- 1Department of Neurosurgery, Affiliated Hospital of Zunyi Medical University, Zunyi, Guizhou, China
- 2Department of Physiology, Zunyi Medical and Pharmaceutical College, Zunyi, Guizhou, China
- 3Department of Urology, The People’s Hospital of Yubei District of Chongqing City, Chongqing, China
- 4Department of Orthopedics, The People’s Hospital of Yubei District of Chongqing City, Chongqing, China
- 5School of Clinical Medicine, Chongqing Medical and Pharmaceutical College, Chongqing, China
Background: Conventional systemic inflammatory biomarkers could predict prognosis in patients with ischemic stroke (IS) admitted to the intensive care unit (ICU). Acute kidney injury (AKI) is common in patients with IS admitted to ICU, but few studies have used systemic inflammatory biomarkers to predict AKI in critically ill patients with IS. This study aimed to establish a risk model based on white blood cell (WBC)-related biomarkers to predict AKI in critically ill patients with IS.
Methods: Data were extracted from the Medical Information Mart for Intensive Care-IV (MIMIC-IV) for a training cohort, and data were extracted from the Medical Information Mart for eICU Collaborative Research Database (eICU-CRD) for a validation cohort. Logistic regression analysis was used to determine the significant predictors of WBC-related biomarkers on AKI prediction, and a risk model was established based on those significant indicators in multivariate logistic regression. The receiver operating characteristics (ROC) curve was utilized to obtain the best cut-off value of the risk model. The Kaplan–Meier curve was used to evaluate the prognosis-predictive ability of the risk model.
Results: The overall incidence of AKI was 28.4% in the training cohort and 33.2% in the validation cohort. WBC to lymphocyte ratio (WLR), WBC to basophils ratio (WBR), WBC to hemoglobin ratio (WHR), and neutrophil to lymphocyte ratio (NLR) could independently predict AKI, and a novel risk model was established based on WLR, WBR, WHR, and NLR. This risk model depicted good prediction performance both in AKI and other clinical outcomes including hemorrhage, persistent AKI, AKI progression, ICU mortality, and in-hospital mortality both in the training set and in the validation set.
Conclusion: A risk model based on WBC-related indicators exhibited good AKI prediction performance in critically ill patients with IS which could provide a risk stratification tool for clinicians in the ICU.
Introduction
Ischemic stroke (IS) is a leading cause of mortality and morbidity, with long-term debilitating effects, which caused huge social and economic costs (1). Acute kidney injury (AKI) is defined by an increase in serum creatinine and a decrease in urine output (2). The epidemiology of AKI following IS is commonly overlooked following stroke events. The incidence of AKI is a common complication among patients with IS, which is tightly linked to poor prognosis (3). A previous review including eight studies reported that the incidence of AKI in the IS setting was 9.62%, and mortality in patients with IS who develop AKI increased 2.5-fold more than in non-AKI (4). The incidence of AKI and mortality in patients with IS admitted to the ICU would be higher. Wang et al. retrospectively recruited 647 patients with stroke from the neurology ICU, 20.9% of critically ill patients with IS developed AKI, and patients in the neurology ICU who developed AKI increased 4.9-folds, 19.7-folds, and 48.6-folds all-cause mortality at AKI-stage 1, AKI-stage 2, and AKI-stage 3, respectively (5). Hence, it is important to find novel biomarkers that can predict AKI to improve post-IS clinical outcomes and reduce mortality rates in these critically ill patients with IS.
A previous study reported that urinary liver-type fatty-acid binding protein (L-FABP) was independently associated with the development of AKI and 90-day mortality in critically ill patients with IS (6). Xiao et al. found that serum neutrophil gelatinase-associated lipocalin (NGAL) could predict AKI in patients with IS (7). However, the above-mentioned biomarkers are not easy to obtain in the ICU setting, and finding relatively clinically often-used indicators may help ICU clinicians in identifying those at high risk. Inflammation and immune responses have been found to be involved in the pathological progression of IS in the ICU setting (8, 9). Under IS conditions, the dynamic balance between the pro-inflammatory and anti-inflammatory responses was disturbed, and inhibited inflammation can alleviate brain injury and improve prognosis (10). Numerous studies reported that blood routine-based inflammatory indicators such as neutrophil to lymphocyte ratio (NLR), platelet to lymphocyte ratio (PLR), and lymphocyte to monocyte ratio (LMR) could predict the prognosis of patients with IS (11–13). However, whether these white blood cell (WBC)-related indicators could predict AKI in the setting of AIS admitted to ICU remains largely unknown.
Hence, this study aims to evaluate the AKI predictive ability of the WBC-related indicators in critically ill patients with IS and then develop a risk model based on those significant WBC-related indicators to facilitate ICU clinicians for better clinical decisions.
Materials and methods
Data source
The data used in this study were obtained from the Medical Information Mart for Intensive Care (MIMIC)-IV database (version: 1.0) from 2008 to 2019 (14) and the eICU Collaborative Research Database (eICU-CRD) (15). eICU-CRD contains data on more than 200 thousand ICU admissions in 2014 and 2015 at 208 US hospitals, while MIMIC-IV includes information on more than 70,000 patients admitted to the ICUs of Beth Israel Deaconess Medical Center in Boston, MA, from 2008 to 2019. The MIMIC-IV database and eICU-CRD database are accessible to individuals who have completed the Collaborative Institutional Training Initiative examination. The study was conducted in accordance with the Declaration of Helsinki. We obtained permission to extract data from the MIMIC-IV database and eICU-CRD database.
The inclusion criteria were as follows: Adult patients with IS, defined as ICD-9 codes of 433, 434, 436, 437.0, and 437.1, or ICD-10 codes of I63, I65, and I66. AKI diagnosis was based on KDIGO-AKI criteria according to serum creatinine in the first 48 h of their ICU admission (16). Patients with one of the following conditions were excluded: (1) Less than 18 years at first admission to ICU; (2) less than 48 h of hospital stay; (3) patients with repeated ICU or hospital admissions; (4) patients with end-stage renal disease (ESRD); and (5) patients with missing values of WBC-related indicators. We selected the MIMIC-IV database as the training cohort and the eICU-CRD database as the validation cohort. The detailed flowchart is shown in Figure 1.
Data collection and outcomes
The following information was collected: General information, vital signs, scoring systems [the sequential organ failure assessment (SOFA) score, oxford acute severity of illness score (OASIS), acute physiology score III (APS III) score, and simplified acute physiology score II (SAPS II)], systemic inflammatory response syndrome (SIRS) score, HAS-BLED score, comorbidities, laboratory data, and drugs usage. All of the laboratory results extracted were from the first test results after the patient entered the ICU. The primary outcome was AKI. The secondary outcomes were AKI severity, AKI progression, intracerebral hemorrhage, in-hospital mortality, and ICU mortality.
Definition
Systemic immune-inflammation index (SII) was calculated as platelet counts* neutrophil count/lymphocyte counts. According to previous reports, AKI progression, AKI recovery, and persistent AKI (pAKI) were diagnosed, in brief, AKI progression was defined as a worsening of AKI-stage: From stage 1 to stage 2 or 3 or from stage 2 to 3. Patients diagnosed with progressive or persisting stage 3 AKI (stage 3 AKI for ≥2 consecutive days) were classified as having AKI progression. AKI recovery was defined as greater than or equal to a 50% decrease in serum creatinine after the diagnosis of AKI and/or return of serum creatinine to the baseline value. Persistent AKI was defined as renal dysfunction without recovery within 2 days or before death (17, 18). Moreover, intracerebral hemorrhage, acute heart failure, sepsis, and acute respiratory failure were defined as the ICD-9/10 codes.
Statistical analysis
Variables included in this study were represented as n (%) or mean ± standard deviation (SD). A comparison of two groups using the Kruskal–Wallis test or the Chi-square test. P < 0.05 was considered to indicate a statistically significant difference on both sides.
A novel AKI prediction risk model [risk = 0.884 × Lg (WLR) + 0.027 × WBR + 1.460 × WHR + 0.013 × NLR] was obtained from the results of a multivariate logistic regression analysis. The decision curve analysis (DCA) was performed to determine the clinical utilities of the risk model. Moreover, univariate and multivariate logistic or Cox regressions were conducted to determine the risk model for the prediction of different clinical outcomes. Subgroup analysis was exhibited by the forest plot. Waterfall plots were used to display the distribution of clinical outcomes and their corresponding risk scores (19).
Results
Baseline characteristics
A total of 1,994 critically ill patients with IS from the MIMIC-IV database and 2,375 critically ill patients with IS from the eICU-CRD database were enrolled in this study, with 567 (28.4%) developed AKI in the training set and 788 (33.2%) developed AKI in the validation set. Compared to those who developed non-AKI, patients who developed AKI had a significantly higher prevalence of congestive heart failure, diabetes, chronic kidney disease, a higher proportion of diuretic use, increased weight, SOFA, OASIS, APS III, HAS-BLED scores, lower MAP, and higher heart rate in both the training and validation sets (P < 0.05 for all comparisons, Table 1). Moreover, patients who developed AKI had markedly higher WBC counts, BUN, creatinine, and potassium, and lower hemoglobin, platelet, albumin, and bilirubin compared to the non-AKI group in both training and validation sets (P < 0.05 for all comparisons, Table 1).
A novel risk model was established based on WBC-related indicators
Multivariate logistic regression was conducted to determine the WBC-related indicators for AKI prediction. As shown in Table 2, Lg (WLR), WBR, WHR, and NLR were independent prognostic predictors of AKI, and a novel risk model was established based on the significant factors of multivariate logistic regression: Risk score = 0.884 × Lg (WLR) + 0.027 × WBR + 1.460 × WHR + 0.013 × NLR.
Verification of the new model’s performance in AKI prediction We first checked the correlations between risk scores with WBC-related biomarkers, severity scores, and clinical outcomes. As shown in Figure 2A, the risk model is tightly related to WBC-related biomarkers, severity scores, and clinical outcomes. ROC curves evaluated the AKI prediction abilities of the risk model and other score systems among critically ill patients with IS. The results indicated that the WBC-related biomarkers-based risk model exhibited better AKI prediction performance (AUC: 0.779, sensitivity: 62.1%, specificity: 79.8%) than other score systems including OASIS score, APS III score, SAPS II score, SIRS score, Charlson index, and HAS-BLED score (Figure 2B). The best cut-off value of the novel risk model is 2.65 according to the ROC analysis, and the low-risk (≤2.65) and high-risk (>2.65) groups were divided according to the best cut-off value. As shown in Table 3, the serum creatinine levels were significantly higher in the high-risk group compared to the low-risk group regardless of before or after the diagnosis of AKI compared to those in the low-risk group (P < 0.05). Critically ill patients with IS at high risk developed AKI, persistent AKI, AKI progression, intracerebral hemorrhage, had higher stage AKI, in-hospital mortality, and ICU mortality both in the training and validation sets (P < 0.05 for all, Table 4 and Supplementary Table 1).
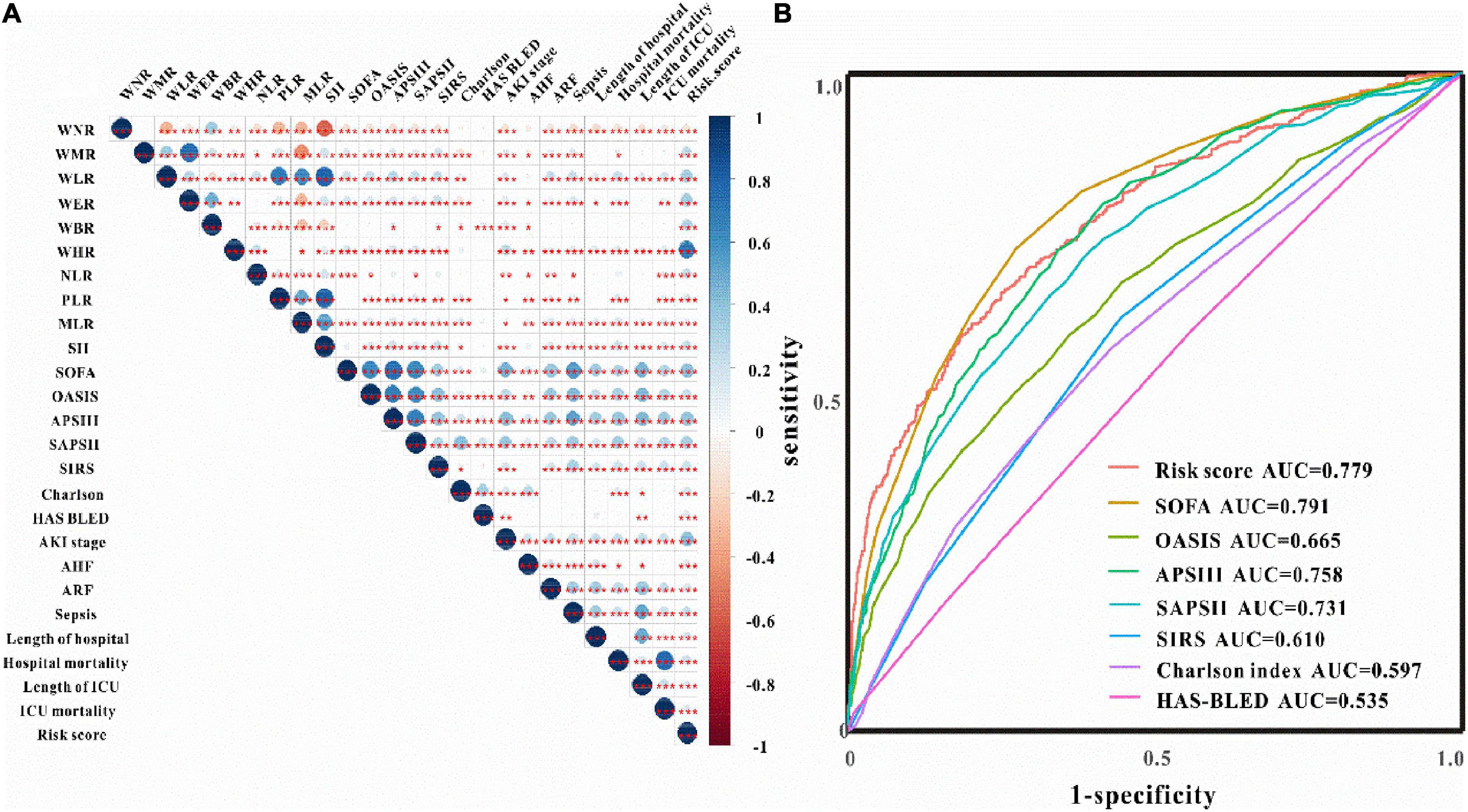
Figure 2. Clinical correlation and predictive ability of WBC-related biomarkers. (A) The correlations between risk score with WBC-related biomarkers, severity score, and clinical outcomes. (B) ROC curves of risk score and severity score for the prediction of AKI among critically ill patients with IS.
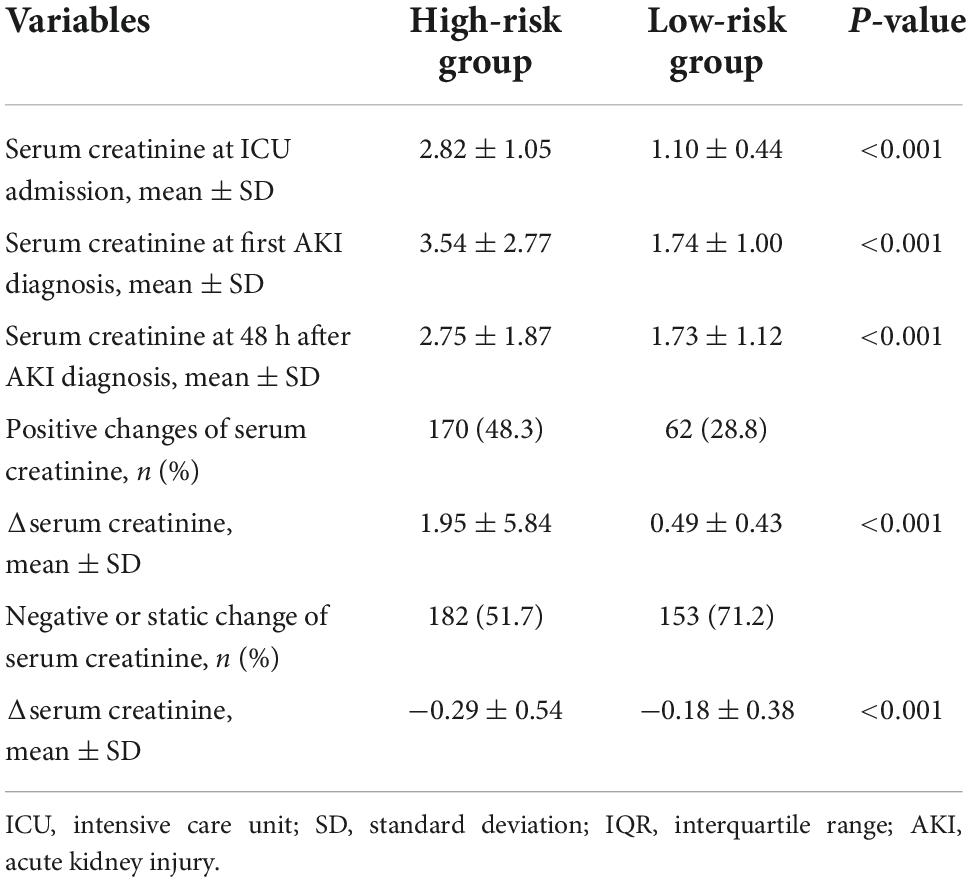
Table 3. Change in serum creatinine before and after the diagnosis of AKI for critically ill patients with IS.
We then utilized univariate and multivariate logistic regression analyses to assess the AKI predictive ability of the risk model. The results indicated that when adjusted for different models, the risk model could remain to predict AKI in both training and validation sets (P < 0.05 for all models, Table 5 and Supplementary Tables 2, 3). Furthermore, the waterfall plots were used to reflect the relationship between the risk score and the prevalence of the developed-AKI proportion. As shown in Figure 3A, a great majority of patients who developed AKI had higher risk scores than those who developed non-AKI. The risk model could independently predict AKI in critically ill patients with IS (OR: 6.45, 95% CI: 5.21–7.98; P < 0.05, Figure 3C). The novel risk model can predict AKI in almost all subgroups except for patients younger than 65 years of age (all P < 0.01, Figure 3C). These results indicated that the WBC-related biomarkers-based risk model could significantly predict AKI in critically ill patients with IS.
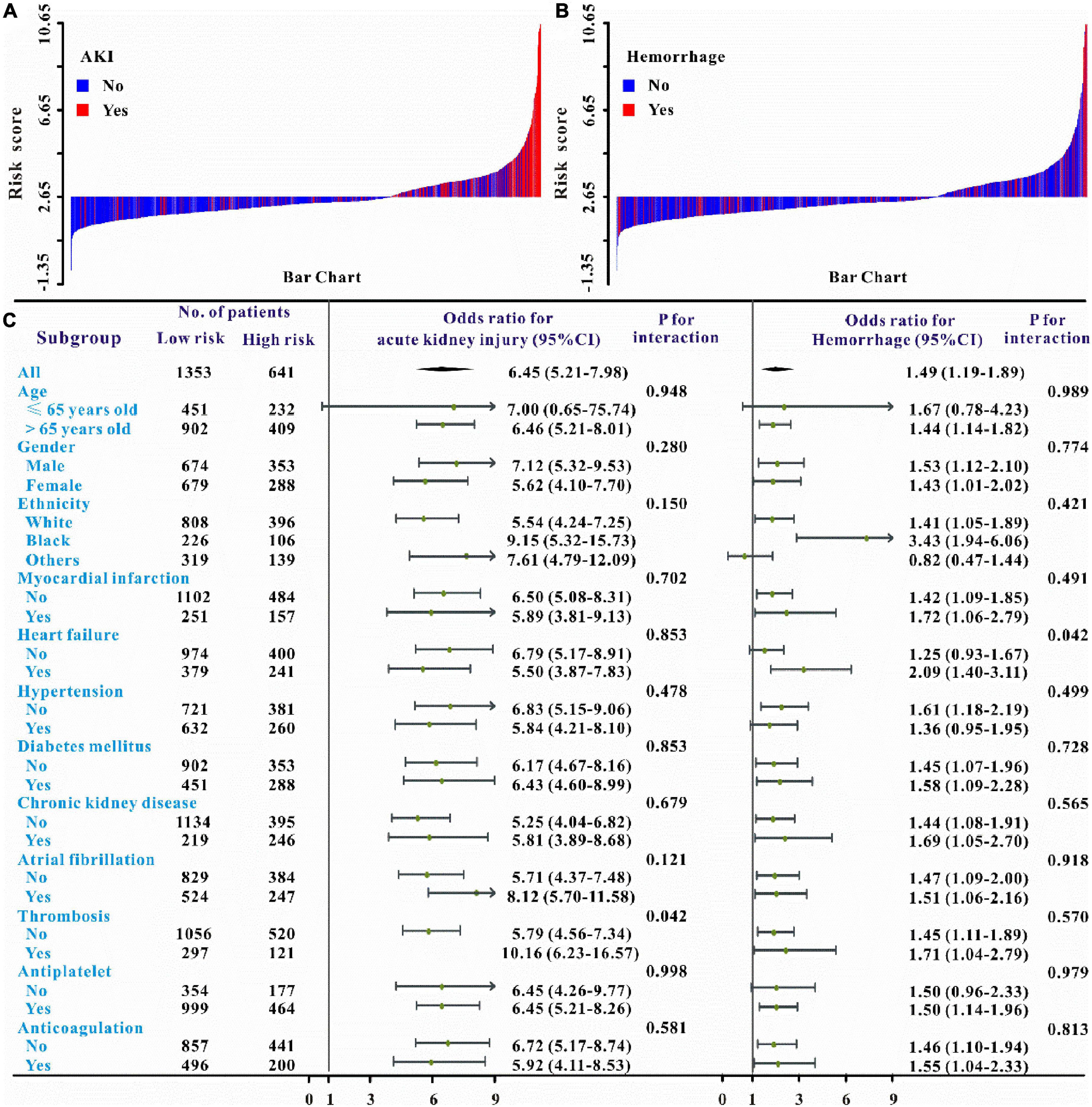
Figure 3. Waterfall plots and forest plots of the high-risk group and low-risk group for the prediction of AKI and intracerebral hemorrhage among critically ill patients with IS. The waterfall plot of the risk score for each patient of AKI (A) and intracerebral hemorrhage (B). The subgroup analysis of the risk score in individuals with critically ill IS for AKI and intracerebral hemorrhage (C).
Verification of the new model’s performance in other clinical outcomes prediction. We also intended to evaluate the predictive abilities of this risk model on the secondary clinical outcomes including hemorrhage, persistent AKI, AKI progression, ICU mortality, and in-hospital mortality. Multivariate logistic or Cox regression analysis revealed that this risk model could also independently predict all secondary clinical outcomes and when adjusted for different models, this result remains solid (Tables 5–6 and Supplementary Table 2). The waterfall plot results verified that higher risk scores were associated with a higher incidence of hemorrhage, persistent AKI, and AKI progression in both training and validation sets (Figures 3B, 4A–B and Supplementary Figure 2). The predictive abilities of the new risk model were further verified across different subgroups: Hemorrhage (OR: 1.49, 95% CI: 1.19–1.89; P < 0.05, Figure 3C), persistent AKI (OR: 2.30, 95% CI: 1.61–3.27; P < 0.05, Figure 4C), and AKI progression (OR: 2.66, 95% CI: 1.74–4.06; P < 0.05, Figure 4C).
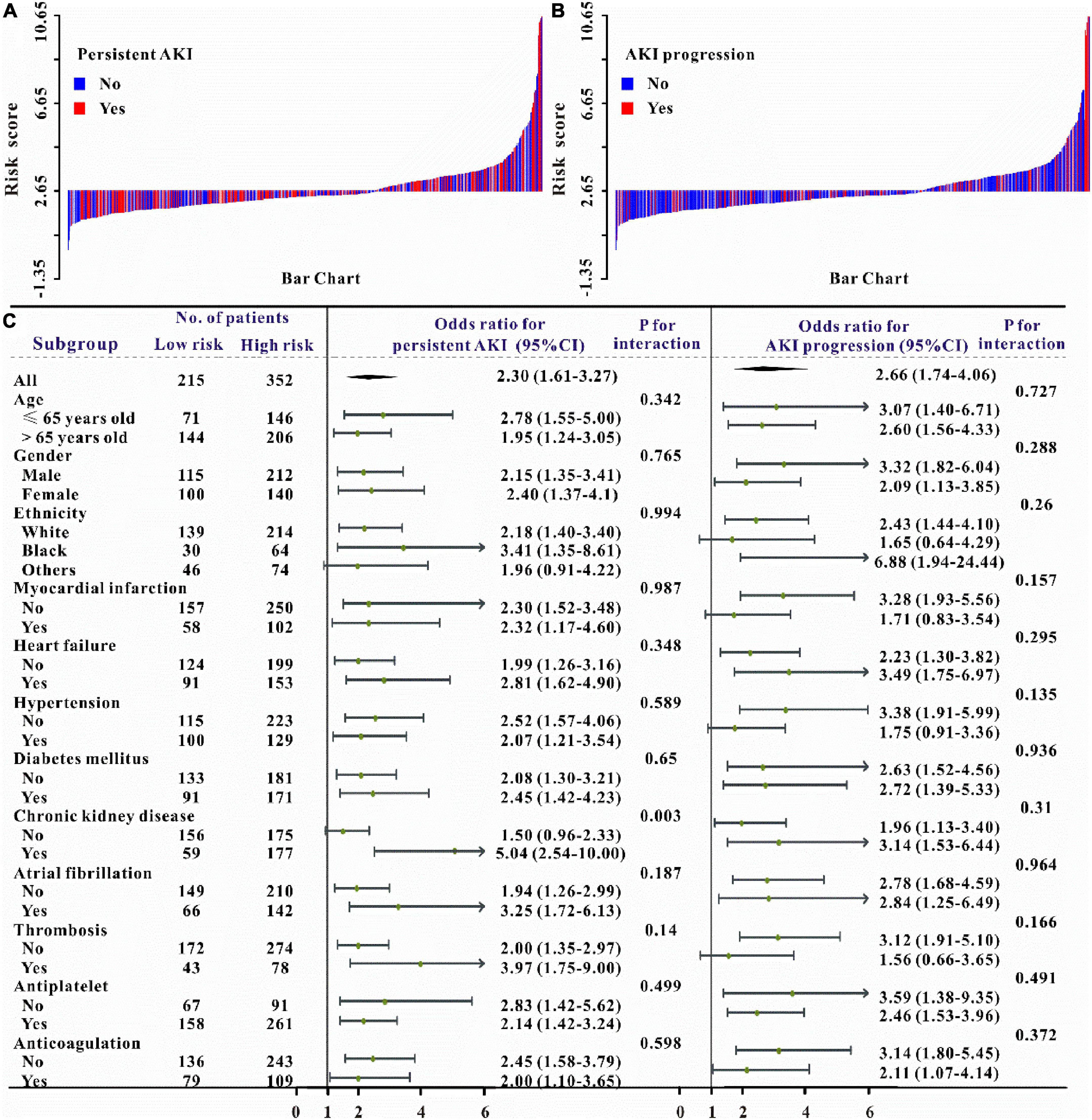
Figure 4. Waterfall plots and forest plots of the high-risk group and low-risk group for the prediction of persistent acute kidney injury (pAKI) and AKI progression. The waterfall plot of the risk score for each patient of pAKI (A) and AKI progression (B). The subgroup analysis of the risk score in individuals with AKI for pAKI and AKI progression (C).
Moreover, as can be seen in Figures 5–6, the risk model depicted good ICU mortality (AUC: 0.763, 95% CI: 0.734–0.792) and in-hospital mortality (AUC:0.741, 95% CI: 0.715–0.767) predictive performance. Kaplan–Meier curve analysis reflected that those in the high-risk group showed lower survival compared to the low-risk group in both training and validation sets (all P < 0.01, Figures 5F, 6F and Supplementary Figure 3). Furthermore, DCA was also performed to determine the clinical utilities of the predictive risk model. The results indicated that the risk model was clinically useful (Figures 5E, 6E). Meanwhile, the novel risk model remains a firmly predictive tool for ICU mortality (OR: 1.96, 95% CI: 1.51–2.55; P < 0.05, Supplementary Figure 1) and in-hospital mortality (OR: 2.10, 95% CI: 1.71–2.58; P < 0.05, Supplementary Figure 1) across different subgroups. These results manifested that except for AKI, WBC-related biomarkers-based risk model could also markedly predict secondary clinical outcomes in critically ill patients with IS.
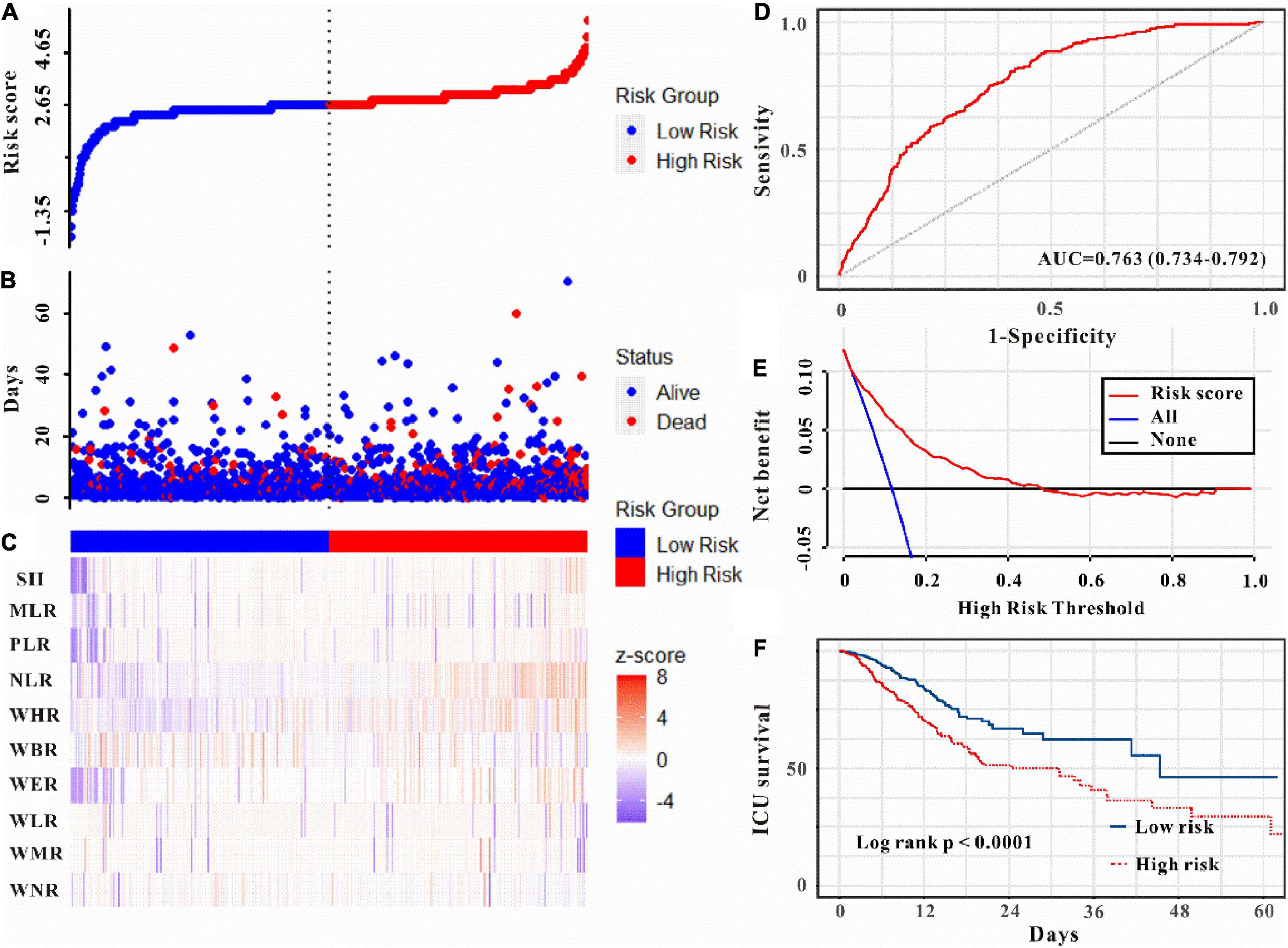
Figure 5. Risk score was established to detect the ICU mortality of critically ill patients with IS. All patients were distinguished into high and low risk based on the risk score (A), the relationship between survival time and prognosis of patients in the two corresponding groups (B), and the heatmap of WBC-related indicators between the two groups (C). Receiver operating characteristic (ROC) curve analysis of the risk score for ICU mortality (D), decision curve analysis of the risk score for ICU mortality (E). Kaplan–Meier curves show the ICU mortality of groups with different risks (F).
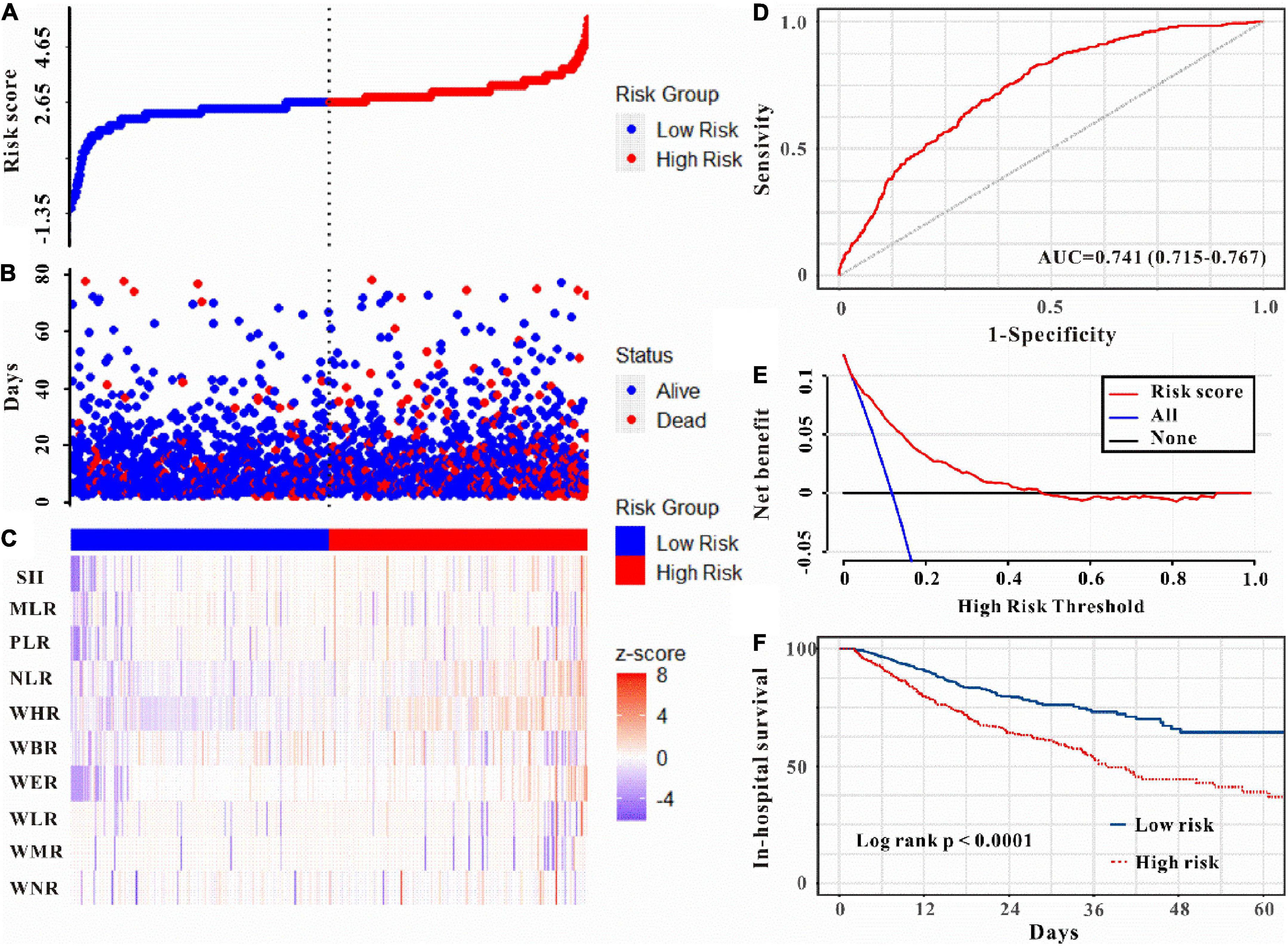
Figure 6. Risk score was established to detect in-hospital mortality in critically ill patients with IS. All patients were distinguished into high and low risk based on the risk score (A), the relationship between survival time and prognosis of patients in the two corresponding groups (B), and the heatmap of inflammatory marks between the two groups (C). Receiver operating characteristic (ROC) curve analysis of the risk score for in-hospital mortality (D) and decision curve analysis of the risk score for in-hospital mortality (E). Kaplan–Meier curves show the in-hospital mortality of groups with different risks (F).
Discussion
Herein, this study for the first time explored a novel risk model based on WBC-related biomarkers to predict AKI and other clinical outcomes in patients with IS admitted to the ICU. This retrospective study indicated that AKI was a common complication of IS admitted to the ICU. WLR, WBR, WHR, and NLR could independently predict AKI, and a novel risk model was established based on WLR, WBR, WHR, and NLR. This risk model exhibited good prediction performance both in AKI and other clinical outcomes (hemorrhage, persistent AKI, AKI progression, ICU mortality, and in-hospital mortality) in both training and validation sets.
Ischemic stroke is a major cause of death and disability worldwide. About 20% of patients with IS require care in an ICU (20). Although advances have been made to improve prognosis among critically ill patients with IS, many complications occurred during admittance to ICU including AKI. According to previous reports, the incidence of AKI following IS ranges from 0.82 to 26.68% (21). The AKI incidence in the ICU would be higher due to more comorbidities. One study investigated the epidemiology of AKI in 381 patients with stroke admitted to ICU and found that 115 (30.18%) patients developed AKI (22). Zhang et al. explored the incidence of AKI in critical care patients with acute cerebrovascular disease and found that 18.3% developed AKI (23). The incidence of AKI in the present study (28.4%) falls along the spectrum described in previous studies (22, 23). Patients with IS who developed AKI exhibited poor prognoses. A meta-analysis reported that patients with stroke who developed AKI increased the risk of mortality, degree of disability, length of hospital stay and hospitalization costs, and cardiovascular events (4). However, the precise mechanism underlying the high incidence of AKI in critically ill patients with IS remains largely unknown.
Previous studies reported that brain–kidney interaction may explain this mechanism. A review concluded that the central pathway of the brain–kidney interaction may be via the central autonomic network and sympathetic nervous system, and the peripheral signaling pathway may be regulated by inflammatory responses (24). The inflammatory response is tightly involved in the progression of a stroke, under stroke conditions, the body is in a pro-inflammatory state, and the C-reactive protein (CRP), IL-1β, IL-6, and tumor necrosis factor-α (TNF-α) were significantly increased following stroke (25, 26), and these inflammatory factors were all reported to be associated with the development of AKI (27, 28). Furthermore, integrated WBC-related inflammatory biomarkers such as NLR, PLR, and LMR have been reported to be involved in the progress of acute IS (11, 12, 29), and NLR, PLR, and LMR could also predict AKI in different settings (30–32). However, whether these WBC-related biomarkers could predict AKI in patients with IS admitted to ICU has been unexplored. Gao et al. previously reported that preoperative WBC to hemoglobin ratio (WHR), WBC to monocyte ratio (WMR), and platelet to lymphocyte ratio (PLR) associated with the prognosis of bladder cancer, and a risk model established based on WHR, WMR, and PLR showed good prognosis-predictive performance (33). In line with the previously reported (33), in this study, WLR, WBR, WHR, and NLR were independent prognostic predictors of AKI, and then WLR, WBR, WHR, and NLR were combined to construct a novel risk model.
Previous studies reported the different risk models to predict AKI in patients with IS. Zhu et al. developed a nomogram that includes blood urea nitrogen (BUN), creatinine, red blood cell distribution width (RDW), heart rate (HR), Oxford Acute Severity of Illness Score (OASIS), the history of congestive heart failure (CHF), the use of vancomycin, contrast agent, and mannitol and found that nomogram showed good predictive ability (34). For ICU patients, the SIRS, OASIS, APS III, SAPS II, and CCI scores are fundamental individualized risk assessments for characterizing disease severity and degree of organ dysfunction. This novel risk model in the present study showed better AKI prediction than those traditional score systems (OASIS, APS III, SAPS II, and SIRS) which are commonly used in the ICU. The SOFA score has been widely used to evaluate organ dysfunction in the ICU. Chang et al. used the SOFA score to predict ICU mortality in patients coexisting with AKI admitted to ICU and indicated that the SOFA score was a good prognosis-predictive tool (35), and this risk model also exhibited comparable AKI predictive ability to the SOFA score. Meanwhile, we also applied this model to predict other clinical outcomes, and the results demonstrated that this model could also predict hemorrhage, persistent AKI, AKI progression, ICU mortality, and in-hospital mortality in critically ill patients with IS.
This study has several strengths. First, to our knowledge, this is the first study to investigate the relationship between a WBC-related biomarkers-based risk model and AKI prediction in critically ill patients with IS. Second, we compared the novel risk model with the other scores system including SOFA score, OASIS score, APS III score, SAPS II score, SIRS score, Charlson index, and HAS-BLED score, and these scores systems were widely used in the ICU setting. Moreover, serum creatinine values were assessed during hospitalization and were used for these analyses. Finally, except for AKI prediction, this risk model was also used to predict other clinical outcomes including hemorrhage, persistent AKI, AKI progression, ICU mortality, and in-hospital mortality. However, some limitations should be addressed in this study. First, it is a retrospective study with data extracted from two public databases, and the results need to be verified by external validation. Second, the incidence of AKI with high heterogeneity according to different define diagnostic criteria. The incidence of AKI was 4.05% based on a coding definition for AKI, and 17.3% in a study utilizing creatinine-based definitions (4); this study used KDIGO-AKI criteria according to serum creatinine, and the incidence of AKI may be overestimated, and the AKI predictive ability of this risk model needed to be confirmed by further studies. Moreover, contrast-induced AKI is one of the common causes of AKI in clinical practice; however, due to the limitations of the MIMIC-IV and eICU-CRD databases, we were unable to obtain information about the contrast materials. Furthermore, stroke-specific characteristics such as NIHSS score, infarction area, and location are not included in the variables in this study due to the limitations of the MIMIC-IV database and the eICU-CRD database.
Conclusion
We found that AKI appears to be a very common complication in patients with ischemic stroke admitted to the ICU. A novel risk model was established based on WBC-related biomarkers WLR, WBR, WHR, and NLR, which is easily accessible from the routine blood test, and this risk model depicted good prognosis-predictive performance including AKI and other clinical outcomes hemorrhage, persistent AKI, AKI progression, ICU mortality, and in-hospital mortality. This risk model may favor clinicians to take early interventions due to its simplicity. Further prospective studies are needed to determine the predictive performance of this study in the future.
Data availability statement
The original contributions presented in this study are included in the article/Supplementary material, further inquiries can be directed to the corresponding authors.
Ethics statement
The study was approved by the Institutional Review Board (IRB) of the Massachusetts Institute of Technology (MIT), and consent was obtained for the original data collection. Therefore, the ethical approval statement and the need for informed consent were waived for this manuscript.
Author contributions
SL and ML conceived and designed the study. ML analyzed and interpreted the data and drafted the work. YY and YC participated in design of the study and assisted with revisions of the manuscript. WW and XZ extracted the data and take responsibility for the content of the manuscript including the data and analysis. All authors have approved the final version of the manuscript for submission and agreed to be accountable for all aspects of the work.
Conflict of interest
The authors declare that the research was conducted in the absence of any commercial or financial relationships that could be construed as a potential conflict of interest.
Publisher’s note
All claims expressed in this article are solely those of the authors and do not necessarily represent those of their affiliated organizations, or those of the publisher, the editors and the reviewers. Any product that may be evaluated in this article, or claim that may be made by its manufacturer, is not guaranteed or endorsed by the publisher.
Supplementary material
The Supplementary Material for this article can be found online at: https://www.frontiersin.org/articles/10.3389/fmed.2022.1043396/full#supplementary-material
References
1. Prabhakaran S, Ruff I, Bernstein R. Acute stroke intervention: a systematic review. JAMA. (2015) 313:1451–62. doi: 10.1001/jama.2015.3058
2. Kellum JA, Lameire N, Aspelin P, Barsoum RS, Burdmann EA, Goldstein SL, et al. KDIGO Clinical practice guideline for acute kidney injury. Kidney Int Suppl. (2012) 2:1–138. doi: 10.1038/kisup.2012.1
3. Qureshi A, Aslam H, Zafar W, Huang W, Lobanova I, Naqvi S, et al. Acute kidney injury in acute ischemic stroke patients in clinical trials. Crit Care Med. (2020) 48:1334–9. doi: 10.1097/CCM.0000000000004464
4. Arnold J, Ng K, Sims D, Gill P, Cockwell P, Ferro C. Incidence and impact on outcomes of acute kidney injury after a stroke: a systematic review and meta-analysis. BMC Nephrol. (2018) 19:283. doi: 10.1186/s12882-018-1085-0
5. Wang D, Guo Y, Zhang Y, Li Z, Li A, Luo Y. Epidemiology of acute kidney injury in patients with stroke: a retrospective analysis from the neurology ICU. Intern Emerg Med. (2018) 13:17–25. doi: 10.1007/s11739-017-1703-z
6. Shimoyama T, Sato T, Sakamoto Y, Nagai K, Aoki J, Suda S, et al. Urinary biomarkers of kidney tubule injury, risk of acute kidney injury, and mortality in patients with acute ischaemic stroke treated at a stroke care unit. Eur J Neurol. (2020) 27:2463–72. doi: 10.1111/ene.14448
7. Xiao W, Chen W, Hu H, Huang X, Luo Y. The clinical significance of neutrophil gelatinase-associated lipocalin in ischemic stroke patients with acute kidney injury. J Clin Lab Anal. (2019) 33:e22907. doi: 10.1002/jcla.22907
8. Zaid Y, Rajeh A, Hosseini Teshnizi S, Alqarn A, Tarkesh F, Esmaeilinezhad Z, et al. Epidemiologic features and risk factors of sepsis in ischemic stroke patients admitted to intensive care: a prospective cohort study. J Clin Neurosci. (2019) 69:245–9. doi: 10.1016/j.jocn.2019.07.031
9. Jin R, Liu L, Zhang S, Nanda A, Li G. Role of inflammation and its mediators in acute ischemic stroke. J Cardiovasc Transl Res. (2013) 6:834–51. doi: 10.1007/s12265-013-9508-6
10. Yilmaz G, Granger D. Cell adhesion molecules and ischemic stroke. Neurol Res. (2008) 30:783–93. doi: 10.1179/174313208X341085
11. Gong P, Liu Y, Gong Y, Chen G, Zhang X, Wang S, et al. The association of neutrophil to lymphocyte ratio, platelet to lymphocyte ratio, and lymphocyte to monocyte ratio with post-thrombolysis early neurological outcomes in patients with acute ischemic stroke. J Neuroinflammation. (2021) 18:51. doi: 10.1186/s12974-021-02090-6
12. Chen C, Gu L, Chen L, Hu W, Feng X, Qiu F, et al. Neutrophil-to-lymphocyte ratio and platelet-to-lymphocyte ratio as potential predictors of prognosis in acute ischemic stroke. Front Neurol. (2021) 11:525621. doi: 10.3389/fneur.2020.525621
13. Quan K, Wang A, Zhang X, Meng X, Chen P, Li H, et al. Neutrophil to lymphocyte ratio and adverse clinical outcomes in patients with ischemic stroke. Ann Transl Med. (2021) 9:1047. doi: 10.21037/atm-21-710
14. Goldberger AL, Amaral LA, Glass L, Hausdorff JM, Ivanov PC, Mark RG, et al. PhysioBank, PhysioToolkit, and PhysioNet: components of a new research resource for complex physiologic signals. Circulation. (2000) 101:e215–20. doi: 10.1161/01.CIR.101.23.e215
15. Pollard TJ, Johnson AEW, Raffa J, Celi LA, Mark RG, Badawi O. The eICU collaborative research database, a freely available multi-center database for critical care research. Sci Data. (2018) 5:180178. doi: 10.1038/sdata.2018.178
16. Ad-hoc working group of Erbp, Fliser D, Laville M, Covic A, Fouque D, Vanholder R, et al. A European renal best practice (ERBP) position statement on the Kidney disease improving global outcomes (KDIGO) clinical practice guidelines on acute kidney injury: part 1: definitions, conservative management and contrast-induced nephropathy. Nephrol Dial Transplant. (2012) 27:4263–72. doi: 10.1093/ndt/gfs375
17. Chawla L, Bellomo R, Bihorac A, Goldstein S, Siew E, Bagshaw S, et al. Acute kidney disease and renal recovery: consensus report of the acute disease quality initiative (ADQI) 16 workgroup. Nat Rev Nephrol. (2017) 13:241–57. doi: 10.1038/nrneph.2017.2
18. Koyner J, Garg A, Coca S, Sint K, Thiessen-Philbrook H, Patel U, et al. Biomarkers predict progression of acute kidney injury after cardiac surgery. J Am Soc Nephrol. (2012) 23:905–14. doi: 10.1681/ASN.2011090907
19. Qamar A, Giugliano R, Keech A, Kuder J, Murphy S, Kurtz C, et al. Interindividual variation in low-density lipoprotein cholesterol level reduction with evolocumab: an analysis of FOURIER trial data. JAMA Cardiol. (2019) 4:59–63. doi: 10.1001/jamacardio.2018.4178
20. Amatangelo M, Thomas S. Priority nursing interventions caring for the stroke patient. Crit Care Nurs Clin North Am. (2020) 32:67–84. doi: 10.1016/j.cnc.2019.11.005
21. Khatri M, Himmelfarb J, Adams D, Becker K, Longstreth W, Tirschwell D. Acute kidney injury is associated with increased hospital mortality after stroke. J Stroke Cerebrovasc Dis. (2014) 23:25–30. doi: 10.1016/j.jstrokecerebrovasdis.2012.06.005
22. Jiang F, Su L, Xiang H, Zhang X, Xu D, Zhang Z, et al. Incidence, risk factors, and biomarkers predicting ischemic or hemorrhagic stroke associated acute kidney injury and outcome: a retrospective study in a general intensive care unit. Blood Purif. (2019) 47:317–26. doi: 10.1159/000499029
23. Zhang X, Chen S, Lai K, Chen Z, Wan J, Xu Y. Machine learning for the prediction of acute kidney injury in critical care patients with acute cerebrovascular disease. Ren Fail. (2022) 44:43–53. doi: 10.1080/0886022X.2022.2036619
24. Zhao Q, Yan T, Chopp M, Venkat P, Chen J. Brain-kidney interaction: renal dysfunction following ischemic stroke. J Cereb Blood Flow Metab. (2020) 40:246–62. doi: 10.1177/0271678X19890931
25. Jiang J, Tan C, Zhou W, Peng W, Zhou X, Du J, et al. Plasma C-reactive protein level and outcome of acute ischemic stroke patients treated by intravenous thrombolysis: a systematic review and meta-analysis. Eur Neurol. (2021) 84:145–50. doi: 10.1159/000514099
26. Lasek-Bal A, Jedrzejowska-Szypulka H, Student S, Warsz-Wianecka A, Zareba K, Puz P, et al. The importance of selected markers of inflammation and blood-brain barrier damage for short-term ischemic stroke prognosis. J Physiol Pharmacol. (2019) 70:209–17. doi: 10.26402/jpp.2019.2.04
27. Chawla L, Seneff M, Nelson D, Williams M, Levy H, Kimmel P, et al. Elevated plasma concentrations of IL-6 and elevated APACHE II score predict acute kidney injury in patients with severe sepsis. Clin J Am Soc Nephrol. (2007) 2:22–30. doi: 10.2215/CJN.02510706
28. Xie Q, Zhou Y, Xu Z, Yang Y, Kuang D, You H, et al. The ratio of CRP to prealbumin levels predict mortality in patients with hospital-acquired acute kidney injury. BMC Nephrol. (2011) 12:30. doi: 10.1186/1471-2369-12-30
29. Hu J, Wang L, Fan K, Ren W, Wang Q, Ruan Y, et al. The association between systemic inflammatory markers and post-stroke depression: a prospective stroke cohort. Clin Interv Aging. (2021) 16:1231–9. doi: 10.2147/CIA.S314131
30. Jiang F, Shi Z, Liu X, Xiang J, Lei J, Yang B, et al. The role of the monocyte-to-lymphocyte ratio in acute ischemic stroke patients with acute kidney injury. Mediators Inflamm. (2022) 2022:7911033. doi: 10.1155/2022/7911033
31. Bi J, Zhang J, Ren Y, Du Z, Wu Z, Lv Y, et al. Neutrophil-to-lymphocyte ratio predicts acute kidney injury occurrence after gastrointestinal and hepatobiliary surgery. World J Gastrointest Surg. (2020) 12:326–35. doi: 10.4240/wjgs.v12.i7.326
32. Jiang F, Lei J, Xiang J, Chen Y, Feng J, Xu W, et al. Monocyte-to-lymphocyte ratio: a potential novel predictor for acute kidney injury in the intensive care unit. Ren Fail. (2022) 44:1004–11. doi: 10.1080/0886022X.2022.2079521
33. Gao M, Yang Q, Xu H, Chen Z, Wang X, Guo H. Preoperative white blood cell-related indicators can predict the prognosis of patients with transurethral resection of bladder cancer. J Inflamm Res. (2022) 15:4139–47. doi: 10.2147/JIR.S373922
34. Zhu G, Fu Z, Jin T, Xu X, Wei J, Cai L, et al. Dynamic nomogram for predicting acute kidney injury in patients with acute ischemic stroke: a retrospective study. Front Neurol. (2022) 13:987684. doi: 10.3389/fneur.2022.987684
Keywords: white blood cell, ischemic stroke, risk model, acute kidney injury, intensive care unit
Citation: Liu S, Li M, Yang Y, Chen Y, Wang W and Zheng X (2022) A novel risk model based on white blood cell-related biomarkers for acute kidney injury prediction in patients with ischemic stroke admitted to the intensive care unit. Front. Med. 9:1043396. doi: 10.3389/fmed.2022.1043396
Received: 13 September 2022; Accepted: 17 November 2022;
Published: 12 December 2022.
Edited by:
Xiaoying Wang, Tulane University, United StatesReviewed by:
Yong Han, Shenzhen Second People’s Hospital, ChinaZuohui Zhang, The University of Iowa, United States
Copyright © 2022 Liu, Li, Yang, Chen, Wang and Zheng. This is an open-access article distributed under the terms of the Creative Commons Attribution License (CC BY). The use, distribution or reproduction in other forums is permitted, provided the original author(s) and the copyright owner(s) are credited and that the original publication in this journal is cited, in accordance with accepted academic practice. No use, distribution or reproduction is permitted which does not comply with these terms.
*Correspondence: Wei Wang, d2FuZ3dlaWNxeWJAMTI2LmNvbQ==; Xiaoyu Zheng, emhlbmd4eWNxQDEyNi5jb20=
†These authors have contributed equally to this work