- 1University Clinic for Internal Medicine II, Paracelsus Medical University, Salzburg, Austria
- 2Cardiovascular Disease in COVID-19, International Research Network, Ufa, Russia
- 3Department of Internal Diseases, Bashkir State Medical University, Ufa, Russia
- 4Department of Biomedical Engineering, Ufa State Aviation Technical University, Ufa, Russia
- 5Scientific Laboratory for the Socio-Economic Region Problems Investigation, Bashkir State University, Ufa, Russia
- 6Department of Urology, Bashkir State Medical University, Ufa, Russia
- 7The Key Laboratory of Cardiovascular Medicine Research, Ministry of Education, Department of Pharmacy at the Second Affiliated Hospital, and Department of Pharmacology at College of Pharmacy, Harbin Medical University, Harbin, China
- 8Department of Internal Medicine, Cardiology, Nephrology and Intensive Care Medicine, Hospital Wiener Neustadt, Wiener Neustadt, Austria
- 9Department of Cardiology I, Coronary and Peripheral Vascular Disease, Heart Failure, University Hospital Munster, Munster, Germany
Aims: While COVID-19 affects the cardiovascular system, the potential clinical impact of cardiovascular biomarkers on predicting outcomes in COVID-19 patients is still unknown. Therefore, to investigate this issue we analyzed the prognostic potential of cardiac biomarkers on in-hospital and long-term post-discharge mortality of patients with COVID-19 pneumonia.
Methods: Serum soluble ST2, VCAM-1, and hs-TnI were evaluated upon admission in 280 consecutive patients hospitalized with COVID-19-associated pneumonia in a single, tertiary care center. Patient clinical and laboratory characteristics and the concentration of biomarkers were correlated with in-hospital [Hospital stay: 11 days (10; 14)] and post-discharge all-cause mortality at 1 year follow-up [FU: 354 days (342; 361)].
Results: 11 patients died while hospitalized for COVID-19 (3.9%), and 11 patients died during the 1-year post-discharge follow-up period (n = 11, 4.1%). Using multivariate analysis, VCAM-1 was shown to predict mortality during the hospital period (HR 1.081, CI 95% 1.035;1.129, p = 0.017), but not ST2 or hs-TnI. In contrast, during one-year FU post hospital discharge, ST2 (HR 1.006, 95% CI 1.002;1.009, p < 0.001) and hs-TnI (HR 1.362, 95% CI 1.050;1.766, p = 0.024) predicted mortality, although not VCAM-1.
Conclusion: In patients hospitalized with Covid-19 pneumonia, elevated levels of VCAM-1 at admission were associated with in-hospital mortality, while ST2 and hs-TnI might predict post-discharge mortality in long term follow-up.
Introduction
The novel coronavirus disease COVID-19 still represents a major clinical challenge to date. COVID-19 primarily involves the respiratory system and severe COVID-19 typically leads to bilateral pneumonia with consequent acute respiratory distress syndrome and high mortality rates (1). As the Spike protein S of the ACE-2 receptor serves as the binding site for SARS-CoV-2, cells with a high expression of the ACE-2 receptor are primarily affected by COVID-19, resulting in a broad range of clinical symptoms in COVID-19. Several clinical parameters including laboratory, electrocardiographic and radiology findings have been used to stratify mortality risk (1–3), yet monitoring parameters and long-term prognostic markers for COVID-19 remain scarce.
COVID-19 was reported to have a considerable impact on the cardiovascular system, including not only cardiac injury but also thromboembolic events. Given the correlation of myocardial injury and disease severity in COVID-19, novel cardiovascular biomarkers might prove to be an effective prognostic tool in COVID-19 patients.
High-sensitive troponin is released in response to myocardial injury and represents the gold standard for cardiovascular risk assessment (4). Previous studies studies found that myocardial injury, defined by increased serum cardiac high-sensitive Troponin (TnI) levels, was associated with a mortality rate of >50% in COVID-19 patients (5), while other studies have reported that 19.7% of all COVID-19 patients presented with myocardial injury. Moreover, these patients had a significantly higher mortality rate compared to COVID-19 patients with normal TnI (51.2 vs. 4.5%) (6). This finding is further emphasized by a meta-analysis which reported significantly higher TnI levels in severe COVID-19 compared with patients with mild COVID-19 (7).
Soluble suppression of tumorgenity-2 (sST2) is a member of the interleukin-1 receptor family and has recently emerged as a potentially useful tool for improving the assessment of cardiovascular disease (8–10). There are two isoforms of ST2, the membrane-bound ST2L, mediating cardio-protective effects through binding of its only known ligand IL-33 (11), as well as sST2, the soluble form of ST2, acting as a decoy receptor for IL-33, thereby inhibiting its potential cardio-protective effects (11). Of note, the IL-33/ST2 axis was also reported to play a potential role in the COVID-19 pathogenesis (12–14). sST2 itself was shown to be elevated in numerous clinical scenarios, including heart failure and cardiac remodeling, myocardial infarction, atherosclerosis as well as in inflammatory disease such as sepsis (8). Recent studies reported high levels of sST2 in COVID-19 patients, also correlating with CRP levels, a standard marker of COVID-19 activity (15). Similarly, Sanches et al. (16) reported a significant correlation between levels of sST2 and ICU admission as well as death, emphasizing its prognostic potential.
Several studies have revealed a potential link between COVID-19 and endothelial dysfunction. On this regard, also the term “Acute Vascular Distress Syndrome” was introduced, to account for the vascular pathophysiology in COVID-19 pneumonia (17, 18). This comprises vasoplegia along with a reduced ventilation-to-perfusion ratio, leading to an increased pulmonary blood flow with intrapulmonary right to left shunt (18). Accordingly, also an analysis of vascular biomarkers might contribute to diagnosis and prognosis in COVID-19.
Vascular cells adhesion molecule-1 (VCAM-1) is a protein acting as a cell adhesion molecule of vascular endothelium for lymphocytes, monocytes, eosinophils, and basophils. In a study on the effect of SARS-CoV-2 spike S1 protein on the activation of human lung microvascular endothelial cells, the incubation of HLMVEC with the S1 protein significantly induced the expression of VCAM-1 (19). In further trials, VCAM-1 was associated with COVID-19-related mortality (20). Moreover, in a meta-analysis (n = 2,213), VCAM-1 levels were linked with increased disease severity in COVID-19 patients (21).
Given the involvement of sST2 and VCAM-1 in inflammatory processes and the previous reports on their role in COVID-19, their potential impact on prognosis also in the long term seems plausible. Accordingly, we undertook a comparative analysis of novel cardiac biomarkers sST2 and VCAM-1 as well as high-sensitive Troponin I (TnI) in a 1-year follow-up of COVID-19 patients. We hypothesized that these cardiovascular biomarkers might be helpful in predicting 1-year mortality. Therefore, the aim of our work was to establish an possible association between the investigated cardiovascular biomarkers and in-hospital as well as post-discharge mortality, which could help to identify patients at risk.
Therefore, the aim of our work was to establish an association between biomarkers and mortality.
Methods
The study was approved by the Local Ethical Committee (N5, 2020) and was performed in accordance with standards of good clinical practice and the principles of the Declaration of Helsinki. Written informed consent was obtained from all study participants prior to inclusion.
This prospective, non-randomized, single-center study enrolled 288 consecutive patients between June 2020 and September 2020, who were hospitalized due to COVID-19-associated pneumonia in a tertiary care center during the 1st “wave” of the COVID-19 pandemic. Initial diagnosis was established via CT-scan, PCR testing and specific antibodies at admission and COVID-19 specific medical treatment was applied according to current national COVID-19 guidelines (22).
Included were patients 18 years or older with confirmed COVID-19 disease and related pneumonia. Exclusion criteria included: acute ST-elevation myocardial infarction at admission, acute stroke at admission, active malignant disease within the last 3 years, and acute kidney failure at admission defined as glomerular filtration rate (GFR) <30 mL/min/1.73 m2, as well as pregnancy or lactation. In total, eight patients met the exclusion criteria and were therefore excluded (acute ST-elevation myocardial infarction: n = 2, acute stroke: n = 1, active malignant disease: n = 2, and acute kidney failure: n = 3).
Patient enrollment and the design of the study are presented in Figure 1. Upon hospital admission, venous blood was drawn, subsequently centrifuged, and the serum frozen at −20° C for further analyses. The concentration of biomarkers ST2, VCAM-1 and TnI was analyzed by enzyme immunoassay as indicated by the manufacturer (Thermo Fisher Scientific, USA for VCAM-1, Critical diagnostics, USA, for ST2, and Hema Ltd, Russia for TnI). In addition to the investigated biomarkers, further laboratory parameters were routinely measured according to current guidelines (Table 1).
A detailed medical history was obtained at admission for all enrolled patients, including current symptoms, as well as previous illnesses and current medications. The study was carried out between June 2020 and September 2020. Follow-up (FU) analysis was conducted during the acute phase of disease defined as the total hospital stay and after hospital discharge for a median of 366 days (356: 373) for the study endpoint with the help of the regional medical information analytical system “ProMed” (23). This web-based medical records system enables remote online monitoring of hospitalization discharge notes including death certificates. The study endpoint was defined as all-cause mortality as indicated by discharge notes and/or death certificate during the FU period. The FU endpoints were analyzed in September 2021.
The mathematical model for statistical analyses is summarized in Figure 2. All data were tested for normality. Normally distributed continuous variables were expressed as mean values (M) and standard deviations (SD). Non-normally distributed data were expressed as median (interquartile range Q1–Q3), and the non-parametric Mann-Whitney test was used for comparison between the two groups. Categorical variables were expressed as frequencies and proportions. Differences among groups were assessed with the Chi-squared test.
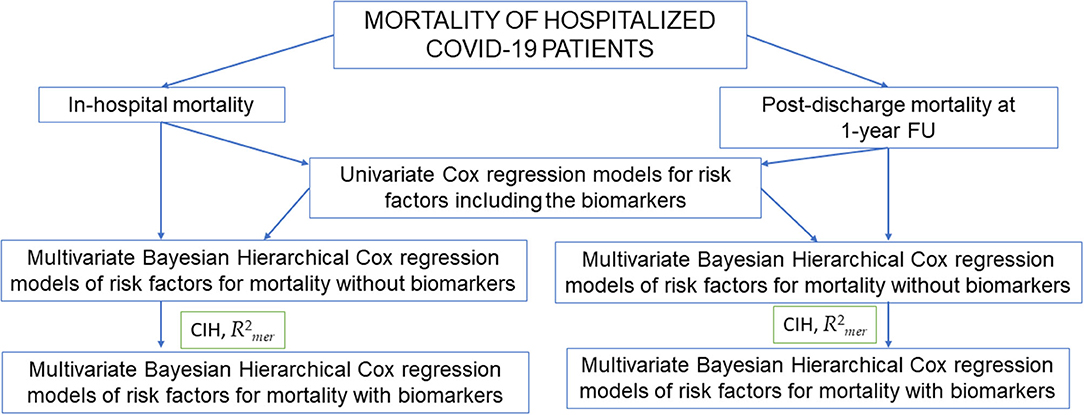
Figure 2. Mathematical model of the statistical analyses. ST2, soluble suppression of tumorigenicity 2; VCAM-1, vascular cells adhesion molecule-1; TnI, high sensitive troponin I.
Prediction of biomarkers for prognosis in COVID-19 patients was analyzed as follows. At the preliminary stage of the analysis, univariate Cox proportional hazards models were evaluated. Biomarkers values were taken as independent variables. To remove the bias of estimates, age of the patients was also considered as a control factor. Then statistically significant factors with p > 0.1 were selected. The first multivariate model of survival was built for all-cause mortality during in-hospital stay, and the second for all-cause mortality after hospital discharge during a FU of 1 year. Gsslasso Cox Bayesian hierarchical models (24) were used to obtain reliable estimates of the models' coefficients. Application of this model was possible since it offers satisfactory results in the case of a correlation between cardiovascular risk predictors. The predictive power of the model was reassured by proximity to the Harrell concordance CI-index. R2mer measure of explained risk was used as model quality metric. The interpretation of the model's results was based on the hazard ratio (HR) of survival for each risk predictor. To determine the predictive value of addressed serum cardiovascular biomarker as mortality risk predictors, two variants of models were considered: 1. a model which included all significant clinical and laboratory risk factors compared to 2. a model which additionally included the investigated cardiovascular serum biomarkers as risk predictors. The predictive value of both models was compared by quality metrics (Harrell concordance CI-index, measure of explained risk) of models with the inclusion/exclusion of biomarker values. A p-value < 0.05 was regarded as statistically significant. All statistical analyses were performed on R software (version 3.6.3, R Foundation for Statistical Computing, Vienna, Austria, https://www.r-project.org).
Results
Demographic characteristics, clinical presentation at admission, relevant concomitant disease, and laboratory markers of our 280 hospitalized COVID-19 patients are presented in Table 1. COVID-19 relevant in-hospital therapies and relevant post-discharge therapies are presented in Supplementary Tables 1, 2. Arterial hypertension (AH) was the most frequent comorbidity. While 64.3% of patients required oxygen therapy, 6.4% needed support by non-invasive mechanical ventilation and 3.2% by endotracheal intubation. Hospital stays averaged 11 (10, 14) days, with an in-hospital mortality rate of 3.9%, whereas overall mortality at 1 year was 7.9%.
In Hospital Survival Analysis
The in-hospital mortality rate was 3.9% (n = 11). This patient group was significantly older than patients surviving COVID-19 pneumonia (p < 0.001) and had lower oxygen saturation (p < 0.003) resulting in higher rates of non-invasive mechanical and invasive mechanical ventilation (both p < 0.001). Similarly, comorbidities were more common in these patients, including significant impairment of renal function. Among the investigated biomarkers, only VCAM-1 (p < 0.001) was significantly elevated in non-survivors, while no significant differences were observed for sST2 but a strong trend toward a statistical significance was evident for TnI (p = 0.05, Table 2).
In the next step, investigated biomarkers were analyzed with the help of univariate Cox regression with age as the control variable. The endpoint in-hospital mortality using each biomarker was further analyzed with the help of univariate Cox regression, where age was the control variable. Table 3 presents coefficients of univariate Cox regression proportional hazards for investigated biomarkers according to in-hospital mortality. Of note, the most accurate mortality risk predictor was VCAM-1 (HR 1.086, p < 0.001). We further analyzed the differences in hospital survival rates in groups, according to the presence / absence of indicator or based on the normal / out of range laboratory and clinical parameters in patients by univariate Cox regression model analysis (Supplementary Table 3). The following variables were associated with hospital mortality with p < 0.1: age, SpO2, arterial hypertension, coronary heart disease, chronic heart failure, procalcitonin and GFR. A significance has been also shown for creatinine, urea, and existence of chronic kidney disease, but considering their direct association with GFR, only the latter was included into the multi-marker model. Furthermore, since WBC and platelets showed significant differences between non-survivors and survivors when applying the Mann-Whitney test (Table 2), we also decided to include them into the multivariable model, although no association (p > 0.1) was revealed when using the univariate Cox regression for patients with high WBC and low platelets (Supplementary Table 3). The variables WBC and platelets were added to the multivariable model as dummy variables (as 1 if WBC was > 8*109 and also as 1 if platelets were <150*109 or as 0 in the rest of the cases).
The Gsslasso Cox Bayesian hierarchical model was based on identified risk factors for in-hospital mortality. It was constructed to assess their combined impact on survival in a multi-marker model. VCAM-1, age and gender as control variables were also added to the pool of risk factors to create the multi-marker model (Figure 3). By creating the preliminary multifactor model, risk factors arterial hypertension, coronary heart disease and chronic heart failure showed no relevant significance (p > 0.05) and were excluded from the model. Harrell's C-index (CIH) of the applied multi-marker model was 0.89, which indicates its satisfactory quality. On the other hand, while removing VCAM-1 from the model, it presented lower Harrell's C-index (CIH) of 0.814 with measure of explained risk of 0.83. Consequently, based on higher model quality metrics we continued by analyzing the model which included VCAM-1. With respect to the variables age, low SpO2, GFR and platelets, WBC and procalcitonin, VCAM-1 remained an indicator associated with fatal events, while the highest HR (3689 *10139) was revealed for procalcitonin. In low SpO2, GFR and platelets variables HR was <1, which indicates that the lower range of the indicators increases in-hospital mortality (Figure 3).
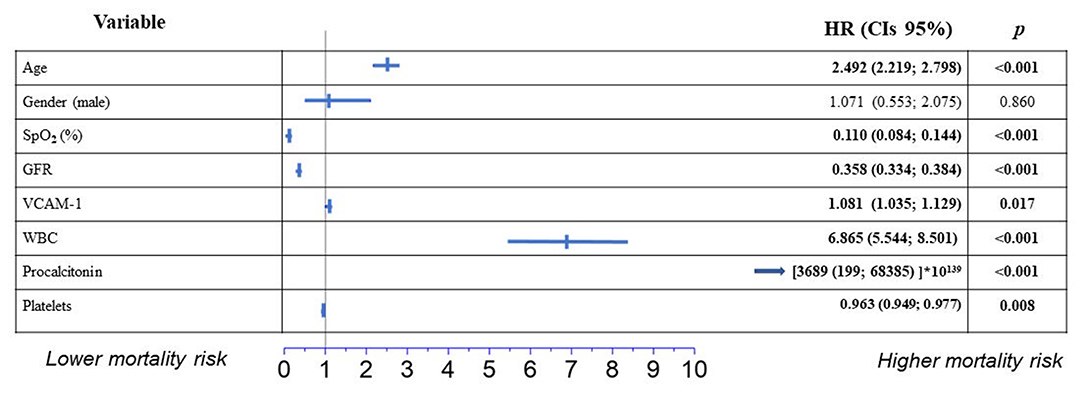
Figure 3. Independent predictors of hospital mortality from COVID-19 in multivariable survival regression (Bayesian Hierarchical Cox model) in a multi-marker model. Results are reported as hazard ratio (HR) and 95% confidence intervals (CIs).
Post Discharge 1-Year Follow-Up Survival Analysis
Among the remaining 269 patients, FU analysis was performed from discharge to 366 days. Death was registered in 11 patients (4.1%; Table 4). The deceased patients were remarkably older: 73 (61; 82, p = 0.002) vs. 59 years (49; 66), and more often had arterial hypertension (p < 0.001) but had no difference in the clinical presentation as well as lower rates of diabetes mellitus (p < 0.001) and obstructive lung disease (p = 0.011). With respect to the investigated biomarkers, only ST2 was significantly higher in the deceased group (p = 0.024). Additionally, this group more often had cardiovascular (CV) and non-CV hospitalizations (p < 0.05, Table 4). The respective biomarkers were analyzed in a next step, with respect to the endpoint 1-year post-discharge mortality, where age was the control variable. Table 5 presents coefficients of Cox univariate regression proportional hazards for investigated mortality biomarkers. According to the univariant Cox regression models, the most accurate mortality risk predictors were ST2 (HR 1.004, p < 0.001) and TnI (HR 1.28, p = 0.011).

Table 5. Univariate Cox regression for biomarkers associated with post-hospital 1-year FU mortality.
Using the Univariate Cox regression, we also analyzed the differences in survival rates of 1-year FU mortality in groups divided according to the presence / absence of risk factor based on the normal / out of range laboratory and clinical parameters. The following variables were shown to be associated with mortality with p < 0.1: procalcitonin, SpO2, urea, WBC, and arterial hypertension (Supplementary Table 4). The Gsslasso Cox Bayesian hierarchical model was constructed based on identified univariate risk factors to assess their combined impact on survival using a multi-marker model. The biomarkers ST2 and TnI as well as age and gender as a control variable were also added to the pool of risk factors to create a multi-marker model for survival. When using a preliminary multifactor model, the risk factors low SpO2, Urea, WBC, and arterial hypertension were not found to be significant (p > 0.1) and were thus excluded from the model. The Harrell's C-index (CIH) of the applied model was 0.856 with a measure of explained risk of 0.81, which indicates its satisfactory quality. While removing TnI and ST2 biomarkers from the model, Harrell's C-index (CIH) was 0.812 with the measure of explained risk of 0.730. Consequently, based on higher model quality metrics we continued by analyzing the model which included ST2 and TnI. Figure 4 presents the results of coefficients of the multivariate Bayesian Hierarchical Cox model for post-discharge all-cause mortality during 1-year FU. Age, TnI, and ST2 remained the indicators associated with post-discharge mortality during 1-year FU (Figure 4).
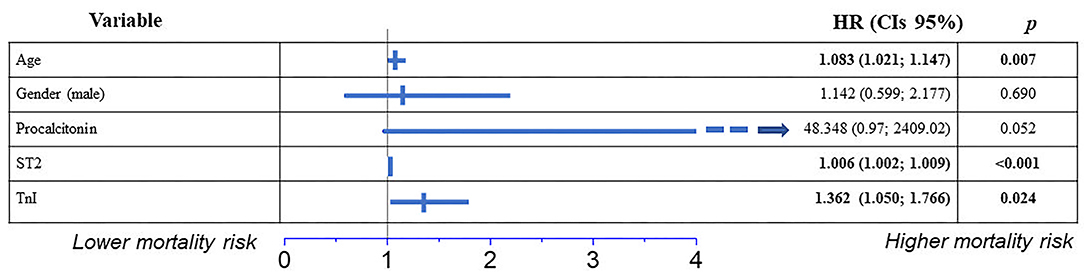
Figure 4. Independent predictors of post-hospital 1-year FU mortality in patients hospitalized with COVID-19, in a multivariate survival analysis using Cox's regression model. Results are reported as hazard ratios (HR) and 95% confidence intervals (CIs).
Discussion
The emergence of COVID-19 has posed an unprecedented challenge to clinicians around the world. COVID-19 exhibits a wide clinical spectrum ranging from asymptomatic or mild respiratory tract symptoms to the development of acute respiratory distress syndrome and death. COVID-19 places a significant strain on healthcare systems, and diagnostic tools to guide decision-making to allocate potentially limited resources are still urgently needed. The disease was shown to be correlated to a high inflammatory burden, in part resulting in multi-organ damage and respiratory failure (25, 26). This systemic inflammatory response generates a “cytokine storm” (27), also affecting endothelial function, emphasized also by histological analyses (28, 29). Thus, a stratification of disease severity through vascular biomarkers seems plausible.
In COVID-19, several clinical parameters including laboratory parameters and radiographic findings were shown to help identify high-risk patients (1). Similarly, several clinical scores have been developed to predict the disease course of COVID-19 patients (30–32). The biomarkers most frequently included in these predictive models are typically indicators of cell damage (LDH, TnT/I) and inflammatory parameters (IL-6, ferritin, or lymphocytes count) (33, 34). Nevertheless, despite promising results, the suitability of biomarkers for the assessment of outcome in COVID-19 patients remains a matter of debate. Furthermore, to maximize diagnostic power, a multi-marker approach has been promoted and also shown to enhance the sensitivity and specificity of prognostic assessments (35).
With these previous studies in mind, we therefore aimed to assess the prognostic impact of the cardiac biomarker high-sensitive TnI, along with sST2 and VCAM-1, on in-hospital mortality as well as on 1-year post discharge survival after a hospitalization due to COVID-19 pneumonia. In-hospital mortality of COVID-19 patients was indeed associated with higher levels of VCAM-1 at hospital admission, while no correlation was evident for sST2 and high-sensitive TnI. Along with VCAM-1, typical risk factors such as old age, low SpO2, GFR and platelet count, high WBC, and high levels of procalcitonin were independent indicators of mortality. Previous studies suggested that VCAM-1, and intracellular cell adhesion molecule-1 (ICAM-1) might promote the interaction between leukocytes and endothelial cells, by serving as ligands for integrins, and thus may play an important role in COVID-19 pathogenesis (36, 37). Impaired endothelial activation can lead to high accumulation of leukocytes and enhanced transmission of intracellular signals, which can result in persistent systemic inflammation and viral-induced endothelial dysfunction. This may be an underlying, unifying mechanism responsible for the widespread systemic manifestations seen with SARSCoV-2 infection (38). On this regard, also term “Acute Vascular Distress Syndrome” was introduced, to account for the quite unique vascular pathophysiology in COVID-19 pneumonia (17, 18). A key feature reported is a low ventilation-to-perfusion ratio, leading to an increased pulmonary blood flow with intrapulmonary right to left shunt (18). This mechanism might also account for the incoherence of clinical and radiographic findings and the in part atypical clinical presentation of dyspnea in COVID-19 patients (18).
Similar to our findings, a recent meta-analysis comprising 349 critically ill and 337 non-critically ill patients described significantly higher rates of VCAM-1 levels in COVID-19 patients with a proposed cut-off point of 2523.7 ng/ml for critically ill and 1921.1 ng/ml for non-critically ill patients, respectively (21). Moreover, Bauer et al. (39) conducted a comparison of critically ill COVID-19 and non-COVID-19 patients requiring intensive care treatment, which again revealed significantly higher VCAM-1 levels in the COVID-19 group (39). Additionally, a direct correlation between VCAM-1 levels and viral RNA load in plasma was noted (40). Based on these and our findings, it can be speculated that vascular involvement is an important promoter of in-hospital mortality in COVID-19. This is also in line with cardio-embolic events as frequent complications especially in severe COVID-19 (41). Furthermore, the positive results of the Recovery trial along with the reduction of cardio-embolic events through use of dexamethasone therapy further emphasize the relevance of vascular complications in the acute setting of COVID-19 (42).
With regards, to high-sensitive TnI levels, we did not find an impact on prognosis. This finding stands in contrast to previous studies (43, 44). Still, the reasons for our contradictory results might be founded in our study design. On the one hand, the lack of a clear association between high-sensitive TnI and in-hospital mortality may partly be explained by the collection of blood samples at the 1st day of hospitalization. However, virally- induced cardiac injury usually requires 1–2 weeks to develop until clinical manifestations and resultant high-sensitive TnI increases occur (5, 45). This is further emphasized by a study of Zhou et al. (5), in which serum high-sensitive TnI median concentrations increased from 57.6 to 290.6 pg/mL during the period between day 16 and day 22 after the onset of COVID-19 infection in non-survivors (5). Similar effects might be responsible for the findings of sST2. On the other hand, high-sensitive TnI levels showed a strong trend toward an increase in non-survivors, with a p-value of 0.050. Accordingly, the lack of prognostic impact might also be attributed to the small sample size of our study, which is a limitation of our study design.
Accordingly, while VCAM-1 had the best prognostic power in the assessment of in-hospital mortality, levels of sST2 were of significant prognostic value with regard to long-term prognosis. This correlates to the results of recent studies which report a significant increase in cardiovascular disease burden over 1-year follow up in COVID-19 survivors (46). Accordingly, the numbers of CV as well as non-CV hospitalizations and stroke were significantly higher in the deceased group (p < 0.05). Similar to our findings, a prognostic benefit of sST2 with respect to disease severity and mortality was also shown in a former study of 100 hospitalized COVID-19 patients (47). As soluble ST2 represents a marker of inflammation and cardiac stress, the reasons for this finding may be diverse (9). For one, higher sST2 levels might be triggered by a higher inflammatory burden (16), potentially resulting in ongoing inflammation, or even virus persistence and long-COVID-19 syndrome. Similarly, higher levels of sST2 might be promoted by diverse comorbidities. sST2 was shown to be an effective prognostic tool in long-term risk stratification in patients with heart failure, myocardial infarction, and stable coronary heart disease (48). Thus, by incorporating different pathophysiological processes, higher levels of sST2 might also point toward a more ill patient collective. The higher rates of CV and non-cardiovascular hospitalizations and stroke in the deceased group during FU (p < 0.001) further supports this suggestion. Moreover, the association of high-sensitive TnI with FU mortality matches previous studies, reporting a correlation of higher TnI/T concentrations with subsequent cardiovascular endpoints including heart failure decompensation, myocardial infarction, and viral myocarditis (49, 50).
With regards to the observed differences regarding short- and long-term prognosis, also the pathophysiological mechanisms have to be considered. VCAM-1 and ICAM-1 as well as sST2 represent circulating biomarkers (43, 44). However, circulating levels might still display differences with regards to their cellular, membrane bound forms. Given the fact, that VCAM-1 acts as a cell adhesion molecule in the context of inflammatory processes, a fast effect of an increase in VCAM-1 can be assumed (51, 52). Of note, changes in levels of VCAM-1 were reported in a comparably short timespan of days to hours (51, 52). Thus, VCAM-1 might represent a promising parameter reflecting short term effects in COVID-19, while long-term prognostic impact is limited. On the other hand, expression of sST2 is influenced by numerous comorbidities, thus reflecting an overall health status, not necessarily limited to ongoing inflammatory processes (53–55). While this might limit its impact on short-time prognosis, the incorporation of different pathophysiologic processes makes it a suitable marker for long term prognosis such as in the context of COVID-19 (53–55). From a cardiovascular aspect, sST2 further represents a marker of cardiac fibrosis and was shown to be elevated in heart failure (55). While cardiac injury was reported in the context of COVID-19, cardiac remodeling and cardiac fibrosis itself represent an ongoing process over months and years. Accordingly, worse outcomes due to cardiac fibrosis and remodeling might primarily induce undesirable long-term effects after a COVID-19 infection.
In conclusion, our study demonstrates the potential of novel cardiovascular biomarkers in the context of COVID-19. Based on the pathophysiological processes involved, VCAM-1 represents a promising prognostic marker for the assessment of in-hospital mortality in COVID-19. On the other hand, sST2 as well as high-sensitive Tn I provided prognostic value in the long-term follow-up.
Limitations
The greatest limitation of our study is the single-center design along with a comparably small sample size. This might limit the significance of our results. Thus, the findings of our study have to be considered as primarily hypothesis generating. Furthermore, the rapid evolution of COVID-19 management during the time of biomarker collection (June to August 2020) should be taken into consideration. Cardiac imaging assessments were not routinely performed in our study. Of note, advanced cardiac imaging including echocardiography, would have provided important information about potential correlations between cardiac functional impairments and the investigated biomarkers. Since only hospitalized patients were included, the results cannot be transferred to milder COVID-19 disease. Moreover, biomarkers were measured only at admission, and no FU values were assessed. Hence, no conclusions with regards to the role of tested biomarkers as potential tools for disease and therapy monitoring can be drawn. Accordingly, our data are only representative for their prognostic ability at baseline. Therefore, despite promising results, routine application of the proposed multi-marker approaches may be limited.
Data Availability Statement
The raw data supporting the conclusions of this article will be made available by the authors, without undue reservation.
Ethics Statement
The studies involving human participants were reviewed and approved by Ethic committee of the Bashkir State Medical University (N5, 2020). The patients/participants provided their written informed consent to participate in this study.
Author Contributions
LM, PJ, DG, PD, RG, IL, AT, RZ, IK, BC, BV, VP, KK, UH, ML, LF, RP, and NZ meet the criteria for authorship and contributorship as defined by the ICMJE. All authors contributed to the article and approved the submitted version.
Funding
This study was supported by grant of Russian Science Foundation No 22-18-20123.
Conflict of Interest
The authors declare that the research was conducted in the absence of any commercial or financial relationships that could be construed as a potential conflict of interest.
Publisher's Note
All claims expressed in this article are solely those of the authors and do not necessarily represent those of their affiliated organizations, or those of the publisher, the editors and the reviewers. Any product that may be evaluated in this article, or claim that may be made by its manufacturer, is not guaranteed or endorsed by the publisher.
Acknowledgments
We acknowledge the help of Ms. Zuhra M. Zaynullina for the technical support.
Supplementary Material
The Supplementary Material for this article can be found online at: https://www.frontiersin.org/articles/10.3389/fmed.2022.906665/full#supplementary-material
References
1. Ibanez B, James S, Agewall S, Antunes MJ, Bucciarelli-Ducci C, Bueno H, et al. 2017 ESC Guidelines for the management of acute myocardial infarction in patients presenting with ST-segment elevation: The Task Force for the management of acute myocardial infarction in patients presenting with ST-segment elevation of the European society of cardiology (ESC). Eur Heart J. (2018) 39:119–77. doi: 10.1093/eurheartj/ehx393
2. Zagidullin NS, Motloch LJ, Musin TI, Bagmanova ZA, Lakman IA, Tyurin AV, et al. J-waves in acute COVID-19: a novel disease characteristic and predictor of mortality? PLoS ONE. (2021) 16:e0257982. doi: 10.1371/journal.pone.0257982
3. Gumerov RM, Gareeva DF, Davtyan PA, Rakhimova RF, Musin TI, Zagidullin SZ, et al. Serum biomarkers of cardiovascular complications in COVID-19. Russian J Cardiol. (2021) 26:4456. (In Russ.) doi: 10.15829/1560-4071-2021-4456
4. Gohar A, Chong JPC, Liew OW, den Ruijter H, de Kleijn DPV, Sim D, et al. The prognostic value of highly sensitive cardiac troponin assays for adverse events in men and women with stable heart failure and a preserved vs. reduced ejection fraction. Eur J Heart Fail. (2017) 19:1638–47. doi: 10.1002/ejhf.911
5. Zhou F, Yu T, Du R, Fan G, Liu Y, Liu Z, et al. Clinical course and risk factors for mortality of adult inpatients with COVID-19 in Wuhan, China: a retrospective cohort study. Lancet. (2020) 395:1054–62. doi: 10.1016/S0140-6736(20)30566-3
6. Shi S, Qin M, Shen B, Cai Y, Liu T, Yang F, et al. Association of CardiacInjury with Mortality in Hospitalized Patients with COVID-19 in Wuhan, China. JAMA Cardiol. (2020) 5:802–10. doi: 10.1001/jamacardio.2020.0950
7. Lippi G, Lavie CJ, Sanchis-Gomar F. Cardiac troponin I in patients with coronavirus disease 2019(COVID-19): evidence from a meta-analysis. Prog Cardiovasc Dis. (2020) 63:390–1. doi: 10.1016/j.pcad.2020.03.001
8. Casula M, Montecucco F, Bonaventura A, Liberale L, Vecchié A, Dallegri F, et al. Update on the role of Pentraxin 3 in atherosclerosis and cardiovascular diseases. Vasc Pharm. (2017) 99:1–12. doi: 10.1016/j.vph.2017.10.003
9. Ciccone MM, Cortese F, Gesualdo M, Riccardi R, Di Nunzio D, Moncelli M, et al. A novel cardiac bio-marker: ST2: a review. Molecules. (2013) 18:15314–28. doi: 10.3390/molecules181215314
10. Zagidullin N, Motloch LJ, Gareeva D, Hamitova A, Lakman I, Krioni I, et al. Combining novel biomarkers for risk stratification of 2-year cardiovascular mortality in patients with ST-elevation myocardial infarction. J Clin Med. (2020) 9:550. doi: 10.3390/jcm9020550
11. Kakkar R, Lee R. The IL-33/ST2 pathway: therapeutic target and novel biomarker. Nat Rev Drug Discov. (2008) 7:827–40. doi: 10.1038/nrd2660
12. Zizzo G, Cohen PL. Imperfect storm: is interleukin-33 the Achilles heel of COVID-19 Lancet Rheumatol. Lancet Rheumatol. (2020) 2:e779–90. doi: 10.1016/S2665-9913(20)30340-4
13. Liang Y, Ge Y, Sun J. IL-33 in COVID-19: friend or foe? Cell Mol Immunol. (2021) 18:1602–4. doi: 10.1038/s41423-021-00685-w
14. Miftode RS, Petris AO, Onofrei Aursulesei V, Cianga C, Costache II, Mitu O, et al. The novel perspectives opened by ST2 in the pandemic: a review of its role in the diagnosis and prognosis of patients with heart failure and COVID-19. Diagnostics. (2021) 11:175. doi: 10.3390/diagnostics11020175
15. Zeng Z, Hong XY, Zhou H, Liao FL, Guo S, Li Y, et al. Serum soluble ST2 as a novel biomarker reflecting inflammatory status and disease severity in patients with COVID-19. SSRN Electron J. (2020) 14:1619–29. doi: 10.2217/bmm-2020-0410
16. Sánchez-Marteles M, Rubio-Gracia J, Peña-Fresneda N, Garcés-Horna V, Gracia-Tello B, Martínez-Lostao L, et al. Early Measurement of Blood sST2 is a good predictor of death and poor outcomes in patients admitted for COVID-19 infection. J Clin Med. (2021) 10:3534. doi: 10.3390/jcm10163534
17. Jounieaux V, Basille D, Toublanc B, Andrejak C, Rodenstein DO, Mahjoub Y. Case reports: bronchial mucosal vasculature is also involved in the acute vascular distress syndrome of COVID-19. Front Med. (2021) 8:710992. doi: 10.3389/fmed.2021.710992
18. Mahjoub Y, Rodenstein DO, Jounieaux V. Severe Covid-19 disease: rather AVDS than ARDS? Crit Care. (2020) 24:327. doi: 10.1186/s13054-020-02972-w
19. de Bruin S, Bos LD, van Roon MA., Tuip-de Boer AM, Schuurman AR, Koel-Simmelinck MJA, et al. Clinical features and prognostic factors in Covid-19: a prospective cohort study. Ebio Med. (2021) 67:103378. doi: 10.1016/j.ebiom.2021.103378
20. Keskinidou C, Vassiliou AG, Zacharis A, Jahaj E, Gallos P, Dimopoulou I, et al. Endothelial, immunothrombotic, and inflammatory biomarkers in the risk of mortality in critically Ill COVID-19 patients: the role of dexamethasone. Diagnostics. (2021) 1249:11. doi: 10.3390/diagnostics11071249
21. Lampsas S, Tsaplaris P, Pantelidis P, Oikonomou E, Marinos G, Charalambous G, et al. The role of endothelial related circulating biomarkers in COVID-19. A systematic review and meta-analysis [published online ahead of print, 2021 Oct 26]. Curr Med Chem. (2021). doi: 10.2174/0929867328666211026124033
22. Russian current national COVID-19 guidelines Version 7 Available online at: https://edu.rosminzdrav.ru/fileadmin/user_upload/specialists/COVID-19/MR_COVID-19_v7.pdf; 2020 (accessed July 3, 2020).
23. Unified Unified digital platform - Republican Medical Information Analytical System of the Republic of Bashkortostan Available online at: https://swantest.promedweb.ru
24. Zaim S, Chong JH, Sankaranarayanan V, Harky A. COVID-19 and multiorgan response. Curr Probl Cardiol. (2020) 45:100618. doi: 10.1016/j.cpcardiol.2020.100618
25. Tang Z, Lei S, Zhang X, et al. Gsslasso Cox: a Bayesian hierarchical model for predicting survival and detecting associated genes by incorporating pathway information. BMC Bioinformatics. (2019) 20:94. doi: 10.1186/s12859-019-2656-1
26. Mokhtari T, Hassani F, Ghaffari N, Ebrahimi B, Yarahmadi A, Hassanzadeh G. COVID-19 and Multiorgan Failure: A Narrative Review on Potential Mechanisms. J Mol Histol. (2020) 3:1–16. doi: 10.1007/s10735-020-09915-3
27. Darif D, Hammi I, Kihel A, El IdrissiSaik I, Guessous F, Akarid K. The pro-inflammatory cytokines in COVID-19 pathogenesis: what goes wrong? Microb Pathog. (2021) 153:104799. doi: 10.1016/j.micpath.2021.104799
28. Varga Z, Flammer AJ, Steiger P, Haberecker M, Andermatt R., Zinkernagel, et al. Endothelial cell infection and endotheliitis in COVID-19. The Lancet. (2020) 395:1417–8. doi: 10.1016/S0140-6736(20)30937-5
29. García de Guadiana-Romualdo L, Calvo Nieves MD, Rodríguez Mulero MD, CalcerradaAlises I, Hernández Olivo M, TrapielloFernández W, et al. MR-ProADM as marker of endotheliitis predicts COVID-19 severity. Eur J Clin Invest. (2021) e13511. doi: 10.1111/eci.13511
30. Gupta RK, Harrison EM, Ho A, Docherty AB, Knight SR, van Smeden M, et al. Development and validation of the ISARIC 4C Deterioration model for adults hospitalised with COVID-19: A prospective cohort study. Lancet Respir Med. (2021) 9:349–59. doi: 10.1016/S2213-2600(20)30559-2
31. Gutiérrez-Gutiérrez B, Del Toro MD, Borobia AM, Carcas A, Jarrín I, Yllescas M, et al. Identification and validation of clinical phenotypes with prognostic implications in patients admitted to hospital with COVID-19: A multicentre cohort study. Lancet Infect Dis. (2021) 21:783–92. doi: 10.1016/S1473-3099(21)00019-0
32. Torres-Macho J, Ryan P, Valencia J, Pérez-Butragueño M, Jiménez E, Fontán-Vela M, et al. The pandemyc score. An easily applicable and interpretable model forpredicting mortality associated with COVID-19. J Clin Med. (3066) 2020:9. doi: 10.3390/jcm9103066
33. Huang C, Wang Y, Li X, Ren L, Zhao J, Hu Y, et al. Clinical features of patients infected with 2019 novel coronavirus in Wuhan, China. Lancet. (2020) 395:497–506. doi: 10.1016/S0140-6736(20)30183-5
34. Guan WJ Ni ZY, Hu Y, Liang WH, Ou CQ, He JX, et al. Clinical characteristics of coronavirus disease 2019 in China. N Engl J Med. (2020) 382:1708–20. doi: 10.1056/NEJMoa2002032
35. Jirak P, Fejzic D, Paar V, Wernly B, Pistulli R, Rohm I, et al. Influences of Ivabradine treatment on serum levels of cardiac biomarkers sST2, GDF-15, suPAR and H-FABP in patients with chronic heart failure. Acta Pharm Sin. (2018) 39:1189–96. doi: 10.1038/aps.2017.167
36. Leite AR, Borges-Canha M, Cardoso R, Neves JS, Castro-Ferreira R, Leite-Moreira A. Novel biomarkers for evaluation of endothelial dysfunction. Angiology. (2020) (5):397–410. doi: 10.1177/0003319720903586
37. Tong M, Jiang Y, Xia D, Xiong Y, Zheng Q, Chen F, et al. Elevated expression of serum endothelial cell adhesion molecules in COVID-19 patients. J Infect Dis. (2020) 222:894–8. doi: 10.1093/infdis/jiaa349
38. Prasad M, Leon M, Lerman LO, Lerman A. Viral endothelial dysfunction: a unifying mechanism for COVID-19. Mayo Clin Proc. (2021) 96:3099–108. doi: 10.1016/j.mayocp.2021.06.027
39. Bauer W, Ulke J, Galtung N, Strasser-Marsik LC, Neuwinger N, Tauber R, et al. Role of cell adhesion molecules for disease development of patients with and without COVID-19 in the emergency department. J Infect Dis. (2021) 223:1497–99. doi: 10.1093/infdis/jiab042
40. Bermejo-Martin JF, González-Rivera M, Almansa R, Micheloud D, Tedim AP, Domínguez-Gil M, et al. Viral RNA load in plasma is associated with critical illness and a dysregulated host response in COVID-19. Crit Care Lond Engl. (2020) 1:691. doi: 10.1186/s13054-020-03398-0
41. Connors JM, Levy JH. COVID-19 and its implications for thrombosis and anticoagulation. Blood. (2020) 135:2033–40. doi: 10.1182/blood.2020006000
42. Jirak P, van Almsick V, Dimitroulis D, Mirna M, Seelmaier C, Shomanova Z, et al. Dexamethasone improves cardiovascular outcomes in critically Ill COVID-19, a real world scenario multicenter analysis. Front Med. (2022) 9:808221. doi: 10.3389/fmed.2022.808221
43. Lala A, Johnson KW, Januzzi JL, et al. Prevalence and impact of myocardial injury in patients hospitalized with COVID-19 infection. J Am Coll Cardiol. (2020) 76:533–46. doi: 10.1016/j.jacc.2020.06.007
44. Maino A, Di Stasio E, Grimaldi MC, Cappannoli L, Rocco E, Vergallo R, et al. Prevalence and characteristics of myocardial injury during COVID-19 pandemic: a new role for high-sensitive troponin. Int J Cardiol. (2021) 338:278–85. doi: 10.1016/j.ijcard.2021.06.028
45. Schultheiss HP, Baumeier C, Aleshcheva G, Bock CT, Escher F. Viral myocarditis-from pathophysiology to treatment. J Clin Med. (2021) 10:5240. Doi :10.3390/jcm10225240
46. Abers MS, Delmonte OM, Ricotta EE, Fintzi J, Fink DL, de Jesus AAA, et al. An immune-based biomarker signature is associated with mortality in COVID-19 patients. JCI Insight. (2021) 6:144455. doi: 10.1172/jci.insight.144455
47. Bayes-Genis A, Richards AM, Maisel AS, Mueller C, Ky B. Multimarker testing with ST2 in chronic heart failure. Am J Cardiol. (2015) 115:76B−80B. doi: 10.1016/j.amjcard.2015.01.045
48. Berg DD, Freedman BL, Bonaca MP, Jarolim P, Scirica BM, Goodrich EL, et al. Cardiovascular biomarkers and heart failure risk in stable patients with atherothrombotic disease: a nested biomarker study from TRA 2°P-TIMI 50. J Am Heart Assoc. (2021) 10:e018673. doi: 10.1161/JAHA.120.018673
49. Thygesen K, Alpert JS, Jaffe AS, Chaitman BR, Bax JJ, Morrow DA, et al. Fourth universal definition of myocardial infarction (2018). J Am Coll Cardiol. (2018) 30:2231–64. doi: 10.1016/j.jacc.2018.08.1038
50. Kontos MC, Turlington JS. High-sensitivity troponins in cardiovascular disease. Curr Cardiol Rep. (2020) 22:30. doi: 10.1007/s11886-020-01279-0
51. Kong DH, Kim YK, Kim MR, Jang JH, Lee S. Emerging roles of vascular cell adhesion molecule-1 (VCAM-1) in immunological disorders and cancer. Int J Mol Sci. (2018) 19:1057. doi: 10.3390/ijms19041057
52. Barreiro O, Yanez-Mo M, Serrador JM, Montoya MC, Vicente-Manzanares M, Tejedor R, et al. Dynamic interaction of VCAM-1 and ICAM-1 with moesin and ezrin in a novel endothelial docking structure for adherent leukocytes. J Cell Biol. (2002) 157:1233–45. doi: 10.1083/jcb.200112126
53. Ip C, Luk KS, Yuen VLC, et al. Soluble suppression of tumorigenicity 2 (sST2) for predicting disease severity or mortality outcomes in cardiovascular diseases: a systematic review and meta-analysis. Int J Cardiol Heart Vasc. (2021) 37:100887. doi: 10.1016/j.ijcha.2021.100887
54. Jirak P, Mirna M, Wernly B, Paar V, Thieme M, Betge S, et al. Analysis of novel cardiovascular biomarkers in patients with peripheral artery disease. Minerva Med. (2018) 109:443–50. doi: 10.23736/S0026-4806.18.05628-8
Keywords: COVID-19, long COVID-19, post-discharge mortality, cardiovascular biomarkers, sST2, VCAM-1
Citation: Motloch LJ, Jirak P, Gareeva D, Davtyan P, Gumerov R, Lakman I, Tataurov A, Zulkarneev R, Kabirov I, Cai B, Valeev B, Pavlov V, Kopp K, Hoppe UC, Lichtenauer M, Fiedler L, Pistulli R and Zagidullin N (2022) Cardiovascular Biomarkers for Prediction of in-hospital and 1-Year Post-discharge Mortality in Patients With COVID-19 Pneumonia. Front. Med. 9:906665. doi: 10.3389/fmed.2022.906665
Received: 28 March 2022; Accepted: 30 May 2022;
Published: 28 June 2022.
Edited by:
Yazine Mahjoub, University Hospital Center (CHU) of Amiens, FranceReviewed by:
Maria Chiara Grimaldi, Catholic University of the Sacred Heart, ItalyMomar Diouf, University Hospital Center (CHU) of Amiens, France
Copyright © 2022 Motloch, Jirak, Gareeva, Davtyan, Gumerov, Lakman, Tataurov, Zulkarneev, Kabirov, Cai, Valeev, Pavlov, Kopp, Hoppe, Lichtenauer, Fiedler, Pistulli and Zagidullin. This is an open-access article distributed under the terms of the Creative Commons Attribution License (CC BY). The use, distribution or reproduction in other forums is permitted, provided the original author(s) and the copyright owner(s) are credited and that the original publication in this journal is cited, in accordance with accepted academic practice. No use, distribution or reproduction is permitted which does not comply with these terms.
*Correspondence: Lukas J. Motloch, bC5tb3Rsb2NoQHNhbGsuYXQ=
†These authors have contributed equally to this work