Effect of SARS-CoV-2 infection on asthma patients
- 1Department of Interventional Therapy, Multidisciplinary Team of Vascular Anomalies, Shanghai Ninth People’s Hospital, Shanghai Jiao Tong University, Shanghai, China
- 2Department of Neurosurgery, Shanghai Ninth People’s Hospital, Shanghai Jiao Tong University, Shanghai, China
- 3Affiliated Hospital of Weifang Medical University, School of Clinical Medicine, Weifang Medical University, Weifang, China
Background: SARS-CoV-2 causes coronavirus disease 2019 (COVID-19), a new coronavirus pneumonia, and containing such an international pandemic catastrophe remains exceedingly difficult. Asthma is a severe chronic inflammatory airway disease that is becoming more common around the world. However, the link between asthma and COVID-19 remains unknown. Through bioinformatics analysis, this study attempted to understand the molecular pathways and discover potential medicines for treating COVID-19 and asthma.
Methods: To investigate the relationship between SARS-CoV-2 and asthma patients, a transcriptome analysis was used to discover shared pathways and molecular signatures in asthma and COVID-19. Here, two RNA-seq data (GSE147507 and GSE74986) from the Gene Expression Omnibus were used to detect differentially expressed genes (DEGs) in asthma and COVID-19 patients to find the shared pathways and the potential drug candidates.
Results: There were 66 DEGs in all that were classified as common DEGs. Using a protein-protein interaction (PPI) network created using various bioinformatics techniques, five hub genes were found. We found that asthma has some shared links with the progression of COVID-19. Additionally, protein-drug interactions with common DEGs were also identified in the datasets.
Conclusion: We investigated possible links between COVID-19 and asthma using bioinformatics databases, which might be useful in treating COVID-19 patients. More studies on populations affected by these diseases are needed to elucidate the molecular mechanism behind their association.
Introduction
SARS-CoV-2 is a serious infectious illness that risks people’s health worldwide (1). Fever, cough, and dyspnea are the most common clinical symptoms of COVID-19 infection. There are also rare cases with unusual symptoms, such as diarrhea, headaches, and a loss of smell (2). Although most patients have mild symptoms, some develop severe pneumonia and multi-organ failure and eventually die (3). COVID-19 spreads primarily through the air and touch, and elderly individuals with weakened immune systems and chronic diseases are more vulnerable to infection and have a higher fatality rate than healthy young people (4). Recurrent episodes of wheezing, shortness of breath, chest tightness, and coughing are among the clinical signs of asthma, which is often linked with high rates of morbidity and death (5, 6). Airway remodeling is an essential feature of asthma, manifested by structural changes in the airways, such as basement membrane thickening, bronchial fibrosis, airway smooth muscle cell hyperplasia, and hypertrophy (6–8). Among COVID-19 patients, uncontrolled asthma was identified as contributing factor to mortality, and patients also had more severe asthma manifestations during the pandemic (9, 10). Therefore, considering the current COVID-19 epidemic and the high prevalence of asthma, the study results are timely. To determine the biological connection between asthma and COVID-19, this study examined two datasets. GSE147507 and GSE74986 were for COVID-19 and asthma, respectively, and were obtained from the Gene Expression Omnibus (GEO) database. Differentially expressed genes (DEGs) were identified for the datasets, and the common DEGs for both diseases were obtained. Following research, including pathway analysis and enrichment analysis, were conducted based on the common DEGs to better understand the biological processes of genome-wide expression studies. Finally, the potential drugs were suggested. Figure 1 displays the entire workflow of our research.
Materials and methods
Identification of the common differentially expressed genes between asthma and COVID-19
The GEO database from the National Center for Biotechnology Information was used to download the gene expression profiles and to determine the common genetic interrelationships (11). The GEO accession number for the SARS-CoV-2 dataset was GSE147507, a transcriptional analysis of the response from COVID-19 lung biopsies to respiratory tract infections by extracting RNA sequences using a high-throughput sequencing Illumina NextSeq 500 platform (12). The asthma dataset was GSE74986, where the experimental platform was based on Agilent’s GPL6480 platform. The GSE74986 dataset contained 86 samples of cellular RNA from the bronchoalveolar lavage fluid of 86 subjects, including 74 samples from asthmatic subjects and 12 from normal subjects. The DEGs analysis was conducted using the R programming language. DEGs were identified using false discovery rate (FDR) ≤ 0.05, P < 0.05 and |log2 (fold change)| ≥ 1. Further, the Lim package in R software was used to analyze the DEGs, and the results were visualized through the R package. An online Venn analysis tool, jvenn, was used to obtain the common DEGs between GSE147507 and GSE74986.
Construction of protein-protein interaction and hub gene screening analysis
Protein interactions are essential for most biological processes, and a PPI network is fundamental in understanding an organism’s functional and structural units. Thus, PPI networks provide a graph of cellular mechanisms (13). The online analysis website Search Tool for the Retrieval of Interacting Genes/Proteins (STRING)1 was used to construct the PPI network derived from the common DEGs and to display the functional and physical interactions between asthma and COVID-19, with a PPI combined score of >0.4 as a threshold condition. After constructing the PPI network of DEGs, the top five hub genes in the network were screened using the degree algorithm of the Cytoscape cytoHubba plugin (14). By examining the connectivity of the nodes in the PPI networks, the hub genes were determined. The cytoHubba plugin was used to distinguish the hub genes from the DEGs based on Maximal Clique Centrality (MCC) algorithm (15). Using the MCC approach of cytoHubba, the top five hub genes from the PPI network were identified.
Gene ontology enrichment and Kyoto encyclopedia of genes and genomes pathway analysis
Functional enrichment analysis is often used in the genome and functional genome research to help researchers gain insights into biological significance, such as biological processes or chromosome positions related to different interrelated diseases (16, 17). The Enrichr database2 integrates 35 databases and offers a wide range of user-friendly enrichment analysis arrangements and visualizations. The enrichment analysis was calculated in three ways: first, using the conventional Fisher test. Second, a modified Fisher test, i.e., ranking using the z-score. Third, combining Fisher’s p-value and z-score in a combined ranking, i.e., the combined score. Fisher’s exact test is a statistical significance test. In this study, the gene ontology (GO) of DEGs and Kyoto encyclopedia of genes and genomes (KEGG) were analyzed by the Enrichr database. P-values < 0.05 were considered a standard metric for quantifying the above-listed pathways.
Recognition of the transcription factors and miRNAs
Transcription factors (TFs) are proteins that bind to specific DNA sequences. In a disease condition, TFs control the expression of genes by interacting with their target genes during the transcription phase (18). The interaction network between DEGs-TFs was constructed using the JASPAR database3 built-in NetworkAnalyst. JASPAR is the most comprehensive publicly available database for transcription factor-DNA binding site motifs. The data in the JASPAR database are rigorously selected for their experimental validity, identified and matched by computer-aided software, and annotated by biological means (19, 20). The data is loaded for reanalysis into NetworkAnalyst, a visual analysis platform for comprehensive gene expression analysis (21). The Tarbase and mirTarbase databases were used to build a gene-miRNAs interaction network through NetworkAnalyst (22–24). In Cytoscape, a gene regulatory network containing DEGs, TFs, and miRNAs was built. This tool screens the top miRNAs with a high degree of biological function and characterization to derive valid biological hypotheses.
Screening of the target drug candidates
The Drug Response Gene Signature Database (DSigDB), which incorporates drug response microarray data from public databases and scientific literature, is accessible via the online enrichment analysis tool Enrichr. It also provides drug and target information by screening the top 500 up-and down-regulated genes for drug signatures to create a DSigDB (25, 26). The current DSigDB has around 1,300 medicines, 7,000 microarrays, and 800 targets, allowing computational drug repositioning to be used to design innovative drugs targeting key genes (26). In this study, DSigDB was used to screen candidate drugs interacting with the hub genes to provide a reference for targeted therapy of diseases. P < 0.05 was considered statistically significant.
Gene-disease association of differentially expressed genes
DisGeNET4 is one of the largest and most comprehensive human gene-disease association repositories, synchronizing relationships from several sources and featuring various biomedical aspects of diseases (27, 28). DisGeNET now has over 24,000 illnesses, 17,000 genes, and 117,000 genomic variations. NetworkAnalyst was also utilized to investigate gene-disease connections in order to find illnesses and chronic issues linked to common DEGs.
Results
Identification of the common differentially expressed genes between asthma and COVID-19
To identify the common DEGs between COVID-19 and asthma patients, we extracted all the all significant DEGs with FDR ≤ 0.05 and |log2 (fold change)| ≥ 1 were extracted. In the GSE74986 dataset, 589 DEGs were identified, of which 43 were highly expressed, and 546 were lowly expressed genes. In the GSE147507 dataset, 1,184 DEGs were identified, of which 293 were highly expressed, and 891 were lowly expressed genes. We identified 66 common DEGs from asthma and SARS-CoV-2 datasets (Figure 2 and Table 1). A Venn diagram was generated using jvenn web.5 This common gene set was used for further experiments. A Venn diagram was generated using jvenn web (see text footnote 5) as previously described (28). This common gene set was used for further experiments.
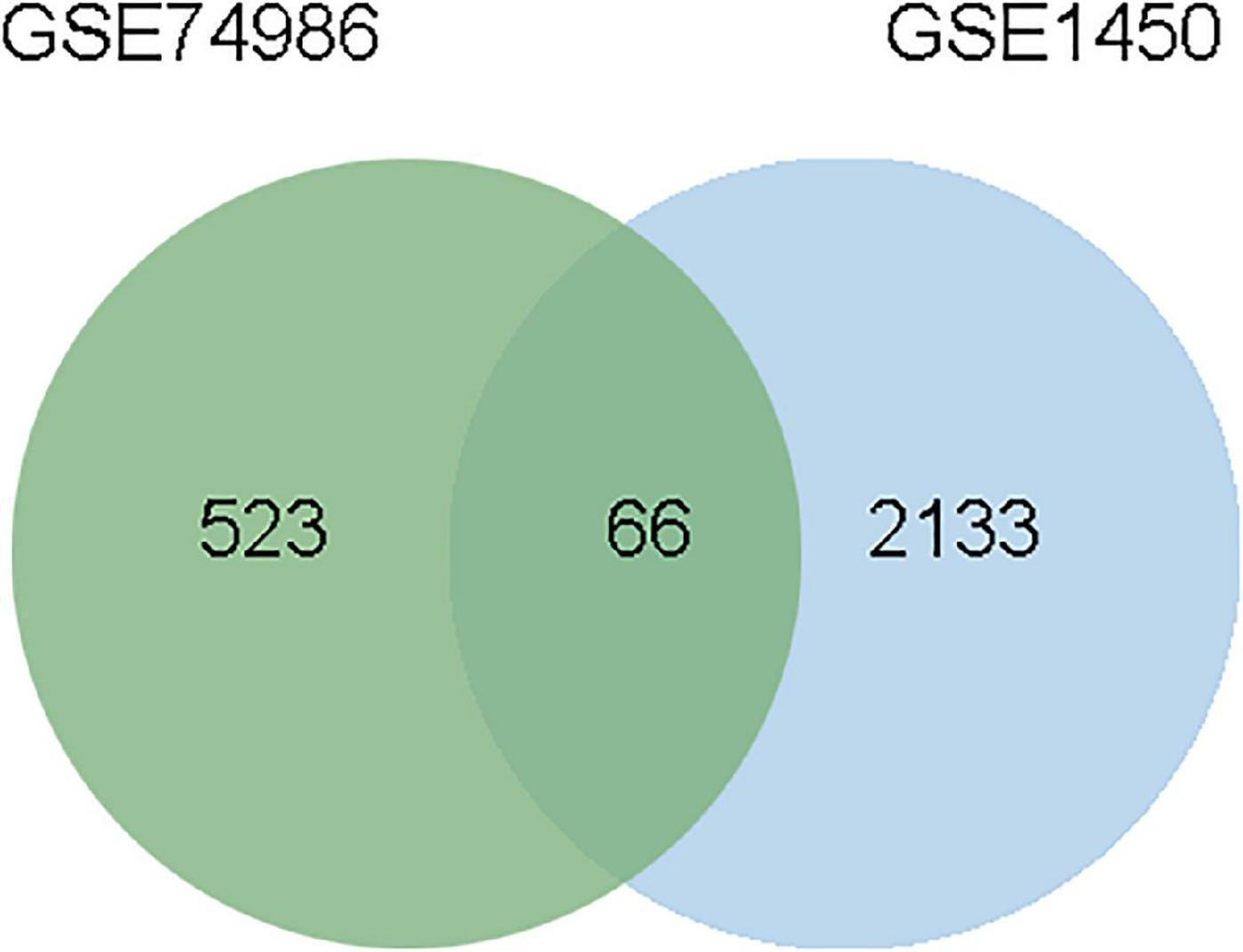
Figure 2. This study incorporates asthma (GSE74986), and SARS-CoV-2 (GSE147507). This integrated analysis revealed 66 common DEGs are shared among SARS-CoV-2 and asthma.
Gene ontology and pathway enrichment analysis
The online tool Enrichr (see text footnote 2) was used for gene enrichment analysis and to determine the biological significance, and enrichment pathways of the DEGs shared in this study (29). The GO database is a comprehensive resource of computable knowledge on the function of genes and gene products (30). GO annotations in biological process (BP), molecular function (MF), and cellular component (CC) were retrieved from the GO database (31). Supplementary Table 1 displays the top 10 enriched GO categories of BP, CC, and MF. For BP, the genes were mainly enriched in regulation of transcription, DNA-templated, regulation of RNA metabolic process, and positive regulation of smooth muscle cell proliferation. For CC, genes were mainly enriched in the nucleus. For MF analysis, the module showed predominant enrichment in cyclin-dependent protein serine/threonine kinase regulator activity. According to the KEGG analysis, the DEGs were primarily abundant in the p53 signaling pathway, legionellosis, transcriptional misregulation in cancer, and PI3K-Akt signaling pathway. Figure 3 represents the pathway enrichment analysis in the bar graphs. In addition, GO, KEGG pathway analysis, Gene counts, and gene symbols were shown in Supplementary Tables 2, 3, respectively.
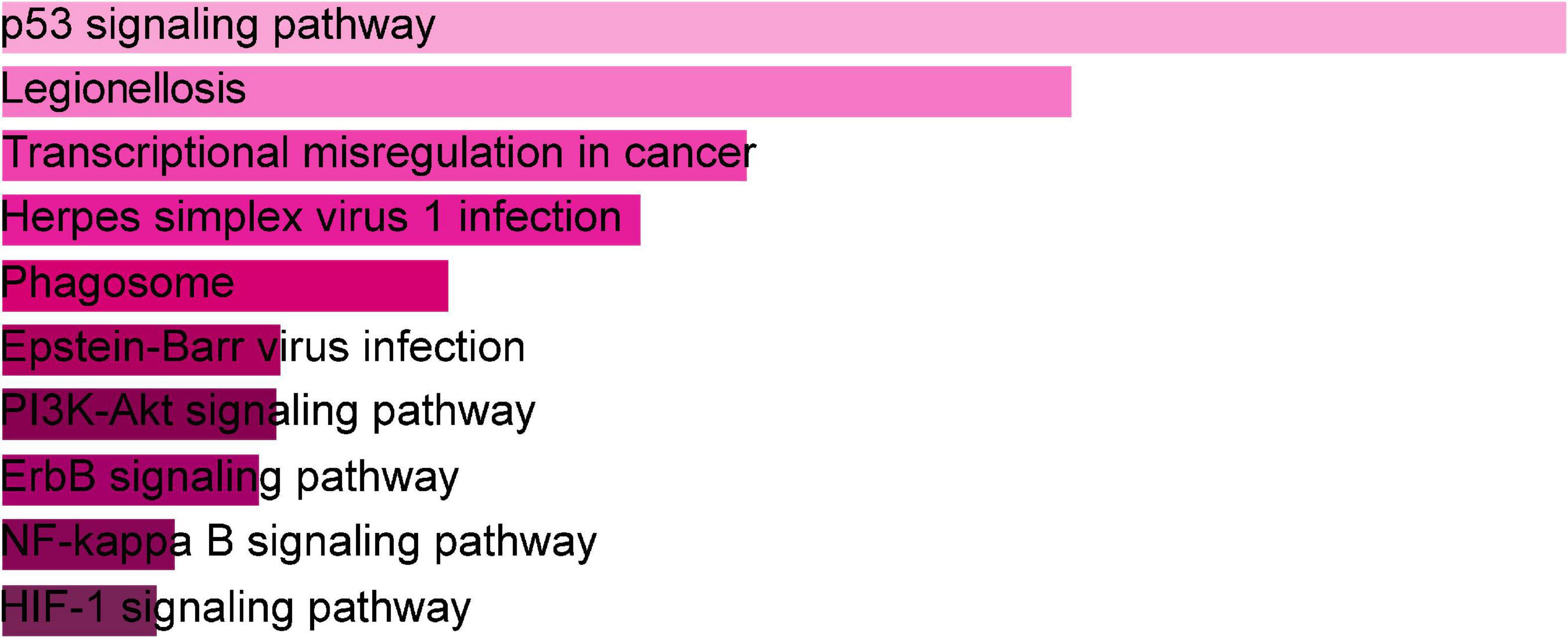
Figure 3. The bar graphs of pathway enrichment analysis of shared DEGs among SARS-CoV-2, asthma performed by the Enricher online tool.
Classification of the hub genes and submodules
To forecast the contacts and adhesion routes of the common DEGs, we investigated the PPI network created by STRING and displayed it in Cytoscape. The PPI network of the DEGs contained 64 nodes and 166 edges, where the most tangled nodes were the hub genes (Figure 4A). The top five hub genes with the highest MCC scores were determined using Cytoscape’s cytoHubba plugin. The hub genes were CLK4, CLK1, CHD1, CCNL1, and CCNT2 (Figure 4B). In addition, we conducted a ROC analysis for asthma and COVID-19 (Supplementary Figure 1). Among these five hub genes in the COVID-19 cohort, all had AUC values larger than 0.65, with 3 hub genes with AUC values larger than 0.7. In the asthma cohort, the AUC values of all hub genes were greater than 0.6. These hub genes could serve as potential biomarkers and might lead to new therapeutic strategies.
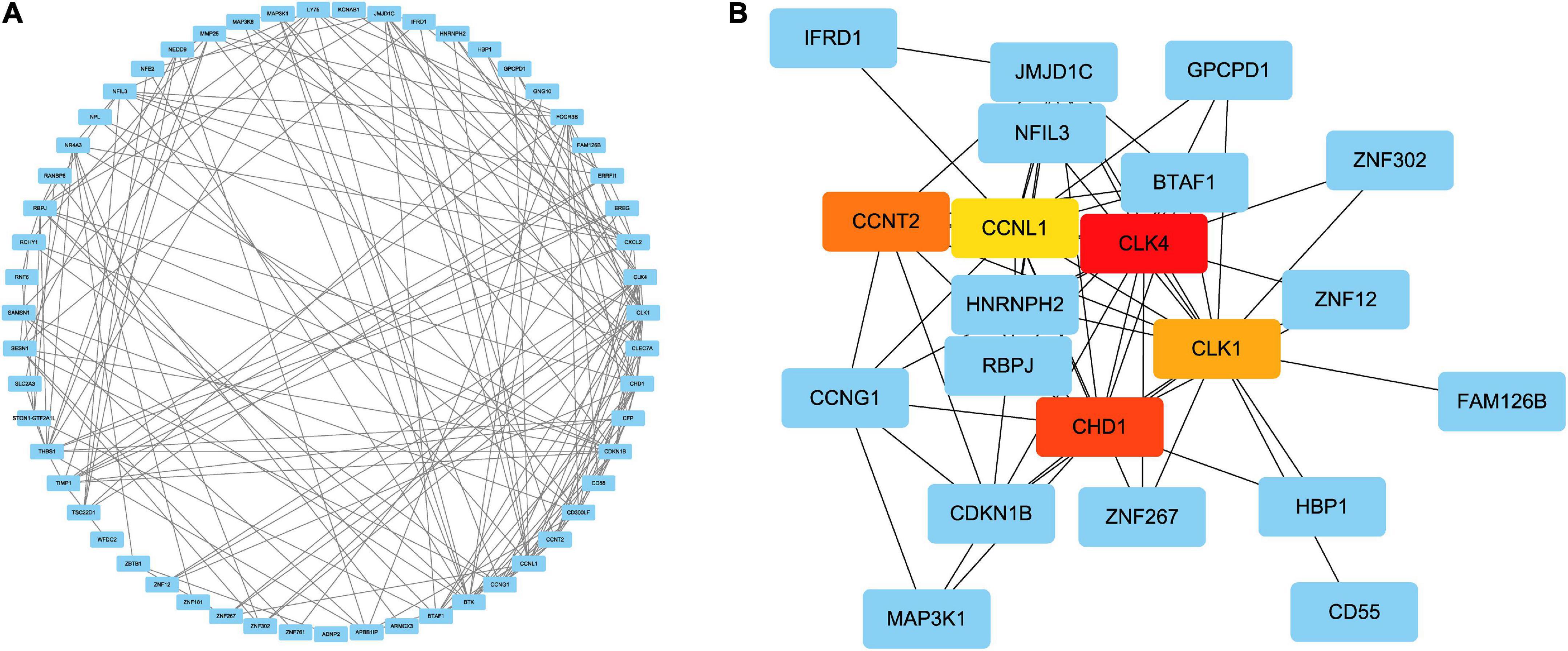
Figure 4. (A) PPI network of common DEGs among SARS-CoV-2 and asthma. In the figure, the circle nodes represent DEGs and edges represent the interactions between nodes. The PPI network has 64 nodes and 166 edges. (B) Determination of hub genes from the PPI network by using the Cytohubba plugin in Cytoscape. The latest MCC procedure of Cytohubba plugin was pursued to obtain hub genes. Here, the red nodes indicate the highlighted top five hub genes and their interactions with other molecules.
Determination of regulatory networks
Transcription factors and miRNAs are critical regulators of gene expression at the transcriptional and post-transcriptional levels (32). TFs and miRNAs can work together to control the same genes. TFs regulate the transcription of miRNAs, and a common set of target lncRNAs are regulated by both TFs and miRNAs. TFs and miRNAs together make up a complex regulatory network (33). From TFs and miRNAs gene interaction network analysis, we established 26 TFs and 1,123 post-transcriptional (miRNAs) regulatory signatures regulated by more than one common DEG, indicating a strong interaction between them (Figure 5). We also constructed and analyzed regulatory networks of target TF and target miRNA genes, including topology tables (Supplementary Tables 4, 5).
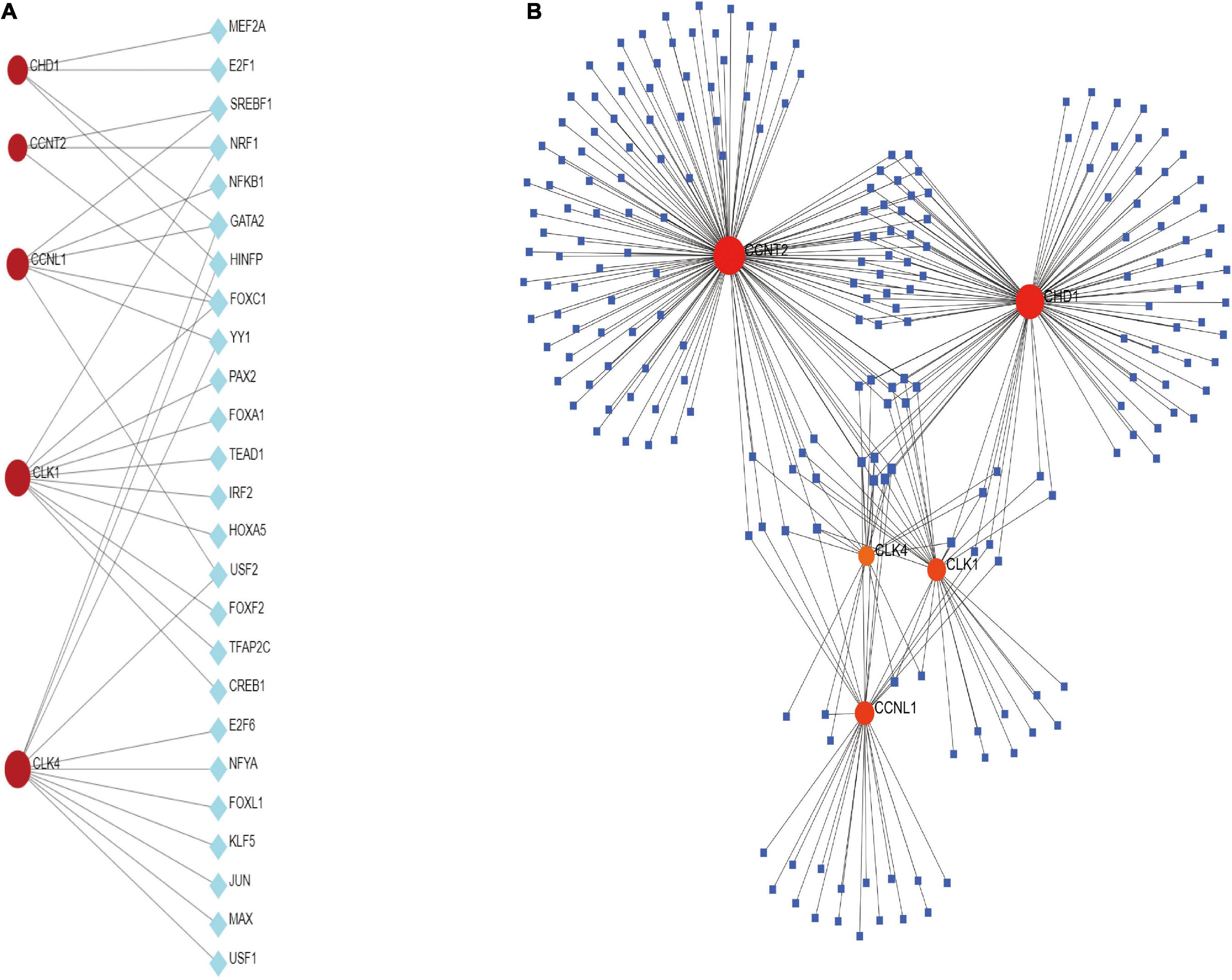
Figure 5. (A) The cohesive regulatory interaction network of DEG–TFs obtained from the Network Analyst. (B) The interconnected regulatory interaction network of DEGs–miRNAs.
Gene-disease associations
Numerous studies have demonstrated that a common or related gene set is involved in similar or related diseases, allowing us to uncover the relationships between genes and diseases (34, 35). NetworkAnalyst was used to analyze the gene-disease association. We found that endometriosis, dermatitis, allergic contact, rheumatoid arthritis, and juvenile arthritis were mainly associated with our reported hub genes (Figure 6).
Identification of candidate drugs
The interaction between the drug and the protein is vital to understand the structural features recommended for receptor sensitivity. DSigDB contains the largest number of drugs/compound-related gene sets to date. According to the DSigDB database, drug molecules were suggested from the common DEGs. The top 10 chemical compounds were extracted based on their p-values (Table 2). Therefore, these drugs could potentially be used as common chemical compounds for treating the two diseases.
Discussion
Mahmud’s work on the impact of SARS-CoV-2 infection in individuals with idiopathic pulmonary fibrosis and chronic obstructive lung disease inspired this investigation (28). We explored a unique perspective of the potential interaction between asthma and COVID-19. Our primary objective was to examine the association between asthma and COVID-19. As a chronic respiratory disease, asthma is characterized by an inherent chronic airway inflammation involving multiple immune cells (eosinophils and mast cells), usually accompanied by increased airway reactivity. Often, acute exacerbations of asthma after pneumonia not only increase patient visits and hospital admissions but also lead to adverse clinical outcomes, such as respiratory failure or death. Additionally, patients with severe asthma take high doses of systemic corticosteroids for long periods, leading to systemic diseases (e.g., hypertension and diabetes) and thus increasing mortality after pneumonia.
The COVID-19 infection is a multi-organ disease characterized by the activation of cellular and innate immunity, often resulting in lung inflammation (36). Hence, COVID-19 infection is a high-risk factor for asthma exacerbations. Meanwhile, studies show that virus-associated respiratory infections are particularly severe in patients with asthma. Some studies also suggest that adults with asthma are at a higher risk of developing severe COVID-19 (37). Furthermore, the Centers for Disease Control and Prevention has identified asthma as a possible risk factor for COVID-19 after treating asthmatic patients with severe viral infections (38). Previous studies have shown that in addition to age, gender, smoking, and several co-morbidities (e.g., obesity, type 2 diabetes, hypertension, etc.), ongoing medication might also be strongly associated with the severity of COVID-19 and adverse clinical outcomes (39). Usually, the prognosis is poor when the co-morbidity is a risk factor, especially in patients with COPD. However, the association of asthma, another major chronic airway disease, with the clinical outcomes of COVID-19 is still unclear.
Here, we developed a general co-expression network-based approach to analyze both gene sets in the microarray data that might act as potential biomarkers of COVID-19. Together, they provide critical information about the disease progression and the development of drug resistance and contribute to discovering new therapeutic approaches. The investigation of asthma and COVID-19 transcriptomics indicated that the common 66 DEGs had comparable expression patterns in both illnesses. Based on the P-values, these DEGs were analyzed using the GO pathway analysis function to give insights into the biological relevance in the etiology of asthma and COVID-19.
Gene ontology is a consortium-based dataset that provides information on the functions of genes. In BP, regulation of transcription and DNA-templated were among the top GO terms. In MF, cyclin-dependent protein serine/threonine kinase regulator activity and protein kinase regulator activity were the top two GO pathways. Under CC, nucleus and intracellular membrane-bounded organelle were the top two GO terms. To establish a comparable pathway for asthma and COVID-19, the KEGG pathway of the 66 shared DEGs was found. The top KEGG human pathway includes the p53 signaling pathway, Legionnaires’ disease, transcriptional misregulation in cancer, herpes simplex virus 1 infection, and phagosome. The host protease TM-PRSS2 cleaves the S protein on the surface of SARS-CoV-2 after the S protein binds to ACE2, prompting virus entry into the host cell (40). Earlier studies reported that p53 inhibits coronavirus replication by degrading ACE2 in humans. Deleting the p53 binding site in porcine kidney cells increases ACE2 promoter activity (41).
A PPI network of DEGs was constructed using the STRING database to uncover the potential association between DEGs. Finding hub genes from common DEGs is of utmost importance because the discovery of drug molecules is primarily dependent upon them. We found five hub proteins (CLK4, CLK1, CHD1, CCNL1, and CCNT2) involved in these diseases. Here, the cutoff of the topological metric for the hub genes was 10 (degrees). The CLK family consists of four isoforms: CLK1, CLK2, CLK3, and CLK4 (42). CLK1 and CLK4 are very similar in their amino-acid sequence (42). CLK1 is vital in splicing the H1N1 influenza virus M2 gene and is a critical anti-influenza target (43). CHD1 is composed of 1,710 amino acids and plays an essential role in transcriptional regulation and development (44). CHD1 controls chromatin structural regions through chromatin accessibility and nucleosome depolymerization and plays a role in the development and progression of prostate cancer (45). CCNL1 is a member of the cell cycle protein family. It is involved in the precursor mRNA shearing and RNA processing and is associated with the action of cell cycle protein-dependent kinases (46). Additionally, CCNL1 may contribute to cell survival during cellular stress, such as cold stimulation (47). CCNT2 is a cyclin involved in the cell cycle and RNA transcription (48). Thus, the identified hub genes could be potential biomarkers and new drug targets. However, more studies are needed to explore the biological insights of COVID-19.
Understanding how miRNAs downregulate target genes and TFs regulate miRNAs during post-transcriptional regulations is critical. Hence, we investigated TFs and miRNAs that potentially regulate the identified DEGs. The identified TFs are associated with different functions, such as cell development and cancer. Further, some miRNAs are involved in lung cancer (hsa-mir-335-5p), infectious diseases (hsa-mir-16-5p), and hematological malignancies (hsa-miR-155-5p) (49). Most miRNAs are associated with cancerous tissues and cause different cancers in the human body. We performed a gene-disease analysis to predict the association of important DEGs with different diseases. The results showed that COVID-19 is associated with various diseases, including lung tumors, allergic diseases, liver, skin, and joints diseases. People with lung diseases are at a higher risk of COVID-19 (50). Our study also identified several genes associated with lung diseases, such as lung cancer. Our network analysis also found some dermatitis, such as allergic contact. Recently, skin diseases in patients with COVID-19 infection were reported in Italy. Side effects of COVID-19 infection include itchy skin. The liver damage from COVID-19 is directly related to the cytopathic effects of the virus, derangement of the immune response, and drug damage. In patients with rheumatoid arthritis, various immune cells are abnormally activated, causing immune dysfunction and making the body susceptible to pathogenic microbial infections, including COVID-19 (51).
Although no standard drugs or vaccines are available for COVID-19, several chemicals and drugs are being investigated as treatment agents (52). We have identified a variety of drugs as potential therapeutic agents. Researchers have found that ouabain is resistant to SARS-CoV-2 and inhibits over 99% of SARS-CoV-2 replication, resulting in suppression of the virus in the post-life cycle entry phase (53). Ouabain might serve as an alternative therapy to COVID-19 and has potential additional therapeutic benefits for patients with cardiovascular disease (53). Fasudil, a selective ROCK inhibitor, plays various roles with T cells (54). Paclitaxel, an anti-tumor drug, can increase the levels of methylglyoxal in cells, providing a powerful reason to repurpose Doxorubicin and Paclitaxel for COVID-19 treatment (55). Daunorubicin is a DNA topoisomerase II, known to disrupt either the catalytic cycle of topoisomerase II (catalytic inhibitors) or stabilize the topoisomerase II–DNA transient cleavage complex (topoisomerase II poisons). M. Bouachrine study suggests Digitoxigenin as an inhibitor against SARS-CoV-2 (56). Mitoxantrone is used in diseases related to anti-malignant disorders, including leukemia, lymphoma, and lung cancer (57). In addition to respiratory tumors, COVID-19 infections involve different tumor sites; therefore, all these drugs could potentially treat COVID-19 infections (58). However, several potential limitations of the study must be acknowledged. No functional and mechanistic experiments were conducted to validate the GO and KEGG results. In addition, we did not perform mechanistic experiments to confirm the results from computational analysis. Therefore, more prospective studies are needed to confirm our results.
Conclusion
Our study used transcriptome analysis to summarize the relationship between asthma and COVID-19. We obtained DEGs from GEO datasets, identified the common genes, and found an association between asthma and SARS-CoV-2. Further, a PPI network was generated using the 66 common genes, and the five most essential hub genes were identified from the PPI network. All hub genes play important roles in different types of respiratory diseases. Therefore, the genes we identified could potentially be a new therapeutic target for developing the COVID-19 vaccine.
Data availability statement
The datasets presented in this study can be found in online repositories. The names of the repository/repositories and accession number(s) can be found in the article/Supplementary material.
Author contributions
All authors made substantial contributions to conception and design, acquisition of data, or analysis and interpretation of data; took part in drafting the article or revising it critically for important intellectual content; agreed to submit to the current journal; gave final approval of the version to be published; and agreed to be accountable for all aspects of the work.
Acknowledgments
We would like to show our respect and gratitude to all the health workers who are at the front line of the outbreak response and fighting against COVID-19 in China. We would also like to thank Bullet Edits Limited for the linguistic editing and proofreading of the manuscript and Figdraw (www.figdraw.com) for its help and support (ID: WATAI44401).
Conflict of interest
The authors declare that the research was conducted in the absence of any commercial or financial relationships that could be construed as a potential conflict of interest.
Publisher’s note
All claims expressed in this article are solely those of the authors and do not necessarily represent those of their affiliated organizations, or those of the publisher, the editors and the reviewers. Any product that may be evaluated in this article, or claim that may be made by its manufacturer, is not guaranteed or endorsed by the publisher.
Supplementary material
The Supplementary Material for this article can be found online at: https://www.frontiersin.org/articles/10.3389/fmed.2022.928637/full#supplementary-material
Supplementary Figure 1 | Validation of the hub gene by ROC analysis. (A) was ROC analysis of COVID-19 cohort; (B) was ROC analysis of asthma cohort.
Supplementary Table 1 | Ontological analysis of common differentially expressed genes (DEGs) among COVID-19 and asthma.
Supplementary Table 2 | Gene ontology (GO) pathway.
Supplementary Table 3 | Kyoto encyclopedia of genes and genomes (KEGG) pathway.
Supplementary Table 4 | Transcription factor (TF)-Gene topology table.
Supplementary Table 5 | MiRNA-gene topology table.
Footnotes
- ^ https://string-db.org/
- ^ http://amp.pharm.mssm.edu/Enrichr/
- ^ http://jaspar.genereg.net
- ^ https://www.disgenet.org/
- ^ http://jvenn.toulouse.inra.fr/app/example.html
References
1. Liu K, Pan X, Li L, Yu F, Zheng A, Du P, et al. Binding and molecular basis of the bat coronavirus RaTG13 virus to ACE2 in humans and other species. Cell. (2021) 184:3438–51. doi: 10.1016/j.cell.2021.05.031
2. Bakouny Z, Hawley JE, Choueiri TK, Peters S, Rini BI, Warner JL, et al. COVID-19 and cancer: current challenges and perspectives. Cancer Cell. (2020) 38:629–46. doi: 10.1016/j.ccell.2020.09.018
3. Case JB, Chen RE, Cao L, Ying B, Winkler ES, Johnson M, et al. Ultrapotent miniproteins targeting the SARS-CoV-2 receptor-binding domain protect against infection and disease. Cell Host Microbe. (2021) 29:1151–61. doi: 10.1016/j.chom.2021.06.008
4. Dhand R, Li J. Coughs and sneezes: their role in transmission of respiratory viral infections, including SARS-CoV-2. Am J Respir Crit Care Med. (2020) 202:651–9. doi: 10.1164/rccm.202004-1263PP
5. Vigetti D, Clerici M, Deleonibus S, Karousou E, Viola M, Moretto P, et al. Hyaluronan synthesis is inhibited by adenosine monophosphate-activated protein kinase through the regulation of HAS2 activity in human aortic smooth muscle cells. J Biol Chem. (2011) 286:7917–24. doi: 10.1074/jbc.M110.193656
6. Haktanir Abul M, Phipatanakul W. Severe asthma in children: evaluation and management. Allergol Int. (2019) 68:150–7. doi: 10.1016/j.alit.2018.11.007
7. Ramratnam SK, Bacharier LB, Guilbert TW. Severe asthma in children. J Allergy Clin Immunol. (2017) 5:889–98. doi: 10.1016/j.jaip.2017.04.031
8. Alwarith J, Kahleova H, Crosby L, Brooks A, Brandon L, Levin SM, et al. The role of nutrition in asthma prevention and treatment. Nutrit Rev. (2020) 78:928–38. doi: 10.1093/nutrit/nuaa005
9. Karlsson Sundbaum J, Konradsen JR, Vanfleteren LEGW, Axelsson Fisk S, Pedroletti C, Sjöö Y, et al. Uncontrolled asthma predicts severe COVID-19: a report from the Swedish National Airway Register. Therapeut Adv Respir Dis. (2022) 16:1621296785. doi: 10.1177/17534666221091183
10. Alabdulkarim N, Gai J, Bost J, Pillai D, Teach SJ, Rastogi D. Effect of the COVID-19 pandemic on morbidity among children hospitalized for an asthma exacerbation. Ann Allergy Asthma Immunol. (2022) [Online ahead of print]. doi: 10.1016/j.anai.2022.03.033
11. Barrett T, Wilhite SE, Ledoux P, Evangelista C, Kim IF, Tomashevsky M, et al. NCBI GEO: archive for functional genomics data sets–update. Nucleic Acids Res. (2013) 41:D991–5. doi: 10.1093/nar/gks1193
12. Blanco-Melo D, Nilsson-Payant BE, Liu W, Uhl S, Hoagland D, Møller R, et al. Imbalanced host response to SARS-CoV-2 drives development of COVID-19. Cell. (2020) 181:1036–45. doi: 10.1016/j.cell.2020.04.026
13. Fujimori S, Hirai N, Masuoka K, Oshikubo T, Yamashita T, Washio T, et al. IRView: a database and viewer for protein interacting regions. Bioinformatics. (2012) 28:1949–50. doi: 10.1093/bioinformatics/bts289
14. Li J, Liu Q, Huang X, Cai Y, Song L, Xie Q, et al. Transcriptional profiling reveals the regulatory role of CXCL8 in promoting colorectal cancer. Front Genet. (2019) 10:1360. doi: 10.3389/fgene.2019.01360
15. Chen S, Gao C, Yu T, Qu Y, Xiao GG, Huang Z. Bioinformatics analysis of a prognostic miRNA signature and potential key genes in pancreatic cancer. Front Oncol. (2021) 11:641289. doi: 10.3389/fonc.2021.641289
16. Collins K, Zhao K, Jiao C, Xu C, Cai X, Wang X, et al. SpinachBase: a central portal for spinach genomics. Database. (2019) 2019:z72. doi: 10.1093/database/baz072
17. Subramanian A, Tamayo P, Mootha VK, Mukherjee S, Ebert BL, Gillette MA, et al. Gene set enrichment analysis: a knowledge-based approach for interpreting genome-wide expression profiles. Proc Natl Acad Sci USA. (2005) 102:15545–50.
18. Pujar MK, Vastrad B, Vastrad C. Integrative analyses of genes associated with subcutaneous insulin resistance. Biomolecules. (2019) 9:37. doi: 10.3390/biom9020037
19. Vlieghe D, Sandelin A, De Bleser PJ, Vleminckx K, Wasserman WW, van Roy F, et al. A new generation of JASPAR, the open-access repository for transcription factor binding site profiles. Nucleic Acids Res. (2006) 34:D95–7. doi: 10.1093/nar/gkj115
20. Castro-Mondragon JA, Riudavets-Puig R, Rauluseviciute I, Berhanu Lemma R, Turchi L, Blanc-Mathieu R, et al. JASPAR 2022: the 9th release of the open-access database of transcription factor binding profiles. Nucleic Acids Res. (2021) 50:D165–73. doi: 10.1093/nar/gkab1113
21. Song G, Xu J, He L, Sun X, Xiong R, Luo Y, et al. Systematic profiling identifies PDLIM2 as a novel prognostic predictor for oesophageal squamous cell carcinoma (ESCC). J Cell Mol Med. (2019) 23:5751–61. doi: 10.1111/jcmm.14491
22. Auwul MR, Zhang C, Rahman MR, Shahjaman M, Alyami SA, Moni MA. Network-based transcriptomic analysis identifies the genetic effect of COVID-19 to chronic kidney disease patients: a bioinformatics approach. Saudi J Biol Sci. (2021) 28:5647–56. doi: 10.1016/j.sjbs.2021.06.015
23. Sethupathy P, Corda B, Hatzigeorgiou AG. TarBase: a comprehensive database of experimentally supported animal microRNA targets. RNA. (2006) 12:192–7.
24. Hsu S, Lin F, Wu W, Liang C, Huang W, Chan W, et al. miRTarBase: a database curates experimentally validated microRNA-target interactions. Nucleic Acids Res. (2011) 39:D163–9. doi: 10.1093/nar/gkq1107
25. Chen EY, Tan CM, Kou Y, Duan Q, Wang Z, Meirelles GV, et al. Enrichr: interactive and collaborative HTML5 gene list enrichment analysis tool. BMC Bioinformat. (2013) 14:128. doi: 10.1186/1471-2105-14-128
26. Federer C, Yoo M, Tan AC. Big data mining and adverse event pattern analysis in clinical drug trials. Assay Drug Dev Technol. (2016) 14:557–66. doi: 10.1089/adt.2016.742
27. Salnikova LE, Chernyshova EV, Anastasevich LA, Larin SS. Gene- and disease-based expansion of the knowledge on inborn errors of immunity. Front Immunol. (2019) 10:2475. doi: 10.3389/fimmu.2019.02475
28. Mahmud SMH, Al-Mustanjid M, Akter F, Rahman MS, Ahmed K, Rahman MH, et al. Bioinformatics and system biology approach to identify the influences of SARS-CoV-2 infections to idiopathic pulmonary fibrosis and chronic obstructive pulmonary disease patients. Briefings Bioinformat. (2021) 22:b115. doi: 10.1093/bib/bbab115
29. Zagorac I, Fernandez-Gaitero S, Penning R, Post H, Bueno MJ, Mouron S, et al. In vivo phosphoproteomics reveals kinase activity profiles that predict treatment outcome in triple-negative breast cancer. Nat Commun. (2018) 9:3501. doi: 10.1038/s41467-018-05742-z
30. Consortium TGO. Expansion of the gene ontology knowledgebase and resources. Nucleic Acids Res. (2017) 45:D331–8. doi: 10.1093/nar/gkw1108
31. Bo X, Wu M, Xiao H, Wang H. Transcriptome analyses reveal molecular mechanisms that regulate endochondral ossification in amphibian Bufo gargarizans during metamorphosis. Biochim Biophys Acta Gene Subj. (2018) 1862:2632–44. doi: 10.1016/j.bbagen.2018.07.032
32. Jiang W, Mitra R, Lin C, Wang Q, Cheng F, Zhao Z. Systematic dissection of dysregulated transcription factor-miRNA feed-forward loops across tumor types. Briefings Bioinformatics. (2016) 17:996–1008. doi: 10.1093/bib/bbv107
33. Zhao S, Chen H, Ding B, Li J, Lv F, Han K, et al. Construction of a transcription factor-long non-coding RNA-microRNA network for the identification of key regulators in lung adenocarcinoma and lung squamous cell carcinoma. Mol Med Rep. (2019) 19:1101–9. doi: 10.3892/mmr.2018.9769
34. Lage K, Karlberg EO, Størling ZM, Olason PI, Pedersen AG, Rigina O, et al. A human phenome-interactome network of protein complexes implicated in genetic disorders. Nat Biotechnol. (2007) 25:309–16. doi: 10.1038/nbt1295
35. Barabási A, Gulbahce N, Loscalzo J. Network medicine: a network-based approach to human disease. Nat Rev Genet. (2011) 12:56–68. doi: 10.1038/nrg2918
36. Francescangeli F, De Angelis ML, Zeuner A. COVID-19: a potential driver of immune-mediated breast cancer recurrence? Breast Cancer Res BCR. (2020) 22:117. doi: 10.1186/s13058-020-01360-0
37. Zhu Z, Hasegawa K, Ma B, Fujiogi M, Camargo CAJ, Liang L. Association of asthma and its genetic predisposition with the risk of severe COVID-19. J Allergy Clin Immunol. (2020) 146:327–9. doi: 10.1016/j.jaci.2020.06.001
38. Kasehagen L, Byers P, Taylor K, Kittle T, Roberts C, Collier C, et al. COVID-19-associated deaths after SARS-CoV-2 infection during pregnancy - Mississippi, March 1, 2020-October 6, 2021. MMWR. (2021) 70:1646–8. doi: 10.15585/mmwr.mm7047e2
39. Zhou F, Yu T, Du R, Fan G, Liu Y, Liu Z, et al. Clinical course and risk factors for mortality of adult inpatients with COVID-19 in Wuhan, China: a retrospective cohort study. Lancet. (2020) 395:1054–62. doi: 10.1016/S0140-6736(20)30566-3
40. Ziegler CGK, Allon SJ, Nyquist SK, Mbano IM, Miao VN, Tzouanas CN, et al. SARS-CoV-2 Receptor ACE2 Is an interferon-stimulated gene in human airway epithelial cells and is detected in specific cell subsets across tissues. Cell. (2020) 181:1016–35. doi: 10.1016/j.cell.2020.04.035
41. Ma-Lauer Y, Carbajo-Lozoya J, Hein MY, Müller MA, Deng W, Lei J, et al. p53 down-regulates SARS coronavirus replication and is targeted by the SARS-unique domain and PLpro via E3 ubiquitin ligase RCHY1. Proc Natl Acad Sci USA. (2016) 113:E5192–201. doi: 10.1073/pnas.1603435113
42. Bowler E, Porazinski S, Uzor S, Thibault P, Durand M, Lapointe E, et al. Hypoxia leads to significant changes in alternative splicing and elevated expression of CLK splice factor kinases in PC3 prostate cancer cells. BMC Cancer. (2018) 18:355. doi: 10.1186/s12885-018-4227-7
43. Khazeei Tabari MA, Iranpanah A, Bahramsoltani R, Rahimi R. Flavonoids as promising antiviral agents against SARS-CoV-2 infection: a mechanistic review. Molecules. (2021) 26:3900. doi: 10.3390/molecules26133900
44. Biswas D, Dutta-Biswas R, Stillman DJ. Chd1 and yFACT act in opposition in regulating transcription. Mol Cell Biol. (2007) 27:6279–87. doi: 10.1128/MCB.00978-07
45. Burkhardt L, Fuchs S, Krohn A, Masser S, Mader M, Kluth M, et al. CHD1 is a 5q21 tumor suppressor required for ERG rearrangement in prostate cancer. Cancer Res. (2013) 73:2795–805. doi: 10.1158/0008-5472.CAN-12-1342
46. Lin X, Wang J, Yun L, Jiang S, Li L, Chen X, et al. Association between LEKR1-CCNL1 and IGSF21-KLHDC7A gene polymorphisms and diabetic retinopathy of type 2 diabetes mellitus in the Chinese Han population. J Gene Med. (2016) 18:282–7. doi: 10.1002/jgm.2926
47. Umehara T, Kagawa S, Tomida A, Murase T, Abe Y, Shingu K, et al. Body temperature-dependent microRNA expression analysis in rats: rno-miR-374-5p regulates apoptosis in skeletal muscle cells via Mex3B under hypothermia. Sci Rep. (2020) 10:15432. doi: 10.1038/s41598-020-71931-w
48. Garcia-Etxebarria K, Jauregi-Miguel A, Romero-Garmendia I, Plaza-Izurieta L, Legarda M, Irastorza I, et al. Ancestry-based stratified analysis of Immunochip data identifies novel associations with celiac disease. Eur J Hum Genet EJHG. (2016) 24:1831–4. doi: 10.1038/ejhg.2016.120
49. Kay M, Soltani BM, Aghdaei FH, Ansari H, Baharvand H. Hsa-miR-335 regulates cardiac mesoderm and progenitor cell differentiation. Stem Cell Res Therapy. (2019) 10:191. doi: 10.1186/s13287-019-1249-2
50. Taz TA, Ahmed K, Paul BK, Kawsar M, Aktar N, Mahmud SMH, et al. Network-based identification genetic effect of SARS-CoV-2 infections to Idiopathic pulmonary fibrosis (IPF) patients. Briefings Bioinformat. (2021) 22:1254–66. doi: 10.1093/bib/bbaa235
51. Fang Q, Zhou C, Nandakumar KS. Molecular and cellular pathways contributing to joint damage in rheumatoid arthritis. Mediat Inflamm. (2020) 2020:3830212. doi: 10.1155/2020/3830212
52. Apio C, Kamruzzaman M, Park T. Confidence intervals for the COVID-19 neutralizing antibody retention rate in the Korean population. Genomics Informat. (2020) 18:e31. doi: 10.5808/GI.2020.18.3.e31
53. Cho J, Lee YJ, Kim JH, Kim SI, Kim SS, Choi B, et al. Antiviral activity of digoxin and ouabain against SARS-CoV-2 infection and its implication for COVID-19. Sci Rep. (2020) 10:16200. doi: 10.1038/s41598-020-72879-7
54. Zhou W, Yang Y, Mei C, Dong P, Mu S, Wu H, et al. Inhibition of Rho-kinase downregulates Th17 cells and ameliorates hepatic fibrosis by schistosoma japonicum infection. Cells. (2019) 8:1262. doi: 10.3390/cells8101262
55. Kalathiya U, Padariya M, Mayordomo M, Lisowska M, Nicholson J, Singh A, et al. Highly conserved homotrimer cavity formed by the SARS-CoV-2 spike glycoprotein: a novel binding site. J Clin Med. (2020) 9:1473. doi: 10.3390/jcm9051473
56. Aanouz I, Belhassan A, El-Khatabi K, Lakhlifi T, El-Ldrissi M, Bouachrine M. Moroccan medicinal plants as inhibitors against SARS-CoV-2 main protease: computational investigations. J Biomol Struct Dynam. (2021) 39:2971–9. doi: 10.1080/07391102.2020.1758790
57. Oyama R, Kito F, Takahashi M, Sakumoto M, Shiozawa K, Qiao Z, et al. Establishment and characterization of a novel dedifferentiated chondrosarcoma cell line, NCC-dCS1-C1. Hum Cell. (2019) 32:202–13. doi: 10.1007/s13577-018-00232-2
Keywords: SARS-CoV-2, asthma, COVID-19, differentially expressed genes, hub genes, drug molecules
Citation: Li X-y, Wang J-b, An H-b, Wen M-z, You J-x and Yang X-t (2022) Effect of SARS-CoV-2 infection on asthma patients. Front. Med. 9:928637. doi: 10.3389/fmed.2022.928637
Received: 13 May 2022; Accepted: 04 July 2022;
Published: 02 August 2022.
Edited by:
Kawsar Ahmed, Mawlana Bhashani Science and Technology University, BangladeshReviewed by:
Md Mamun Ali, Daffodil International University, BangladeshTasnimul Alam Taz, Wayne State University, United States
Danial Kahrizi, Razi University, Iran
Copyright © 2022 Li, Wang, An, Wen, You and Yang. This is an open-access article distributed under the terms of the Creative Commons Attribution License (CC BY). The use, distribution or reproduction in other forums is permitted, provided the original author(s) and the copyright owner(s) are credited and that the original publication in this journal is cited, in accordance with accepted academic practice. No use, distribution or reproduction is permitted which does not comply with these terms.
*Correspondence: Xi-tao Yang, xitao123456@126.com
†These authors have contributed equally to this work