- 1First Teaching Hospital of Tianjin University of Traditional Chinese Medicine, Tianjin, China
- 2National Clinical Research Center for Chinese Medicine Acupuncture and Moxibustion, Tianjin, China
Background: Stroke-associated pneumonia (SAP) is one of the major causes of death after suffering a stroke. Several scoring systems have been developed for the early prediction of SAP. However, it is unclear which scoring system is more suitable as a risk prediction tool. We performed this Bayesian network meta-analysis to compare the prediction accuracy of these scoring systems.
Methods: Seven databases were searched from their inception up to April 8, 2022. The risk of bias assessment of included study was evaluated by the QUADAS-C tool. Then, a Bayesian network meta-analysis (NMA) was performed by R 4.1.3 and STATA 17.0 software. The surface under the cumulative ranking curve (SUCRA) probability values were applied to rank the examined scoring systems.
Results: A total of 20 cohort studies involving 42,236 participants were included in this analysis. The results of the NMA showed that AIS-APS had excellent performance in prediction accuracy for SAP than Chumbler (MD = 0.030, 95%CI: 0.004, 0.054), A2DS2 (MD = 0.041, 95% CI: 0.023, 0.059), ISAN (MD = 0.045, 95% CI: 0.022, 0.069), Kwon (MD = 0.077, 95% CI: 0.055, 0.099) and PANTHERIS (MD = 0.082, 95% CI: 0.049, 0.114). Based on SUCRA values, AIS-APS (SUCRA: 99.8%) ranked the highest.
Conclusion: In conclusion, the study found that the AIS-APS is a validated clinical tool for predicting SAP after the onset of acute ischemic stroke.
Systematic review registration: https://www.crd.york.ac.uk/PROSPERO/display_record.php?RecordID=292375, identifier: CRD42021292375.
Introduction
Stroke-associated pneumonia (SAP) which was introduced for the first time by Hilker et al. (1) in 2003 is one of the major causes of death after suffering a stroke. The morbidity of SAP varies from 8.6% (2) to 21.4% (3). It significantly increased the mortality, length of hospitalization, and economic burden among these patients (4–7). Therefore, early identification of SAP high-risk groups and timely therapy are crucial.
As various approaches (8, 9) were well-recognized in research and clinical practice which may lead to delayed or inappropriate antibiotic therapy, Pneumonia in Stroke Consensus [PISCES] Group defined SAP as the spectrum of lower respiratory tract infections within the first 7 days after stroke onset in 2015 (10). Currently, the modified criteria of the Centers for Disease Control and Prevention (mCDC criteria) (11) was widely used for the diagnosis of SAP (12, 13). Meanwhile, various risk factors for SAP have been reported in recent years, which include mechanical ventilation, atrial fibrillation, pre-existing respiratory disease, smoking, pre-existing heart disease, stroke severity, stroke-induced immunodepression and dysphasia etc. (14, 15). Combined with the SAP risk factors, several scoring systems such as A2DS2 (Age, Atrial fibrillation, Dysphagia, Sex, Stroke Severity) in Germany (16), ISAN (Prestroke Independence, Sex, Age, National Institutes of Health Stroke Scale) in UK (4), AIS-APS (Acute Ischaemic Stroke-Associated Pneumonia Score) in China (17), Kwon (Pneumonia Score) in Korea (18), PANTHERIS (Preventive Antibacterial Therapy in Acute Ischaemic Stroke) in Germany (19), Chumbler (Veteran's Health Administration cohort score) in USA (20) have been developed for the early prediction. On the one hand, some original research papers have compared several of these scoring systems but got contradictory results. The ranking of these scoring systems varies. On the other hand, the head-to-head clinical trials comparing the accuracy of these scoring systems are lacking up to now. Only a few original research papers (21) compared all scoring systems. Two systematic reviews (22, 23) determined the predictive performance of A2DS2, ISAN and AIS-APS, but do not compare the predictive accuracy among these scoring systems. It is still unclear which scoring system is more suitable as a risk prediction tool for SAP.
The network meta-analysis allows a quantitative comparison of multiple interventions to select the best option. Therefore, we compared the six scoring systems for rational predicting the risk of SAP based on the Bayesian network meta-analysis method.
Materials and methods
Study registration
This study was prepared under the guidance of the Preferred Reporting Items for Systematic Review and Meta-Analysis (PRISMA) guidelines. The study was prospectively registered on the PROSPERO platform (https://www.crd.york.ac.uk/prospero/) with an assigned registration number CRD42021292375.
Inclusion and exclusion criteria
Inclusion criteria
1) Retrospective or prospective cohort studies.
2) Age ≥15 years, ischemic stroke patient.
3) The reference standards for SAP diagnosis according to the clinical, laboratory, and radiological examinations according to the modified criteria of the Centers for Disease Control and Prevention.
4) All studies compared the prediction accuracy of two or more selected scoring systems (A2DS2, ISAN, AIS-APS, Kwon, PANTHERIS, and Chumbler) for patients with SAP.
Exclusion criteria
1) Transient ischemic attack (TIA) Patients.
2) Studies included special populations (oncology patients, pregnant women, patients using immunosuppressive drugs, liver cirrhosis, etc.).
3) Studies with incomplete research data, unable to extract valid data.
4) Studies with duplicate publications or duplicate data.
Search strategy
A systematic and comprehensive search was performed using electronic databases of PubMed (MEDLINE), Embase, Web of Science, China National Knowledge Infrastructure (CNKI), China Science and Technology Journal Database (VIP), China Biology Medicine disc (CBM) and Wanfang data from their inception up to April 8th, 2022. In addition, relevant Meta-analysis and systematic review were manually retrieved to track references of included literatures. As for studies with incomplete data, we would contact the authors of the studies. If valid data was still not available, the study would be excluded.
Literature selection and data extraction
Two investigators (XM Zhang and L Xiao) independently screened the papers by checking the titles, abstracts, and keywords. Then, full texts were read to select studies meeting eligibility criteria. Any inconsistencies during the entire study selection were resolved by thorough discussion or the third investigator (YC Tian). The information including eligible study characteristics (e.g., first author and year of publication), participant characteristics (e.g., gender, age, and sample), details of interventions (e.g., scoring systems), outcome data, and factors to evaluate risk of bias were extracted and entered into the spreadsheet.
Risk of bias assessment
The risk of bias was evaluated using the QUADAS-C (24) risk of bias assessment tool (http://www.bristol.ac.uk/population-health-sciences/projects/quadas/quadas-c). QUADAS-C (C stands for comparative) is an extension to QUADAS-2 for assessing risk of bias in comparative accuracy studies. The QUADAS-C tool retains the same 4-domain structure of QUADAS-2 (Patient Selection, Index Test, Reference Standard, and Flow and Timing) and comprises additional questions to each QUADAS-2 domain. A risk-of-bias judgment for comparative accuracy requires a risk-of-bias judgment for the accuracy of each test (resulting from QUADAS-2) and additional criteria specific to test comparisons. Two investigators (XM Zhang and L Xiao) independently evaluated the risk of bias of the included studies and cross-checked the results.
Statistical analysis
R software version 4.1.3 and STATA software were employed to compute calculations and prepare graphs. The gemtc packages and rjags packages were utilized to compute a Markov chain Monte Carlo (MCMC) simulation. Odds ratios (OR) with 95% confidence intervals (CIs) were used for dichotomous data. For continuous variables, mean differences (MD) with 95% CIs. Initially, both fixed effects and random effects models were fitted. Preset model parameters: four chains were used for simulation analysis, 20,000 annealing times, a step size of one, and 50,000 simulation iterations. The deviance information criterion (DIC) was used to judge the degree of model fit (25). A lower DIC score meant a better fit. After the subsequent analysis was performed. Consistency in the entire network was evaluated by calculating the unrelated mean effects (UME) model (26, 27). Consistency between direct and indirect comparison was analyzed by the node-splitting method (28), and P < 0.05 indicated a significant inconsistency for a specific comparison. The I2 statistic was used to assess the heterogeneity between studies, with a cut-off point of 50%. The network graph constructed by STATA software represented a comparative relationship between different interventions. The surface under the cumulative ranking curves (SUCRA) were calculated to present the ranking probability of different scoring systems. The range of SUCRA was from 0 to 100% (29, 30). After that, publication bias were reflected by funnel plots (31, 32).
Results
A total of 2,040 studies were identified from the search at first. After removing duplicates, 1,395 remained. By screening titles and abstracts, 1,308 studies were excluded because they were reviews, irrelevant studies, and animal experiments. Afterwards, 87 relevant studies were reviewed for eligibility by full-text evaluations. Finally, 20 studies that met the inclusion criteria were included in our Bayesian NMA. 67 records were excluded for the following reasons: (1) intracerebral hemorrhage patients (n = 4); (2) The study examined only one of the selected scoring systems (n = 45); (3) incomplete data (n = 18). The literature selection process was illustrated in Figure 1.
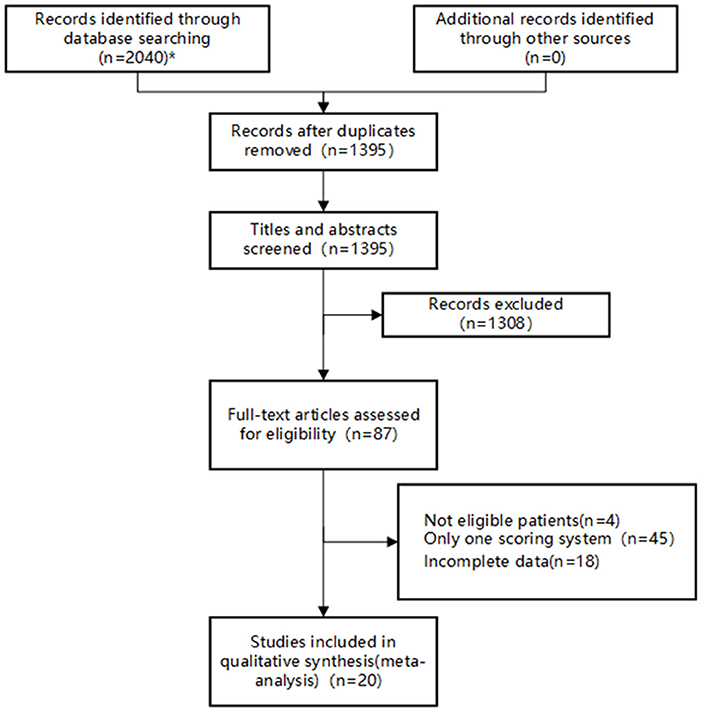
Figure 1. Flow diagram of study inclusion. *The databases searched and the number of studies retrieved are as follows: PubMed (n = 826), Embase (n = 48), Web of Science (n = 769), CNKI (n = 40), Wan Fang Data (n =70), VIP (n = 254), and SinoMed (n = 33).
Study characteristics
The Bayesian NMA was performed using 20 cohort studies with a total of 42,236 patients and their sample sizes varying from 40 to 14,400 participants. Twenty studies were conducted in China (13) (17, 21, 33–43), Singapore (1) (44), Spain (1) (45), Egypt (1) (13), Indonesia (1) (46), the United Arab Emirates (1) (47), the United Kingdom (1) (48), and France (1) (2) and published between 2013 and 2021. The study by Ji et al. (17) contained three validation cohorts, which were split to represent. The details of the study characteristics were depicted in Table 1. The network diagram is presented in Figure 2. Lines width is proportional to the number of references including the comparison. Dots area is proportional to the number of patients in the scoring systems.
Quality assessments of studies
We used the QUADAS-C tools to conduct the quality evaluation. All of the studies were in full paired design (each patient receiving all of the index tests in the studies). Six studies (33, 34, 36–39) were assessed as “unclear risk” in terms of patient selection due to unreported whether the selection of patients was consecutive or not. Six studies (2, 21, 33, 36, 37, 39) were assessed as “high risk” in terms of index test due to unable to determine whether to interpret the index tests results without knowledge of the results of the reference standard. Further details of the risk of bias assessment are shown in Figure 3.
Model selection
The preliminary model fit showed fixed effects model DIC= 165.8, I2 = 50% and random effects model DIC = 129.5, I2 = 12%, so a random effects model was used for the network meta-analysis. The convergence diagnosis graph and trajectory and density graph of the random effects model were shown in Figures 4, 5.
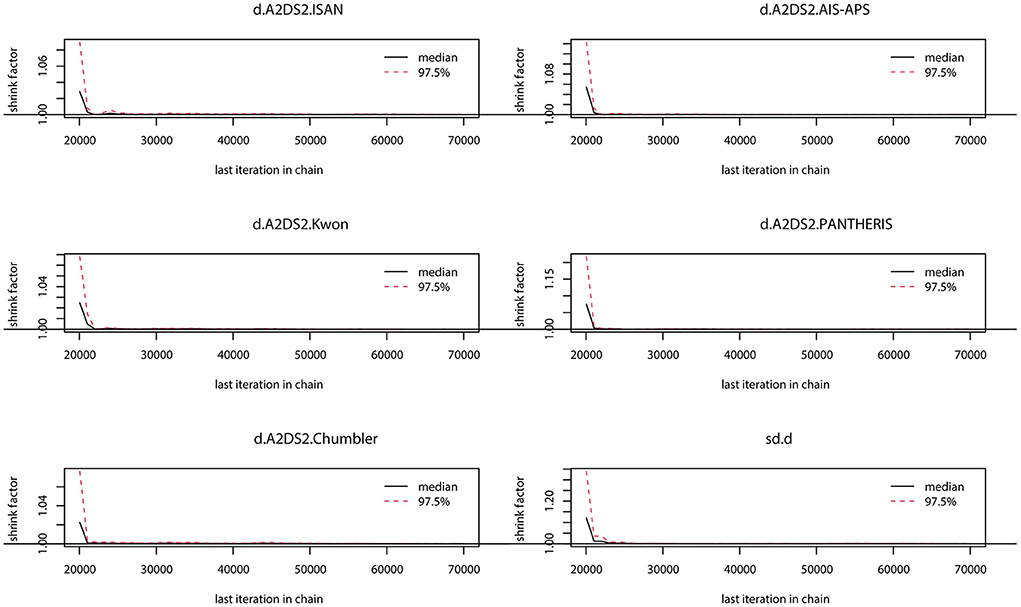
Figure 4. Convergence diagnosis graph. The value of the potential scale reduction factor (PSRF) tends to 1 indicating a satisfactory degree of model convergence.
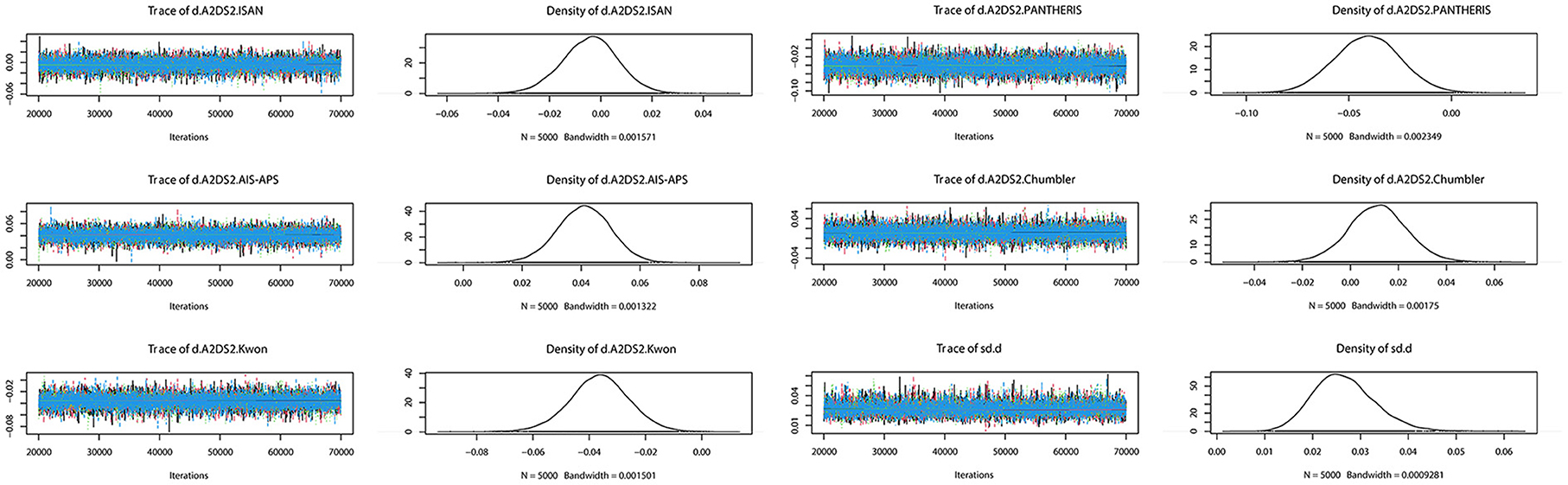
Figure 5. Trajectory and density graph. Each MCMC chain in the trajectory diagram has reached stable fusion from the beginning part, and the overlapping area accounts for most of the chain fluctuation range in the subsequent calculation, and the fluctuation of a single chain cannot be recognized by the naked eye, so the model convergence degree is satisfactory. The distribution of the graphs in the density map is normal, and the Bandwidth value tends to 0 and reaches stability, and the model convergence degree is satisfactory when combined with the results of the trajectory map.
Inconsistency test
The consistency in the entire network was evaluated by calculating the UME model, the results showed DIC= 129.5. The local inconsistency test used the node-splitting method, and all the results showed P > 0.05 (Table 2). Overall, no inconsistency was found. The results of NMA are reliable.
Network meta-analysis results
The network meta-analysis results were shown in Figure 6. AIS-APS had excellent performance in prediction accuracy for SAP than Chumbler (MD = 0.030, 95%CI: 0.004, 0.054), A2DS2 (MD = 0.041, 95% CI: 0.023, 0.059), ISAN (MD = 0.045, 95% CI: 0.022, 0.069), Kwon (MD = 0.077, 95% CI: 0.055, 0.099) and PANTHERIS (MD = 0.082, 95% CI: 0.049, 0.114).
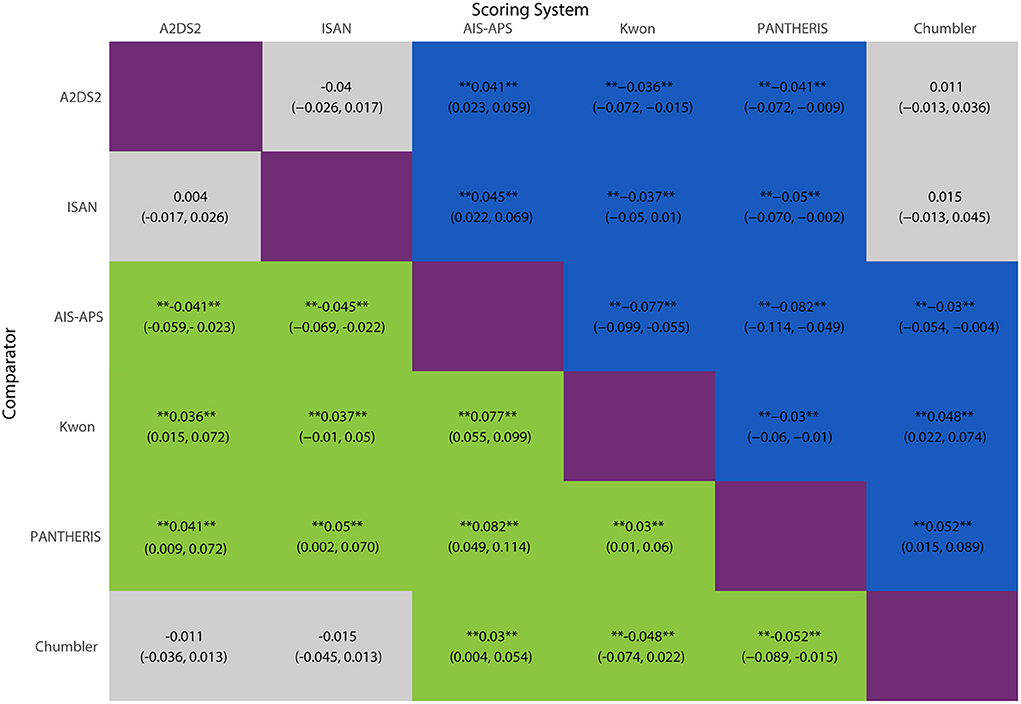
Figure 6. MD with 95% CIs of AUC of six risk scores for SAP. The values in each cell represent the relative effect of the risk score on the top, compared to the risk score on the left. **Indicates statistical significance.
However, the results showed no significant differences between Chumbler, A2DS2 and ISAN (Chumbler: MD = 0.015, 95%CI: −0.013, 0.045, A2DS2: MD = 0.004, 95% CI: −0.017, 0.026, compared with ISAN), no significant differences between Kwon and PANTHERIS (Kwon: MD = 0.005, 95% CI: −0.031, 0.039, compared with PANTHERIS).
Prediction accuracy ranking based on SUCRA values, were as follows: AIS-APS, Chumbler, A2DS2, ISAN, KWON, PANTHERIS. The details are depicted in Figure 7.
Funnel plot characteristics
The funnel plot result was displayed in Figure 8. There were roughly symmetrical in visual, but some points lay outside the funnel, which revealed there was a small sample size and publication bias.
Discussion
As the increasing morbidity and mortality of SAP, it is necessary to predict the SAP risk at the early stage. However, which scoring system of prediction is better has long been matter of debate. To the best of our knowledge, this is the first and the most comprehensive Bayesian NMA evaluating the scores for predicting SAP. The most important finding of our study is that the AIS-APS score showed better value in predicting the risk of SAP.
The AIS-APS score was derived by Ji Ruijun et al. in 2013, based on the China National Stroke Registry (CNSR), a nationwide, multicenter, and prospective registry of consecutive patients with acute cerebrovascular events. The following items were selected: age, past medical history, current smoking, modified Rankin Scale (mRS), Glasgow Coma Scale (GCS), National Institutes of Health Stroke Scale (NIHSS), Oxfordshire Community Stroke Project (OCSP), dysphagia, and admission glucose, with 5 risk stratifications [very low (0–6), low (7–13), intermediate (14–20), high (21–27), and very high (28–35)]. In the previous cohort (17), the AIS-APS had the highest associated sensitivity, specificity, positive predictive value and negative predictive value in the internal and external validation. The results of our study corroborate growing recent evidence that suggest the AIS-APS score had better predictive accuracies for SAP in comparative diagnostic accuracy studies. Zhang et al. (43) found that the AIS-APS [AUC: 0.79 (95% CI: 0.78–0.80)] performed superior to the A2DS2 score [AUC: 0.74 (95% CI: 0.73–0.75)] and the ISAN score [AUC: 0.76 (95% CI: 0.75–0.78)] to in predicting in-hospital pneumonia after AIS. Siregar et al. (46) found the AIS-APS [AUC: 0.792 (95% CI: 0.761–0.823)] performed a higher AUC value than the A2DS2 score for the Indonesia patients. The reliability and structural validity of the AIS-APS score were validated in the study of Qinxia et al. (49). The result of internal consistency (Cronbach's α) was 0.831. The results of the structural validity test were consistent with the ideal structure of the score. The Chinese consensus statement suggests using AIS-APS as a prediction tool for SAP. With several risk stratification, most reliable and accurate scoring system, AIS-APS would be greatly beneficial to patients and clinicians. However, there were little external validations of AIS-APS for non-Asian populations. The AIS-APS should be externally validated in geographically distinct population.
Chumbler et al. developed a 3-category scoring system (Chumbler score) to better identify patients at high risk of SAP, from a secondary analysis of a retrospective cohort study based on the Veterans Health Administration (VHA), which included the medical history of pneumonia, dysphagia, increasing NIHSS score, being found down at symptom onset, and age >70 years. The main difference among Chumbler's, AIS-APS and A2DS2 is the weighted value of NIHSS score, which are 14/19, 5/9 and 8/35, respectively. A meta-analysis had shown that patients with an NIHSS score >15 points had a 14.63-fold increased risk for pulmonary infection compared with patients whose NIHSS scores were <15 points (50). Besides, Chumbler's score has a unique valuable “being found down at symptomonset.” Falling down after stroke may lead to bone fracture and then resting in bed for long time which would increase the risk of SAP. However, the Chumbler score was from a secondary analysis of a retrospective cohort study, the score needs more validations.
The A2DS2 score was derived by Hoffmann et al in 2012. The score was performed in a large derivation and validation sample based on the Berlin Stroke Register (BSR) data. It did not include meaningful risk categories. The risk score showed good discrimination properties. Although the A2DS2 score had been externally validated in Asian and non-Asian populations, it was not always performing better than other scores. Ye et al. (34) found that A2DS2 (AUC: 0.776 (95% CI: 0.694–0.859)] performed inferior to AIS-APS (AUC: 0.829 (95% CI: 0.769–0.889)] and PANYHERIS [AUC: 0.818 (95% CI:0.750–0.885)]. Helmy et al. (13) reported that the A2DS2 score showed the highest AUC [0.85 (95% CI: 0.74–0.92)] compared with AIS-APS [0.798 (95% CI: 0.685–0.884)] and PANTHERIS [0.715 (95% CI: 0.595–0.817)] in Egypt populations. The A2DS2 score should be externally validated.
To simplify the scoring system, Harms et al. developed the PANTHERIS score based on the Berlin Neurological Intensive Care Unit. The leukocyte count was selected as one of the predictors. However, it was limited by the small sample size and all patients with middle cerebral artery infarction. This score did not include medical history and stroke patterns. The PANTERIS score was originally developed for patients with severe strokes admitted to neurocritical care units. Current published studies almost were designed for emergency department or neurology department patients. Further comparative diagnostic accuracy studies for different stroke severity patients are required.
Overall, all scores were derived from a retrospective analysis of registry-based studies. Two scores (AIS-APS and PANTHERIS) required laboratory variables. Two scores (Kwon score and PANTHERIS) were derived from relatively small single-center studies limiting their applicability. Two scores (AIS-APS and ISAN) selected pre-stroke neurological status as one of the predictors. Several scores (Chumbler, AIS-APS and ISAN) provided risk stratification. All of the scores did not include meaningful predictions of outcomes. The details of the six scoring systems were shown in Table 3. Most of the scores can be directly performed after admission or soon after admission. The PANTHERIS score needs more time to be evaluated because of the inclusion of systolic blood pressure within 24 h after admission. Dysphagia almost was preliminary evaluated soon after admission with water swallow test. If patients already suffered SAP according to the diagnosis criteria of guideline, risk scores could help us to prove the effect of these scores. The addition of items such as WBC and systolic blood pressure within 24 h after admission could improve the predictive performance, but have a chance of delay for predict SAP. However, beside PANTHERIS score, other scores could be evaluated at similar time after admission. The balance between better accuracy, additive items and time warrants further consideration.
Initially, we wanted to analyze the sensitivity and specificity of each score. However, most of the studies did not provide 95%CIs and the standard deviation of sensitivity and specificity. Meanwhile, true positive rate, false positive rate, false negative rate, and true negative rate were also missing in several studies. After trying to contact with authors, we still did not have enough data available for the analysis. And the network meta-analysis methodology of diagnostic accuracy studies was still inadequate. We finally gave up on the analysis.
Preventive antibiotics had not shown any effect neither in reducing the incidence of stroke-associated pneumonia nor decreasing the mortality or improving the proportion of good outcomes within this field for the last decade. A systematic review (51) made an analysis of preventive antibiotics in patients with different risk score (A2DS2 or ISAN) and found risk scores did not significantly influence treatment response of preventive antibiotic therapy. However, few clinical trials have compared patients with different risk scores. None of the studies investigated the analysis of the impact of risk scores on clinical outcomes. We expected more future clinical trials could include risk scores as an inclusion criterion and prove the effect of preventive antibiotics in patients with stroke. In addition, except preventive antibiotics, lots of other intervention could prevent SAP in patients with stroke of high risk. For instance, dysphagia assessment and management (52), modes of nutritional support (53, 54) and care programme (55) also play important parts in the prevention of SAP.
Limitation
This study also has some limitations. First, most of the studies are based on Chinese patients, which may lead to publication bias and affect the validity and reliability of this systematic review. Second, the number of studies for some scoring systems are low, which may affect the comparison with others.
Conclusion
In conclusion, the AIS-APS is a validated clinical tool for predicting SAP after the onset of acute ischemic stroke. Due to the limitations of this study, the results should be verified by more multi-center and large-sample prospective studies and geographically distinct populations.
Data availability statement
The original contributions presented in the study are included in the article/Supplementary material, further inquiries can be directed to the corresponding author/s.
Author contributions
XZ: conceptualization, methodology, software, formal analysis, quality assessment, investigation, resources, data curation, writing—original draft preparation, review and editing, and visualization. LX: conceptualization, methodology, validation, formal analysis, quality assessment, investigation, data curation, writing—original review and editing, and visualization. LN: investigation, resources, data curation, and software. YT: methodology, validation, data curation, quality assessment, writing—original review and editing, and supervision. KC: writing—original review and editing, supervision, and project administration. All data were generated in-house and no paper mill was used. All authors listed have made a substantial, direct, and intellectual contribution to the work and approved it for publication.
Conflict of interest
The authors declare that the research was conducted in the absence of any commercial or financial relationships that could be construed as a potential conflict of interest.
Publisher's note
All claims expressed in this article are solely those of the authors and do not necessarily represent those of their affiliated organizations, or those of the publisher, the editors and the reviewers. Any product that may be evaluated in this article, or claim that may be made by its manufacturer, is not guaranteed or endorsed by the publisher.
Supplementary material
The Supplementary Material for this article can be found online at: https://www.frontiersin.org/articles/10.3389/fmed.2022.964616/full#supplementary-material
Attachment 1. PRISMA NMA checklist.
Attachment 2. Search strategy.
Abbreviations
A2DS2, Age, Atrial fibrillation, Dysphagia, Sex, Stroke Severity; ISAN, Prestroke Independence, Sex, Age, National Institutes of Health Stroke Scale; AIS-APS, Acute Ischaemic Stroke-Associated Pneumonia Score; PANTHERIS, Preventive Antibacterial Therapy in Acute Ischaemic Stroke; AUC, Area under the receiver operating characteristic; BSR, Berlin Stroke Register; CI, Confidence interval; CNSR, China National Stroke Registry; COPD, indicates chronic obstructive pulmonary disease; DIC, Deviance information criterion; GCS, Glasgow Coma Scale; MCMC, Markov chain Monte Carlo; MD, Mean differences; mRS, modified Rankin Scale; NICU, Neurological Intensive Care Unit; NIHSS, National Institutes of Health Stroke Scale; NMA, Network meta-analysis; OCSP, Oxfordshire Community Stroke Project; OR, Odds ratios; POCI, Posterior circulation infarct; PRISMA, Preferred Reporting Items for Systematic reviews and Meta-Analyses; PSRF, Potential scale reduction factor; SAP, Stroke-associated pneumonia; SUCRA, Surface under the cumulative ranking curves; SSNAP, Sentinel Stroke National Audit Programme; TACI, Total anterior circulation infarct; TIA, Transient ischemic attack; UME, Unrelated mean effects; VHA, Veterans Health Administration.
References
1. Hilker R, Poetter C, Findeisen N, Sobesky J, Jacobs A, Neveling M, et al. Nosocomial pneumonia after acute stroke: implications for neurological intensive care medicine. Stroke. (2003) 34:975–81. doi: 10.1161/01.STR.0000063373.70993.CD
2. Cugy E, Sibon I. Stroke-associated pneumonia risk score: validity in a french stroke unit. J Stroke Cerebrovasc Dis. (2017) 26:225–9. doi: 10.1016/j.jstrokecerebrovasdis.2016.09.015
3. Chaves ML, Gittins M, Bray B, Vail A, Smith CJ. Variation of stroke-associated pneumonia in stroke units across England and Wales: a registry-based cohort study. Int J Stroke. 2021:17474930211006297. doi: 10.1177/17474930211006297
4. Smith CJ, Bray BD, Hoffman A, Meisel A, Heuschmann PU, Wolfe CD, et al. Can a novel clinical risk score improve pneumonia prediction in acute stroke care? A UK multicenter cohort study. J Am Heart Assoc. (2015) 4:e001307. doi: 10.1161/JAHA.114.001307
5. Finlayson O, Kapral M, Hall R, Asllani E, Selchen D, Saposnik G. Risk factors, inpatient care, and outcomes of pneumonia after ischemic stroke. Neurology. (2011) 77:1338–45. doi: 10.1212/WNL.0b013e31823152b1
6. Ali AN, Howe J, Majid A, Redgrave J, Pownall S, Abdelhafiz AH. The economic cost of stroke-associated pneumonia in a UK setting. Top Stroke Rehabil. (2018) 25:214–23. doi: 10.1080/10749357.2017.1398482
7. Teh WH, Smith CJ, Barlas RS, Wood AD, Bettencourt-Silva JH, Clark AB, et al. Impact of stroke-associated pneumonia on mortality, length of hospitalization, and functional outcome. Acta Neurol Scand. (2018) 138:293–300. doi: 10.1111/ane.12956
8. Kishore AK, Vail A, Chamorro A, Garau J, Hopkins SJ, Di Napoli M, et al. How is pneumonia diagnosed in clinical stroke research? A systematic review and meta-analysis. Stroke. (2015) 46:1202–9. doi: 10.1161/STROKEAHA.114.007843
9. Harms H, Hoffmann S, Malzahn U, Ohlraun S, Heuschmann P, Meisel A. Decision-making in the diagnosis and treatment of stroke-associated pneumonia. J Neurol Neurosurg Psychiatry. (2012) 83:1225–30. doi: 10.1136/jnnp-2012-302194
10. Smith CJ, Kishore AK, Vail A, Chamorro A, Garau J, Hopkins SJ, et al. Diagnosis of stroke-associated pneumonia: recommendations from the pneumonia in stroke consensus group. Stroke. (2015) 46:2335–40. doi: 10.1161/STROKEAHA.115.009617
11. Horan TC, Andrus M, Dudeck MA. CDC/NHSN surveillance definition of health care-associated infection and criteria for specific types of infections in the acute care setting. Am J Infect Control. (2008) 36:309–32. doi: 10.1016/j.ajic.2008.03.002
12. Wang Y, Chen Y, Lu C, Zhao X, Guo W, Bi Q, et al. An updated chinese consensus statement on stroke-associated pneumonia 2019. Chin J Stroke. (2019) 14:1251–62. doi: 10.4103/1995-7645.271937
13. Helmy TA, Abd-Elhady MAE, Abdou M. Prediction of ischemic stroke-associated pneumonia: a comparison between 3 scores. J Stroke Cerebrovasc Dis. (2016) 25:2756–61. doi: 10.1016/j.jstrokecerebrovasdis.2016.07.030
14. Chapman C, Morgan P, Cadilhac DA, Purvis T, Andrew NE. Risk factors for the development of chest infections in acute stroke: a systematic review. Top Stroke Rehabil. (2018) 25:445–58. doi: 10.1080/10749357.2018.1481567
15. Hannawi Y, Hannawi B, Rao CP, Suarez JI, Bershad EM. Stroke-associated pneumonia: major advances and obstacles. Cerebrovasc Dis. (2013) 35:430–43. doi: 10.1159/000350199
16. Sarah H, Uwe M, Hendrik H, Hans-Christian K, Klaus B, Marianne K, et al. Development of a clinical score (A2DS2) to predict pneumonia in acute ischemic stroke. Stroke. (2012) 43:2617–23. doi: 10.1161/strokeaha.112.653055
17. Ji RJ, Shen HP, Pan YS, Wang PL, Liu GF, Wang YL, et al. Novel risk score to predict pneumonia after acute ischemic stroke. Stroke. (2013) 44:1303. doi: 10.1161/STROKEAHA.111.000598
18. Kwon HM, Jeong SW, Lee SH, Yoon BW. The pneumonia score: a simple grading scale for prediction of pneumonia after acute stroke. Am J Infect Control. (2006) 34:64–8. doi: 10.1016/j.ajic.2005.06.011
19. Harms H, Reimnitz P, Bohner G, Werich T, Klingebiel R, Meisel C, et al. Influence of stroke localization on autonomic activation, immunodepression, and post-stroke infection. Cerebrovasc Dis. (2011) 32:552–60. doi: 10.1159/000331922
20. Chumbler NR, Williams LS, Wells CK, Lo AC, Nadeau S, Peixoto AJ, et al. Derivation and validation of a clinical system for predicting pneumonia in acute stroke. Neuroepidemiology. (2010) 34:193–9. doi: 10.1159/000289350
21. Jing H, Xiding P, Niannian G, Yang Y, Junshan Z, Youyong T. Prediction of stroke-associated pneumonia in patients with acute ischemic stroke:comparison of 6 scores. Int J Cerebrovasc Dis. (2017) 25:972–78. doi: 10.3760/cma.j.issn.1673-4165.2017.11.002
22. Ni JC, Shou WQ, Wu XP, Sun JH. Prediction of stroke-associated pneumonia by the A2DS2, AIS-APS, and ISAN scores: a systematic review and meta-analysis. Expert Rev Respir Med. (2021) 15:1461–72. doi: 10.1080/17476348.2021.1923482
23. Jie H, Ming L, Weiliang H, Feifei L, Jinming C, Hebo W. Use of the A2DS2 scale to predict morbidity in stroke-associated pneumonia: a systematic review and meta-analysis. BMC Neurol. (2021) 21:33. doi: 10.1186/s12883-021-02060-8
24. Yang B, Mallett S, Takwoingi Y, Davenport CF, Hyde CJ, Whiting PF, et al. QUADAS-C: a tool for assessing risk of bias in comparative diagnostic accuracy studies. Ann Intern Med. (2021) 174:1592–9. doi: 10.7326/M21-2234
25. Lunn D JC, Best N, Thomas A, Spiegelhalter D. The BUGS Book: A Practical Introduction to Bayesian Analysis. Boca Raton, FL: CRC Press (2012). doi: 10.1201/b13613
26. Efthimiou O, Debray TP, van Valkenhoef G, Trelle S, Panayidou K, Moons KG, et al. GetReal in network meta-analysis: a review of the methodology. Res Synth Methods. (2016) 7:236–63. doi: 10.1002/jrsm.1195
27. Veroniki AA, Mavridis D, Higgins JP, Salanti G. Characteristics of a loop of evidence that affect detection and estimation of inconsistency: a simulation study. BMC Med Res Methodol. (2014) 14:106. doi: 10.1186/1471-2288-14-106
28. van Valkenhoef G, Dias S, Ades AE, Welton NJ. Automated generation of node-splitting models for assessment of inconsistency in network meta-analysis. Res Synth Methods. (2016) 7:80–93. doi: 10.1002/jrsm.1167
29. Salanti G, Ades AE, Ioannidis JP. Graphical methods and numerical summaries for presenting results from multiple-treatment meta-analysis: an overview and tutorial. J Clin Epidemiol. (2011) 64:163–71. doi: 10.1016/j.jclinepi.2010.03.016
30. Riley RD, Jackson D, Salanti G, Burke DL, Price M, Kirkham J, et al. Multivariate and network meta-analysis of multiple outcomes and multiple treatments: rationale, concepts, and examples. BMJ. (2017) 358:j3932. doi: 10.1136/bmj.j3932
31. Chaimani A, Higgins JP, Mavridis D, Spyridonos P, Salanti G. Graphical tools for network meta-analysis in STATA. PLoS ONE. (2013) 8:e76654. doi: 10.1371/journal.pone.0076654
32. Salanti G, Del Giovane C, Chaimani A, Caldwell DM, Higgins JP. Evaluating the quality of evidence from a network meta-analysis. PLoS ONE. (2014) 9:e99682. doi: 10.1371/journal.pone.0099682
33. Na W, Lu-shan L, Feng-rong W. Comparison of four scoring systems in predicting risk of stroke-associated pneumonia in patients with acute ischemic stroke. Chin J Rehabil Theory Pract. (2018) 24:696–701. doi: 10.3969/j.issn.1006-9771.2018.06.014
34. Ye S, Dongqin C. Comparison of different stroke-associated pneumonia prediction scores in patients with ischemic stroke. J Nurs. (2018) 25:43–6. doi: 10.16460/j.issn1008-9969.2018.24.043
35. Yapeng Z, Aiming Y. Comparison of clinical predictive value of A2DS2 and AIS-APS scale for acute ischemic stroke-associated pneumonia. J Cardiovasc Pulmon Dis. (2019) 38:624–8. doi: 10.3969/j.issn.1007-5062.2019.06.008
36. Xuefu H, Fenlian H, Yongmei Q, Wenjun R. Predictive value of different risk scoring systems for stroke-associated pneumonia in the patients with acute stroke. Chin J Crit Care Med. (2020) 40:976–80. doi: 10.3969/j.issn.1002-1949.2020.10.014
37. Xiaona L, Xinsheng L, Kun W, Shiguang L, Jiangbo C, Lijun F, et al. Clinical value of A2DS2 and AIS-APS in prdicting stroke-associated pneumonia. Chin J Geriatr Heart Brain Vessel Dis. (2018) 20:1183–86. doi: 10.3969/j.issn1009-0126.2018.11.015
38. Rui Y, Hao L, Xiaopeng W, Jiawei M, Qinglian M, Yujie Y. Comparison of A2DS2 and AIS-APS scores in the predictive accuracies of stroke-associated pneumonia. Contempor Med. (2019) 25:164–6. doi: 10.3969/j.issn.1009-4393.2019.09.076
39. Lei H, Yong L, Qiuxia Z. Comparison of A2DS2 and AIS-APS scores in prediction of stroke-associated pneumonia in the elderly. Chin J Lung Dis. (2021) 14:335–7. doi: 10.3877/cma.j.issn.1674-6902.2021.03.018
40. Fang L, Lihua X, Ruijun J, Kai Y, Hongna Y, Yinglin Y, et al. Analysis of clinical value of acute ischemic stroke-associated pneumonia score. Chin J Stroke. (2020) 15:1204–09. doi: 10.3969/j.issn.1673-5765.2020.11.009
41. Huang GQ, Lin YT, Wu YM, Cheng QQ, Cheng HR, Wang Z. Individualized prediction of stroke-associated pneumonia for patients with acute ischemic stroke. Clin Interv Aging. (2019) 14:1951–62. doi: 10.2147/CIA.S225039
42. Jiao J, Geng L, Zhang Z. Do we need to distinguish thrombolysis and nonthrombolysis patients when applying stroke-associated pneumonia predicting scores? An external validation from a 2-center database. Med Sci Monit. (2020) 26:e924129. doi: 10.12659/MSM.924129
43. Zhang R, Ji R, Pan Y, Jiang Y, Liu G, Wang Y, et al. External validation of the prestroke independence, sex, age, national institutes of health stroke scale score for predicting pneumonia after stroke using data from the China National Stroke Registry. J Stroke Cerebrovasc Dis. (2017) 26:938–43. doi: 10.1016/j.jstrokecerebrovasdis.2016.10.043
44. Ming TT, Sh PS, Sanchalika A, May NW, Ct OD. Predicting pneumonia in acute ischaemic stroke: comparison of five prediction scoring models. Ann Acad Med. (2017) 46:237–44. doi: 10.47102/annals-acadmedsg.V46N6p237
45. Ramírez-Moreno JM, Martínez-Acevedo M, Cordova R, Roa AM, Constantino AB, Ceberino D, et al. External validation of the A2DS2 and ISAN scales for predicting infectious respiratory complications of ischaemic stroke. Neurología. (2018) 34:14–21. doi: 10.1016/j.nrleng.2018.09.012
46. Siregar DH, Sjahrir H, Nasution IK. Accuracy comparison of age, atrial fibrillation, dysphagia, stroke severity, sex (A2DS2) and acute ischemic stroke-associated pneumonia score (AIS-APS) to predict pneumonia in acute ischemic stroke. J Neurol Sci. (2017) 381:998. doi: 10.1016/j.jns.2017.08.2816
47. Elhasin H, Szolics M, Hassan A, Soliman N, Kaasch A. Risk scores for predicting stroke associated pneumonia: validity of A2DS2 and ISAN scores. J Neurol Sci. (2019) 405:41–42. doi: 10.1016/j.jns.2019.10.501
48. Rehan J, Kilner K, Redgrave J, Majid A, Ali A. External validation of pneumonia prediction scores after stroke in a prospective UK cohort study. Eur Stroke J. (2018) 3:204. doi: 10.1177/2396987318770127
49. Qingxia D, Ning D, Weiyi L, Jiebin L, Xulian Y, Meng Z, et al. The value of ischemic stroke acute ischemic stroke-associated pneumonia score in predicting stroke-associated pneumonia. Chin J Emerg Med. (2016) 25:1268–71. doi: 10.3760/cma.j.issn.1671-0282.2016.10.011
50. Yuan MZ, Li F, Tian X, Wang W, Jia M, Wang XF, et al. Risk factors for lung infection in stroke patients: a meta-analysis of observational studies. Expert Rev Anti Infect Ther. (2015) 13:1289–98. doi: 10.1586/14787210.2015.1085302
51. Westendorp WF, Vermeij JD, Smith CJ, Kishore AK, Hodsoll J, Kalra L, et al. Preventive antibiotic therapy in acute stroke patients: a systematic review and meta-analysis of individual patient data of randomized controlled trials. Eur Stroke J. (2021) 6:385–94. doi: 10.1177/23969873211056445
52. Eltringham SA, Kilner K, Gee M, Sage K, Bray BD, Pownall S, et al. Impact of dysphagia assessment and management on risk of stroke-associated pneumonia: a systematic review. Cerebrovasc Dis. (2018) 46:99–107. doi: 10.1159/000492730
53. Lyons M, Smith C, Boaden E, Brady MC, Brocklehurst P, Dickinson H, et al. Oral care after stroke: where are we now? Eur Stroke J. (2018) 3:347–54. doi: 10.1177/2396987318775206
54. Heyland DK, Drover JW, Dhaliwal R, Greenwood J. Optimizing the benefits and minimizing the risks of enteral nutrition in the critically ill: role of small bowel feeding. J Parenter Enteral Nutr. (2002) 26(6 Suppl.):S51–5. Discussion S6–7. doi: 10.1177/014860710202600608
Keywords: risk prediction, risk score, ischemic stroke (IS), network meta-analysis, stroke-associated pneumonia (SAP)
Citation: Zhang X, Xiao L, Niu L, Tian Y and Chen K (2022) Comparison of six risk scores for stroke-associated pneumonia in patients with acute ischemic stroke: A systematic review and Bayesian network meta-analysis. Front. Med. 9:964616. doi: 10.3389/fmed.2022.964616
Received: 08 June 2022; Accepted: 26 September 2022;
Published: 12 October 2022.
Edited by:
Ming Yang, Sichuan University, ChinaReviewed by:
Qingyu Dou, Sichuan University, ChinaZhiyan Liu, Peking University First Hospital, China
Copyright © 2022 Zhang, Xiao, Niu, Tian and Chen. This is an open-access article distributed under the terms of the Creative Commons Attribution License (CC BY). The use, distribution or reproduction in other forums is permitted, provided the original author(s) and the copyright owner(s) are credited and that the original publication in this journal is cited, in accordance with accepted academic practice. No use, distribution or reproduction is permitted which does not comply with these terms.
*Correspondence: Kuang Chen, MTg2MjIxNTAyODFAMTYzLmNvbQ==
†These authors have contributed equally to this work and share first authorship