- Department of Clinical Laboratory, The First Hospital of Jiaxing and The Affiliated Hospital of Jiaxing University, Jiaxing, China
Purpose: A series of complications caused by severe COVID-19 can significantly affect short-term results. Therefore, early diagnosis is essential for critically COVID-19 patients. we aimed to investigate the correlation among D-dimer levels, lymphocyte subsets, cytokines, and disease severity in COVID-19 patients.
Methods: Systematic review and meta- analysis of PubMed, Scopus, Web of Science, Cochrane Central Register of Controlled Trials, Embase, clinical trials, and China National Knowledge Infrastructure (CNKI) until 1 August 2022. We considered case-control, and cohort studies that compared laboratory parameters between patients with severe or non-serious diseases or between survivors and non-survivors. Pooled data was assessed by use of a random-effects model and used I2 to test heterogeneity. We assessed the risk of bias using the Newcastle- Ottawa Scale.
Results: Of the 5,561 identified studies, 32 were eligible and included in our analysis (N = 3,337 participants). Random-effect results indicated that patients with COVID-19 in severe group had higher levels for D-dimer (WMD = 1.217 mg/L, 95%CI=[0.788, 1.646], P < 0.001), neutrophil-to-lymphocyte ratio (NLR) (WMD = 6.939, 95%CI = [4.581, 9.297], P < 0.001), IL-2 (WMD = 0.371 pg/ml, 95%CI = [−0.190, 0.932], P = 0.004), IL-4 (WMD = 0.139 pg/ml, 95%CI = [0.060, 0.219], P = 0.717), IL-6 (WMD = 44.251 pg/ml, 95%CI = [27.010, 61.493], P < 0.001), IL-10 (WMD = 3.718 pg/ml, 95%CI = [2.648, 4.788], P < 0.001) as well as lower levels of lymphocytes (WMD = −0.468(× 109/L), 95%CI = [−0.543, −0.394], P < 0.001), T cells (WMD = −446.746(/μL), 95%CI = [−619.607, −273.885], P < 0.001), B cells (WMD = −60.616(/μL), 95%CI = [−96.452, −24.780], P < 0.001), NK cells (WMD = −68.297(/μL), 95%CI = [−90.600, −45.994], P < 0.001), CD3+T cells (WMD = −487.870(/μL), 95%CI = [−627.248, −348.492], P < 0.001), CD4+T cells (WMD = −290.134(/μL), 95%CI = [−370.834, −209.435], P < 0.001), CD8+T cells (WMD = −188.781(/μL), 95%CI = [−227.806, −149.757], P < 0.001).
Conclusions: There is a correlation among higher levels of D-dimer, cytokines, lower levels of lymphocyte subsets, and disease severity in COVID-19 patients. These effective biomarkers may help clinicians to evaluate the severity and prognosis of COVID-19. This study is registered with PROSPERO, number CRD42020196659.
Systematic review registration: https://www.crd.york.ac.uk/PROSPERO/display_record.php?RecordID=196659; PROSPERO registration number: CRD42020196659.
Introduction
The current 2019 Novel Coronavirus (2019-nCoV) infection reportedly originated in Wuhan, Hubei Province, China in December 2019. After being declared a pandemic on March 11, 2020, it has affected more than 200 countries / Areas of 40 million people (1). Countries around the world have entered a state of emergency, and everyone can feel the impact on health, business, and other aspects of daily life. According to reports, the most common initial symptoms of COVID-19 are fever, cough, fatigue, anorexia, and diarrhea (2). Shortness of breath occurs on average 5 to 8 days after the initial symptoms appear; its appearance indicates that the condition has deteriorated (3). Although most patients with COVID-19 pneumonia have a good prognosis, some patients develop to acute respiratory distress syndrome (ARDS), coagulation disorders, or multiple organ failure, with a mortality rate between 4 and 15% (4, 5). More timely and effective early intervention for severe patients is a priority. And early diagnosis is the best approach to achieve this aim. Therefore, we believe that comprehensive monitoring of the severity of COVID-19 and effective early intervention are basic measures to reduce the mortality rate. Some studies have reported several abnormal hematological parameters in patients with COVID-19, including lymphopenia, neutrophilia, elevated levels of D-dimer, and fibrinogen (6–9).
Besides, inflammatory cytokine levels are obvious laboratory abnormalities observed during infection with COVID-19 (10, 11). However, the clinical significance of these biomarkers has not been fully clarified. This meta-analysis aims to reveal the characteristics of laboratory test results in patients with COVID-19 through the included articles, especially the changes in severely ill patients, to define which parameters can distinguish those who are at higher risk of severe and non-serious diseases.
Methods
Search strategy and selection criteria
This meta-analysis is reported following with the Preferred Reporting Items for Systematic Reviews and Meta-Analyses (PRISMA) Statement and has been registered at the International Prospective Register of Systematic Reviews (numberCRD42020196659) (12).
We search PubMed/MEDLINE, Scopus, Web of Science, Cochrane Central Register of Controlled Trials, Embase, clinical trials, China National Knowledge Infrastructure (CNKI) and Google Scholar databases for articles published until 1 August 2022, using the keywords. “coronavirus,” “2019-nCoV,” “COVID-19,” “SARS-CoV-2,” “2019 novel coronavirus disease,” “coronavirus disease 2019,” “laboratory,” “clinical characteristics.” In addition, the WHO publication database, lancet, New England Journal of Medicine, JAMA, BMJ were screened to find potentially relevant publications. To ensure the comprehensiveness and accuracy of the research, we also consulted the references of the attached literature.
Study designs eligible for inclusion were cross sectional, case-control, and cohort studies. To be eligible, the studies must clearly indicate that the patients have been diagnosed as COVID-19 and were positive for SARS-CoV-2RNA. Studies were excluded if patients were asymptomatic carriers and did not fulfill the inclusion criteria. Studies were considered eligible for inclusion in this meta-analysis if they compared laboratory parameters between patients with severe or non-serious diseases or between survivors and non-survivors. Since the pre-prints have not been peer-reviewed, we did not include these papers in our analysis to avoid the spread of any potential misinformation. The following studies were excluded: duplicate publications, reviews, editorials, single case reports, small case series (< 10 cases), studies did not include the biomarkers required for the meta-analysis or missing research data. Two authors independently screened the title and abstract based on these selection criteria, discussed the differences with another author and subsequently resolved through negotiation.
Data extraction and quality assessment
Two authors (HYZ, HJW) independently extracted the data, differences were resolved in a discussion, or, if a consensus could not be reached, the third author resolved (WFS). We extracted the following variables: first author; publication year; study design; country; the number of participants in severe and non-severe disease groups; levels of laboratory indexes (d-dimer levels, lymphocyte subsets, cytokines) in different groups. If further information is needed, we will email the corresponding author, if there is no response, we will exclude the study. Stratified data or interquartile range (IQR) were converted to mean (±SD) using mathematical formulas for meta-analysis (13, 14). We planned to use the Cochrane Risk of Bias tool to assess the risk of bias in randomized trials, but our search did not find any eligible randomized trials. We used the Newcastle-Ottawa scale to rate the risk of bias in the non-randomized study. There are 8 criteria for NOS, with scores ranging from 0 (high-risk deviation) to 9 (low-risk deviation). Studies with a NOS score> 7 were considered high- quality.
Statistical analysis
We used Stata (version 12.0) for all statistical analysis. We used the I2 statistic and Cochran's Q-test to assess statistical heterogeneity. We believe that an I2 statistics of 0–25% shows a low heterogeneity, the medium heterogeneity is 26–75%, and the high heterogeneity is 76–100%, the heterogeneity p-value is < 0.05. If there is heterogeneity, the random effect model was used, otherwise, the fixed-effect model was used. Weighted mean difference (WMD) with 95% confidence intervals (95% CI) was calculated for D-dimer, lymphocyte subsets, and cytokines. We studied the influence of a single study on the overall risk estimate and eliminated each study in each round to test the robustness of the main results. If a meta- analysis included more than ten studies, publication bias was assessed by Begg and Egger test. We defined significant publication bias as a p-value < 0.05.The trim-and-fill computation was used to estimate the effect of publication bias on the interpretation of the results.
Results
Literature search and studies characteristics
We identified 5561 studies, of which 32 (data from 3337 participants) were included in our analysis (10, 11, 15–44) (Figure 1). The basic characteristics of the articles included in the study are shown in Table 1. Five of the 32 trials were published in 2022, four trials in 2021, and 23 trials were published in 2020. Two studies were from Italy, two studies were from Turkey, one study was from Germany, one study was from the United States, one study was from Spain, 1 study was from India, one study was from the Republic of North Macedonia, and the rest were from China. Twenty-eight studies were grouped by severity and non-severity, and four studies were grouped by survivors and non-survivors of COVID-19. Among these articles, 21 articles described the D-dimer levels of COVID-19 patients, 28 articles described the lymphocyte subsets levels of COVID-19 patients, 17 articles described the cytokine levels of COVID-19 patients. All the included studies were rated high quality according to the NOS scores and details are shown in Table 2.
Association of d-dimer levels in the peripheral blood with the severity of COVID-19
Twenty-one articles showed that compared with patients in non-severe group, patients in severe group had higher levels of D-dimer (WMD = 1.217 mg/L, 95%CI = [0.788, 1.646], P < 0.001) (Figure 2). A sensitivity analysis was carried out by excluding one study at a time and reanalyzing the entire data set. We found that the results were not influenced by excluding any one specific study (Supplementary material 1A). A funnel plot based on the d-dimer levels of patients showed p-values of 0.001 in Egger's test (Supplementary material 1B). There was publication bias in the studies included in the meta-analysis. After filling one trial, the revised result was still consistent using random model (WMD = 0.306 mg/L, 95%CI = [−0. 167, 0.778], P = 0.205) or fix model (WMD = 0.127 mg/L, 95%CI = [0.058, 0.195], P < 0.001) (Supplementary material 1C). Besides, using standard mean difference (SMD) for the meta-analysis still did not change the conclusion (Table 3).
The decrease of lymphocyte subsets in severe patients with COVID-19
A random-effects model was used to analyze the correlation between the level of patient lymphocyte subsets and the severity of COVID-19. Compared with the non-severe group, patients in the severe group had lower levels for lymphocytes (WMD = −0.468(× 109/L), 95%CI = [−0.543, −0.394], P < 0.001) (Figure 3), T cells (WMD = −446.746 (/μL), 95%CI = [−619.607, −273.885], P < 0.001), B cells(WMD = −60.616(/μL), 95%CI = [−96.452, −24.780], P < 0.001), NK cells (WMD = −68.297(/μL), 95%CI = [−90.600, −45.994], P < 0.001), CD3+T cells (WMD = −487.870(/μL), 95%CI = [−627.248, −348.492], P < 0.001), CD4+T cells (WMD = −290.134(/μL), 95%CI = [−370.834, −209.435], P < 0.001), CD8+T cells (WMD = −188.781(/μL), 95%CI = [−227.806, −149.757], P < 0.001) (Supplementary materials 2A–F). Furthermore, the neutrophil-to-lymphocyte ratio (NLR) (WMD = 6.939, 95%CI = [4.581, 9.297], P < 0.001) (Figure 4) of severe patients was higher than that of non-severe patients. Sensitivity analyses indicated that the results were robustness by removing any one specific study in all the lymphocyte subsets and NLR between non-severe and severe groups (Supplementary materials 2G–N). No significant publication bias was detected in most of the studies except for NK cells (p = 0.034) and neutrophil-to-lymphocyte ratio (NLR) (p = 0.016) (Supplementary materials 2O–T). When applying the trim-and-fill method, there were no any trials trimmed and filled in NK cells and neutrophil-to-lymphocyte ratio(NLR) (Supplementary materials 2U,V). Besides, using standard mean difference (SMD) for the meta-analysis still did not change the conclusion (Table 3).
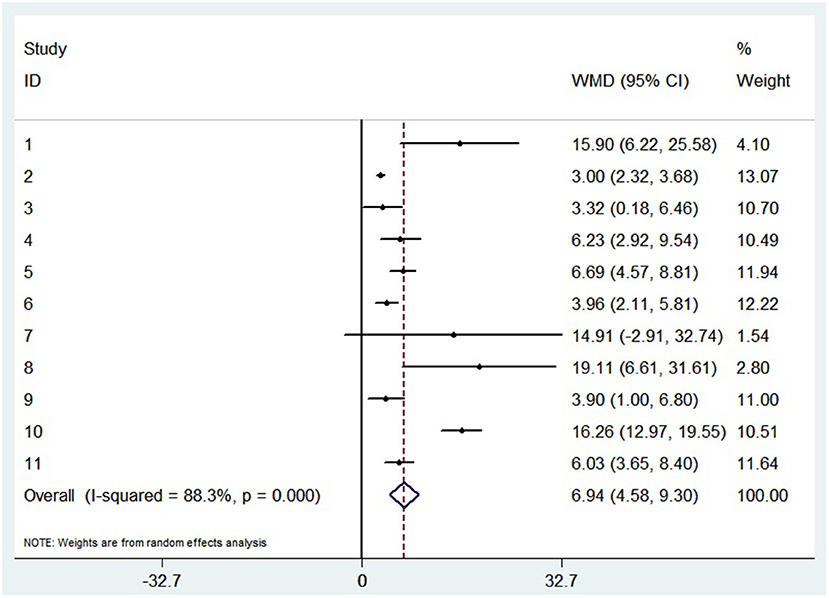
Figure 4. Forest plot between non-severe and severe groups for levels of neutrophil-to-lymphocyte ratio (NLR).
The increase of cytokines in peripheral blood of patients with COVID-19
The random-effect results demonstrated that compared with patients in the non-severe group, patients in the severe group had higher levels for interleukin (IL)-2 (WMD = 0.371 pg/ml, 95%CI = [−0.190,0.932], P = 0.004), IL-4 (WMD = 0.139 pg/ml, 95%CI = [0.060, 0.219], P = 0.717) (Supplementary materials 3A,B), IL-6 (WMD = 44.251 pg/ml, 95%CI = [27.010, 61.493], P < 0.001) (Figure 5), IL-10 (WMD = 3.718 pg/ml, 95%CI = [2.648, 4.788], P < 0.001) (Supplementary material 3C). Sensitivity analysis by removing one study in each turn, the result indicated that the main result was robustness (Supplementary materials 3D–G). A funnel plot based on the IL-6 levels of patients showed p-values of 0.031 in Egger's test (Supplementary material 3H). No significant publication bias was detected in IL-10 (Supplementary material 4I). When applying the trim-and-fill method, there were no any trials trimmed and filled in IL-6 (Supplementary material 3J). Besides, using standard mean difference (SMD) for the meta-analysis still did not change the conclusion (Table 3).
Discussion
This meta-analysis showed that higher levels of d-dimer, cytokines, NLR, and lower levels of lymphocyte subsets were associated with the severity of COVID-19 infection.
Several studies have shown that the inflammatory response plays a key role in COVID-19, excessive elevation of inflammatory cytokines can cause the so-called “cytokine storm,” and the inflammatory cytokine storm increases the severity of COVID-19 (45, 46). The fifth edition of “Diagnosis and Treatment of COVID-19” recommends monitoring the cytokine levels to improve treatment effectiveness and reduce mortality (47). Siddiqi et al. (48) found that inflammatory cytokines and biomarkers are significantly elevated during the systemic inflammation stage of COVID-19 and excessive hyper inflammation may lead to cardiopulmonary collapse and multi-organ failure. Several studies have shown that the blood coagulation function is significantly activated during severe COVID-19 infection, which may be related to the sustained inflammation response caused by the release of cytokines induced by virus invasion. Recent lung pathological anatomy evidence showed that pulmonary small vessel occlusion and micro thrombosis in critically ill patients with COVID-19 (7). The most significant coagulation marker is the significant and dynamic increase of D-dimer levels. However, the etiology of elevated serum D-dimer levels is multifactorial. The significant inflammatory response in patients with severe COVID-19 may increase the possibility of thromboembolic disease, which may explain the increase in serum d-dimer levels. COVID-19-related coagulopathy needs special attention and treatment. In the absence of contraindications, it is recommended to use a preventive dose of anticoagulant for all COVID-19 patients.
Many studies have reported lymphopenia in patients with COVID-19, because SARS-CoV-2 particles may destroy the cytoplasmic components of lymphocytes and cause apoptosis (8). For the SARS virus, He et al. (9) suspected that lymphocytes and their subsets are essential to eliminating virus-infected cells, while for COVID-19 Henry B hypothesized that survival may depend on the ability to replenish lymphocytes and their subsets killed by the virus (31). Therefore, lymphocyte count may be used as a clinical predictor of severity and prognosis. Previous studies have shown that the severity of SARS pathological damage is related to the extensive infiltration of neutrophils in the lung and the increase in the number of peripheral blood neutrophils (49). Therefore, the increase in neutrophil count may indicate the intensity of the inflammatory responses in patients with COVID-19. Besides, the degree of lymphopenia also indicates course and severity of the COVID-19. Thus, NLR may have a potential value in monitoring the condition of severe COVID-19 patients.
In terms of immune biomarkers, elevated IL-6, IL-10, along with elevated C-reactive protein (CRP) and procalcitonin (PCT), indicating that patients with severe diseases have systemic inflammatory response syndrome (SIRS). Additionally, elevated IL-10 may be related to compensatory anti-inflammatory response (CARS), which may be the cause of secondary infection in the severe group and non-survival group (50). Therefore, we suggest that these parameters can be used to monitor the prognosis of COVID-19 patients during hospitalization.
However, some limitations in our meta-analysis should be mentioned. Firstly, the number of cases is small. Secondly, Most of the included studies were from China. As more data from other countries becomes available, further investigation is needed. Lastly, the language of studies was limited to English, which may result in potential language bias.
In conclusion, our analysis showed that higher levels of D-dimer, NLR, cytokines (IL-2, IL-4, IL-6, IL-10) and lower levels of lymphocyte subsets in severe patients, which are of great significance for predicting disease changes. For hospitalized patients, we recommend that clinicians closely monitor D-dimer levels, lymphocyte subsets, and cytokines as markers for potential progression to severe disease. Finally, these parameters should continue to be re-evaluated in the future, because more data can be obtained in future large cohort studies.
Data availability statement
The original contributions presented in the study are included in the article/Supplementary material, further inquiries can be directed to the corresponding author/s.
Author contributions
HZ and WS were responsible for study design. HZ and DP were involved in data collection. HZ and HW analyzed the data. HZ wrote the manuscript. HZ, HW, DP, and WS revised the manuscript. All authors contributed to the article and approved the submitted version.
Funding
This work was funded by the Zhejiang Medicine and Health under Grant 2022RC077, the Venus Talent Training of the First Hospital of Jiaxing of Zhejiang Province of China under Grant 2020-QMX-25.
Conflict of interest
The authors declare that the research was conducted in the absence of any commercial or financial relationships that could be construed as a potential conflict of interest.
Publisher's note
All claims expressed in this article are solely those of the authors and do not necessarily represent those of their affiliated organizations, or those of the publisher, the editors and the reviewers. Any product that may be evaluated in this article, or claim that may be made by its manufacturer, is not guaranteed or endorsed by the publisher.
Supplementary material
The Supplementary Material for this article can be found online at: https://www.frontiersin.org/articles/10.3389/fmed.2022.988666/full#supplementary-material
References
1. Lai CC, Shih TP, Ko WC, Tang HJ, Hsueh PR. Severe acute respiratory syndrome coronavirus 2 (SARS-CoV-2) and coronavirus disease-2019 (COVID-19): the epidemic and the challenges. Int J Antimicrob Agents. (2020) 55:105924. doi: 10.1016/j.ijantimicag.2020.105924
2. Berlin DA, Gulick RM, Martinez FJ. Severe Covid-19. N Engl J Med. (2020) 383:2451–60. doi: 10.1056/NEJMcp2009575
3. Gandhi RT, Lynch JB, Del Rio C. Mild or moderate Covid-19. N Engl J Med. (2020) 383:1757–66. doi: 10.1056/NEJMcp2009249
4. Huang C, Wang Y, Li X, Ren L, Zhao J, Hu Y, et al. Clinical features of patients infected with 2019 novel coronavirus in Wuhan, China. Lancet. (2020) 395:497—506. doi: 10.1016/S0140-6736(20)30183-5
5. Wang D, Hu B, Hu C, Zhu F, Liu X, Zhang J, et al. Clinical characteristics of 138 hospitalized patients with 2019 Novel coronavirus-infected pneumonia in Wuhan, China. JAMA. (2020) 323:1061–9. doi: 10.1001/jama.2020.1585
6. Guan, WJ, Zhong NS. Clinical Characteristics of Covid-19 in China. Reply. N Eng J Med. (2020) 382:1861–2. doi: 10.1056/NEJMc2005203
7. Luo W, Yu H, Gou J, Li X, Sun Y, Li J, et al. Clinical pathology of critical patient with novel coronavirus pneumonia (COVID-19). Preprints. doi: 10.1097/TP.0000000000003412
8. Gu J, Gong E, Zhang B, Zheng J, Gao Z, Zhong Y, et al. Multiple organ infection and the pathogenesis of SARS. J Exp Med. (2005) 202:415–24. doi: 10.1084/jem.20050828
9. He Z, Zhao C, Dong Q, Zhuang H, Song S, Peng G, et al. Effects of severe acute respiratory syndrome (SARS) coronavirus infection on peripheral blood lymphocytes and their subsets. Int J Infect Dis. (2005) 9:323–30. doi: 10.1016/j.ijid.2004.07.014
10. Qin C, Zhou L, Hu Z, Zhang S, Yang S, Tao Y, et al. Dysregulation of Immune Response in Patients With Coronavirus 2019 (COVID-19) in Wuhan, China. Clin Infect Dis. (2020) 71:762–8. doi: 10.1093/cid/ciaa248
11. Chen G, Wu D, Guo W, Cao Y, Huang D, Wang H, et al. Clinical and immunological features of severe and moderate coronavirus disease 2019. J Clin Invest. (2020) 130:2620–9. doi: 10.1172/JCI137244
12. Moher D, Liberati A, Tetzlaff J, Altman DG, PRISMA Group. Preferred reporting items for systematic reviews and meta-analyses: the PRISMA statement. PLoS Med. (2009) 6:e1000097. doi: 10.1371/journal.pmed.1000097
13. Luo D, Wan X, Liu J, Tong T. Optimally estimating the sample mean from the sample size, median, mid-range, and/or mid-quartile range. Statist Methods Med Res. (2018) 27:1785–1805. doi: 10.1177/0962280216669183
14. Wan X, Wang W, Liu J, Tong T. Estimating the sample mean and standard deviation from the sample size, median, range and/or interquartile range. BMC Med Res Methodol. (2014) 14:135. doi: 10.1186/1471-2288-14-135
15. Idiz UO, Yurttas TT, Degirmencioglu S, Orhan B, Erdogan E, Sevik H, et al. Immunophenotyping of lymphocytes and monocytes and the status of cytokines in the clinical course of Covid-19 patients. J Med Virol. (2022) 94:4744–53. doi: 10.1002/jmv.27917
16. Koc I, Unalli Ozmen S. Eosinophil levels, neutrophil-lymphocyte ratio, and platelet-lymphocyte ratio in the cytokine storm period of patients with COVID-19. Int J Clin Pract. (2022) 2022:7450739. doi: 10.1155/2022/7450739
17. Zendelovska D, Petrushevska M, Atanasovska E, Spasovska K, Gjorgjievska K, Pavlovska K, et al. COVID-19 disease induced alteration of oxidative stress and clinical laboratory parameters in moderate and severe patients. Eur Rev Med Pharmacol Sci. (2022) 26:5611–7. doi: 10.26355/eurrev_202208_29434
18. Wang H, Zhang Y, Mo P, Liu J, Wang H, Wang F, et al. Neutrophil to CD4+ lymphocyte ratio as a potential biomarker in predicting virus negative conversion time in COVID-19. Int Immunopharmacol. (2020) 85:106683. doi: 10.1016/j.intimp.2020.106683
19. Masotti L, Grifoni E, Pelagalli G, Cioni E, Mattaliano C, Cioffi E, et al. Prognostic role of Interleukin-6/lymphocytes ratio in SARS-CoV2 related pneumonia. Int Immunopharmacol. (2022) 103:108435. doi: 10.1016/j.intimp.2021.108435
20. Zhang J, Wang Z, Wang X, Hu Z, Yang C, Lei P. Risk factors for mortality of COVID-19 patient based on clinical course: a single center retrospective case-control study. Front Immunol. (2021) 12:581469. doi: 10.3389/fimmu.2021.581469
21. Tang Y, Sun J, Pan H, Yao F, Yuan Y, Zeng M, et al. Aberrant cytokine expression in COVID-19 patients: associations between cytokines and disease severity. Cytokine. (2021) 143:155523. doi: 10.1016/j.cyto.2021.155523
22. Pujani M, Raychaudhuri S, Singh M, Kaur H, Agarwal S, Jain M, et al. An analysis of hematological, coagulation and biochemical markers in COVID-19 disease and their association with clinical severity and mortality: an Indian outlook. Am J Blood Res. (2021) 11:580–91.
23. García-Gasalla M, Ferrer JM, Fraile-Ribot PA, Ferre-Beltrán A, Rodríguez A, Martínez-Pomar N, et al. predictive immunological, virological, and routine laboratory markers for critical COVID-19 on admission. J canadien des maladies infectieuses et de la microbiologie medicale. (2021) 2021:9965850. doi: 10.1155/2021/9965850
24. Li X, Wang L, Yan S, Yang F, Xiang L, Zhu J, et al. Clinical characteristics of 25 death cases with COVID-19: A retrospective review of medical records in a single medical center, Wuhan, China. Int J Infect Dis. (2020) 94:128–32. doi: 10.1016/j.ijid.2020.03.053
25. Chen T, Wu D, Chen H, Yan W, Yang D, Chen G, et al. Clinical characteristics of 113 deceased patients with coronavirus disease 2019: retrospective study. BMJ (Clinical research ed). (2020) 368:m1091. doi: 10.1136/bmj.m1091
26. Dong X, Wang M, Liu S, Zhu J, Xu Y, Cao H, et al. Immune Characteristics of Patients with Coronavirus Disease 2019 (COVID-19). Aging Dis. (2020) 11:642–8. doi: 10.14336/AD.2020.0317
27. He R, Lu Z, Zhang L, Fan T, Xiong R, Shen X, et al. The clinical course and its correlated immune status in COVID-19 pneumonia. J Clin Virol. (2020) 127:104361. doi: 10.1016/j.jcv.2020.104361
28. Sun Y, Dong Y, Wang L, Xie H, Li B, Chang C, et al. Characteristics and prognostic factors of disease severity in patients with COVID-19: The Beijing experience. J Autoimmun. (2020) 112:102473. doi: 10.1016/j.jaut.2020.102473
29. Liu J, Li S, Liu J, Liang B, Wang X, Wang H, et al. Longitudinal characteristics of lymphocyte responses and cytokine profiles in the peripheral blood of SARS-CoV-2 infected patients. EBioMedicine. (2020) 55:102763. doi: 10.1016/j.ebiom.2020.102763
30. Liu Y, Liao W, Wan L, Xiang T, Zhang W. Correlation between relative nasopharyngeal virus RNA load and lymphocyte count disease severity in patients with COVID-19. Viral Immunol. (2021) 34:330–5. doi: 10.1089/vim.2020.0062
31. Fu J, Kong J, Wang W, Wu M, Yao L, Wang Z, et al. The clinical implication of dynamic neutrophil to lymphocyte ratio and D-dimer in COVID-19: A retrospective study in Suzhou China. Thromb Res. (2020) 192:3–8. doi: 10.1016/j.thromres.2020.05.006
32. Shi H, Wang W, Yin J, Ouyang Y, Pang L, Feng Y, et al. The inhibition of IL-2/IL-2R gives rise to CD8+ T cell and lymphocyte decrease through JAK1-STAT5 in critical patients with COVID-19 pneumonia. Cell Death Dis. (2020) 11:429. doi: 10.1038/s41419-020-2636-4
33. Yang AP, Li HM, Tao WQ, Yang XJ, Wang M, Yang WJ, et al. Infection with SARS-CoV-2 causes abnormal laboratory results of multiple organs in patients. Aging. (2020) 12:10059–69. doi: 10.18632/aging.103255
34. Wan S, Yi Q, Fan S, Lv J, Zhang X, Guo L, et al. Relationships among lymphocyte subsets, cytokines, and the pulmonary inflammation index in coronavirus (COVID-19) infected patients. Br J Haematol. (2020) 189:428–37. doi: 10.1111/bjh.16659
35. Liu R, Wang Y, Li J, Han H, Xia Z, Liu F, et al. Decreased T cell populations contribute to the increased severity of COVID-19. Clin Chim Acta. (2020) 508:110–4. doi: 10.1016/j.cca.2020.05.019
36. Zhu Z, Cai T, Fan L, Lou K, Hua X, Huang Z, et al. Clinical value of immune-inflammatory parameters to assess the severity of coronavirus disease 2019. Int J Infect Dis. (2020) 95:332–9. doi: 10.1016/j.ijid.2020.04.041
37. Sun DW, Zhang D, Tian RH, Li Y, Wang YS, Cao J, et al. The underlying changes and predicting role of peripheral blood inflammatory cells in severe COVID-19 patients: a sentinel?. Clinica chimica acta Int J Clin Chem. (2020) 508:122–9. doi: 10.1016/j.cca.2020.05.027
38. Chen R, Sang L, Jiang M, Yang Z, Jia N, Fu W, et al. Longitudinal hematologic and immunologic variations associated with the progression of COVID-19 patients in China. J Allergy Clin Immunol. (2020) 146:89–100. doi: 10.1016/j.jaci.2020.05.003
39. d'Alessandro M, Cameli P, Refini RM, Bergantini L, et al. Serum KL-6 concentrations as a novel biomarker of severe COVID-19. J Med Virol. (2020) 92:2216–20. doi: 10.1002/jmv.26087
40. Chi Y, Ge Y, Wu B, Zhang W, Wu T, Wen T, et al. Serum cytokine and chemokine profile in relation to the severity of coronavirus disease 2019 in China. J Infect Dis. (2020) 222:746–54. doi: 10.1093/infdis/jiaa363
41. Xu B, Fan CY, Wang AL, Zou YL, Yu YH, He C, et al. Suppressed T cell-mediated immunity in patients with COVID-19: a clinical retrospective study in Wuhan, China. J Infect. (2020) 81:e51–60. doi: 10.1016/j.jinf.2020.04.012
42. Ni M, Tian FB, Xiang DD, Yu B. Characteristics of inflammatory factors and lymphocyte subsets in patients with severe COVID-19. J Med Virol. (2020) 92:2600–6. doi: 10.1002/jmv.26070
43. Yang, Y., Shen, C., Li, J., Yuan, J., Wei, J., Huang, F., et al. Plasma IP-10 and MCP-3 levels are highly associated with disease severity and predict the progression of COVID-19. J Aller Clin Immunol. (2020) 146:119–27. doi: 10.1016/j.jaci.2020.04.027
44. Wang H, Yan D, Li Y, Gong Y, Mai Y, Li B, et al. Clinical and antibody characteristics reveal diverse signatures of severe and non-severe SARS-CoV-2 patients. Infectious Dis Poverty. (2022) 11:15. doi: 10.1186/s40249-022-00940-w
45. Yang P, Ding Y, Xu Z, Pu R, Li P, Yan J, et al. Epidemiological and clinical features of COVID-19 patients with and without pneumonia in Beijing, China. Preprints. (2020). doi: 10.1101/2020.02.28.20028068
46. Zumla A, Hui DS, Azhar EI, Memish ZA, Maeurer M. Reducing mortality from 2019-nCoV: host-directed therapies should be an option. Lancet (London, England). (2020) 395:e35–6. doi: 10.1016/S0140-6736(20)30305-6
47. National Health Health Commission of the people's Republic of China. (2020). Diagnosis and Treatment of Pneumonia of New Coronavirus Infection (Trial Version 5). Available online at: http://www.nhc.gov.cn/jkj/s3578/202002/dc7f3a7326e249c0bad0155960094b0b.shtml (accessed February 21, 2020).
48. Siddiqi HK, Mehra MR. COVID-19 illness in native and immunosuppressed states: a clinical-therapeutic staging proposal. J Heart Lung Transplant. (2020) 39:405–7. doi: 10.1016/j.healun.2020.03.012
49. Henry BM. COVID-19, ECMO, and lymphopenia: a word of caution. The Lancet. Resp Med. (2020) 8: e24. doi: 10.1016/S2213-2600(20)30119-3
Keywords: COVID-19, D-dimer, lymphocyte subsets, cytokine, meta-analysis
Citation: Zhang H, Wu H, Pan D and Shen W (2022) D-dimer levels and characteristics of lymphocyte subsets, cytokine profiles in peripheral blood of patients with severe COVID-19: A systematic review and meta-analysis. Front. Med. 9:988666. doi: 10.3389/fmed.2022.988666
Received: 07 July 2022; Accepted: 12 September 2022;
Published: 05 October 2022.
Edited by:
Reza Lashgari, Shahid Beheshti University, IranReviewed by:
Ufuk Oguz Idiz, Istanbul Egitim ve Araştirma Hastanesi, TurkeyFrancisco Fanjul, Hospital Universitario Son Espases, Spain
Copyright © 2022 Zhang, Wu, Pan and Shen. This is an open-access article distributed under the terms of the Creative Commons Attribution License (CC BY). The use, distribution or reproduction in other forums is permitted, provided the original author(s) and the copyright owner(s) are credited and that the original publication in this journal is cited, in accordance with accepted academic practice. No use, distribution or reproduction is permitted which does not comply with these terms.
*Correspondence: Weifeng Shen, anl6aHk1MjZAMTYzLmNvbQ==