- 1Department of Nursing, Sir Run Run Shaw Hospital, Zhejiang University School of Medicine, Hangzhou, China
- 2Deepwise Artificial Intelligence Laboratory, Hangzhou, China
- 3Department of Pathology, Sir Run Run Shaw Hospital, Zhejiang University School of Medicine, Hangzhou, China
- 4Department of General Surgery, Sir Run Run Shaw Hospital, Zhejiang University School of Medicine, Hangzhou, China
- 5Faculty of Engineering, The University of Hong Kong, Hong Kong, China
Objective: Post-hepatectomy liver failure (PHLF) remains clinical challenges after major hepatectomy. The aim of this study was to establish and validate a deep learning model to predict PHLF after hemihepatectomy using preoperative contrast-enhancedcomputed tomography with three phases (Non-contrast, arterial phase and venous phase).
Methods: 265 patients undergoing hemihepatectomy in Sir Run Run Shaw Hospital were enrolled in this study. The primary endpoint was PHLF, according to the International Study Group of Liver Surgery’s definition. In this study, to evaluate the proposed method, 5-fold cross-validation technique was used. The dataset was split into 5 folds of equal size, and each fold was used as a test set once, while the other folds were temporarily combined to form a training set. Performance metrics on the test set were then calculated and stored. At the end of the 5-fold cross-validation run, the accuracy, precision, sensitivity and specificity for predicting PHLF with the deep learning model and the area under receiver operating characteristic curve (AUC) were calculated.
Results: Of the 265 patients, 170 patients with left liver resection and 95 patients with right liver resection. The diagnosis had 6 types: hepatocellular carcinoma, intrahepatic cholangiocarcinoma, liver metastases, benign tumor, hepatolithiasis, and other liver diseases. Laparoscopic liver resection was performed in 187 patients. The accuracy of prediction was 84.15%. The AUC was 0.7927. In 170 left hemihepatectomy cases, the accuracy was 89.41% (152/170), and the AUC was 82.72%. The accuracy was 77.47% (141/182) with liver mass, 78.33% (47/60) with liver cirrhosis and 80.46% (70/87) with viral hepatitis.
Conclusion: The deep learning model showed excellent performance in prediction of PHLF and could be useful for identifying high-risk patients to modify the treatment planning.
1. Introduction
Liver resections are performed for both benign and malignant liver diseases. Hemihepatectomy is one type of major liver resection for the treatment of liver disease (1–3). Posthepatectomy liver failure (PHLF) is the most worrisome complication after major hepatectomy and is the leading cause of postoperative mortality (4–8). Previous reports have shown that the incidence of PHLF after liver resection varies and ranges from 0.7 to 39.6% (9, 10). Moreover, PHLF is the major cause of prolonged hospitalization, increased costs, and poor long-term outcomes in patients undergoing hepatectomy. In 2011, the International Study Group of Liver Surgery (ISGLS) proposed a standardized definition and severity grading for PHLF. The grade A, B, and C definitions are associated with mortality rates of 0, 12 and 54%, respectively, (7, 11).
The prediction of PHLF before hemihepatectomy should be a major concern for hepatobiliary surgeons and patients. A tool to accurately predict the risk for PHLF preoperatively will assist with patient selection and earlier intervention to potential PHLF patients. Currently, there are several predictors of PHLF reported, such as indocyanine green (ICG) clearance (12, 13), “50–50 Criteria” (4, 14), model for end-stage liver disease (MELD) system (15), Child-Pugh grade (16) and Future liver remnant (FLR) volume (17, 18). In addition, multivariable models are created to predict the risk of PHLF. But, there is still no standard model for clinical application due to limitations of each current models.
Deep learning with convolutional neural networks (CNNs) has been proven to have clinical significance in various medical image interpretation tasks, such as identifying and grading diabetic retinopathy, classifying skin lesions, classifying liver masses as benign or malignant and grading breast nodules based on BI-RADS, with accuracy comparable to experts (19–23). Recently, a research showed that deep learning model, based on medical data, could be used for preoperative prediction of severe liver failure after hemihepatectomy in patients with hepatocellular carcinoma (24). But there was no study on deep learning for preoperative prediction of PHLF with liver images.
Contrast-enhanced computed tomography (CT) is a common examination for the assessment of liver disease, because the vascularity and contrast agent enhancement patterns of liver lesions provide useful information for evaluation. A previous study reported that a nomogram combining CT image, serum albumin (Alb) and serum total bilirubin (Tbil) showed a good performance for PHLF preoperative prediction in patients with resectable HCC (25).
In this study, we aimed to investigate the prediction performance of deep learning model for PHLF after hemihepatectomy on preoperative contrast-enhanced CT images.
2. Methods
2.1. Patients
This retrospective study was approved by the Institutional Review Board of Sir Run Run Shaw Hospital (SRRSH). Informed consent was waived.
Between January 2017 and December 2021, consecutive patients who underwent hemihepatectomy at SRRSH were reviewed retrospectively. A total of 266 patients who met the inclusion criteria were enrolled. The inclusion criteria were as follows: (1) patients who underwent hemihepatectomy, (2) Patients above the age of 14, and (3) patients who underwent contrast-enhanced CT and serum liver function and coagulating function testing within 1 week before operation. The exclusion criteria were as follows: (1) patients with any antitumor therapy before surgery and (2) patients who had minor liver resections or more than hemihepatectomy.
2.2. Data collection and imaging quality control
Preoperative data were collected, including age, gender, the presence of viral hepatitis and liver cirrhosis, grade of Child-Pugh, pathological diagnosis, American Society of Anesthesiologists Score (ASA score) and preoperative contrast-enhanced CT image. Perioperative laboratory data were recorded, including total bilirubin (Tbil), international normalized ratio (INR) before operation and on or after postoperative day 5 (POD 5). Intraoperative variables, related to postoperative morbidity, were also collected. Blood loss was recorded as binary classification (≥ 400 or less). Besides, surgical approach (laparoscopy or laparotomy), extent of resection (left or right) and operation time were used in this study.
2.3. CT image
Preoperative contrast-enhanced CT images with three phases (Non-contrast, arterial phase and venous phase) were used for this model.
CT scans were performed from three manufacturers: Siemens, General Electric (GE) and United Imaging Healthcare (UIH).
The CT scans were acquired using a slice collimation of 5/7 mm, a matrix of 512 × 512 pixels, and an in-plane resolution of 0.516–0.975 mm. Each multi-phase CT image consists of three phases before and after the injection of contrast agent. A Non-Contrast scan is performed before injecting the contrast agent. The post-injection phases include the arterial phase (25–40 s after the injection) and the portal venous phase (60–80 s after the injection). For each patient, all slices containing lesions were used to construct the image dataset.
2.4. Outcomes
The primary outcome was PHLF, as defined by the International Study Group of Liver Surgery (ISGLS) as an increased INR (or need of clotting factors to maintain normal INR) and hyperbilirubinemia (according to the normal cut-off levels defined by the local laboratory) on or after postoperative day 5 (POD 5) (7).
2.5. Deep learning model development
2.5.1. Data pre-processing
In this study, we first extracted liver regions from input liver CT images using via an in-house trained liver segmentation model (Figure 1). This process can be replaced by manual region of interest (ROI) cropping using commercial image annotation tools. Then, the extracted image patches were resized to 16 × 128 × 128 due to different sizes of liver regions in the images. Finally, during training, we randomly cropped 12 × 112 × 112 from the resized image patches and mirrored the crop images to augment the data.
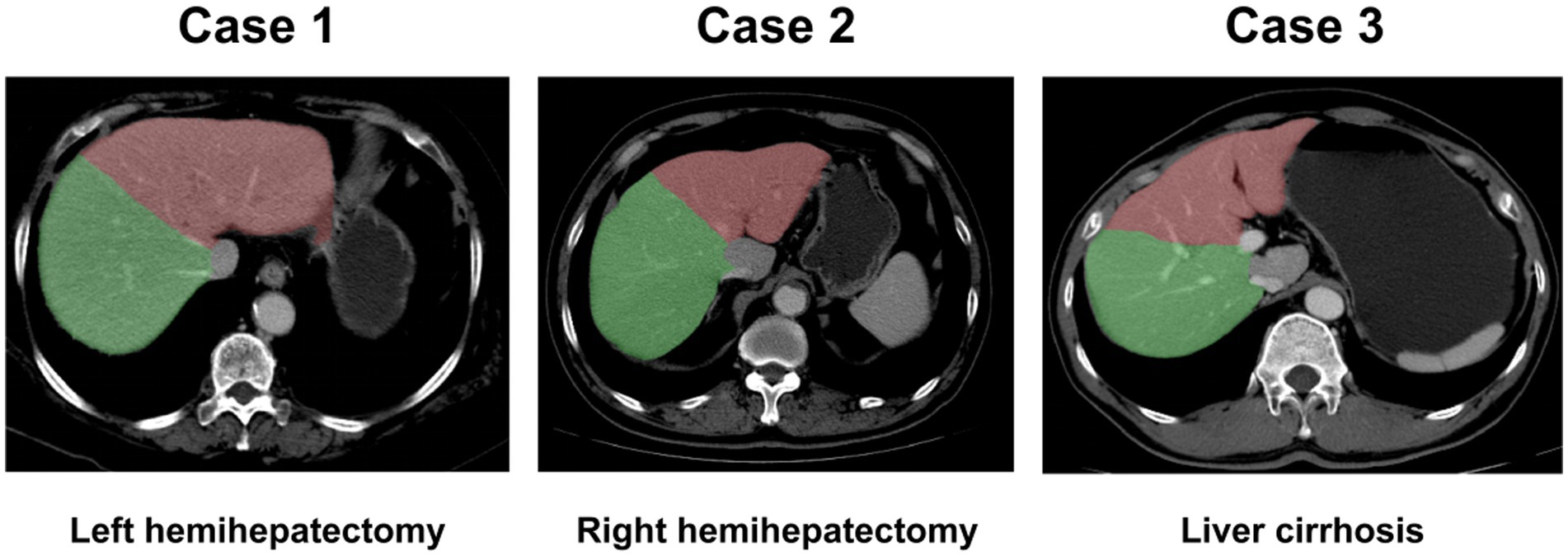
Figure 1. Liver segmentation images. Liver could be segmented automatically. Case 1: left hemihepatectomy; Case 2: right hemihepatectomy; Case 3: liver with cirrhosis. Green region: right liver lobe; Red region: left liver lobe.
2.5.2. Model architecture framework
For each liver CT examination, there were three phases, including non-contrast phase, arterial phase, and venous phase. To leverage the multi-phase information, we developed a three-phase model framework that utilized the non-contrast, arterial and venous phases of the liver region as inputs. The framework consisted of three stages. The first stage was a feature extraction backbone, which aimed to generate feature maps for the liver region of each of the three-phases. In the second stage, phase-interaction feature fusion was performed by obtaining correlation features between different pairs of phases through the Hadamard product operation. The inputs for this stage were three feature maps with dimensions of 92(C) × 12(D) × 112(H) × 112(W). The resulting correlation feature maps were then passed through 3D average pooling layers, resulting in three one-dimensional feature vector of size 92. In the third stage, the pooled feature vectors were concatenated into a vector of size 276, which was then connected to a fully connected layer to obtain the final classification result, indicating whether the patient had liver failure or not. The overall pipeline of the proposed model framework is illustrated in Figure 2A. It is worth mentioning that precise registration of the three-phase 3D images of the liver region is not necessary.
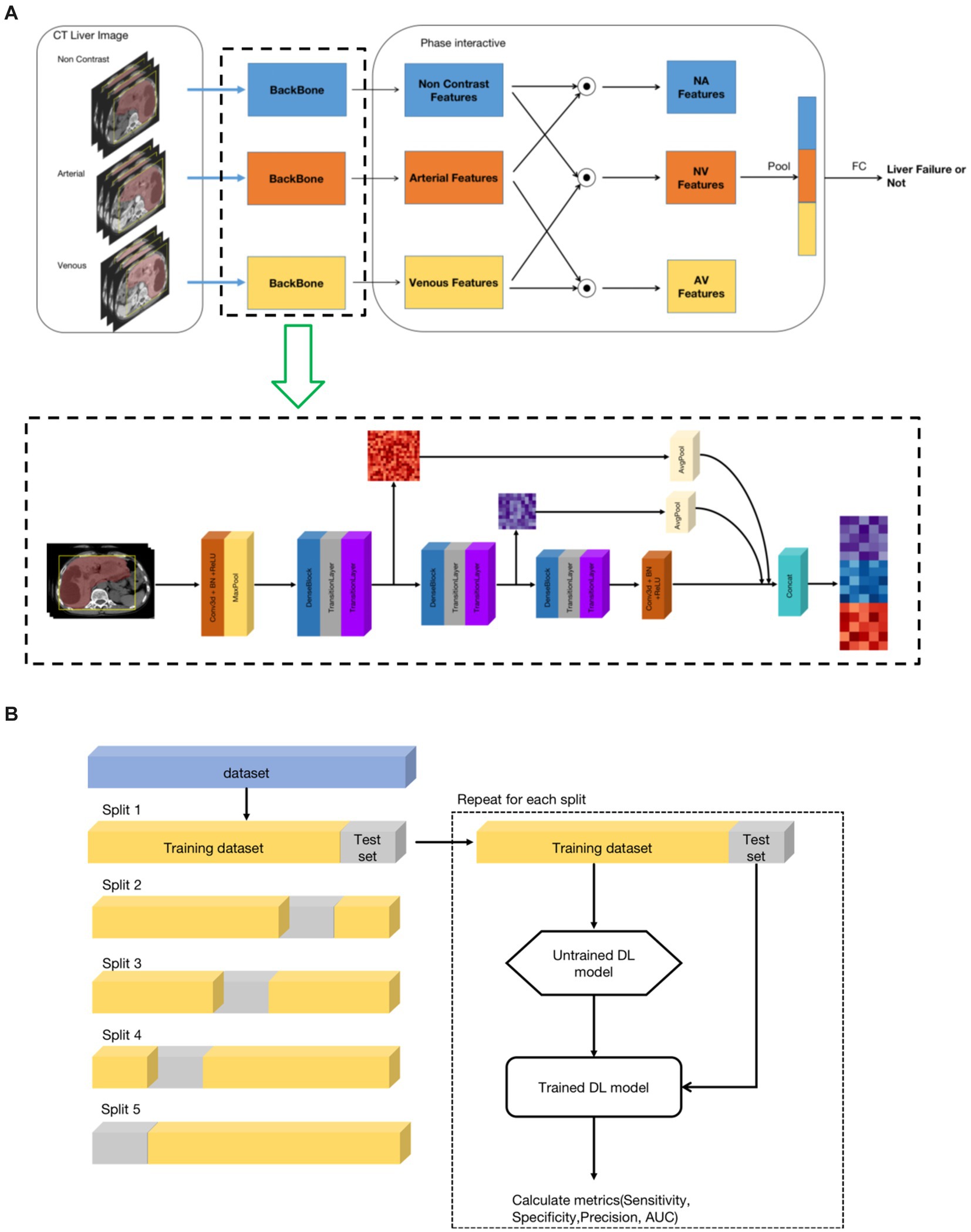
Figure 2. The architecture of the deep learning model. (A) Model architecture framework and implementation details. (B) Training and validation of the models. A 5-fold cross-validation technique was used.
2.5.3. Model implementation details
As shown in Figure 2A, the feature extraction backbone of the first stage of the framework was based on 3D convolution layers. It was composed of three similar structures, consisting of one dense neural network block, one transition layer, and one SE layer. The dense neural network block origins from the DenseNet (26), inspired by the ResNet (27), which bypassed information from one layer to the next layer via identity connections. The DenseNet architecture distilled shortcut insights into a simple connectivity pattern: ensuring maximum information flow between layers within layers. In this mode, each layer obtained additional inputs from preceding layers and passed its own output to subsequent layers. The DenseNet improved information flow, alleviated the gradient-vanishing problem, enhanced feature reuse, and substantially reduced the number of parameters. The transition layers consisted of 1*1*1 3D convolution and max-pool layer, which reduced the feature map, including reducing the number of feature channels and the size of the feature map. The SE layer used the Squeeze-and-Excitation (SE) block (28), which explicitly modeled the inter-dependencies between channels. Using SE blocks, the network learned to selectively strengthen discriminative features and suppress less informative ones. Finally, the feature map outputs of the three sub-structures of the first stage were concatenated by average pooling to combine high-level and low-level features, thereby improving the feature representation.
2.5.4. Training and validation of the models
In this study, to evaluate the proposed method, we used a 5-fold cross-validation technique. In detail, the dataset was split into 5 folds of equal size, and each fold was used as a test set once, while the other folds were temporarily combined to form a training set. Performance metrics on the test set were then calculated and stored. The process was repeated for the number of folds that have been generated. In each iteration, a new model was trained and tested. At the end of the 5-fold cross-validation run, the collected metrics of the 5 generated DL models were summarized. Finally, the following metrics were calculated: sensitivity, specificity, precision and area under the curve receiver operator characteristic (AUC-ROC). The illustration of the 5-fold cross validation is show in Figure 2B.
2.6. Statistical analysis
AUC are created by varying the threshold of the predicted probability and plotting the true positive rate (sensitivity) against the false positive rate (1-specificity). Accuracy, precision, sensitivity, and specificity are also used to evaluate the performance of DL model. All statistical tests use two-tailed tests and p-values less than or equal to 0.05 will be considered statistically significant. Statistical analysis was conducted using Python version 3.7.6.
3. Results
3.1. Characteristics of patients
265 patients with liver CT examination who underwent hemihepatectomy from SRRSH were analyzed, including 170 patients with left liver and 95 patients with right liver. Mean age was 60 ± 13 years. Liver function was evaluated, with only 14 patients (5.3%) classified as Child-Pugh B. 87 patients had viral hepatitis, and 60 patients had liver cirrhosis. The diagnosis was divided into 6 types: 92 (34.7%) hepatocellular carcinoma, 58 (21.9%) intrahepatic cholangiocarcinoma, 5 (1.9%) liver metastases, 27 (10.2%) benign tumor, 78 (29.4%) hepatolithiasis and 5 (1.9%) other liver diseases. 78 (29.4%) patients received open liver resection, and 187 (70.6%) patients with laparoscopic liver resection. 83 (31.3%) patients had intraoperative blood loss more than 400 mL (Table 1). More data was showed in Supplementary Table S1.
3.2. Posthepatectomy liver failure
Of all the patients, 91 (34.3%) patients developed PHLF (grade A: n = 38; grade B: n = 45; grade C: n = 8, Table 1). There was no significant difference between PHLF group and no PHLF group on age, ASA, Child-Pugh score and surgical approach. Sex, Viral hepatitis, liver cirrhosis, diagnosis, operation time, blood loss, and extent of resection were associated with PHLF (Table 1, p < 0 0.050 for all).
3.3. Performance of the DL model on prediction of PHLF
The performance of the DL model on prediction of PHLF was good, with an accuracy value of 84.15% and an AUC value of 79.27% (Figure 3A). The sensitivity was 72.53% (66/91). The specificity was 90.23% (157/174). The precision was 79.52% (66/83) (Table 2).
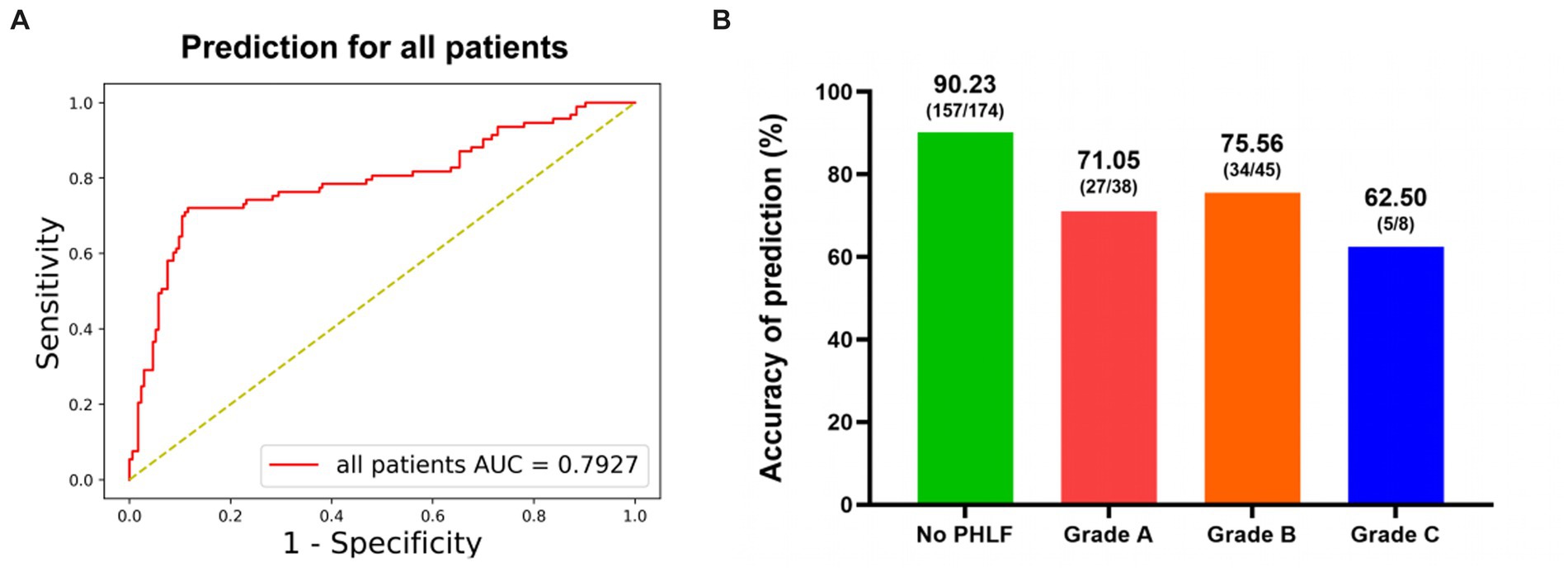
Figure 3. The performance of DL model for prediction of PHLF. (A) Receiver-operating characteristic curves and AUC of the predictive models of PHLF for all patients. (B) The accuracy for different PHLF grades.
The prediction performance of PHLF in different grades was showed in Figure 3B. The accuracy was 71.05% in PHLF Grade A. In patients with severe PHLF (Grade B and Grade C), the accuracy was 73.58% (Grade B: 75.56%, Grade C: 62.50%).
3.4. Subgroup analysis
3.4.1. Performance for different extent of resection (left or right hemihepatectomy)
In 170 left hemihepatectomy cases, the performance of the DL model was better than that in right hemihepatectomy cases (left vs. right: accuracy 89.41% (152/170)vs. 74.74% (71/95); AUC 82.72% vs. 74.03%) (Figures 4A,B). The sensitivity was 78.79% (26/33) inleft hemihepatectomy cases, and 68.97% (40/58) in right hemihepatectomy cases. The specificity was 91.97% (126/137) in the left, 83.78% (31/37) in the right. The precision was 70.27% (26/37) in the left, 86.96% (40/46) in the right.
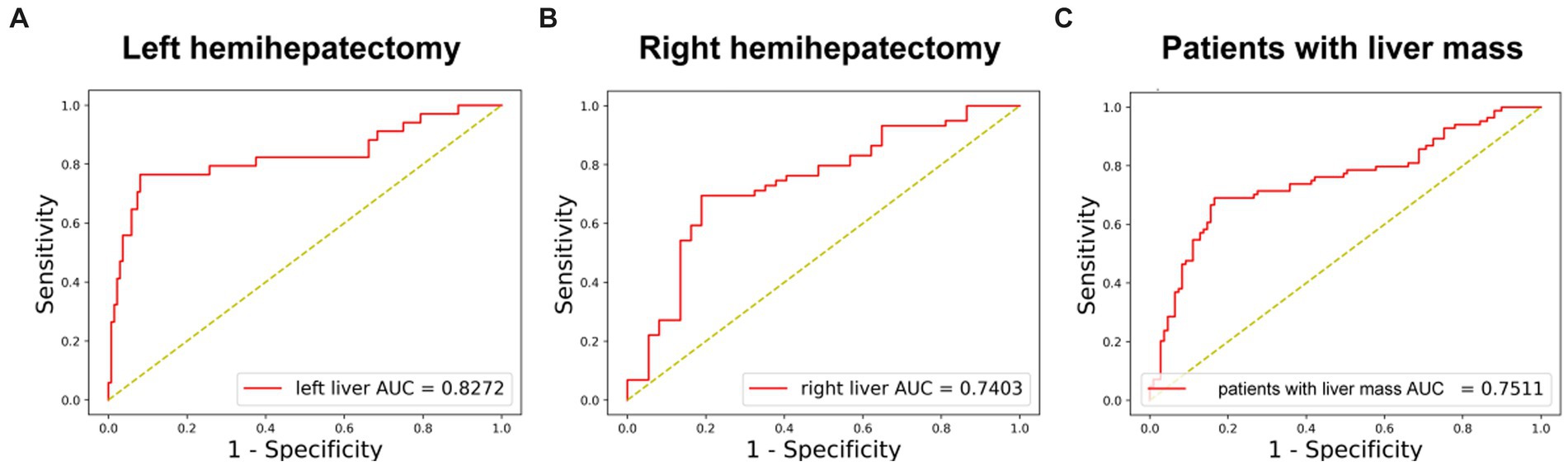
Figure 4. The performance of DL model in subgroups. (A) Receiver-operating characteristic curves and AUC of the predictive models of PHLF for left hemihepatectomy patients. (B) Receiver-operating characteristic curves and AUC of the predictive models of PHLF for right hemihepatectomy patients. (C) Receiver-operating characteristic curves and AUC of the predictive models of PHLF for patients with liver mass (HCC, ICC, liver metastasis or benign tumor).
3.4.2. Performance for patients with liver mass
In 182 patients with liver mass (hepatocellular carcinoma, intrahepatic cholangiocarcinoma, liver metastases and benign tumor), the accuracy was 77.47% (141/182), and AUC was 75.11% (Figure 4C).
3.4.3. Performance for patients with liver cirrhosis or viral hepatitis
The accuracy was 78.33% (47/60) in patients with liver cirrhosis and 80.46% (70/87) with viral hepatitis.
All the results were showed in Table 2.
4. Discussion
PHLF is responsible for more than 60% of mortalities after hepatectomy. There were several researches for development of PHLF predictors. Previous study reported that PLT count was related to postoperative liver regeneration, postoperative liver function recovery, and PHLF risk (29, 30). The“50–50 criteria,” an predictor of PHLF and mortality, predicts>50% mortality rate if prothrombin time < 50% and serum bilirubin ≥50 μmoL/L on POD 5 (4). Indocyanine green retention test at 15 min (ICG-R15) is another tool used to evaluate liver quality (12). Mathieu Prodeau et al. (6), reported an ordinal PHLF prediction model for patients with cirrhosis based on 3 variables (i.e., platelet count, RTLV and ITT laparoscopy). Yangling Peng et al. (25), showed a nomogram based on CT–derived extracellular volume for the prediction of PHLF in patients with HCC. Rong-yun Mai et al. (24), developed and validated a multivariate deep learning model based on baseline characteristics, laboratory indicators and surgical situation for predicting the risk of severe PHLF in patients with HCC who underwent hemihepatectomy.
In this study, we first investigated whether PHLF could be predicted by deep learning model based on preoperative enhanced CT. We successfully established a deep learning model and found that it was useful to predict PHLF after hemihepatectomy (precision: 83.40%, AUC: 79.26%). Thus, our findings and the deep learning model may help to select patients who need hemihepatectomies and do a better preparation before operation. In addition, some preoperative characteristics including viral hepatitis, liver cirrhosis, diagnosis and extent of hemihepatectomy were important factors with PHLF. In patients with liver masses, the model showed a performance with AUC 75.10% and precision 77.47% (141/182), sensitivity 68.75% (55/80), specificity 84.31% (86/102). In China, there are many liver resection cases with chronic hepatitis or liver cirrhosis. So, we also evaluated the performance of this model in patients with hepatitis or liver cirrhosis and had a good performance with the precision (chronic hepatitis: 80.46% (70/87); liver cirrhosis: 78.33% (47/60)). In different extent of resection, the result showed that the predictive ability in left hemihepatectomy was better than that in right hemihepatectomy (precision: 89.41% vs. 72.63%; AUC: 82.72% vs. 74.03%). These results suggested that deep learning model could be useful for prediction of PHLF based on CT images, and multiple characteristics were related to the prediction performance of the model.
This model showed a good performance for prediction of PHLF after hemihepatectomy (precision: 83.40%), and it could help to improve the selection of patients with the best risk–benefit profiles for hemihepatectomy. The patients with high PHLF risk, which were selected by the model, could receive other options such as portal vein embolization (PVE), associating liver partition and portal vein ligation for staged hepatectomy (ALPPS), radiofrequency ablation (RFA) or transcatheter arterial chemoembolization (TACE) (31–33). Besides, the model could help surgeons modify the perioperative treatment plan for the high PHLF risk patients. Enhanced recovery after surgery (ERAS) and prehabilitation could be used for high risk patients who were selected by the model. Furthermore, the prediction data of the model could also help patients understand the risk–benefit before surgery, and be useful for preoperative conversation and seeking informed consent.
There are some limitations in our study. First, the more cases from different centers were needed. Second, the precision and AUC in right hemihepatectomy were not as good as that in left hemihepatectomy. Possible reasons included small number of right hemihepatectomy cases and more complicated surgical situation. Third, this study was not designed to predict other outcomes such as 30-day, 90-day or 1-year mortality. Forth, patients’ basic characteristics, laboratory indicators and surgical situation were not included in our model. Thus, multimodal algorithm based on more effective medical data, would be developed to achieve adequate performance. Fifth, a prospective and multicenter study is required to clarify the reliability and adaptability of the deep learning model.
In conclusion, this preliminary study obtains a deep learning model for prediction of PHLF (as defined by the International Study Group of Liver Surgery (ISGLS)), which can be accomplished with a high precision based on preoperative contrast-enhanced CT images. However, further study would be necessary to improve performance for prediction of PHLF.
Data availability statement
The original contributions presented in the study are included in the article/Supplementary material, further inquiries can be directed to the corresponding authors.
Ethics statement
Ethical review and approval was not required for the study on human participants in accordance with the local legislation and institutional requirements. Written informed consent from the participants was not required to participate in this study in accordance with the national legislation and the institutional requirements.
Author contributions
HY, YY, XL, and XX contributed to the study concept and design. HY, YR, XX, and YT contributed to acquisition of data. ZiX, SW, and XLiu contributed to analysis and interpretation of data. XX, HY, and XLiu contributed to writing, reviewing, and approval of the final version of this work. All authors contributed to the article and approved the submitted version.
Funding
This research was supported in part by National Key Research and Development Program of China (No. 2019YFC0118100), the Zhejiang Provincial Key Research & Development Program (No. 2020C03073), Medical Health Science and Technology Project of Zhejiang Provincial Health Commission (2020RC069).
Acknowledgments
The authors thank Xi Hu and Hui Liu for his assistance with data keeping, processing and statistical analysis.
Conflict of interest
The authors declare that the research was conducted in the absence of any commercial or financial relationships that could be construed as a potential conflict of interest.
Publisher’s note
All claims expressed in this article are solely those of the authors and do not necessarily represent those of their affiliated organizations, or those of the publisher, the editors and the reviewers. Any product that may be evaluated in this article, or claim that may be made by its manufacturer, is not guaranteed or endorsed by the publisher.
Supplementary material
The Supplementary material for this article can be found online at: https://www.frontiersin.org/articles/10.3389/fmed.2023.1154314/full#supplementary-material
References
1. Heimbach, JK, Kulik, LM, Finn, RS, Sirlin, CB, Abecassis, MM, Roberts, LR, et al. AASLD guidelines for the treatment of hepatocellular carcinoma. Hepatology. (2018) 67:358–80. doi: 10.1002/hep.29086
2. Omata, M, Cheng, AL, Kokudo, N, Kudo, M, Lee, JM, Jia, J, et al. Asia-Pacific clinical practice guidelines onthe management of hepatocellular carcinoma: a 2017 update. Hepatol Int. (2017) 11:317–70. doi: 10.1007/s12072-017-9799-9
3. European Association for the Study of the Liver. European Association for the Study of the Liver. EASL clinical practice guidelines: management of hepatocellular carcinoma. J Hepatol. (2018) 69:182–236. doi: 10.1016/j.jhep.2018.03.019
4. Balzan, S, Belghiti, J, Farges, O, Ogata, S, Sauvanet, A, Delefosse, D, et al. The "50–50 criteria" on postoperative day 5:an accurate predictor of liver failure and death after hepatectomy. Ann Surg. (2005) 242:824–9. doi: 10.1097/01.sla.0000189131.90876.9e
5. Poon, RT, Fan, ST, Lo, CM, Liu, CL, Lam, CM, Yuen, WK, et al. Improving perioperative outcome expands the role of hepatectomy in management of benign and malignant hepatobiliary diseases: analysis of 1222 consecutive patients from a prospective database. Ann Surg. (2004) 240:698–710. doi: 10.1097/01.sla.0000141195.66155.0c
6. Prodeau, M, Drumez, E, Duhamel, A, Vibert, E, Farges, O, Lassailly, G, et al. An ordinal model to predict the risk of symptomatic liver failure in patients with cirrhosis undergoing hepatectomy. J Hepatol. (2019) 71:920–9. doi: 10.1016/j.jhep.2019.06.003
7. Rahbari, NN, Garden, OJ, Padbury, R, Brooke-Smith, M, Crawford, M, Adam, R, et al. Posthepatectomy liver failure: a definition and grading by the International Study Group of Liver Surgery(ISGLS). Surgery. (2011) 149:713–24. doi: 10.1016/j.surg.2010.10.001
8. Wei, AC, Tung-Ping, PR, Fan, ST, and Wong, J. Risk factors for perioperative morbidity and mortality after extended hepatectomy for hepatocellular carcinoma. Br J Surg. (2003) 90:33–41. doi: 10.1002/bjs.4018
9. Soreide, JA, and Deshpande, R. Post hepatectomy liver failure (PHLF) – recent advances in prevention and clinical management. Eur J Surg Oncol. (2021) 47:216–24. doi: 10.1016/j.ejso.2020.09.001
10. Tsujita, Y, Sofue, K, Komatsu, S, Yamaguchi, T, Ueshima, E, Ueno, Y, et al. Prediction of posthepatectomy liver failure using gadoxetic acid-enhanced magnetic resonance imaging for hepatocellular carcinoma with portal vein invasion. Eur J Radiol. (2020) 130:109189–9. doi: 10.1016/j.ejrad.2020.109189
11. Reissfelder, C, Rahbari, NN, Koch, M, Kofler, B, Sutedja, N, Elbers, H, et al. Postoperative course and clinical significance of biochemical blood tests following hepatic resection. Br J Surg. (2011) 98:836–44. doi: 10.1002/bjs.7459
12. Pind, ML, Møller, S, Faqir, N, and Bendtsen, F. Predictive value of indocyanine green retention test and indocyanine green clearance in child-Pugh class a patients. Hepatology. (2015) 61:2112–3. doi: 10.1002/hep.27569
13. Kudo, M, Matsui, O, Izumi, N, Iijima, H, Kadoya, M, Imai, Y, et al. JSH consensus-based clinical practice guidelines for the Management of Hepatocellular Carcinoma: 2014 update by the liver Cancer study Group of Japan. Liver Cancer. (2014) 3:458–68. doi: 10.1159/000343875
14. Rahbari, NN, Reissfelder, C, Koch, M, Elbers, H, Striebel, F, Büchler, MW, et al. The predictive value of postoperative clinical risk scores for outcome after hepatic resection: a validation analysis in 807 patients. Ann Surg Oncol. (2011) 18:3640–9. doi: 10.1245/s10434-011-1829-6
15. Malinchoc, M, Kamath, PS, Gordon, FD, Peine, CJ, Rank, J, and ter Borg, PC. A model to predict poor survival in patients undergoing transjugular intrahepatic portosystemic shunts. Hepatology. (2000) 31:864–71. doi: 10.1053/he.2000.5852
16. Durand, F, and Valla, D. Assessment of the prognosis of cirrhosis: child-Pugh versus MELD. J Hepatol. (2005) 42:S100–7. doi: 10.1016/j.jhep.2004.11.015
17. Simpson, AL, Geller, DA, Hemming, AW, Jarnagin, WR, Clements, LW, D’Angelica, MI, et al. Liver planning software accurately predicts postoperative liver volume and measures early regeneration. J Am Coll Surg. (2014) 219:199–207. doi: 10.1016/j.jamcollsurg.2014.02.027
18. Wigmore, SJ, Redhead, DN, Yan, XJ, Casey, J, Madhavan, K, Dejong, CHC, et al. Virtual hepatic resection using three dimensional reconstruction of helical computed tomography angioportograms. Ann Surg. (2001) 233:221–6. doi: 10.1097/00000658-200102000-00011
19. Ozdemir, O, Russell, R, and Berlin, A. A 3D probabilistic deep learning system for detection and diagnosis of lung Cancer using low-dose CT scans. IEEE Trans Med Imaging. (2020) 39:1419–29. doi: 10.1109/TMI.2019.2947595
20. Xi, I, Zhao, Y, Wang, R, Chang, M, Purkayastha, S, Chang, K, et al. Deep learning to distinguish benign from malignant renal lesions based on routine MR imaging. Clin Cancer Res. (2020) 26:1944–52. doi: 10.1158/1078-0432.CCR-19-0374
21. Aboutalib, S, Mohamed, A, Berg, W, Zuley, ML, Sumkin, JH, and Wu, S. Deep learning to distinguish recalled but benign mammography images in breast Cancer screening. Clin Cancer Res. (2018) 24:5902–9. doi: 10.1158/1078-0432.CCR-18-1115
22. Gulshan, V, Peng, L, Coram, M, Stumpe, MC, Wu, D, Narayanaswamy, A, et al. Development and validation of a deep learning algorithm for detection of diabetic retinopathy in retinal fundus photographs. JAMA. (2016) 316:2402–10. doi: 10.1001/jama.2016.17216
23. Esteva, A, Kuprel, B, Novoa, R, Ko, J, Swetter, SM, Blau, HM, et al. Dermatologist-level classification of skin cancer with deep neural networks. Nature. (2017) 542:115–8. doi: 10.1038/nature21056
24. Mai, R-y, Lu, HZ, Bai, T, Liang, R, Lin, Y, Ma, L, et al. Artificial neural network model for preoperative prediction of severe liver failure after hemihepatectomy in patients with hepatocellular carcinoma. Surgery. (2020) 168:643–52. doi: 10.1016/j.surg.2020.06.031
25. Peng, Y, Shen, H, Tang, H, Huang, Y, Lan, X, Luo, X, et al. Nomogram based on CT–derived extracellular volume for the prediction of post-hepatectomy liver failure in patients with resectable hepatocellular carcinoma. Eur Radiol. (2022) 32:8529–39. doi: 10.1007/s00330-022-08917-x
26. Huang, G, Liu, Z, Van Der Maaten, L, et al. (2017). Densely connected convolutional networks. Proceedings of the IEEE conference on computer vision and pattern recognition. 4700–4708.
27. He, K, Zhang, X, Ren, S, et al. (2016). Deep residual learning for image recognition. Proceedings of the IEEE conference on computer vision and pattern recognition. 770–778.
28. Hu, J, Shen, L, and Sun, G. (2018). Squeeze-and-excitation networks. Proceedings of the IEEE conference on computer vision and pattern recognition. 7132–7141.
29. Cichoż-Lach, H, Celiński, K, Prozorow-Król, B, Swatek, J, Słomka, M, and Lach, T. The BARD score and the NAFLD fibrosis score in the assessment of advanced liver fibrosis in nonalcoholic fatty liver disease. Med Sci Monit. (2012) 18:CR735–40. doi: 10.12659/MSM.883601
30. Tomimaru, Y, Eguchi, H, Gotoh, K, Kawamoto, K, Wada, H, Asaoka, T, et al. Platelet count is more useful for predicting posthepatectomy liver failure at surgery for hepatocellular carcinoma than indocyanine green clearance test. J Surg Oncol. (2016) 113:565–9. doi: 10.1002/jso.24166
31. Chan, A, Zhang, WY, Chok, K, Dai, J, Ji, R, Kwan, C, et al. ALPPS versus portal vein embolization for hepatitis-related hepatocellular carcinoma: a changing paradigm in modulation of future liver remnant before major hepatectomy. Ann Surg. (2021) 273:957–65. doi: 10.1097/SLA.0000000000003433
32. Clavien, PA, and Lillemoe, KD. Associating liver partition and portal vein ligation for staged hepatectomy. Ann Surg. (2016) 263:835–6. doi: 10.1097/SLA.0000000000001534
Keywords: deep learning, hemihepatectomy, liver failure, prediction model, contrast – enhanced CT
Citation: Xu X, Xing Z, Xu Z, Tong Y, Wang S, Liu X, Ren Y, Liang X, Yu Y and Ying H (2023) A deep learning model for prediction of post hepatectomy liver failure after hemihepatectomy using preoperative contrast-enhanced computed tomography: a retrospective study. Front. Med. 10:1154314. doi: 10.3389/fmed.2023.1154314
Edited by:
Jagannath M., Vellore Institute of Technology (VIT), IndiaReviewed by:
Vishal G. Shelat, Tan Tock Seng Hospital, SingaporeYun Long, Peking Union Medical College Hospital (CAMS), China
Shouliang Qi, Northeastern University, China
Copyright © 2023 Xu, Xing, Xu, Tong, Wang, Liu, Ren, Liang, Yu and Ying. This is an open-access article distributed under the terms of the Creative Commons Attribution License (CC BY). The use, distribution or reproduction in other forums is permitted, provided the original author(s) and the copyright owner(s) are credited and that the original publication in this journal is cited, in accordance with accepted academic practice. No use, distribution or reproduction is permitted which does not comply with these terms.
*Correspondence: Hanning Ying, aGVucnlfeWluZ2huQHpqdS5lZHUuY24=; Xiao Liang, c3Jyc2hseEB6anUuZWR1LmNu; Yizhou Yu, eWl6aG91eUBhY20ub3Jn
†These authors have contributed equally to this work