Gut microbiota and atopic dermatitis: a two-sample Mendelian randomization study
- 1The Third People’s Hospital of Chengdu, Clinical College of Southwest Jiao Tong University, Chengdu, China
- 2School of Basic Medical Sciences, Chengdu University of Traditional Chinese Medicine, Chengdu, China
- 3North Sichuan Medical College, Nanchong, Sichuan, China
Background: Accumulating evidence suggests that alterations in gut microbiota composition and diversity are associated with Atopic dermatitis (AD). But until now, the causal association between them has been unclear.
Methods: We employed a two-sample Mendelian Randomization (MR) study to estimate the potential causality of gut microbiota on AD risk. The summary statistics related to the gut microbiota were obtained from a large-scale genome-wide genotype and 16S fecal microbiome dataset from 18,340 individuals (24 cohorts) analyzed by the MiBioGen Consortium, comprising 211 gut microbiota. AD data were also derived from strictly defined AD data collected by FinnGen biobank analysis, which included 218,467 European ancestors (5,321 AD patients and 213,146 controls). The inverse variance weighted method (IVW), weighted median (WME), and MR-Egger were used to determine the changes of AD pathogenic bacterial taxa, followed by sensitivity analysis including horizontal pleiotropy analysis, Cochran’s Q test, and the leave-one-out method to assess the reliability of the results. In addition, MR Steiger’s test was used to test the suppositional relationship between exposure and outcome.
Results: A total of 2,289 SNPs (p < 1 × 10−5) were included, including 5 taxa and 17 bacterial characteristics (1 phylum, 3 classes, 1 order, 4 families, and 8 genera), after excluding the IVs with linkage disequilibrium (LD). Combining the analysis of the results of the IVW models, there were 6 biological taxa (2 families, and 4 genera) of the intestinal flora positively associated with the risk of AD and 7 biological taxa (1 phylum, 2 classes, 1 order, 1 family, and 2 genera) of the intestinal flora negatively associated. The IVW analysis results showed that Tenericutes, Mollicutes, Clostridia, Bifidobacteriaceae, Bifidobacteriales, Bifidobacterium, and Christensenellaceae R 7 group were negatively correlated with the risk of AD, while Clostridiaceae 1, Bacteroidaceae, Bacteroides, Anaerotruncus, the unknown genus, and Lachnospiraceae UCG001 showed the opposite trend. And the results of the sensitivity analysis were robust. MR Steiger’s test showed a potential causal relationship between the above intestinal flora and AD, but not vice versa.
Conclusion: The present MR analysis genetically suggests a causal relationship between changes in the abundance of the gut microbiota and AD risk, thus not only providing support for gut microecological therapy of AD but also laying the groundwork for further exploration of the mechanisms by which the gut microbiota contributes to the pathogenesis of AD.
1. Introduction
Atopic dermatitis (AD) (1, 2), also known as atopic eczema or hereditary allergic eczema, is a chronic inflammatory skin lesion characterized by dry, itchy skin as well as recurrent episodes. AD can occur at all ages (3), with the majority having an onset in infancy; some severe impairments can extend chronically into adulthood. Davies et al. (4) outlined 15 studies published in 2015 and found that the global prevalence of AD in childhood was 7.89% and that people with AD were four times more likely to have allergic rhinitis and asthma than the normal population. The WHO global burden of disease data suggests that at least 230 million people worldwide suffer from AD and that AD has jumped to become the fourth leading cause of non-fatal disease (5). Almost 20% of children in Western countries suffer from AD (6).
Multiple factors, including genetics, the environment, and immunity, influence AD (7), and its etiology and pathogenesis are unknown. Many researchers found that changes in intestinal flora abundance may be related to the occurrence and development of AD, but the specific relationship between the two remains unclear (8, 9). Early hypotheses were used to posit that excessive hygiene disrupts skin surface flora and thereby renders probiotics less protective for the host immune system. And then, with the rise of the “gut-skin axis “and the “gut-brain axis” (10, 11), accumulating evidence has proved that altered gut microbiota composition and diversity can affect skin immunity and metabolisms (12, 13), such as promoting cytokine responses and regulatory T cell differentiation (14). Besides, it also plays a key role in immune activation and tolerance. Short-chain fatty acids (SCFAs, such as propionate, and butyrate) bind to short-chain fatty receptors 2 (FFA2) and 3(FFA3) to induce Treg cells to regulate immune balance (15), and amino acid metabolites (tryptophan, kynurenic acid, etc.) can also play an anti-inflammatory role by binding to G-protein receptors (such as GPR142, GPR35) (16, 17).
In 2018, a systematic review of the literature on gut microbiota versus AD by Petersen (18) found that nearly half of the findings agreed that gut microbiota diversity, as well as the colonization of specific flora (such as Clostridium species, Lactobacillus paracasei, and Bifidobacterium), was inversely associated with AD risk, while the remaining studies suggested no clear association between the two based on PRISMA guidelines. A previous birth cohort study with a 16S ribosomal RNA gene sequencing approach revealed reduced gut microbiota diversity in AD patients (19). The scholar Abrahamsson also reached a similar conclusion (20) and showed that Bacteroidetes decreased at both phylum and genus levels in 1-month-old children with AD. Moreover, a study published by Nowrouzian and colleagues also found that intestinal colonization with Staphylococcus aureus (S. aureus) strains carrying a certain combination of superantigen and adhesin genes was not only negatively associated with the subsequent development of atopic eczema but also, to some extent, able to promote the maturation of the infant immune system (21). However, the potential causal relationship between gut microbiota and the risk of AD has not been clearly established.
Most observational studies have shown that patients with specific dermatitis often have intestinal flora disturbance, but this may only be a clinical symptom of specific dermatitis and cannot prove a causal relationship (observational studies may have differences in intestinal flora due to grouping requirements, sex ratio, ethnicity, etc.). Therefore, MR is adopted to eliminate the interference of confounding factors on the one hand and avoid the influence of reverse causality on the other hand, so as to make the study more rigorous and credible (22).
From a genetic perspective, Mendelian randomization (MR) utilizes the genetic law of random distribution of gamete alleles to analyze potential causal relationships between exposures and outcomes. MR requires a genetic variant that is robustly associated with exposure as an instrumental variable, and the genetic variants have and can only act on the outcome indicators by influencing the exposure factors, largely reducing the interference of confounding and reverse causal associations. In this study, the two-sample Mendelian randomization was used to analyze the potential causal relationship between the gut microbiota and AD, using the abundance of the gut microbiota as the exposure factor and the occurrence of AD as the outcome, and explore the relationship between them genetically.
2. Materials and methods
2.1. Study design
This study utilized gut microbiota abundance as an exposure factor, and single nucleotide polymorphisms (SNPs) screened separately under the comprehensive threshold (1 × 10−5) and the traditional threshold (5 × 10−8) were used as instrumental variables (IVs).The outcome measure was atopic dermatitis (AD). Causal analysis was performed using a two-sample MR analysis approach (Figure 1).
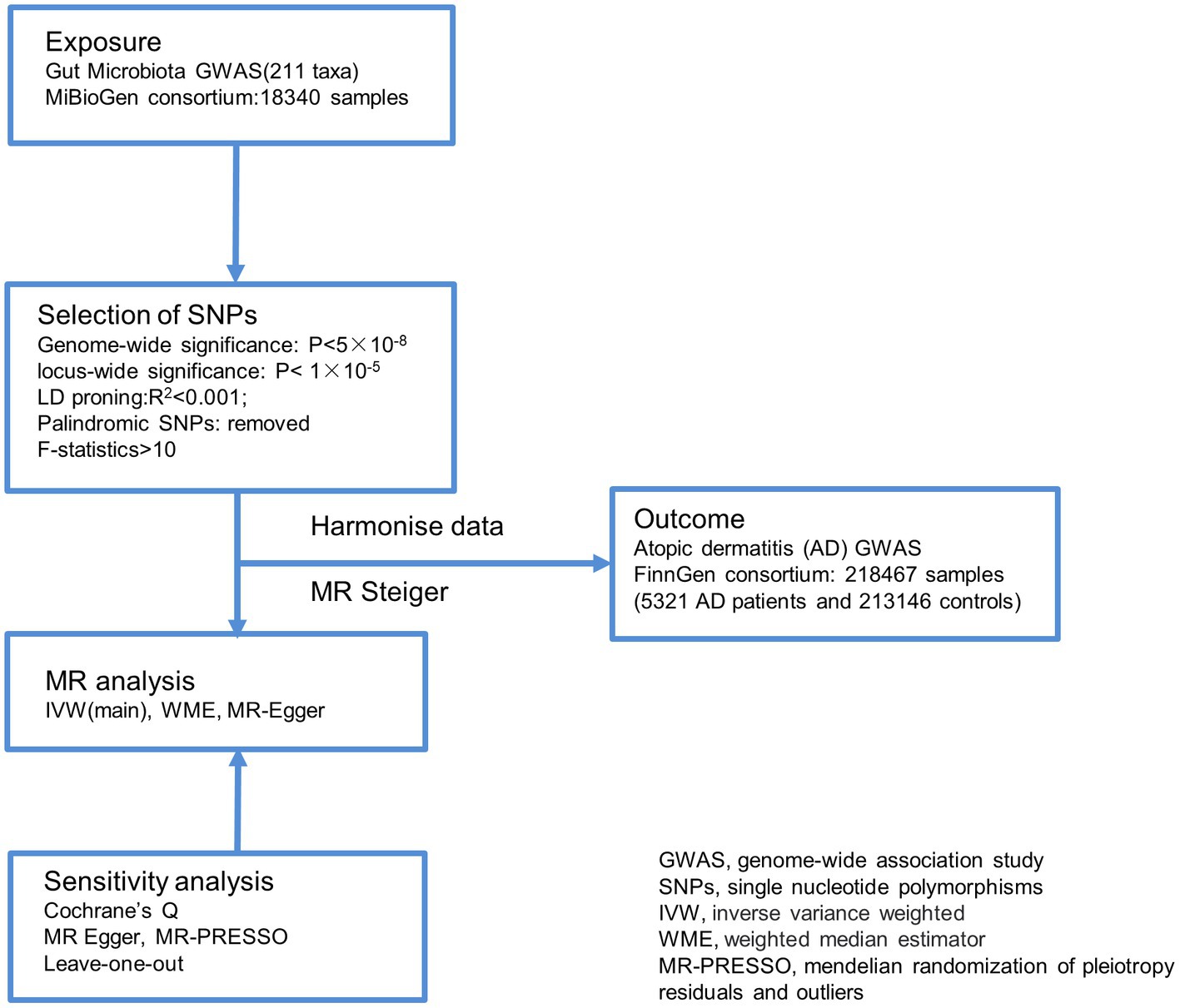
Figure 1. Overview of the analysis process of the causal relationship between the gut microbiome and Atopic dermatitis through MR analyzes. GWAS, genome-wide association study; SNPs, single nucleotide polymorphisms; IVW, inverse variance weighted; WME, weighted median estimator; MR-PRESSO, mendelian randomization of pleiotropy residuals and outliers.
2.2. Data sources
Both gut microbiota and AD data were obtained from genome-wide association study (GWAS) datasets. The intestinal microbiome data were derived from a large MiBioGen consortium GWAS analysis of 18,340 people, including 24 cohorts for whole-genome genotypes and 16S fecal microbiome data; the AD data were derived from strictly defined AD data collected by FinnGen biobank analysis, consisting of 5,321 AD patients and 213,146 controls (European descent).
2.3. Outcome measure
The current diagnostic criteria for AD are not uniform (23–25). This case refers to the Hanifin and Rajka diagnostic criteria (gold standard).
Hanifin and Rajka’s diagnostic criteria include four cardinal and 23 minor features (26). The basic characteristics are: 1. Itching; 2. Typical shape and distribution of the rash: children with facial and extensors involved; 3. Chronic or chronic recurrent dermatitis; 4. Personal or family history of atopic disease. The diagnosis of atopic dermatitis in children requires meeting any three of the basic characteristics plus any three of the secondary characteristics (Supplementary Table S1).
2.4. Instrumental variables
First, for the 1,000 Genomes project, we were originally going to choose SNPs that were significantly associated with gut microbiota (p < 5 × 10−8) of SNPs. If the number of screening is relatively small under the traditional threshold, a relaxation statistical threshold(p < 1 × 10−5) was commonly implemented in MR studies (27–30) to account for greater variation when few genome-wide significant SNPs were available for exposure (30–32). We set the threshold of R2 as 0.001, KB = 10,000 (clump step was performed using two-sample MR of R software to remove SNPs within 10 MB that were in LD with the most significant SNP with an R2 of more than 0.001 to exclude linkage disequilibrium effects). Second, based on the principle that the effects of the selected SNPs on exposure and outcome are due to the same alleles, palindromic SNPs that do not possess the A/T or C/G polymorphisms were excluded from IVs (33). The proportion of variation (R2) explained by SNPs in the exposure database was calculated by the following formula: R2 = 2 × β2 × (1-EAF) × EAF. In this formula, β identifies the estimated effect of the genetic variant and EAF represents the effect allele frequency, and then F = R2 (N-k-1)/k(1-R2) was calculated based on the sample size (N), the number of included SNPs (k), and R2 (34).When the F-statistic >10, then indicating the absence of instrumental variable bias [F for a single SNP equals β 2/SE2 (35)].
2.5. Mendelian randomization analysis
Inverse variance weighted (IVW), MR-Egger regression (36), weighted median estimator (WME) and Wald ratio was used for MR Analysis. The IVW method suggests that there is no level of pleiotropy, which avoids the influence of confounding factors to a certain extent and thus gets unbiased estimation (27, 37). The applicability of the MR-Egger hypothesis is strong, and it can withstand the polytropy of more than 50% SNP. However, WME was still applicable when the pleiotropy was less than 50%, which improved the accuracy of the results to some extent. In this study, IVW method was adopted as the primary causal effect estimation. IVW method is a relatively ideal estimation, which is an effective analysis under the basic premise that all genetic variations are valid instrumental variables, and has a strong ability to detect causality. Besides, another four methods were adopted to supplementary the results, namely MR-Egger regression, WME, simple modal-based estimation, and weighted modal-based estimation. It has been reported that, under certain conditions, the IVW method is more powerful and reliable than other methods (38). The Wald ratio (WR) method is often used when examining the effect of individual IV on causal estimation.
2.6. Horizontal pleiotropy and heterogeneity evaluation
Instrumental variables pleiotropy detection: the intercept term of MR-Egger regression tests for the presence of directional pleiotropy, if the intercept term egger intercept is close to zero, there is no pleiotropy for instrumental variables and vice versa. In addition, outliers can be detected for pleiotropy bias through Mendelian randomization of pleiotropy residuals and outliers (MR PRESSO) or by Cochran’s Q test to quantify the heterogeneity among the selected SNPs (p < 0.05 was considered as possible heterogeneity in IVs) (39). A leave-one-out sensitivity analysis was performed on the results by observing whether there was a statistical difference before and after removing each SNP one by one. If there is little change in the results before and after removing this SNP, which indicates that removing this SNP would maynot have a nonspecific effect on the effect estimate. MR Steiger test was used to determine the directivity of the impact of exposure on the outcome, and the result of “TRUE” predicts the association in the expected direction.The statistical treatments described above were carried out using R version 4.2.1 and implemented in the TwosampleMR package (version 0.5.6) with a 0.05 check level. Full documentation is available here: https://mrcieu.github.io/TwoSampleMR.
3. Results
3.1. Instrumental variables selection
The intestinal flora (exposure) and AD (outcome) used in this analysis were summarized data collected by the MiBioGen Consortium and the Fingen Biobank analysis, respectively. Gut microbiota exposure was obtained from 24 cohort studies in the United States, Canada, Israel, South Korea, Germany, Denmark, the Netherlands, Belgium, Sweden, Finland, and the United Kingdom. These data included 16 s ribosomal RNA gene sequencing profiles and genotyping data from 18,340 participants. Seven different fecal DNA extraction methods and three different 16S rRNA regions (V4 (13 cohorts), V3-V4 (6 cohorts), and V1-V2 (5 cohorts)) were used. The exposure data included 211 intestinal biological groups including Bifidobacterium, Bacteroides, Clostridia, etc., which broadly summarized the distribution of human intestinal flora and was used for subsequent analysis by many studies. Detailed information was obtained from the study of the scholar Kurilshikov (40). The outcome included 5,321 AD patients and 213,146 control members (European), with a total number of SNPS of 16,380,466.After removing IVs in linkage disequilibrium, a total of 2,289 SNPs (p < 1 × 10−5) were included, including 5 taxa and 17 bacterial characteristics (1 phylum, 3 classes, 1 order, 4 families, and 8 genera). In addition, we also collected more information about SNPs (such as effect alleles, beta, SE, and p values), and all F-statastics >10, as shown in the Supplementary Table S2. Under the traditional threshold value (p < 5 × 10−8), we screened only 25 SNPs through a series of quality control, including 5 taxa and 22 bacterial characteristics(1 phylum, 1 class, 2 orders, 5 families, and 13 genera), and all F statistics were greater than 10 (Figure 2; Supplementary Table S3).
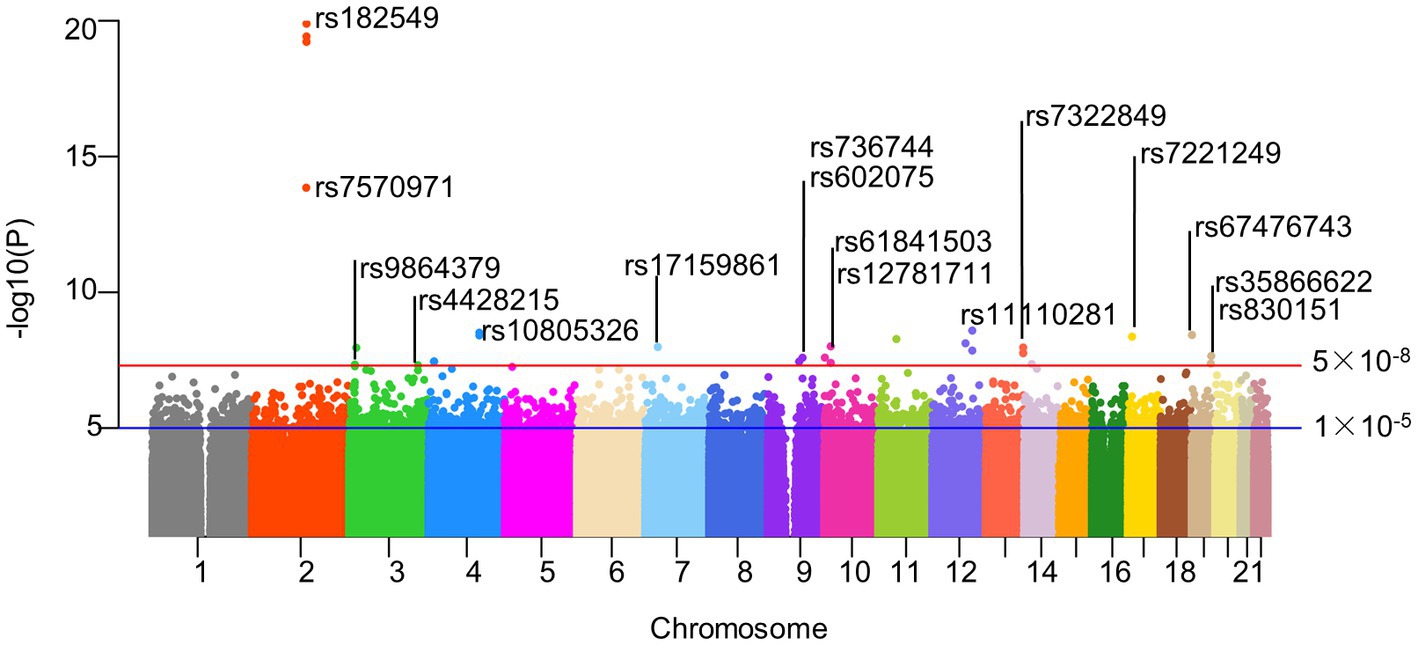
Figure 2. Manhattan plot of SNPs screening results. Horizontal lines define nominal genome-wide significance (p = 5 × 10−8. red) and suggestive genome-wide (p = 1 × 10−5. blue) thresholds. As described in our paper, 25 significant SNPs were included in our follow-up study after excluding SNP loci with palindromic structures under genome-wide association thresholds. Actinobacteria, Bifidobacteriaceae, Bifidobacterium, and Bifidobacteriales all contained rs182549, so we only identified them at the top of the corresponding SNPs. Similarly, rs61841503 (2), rs11110281 (2) and rs7322849 (3) were also identified only at the top. And the corresponding SNPs information could be read in the corresponding Supplementary Tables S2, S3. rs182549, rs7570971 (red); rs9864379, rs4428215 (limegreen); rs10805326 (dodgerblue); rs17159861 (lightskyblue); rs736744, rs602075 (purple2); rs61841503, rs12781711 (maroon2); rs11110281 (slateblue2); rs7322849 (tomato1); rs7221249 (gold1); rs67476743, rs35866622, rs830151(tan).
3.2. Mendelian randomization analysis of gut microbiota and AD
3.2.1. Mendelian randomization analysis of gut microbiota and AD obtained at suggestive genome-wide significance (1 × 10−5)
Based on several methods of MR analysis, we observed evidence of a potential causal association between gut microbiota and AD risk. Bounded by a significance of 0.05. The IVW analysis showed that Tenericutes, Mollicutes, Clostridia, Bifidobacteriaceae, Bifidobacteriales, Bifidobacterium, and Christensenellaceae R 7 group were negatively correlated with the risk of AD (Figure 3), while Clostridiaceae_1, Bacteroidaceae, Bacteroides, Anaerotruncus, unknown genus, and Lachnospiraceae UCG001 showed the opposite trend (Figure 4; Supplementary Figures S1–S13).
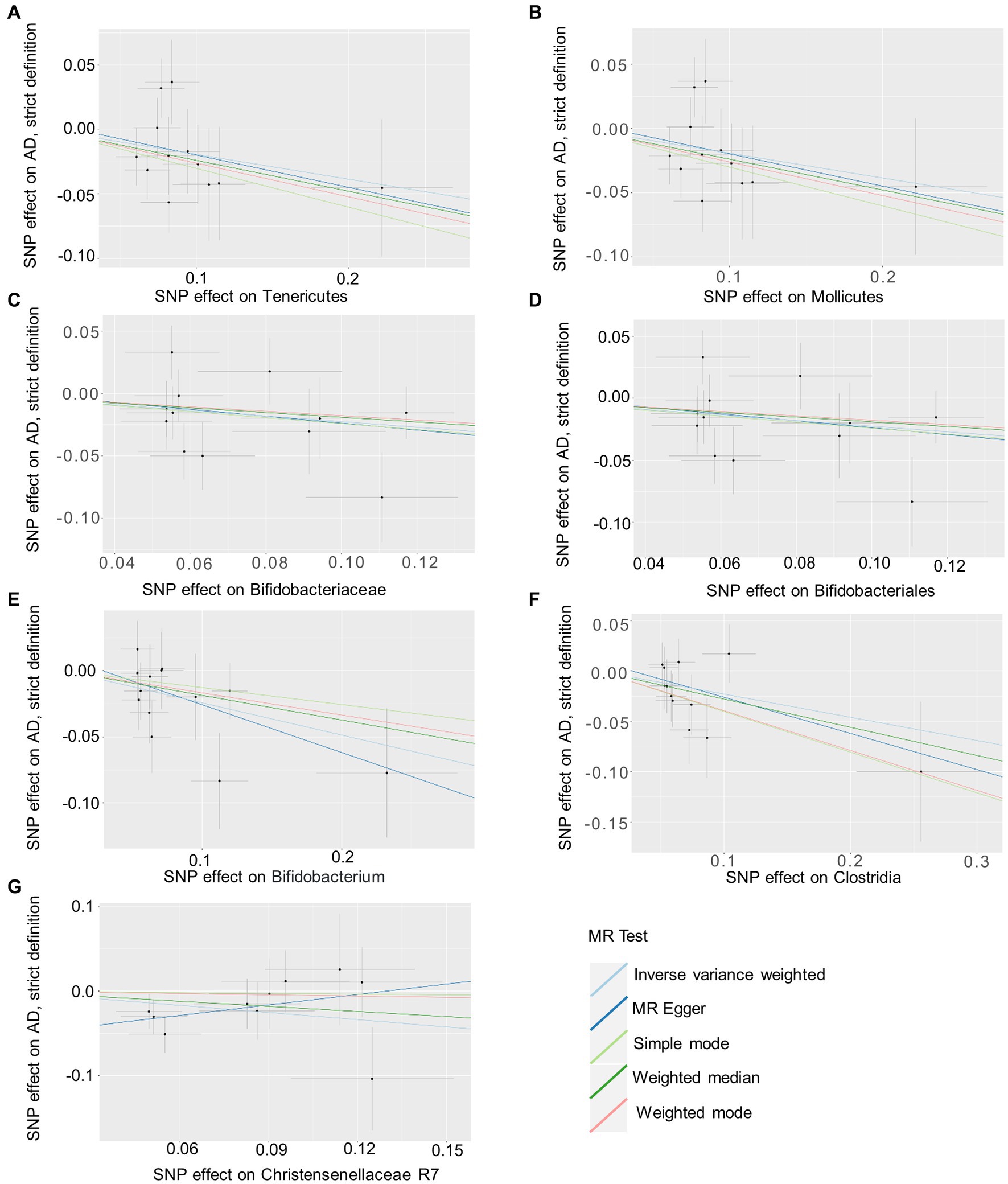
Figure 3. Summary of scatter plots of potential negative associations between intestinal flora and AD risk (A–G). Each dot in the graph represents an SNP locus. The vertical axis of the graph is the effect of the instrumental variable on the outcome, the horizontal axis is the effect of the instrumental variable on the exposure, and the ratio of the two effects is the effect of exposure on the outcome, that is, the slope of the regression line corresponds to the causal effect of exposure on the outcome in the graph. Horizontal and vertical crosses show a 95% confidence interval for each association. The estimates for the MR Analysis were slightly different, but the overall downward-sloping trend suggests that exposure (this gut microbiome) may have a negative causal effect on the outcome (AD). AD, atopic dermatitis; SNPs, single nucleotide polymorphisms; MR, Mendelian randomization; IVW, inverse variance weighted.
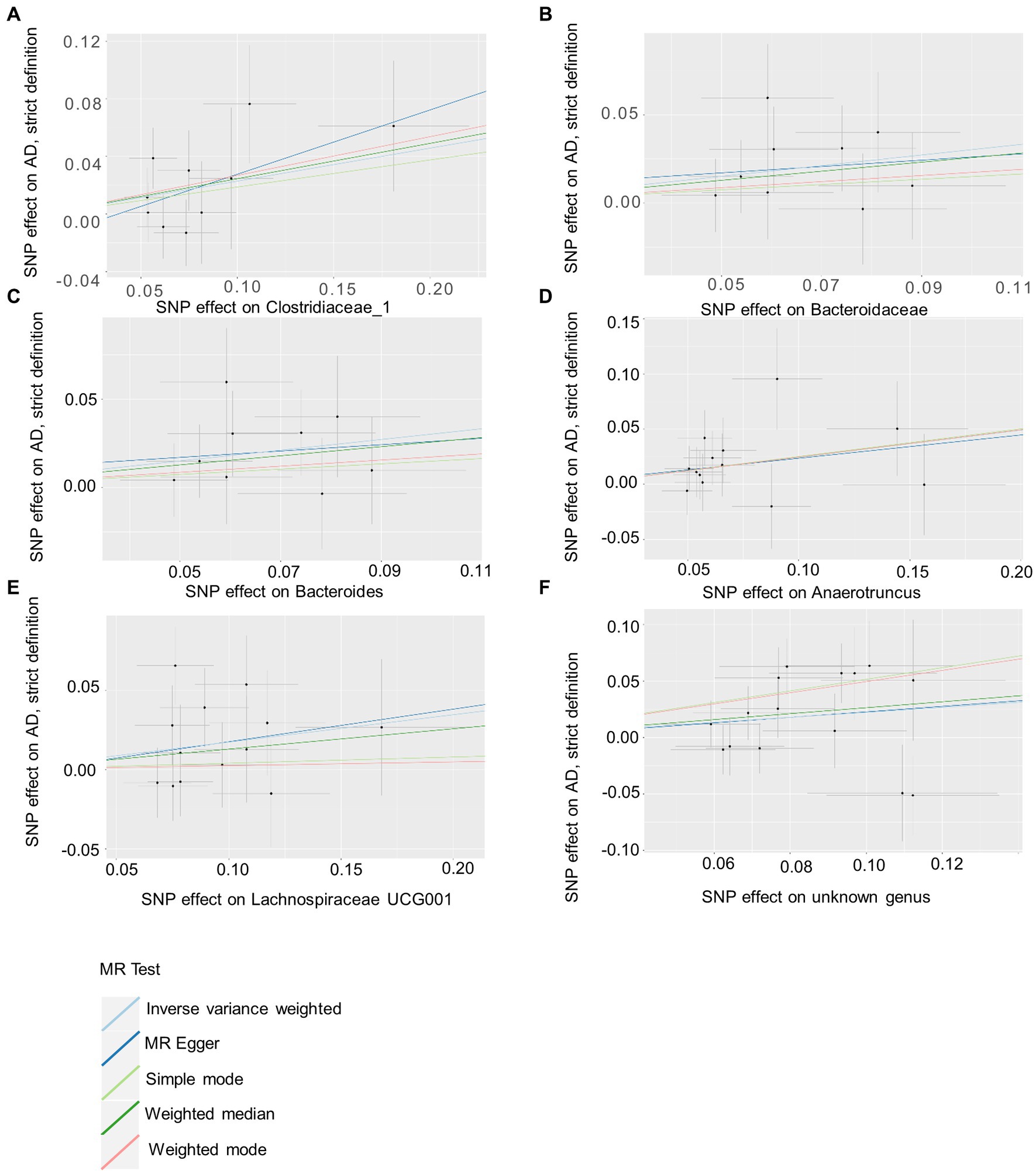
Figure 4. Summary of scatter plots of potential positive associations between intestinal flora and AD risk (A–F). IVW estimates show that Clostridiaceae_1, Bacteroidaceae, Bacteroides, Anaerotruncus, unknown genus, and Lachnospiraceae UCG001 and AD show an upward trend, suggesting a potential positive correlation between them. AD, atopic dermatitis; SNPs, single nucleotide polymorphisms; MR, Mendelian randomization; IVW, inverse variance weighted.
In addition, WME analysis results suggested that the Eubacterium hallii group (OR = 1.295, 95%CI, 1.012–1.659, p = 0.040; Supplementary Figure S14) was a risk factor for AD. MR-Egger estimated that genetically related Rhodospirillaceae were positively correlated with AD risk (OR = 2.163, 95%CI, 1.194–3.920, p = 0.024; Supplementary Figure S15), and Bacilli (OR = 0.556, 95%CI, 0.337–0.918, p = 0.036, Supplementary Figure S16), Anaerostipes(OR = 0.345, 95%CI, 0.152–0.782, p = 0.027; Supplementary Figure S17) had a potential protective effect on AD (Figure 5; Supplementary Table S4). The detailed information on the included SNPs were shown in the Supplementary Table S2.
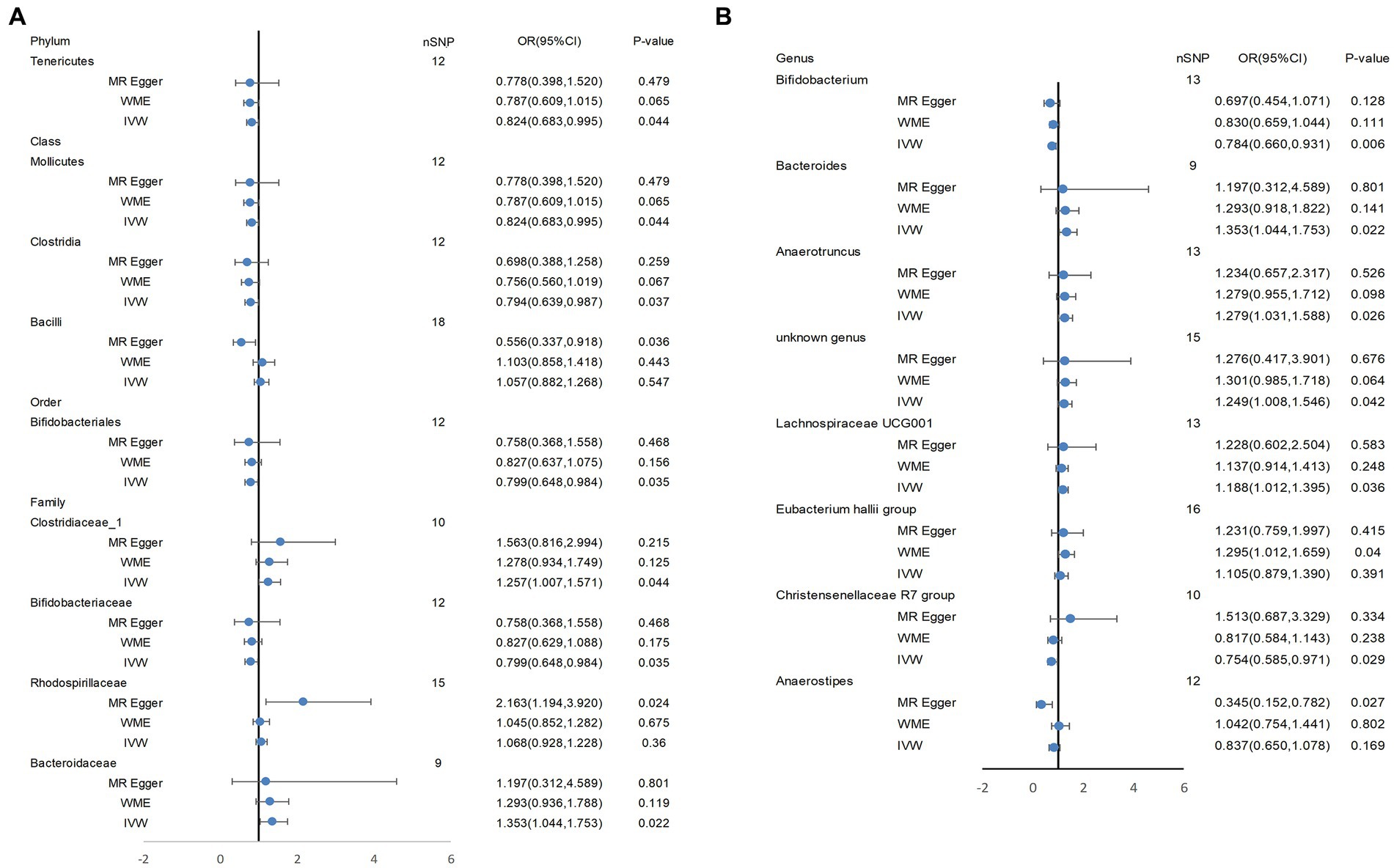
Figure 5. Tenericutes, Mollicutes, Clostridia, Bifidobacteriaceae, Bifidobacteriales, Bifidobacterium, and Christensenellaceae R 7 group, Clostridiaceae_1, Bacteroidaceae, Bacteroides, Anaerotruncus, unknown genus, and Lachnospiraceae UCG001 showed a potential causal relationship with AD risk. In (A), the three most classical MR Analysis methods are used to demonstrate the potential causal relationship between some bacterial groups and AD risk at four levels (phylum, class, order, family). (B) shows the potential causal association at the genus level between them. IVW, inverse variance weighted; WME, weighted median estimator.
MR-Egger regression estimated that the p values of the horizontal pleiotropy results for the three kinds of bacteria were all less than 0.05, but the MR-PRESSO test results suggested that there was no horizontal pleiotropy and outlier values, which need further discussion here. In addition, no horizontal pleiotropy was found in the other bacteria. The statistical values of SNPs were all greater than 10, indicating that there was no weak IVs bias in this study, and the analysis results were more credible. In addition, no SNPs that had a significant effect on causal association were found in the leave-one-out analysis (Supplementary Table S4). After MR Analysis of intestinal flora and atopic dermatitis, we concluded that significant matches between 2 × 10−4 (0.05/211) and 0.05 of intestinal flora may have a potential causal association with atopic dermatitis to some extent (29). MR Steiger’s test verified the causal hypothesis between intestinal flora exposure and AD, and the results showed that the effect of intestinal flora on AD was in a expected causal direction.
3.2.2. Mendelian randomization analysis of gut microbiota and AD obtained at nominal genome-wide significance (5 × 10−8)
The MR Results included a total of 22 gut microbiota abundance information related to genetic prediction, including 1 phylum (1 SNP), 1 class (1 SNP), 2 orders (3 SNPs), 5 families (6 SNPs), and 13 genera (14 SNPs), whose details were shown in Supplementary Table S3. Unknown genus, unknown family, and order Gastranaerophilales (all rs9864379) might be positively associated with AD risk (Wald ratio: OR,95%CI = 1.509 (1.057, 2.152), p = 0.023), as shown in Supplementary Table S5. The remaining taxa did not show a potential causal relationship with AD risk. Moreover, due to the small number of SNPs included under each gut microbiota category (1 or 2 SNPs), it was not possible to perform subsequent sensitivity analysis.
4. Discussion
Normal gut microbiology is mostly composed of firmicutes, bacteroidetes, actinobacteria, and proteobacteria (29), which remain relatively conserved and stable at the phylum level but differ considerably at the species level. We found in this MR analysis that Tenericutes, Mollicutes, Clostridia, Bifidobacteriaceae, Bifidobacteriales, Bifidobacterium, Christensenellaceae R7, Bacilli, and Anaerostipes levels were inversely associated with the risk of AD, and Eubacterium hallii group, Clostridiaceae_1, Bacteroidaceae, Bacteroides, Anaerotruncus, unknown genus, and Lachnospiraceae UCG 001 were potential risk factors for AD.
The gut microbiota plays an important role not only in immune, and metabolic regulation but also in neurodevelopment and feedback mechanisms, among others, from the period when the infant begins colonization after birth until the entire human life span (41). Some studies have found that gut microbiota diversity and abundance of specific bacteria are closely related to the onset age and severity of AD, but some scholars also do not recognize the former view. Therefore, whether gut microbiota abundance change is a key reason for the occurrence and development of AD becomes the main entry point for this MR.
Colonization with specific flora may interfere with the course of AD by affecting the intestinal barrier and the balance of intestinal microbiology. For example, a birth cohort for childhood origin of asthma and allergic diseases (COCOA) found that low levels of Streptococcus and high levels of Akkermansia (Akkermansia family, Verrucomicrobia order) were found in transient AD cases, while the opposite was true in AD children with a persistent system (42). Furthermore, the abundance of the Clostridium genus increased, while the abundance of gut microbial functional genes related to energy metabolism and SCFA decreased. Lee reached a similar conclusion through metagenomic analysis (9).
Surprisingly, certain specific gut flora associated with AD also play important roles in cognitively dysfunctional populations, further driving the hypothesis of a “gut-brain-skin axis” (43). Increased abundances of Clostridiaceae were also strongly associated with AD risk in our analysis (Supplementary Table S4), among which increased abundance of clostridia is strongly associated with AD risk, possibly through the release of toxins that inhibit the chemotaxis of neutrophils and inactivate eosinophils, aggravating intestinal inflammation. On the one hand, the impaired intestinal barrier enters the bloodstream by releasing substances such as tryptophan and serotonin, and atopic march to distant skin (44). On the other hand, activates neuroimmune pathways to transmit signals to the brain, causing emotions such as anxiety and depression, and further releases neurotransmitters such as 5-hydroxytryptamine (5-HT, also known as serotonin) and tryptophan to affect the status of distant skin. The findings support the hypothesis of the “gut-skin-axis” and “gut-brain-skin axis” and add to the potential mechanisms explaining the causal association of gut flora with AD risk (Supplementary Figure S18). We found that, Anaerostipes could be used as a potential protective factor for AD in this study. Similar to what was reported by scholar Taylor, Anaerostipes could participate in the regulation of food anti-allergic reaction (45), and protect the body from the attack of foreign antigens. Rhodospirllaceae belongs to the alpha-proteus family, and it has been found that Rhodospirllaceae is involved in the pathogenesis of cognitive function and neuropsychiatric symptoms of Alzheimer’s disease (46), suggesting the potential background of Rhodospirllaceae in the gut-brain axis. In this paper, it was also found that Rhodospirllaceae is closely related to the pathogenesis of AD. It provides a new way of thinking for us to search for the specific mechanism of the “gut-brain-skin axis.”
In addition, it has also been reported that Enterococcus and Shigella are also significantly higher in children with early AD and that serotonin production aggravates skin pigmentation, whereas Bifidobacterium abundance decreases (47). As well-known probiotics, Bifidobacteria and Bifidobacterium play important roles in protein synthesis, preventing the invasion of foreign bacteria, and stimulating immunity (48). Bifidobacteria, on the other hand, can promote Treg differentiation while inhibiting the Th2 response. It also increases the proportion of Lactobacillus positively associated with increased propionic acid production (49, 50), on the other hand, it alleviates AD symptoms by regulating tryptophan metabolites (indole derivatives) to activate the aryl hydrocarbon receptor (AHR) signaling pathway (44). Bacilli include Bacillus and Lactobacillus, of which Lactobacillus has been reported to involved in eosinophilic regulation, reducing eosinophil count and serum IgE concentration, activating regulatory T cells and TH1/TH2 balance, and contributing to intestinal microecological balance (51–53). Like Lactobacillus, Bacillus subgroups also play a protective role by regulating intestinal microecology. Some of the Bacillus species (such as Bacillus coagulans TL3, Bacillus velezensis A2) can reduce oxidative stress and inflammatory damage by participating in the regulation of signaling pathways, and also participate in the regulation of intestinal flora abundance, increasing the relative abundance of beneficial bacteria and the expression of intestinal barrier tight junction protein (54–56).
Christensenellaceae R7 belongs to the phylum firmicutes and is widely found in the intestinal mucosa of humans and animals. Some scholars have found that Christensenellaceae R7 is significantly negatively correlated with Body Mass Index (BMI) (57), inflammation (58), and other metabolic diseases (59, 60). Similarly, when scholar Zhao (61) established acute colitis models in sensitized pigs and Yorkshire pigs, he found potentially beneficial microorganisms related to intestinal barrier function, such as Lactobacillus, Eubacillus, and Christensenellaceae, through microbial sequencing analysis. Once again, the potential protective effect of Christensenellaceae was verified. Desulfurizing bacteria, Bacteroides, and Streptococcus still play dangerous roles, as discussed earlier. In the results of this study, the Eubacterium hallii group is considered a risk factor for AD, which has been proven in many autoimmune diseases such as Hashimoto’s thyroiditis (62), clear cell renal cell carcinoma (63), rheumatoid arthritis (RA) (64). Eubacterium hallii group and Lachnospiraceae UCG001 belong to the Lachnospiraceae family and can both produce butyrate and participate in the metabolism of amino acids and lipids. In the study of intestinal flora dysbiosis and hyperuricemia, scholar Song found that the enrichment of Lachnospiraceae UCG001 and Anaerotruncus led to intestinal dysbiosisand intestinal barrier damage, which interfered with amino acid metabolism and increased blood uric acid level and CD4 Th17-driven inflammation (65).
In addition, we found that as the risk of AD increased, the abundance of Bacteroidaceae and Bacteroides gradually increased. In line with our findings, the author Siqi Ye mentioned that the α diversity of intestinal flora in AD patients was decreased compared with healthy controls, but the relative abundance of parabacteroides, Bacteroides ovoides, and homobacteroides was significantly increased (66) The change in Bacteroides abundance is closely associated with gout (67), inflammatory bowel disease (68), autoimmune thyroid disease (AITD) (69), multiple sclerosis (MS) (66), rheumatoid arthritis (RA) (70), etc. Therefore, we speculate that Bacteroides may be used as diagnostic markers for autoimmune diseases such as AD in the future.
Tenericutes belong to the one of the placental-specific flora,part of which is involved in the composition of non-pathogenic symbiotic microbiota (71). Tenericutes may show different associations with health and disease (72–74). Although it is considered in this study that Tenericutes and Mollicutes mayhave potential protective effects on AD, the correlation effect of pairing them has not been observed at a more detailed level, so deeper studies are needed to explain clearly.
The microbiome may be a target for the treatment of immune diseases (75). Most observational studies believe that SCFA (such as acetate, butyrate, and kynurenic acid) produced by Akkermansia, Bifidobacteria, Facalibacterium, and other bacteria in the gut. is closely related to the diversity of the skin microbiome (76–78). SCFAs inhibit immune response by inhibiting the proliferation of inflammatory cells and the production of cytokines. In addition, SCFAs also regulate apoptosis and activation of immune cells by inhibiting histone deacetylase (HDAC) (79).
Therefore, it is intended to correlate the changes in intestinal microbiome abundance with AD, psoriasis, common eczema, and other skin lesions and develop new immunomodulators to promote skin improvement. In our analysis, it can also be observed that Bacteroides, Lachnospiraceae, and Bifidobacteriaceae(these three intestinal bacteria are related to SCFA synthesis) have a potential causal relationship with AD. Song et al. combined with whole genome sequencing did not observe the reduction of evolutionary branches of Lactobacillus and Bifidobacterium in the analysis (80), and some reports believed that the increase of some groups in the intestinal flora of children with AD was not related to AD, but to the potential harmful flora in the damaged intestinal environment (81). For acute AD, changes in the composition of some intestinal flora may be less obvious, leading to different conclusions. In order to avoid the interference of confounding factors, we strictly abide by the three principles of MR and verify the causal association between the changes in the abundance of intestinal flora and the risk of AD from the genetic perspective.
Some researchers found that Gastranaerophilales was one of the main indole-producing bacteria, and the concentration of indole and its derivatives was increased in patients with Alzheimer’s disease (82), suggesting that this bacterium might be involved in the formation of the gut-brain axis. Furthermore, scholar wang used enterotoxigenic Escherichia coli (ETEC) to damage the intestinal epithelial barrier function, causing severe intestinal diarrhea in mice. In the feces of the disease group, the abundance of pathogenic bacteria(Gastranaerophilales and Escherichia coli) in the intestine was found to increase (83). In a study based on a GWAS dataset, Cao and colleagues used microbiota-related gene set enrichment analysis to explore the relationship between the gut microbiota and six autoimmune diseases, including chylous diarrhea (CeD), inflammatory bowel disease (IBD), multiple sclerosis (MS), primary biliary cirrhosis (PBC), type 1 diabetes (T1D), and primary sclerosing cholangitis (PSC), and osteoporosis (OP). Surprisingly, Peptostreptococcaceae, Gastanaerophilales, and Romboutsia were found in the flora of the other six autoimmune diseases except for PBC (84). In addition, there was a possible association between AD and autoimmune diseases (85), so we suspected that Gastranaerophilales may also be involved in the pathogenesis of AD.
Host genetics may also play an important role in the construction of intestinal flora. Host genetic distance was found to be associated with BMI and the combination of intestinal flora diversity (86), and animal experiments also identified genes involved in metabolism, immunity, and behavior, such as myeloid differentiation primary response protein 88 (MYD88) (87), human leukocyte antigen (HLA) may be associated with intestinal flora (88). The combination of environment and heredity on intestinal flora provides an idea for further understanding of “gut-skin” axis.
To the best of our knowledge, this is the first MR study to look at the genetic link between the gut microbiome and atopic dermatitis. According to this MR analysis, we recognized the causal relationship between intestinal flora and AD and found that some specific intestinal flora participated in the pathogenesis of AD. The present MR analysis of the causal relationship between the gut microbiota and AD, which both reduces the possibility of the presence of confounders in the outcome and negates the reverse causal association of the two, is more convincing than observational studies.
But in fact, there are still some deficiencies: First, the data included in this MR analysis are all of European ancestry, and different ethnicities do not share the same dominant gut flora due to different exposure factors such as dietary environment (9), so generalizing the results to other ethnic populations is limited; Second, we adopted linear MR analysis because specific sample data were not available, resulting in the inability to perform subgroup analyzes based on age and gender; Then, we included SNPs of gut microbiota at the phylum, class, order, family, and genus levels, which could not be analyzed at a more specific species level. What’s more, because our study was based on GWAS summarized data, we were unable to obtain detailed clinical differences within each group of patients with specific dermatitis, and therefore could not combine clinical differences among each group for further study. Finally, the composition of the infant gut microbiota is highly dynamic, with certain species genera exhibiting opposite states at the beginning versus the continuing stages of AD, but our original hypothesis is that the emergence and change of certain species abundances is relatively stable.
5. Conclusion
Using a two-sample Mendelian randomization approach, our study provides a potential causal association between the abundance of gut microbes and the risk of AD and suggests a genetic relationship between the two. The results of our MR analysis will provide beneficial support for gut microecological-based therapy of AD and lay a solid foundation for further exploration of the pathogenesis of gut microbiota leading to AD.
Data availability statement
The datasets presented in this study can be found in online repositories. The names of the repository/repositories and accession number(s) can be found in the article/Supplementary material.
Author contributions
YX, LZ, YC, HW, and JX: study design. YX, HW, LZ, and YC: data collection and data analysis. YX, LZ, and YC: drafting manuscript. All authors take responsibility for the integrity of the data analysis and read and approved the article.
Funding
This work was funded by the Science and Technology Planning Project of Sichuan Province (2021YJ0170) and the Project of Chengdu Science and Technology Bureau (2019-YF05-00498-SN).
Conflict of interest
The authors declare that the research was conducted in the absence of any commercial or financial relationships that could be construed as a potential conflict of interest.
Publisher’s note
All claims expressed in this article are solely those of the authors and do not necessarily represent those of their affiliated organizations, or those of the publisher, the editors and the reviewers. Any product that may be evaluated in this article, or claim that may be made by its manufacturer, is not guaranteed or endorsed by the publisher.
Supplementary material
The Supplementary material for this article can be found online at: https://www.frontiersin.org/articles/10.3389/fmed.2023.1174331/full#supplementary-material
References
2. Langan, SMAD, and Weidinger, IS. Atopic dermatitis. Lancet. (2020) 396:345–60. doi: 10.1016/S0140-6736(20)31286-1
3. Perkin, MRDP, Strachan, HC, Williams, CT, and Golding, KJ. Natural history of atopic dermatitis and its relationship to serum total immunoglobulin E in a population-based birth cohort study. Pediatr Allergy Immunol. (2004) 15:221–9. doi: 10.1111/j.1399-3038.2004.00160.x
4. Davies, EN, Rogers, A, Lloyd-Lavery, DJC, and Thomas, GKS. What's new in atopic eczema? An analysis of systematic reviews published in 2015. Part 1: epidemiology and methodology. Clin Exp Dermatol. (2018) 43:375–9. doi: 10.1111/ced.13377
5. Weidinger, SLA, Beck, T, Bieber, K, and Irvine, KAD. Atopic dermatitis. Nat Rev Dis Primers. (2018) 4:1. doi: 10.1038/s41572-018-0001-z
6. Drucker, AMAR, Wang, WQ, Li, E, Sevetson, JK, and Qureshi, BAA. The burden of atopic dermatitis: summary of a report for the National Eczema Association. J Invest Dermatol. (2017) 137:26–30. doi: 10.1016/j.jid.2016.07.012
7. Sroka-Tomaszewska, J, and Trzeciak, M. Molecular mechanisms of atopic dermatitis pathogenesis. Int J Mol Sci. (2021) 22:4130. doi: 10.3390/ijms22084130
8. Reddel, SF, Del Chierico, A, Quagliariello, S, Giancristoforo, P, Vernocchi, A, Russo, A, et al. Gut microbiota profile in children affected by atopic dermatitis and e valuation of intestinal persistence of a probiotic mixture. Sci Rep. (2019) 9:4996. doi: 10.1038/s41598-019-41149-6
9. Lee, MJMJ, Kang, SY, Lee, E, Lee, K, Kim, S, Won, DI, et al. Perturbations of gut microbiome genes in infants with atopic dermatitis according to feeding type. J Allergy Clin Immunol. (2018) 141:1310–9. doi: 10.1016/j.jaci.2017.11.045
10. Mahmud, MRS, Akter, SK, Tamanna, L, Mazumder, IZ, Esti, S, Banerjee, S, et al. Impact of gut microbiome on skin health: gut-skin axis observed through the lenses of therapeutics and skin diseases. Gut Microbes. (2022) 14:2096995. doi: 10.1080/19490976.2022.2096995
11. De Pessemier, BL, Grine, M, Debaere, A, Maes, B, and Callewaert, PC. Gut-skin Axis: current knowledge of the interrelationship between microbial Dysbiosis and skin conditions. Microorganisms. (2021) 9:353. doi: 10.3390/microorganisms9020353
12. Wang, MC, Karlsson, C, Olsson, I, Adlerberth, AE, Wold, DP, Strachan, PM, et al. Reduced diversity in the early fecal microbiota of infants with atopic eczema. J Allergy Clin Immunol. (2008) 121:129–34. doi: 10.1016/j.jaci.2007.09.011
13. Galazzo, GN, van Best, L, Bervoets, IO, Dapaah, PH, Savelkoul, MW, Hornef, S, et al. Development of the microbiota and associations with birth mode, diet, and atopic disorders in a longitudinal analysis of stool samples, collected from infancy through early childhood. Gastroenterology. (2020) 158:1584–96. doi: 10.1053/j.gastro.2020.01.024
14. Fujimura, KEAR, Sitarik, S, Havstad, DL, Lin, S, Levan, D, Fadrosh, AR, et al. Neonatal gut microbiota associates with childhood multisensitized atopy and T cell differentiation. Nat Med. (2016) 22:1187–91. doi: 10.1038/nm.4176
15. Kespohl, MN, Vachharajani, M, Luu, H, Harb, S, Pautz, S, Wolff, N, et al. The microbial metabolite butyrate induces expression of Th1-associated factors in CD4(+) T cells. Front Immunol. (2017) 8:1036. doi: 10.3389/fimmu.2017.01036
16. Maravillas-Montero, JLAM, Burkhardt, PA, Hevezi, CD, Carnevale, MJ, and Zlotnik, SA. Cutting edge: GPR35/CXCR8 is the receptor of the mucosal chemokine CXC L17. J Immunol. (1950) 194:29–33. doi: 10.4049/jimmunol.1401704
17. S.A J Scott. Chang, FPV, Microbial tryptophan metabolites regulate gut barrier function via the aryl hydrocarbon receptor. Proc Natl Acad Sci U S A, (2020), 117, 19376–19387. doi: 10.1073/pnas.2000047117
18. Petersen, EBML, Skov, JP, and Jensen, TP. Role of the gut microbiota in atopic dermatitis: a systematic review. Acta Derm Venereol. (2019) 99:5–11. doi: 10.2340/00015555-3008
19. Marrs, TC. Flohr, the role of skin and gut microbiota in the development of atopic eczema. Br J Dermatol. (2016) 175:13–8. doi: 10.1111/bjd.14907
20. Abrahamsson, TRHE, Jakobsson, AF, Andersson, B, Björkstén, L, and Jenmalm, EMC. Low diversity of the gut microbiota in infants with atopic eczema. J Allergy Clin Immunol. (2012) 129:434–40. doi: 10.1016/j.jaci.2011.10.025
21. Nowrouzian, FLG, Lina, E, Hodille, E, Lindberg, B, Hesselmar, R, Saalman, I, et al. Superantigens and adhesins of infant gut commensal Staphylococcus aureus strains and association with subsequent development of atopic eczema. Br J Dermatol. (2017) 176:439–45. doi: 10.1111/bjd.15138
22. Cheng, BS, Cheng, C, Li, W, Wei, L, Liu, P, Meng, X, et al. Treatment resistance in schizophrenia is associated with attention deficit/hyperactivity disorder and gut microbiota: a genetic correlation and Mendelian randomization study. Neuropsychobiology. (2023) 82:24–32. doi: 10.1159/000528316
23. Lahouel, MI, Lahouel, H, Ben, BM, Hammouda, M, and Zili, YJ. A comparative case-control study of diagnostic criteria for atopic dermatitis and proposal of new diagnostic criteria from Tunisia. Int J Dermatol. (2020) 59:962–8. doi: 10.1111/ijd.14985
24. Samochocki, ZJ. Dejewska, a comparison of criteria for diagnosis of atopic dermatitis in children. World J Pediatr. (2012) 8:355–8. doi: 10.1007/s12519-012-0381-1
25. Silverberg, JID, Lei, M, Yousaf, SR, Janmohamed, PP, Vakharia, R, Chopra, R, et al. Measurement properties of the Rajka-Langeland severity score in children and adults with atopic dermatitis. Br J Dermatol. (2021) 184:87–95. doi: 10.1111/bjd.19159
26. Rudzki, EZ, Samochocki, P, Rebandel, E, Saciuk, W, Gałecki, A, and Szmurło, RA. Frequency and significance of the major and minor features of Hanifin and Rajka among patients with atopic dermatitis. Dermatology. (1994) 189:41–6. doi: 10.1159/000246781
27. Li, PH, Wang, L, Guo, X, Gou, G, Chen, D, Lin, D, et al. Association between gut microbiota and preeclampsia-eclampsia: a two-sample Mendelian randomization study. BMC Med. (2022) 20:443. doi: 10.1186/s12916-022-02657-x
28. Liu, KPWJ, Zou, H, Fan, HHY, Cheng, F, He, J, and You, LZ. Mendelian randomization analysis reveals causal relationships between gut microbiome and optic neuritis. Hum Genet. (2022) 2022:514. doi: 10.1007/s00439-022-02514-0
29. Liu, KJ, Zou, H, Fan, H, and You, HZ. Causal effects of gut microbiota on diabetic retinopathy: a Mendelian randomization study. Front Immunol. (2022) 13:930318. doi: 10.3389/fimmu.2022.930318
30. Liu, XX, Tong, Y, Zou, X, Lin, H, Zhao, L, Tian, Z, et al. Mendelian randomization analyses support causal relationships between blood metabolites and the gut microbiome. Nat Genet. (2022) 54:52–61. doi: 10.1038/s41588-021-00968-y
31. Feng, YR, Wang, C, Li, X, Cai, Z, Huo, Z, et al. Causal effects of genetically determined metabolites on cancers included lung, breast, ovarian cancer, and glioma: a Mendelian randomization study. Transl lung. Cancer Res. (2022) 11:1302–14. doi: 10.21037/tlcr-22-34
32. Lv, WQX, Lin, H, Shen, HM, Liu, X, Qiu, BY, Li, WD, et al. Human gut microbiome impacts skeletal muscle mass via gut microbial synthesis of the short-chain fatty acid butyrate among healthy menopausa l women. J Cachexia Sarcopenia Muscle. 12:1860–70. doi: 10.1002/jcsm.12788
33. Zhang, ZJHLQN, Zhao, J, Wang, XY, Wang, R, and Li, HB. Assessment of causal direction between gut microbiota and inflammatory bowel disease: a Mendelian randomization analysis. Front Genet. (2021) 12:631061. doi: 10.3389/fgene.2021.631061
34. Palmer, TMDA, Lawlor, RM, Harbord, NA, Sheehan, JH, Tobias, NJ, Davey, TG, et al. Using multiple genetic variants as instrumental variables for modifiable risk factors. Stat Methods Med Res. (2012) 21:223–42. doi: 10.1177/0962280210394459
35. Hirtz, RC, Hars, R, Naaresh, BH, Laabs, J, Antel, C, Grasemann, A, et al. Causal effect of age at menarche on the risk for depression: results from a two-sample multivariable Mendelian randomization study. Front Genet. (2022) 13:918584. doi: 10.3389/fgene.2022.918584
36. Bowden, JG, and Burgess, DSS. Mendelian randomization with invalid instruments: effect estimation and bias detection through egger regression. Int J Epidemiol. (2015) 44:512–25. doi: 10.1093/ije/dyv080
37. Bowden, JMF, Del Greco, C, Davey, MG, Smith, N, and Thompson, SJ. A framework for the investigation of pleiotropy in two-sample summary data Mendelian randomization. Stat Med. (2017) 36:1783–802. doi: 10.1002/sim.7221
38. Bowden, JG, Davey Smith, PC, and Burgess, HS. Consistent estimation in Mendelian randomization with some invalid instruments using a weighted median estimator. Genet Epidemiol. (2016) 40:304–14. doi: 10.1002/gepi.21965
39. Xiang, KP, Wang, Z, Xu, YQ, Hu, YS, He, Y, Chen, YT, et al. Causal effects of gut microbiome on systemic lupus Erythematosus: a two-sample Mendelian randomization study. Front Immunol. (2021) 12:667097. doi: 10.3389/fimmu.2021.667097
40. Kurilshikov, AC, Medina-Gomez, R, Bacigalupe, D, Radjabzadeh, J, Wang, A, Le, DCI, et al. Large-scale association analyses identify host factors influencing human gut microbiome composition. Nat Genet. (2021) 53:156–65. doi: 10.1038/s41588-020-00763-1
41. Zhuang, LH, Chen, S, Zhang, J, Zhuang, Q, and Feng, LZ. Intestinal microbiota in early life and its implications on childhood health. Genomics Proteomics Bioinformatics. (2019) 17:13–25. doi: 10.1016/j.gpb.2018.10.002
42. Park, YMS, Lee, MJ, Kang, BS, Kim, MJ, Lee, SS, Jung, JS, et al. Imbalance of gut Streptococcus, Clostridium, and Akkermansia determines the natural course of atopic dermatitis in infant. Allergy Asthma Immunol Res. (2020) 12:322–37. doi: 10.4168/aair.2020.12.2.322
43. Zhan, GD, Hua, N, Huang, Y, Wang, S, Li, Z, Zhou, N, et al. Anesthesia and surgery induce cognitive dysfunction in elderly male mice: the role of gut microbiota. Aging (Albany NY). (2019) 11:1778–90. doi: 10.18632/aging.101871
44. Lee, ESY, Lee, MJ, Kang, K, Kim, S, Won, BJ, Kim, KY, et al. Clostridia in the gut and onset of atopic dermatitis via eosinophilic inflammation. Ann Allergy Asthma Immunol. (2016) 117:91–92.e1. doi: 10.1016/j.anai.2016.04.019
45. Feehley, TCH, Plunkett, R, Choi, BSM, Hong, E, Culleen, P, Belda-Ferre, E, et al. Healthy infants harbor intestinal bacteria that protect against food allergy. Nat Med. (2019) 25:448–53. doi: 10.1038/s41591-018-0324-z
46. Zhou, YY, Wang, M, Quan, H, and Jia, ZJ. Gut microbiota changes and their correlation with cognitive and neuropsychiatric symptoms in Alzheimer's disease. J Alzheimers Dis. (2021) 81:583–95. doi: 10.3233/JAD-201497
47. Yap, GCEX, Loo, M, Aw, QLLP, and Lee, SBW. Molecular analysis of infant fecal microbiota in an Asian at-risk cohort-correlates with infant and childhood eczema. BMC Res Notes. (2014) 7:166. doi: 10.1186/1756-0500-7-166
48. Rivière, AM, Selak, D, Lantin, F, and De Vuyst, LL. Bifidobacteria and butyrate-producing Colon Bacteria: importance and strategies for their stimulation in the human gut. Front Microbiol. (2016) 7:979. doi: 10.3389/fmicb.2016.00979
49. Fang, ZL, Li, J, Zhao, H, Zhang, YK, Lee, W, and Chen, LW. Bifidobacteria adolescentis regulated immune responses and gut microbial composition to alleviate DNFB-induced atopic dermatitis in mice. Eur J Nutr. (2020) 59:3069–81. doi: 10.1007/s00394-019-02145-8
50. Fang, ZT, Pan, H, Wang, J, Zhu, H, Zhang, J, Zhao, W, et al. Limosilactobacillus reuteri attenuates atopic dermatitis via changes in gut Bacteria and Indole derivatives from tryptophan metabolism. Int J Mol Sci. (2022) 23:7735. doi: 10.3390/ijms23147735
51. Jeong, KM, Kim, SA, Jeon, Y-H, and Lee, KS. A randomized trial of Lactobacillus rhamnosus IDCC 3201 tyndallizate (RHT3201) for treating atopic dermatitis. Pediatr Allergy Immunol. 31:783–92. doi: 10.1111/pai.13269
52. Inoue, YT, Kambara, N, Murata, J, Komori-Yamaguchi, S, Matsukura, Y, Takahashi, Z, et al. Effects of oral administration of Lactobacillus acidophilus L-92 on the symptoms and serum cytokines of atopic dermatitis in Japanese adults: a double-blind, randomized, clinical trial. Int Arch Allergy Immunol. (2014) 165:247–54. doi: 10.1159/000369806
53. Kim, W-KYJ, Jang, DH, Han, K, Jeon, C, Lee, HS, and Ko, HG. Lactobacillus paracasei KBL382 administration attenuates atopic dermatitis by modulating immune response and gut microbiota. Gut Microbes. 12:1–14. doi: 10.1080/19490976.2020.1819156
54. Wang, YJ, Lin, Z, Cheng, T, Wang, J, and Long, CM. Bacillus coagulans TL3 inhibits LPS-induced Caecum damage in rat by regulating the TLR4/MyD88/NF-κB and Nrf2 signal pathways and modulating intestinal microflora. Oxidative Med Cell Longev. (2022) 2022:5463290. doi: 10.1155/2022/5463290
55. Cai, JN, Wang, J, Chen, A, Wu, E, Nepovimova, M, Valis, M, et al. Bacillus velezensis A2 inhibited the Cecal inflammation induced by Zearalenone by regulating intestinal Flora and Short-chain fatty a cids. Front Nutr. 9:806115. doi: 10.3389/fnut.2022.806115
56. Luo, RJ, Zhang, X, Zhang, Z, Zhou, W, Zhang, Z, Zhu, H, et al. Bacillus subtilis HH2 ameliorates TNBS-induced colitis by modulating gut microbiota composition and improving intestinal barrier function in rabbit model. J Funct Foods. 74:104167. doi: 10.1016/j.jff.2020.104167
57. Goodrich, JKJL, Waters, AC, Poole, JL, Sutter, O, Koren, R, Blekhman, M, et al. Human genetics shape the gut microbiome. Cells. 159:789–99. doi: 10.1016/j.cell.2014.09.053
58. Mancabelli, LC, Milani, GA, Lugli, F, Turroni, D, Van Cocconi, D, and Ventura, SM. Identification of universal gut microbial biomarkers of common human intestinal diseases by meta-analysis. FEMS Microbiol Ecol. (2017) 93:153. doi: 10.1093/femsec/fix153
59. Hill-Burns, EMJW, Debelius, JT, Morton, WT, Wissemann, MR, Lewis, ZD, and Wallen, SD. Parkinson's disease and Parkinson's disease medications have distinct signatures of the gut microbiome. Mov Disord. 32:739–49. doi: 10.1002/mds.26942
60. Coello, KTH, Hansen, N, Sørensen, NM, Ottesen, KW, Miskowiak, O, Pedersen, LV, et al. Affective disorders impact prevalence of Flavonifractor and abundance of Christensenellaceae in gut microbiota. Prog Neuropsychopharmacol Biol Psychiatry. (2021) 110:110300. doi: 10.1016/j.pnpbp.2021.110300
61. Zhao, XL, Jiang, X, Fang, Z, Guo, X, Wang, B, and Meng, SQ. Host-microbiota interaction-mediated resistance to inflammatory bowel disease in pigs. Microbiome. (2022) 10:115. doi: 10.1186/s40168-022-01303-1
62. Zhao, FJ, Feng, J, Li, L, Zhao, Y, Liu, H, Chen, Y, et al. Alterations of the gut microbiota in Hashimoto's thyroiditis patients. Thyroid. (2018) 28:175–86. doi: 10.1089/thy.2017.0395
63. Chen, YJ, Ma, Y, Dong, Z, Yang, N, Zhao, Q, Liu, W, et al. Characteristics of gut microbiota in patients with clear cell renal cell carcinoma. Front Microbiol. (2022) 13:913718. doi: 10.3389/fmicb.2022.913718
64. Zhu, JT, Wang, Y, Lin, M, Xiong, J, Chen, C, Jian, J, et al. The change of plasma metabolic profile and gut microbiome dysbiosis in patients with rheumatoid arthritis. Front Microbiol. (2022) 13:931431. doi: 10.3389/fmicb.2022.931431
65. Song, SY, Lou, Y, Mao, X, Wen, M, Fan, Z, He, Y, et al. Alteration of gut microbiome and correlated amino acid metabolism contribute to Hyperuricemia and Th17-driven inflammation in Uox-KO mice. Front Immunol. (2022) 13:804306. doi: 10.3389/fimmu.2022.804306
66. Schepici, GS, Silvestro, P, and Mazzon, BE. The gut microbiota in multiple sclerosis: an overview of clinical trials. Cell Transplant. (2019) 28:1507–27. doi: 10.1177/0963689719873890
67. Chu, YS, Sun, Y, Huang, Q, Gao, X, Xie, P, Wang, J, et al. Metagenomic analysis revealed the potential role of gut microbiome in gout. NPJ Biofilms Microbiomes. (2021) 7:66. doi: 10.1038/s41522-021-00235-2
68. Guo, XC, Huang, JXHXL, Liu, H, Zhao, J, Wang, W, Huang, W, et al. Gut microbiota is a potential biomarker in inflammatory bowel disease. Front Nutr. (2021) 8:818902. doi: 10.3389/fnut.2021.818902
69. Cayres, LCFLVV, de Salis, GSP, Rodrigues, AVH, Lengert, APC, Biondi, LDB, Sargentini, JL, et al. Detection of alterations in the gut microbiota and intestinal permeability in patients with Hashimoto thyroiditis. Front Immunol. (2021) 12:579140. doi: 10.3389/fimmu.2021.579140
70. Chen, YC, Ma, L, Liu, J, He, C, Zhu, F, Zheng, W, et al. Analysis of gut microbiota and metabolites in patients with rheumatoid arthritis and identification of potential biomarkers. Aging. (2021) 13:23689–701. doi: 10.18632/aging.203641
71. Aagaard, KJ, Ma, KM, Antony, R, Ganu, J, and Versalovic, PJ. The placenta harbors a unique microbiome. Sci Transl Med. (2014) 6:237ra65. doi: 10.1126/scitranslmed.3008599
72. Puri, KDH, Taft, N, Ambalavanan, KR, Schibler, AL, and Kallapur, MSG. Association of Chorioamnionitis with aberrant neonatal gut Colonizatio n and adverse clinical outcomes. PLoS One. 11:e0162734. doi: 10.1371/journal.pone.0162734
73. Ferri, CM, Castellazzi, N, Merli, M, Laudisi, E, Baldin, E, Baldi, L, et al. Gut microbiota changes during dimethyl Fumarate treatment in patients with multiple sclerosis. Int J Mol Sci. 24:2720. doi: 10.3390/ijms24032720
74. Crovesy, LD, and Rosado, MEL. Profile of the gut microbiota of adults with obesity: a systematic rev iew. Eur J Clin Nutr. 74:1251–62. doi: 10.1038/s41430-020-0607-6
75. Peroni, DGG, Nuzzi, I, Di, TME, and Comberiati, CP. Microbiome composition and its impact on the development of allergic diseases. Front Immunol. (2020) 11:700. doi: 10.3389/fimmu.2020.00700
76. Tan, LS, Zhao, W, Zhu, L, Wu, J, Li, M, Shen, L, et al. The Akkermansia muciniphila is a gut microbiota signature in psoriasis. Exp Dermatol. 27:144–9. doi: 10.1111/exd.13463
77. Matsumoto, MT, Ebata, J, Hirooka, R, Hosoya, N, Inoue, S, Itami, K, et al. Antipruritic effects of the probiotic strain LKM512 in adults with ato pic dermatitis. Ann Allergy Asthma Immunol. 113:209–216.e7. doi: 10.1016/j.anai.2014.05.002
78. Louis, PP, Young, G, and Flint, HHJ. Diversity of human colonic butyrate-producing bacteria revealed by analysis of the butyryl-CoA:acetate CoA-transferase gene. Environ Microbiol. (2010) 12:304–14. doi: 10.1111/j.1462-2920.2009.02066.x
79. Shi, YMXS, Pan, S, Gao, J, Ren, R, Bai, H, Li, C, et al. Induction of the apoptosis, degranulation and IL-13 production of human basophils by butyrate and propionate via suppression of histone deacetylation. Immunology. (2021) 164:292–304. doi: 10.1111/imm.13370
80. Song, HY, Yoo, J, Hwang, YC, and Kim, NHS. Faecalibacterium prausnitzii subspecies-level dysbiosis in the human gut microbiome underlying atopic dermatitis. J Allergy Clin Immunol. (2016) 137:852–60. doi: 10.1016/j.jaci.2015.08.021
81. Stecher, BL, and Hardt, MWD. 'Blooming' in the gut: how dysbiosis might contribute to pathogen evolution. Nat Rev Microbiol. (2013) 11:277–84. doi: 10.1038/nrmicro2989
82. Rosario, DG, Bidkhori, S, Lee, J, Bedarf, F, Hildebrand, E, Le Chatelier, M, et al. Systematic analysis of gut microbiome reveals the role of bacterial fo late and homocysteine metabolism in Parkinson's disease. Cell Rep. 34:108807. doi: 10.1016/j.celrep.2021.108807
83. Wang, DY, He, K, Liu, S, Deng, Y, and Liu, FY. Sodium Humate alleviates Enterotoxigenic Escherichia coli-induced intestinal dysfunction via alteration of intestinal microbiota and metabolites in mice. Front Microbiol. (2022) 13:809086. doi: 10.3389/fmicb.2022.809086
84. Cao, RRP, and Lei, HSF. Novel microbiota-related gene set enrichment analysis identified osteoporosis associated gut microbiota from autoimmune diseases. J Bone Miner Metab. (2021) 39:984–96. doi: 10.1007/s00774-021-01247-w
85. Ivert, LUCF, Wahlgren, B, Dal Lindelöf, H, Bradley, M, and Johansson, EK. Association between atopic dermatitis and autoimmune diseases: a population-based case-control study. Br J Dermatol. (2021) 185:335–42. doi: 10.1111/bjd.19624
86. Suzuki, TAM, Phifer-Rixey, KL, Mack, MJ, Sheehan, D, Lin, K, and Nachman, BMW. Host genetic determinants of the gut microbiota of wild mice. Mol Ecol. (2019) 28:3197–207. doi: 10.1111/mec.15139
87. Asquith, MJO, Boulard, F, and Maloy, PKJ. Pathogenic and protective roles of MyD88 in leukocytes and epithelial cells in mouse models of inflammatory bowel disease. Gastroenterology. (2010) 139:519–29. doi: 10.1053/j.gastro.2010.04.045
Keywords: gut flora, gut microbiota, atopic dermatitis, Mendelian randomization, single nucleotide polymorphisms
Citation: Xue Y, Zhang L, Chen Y, Wang H and Xie J (2023) Gut microbiota and atopic dermatitis: a two-sample Mendelian randomization study. Front. Med. 10:1174331. doi: 10.3389/fmed.2023.1174331
Edited by:
Robert Gniadecki, University of Alberta, CanadaReviewed by:
Daria Kashtanova, Centre for Strategic Planning, RussiaAna Lucia Carvalho, University of Liverpool, United Kingdom
Copyright © 2023 Xue, Zhang, Chen, Wang and Xie. This is an open-access article distributed under the terms of the Creative Commons Attribution License (CC BY). The use, distribution or reproduction in other forums is permitted, provided the original author(s) and the copyright owner(s) are credited and that the original publication in this journal is cited, in accordance with accepted academic practice. No use, distribution or reproduction is permitted which does not comply with these terms.
*Correspondence: Han Wang, wanghan@swjtu.edu.cn; Jiang Xie, xiejiang@swjtu.edu.cn
†These authors have contributed equally to this work and share first authorship