Global research of artificial intelligence in strabismus: a bibliometric analysis
- 1Eye Center, The Second Affiliated Hospital, School of Medicine, Zhejiang University, Zhejiang Provincial Key Laboratory of Ophthalmology, Zhejiang Provincial Clinical Research Center for Eye Diseases, Zhejiang Provincial Engineering Institute on Eye Diseases, Hangzhou, China
- 2Institute for Research in Ophthalmology, Foundation for Ophthalmology Development, Poznan, Poland
Purpose: To analyze the global publications on artificial intelligence (AI) in strabismus using a bibliometric approach.
Methods: The Web of Science Core Collection (WoSCC) database was used to retrieve all of the publications on AI in strabismus from 2002 to 2023. We analyzed the publication and citation trend and identified highly-cited articles, prolific countries, institutions, authors and journals, relevant research domains and keywords. VOSviewer (software) and Bibliometrix (package) were used for data analysis and visualization.
Results: By analyzing a total of 146 relevant publications, this study found an overall increasing trend in the number of annual publications and citations in the last decade. USA was the most productive country with the closest international cooperation. The top 3 research domains were Ophthalmology, Engineering Biomedical and Optics. Journal of AAPOS was the most productive journal in this field. The keywords analysis showed that “deep learning” and “machine learning” may be the hotspots in the future.
Conclusion: In recent years, research on the application of AI in strabismus has made remarkable progress. The future trends will be toward optimized technology and algorithms. Our findings help researchers better understand the development of this field and provide valuable clues for future research directions.
1. Introduction
Strabismus, featured by misalignment of the eyes, is one of the most common ocular diseases (1). It can occur at all ages, while children are diagnosed more commonly, with a prevalence ranging from 2.40 to 5.65% (2–6). For young children, it is typical that strabismus occurs spontaneously, probably stemming from inheritance, low birth rate, prematurity and etc. (7). Individuals suffering from strabismus later in life may have gone through head injury, stroke, eye muscle damage during surgery or developed diseases, such as Graves’ disease, diabetes and myasthenia gravis (1, 8, 9). Traditionally, the diagnosis of strabismus requires for specialized examinations like the cover and uncover test, the Hirschberg test and the alternate prism cover test conducted by ophthalmologists (3, 10). In addition to resulting in amblyopia and irreversible vision loss, strabismus plays a significant role in developing mental illness and reducing quality of life (11–13). Therefore, conducting researches on strabismus is of great significance.
Known as the fourth industrial revolution in the history of humanity (14), artificial intelligence (AI) is an incredibly hot topic, referring to the area of computer science devoting to creating machine that can undertake behaviors that humans consider intelligent (15). In the field of medicine, AI has its unique advantages in medical imaging analysis (16). It is AI technology that enables computers and systems to obtain useful information from digital images, videos and other visual inputs and carry out analysis (17, 18). Since ocular images have vital clinical implications, ophthalmology has always been in a leading position in artificial intelligence and technological applications (19). And strabismus is a suitable field for the application of AI technology because a significant portion of investigations are image-based. A certain number of researches concerning automatic strabismus diagnosis, quantitative measurement and other relevant achievements have been reported.
Bibliometric analysis is a statistical method to exhibit previous research achievements and identify research hotspots by quantitatively assess the research status of countries, institutions, authors, journals, which cannot be replaced by other methods including traditional reviews, meta-analyses (20, 21). It has been applied in numerous subjects and disciplines, such as economics, agriculture, engineering, medicine and so forth (22–25). Bibliometrics has been commonly utilized in analyzing scientific publications on AI in ophthalmology, such as glaucoma, diabetic retinopathy and macular edema (26–28). However, no bibliometric analysis of AI in strabismus has been conducted before. Hence, this is the first study aiming to present an overview regarding the global publications of AI in strabismus and to provide a prediction of future research directions in this field.
2. Materials and methods
2.1. Data collection
Based on the recommendation of collecting bibliometric data from single database (20), we used Web of Science Core Collection (WoSCC) as the research database, which is the most commonly used and approved database for bibliometric analysis. To search for relevant data, at least one keyword related to strabismus and at least one keyword related to AI were combined to form query formulation. The detailed query formulation was described as follows: TS = (AI OR “artificial intelligence” OR intelligent OR “data learning” OR “robotic*” OR “computer vision” OR “machine learning” OR “deep learning” OR “deep network*” OR “neural learning” OR automat* OR algorithm OR “neural network*” OR “expert* system*”) AND TS = (strabismus OR esotropia OR exotropia OR hypertropia OR hypotropia OR heterotropia OR esophoria OR exophoria OR hyperphoria OR hypophoria OR heterophoria OR “dissociated vertical deviation” OR “dissociated horizontal deviation” OR “dissociated torsional deviation” OR “[(eye OR ocular) AND (motility OR movement*) AND disorder*]” OR “[(eye OR ocular) AND (alignment OR deviation)]” OR “media rectus” OR “lateral rectus” OR “superior rectus” OR “inferior rectus” OR “superior oblique” OR “inferior oblique” OR “third nerve” OR “fourth nerve” OR “sixth nerve” OR “abducens nerve” OR “oculomotor nerve” OR “trochlear nerve”). The time span was from January 1, 2002 to March 31, 2023, and the document types were limited to articles, reviews and proceedings papers. The last search was conducted on April 29, 2023. A total of 280 retrieved documents were prepared for the following screening.
2.2. Data screening
To exclude irrelevant documents in retrieved documents, we set the practical inclusion criteria as follows: (i) involvement of AI technology, including deep learning, machine learning and automated devices; (ii) involvement of strabismus, including: (1) researches focused on strabismus; (2) researches focused on the characterized clinical features of strabismus; (3) researches focused on multiple diseases and strabismus. After reading the titles and abstracts of each document carefully, 148 documents were included for the bibliometric analysis.
2.3. Data analysis and visualization
Publications and citations of each year, countries, institutions, authors, journals, research domain and H-index were acquired from WoSCC. H-index, as a reference index, indicates the impact of a researcher, country, institution, journal on the development of the certain scientific field (29, 30). The WoSCC intrinsic toolkits help to analyze general features mentioned above. Microsoft Excel 2019 was used to conduct polynomial regression analysis and to export charts and tables of publications, top-cited documents, productive countries, institutions, authors and journals, and hot research domain. The average growth rate of publications was calculated as follows:
Where : first year; : last year; : publication count of the first year; : publication count of the last year.
We used VOSviewer (software, version 1.6.19) (31) to visualize the collaboration of countries, institutions, authors and co-occurrence of keywords, and calculate the total link strength. Bibliometrix (package, version 4.1.2) of R (programming language, version 4.3.0) (32) was applied to create WordCloud of keywords and calculate fractionalized frequency of an author which reflect one’s contribution to the publications. Fractionalized frequency was calculated as follows:
Where : the set of documents co-authored by the author j; h: a document included in .
3. Results
3.1. Analysis of publications and citations
On the basis of search strategy and inclusion criteria, we included 146 documents (Figure 1), including 110 articles, 26 proceedings and 10 reviews. The detailed publication numbers of different document types during 2002 and 2023 were shown in Supplementary Table S1. And Supplementary Figure S1 illustrated the annual trends of publications and citations on AI in strabismus from 2002 to 2023. From 2002 to 2012, the number of publications was no more than 5 documents per year, seeming to be relatively small. Since 2013, there has been an overall rising trend and at the year of 2021 the number of publications per year approached the peak value of 25. The average growth rate from 2002 to 2022 was 16.71%. Especially the last 5 years (2018–2022), the research has developed rapidly, contributing 59.12% (81/137) of all documents from 2002 to 2022. The total citations were up to 1,222 times, and average citations per item and H-index were 8.37 and 18, respectively. To model the publication and citation trends, polynomial regression analysis was conducted (2023 was excluded due to incomplete indexing). The fitted curves of y1 = −2E-05x6 + 0.0013x5–0.0332x4 + 0.3959x3 – 2.2868x2 + 5.8559x − 2.627(R2 = 0.8423) and y2 = −0.0003x6 + 0.0212x5 – 0.498x4 + 5.5629x3 – 29.853x2 + 70.569x − 50.265(R2 = 0.9642) indicated changes in the quantities of publications and citations with time, respectively. The trend of citations showed a similar tendency as publications, indicating AI in strabismus drew researchers’ attention in the last decade and is in the developing phase.
Additionally, we listed the top 10 documents ranked by annual citations count in Table 1. There were 7 articles, 2 reviews and a proceedings paper. Eight documents were published in the last 5 years. The earliest document, also the most impactful one, was by Donahue et al. (33). It drew up guidelines for automated preschool vision screening, including the identification of children with strabismus. The Pediatric Vision Scanner, detecting the absence of foveal fixation as a harbinger of strabismus and amblyopia, was regarded as a potentially recommended instrument. The top 10 documents ranked by total citations count were also listed in Table 1. Four articles and 1 proceedings paper published before 2014 were newly on the list.
3.2. Analysis of top productive countries and collaboration networks of countries
A total of 28 countries had published related research on this topic. Countries ranked by publication count were listed in the Table 2. Accounting for 35.62% (52/146) of included documents, USA was the most productive country with the most publications and the highest citations (687 times) and H-index (15). According to publication count, China (29/146, 19.86%) was the second leading country, followed by South Korea (11/146, 7.53%). When it came to collaboration networks of countries, we visualized it by means of VOSviewer (Supplementary Figure S2). The number of publications determined the size of the circle and the lines between circles stood for the co-authorship between countries. The thickness of lines indicated the strength of cooperation (termed total link strength). USA had the highest total link strength, followed by England and China. Seventeen countries with cooperative relationships were presented, while 11 countries lacking international cooperation were not shown in Supplementary Figure S2, like South Korea, Brazil etc.
3.3. Analysis of top productive institutions and collaboration networks of institutions
There were 248 institutions participating in related research. Table 2 summarized institutions ranked by publication count. Johns Hopkins University had the greatest contribution with 11 documents in total, followed by Vanderbilt University, Universidade Federal do Maranhao and Seoul National University, each of which published 6 documents. Although the number of documents was inferior to Johns Hopkins University, Vanderbilt University had the highest citations. Figure 2 visualized the co-authorship network of institutions. While some institutions were not connected to each other, Figure 2 only demonstrated the largest set of connected items consisting of 48 items. University College London had the highest total link strength, followed by Moorfields Eye Hospital and Harvard Medical School. Nevertheless, the majority of institutions were dispersive and lack of cooperation (Supplementary Figure S3).
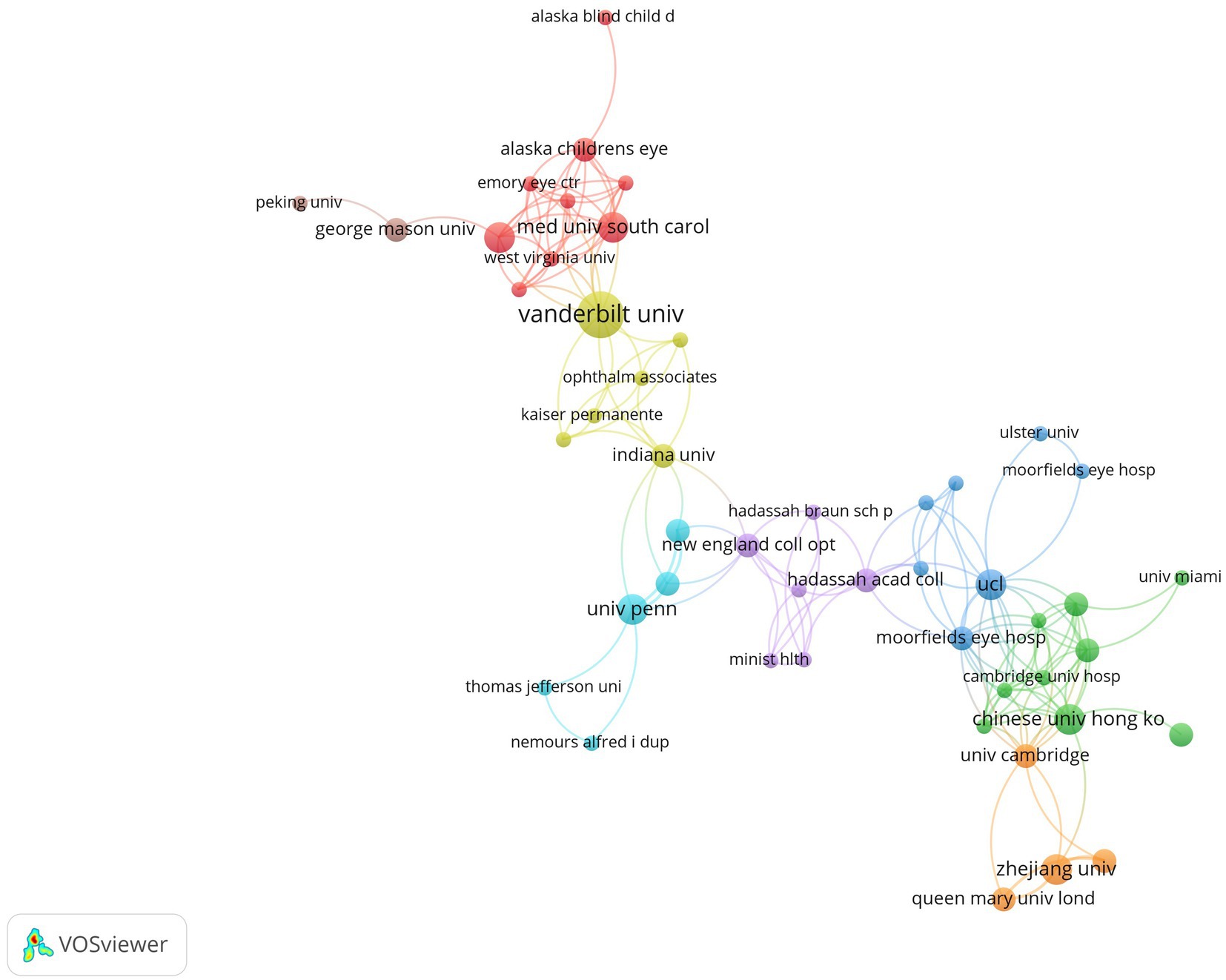
Figure 2. Co-authorship network visualization map of institutions. Each node represents one institution. The number of publications determines the size of the circle. Connecting lines represent collaboration between institutions.
3.4. Analysis of top productive authors and collaboration networks of authors
Top productive authors were listed in Table 3 and the collaboration relationship among authors were displayed in Figure 3. Because some authors were not connected to each other, the largest set of connected items consisting of 30 authors were visualized. According to Figure 3, we could see that authors from the same country were more likely to have close cooperation.
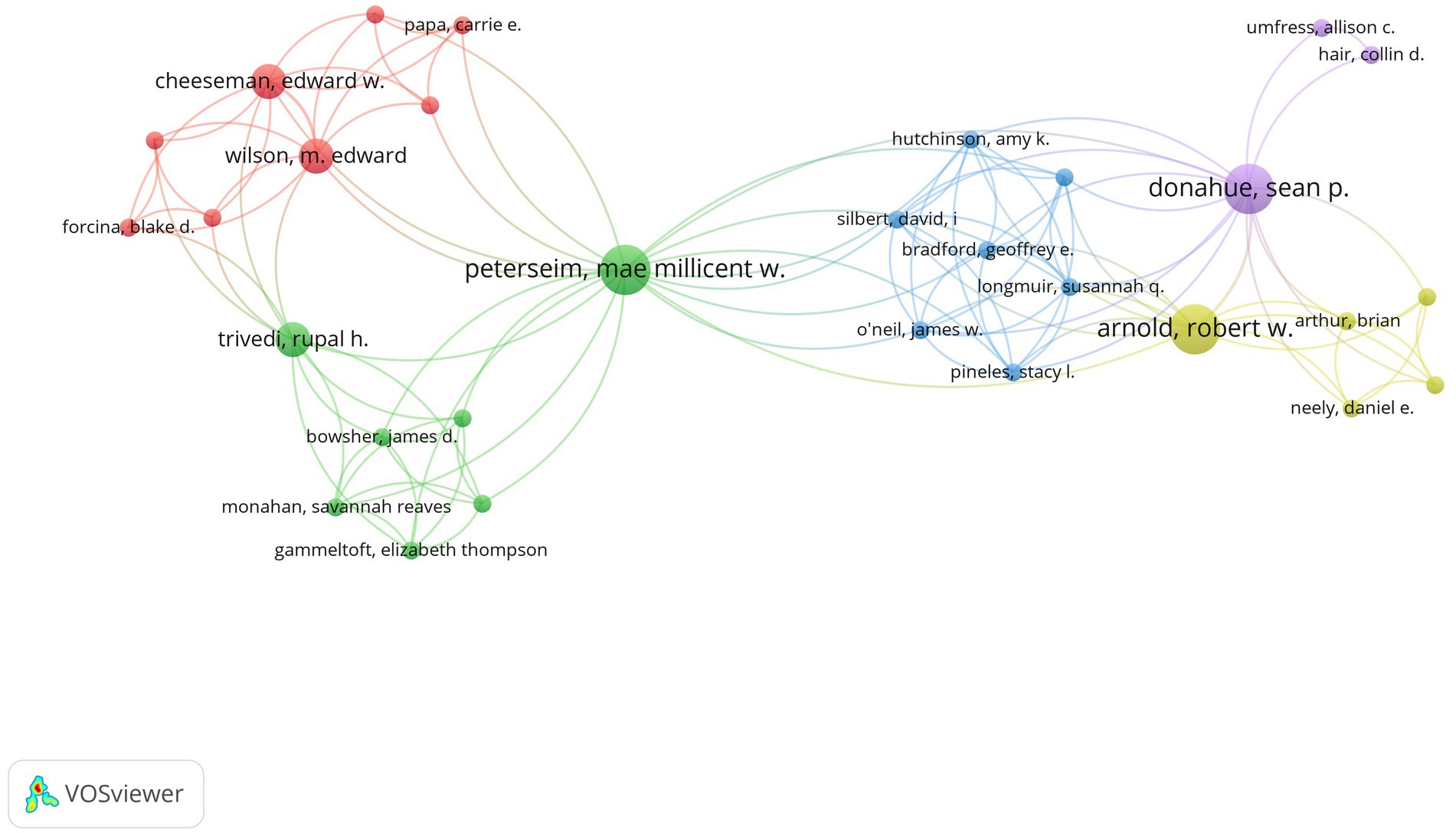
Figure 3. Co-authorship network visualization map of authors. Each node represents one author. The number of publications determines the size of the circle. Connecting lines represent collaboration between authors.
3.5. Analysis of research domains and top productive journals
The 10 most common research domains were listed in Table 4. The top 3 research domains were Ophthalmology (59/146, 40.41%), Engineering Biomedical (21/146, 14.38%), and Optics (12/146, 8.22%).
Top 10 productive journals were listed in Table 5. To evaluate the academic influence of journals, we consulted impact factor (IF) and the Journal Citation Reports (JCR) (2021) assessment system. Among these journals, JAMA Ophthalmology had the highest IF with 8.253. Journal of AAPOS (IF: 1.325, Q4) had the most publications and highest citations. Four journals ranked Q1 in JCR (2021), three journals ranked Q2, two journals ranked Q3 and one journal ranked Q4.
3.6. Analysis of keywords
In order to have a better understanding of research orientation and prospect, we conducted a keyword co-occurrence analysis by VOSviewer. For the total of 663 automatically identified keywords, 40 keywords occurred at least 4 times, which were displayed in Figure 4. All included keywords were divided into 5 clusters, indicated by red, yellow, blue, green, and purple colors, representing the diagnosis of strabismus (e.g., “cover test”, “hirschberg test”, “recognition”, etc.), diseases related to strabismus (e.g., “graves ophthalmopathy”, “diplopia”, etc.), terms related to AI (e.g., “machine learning”, “deep learning”, etc.), terms related to epidemiology (e.g., “children”, “prevalence”, “risk factors”, etc.), and technical key point (e.g., “automated detection”, “vision screening”, etc.).
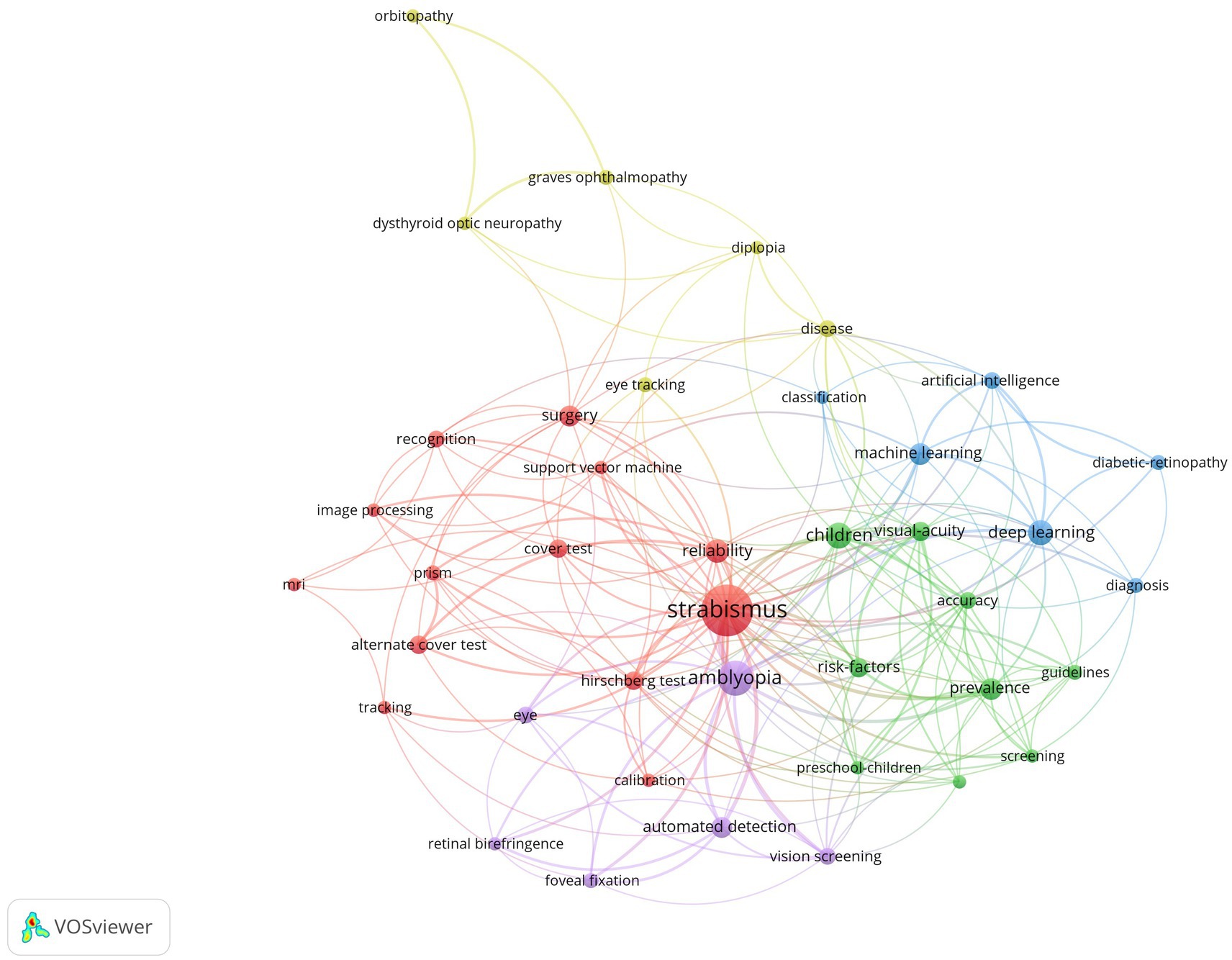
Figure 4. The co-occurrence map of keywords. It reveals 5 clusters (in 5 colors): the diagnosis of strabismus (in red), diseases related to strabismus (in yellow), terms related to AI (in blue), terms related to epidemiology (in green) and technical key point (in purple). Each node represents one keyword. The number of occurrences determines the size of the circle. Connecting lines represent co-occurrence between different keywords.
To understand when these hotspots emerged and how they evolved, we divided the documents into 3 group based on publication count and publication time: (i) 2002–2012 (during this period the academic output was relatively small, so time interval was extended); (ii) 2013–2017; (iii) 2018–2023. Three corresponding WordCloud of keywords (Supplementary Figure S4) were conducted by Bibiometrix Package in R. The more frequently-occurred, the bigger scale. “Strabismus” was the most dominant keyword for the entire period, followed by “amblyopia”. During 2002 and 2012, “polarization optics”, “vision screening” was ascendent. During 2013 and 2017, “retina birefringence” and “fixation detection” were more prevailing compared with “artificial neural networks”. During 2018 and 2023, “deep learning” and “machine learning” were dominant.
4. Discussion
In this study, we performed a bibliometric analysis to explore the features of the publications concerning the application of AI in strabismus from 2002 to 2023 and gave a comprehensive look at this research trends for the first time.
The production and growth rates of the scientific papers reflect how a particular subject of study is progressing. There was a relatively slow stage of development from 2002 to 2012. The number of publications during this period accounted for 18.49% (27/146) of the total. Then, there has been an overall rising tendency since 2013. Especially the last 5 years (2018–2022), the research has developed rapidly, contributing 59.12% (81/137) of all articles from 2002 to 2022. The advancements in AI technology and its use in ophthalmology were primarily the reason for the developing trend. The most influential document was guideline for automated preschool vision screening by Donahue et al. (33). Its high citations were closely related to its guiding value. And other impactful documents were focused on aspects including strabismus recognition using eye-tracking data and convolutional neural networks, instrument-based vision screen and eye alignment measurement using smartphone app (34–37, 39, 40, 44, 47).
USA, China and South Korea were the main forces in researches concerning the application of AI in strabismus. USA was the most productive country with the most publications and the highest citations and H-index. A significant gap between the number of publications of USA and China was noticed, while China ranked second in the number of publications. This was intimately tied to the fact that USA had the most advanced research equipment and a large number of scientific researchers in the whole world. Meanwhile, USA had the closest international cooperation, while other countries such as South Korea and Brazil sustained high scientific productions with poor international collaboration. Publications from USA in recent years inclined to study new screening devices which were able to detect amblyopia risk factors or directly detect strabismus (48, 49). Publications from China brought more focus on deep learning-based image analysis. Zhang et al. (50) proposed multi-feature fusion model which achieved an accuracy of 97.17%, sensitivity of 96.06%, specificity of 97.79%, and AUC of 0.969 in detecting strabismus. Shi and Tang (51) proposed a multitask deep learning model based on deep snake to improve strabismus iris recognition in complicated scenes. Publications from South Korea also paid more attention to automated detection of strabismus (52, 53).
Johns Hopkins University had excellent academic reputation and played a dominant role in this field. Research groups from here developed and reported a pediatric vision screener which was an efficient tool for detecting central fixation based on retinal birefringence scanning, and Gramatikov and Guyton did dedicate themselves to this research direction and exert great influence (54, 55). Researchers from Vanderbilt University which had the highest citations among all institutions, sought to a novel automated method of CT metrics of extraocular muscle in thyroid eye disease (56, 57) and made contribution to automated vision screening guidelines (33, 35). However, the majority of institutions were dispersive and lack of cooperation. It is crucial that the cooperation among countries and institutions needs to be reinforced. Authors from Brazil, including Jo o Dallyson Sousa de Almeida, Jorge Antonio Meireles Teixeira and Aristófanes Correa Silva, devoted themselves to researching the diagnosis of strabismus based on digital videos and surgical planning for horizontal strabismus by Support Vector Regression (58–60).
The top 3 research domains were Ophthalmology, Engineering Biomedical and Optics. Journal of AAPOS was ranked the first in the most productive journal. By analyzing keywords, “deep learning” and “machine learning” may be the hotspots in the near future. Meanwhile, “screening” and “smartphone app” had been the common interests of researchers. This may be due to the fact that the diagnosis of strabismus was important in clinical procedure and it was image-dependent which was suitable for AI technology to handle. AI-based algorithms can extract characteristics from ocular images to construct models to facilitate diagnosis and quantitively assessment.
By analyzing recent publications, the latest advances were manifested in different ways. When it came to screening devices, Monahan et al. (49) held the view that the blinq™ Vision Screener was suitable for detecting strabismus in children with sensitivity of 87.5% and specificity of 51.3%. As for new algorithms, Huang et al. (61) proposed a method that could automatically identify the oculomotor nerve from dMRI tractography and Xie et al. (62) put forward a novel multimodal deep-learning-based multi-class network for automated cranial nerves tract segmentation called CNTSeg, which would be beneficial to the diagnosis of incomitant strabismus. Lou et al. (63) proposed a novel deep learning-based approach to automatically evaluate the amount of inferior oblique overaction from frontal facial images via GAR2U-Net.
With the prosperity of AI technology, the development direction is changing from digital devices [like photoscreener, eye trackers, virtual reality headsets (39, 44, 64)] to automatic screening methods, which is more economical and practical in undeveloped areas. In the future, optimizing algorithms and proposing new techniques will become the focus of this field. For one thing, with the development of instrument-based vision screening technology, promoting the application of efficient and economical devices in strabismus screening is an important topic. For another, as artificial intelligence is racing ahead, it is vital to develop segmentation algorithms with higher accuracy and models with better performance. Also, an automated integrated platform with functions such as diagnosis, evaluation and postoperative prediction is highly expected.
Compared with AI applications in diabetic retinopathy, glaucoma and other eye diseases, AI applications in strabismus was relatively fewer. It may be because of the following reasons. On the one hand, the dynamic tests are required during the diagnosis of strabismus while a static fundus photograph is of great value for the diagnosis of eye diseases like diabetic retinopathy. On the other hand, public large-scale datasets of strabismus are rare, which is inconvenient for academic groups worldwide to test their models and algorithms. As a result, it is essential to grasp the specific characteristics of strabismus and promote the establishment of public large-scale datasets so as to provide opportunity for researchers worldwide to train their models. Research on the application of AI in strabismus has made significant progress in recent years, but there is still a distance between the current AI in strabismus and the clinical practice. We anticipate a more effective use of artificial intelligence in strabismus.
There are several limitations of the study. First, we only retrieve data from WoSCC. Other databases like Pubmed, Embase, and Scopus were not obtained. Secondly, our query formulation may not be perfect enough to retrieve all publications in the research field. Third, when analyzing keywords, similar keywords like “image processing” and “image processing techniques” were not merged.
5. Conclusion
This study presented an overview regarding the global researches of AI in strabismus. It was the first bibliometric analysis of this field. Our findings help researchers better understand the development of this field and provide valuable clues for future research directions. Research on the application of AI in strabismus has made remarkable progress in recent years. The future trends will be toward optimized technology and algorithms.
Data availability statement
The original contributions presented in the study are included in the article/Supplementary material, further inquiries can be directed to the corresponding authors.
Author contributions
ZZ, XZ, XT, JY, and LL: conception and design. JY and LL: administrative support. ZZ and LL: collection and assembly of data. ZZ, XZ, AG, and LL: data analysis and interpretation. All authors contributed to the article and approved the submitted version.
Funding
This work was supported by National Natural Science Foundation of China [No. 82000948 to LL, No. U20A20386 to JY, No. 81870635 to JY]; National Key Research and Development Program of China [No. 2019YFC0118400 to JY]; and Zhejiang Provincial Key Research and Development Plan [No. 2019C03020 to JY].
Conflict of interest
The authors declare that the research was conducted in the absence of any commercial or financial relationships that could be construed as a potential conflict of interest.
Publisher’s note
All claims expressed in this article are solely those of the authors and do not necessarily represent those of their affiliated organizations, or those of the publisher, the editors and the reviewers. Any product that may be evaluated in this article, or claim that may be made by its manufacturer, is not guaranteed or endorsed by the publisher.
Supplementary material
The Supplementary material for this article can be found online at: https://www.frontiersin.org/articles/10.3389/fmed.2023.1244007/full#supplementary-material
References
2. Chen, X, Fu, Z, Yu, J, Ding, H, Bai, J, Chen, J, et al. Prevalence of amblyopia and strabismus in eastern China: results from screening of preschool children aged 36–72 months. Br J Ophthalmol. (2016) 100:515–9. doi: 10.1136/bjophthalmol-2015-306999
3. Hull, S, Tailor, V, Balduzzi, S, Rahi, J, Schmucker, C, Virgili, G, et al. Tests for detecting strabismus in children aged 1 to 6 years in the community. Cochrane Database Syst Rev. (2017) 2017:CD011221. doi: 10.1002/14651858.CD011221.pub2
4. McKean-Cowdin, R, Cotter, SA, Tarczy-Hornoch, K, Wen, G, Kim, J, Borchert, M, et al. Prevalence of amblyopia or strabismus in Asian and non-Hispanic white preschool children: multi-ethnic pediatric eye disease study. Ophthalmology. (2013) 120:2117–24. doi: 10.1016/j.ophtha.2013.03.001
5. Multi-ethnic Pediatric Eye Disease Study Group. Prevalence of amblyopia and strabismus in African American and Hispanic children ages 6 to 72 months: the multi-ethnic pediatric eye disease study. Ophthalmology. (2008) 115:1229–1236.e1. doi: 10.1016/j.ophtha.2007.08.001
6. Robaei, D, Rose, KA, Kifley, A, Cosstick, M, Ip, JM, and Mitchell, P. Factors associated with childhood strabismus: findings from a population-based study. Ophthalmology. (2006) 113:1146–53. doi: 10.1016/j.ophtha.2006.02.019
7. Maconachie, GDE, Gottlob, I, and McLean, RJ. Risk factors and genetics in common comitant strabismus: a systematic review of the literature. JAMA Ophthalmol. (2013) 131:1179–86. doi: 10.1001/jamaophthalmol.2013.4001
8. Schiavi, C. Paralytic strabismus. Curr Opin Ophthalmol. (1995) 6:22–6. doi: 10.1097/00055735-199510000-00005
9. VIS group UK, and Rowe, F. The profile of strabismus in stroke survivors. Eye. (2010) 24:682–5. doi: 10.1038/eye.2009.138
10. Choi, RY, and Kushner, BJ. The accuracy of experienced strabismologists using the Hirschberg and Krimsky tests. Ophthalmology. (1998) 105:1301–6. doi: 10.1016/S0161-6420(98)97037-3
11. Lee, YH, Repka, MX, Borlik, MF, Velez, FG, Perez, C, Yu, F, et al. Association of strabismus with mood disorders, schizophrenia, and anxiety disorders among children. JAMA Ophthalmol. (2022) 140:373–81. doi: 10.1001/jamaophthalmol.2022.0137
12. McBain, HB, Au, CK, Hancox, J, MacKenzie, KA, Ezra, DG, Adams, GGW, et al. The impact of strabismus on quality of life in adults with and without diplopia: a systematic review. Surv Ophthalmol. (2014) 59:185–91. doi: 10.1016/j.survophthal.2013.04.001
13. Mohney, BG, McKenzie, JA, Capo, JA, Nusz, KJ, Mrazek, D, and Diehl, NN. Mental illness in young adults who had strabismus as children. Pediatrics. (2008) 122:1033–8. doi: 10.1542/peds.2007-3484
14. Ting, DSW, Pasquale, LR, Peng, L, Campbell, JP, Lee, AY, Raman, R, et al. Artificial intelligence and deep learning in ophthalmology. Br J Ophthalmol. (2019) 103:167–75. doi: 10.1136/bjophthalmol-2018-313173
15. Russell, S, and Norvig, P. Artificial intelligence: A modern approach. 2nd edn. Upper Saddle River, New Jersey: Prentice Hall (2002).
16. Yu, K-H, Beam, AL, and Kohane, IS. Artificial intelligence in healthcare. Nat Biomed Eng. (2018) 2:719–31. doi: 10.1038/s41551-018-0305-z
17. Kermany, DS, Goldbaum, M, Cai, W, Valentim, CCS, Liang, H, Baxter, SL, et al. Identifying medical diagnoses and treatable diseases by image-based deep learning. Cells. (2018) 172:1122–1131.e9. doi: 10.1016/j.cell.2018.02.010
18. Li, J-PO, Liu, H, Ting, DSJ, Jeon, S, Chan, RVP, Kim, JE, et al. Digital technology, tele-medicine and artificial intelligence in ophthalmology: a global perspective. Prog Retin Eye Res. (2021) 82:100900. doi: 10.1016/j.preteyeres.2020.100900
19. Jin, K, and Ye, J. Artificial intelligence and deep learning in ophthalmology: current status and future perspectives. Adv Ophthalmol Pract Res. (2022) 2:100078. doi: 10.1016/j.aopr.2022.100078
20. Donthu, N, Kumar, S, Mukherjee, D, Pandey, N, and Lim, WM. How to conduct a bibliometric analysis: an overview and guidelines. J Bus Res. (2021) 133:285–96. doi: 10.1016/j.jbusres.2021.04.070
21. Zhang, D, Zhu, W, Guo, J, Chen, W, and Gu, X. Application of artificial intelligence in glioma researches: a bibliometric analysis. Front Oncol. (2022) 12:978427. doi: 10.3389/fonc.2022.978427
22. Fahimnia, B, Sarkis, J, and Davarzani, H. Green supply chain management: a review and bibliometric analysis. Int J Prod Econ. (2015) 162:101–14. doi: 10.1016/j.ijpe.2015.01.003
23. Faust, O, Hagiwara, Y, Hong, TJ, Lih, OS, and Acharya, UR. Deep learning for healthcare applications based on physiological signals: a review. Comput Methods Prog Biomed. (2018) 161:1–13. doi: 10.1016/j.cmpb.2018.04.005
24. Giraldo, P, Benavente, E, Manzano-Agugliaro, F, and Gimenez, E. Worldwide research trends on wheat and barley: a bibliometric comparative analysis. Agronomy. (2019) 9:352. doi: 10.3390/agronomy9070352
25. Mao, G, Zou, H, Chen, G, Du, H, and Zuo, J. Past, current and future of biomass energy research: a bibliometric analysis. Renew Sust Energ Rev. (2015) 52:1823–33. doi: 10.1016/j.rser.2015.07.141
26. Feng, H, Chen, J, Zhang, Z, Lou, Y, Zhang, S, and Yang, W. A bibliometric analysis of artificial intelligence applications in macular edema: exploring research hotspots and Frontiers. Front Cell Dev Biol. (2023) 11:1174936. doi: 10.3389/fcell.2023.1174936
27. Saeed, AQ, Sheikh Abdullah, SNH, Che-Hamzah, J, and Abdul Ghani, AT. Accuracy of using generative adversarial networks for Glaucoma detection: systematic review and bibliometric analysis. J Med Internet Res. (2021) 23:e27414. doi: 10.2196/27414
28. Shao, A, Jin, K, Li, Y, Lou, L, Zhou, W, and Ye, J. Overview of global publications on machine learning in diabetic retinopathy from 2011 to 2021: bibliometric analysis. Front Endocrinol. (2022) 13:1032144. doi: 10.3389/fendo.2022.1032144
29. Hirsch, JE, and Buela-Casal, G. The meaning of the h-index. Int J Clin Health Psychol. (2014) 14:161–4. doi: 10.1016/S1697-2600(14)70050-X
30. Lu, A, Liu, Z, Su, G, and Yang, P. Global research status regarding uveitis in the last decade. Ocul Immunol Inflamm. (2023) 1–10:1–10. doi: 10.1080/09273948.2023.2170251
31. Van Eck, NJ, and Waltman, L. Software survey: VOSviewer, a computer program for bibliometric mapping. Scientometrics. (2010) 84:523–38. doi: 10.1007/s11192-009-0146-3
32. Aria, M, and Cuccurullo, C. Bibliometrix: an R-tool for comprehensive science mapping analysis. J Informet. (2017) 11:959–75. doi: 10.1016/j.joi.2017.08.007
33. Donahue, SP, Arthur, B, Neely, DE, Arnold, RW, Silbert, D, and Ruben, JB. Guidelines for automated preschool vision screening: a 10-year, evidence-based update. J Am Assoc Pediatr Ophthalmol Strabismus. (2013) 17:4–8. doi: 10.1016/j.jaapos.2012.09.012
34. Chen, Z, Fu, H, Lo, WL, and Chi, Z. Strabismus recognition using eye-tracking data and convolutional neural networks. J Healthcare Eng. (2018) 2018:1–9. doi: 10.1155/2018/7692198
35. Arnold, RW, Donahue, SP, Silbert, DI, Longmuir, SQ, Bradford, GE, Peterseim, MMW, et al. AAPOS uniform guidelines for instrument-based pediatric vision screen validation 2021. J Am Assoc Pediatr Ophthalmol Strabismus. (2022) 26:1.e1–6. doi: 10.1016/j.jaapos.2021.09.009
36. Chen, ZH, Fu, H, Lo, WL, Chi, Z, and Xu, B. Eye-tracking-aided digital system for strabismus diagnosis. Healthcare Technol Lett. (2018) 5:1–6. doi: 10.1049/htl.2016.0081
37. Pundlik, S, Tomasi, M, Liu, R, Houston, K, and Luo, G. Development and preliminary evaluation of a smartphone app for measuring eye alignment. Trans Vis Sci Tech. (2019) 8:19. doi: 10.1167/tvst.8.1.19
38. Reid, JE, and Eaton, E. Artificial intelligence for pediatric ophthalmology. Curr Opin Ophthalmol. (2019) 30:337–46. doi: 10.1097/ICU.0000000000000593
39. Miao, Y, Jeon, JY, Park, G, Park, SW, and Heo, H. Virtual reality-based measurement of ocular deviation in strabismus. Comput Methods Prog Biomed. (2020) 185:105132. doi: 10.1016/j.cmpb.2019.105132
40. Yehezkel, O, Belkin, M, and Wygnanski-Jaffe, T. Automated diagnosis and measurement of strabismus in children. Am J Ophthalmol. (2020) 213:226–34. doi: 10.1016/j.ajo.2019.12.018
41. Ji, Y, Wang, S, Lu, Y, Wei, J, and Zhao, Y. Eye and mouth state detection algorithm based on contour feature extraction. J Electron Imag. (2018) 27:1. doi: 10.1117/1.JEI.27.5.051205
42. Strianese, D. Update on graves disease: advances in treatment of mild, moderate and severe thyroid eye disease. Curr Opin Ophthalmol. (2017) 28:505–13. doi: 10.1097/ICU.0000000000000402
43. Ben Simon, GJ, Syed, AM, Lee, S, Wang, DY, Schwarcz, RM, McCann, JD, et al. Strabismus after deep lateral wall orbital decompression in thyroid-related orbitopathy patients using automated Hess screen. Ophthalmology. (2006) 113:1050–5. doi: 10.1016/j.ophtha.2006.02.015
44. Schaeffel, F. Kappa and Hirschberg ratio measured with an automated video gaze tracker. Optom Vis Sci. (2002) 79:329–34. doi: 10.1097/00006324-200205000-00013
45. Han, SJ, Guo, Y, Granger-Donetti, B, Vicci, VR, and Alvarez, TL. Quantification of heterophoria and phoria adaptation using an automated objective system compared to clinical methods: quantification of heterophoria. Ophthalmic Physiol Opt. (2010) 30:95–107. doi: 10.1111/j.1475-1313.2009.00681.x
46. Hunter, DG, Nassif, DS, Piskun, NV, Winsor, R, Gramatikov, BI, and Guyton, DL. Pediatric vision screener 1: instrument design and operation. J Biomed Opt. (2004) 9:1363–8. doi: 10.1117/1.1805560
47. Ransbarger, KM, Dunbar, JA, Choi, SE, and Khazaeni, LM. Results of a community vision-screening program using the spot photoscreener. J Ame Assoc Pediatr Ophthalmol Strabismus. (2013) 17:516–20. doi: 10.1016/j.jaapos.2013.06.013
48. Arnold, RW. Comparative validation of PlusoptiX and AI-optic photoscreeners in children with high amblyopia risk factor prevalence. Clin Ophthalmol. (2022) 16:2639–50. doi: 10.2147/OPTH.S378777
49. Monahan, SR, Smith, SM, Gammeltoft, ET, Trivedi, RH, Wilson, ME, Bowsher, JD, et al. The blinq™ vision screener in detection of amblyopia and strabismus. Am J Ophthalmol. (2023) 250:20–4. doi: 10.1016/j.ajo.2023.01.013
50. Zhang, G, Xu, W, Gong, H, Sun, L, Li, C, Chen, H, et al. Multi-feature fusion-based strabismus detection for children. IET Image Process. (2023) 17:1590–602. doi: 10.1049/ipr2.12740
51. Shi, D, and Tang, H. Research on strabismus iris segmentation model based on deep snake multitask learning. J Electron Imag. (2022) 31:063018. doi: 10.1117/1.JEI.31.6.063018
52. Huang, X, Lee, SJ, Kim, CZ, and Choi, SH. An automatic screening method for strabismus detection based on image processing. PLoS One. (2021) 16:e0255643. doi: 10.1371/journal.pone.0255643
53. Lee, D, and Kim, H. Automated detection of horizontal strabismus and home control evaluation using a Mobile phone app. J Korean Ophthalmol Soc. (2022) 63:994–1000. doi: 10.3341/jkos.2022.63.12.994
54. Gramatikov, BI. Detection of central fixation using short-time autoregressive spectral estimation during retinal birefringence scanning. Med Eng Phys. (2015) 37:905–10. doi: 10.1016/j.medengphy.2015.06.007
55. Gramatikov, BI. Detecting central fixation by means of artificial neural networks in a pediatric vision screener using retinal birefringence scanning. Biomed Eng Online. (2017) 16:52. doi: 10.1186/s12938-017-0339-6
56. Chaganti, S., Nelson, K., Mundy, K., Luo, Y., Harrigan, R. L., Damon, S., et al. (2016). Structural functional associations of the orbit in thyroid eye disease: Kalman filters to track extraocular rectal muscles. In: M. A. Styner and E. D. Angelini (Eds), San Diego, California: Proceedings of SPIE--the International Society for Optical Engineering (2016). p. 97841G.
57. Law, JJ, Mundy, KM, Kupcha, AC, Chaganti, S, Nelson, KM, Harrigan, RL, et al. Correlation of automated computed tomography volumetric analysis metrics with motility disturbances in thyroid eye disease. Ophthalmic Plast Reconstr Surg. (2020) 37:372–6. doi: 10.1097/IOP.0000000000001880
58. Almeida, JDSD, Silva, AC, Teixeira, JAM, Paiva, AC, and Gattass, M. Surgical planning for horizontal strabismus using support vector regression. Comput Biol Med. (2015) 63:178–86. doi: 10.1016/j.compbiomed.2015.05.025
59. Da Costa, PB, De Almeida, JDS, Teixeira, JAM, Braz, G, De Paiva, AC, and Silva, AC. Computational method for aid in the diagnosis of sixth optic nerve palsy through digital videos. Comput Biol Med. (2022) 150:106098. doi: 10.1016/j.compbiomed.2022.106098
60. Valente, TLA, De Almeida, JDS, Silva, AC, Teixeira, JAM, and Gattass, M. Automatic diagnosis of strabismus in digital videos through cover test. Comput Methods Prog Biomed. (2017) 140:295–305. doi: 10.1016/j.cmpb.2017.01.002
61. Huang, J, Li, M, Zeng, Q, Xie, L, He, J, Chen, G, et al. Automatic oculomotor nerve identification based on data-driven fiber clustering. Hum Brain Mapp. (2022) 43:2164–80. doi: 10.1002/hbm.25779
62. Xie, L, Huang, J, Yu, J, Zeng, Q, Hu, Q, Chen, Z, et al. CNTSeg: a multimodal deep-learning-based network for cranial nerves tract segmentation. Med Image Anal. (2023) 86:102766. doi: 10.1016/j.media.2023.102766
63. Lou, L, Huang, X, Sun, Y, Cao, J, Wang, Y, Zhang, Q, et al. Automated photographic analysis of inferior oblique overaction based on deep learning. Quant Imaging Med Surg. (2023) 13:329–38. doi: 10.21037/qims-22-467
Keywords: strabismus, artificial intelligence, bibliometric analysis, global publications, keywords analysis
Citation: Zhou Z, Zhang X, Tang X, Grzybowski A, Ye J and Lou L (2023) Global research of artificial intelligence in strabismus: a bibliometric analysis. Front. Med. 10:1244007. doi: 10.3389/fmed.2023.1244007
Edited by:
Arjun Singh, Memorial Sloan Kettering Cancer Center, United StatesCopyright © 2023 Zhou, Zhang, Tang, Grzybowski, Ye and Lou. This is an open-access article distributed under the terms of the Creative Commons Attribution License (CC BY). The use, distribution or reproduction in other forums is permitted, provided the original author(s) and the copyright owner(s) are credited and that the original publication in this journal is cited, in accordance with accepted academic practice. No use, distribution or reproduction is permitted which does not comply with these terms.
*Correspondence: Lixia Lou, loulixia110@zju.edu.cn; Juan Ye, yejuan@zju.edu.cn