- 1Department of Anesthesiology, Shizhu Tujia Autonomous County People's Hospital, Chongqing, China
- 2Department of Anesthesiology, Chongqing University Cancer Hospital, Chongqing, China
Objective: The incidence of pulmonary infection following major orthopedic surgery in the elderly is high, significantly affecting prognosis. Identifying high-risk factors and stratifying patient risk more effectively is an urgent problem that needs to be addressed. This study aims to develop a nomogram for predicting postoperative pulmonary infection (PPI) in elderly patients undergoing major orthopedic surgery.
Methods: Data from preoperative variables, surgical procedures, and anesthesia factors of 814 elderly patients who underwent major orthopedic surgery between January 2020 and October 2023 were retrospectively collected to develop a nomogram. The primary outcome was PPI. Stata 16 and R 4.1.2 software were used for statistical analysis.
Results: Multivariate logistic regression revealed that gender (OR = 2.336, 95% CI1.135–4.807, p = 0.021), preoperative pulmonary disease (OR = 6.042, 95% CI 2.849–12.814, p = 0.000), preoperative sedation and analgesia (OR = 0.159, 95% CI 0.037–0.689, p = 0.014), intraoperative infusion volume ≥ 1,200 mL (OR = 2.530, 95% CI 1.166–5.489, p = 0.019) were identified as independent risk factors for PPI in elderly orthopedic patients. The risk factors in the nomogram included ASA, gender, preoperative pulmonary disease, cognitive impairment, and non-preoperative sedation and analgesia, and intraoperative infusion. Area under the curve (AUC) of the nomogram was 0.834, the slope was 1.000, and the net benefit of the decision curve analysis (DCA) curve was 0.01–0.60.
Conclusion: Researchers have developed and validated a predictive nomogram for PPI in elderly patients undergoing major orthopedic surgery, identifying 6 key variables, which can be used to predict PPI of aged patients undergoing major orthopedic surgery and identify high risk groups.
1 Introduction
Among countries, China has one of the fastest aging populations (1). The risks and challenges of surgical anesthesia for aged patients are substantial, especially for aged patients undergoing major orthopedic surgery. These patients are more susceptible to postoperative pulmonary infections (PPIs) because of preoperative frailty, numerous underlying systemic conditions, and restricted perioperative activity. The incidence of PPI in major orthopedic surgery in the elderly ranges from 3.5% to 14.4% (2, 3). Postoperative pulmonary complications have a significant impact on perioperative morbidity and mortality, prolong postoperative hospital stays, and greatly increase hospital costs (4).
Numerous studies have investigated the high-risk factors for PPI in aged patients, leading to the development of predictive models to identify susceptible individuals early. However, most studies to date have concentrated on hip replacement surgery (5, 6), leaving uncertainly regarding the applicability of these PPI prediction models to major orthopedic surgeries. Preoperative pain, stress and sleep disturbances can increase postoperative complications, which may affect PPI (7). And preoperative pain control can alleviate postoperative pain and improve sleep disorders (8). However, most studies only include patient factors and surgical-related factors, neglecting preoperative pain control and anesthesia-related perioperative factors. Numerous studies have shown that anesthesia and analgesia methods are associated with PPI (9, 10). Therefore, this study retrospectively analyzed the perioperative clinical data, including patient demographics, surgical procedures and anesthesia-related perioperative factors, and specifically preoperative sedative and analgesia practices, among aged patients undergoing major orthopedic surgery in our hospital. The aim was to develop a risk prediction model for PPI in aged patients undergoing major orthopedic surgery, to aid medical staff in early identification of high-risk patients and to offer theoretical support and a simple practical tool.
2 Materials and methods
2.1 Study design
This study was approved by the Institutional Ethics Committee of Shizhu Tujia Autonomous County People’s Hospital, China (Scientific Ethics Review No. 18 in 2022). Due to the retrospective nature of the study and the anonymity of the data, informed consent was not necessary. To ensure anonymity, names and admission and surgical dates were removed during data extraction.
2.2 Patients and sample size
According to Harrell guidelines and reference study from Riley et al. (11), the number of outcome variables should be at least 10–20 times the number of variables. Based on prior studies, approximately 15% of elderly patients undergoing major orthopedic surgery developed PPI. In our study design, we aim to incorporate 12 predictive factors to enhance the accuracy of our prognosis models. To achieve this, we determine that a minimum of 800 patients was necessary to ensure the robustness and statistical significance of our findings. Accounting for a 20% dropout rate, we aimed to enroll at least 960 patients in the study.
Patients who underwent major orthopedic surgery at Shizhu Tujia Autonomous County People’s Hospital spanning from January 2020 to October 2023 were enrolled. The inclusion criteria were as follows: (1) patients who underwent major orthopedic surgery (surgical time >60 min) in our hospital; and (2) age ≥ 65 years old. The exclusion criteria were as follows: (1) patients who underwent orthopedic surgery under local anesthesia; (2) superficial and minimally invasive surgeries such as mass excisions, tendon repairs, and arthroscopies; (3) severe trauma with brain trauma, unconsciousness, pulmonary contusion, hemothorax and pneumothorax, rib fractures, etc.; and (4) incomplete clinical medical records.
2.3 Data collection
In our hospital, the electronic medical record system and surgical anesthesia system were queried using special medical terms such as “fracture” or “arthroplasty” or “replacement” or “vertebra” or “spine” to identified relevant patient records and surgical-anesthesia procedure. Six researchers used a retrospective study method to extract data from patients’ electronic medical records, test records, examination reports, and nursing records. All raw data were collected using self-designed case report form. Two investigators reviewed the data to ensure its accuracy and completeness. Any disagreement was settled by discussion among all researchers.
Observation indicators included (1) patient factors: age, sex, New York Heart Association (NYHA) classification, smoking history, combined pulmonary disease [chronic obstructive pulmonary disease (COPD), asthma, pulmonary infection, tuberculosis], cerebrovascular disease (history of stroke, brain atrophy), cognitive impairment (diagnosed dementia or Montreal Cognitive Assessment Scale < 26 points), diabetes, coronary heart disease, hypertension, preoperative arterial partial pressure of oxygen, pulmonary function, hemoglobin, leukocyte, neutrophil-to-lymphocyte ratio (NLR), albumin, and creatinine; and (2) surgery procedure and anesthesia-related perioperative factors: preoperative sedation and analgesia, anesthesia method, surgical duration, blood loss (ml), fluid volume (ml), blood transfusion, postoperative pain (defined as a VAS score > 3 points within 48 h after operation), postoperative analgesia method, and intensive care unit (ICU) stay.
2.4 Outcomes
The primary outcome was the incidence of pulmonary infection within 7 days of surgery, and the secondary outcomes were length of postoperative hospital stay (LOS) and in-hospital mortality. PPI refers to the diagnosis of postoperative pneumonia defined in the systematic review conducted by Abbott et al. (12): chest X-ray (without underlying cardiopulmonary disease) with at least 1 of the following imaging features: new or progressive persistent pulmonary infiltrates, consolidations, or cavities; at least 1 of the following symptoms: unexplained fever (>38°C), leukocyte (<4 × 109/L) or leukocyte (>12 × 109/L) and, for elderly individuals over 70 years old, disturbance of consciousness with no other explanation; at least 2 reasons: new onset cough, aggravated sputum, change in sputum color, increased respiratory secretions, increased need for sputum suction; new onset or aggravated cough, dyspnoea, shortness of breath; pulmonary rales or bronchial breath sounds; and worsening of gas exchange (hypoxemia, increased oxygen demand, and increased need for mechanical ventilation).
2.5 Missing data
Data cleaning was performed before statistical analyses, and variables with more than 10% missing values were not included. For continuous variable values that were missing (within 10%), the mean or median was used instead according to whether the data follows a normal distribution or not.
2.6 Statistical analysis
Categorical variables are represented by the number of cases, and were analyzed using the chi-square test. Continuous variables are expressed as medians and interquartile ranges (IQRs), and were analyzed using the t test or rank-sum test. Univariate logistic regression analysis was performed to screen the risk factors, and the variables with p < 0.1 were included in the multivariate logistic analysis with forward-backward stepwise method based on the AIC criteria to screen the variables that were ultimately included in the nomogram model. Then, a nomogram was constructed based on the results of the multiple regression.
The area under the curve (AUC) and the calibration curve were used to evaluate the discrimination and accuracy of the nomogram model. Decision curve analysis (DCA) was used to assess the range of clinical validity of the model. All analyses were conducted using Stata 16 (Stata Corp) and R Software 4.1.2 (R Foundation for statistical computing) (Supplementary material 1). For all analyses, p < 0.05 was considered statistically significant.
3 Results
In this study, 10 patients were excluded due to severe trauma, and 4 patients were excluded due to incomplete data. Ultimately, 814 patients were enrolled in the analysis.
3.1 General characteristic of the included patients
The incidence of PPI in this study was 4.7% (38 cases), and 1 patient died due to a PPI. The median LOS in the PPI group was 14 days, and the median LOS in the non-PPI group was 10 days; the difference between the 2 groups was significant (p = 0.003). Preoperative arterial partial pressure of oxygen, pulmonary function and albumin were excluded from the analysis because the missing data exceeded 10%. Between the two groups of patients, there were significant differences in gender, ASA classification, combined pulmonary disease and cognitive impairment, preoperative sedation and analgesia, operation time, blood loss, intraoperative fluid infusion, blood transfusion, and postoperative ICU stay (p < 0.05, Table 1).
3.2 Univariate and multivariate logistic regression results
Univariate analysis showed that ASA classification, gender, combined pulmonary disease and cognitive impairment, preoperative sedation and analgesia, intraoperative fluid infusion, and blood transfusion were associated with PPI (p < 0.05). The multivariate analysis results showed that gender (OR = 2.336, 95% CI 1.135, 4.807, p = 0.021), preoperative pulmonary disease (OR = 6.042, 95% CI 2.849, 12.814, p = 0.000), intraoperative fluid infusion volume ≥1,200 mL (OR = 2.530, 95% CI 1.166, 5.489, p = 0.019) were independent risk factors and preoperative sedation and analgesia (OR = 0.159, and 95% CI 0.037, 0.689, p = 0.014) was independent protective factor for PPI in elderly patients undergoing major orthopedic surgery (Table 2).
3.3 Development of a nomogram for PPI
Eight variables, i.e., ASA, gender, pulmonary disease, cognitive impairment, preoperative sedation, intraoperative fluid infusion, surgical time, and blood transfusion were included in stepwise regression. Finally, a nomogram was constructed incorporating 6 risk factors: ASA, gender, pulmonary disease, cognitive impairment, absence of preoperative sedation and analgesia, and intraoperative fluid infusion (Figure 1). The scores for these indicators in the nomogram were 23.8, 45.5, 98, 56.1, 100, and 52.5, respectively.
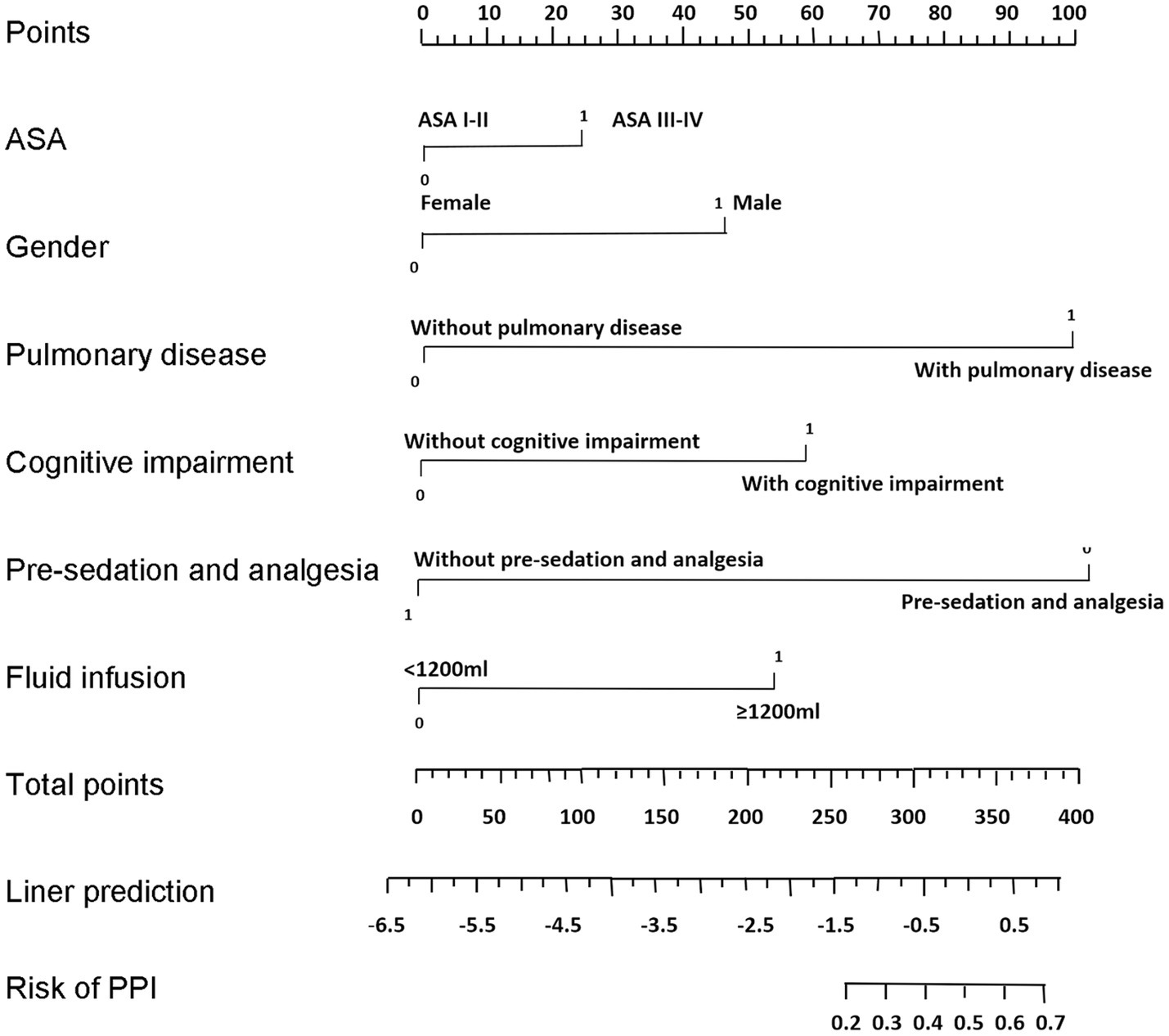
Figure 1. Nomogram for predicting the risk of PPI in elderly patients undergoing major orthopedic surgery. To use the nomogram, a vertical line is drawn up to the top point row to assign points for each variable. Then, the total number of points is calculated, and a vertical line is drawn downward from the total point row to obtain the probability of PPI.
3.4 Validation of the nomogram for PPI
The AUC was 0.834, indicating that the model exhibited strong discriminatory ability (Figure 2). A calibration curve was drawn, and the Hosmer–Lemeshow (HL) goodness-of-fit test was also conducted. The HL test result showed that p = 0.696, and the calibration curve was straight with a slope of 1.0, indicating good consistency between the predicted values and the observed results (Figure 3). The DCA indicated that, when the threshold probability was within a range of 0.01–0.60, the nomogram added more net benefit than the “treat all” or “treat none” strategies (Figure 4).
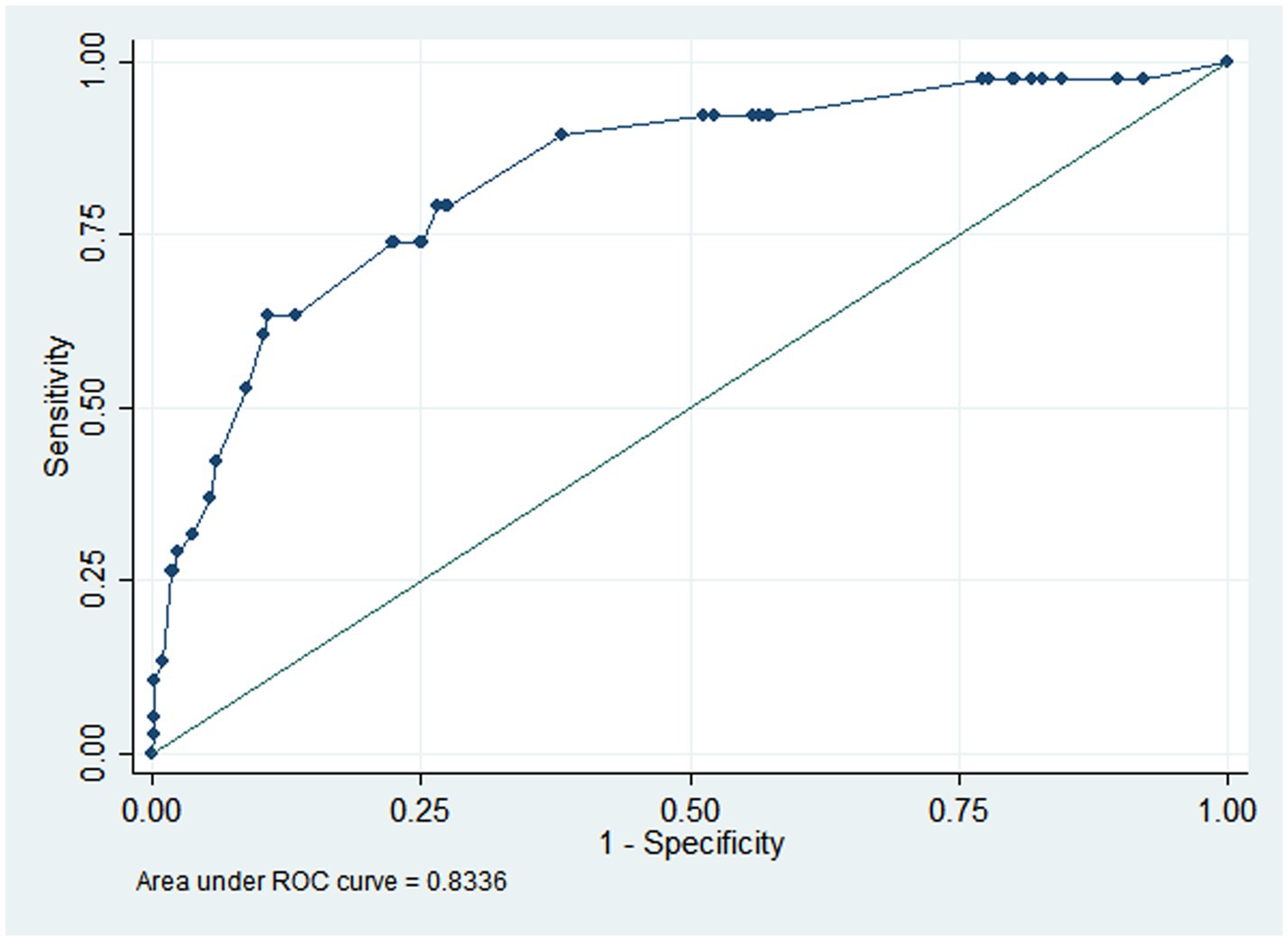
Figure 2. AUC of nomogram for predicting PPI in elderly patients undergoing major orthopedic surgery. The AUC was 0.834, indicating high discrimination. AUC, area under receiver-operating characteristic curve; PPI, postoperative pulmonary infection.
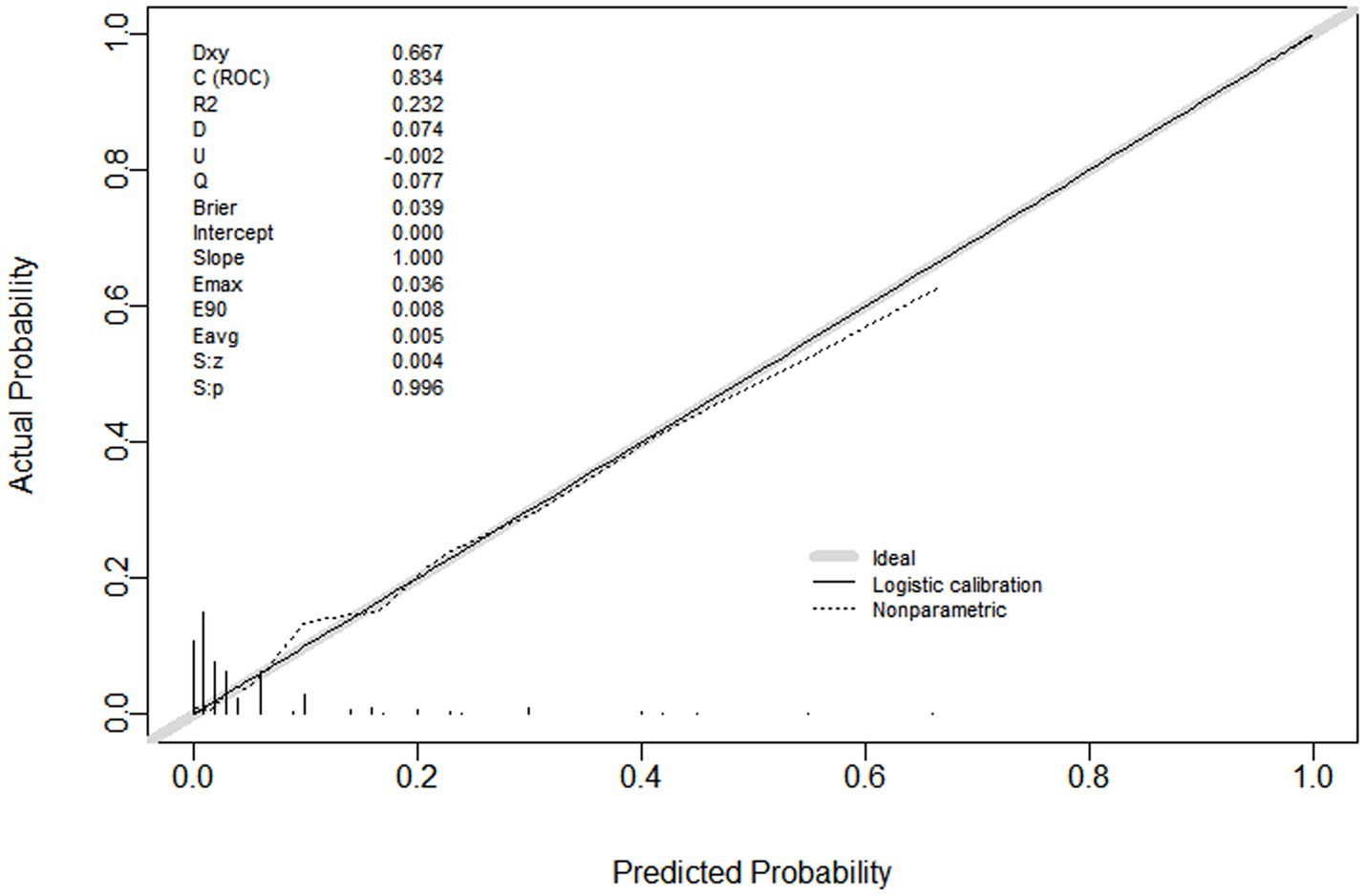
Figure 3. Calibration curve of nomogram for predicting PPI in elderly patients undergoing major orthopedic surgery. The slope was 1.0, the R2 was 0.232. The calibration curve showed good concordance between predicted probability and actual probability. PPI, postoperative pulmonary infection.
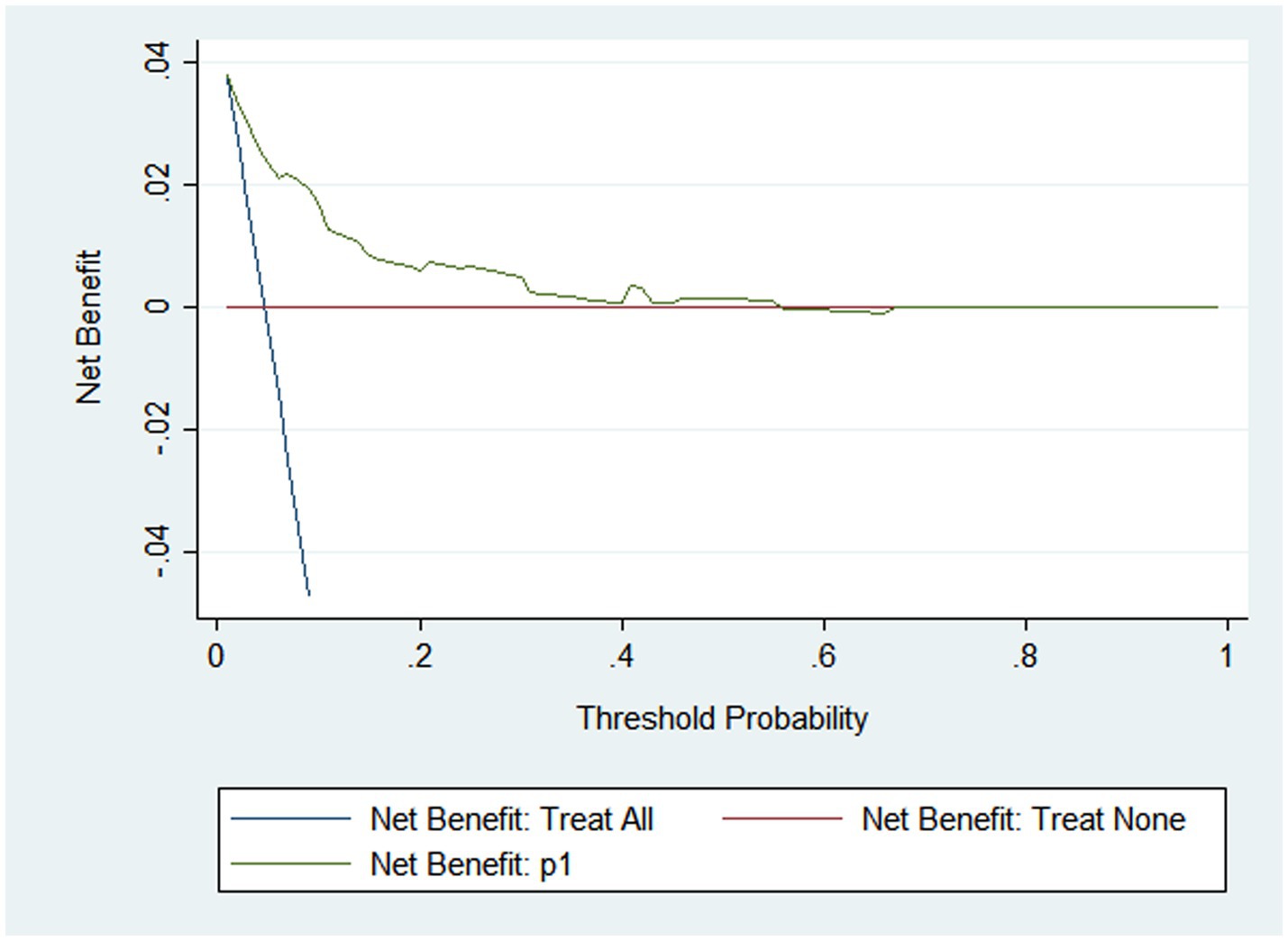
Figure 4. DCA of nomogram for predicting PPI in elderly patients undergoing major orthopedic surgery. DCA, decision curve analysis; PPI, postoperative pulmonary infection.
4 Discussion
Postoperative pulmonary infection (PPI) is a severe complication for elderly patients undergoing major orthopedic surgery, as evidenced by studies indicating that it not only prolongs the postoperative hospital stay but also increases the risk of readmission and death (13). One study revealed that nearly 25% of deaths in the first week after surgery were related to PPIs (14). In this study, the incidence of PPI was 4.7%, and only 1 patient died due to a PPI. Univariate and multivariate logistic regressions were used to analyze the effects of patient and surgical anesthesia factors on PPI in elderly orthopedic major surgery patients, and 6 variables were identified and incorporated into the nomogram for predicting PPI: ASA, gender, combined pulmonary disease, cognitive impairment, preoperative sedation and analgesia, and intraoperative fluid infusion. In addition, the AUC, calibration plot, and DCA showed satisfactory performance for the prediction model.
Many studies have shown that anemia, diabetes, number of comorbidities, ASA ≥ III and some specific laboratory biomarkers and significant clinical interventions are important risk factors for PPI (6, 15, 16). The risk factors incorporated into the prediction model of this study are largely consistent with those identified in prior research (17–19). Our results also found that preoperative sedation and analgesia was a protective factor for elderly orthopedic major surgery patients. Patients with orthopedic surgery often suffer from pain before operation, which affects exercise and sleep quality, and also increases preoperative stress and inflammation, which are closely related to the postoperative pain (7). Surgery also can initiate the pain process, evokes hyperalgesia, releases inflammatory factors, and leads to reduce immunity (20). Several clinical studies have shown that pre-emptive sedation and analgesia can decrease the level of postoperative pain, and allevate postoperative pain (6, 21, 22). The mechanism may involve preoperative pain control, which can help reduce perioperative IL-6 levels and hs-CRP levels, alleviate postoperative pain and improve sleep disorders (8, 21). Therefore, sedatives and analgesics should be used to improve the preoperative state of patients before orthopedic surgery.
ASA classification ≥ III means patients exhibit frailty and many comorbidities, resulting on a lower cardiopulmonary function compared to those classified as ASA I-II (22). Therefore, pulmonary complications are likely to occur after surgery. Male patients are mostly affected by long-term smoking and are often accompanied by COPD, asthma and pneumonia, which affect lung function (23). Anesthesia and surgery can reduce lung volumes, which is the primary physiologic mechanism that contributes to the development of atelectasis and other postoperative pulmonary complications (24). Compared with systemic opioids, epidural local anesthetics increased arterial partial pressure of oxygen and decreased the incidence of PPI and pulmonary complications (4, 7). But the results of this study showed that the mode of anesthesia had no effect on PPI. The reason might be that most high-risk patients underwent epidural anesthesia or nerve block, or other local anesthesia technique. The commonly used sedatives and analgesics in wards in our hospital are mainly dezocine, tramadol, or NSAIDs, which have minimal impact on respiration. Aspiration is a factor that will be strongly linked to PPI. In our study, the incidence of postoperative vomiting was 11.3%, but no aspiration was recorded in any of the groups.
Studies reported that preoperative cognitive impairment associated with morbidity and mortality, including pulmonary complications after surgery (25, 26). The mechanism may involve cognitive impairment due to ineffective respiratory exercise, leading to higher rates of moderate-deep residual sedation during anesthesia recovery. This can result in prolonged postoperative ventilation, an increased incidence of delirium, and subsequently, ineffective coughing and atelectasis (27, 28).
We used data from elderly orthopedic surgery patients at our hospital to validate two other models from studies by Zhang et al. (5) and Tian et al. (29) The results showed that the accuracy of these models was relatively low, with AUC value of 0.674 and 0.758 (Supplementary Figures 1, 2). The HL calibration test results showed p values of 0.010 and 0.065, respectively. Variations in regional practices and the technical abilities of surgeons and anesthesiologists may contribute to differences in model outcomes derived from data collected across various hospitals. Furthermore, discrepancies in regional healthcare standards and hospital levels can lead to variation in the extension and comprehensiveness of patient examinations, which may result in certain indicators no being part of routine examinations, thus making it challenging to obtain relevant data. Studies have indicated that preoperative oxygen partial pressure below 72.5% in elderly patients undergoing hip fracture surgery is associated with PPI (30). Certain studies suggest that pulmonary function, as well as preoperative and early postoperative hypoalbuminemia, are linked to PPI in major orthopedic surgeries (31, 32). Elevated CRP levels can also serve as a predictor for PPI in some research (6). However, since many patients in this study did not routinely receive arterial blood gas analysis, pulmonary function tests, albumin or CRP level measurements prior to surgery, these factors were excluded from the analysis. Nonetheless, their potential impact on PPI cannot be overlooked.
In our study, the nomogram’s Area Under the Curve (AUC) was 0.834, and the calibration curve’s slope was 1, p value was 0.696 from HL test, suggesting good consistency and calibration. The Decision Curve Analysis (DCA) demonstrated the clinical practicability of the prediction model, indicating that its discrimination ability for individual probabilities was satisfactory. Therefore, the prediction model with 6 simple clinical factors can assist clinicians in identifying high-risk patients with PPI before surgery.
However, this study has certain limitations. Firstly, the data were collected retrospectively, which may compromise the reliability of all information, potentially introducing bias into the results and increasing the risk of misdiagnosis and missed diagnosis. Some cases of postoperative atelectasis may also fulfill the diagnostic criteria of PPI and thus confound the results. Secondly, being a single-center study, the sample size was inadequate, and some risk factors were not included due to incomplete data, potentially undermining the robustness of the results. Thirdly, prospective external verification was not performed, and the application value of the model needs to be confirmed by further research.
In conclusion, this study developed a 6-factor nomogram prediction model for predicting PPI in elderly patients undergoing major orthopedic surgery, considering patients, surgical and anesthesia related factors. The model can help in the identification of high-risk individuals early and in the formulation of optimal anesthesia and perioperative management strategies to reduce the occurrence of PPIs. However, a larger sample size and a multicenter study are needed to confirm these conclusions.
Data availability statement
The original contributions presented in the study are included in the article/Supplementary material, further inquiries can be directed to the corresponding authors.
Ethics statement
The studies involving humans were approved by Ethical Committee of Clinical Research of the Shizhu Tujia Autonomous County People’s Hospital. The studies were conducted in accordance with the local legislation and institutional requirements. Written informed consent for participation was not required from the participants or the participants’ legal guardians/next of kin because this study utilizes medical records/biological samples obtained from previous clinical treatments. Written informed consent was not obtained from the individual (s) for the publication of any potentially identifiable images or data included in this article because this study utilizes medical records/biological samples obtained from previous clinical treatments.
Author contributions
YLi: Conceptualization, Formal analysis, Writing – original draft. YF: Investigation, Methodology, Writing – original draft. XY: Investigation, Writing – original draft. HG: Investigation, Writing – original draft. XL: Investigation, Writing – original draft. YLu: Investigation, Writing – original draft. QP: Formal analysis, Software, Writing – review & editing. TY: Project administration, Supervision, Writing – review & editing.
Funding
The author(s) declare that no financial support was received for the research and/or publication of this article.
Conflict of interest
The authors declare that the research was conducted in the absence of any commercial or financial relationships that could be construed as a potential conflict of interest.
Generative AI statement
The authors declare that no Gen AI was used in the creation of this manuscript.
Publisher’s note
All claims expressed in this article are solely those of the authors and do not necessarily represent those of their affiliated organizations, or those of the publisher, the editors and the reviewers. Any product that may be evaluated in this article, or claim that may be made by its manufacturer, is not guaranteed or endorsed by the publisher.
Supplementary material
The Supplementary material for this article can be found online at: https://www.frontiersin.org/articles/10.3389/fmed.2025.1537697/full#supplementary-material
SUPPLEMENTARY MATERIAL 1 | Codes used in Stata and R software.
SUPPLEMENTARY FIGURE 1 | AUC of Zhang's research model for external validation with data from our hospital. The AUC was 0.674, indicating low accuracy.
SUPPLEMENTARY FIGURE 2 | AUC of Tian's research model for external validation with data from our hospital. The AUC was 0.758, indicating medium accuracy.
References
1. Man, W, Wang, S, and Yang, H. Exploring the spatial-temporal distribution and evolution of population aging and social-economic indicators in China. BMC Public Health. (2021) 21:966. doi: 10.1186/s12889-021-11032-z
2. Zhao, K, Zhang, J, Li, J, Guo, J, Meng, H, Zhu, Y, et al. In-hospital postoperative pneumonia following geriatric intertrochanteric fracture surgery: incidence and risk factors. Clin Interv Aging. (2020) 15:1599–609. doi: 10.2147/CIA.S268118
3. Song, K, Rong, Z, Yang, X, Yao, Y, Shen, Y, Shi, D, et al. Early pulmonary complications following Total knee arthroplasty under general anesthesia: a prospective cohort study using CTScan. Biomed Res Int. (2016) 2016:1–5. doi: 10.1155/2016/4062043
4. Sameed, M, Choi, H, Auron, M, and Mireles-Cabodevila, E. Preoperative pulmonary risk assessment. Respir Care. (2021) 66:1150–66. doi: 10.4187/respcare.09154
5. Zhang, X, Shen, ZL, Duan, XZ, Zhou, QR, Fan, JF, Shen, J, et al. Postoperative pneumonia in geriatric patients with a hip fracture: incidence, risk factors and a predictive nomogram. Geriatr Orthop Surg Rehabil. (2022) 13:21514593221083824. doi: 10.1177/21514593221083824
6. Xiang, G, Dong, X, Xu, T, Feng, Y, He, Z, Ke, C, et al. A nomogram for prediction of postoperative pneumonia risk in elderly hip fracture patients. Risk Manag Healthc Policy. (2020) 13:1603–11. doi: 10.2147/RMHP.S270326
7. Wluka, AE, Yan, MK, Lim, KY, Hussain, SM, and Cicuttini, FM. Does preoperative neuropathic-like pain and central sensitisation affect the post-operative outcome of knee joint replacement for osteoarthritis? A systematic review and meta analysis. Osteoarthr Cartil. (2020) 28:1403–11. doi: 10.1016/j.joca.2020.07.010
8. Wilson, JM, Yoon, J, Mun, CJ, Meints, SM, Campbell, CM, Haythornthwaite, JA, et al. The association between changes in clinical pain severity and IL-6 reactivity among patients undergoing total knee arthroplasty: the moderating role of change in insomnia. Brain Behav Immun. (2024) 120:199–207. doi: 10.1016/j.bbi.2024.06.001
9. Ballantyne, JC, Carr, DB, de Ferranti, S, Suarez, T, Lau, J, Chalmers, TC, et al. The comparative effects of postoperative analgesic therapies on pulmonary outcome: cumulative meta-analyses of randomized, controlled trials. Anesth Analg. (1998) 86:598–612.
10. Menendez, ME, Ring, D, and Bateman, BT. Preoperative opioid misuse is associated with increased morbidity and mortality after elective Orthopaedic surgery. Clin Orthop Relat Res. (2015) 473:2402–12. doi: 10.1007/s11999-015-4173-5
11. Riley, RD, Ensor, J, Snell, KIE, Harrell, FE Jr, Martin, GP, Reitsma, JB, et al. Calculating the sample size required for developing a clinical prediction model. BMJ. (2020) 368:m441. doi: 10.1136/bmj.m441
12. Abbott, TEF, Fowler, AJ, Pelosi, P, Gama de Abreu, M, Møller, AM, Canet, J, et al. A systematic review and consensus definitions for standardised end-points in perioperative medicine: pulmonary complications. Br J Anaesth. (2018) 120:1066–79. doi: 10.1016/j.bja.2018.02.007
13. Bohl, DD, Sershon, RA, Saltzman, BM, Darrith, B, and Della Valle, CJ. Incidence, risk factors, and clinical implications of pneumonia after surgery for geriatric hip fracture. J Arthroplast. (2018) 33:1552–1556.e1. doi: 10.1016/j.arth.2017.11.068
14. Chandler, D, Mosieri, C, Kallurkar, A, Pham, AD, Okada, LK, Kaye, RJ, et al. Perioperative strategies for the reduction of postoperative pulmonary complications. Best Pract Res Clin Anaesthesiol. (2020) 34:153–66. doi: 10.1016/j.bpa.2020.04.011
15. Chang, SC, Lai, JI, Lu, MC, Lin, KH, Wang, WS, Lo, SS, et al. Reduction in the incidence of pneumonia in elderly patients after hip fracture surgery: An inpatient pulmonary rehabilitation program. Medicine. (2018) 97:e11845. doi: 10.1097/MD.0000000000011845
16. Lv, H, Yin, P, Long, A, Gao, Y, Zhao, Z, Li, J, et al. Clinical characteristics and risk factors of postoperative pneumonia after hip fracture surgery: a prospective cohort study. Osteoporos Int. (2016) 27:3001–9. doi: 10.1007/s00198-016-3624-5
17. Gu, A, Wei, C, Chen, AZ, Malahias, MA, Fassihi, SC, Ast, MP, et al. Operative time greater than 120 minutes is associated with increased pulmonary and thromboembolic complications following revision total hip arthroplasty. Eur J Orthop Surg Traumatol. (2020) 30:1393–400. doi: 10.1007/s00590-020-02712-4
18. Huang, Q, Weng, D, Yuan, J, Liang, Y, Cai, S, and Jiang, R. Clinical analysis of different anesthesia methods for pulmonary infection after perioperative operation. J Infect Public Health. (2021) 14:71–6. doi: 10.1016/j.jiph.2019.09.011
19. Shen, JW, Zhang, PX, An, YZ, and Jiang, BG. Prognostic implications of preoperative pneumonia for geriatric patients undergoing hip fracture surgery or arthroplasty. Orthop Surg. (2020) 12:1890–9. doi: 10.1111/os.12830
20. Yin, S, Tao, H, Du, H, Feng, C, Yang, Y, Yang, W, et al. Postoperative pulmonary complications following posterior spinal instrumentation and fusion for congenital scoliosis. PLoS One. (2018) 13:e0207657. doi: 10.1371/journal.pone.0207657
21. Liszka, H, Zając, M, and Gądek, A. Pre-emptive analgesia with methylprednisolone and gabapentin in total knee arthroplasty in the elderly. Sci Rep. (2022) 12:2320. doi: 10.1038/s41598-022-05423-4
22. Hamal, PK, Shrestha, AB, and Shrestha, RR. Efficacy of preemptive gabapentin for lower extremity orthopedic surgery under subarachnoid block. JNMA J Nepal Med Assoc. (2015) 53:210–3. doi: 10.31729/jnma.2732
23. Xu, BY, Yan, S, Low, LL, Vasanwala, FF, and Low, SG. Predictors of poor functional outcomes and mortality in patients with hip fracture: a systematic review. BMC Musculoskelet Disord. (2019) 20:568. doi: 10.1186/s12891-019-2950-0
24. Numata, T, Nakayama, K, Fujii, S, Yumino, Y, Saito, N, Yoshida, M, et al. Risk factors of postoperative pulmonary complications in patients with asthma and COPD. BMC Pulm Med. (2018) 18:4. doi: 10.1186/s12890-017-0570-8
25. Odor, PM, Bampoe, S, Gilhooly, D, Creagh-Brown, B, and Moonesinghe, SR. Perioperative interventions for prevention of postoperative pulmonary complications: systematic review and meta-analysis. BMJ. (2020) 368:m540. doi: 10.1136/bmj.m540
26. Aykut, K, Albayrak, G, Guzeloglu, M, Baysak, A, and Hazan, E. Preoperative mild cognitive dysfunction predicts pulmonary complications after coronary artery bypass graft surgery. J Cardiothorac Vasc Anesth. (2013) 27:1267–70. doi: 10.1053/j.jvca.2013.01.028
27. McHugh, MA, Wilson, JL, Schaffer, NE, Olsen, EC, Perdue, A, Ahn, J, et al. Preoperative comorbidities associated with early mortality in hip fracture patients: a multicenter study. J Am Acad Orthop Surg. (2023) 31:81–6. doi: 10.5435/JAAOS-D-21-01055
28. Kruthiventi, SC, Laporta, ML, Deljou, A, Knopman, DS, Petersen, RC, Schroeder, DR, et al. Preoperative cognitive impairment associated with oversedation during recovery from anesthesia. J Anesth. (2020) 34:390–6. doi: 10.1007/s00540-020-02764-0
29. Tian, Y, Zhu, Y, Zhang, K, Tian, M, Qin, S, Li, X, et al. Incidence and risk factors for postoperative pneumonia following surgically treated hip fracture in geriatric patients: a retrospective cohort study. J Orthop Surg Res. (2022) 17:179. doi: 10.1186/s13018-022-03071-y
30. Ji, Y, Li, X, Wang, Y, Cheng, L, Tian, H, Li, N, et al. Partial pressure of oxygen level at admission as a predictor of postoperative pneumonia after hip fracture surgery in a geriatric population: a retrospective cohort study. BMJ Open. (2021) 11:e048272. doi: 10.1136/bmjopen-2020-048272
31. Shin, KH, Kim, JJ, Son, SW, Hwang, KS, and Han, SB. Early postoperative Hypoalbuminaemia as a risk factor for postoperative pneumonia following hip fracture surgery. Clin Interv Aging. (2020) 15:1907–15. doi: 10.2147/CIA.S272610
Keywords: orthopedic surgery, postoperative infection, pulmonary, aged, nomogram
Citation: Liu Y, Fan Y, Yang X, Gan H, Li X, Luo Y, Pang Q and Yang T (2025) Nomogram for predicting postoperative pulmonary infection in elderly patients undergoing major orthopedic surgery. Front. Med. 12:1537697. doi: 10.3389/fmed.2025.1537697
Edited by:
Zhaolan Hu, Central South University, ChinaReviewed by:
Christian Bohringer, UC Davis Medical Center, United StatesNing Na, Third Affiliated Hospital of Sun Yat-sen University, China
Zhiming Zhang, The First People’s Hospital of Chenzhou, China
Copyright © 2025 Liu, Fan, Yang, Gan, Li, Luo, Pang and Yang. This is an open-access article distributed under the terms of the Creative Commons Attribution License (CC BY). The use, distribution or reproduction in other forums is permitted, provided the original author(s) and the copyright owner(s) are credited and that the original publication in this journal is cited, in accordance with accepted academic practice. No use, distribution or reproduction is permitted which does not comply with these terms.
*Correspondence: Qianyun Pang, cHF5MDQ3NDE3QDE2My5jb20=; Tingjun Yang, NjU3NTU2MDRAcXEuY29t
†These authors have contributed equally to this work