- 1Programa de Inmunología Molecular Microbiana, Departamento de Microbiología y Parasitología, Facultad de Medicina, Universidad Nacional Autónoma de México, Mexico City, Mexico
- 2Programa de Genómica Evolutiva, Centro de Ciencias Génomicas, Universidad Nacional Autónoma de México, Cuernavaca, Mexico
Sigma factors are an essential part of bacterial gene regulation and have been extensively studied as far as their molecular mechanisms and protein structure are concerned. However, their molecular evolution, especially for the alternative sigma factors, is poorly understood. Here, we analyze the evolutionary forces that have shaped the rpoH sigma factors within the alphaproteobacteria. We found that an ancient duplication gave rise to two major groups of rpoH sigma factors and that after this event horizontal gene transfer (HGT) occurred in rpoH1 group. We also noted that purifying selection has differentially affected distinct parts of the gene; singularly, the gene segment that encodes the region 4.2, which interacts with the −35 motif of the RpoH-dependent genes, has been under relaxed purifying selection. Furthermore, these two major groups are clearly differentiated from one another regarding their promoter selectivity, as rpoH1 is under the transcriptional control of σ70 and σ32, whereas rpoH2 is under the transcriptional control of σ24. Our results suggest a scenario in which HGT, gene loss, variable purifying selection and clear promoter specialization occurred after the ancestral duplication event. More generally, our study offers insights into the molecular evolution of alternative sigma factors and highlights the importance of analyzing not only the coding regions but also the promoter regions.
Introduction
Bacteria face many different environmental challenges and a key element to cope with this is the capacity to modulate gene expression. There are several ways in which a bacterium can regulate gene expression, one of them being the use of different sigma factors to rapidly mount a response to environmental changes. Bacteria have one essential housekeeping sigma factor, which controls the transcription of many genes, and some species additionally might have one or more alternative sigma factors that promote very specific sets of genes required for particular stress conditions (Helmann, 2002; Gruber and Gross, 2003; Osterberg et al., 2011; Feklistov et al., 2014). One of these alternative sigma factors is σ32, which is encoded by the rpoH gene; this sigma factor plays a key role in the heat shock stress response in many bacteria (Gruber and Gross, 2003; Osterberg et al., 2011; Feklistov et al., 2014). Many of the heat-shock proteins that define the heat-shock stimulon are regulated by σ32. This sigma factor is found as a single copy gene in Escherichia coli, as well as in other gammaproteobacteria (Bukau, 1993); nonetheless, it is has been found as a multiple copy gene in many alphaproteobacteria genomes (Narberhaus et al., 1997; Galibert et al., 2001; Kaneko et al., 2002; Gonzalez et al., 2006; Green and Donohue, 2006; Martinez-Salazar et al., 2009a). For instance, two rpoH-like genes have been found in Rhodobacter sphaeroides (Green and Donohue, 2006), whereas Bradyrhizobium japonicum has three copies (Narberhaus et al., 1997). In the case of Rhizobium etli, which has two copies, one of them, namely rpoH1, deals with the heat-shock response, whereas the second copy (rpoH2) is needed for the osmotic-shock response (Martinez-Salazar et al., 2009a). This trend seems to apply to other alphaproteobacteria, where one of the rpoH genes (generally named rpoH1) is involved in the heat-shock response, whereas the other (s) copies are involved in other stress conditions (Narberhaus et al., 1997; Green and Donohue, 2006). Although in alphaproteobacteria the different rpoH genes are differentially regulated, it has also been found that they can partially or completely complement the temperature-sensitive phenotype of an E. coli rpoH mutant (Narberhaus et al., 1997; Green and Donohue, 2006; Martinez-Salazar et al., 2009a). This might suggest that the functional properties of the different RpoH proteins coded in these genomes are not that different after all. Despite this, it has also been shown that different RpoH copies target a common shared set of genes within a genome but also each copy has a set of exclusive targets (Dufour et al., 2012). This implies that although the functions of the RpoH copies may be similar, they are not exactly the same. It is possible that, to some extent, the differentiation between these copies could lay within the parts of the protein that recognize the upstream regions of the genes regulated by these RpoH sigma factors.
Sequence analyses have shown that RpoH sigma factors form a monophyletic group and belong to one of the main four groups in which the σ70 family is divided into (Helmann, 2002; Paget and Helmann, 2003). The RpoH sigma factors are within the 3rd group of this family and are distantly related to the first group, which is composed of essential housekeeping factors σ70. This third group has been implicated in stress responses and developmental programs such as flagella biosynthesis or sporulation (Helmann, 2002; Paget and Helmann, 2003). The RpoH sigma factors have two conserved amino acid regions, namely 2.4 and 4.2, that contact the promoter sequences (2.4 contacts the −10 motif, whereas 4.2 interacts with the −35 motif) of the genes controlled by these sigma factors; the 4.2 resides in the carboxy-terminal section of the protein, whereas region 2.4 lies within the amino-terminal part of the protein (Feklistov et al., 2014). Compared to other members of the σ70 family, the RpoH sigma factors are characterized by a region of highly conserved amino acids—involved in RNA polymerase interactions—that is known as the “RpoH box” (Nakahigashi et al., 1995). Previous sequence analyses have focused on the whole σ70 family mainly at the level of protein sequences. To date, no single study has focused on the homologous genes from the rpoH sigma factor subfamily. Most studies instead have either grouped the sigma factors into different subgroups and/or determined which parts of the protein sequences were more conserved. Notably, much less is known about the evolutionary forces that have shaped the genetic variation of the genes that code for these proteins. For instance, there has been little attention paid to the role of natural selection (either purifying or diversifying) as a potential major evolutionary driver for the sigma factors or the impact of horizontal gene transfer (HGT) on these genes.
Nowadays, with the availability of so many bacterial genomes, evolutionary studies can be carried out much more easily than a couple of decades ago, when the shortage of sequences was one of the major shortcomings. Therefore, given the scarcity of evolutionary studies concerning sigma factors, the aim of our work was to study the molecular evolution of the rpoH sigma factors. Our results show that this family has a very particular evolutionary history, where after an ancient gene duplication event—with subsequent gene loss and HGT events—variable purifying selection across the gene and functional differentiation of the promoter regions have occurred.
Materials and Methods
Genomes Used and RpoH Homologous Genes
To carry out our study 53 bacterial genomes were considered (Additional Material 1), 46 of them belong to the alphaproteobacteria; however, we also included 7 gammaproteobacteria genomes. We chose these 46 alphaproteobacteria genomes as previous phylogenomic analyses have established the species tree for them (Gupta and Mok, 2007; Castillo-Ramirez and Gonzalez, 2008) and this was relevant for some of the phylogenetic analyses that we conducted. Furthermore, these genomes cover the 7 main orders, and many families, of the alphaproteobacteria. These genomes were downloaded between November and December 2015 from the NCBI and are listed in Additional Material 1. We used the RpoH protein from E. coli K12 as a seed in the blast searches (Altschul et al., 1990), setting an E-value cutoff of 1.0e-15, against the proteomes encoded by the other genomes. All the proteins that showed an E-value lower than the cutoff and that aligned at least 70% of their length were kept for further analysis. Then, a protein Multiple Sequence Alignment (MSA) was created through MUSCLE (Edgar, 2004), specifying 20 iterations. To create a DNA alignment in frame, we used the program TRANALING, which is part of the EMBOSS suite (Rice et al., 2000), that aligns coding regions based on the based on the corresponding translations of the genes considered.
Phylogenetic Inferences and among-rpoH Divergence
We conducted a Maximum Likelihood (ML) phylogeny on the protein MSA and chose the most adequate model using ProtTest (Abascal et al., 2005) that in this case was: LG (as the model of amino acid substitution), a correction for among site variation (G) and allowed a proportion of invariable sites (I). We set a non-parametric bootstrap analysis (100 replicates) to establish the support for the clades. In order to have a proxy for the species tree of the species of the genomes here analyzed, we considered the set of 31 orthologs used by Ciccarelli et al. (2006) to reconstruct a tree of life. Of the 31 orthologs we only kept those cases that are single gene families (only one gene per genome) and that are also present in each of the genomes employed in this study; this reduced the number of cases to 8 orthologs (see Additional Material 2). None of these genes had signals of recombination. The protein alignments of these genes were concatenated and, on this concatenate alignment, a Maximum Likelihood phylogeny was constructed. We ran the ML phylogeny with the model LG+G+I, as determined by ProtTest (Abascal et al., 2005), this phylogeny is provided in Additional Material 3.
We ran several topology tests to establish whether or not the topology obtained for the proxy for the species tree provides an equivalent explanation for the two main groups identified in the RpoH family. For this analysis, we considered the RpoH sequences from 15 genomes (Additional Material 4) that have one copy in each one of both RpoH groups. The protein phylogenies for these two groups were conducted via PhyML (Guindon et al., 2010), we carried out statistical model selection as before, via ProtTest (Abascal et al., 2005). The best models in these cases were as follows: JTT+G+I+F for RpoH1 and LG+G+I+F for RpoH2. We conducted two topology tests, the Kishino-Hasegawa (KH) and the Shimodaira-Hasewaga (SH), by means of the program codeml (seqtype = 2), from PAML (Yang, 2007). We ran codeml considering the appropriate substitution rate matrix and among site rate variation estimating the alpha parameter for the gamma distribution. Two topologies were tested in each case, one was the ML topology obtained for the proxy for the species tree and the other was the ML phylogeny of either RpoH1 or RpoH2. The trees used for this analysis are shown in Additional Material 9.
We also ran a molecular dating analysis on the RpoH protein MSA, using the Bayesian Evolutionary Analysis by Sampling Trees (BEAST) program (Drummond and Rambaut, 2007). We employed an uncorrelated log-normal relaxed clock with site model specifications equal to those of the RpoH ML phylogeny. We calibrated the clock using information from the TimeTree database (Hedges et al., 2006, 2015); we used the dates of the most recent common ancestor of (1) the Enterobacteriales (425 million years ago [mya]), (2) the gammaproteobacteria (1787.2 mya), and (3) the gamma and alphaproteobacteria (2472.1 mya). We specified normal prior distributions on the calibrated nodes centered at the values mention above and with 20 standard deviations. The analysis was run twice for 50,000,000 generations, sampling every 5000 generations and discarding the first 12,500,00 generations as burn-in. We are confident about our results as the Effective Sample Size for all the parameters—except for the calibrated Yule model that was 195.176—were >200 and because the 2 BEAST runs converged on similar posterior distributions.
Selection Analysis and Tajima'S Relative Test
All the selection analyses were run using codeml from PAML (Yang, 2007). We tested for variability of selection (type and magnitude) across the codons of the gen using some site models; three pairs of models were employed. The first pair considers M0 (just one dN/dS ratio) and M3 (“n” discrete categories of dN/dS) and has 4 degrees of freedom (df); the second pair includes M1a (two classes of codons, conserved [dN/dS < 1] and neutral [dN/dS = 1]) and M2a (the same as M1a plus another category that allows for dN/dS > 1), this has 2 df; and the third pair encompasses M7 (a beta distribution that allows dN/dS to vary in the interval [0, 1]) and M8 (the same beta distribution as in M7 but adding an extra class for codons with dN/dS >1), with 2 df. We used MEGA6 (Tamura et al., 2013) to conduct the Tajima's relative test (Tajima, 1993) to evaluate the hypothesis of equal rates between two rpoH groups. The analysis involved the amino acid sequences of the two copies of R. etli CFN42 and E. coli K-12 as an out group. Similar analyses were carried out with the two RpoH copies of B. abortus 9-941, Jannaschia sp. CCS1, and R. sphaeroides 2.4.1 in all these cases also using E. coli K-12 as an out group.
Promoter Analysis
To carry out promoter identification, we used the upstream regions of rpoH1 and rpoH2 from a set of Rhizobiales genomes (Additional Material 6). For both rpoH1 and rpoH2, two data sets were created collecting the upstream non-coding regions using a custom PERL script. One set contained the first upstream 150 nucleotides from the ATG that defines the translation start site, whereas the other contained the whole upstream region until the next gene. We used RSAT, Regulatory Sequence Analysis Tools (Medina-Rivera et al., 2015), to conduct promoter identification on these data sets. First, by means of spaced dyads analysis de novo promoter identification was conducted. Different motifs were created with a dyad spacing parameter of 13–22, other than this default parameters were used (Additional Material 7). However, in the case of the rpoH2 group we used a minimum weight of 6 for the assembled motifs. Alternatively, we also used matrices for promoter identification. We created matrices employing the program info-gibbs also from RSAT and using the information of previously reported promoters for alphaproteobacteria (Martinez-Salazar et al., 2009a,b; Barnett et al., 2012; Jans et al., 2013; Schluter et al., 2013). The matrices were constructed independently for the −35 and −10 promoter motifs—when considering the σ70 family promoters class (SigA, RpoH1, RpoH2, RpoE2, RpoE4, and RpoE1)—and −24 and −12 motifs for the σ54 family promoters class. We used the matrices to locate potential promoter motifs using matrix-scan (also from RSAT) with a p-value cutoff of 0.001 for the −35 motifs and in some cases a p-value of 0.0025 for the −10 motifs, we used different p-values as it has been shown that the −10 motif tends to be less conserved (Ramirez-Romero et al., 2006). In the case of the potential promoter that belonged to σ70 family, we retained all the cases that had a spacer of 13–23 nt between −35 and −10 motifs (Additional Material 8).
Results
A Mixture of Gene Duplication, HGT, and Gene Loss Events
In order to understand the molecular evolution of the rpoH genes we used more than 50 genomes (see Additional Material 1), most of which are classified as alphaproteobacteria, although we also considered few gammaproteobacteria genomes as outgroup. Notably these genomes represent the main orders of the alphaproteobacteria (videlicet Rhizobiales, Rhodobacterales, Sphingomonadales, Rhodospiralelles, Rickettsiales, Parvularculales, Caulobacterales). We first wanted to quantify the number of RpoH homologs per genome and, therefore, we conducted BLAST searches using the RpoH sequence from E. coli K12 as seed to identify the RpoH protein sequences in the rest of the genomes (see Section Materials and Methods). A total of 76 RpoH protein sequences were found and to visualize their phylogenetic relationships we constructed a Maximum Likelihood (ML) phylogeny (Figure 1). We first note that, whereas there is only one copy of RpoH in the gammaproteobacteria genomes (pink labels, Figure 1), there are many alphaproteobateria that have more than one copy per genome (light blue, blue, gray, and red labels, Figure 1). For example, R. etli CFN42 has two copies and the same applies for other Rhizobia such as S. meliloti or M. loti but also for species from the genera Bartonella and Rhodobacter. The most extreme case is B. japonicum USDA, which shows three copies; at the other extreme—with just one copy—there are some strains (CGA009, BisA53, BisB5, and BiisB18) from the species Rhodopseudomonas palustris but also S. alaskensis RB2256, Z. mobilis ZM4, E. litoralis HTCC2594, and Sphingomonas_sp. MM-1 (green labels). Notably, all the alphaprotebacteria RpoH sequences form a monophyletic group, which is very well supported (i.e., a bootstrap value of 100, see Figure 1) and well differentiated from the gammaproteobacteria RpoH sequences. Furthermore, the RpoH sequences from the alphaproteobacteria and gammaproteobacteria do not mix together whatsoever. This implies that there has been no HGT between these two classes (alphaproteobacteria and gammaproteobacteria). Together these data suggest that the presence of more than one rpoH gene per genome is a common occurrence in the alphaproteobacteria and that HGT involving these genes has no occurred between the alphaproteobacteria and gammaproteobacteria.
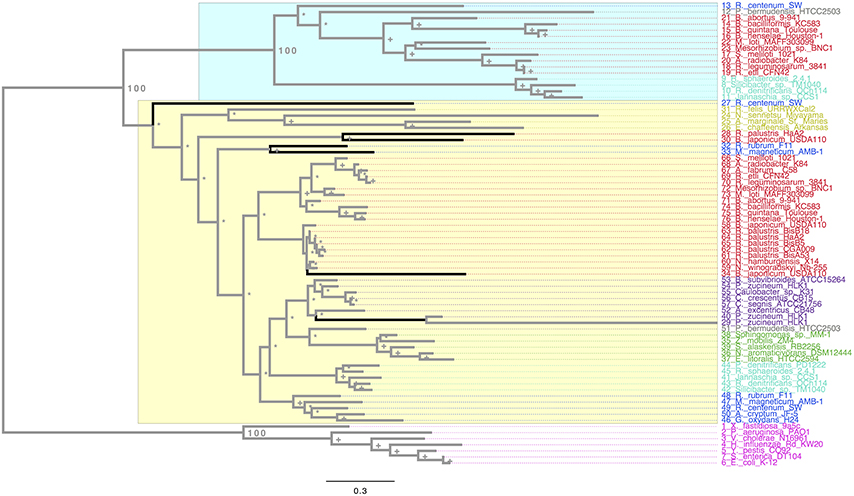
Figure 1. Maximum Likelihood phylogeny of the RpoH. The ML phylogeny is based on the protein alignment. We colored the RpoH sequences to denote the different orders of the alphaproteobacteria: red, Rhizobiales; light blue (cyan), Rhodobacterales; green, Sphingomonadales; blue, Rhodospirillales; yellow, Rickettsiales, gray, Parvularculales; violet, Caulobacterales, and magenta for the gammaproteobacteria. The numbers next to some nodes are the bootstrap values for some of the main groups, plus signs show nodes with 70 or higher bootstrap support, whereas asterisks denote nodes with less than 70 bootstrap support. The black branches denote HGT events. The yellow rectangle shows all the sequences within the rpoH1 group, whereas the blue one covers the sequences composing the rpoH2 group. The scale bar describes the number of substitution per site.
In order to a have a reference to identify HGT and duplication events, we constructed a proxy for the species tree of the genomes considered here using a previous set of orthologous genes (see Section Materials and Methods and Additional Material 2). Given this proxy for the species tree (see Supplementary Material 3), the positions of some sequences on the RpoH tree are better explained as cases of HGT; these are marked on the tree (see black branches). Such is the case of one of the RpoH copies of Rhodospirillum centenum SW, which clusters basally to the Rickettsiales. Another two cases are two of the copies of B. japonicum USDA and one of the copies of Rhodopseudomonas palustris HaA2. However all these cases of HGT seem to have occurred within the alphaproteobacteria. On the other hand, Figure 1 clearly shows that there are two main groups (rpoH1, yellow rectangle, and rpoH2,blue rectangle) in the alphaproteobacteria group; notably, the phylogenetic relationships among the sequences within the two groups—if one does not take into account the HGT events previously mentioned—are similar to those found in the species trees of the Rhizobiales order (Castillo-Ramirez and Gonzalez, 2008) and to our proxy for the species tree. This might suggest that a duplication of the rpoH gene might have occurred some time ago and then each copy has accumulated changes that reflect a history of the species. To gain further insight into this, we tested whether the topologies for a common set of taxa (see Additional Material 4) within the two groups where similar to that of the proxy for the species tree (see Section Materials and Methods). We did not find significant differences (at an alpha of 0.01) between the topology of either group and that of the proxy for the species tree (see Table 1); this was true irrespective of the test used—we employed the Kishino–Hasegawa test but also the Shimodaira–Hasewaga test. From these results we conclude that the phylogenetic relationships within each group (not taking into account the HGT events in rpoH1 group) are pretty similar to those of the species tree, which further corroborates the view that a duplication event gave rise to the two groups. Of note the HGT events seemed to be found exclusively in the rpoH1 group. Although, a duplication event has generated two rpoH groups, the genes belonging to each one of them have no been equally conserved; clearly, the rpoH1 group had more taxa than the rpoH2 group (see Figure 1). Actually, whereas all the alphaproteobacteria had one member of the rpoH1 group, not all the alphaproteobacteria had a member of the rpoH2 group. For instance, the two species from the genus Nitrobacter, many R. palustris strains, A. tumefaciens C58, S. alaskensis RB2256, Z. mobilis ZM4, E. litoralis HTCC2594, and Sphingomonas_sp. MM-1 just had one rpoH homolog and in all the cases it belongs to the rpoH1 group. This implies that several gene loss events have occurred over time but these have only affected the members of the rpoH2 group.
Our ML phylogeny suggests that the duplication event that gave origin to the rpoH2 group is rather ancient; therefore, in order to further explore this, we constructed a calibrated phylogeny using BEAST (Drummond and Rambaut, 2007; see Section Materials and Methods; Additional Material 5). Our calibrated phylogeny suggests that the duplication occurred some 2214 million years ago (mya) (Figure 2), 95% highest posterior density interval (HPD) 1812–2491 mya—the whole calibrated phylogeny is provided in Additional Material 5. Therefore, our dating analysis tells us that this duplication event is ancient; more than 1800 mya even if one takes the lower bound (1812 mya) of the 95% HPD. To summarize, the ML phylogeny, the topology tests and the molecular dating analysis indicate that an ancestral duplication of the rpoH gene occurred some 2214 mya within the alphaproteobacteria, after the split between the gamma and the alphaproteobacteria, and yielded two rpoH groups. Furthermore, whereas the rpoH1 group has experienced cases of HGT no such cases were found in the rpoH2 group. However this latter group appears to have endured several instances of gene loss.
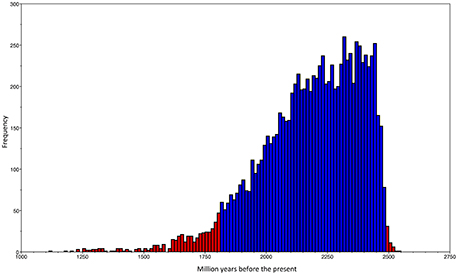
Figure 2. Dating of the duplication event. Frequency histogram of the traces of the origin of the duplication event that gave rise to the two rpoH groups.The blue bars show the 95% highest posterior density (HPD) interval, whereas the red bars give all the values not included within the HPD.
Variable Purifying Selection along the rpoH Gene and Similar Amino Acid Rates between the rpoH Groups
Next, we wanted to know if any of the rpoH groups have signals for positive selection and to that end we used the ratio dN/dS (see methods). This ratio is commonly used to infer the type and intensity of selection: where dN/dS >1 implies diversifying (positive) selection; dN/dS < 1 indicates purifying (negative) selection and dN/dS = 1 means neutral evolution. The program codeml implements different models of codon evolution and these models can be compared by means of likelihood ratio tests (LRT). Therefore, we employed this program to conduct several types of analysis to infer the type—and magnitude—of selection acting on these genes. We first obtained a Maximum Likelihood (ML) estimate of dN/dS for the whole gene. This value was 0.09446, which indicates a very strong purifying selection. However, this is an average estimate that cannot reflect how selection varies across time or among sites. Importantly, as different parts of a gene could be subject to different selection pressures, we also ran other models that allowed us to test for variability in dN/dS across codons; these are known as “site models” (Yang, 2007). We carried out three LRTs that allowed us to compare pairs of models as follows: (1) LRT for testing variable selection among codons (M0 vs. M3); (2) LRT for testing the presence of codons subject to positive selection (M1a vs. M2a); (3) alternative LRT to test for the presence of codons subject to positive selection (M7 vs. M8). The test for variable selection among codons (M0 vs. M3) was significant (LRT = 4347.887, prob = 0) implying that different parts of the gene have experienced different levels of purifying selection (see below). However, neither of the tests for codons subject to positive selection was significant: M1a vs. M2a LRT = 0 (prob = 1)—similar results were obtained with the other LRT (M7 vs. M8). Hence, we did not find any signals of codons under diversifying selection. To further explore how selection has changed across the gene we plotted the mean posterior probability values of dN/dS for every codon (see Figure 3). The level of purifying selection shows no constancy among the codons of the gene (see Figure 3), as there were some codons under very strong selection—values of dN/dS lower than 0.1 and approaching to 0-, whereas others were under weak purifying selection (dN/dS higher than 0.2). Furthermore, many sites (codons) in the amino-terminus of the protein seem to have experienced mild purifying selection as their dN/dS values are higher than 0.1 (see Figure 3). Notably, the codons that codify for the RpoH box and the region 2.4 were under strong purifying selection. On the other hand, we found clear evidence of relaxed purifying selection in the region 4.2—this is the region that interacts with the −35 motif of the RpoH-dependent genes. This analysis shows that strong purifying selection is the main type of selection. However, the intensity of purifying selection is not uniform along the gene.
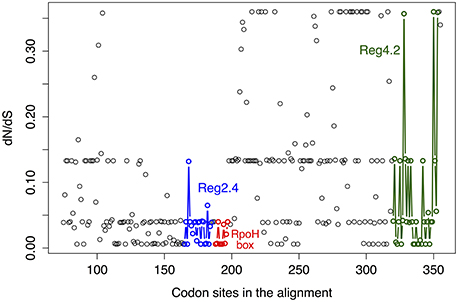
Figure 3. Variability in dN/dS among codons. Mean posterior probability values of dN/dS for the codon alignment. These values depend on the maximum likelihood estimates for dN/dS under the M3 model and were calculated via the Naïve Empirical Bayes method. The color code shows regions codifying some relevant parts of the RpoH protein and is as follows: blue circles, region 2.4 that interacts with the −10 motif of the RpoH-dependent genes; red circles, conserved region that is particular to rpoH genes; green circles, region 4.2 that interacts with the −35 motif of the RpoH-dependent genes.
In order to test the equality of amino acid evolutionary rate between the two rpoH groups, we conducted the Tajima's relative test (Tajima, 1993). For this test we employed the two copies of R. etli CFN42 and E. coli K-12 as an out group, the null hypothesis of equal rates between the rpoH groups was not rejected as the chi-square (chisq) test statistic was 0.49 (prob = 0.48384, 1 df). We also carried out Tajima's relative test using the two rpoH copies of other species—B. abortus 9-941 (chisq = 1.86, prob = 0.17245), Jannaschia sp. CCS1 (chisq = 3.46, prob = 0.06289), and R. sphaeroides 2.4.1 (chisq = 2.80, prob = 0.09426)—and again the null hypothesis of equality of evolutionary rate could not be rejected. Therefore, it seems that the amino acid evolutionary rate of RpoH has remained rather constant over time. To sum up, the dN/dS analysis indicates that although strong purifying selection is the main type of selection, its intensity is not uniform along the gene and the Tajima's relative test implies that similar amino acid evolutionary rate between the two rpoH groups.
Different Promoter Organization for rpoH1 and rpoH2
Thus far, we have only considered the coding region of the gene—either the proteins sequences for the ML phylogeny, dating analysis, Tajima's relative test or DNA for the selection analysis—but a fundamental aspect of any gene is its transcriptional regulation. Therefore, to understand how the expression of rpoH1 and rpoH2 might have changed we conducted a promoter analysis. For this analysis we decided to focus only on the Rhizobiales order for two reasons: first, because non-coding regions are more difficult to align and, therefore, we restrained this analysis to a small evolutionary scale and, second, because previous experimental studies have mapped and characterize the promoters of these genes in some species from this order (Martinez-Salazar et al., 2009a; Barnett et al., 2012; Schluter et al., 2013). We used two approaches to identify the potential promoter signals (see Section Materials and Methods): first, we used a set of matrices constructed from promoters previously characterized (MacLellan et al., 2006; Martinez-Salazar et al., 2009b; Barnett et al., 2012; Schluter et al., 2013) and, secondly, we assembled de novo promoters from our set of upstream regions. The use of these two approaches allowed us to identify promoters that were highly represented (de novo promoters) and those that were poorly represented (using matrices). First, we found that all members from the rpoH1 group had promoter signals within the first 80–100 nt of the upstream region (see Figure 4), whereas most of the members of the rpoH2 group showed promoter signals far apart (>200 nt of the upstream region); the only exception was R. tropici CIAT899 which had promoter signals in the first 30–40 nt of the upstream region. Secondly, we noted that 75% of members of the rpoH1 group had promoter signals that indicate that it could be very likely under transcriptional control of σ70 and σ32 (see Figure 4). Notably, there is a considerably overlap between the promoter signals for σ70 and σ32. There were only four cases that just had promoter signals for σ32. In contrast, most members (80%) of the rpoH2 group only had promoter signals for just one sigma factor, namely σ24 (see Figure 4). A particular case is R. tropici CIAT899 that also had promoter signals for σ70 (see Figure 4). However, 4 strains did not show promoter signals for any of the sigmas that we considered; three of them are R. etli strains (MiM1, CFN42, and CIAT652) and S. fredi 257. Taken together these results indicate that very likely the two rpoH groups are under the control of different sigmas; even more, whereas the rpoH2 group has promoter signals for just one sigma, the rpoH1 group not only has promoter signals for two sigmas but these signals tend to overlap to a considerable extent. Additionally, the promoter signals are located at different distances for each rpoH group. In considering these results, it is clear that rpoH1 and rpoH2 likely have a different promoter organization and different promoter selectivity.
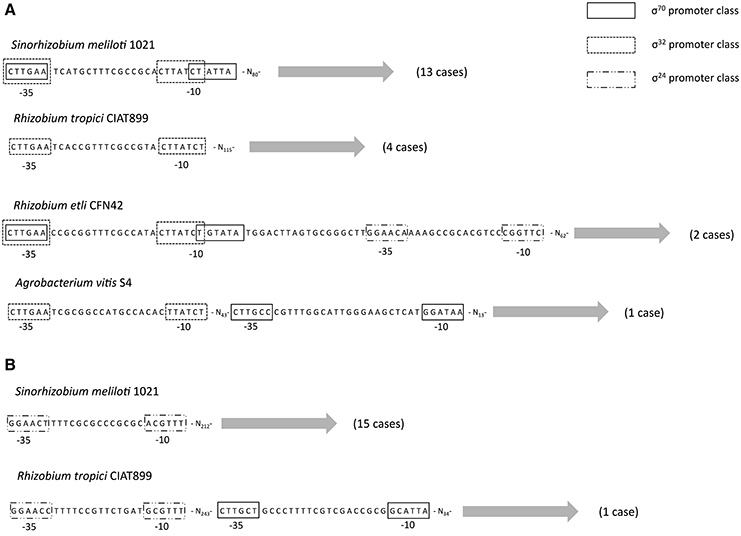
Figure 4. Potential promoter organization. Schematic representation of the potential promoter organization for rpoH1 (A) and rpoH2 (B). Putative promoters classes for σ70, σ32, and σ24 are shown as boxes. The most common promoter organization is shown first—exemplified by S. meliloti 1021 in both cases (A,B)—and then the least frequent cases are shown below, in parentheses are given the number of strains that show such promoter organization.
Discussion
We decided to focus on the alphaproteobacteria for a couple of reasons. First, there are previous reports that have shown that in this class some genomes have more than one rpoH gene (Narberhaus et al., 1997; Green and Donohue, 2006; Martinez-Salazar et al., 2009a). Secondly, previous phylogenomic analyses have established the species tree of some species from this class (Gupta and Mok, 2007; Castillo-Ramirez and Gonzalez, 2008) and this was of paramount importance for some part of the analyses that we carried out. The data set that we gathered covers the main orders of the alphaproteobacteria. In order to better understand the origins of the rpoH homologs, we used fully sequenced genomes, which have the virtue of providing an entire and unbiased view of the number of rpoH copies within each strain. To the best of our knowledge, this is the first study that has tried to establish the evolutionary forces that have molded the evolutionary history of any sigma factor.
It is a commonplace occurrence in alphaproteobacteria to have more than one rpoH homologs per genome. We found that 53% of the alphaproteobacteria genomes have two (or more) rpoH homologs and only few of them presented just one homolog. This is in agreement with previous experimental studies that have found that bacteria, such as R. etli (Martinez-Salazar et al., 2009a) or R. sphaeroides (Green and Donohue, 2006), have two members of the RpoH family. There are two possible explanations for the occurrence of extra rpoH homologs in a genome: they could have been introduced by HGT or they could have been originated through duplication. Our phylogenetic analysis shows that both processes have shaped the evolutionary history of rpoH. We note that most the rpoH homologs fall within two major groups and, given that the species relationships within each group is similar to that of the species tree—apart from the HGT cases-, we think that an ancient gene duplication is the most parsimonious explanation. Even more, our topology test analysis further reinforces this possibility. It is interesting to note that, according to our estimates, the ancient duplication occurred some time (95% HPD 1812–2491) after the Great Oxidation Event (the first significant increase in atmospheric oxygen) that is thought to have occurred around 2450–2320 mya (Bekker et al., 2004; Bekker and Kaufman, 2007)—even more so, considering that some rpoH2 genes are involved in the oxidative stress response (Martinez-Salazar et al., 2009a; Nuss et al., 2009; Dufour et al., 2012; Jans et al., 2013). However, the pattern generated by this ancient duplication has been peppered with HGT events. We did not detect any HGT between the gamma and alphaproteobacteria, which is expected given that this gene is highly connected and recently it has been shown that high gene connectivity curbs HGT (Cohen et al., 2011). However, we did find some events of HGT within the alphaproteobacteria and more specifically within the rpoH1 group.
While rpoH1 is clearly involved in heat-shock stress, experimental studies have shown that rpoH2 is related to other stress conditions in different bacteria; for instance, in R. sphaeroides rpoH2 has been implicated in oxygen stress (Dufour et al., 2012), while in R. etli (Martinez-Salazar et al., 2009a) it has been shown to be involved in the osmotic stress. Although RpoH2 proteins have shown not to be essential, they are bound to be relevant in the environment in which these bacteria dwell; along these lines we found that most of them are located on the chromosome and not on plasmids. Furthermore, in the case of R. etli it is known that genes that code for RpoH1 and RpoH2 belong to the core genome (Rosa Isela Santamaría personal communication). Notably, as far as the gammaproteobacteria are concerned, only one rpoH homolog per genome was found, suggesting that duplication events or even HGT introducing new rpoH genes have not been successful. Another salient trend that emerges from our analysis is the fact that rpoH homologs have been differentially conserved in each group. Clearly, whereas almost all the alphaproteobacteria genomes here analyzed have rpoH1 homologs, not all of them present rpoH2 homologs; in this regard, both the Sphingomonadales and the Rickettsiales only have rpoH1. Therefore, these gene loss events only affected the rpoH2 homologs and we think this also reflects the more essential nature of rpoH1 homologs. Of note, there was only one genome, Rickettsia bellis OSU, for which it was not possible to find either of the rpoH genes. Although the BLAST search found a hit for rpoH1 group, this hit did not pass our alignment criteria and therefore was not considered in our analysis. The presence of just rpoH1 in the Rickettsiales is not unexpected, as this group of obligate intracellular bacteria is known to have endured severe reductive genome processes (Darby et al., 2007; Renvoise et al., 2011).
Our selection analysis indicates that for most of the history of rpoH selection has acted to eliminate non-synonymous mutations—most of which are likely to be deleterious. Remarkably, we did not find evidence for sites under positive selection nor did we find evidence for a change in the amino acid evolutionary rate over time. Hence, the strong level of purifying selection is conserved over time. This notion that purifying selection is the main form of selection acting on this gene agrees with the findings that rpoH1 and rpoH2 are able to complement the temperature sensitivity of an E. coli rpoH mutant (Narberhaus et al., 1997; Green and Donohue, 2006; Martinez-Salazar et al., 2009a); in other words, selection has purged many of the changes that affect the protein initial function and, therefore, even rpoH2, which has been implicated in several stress conditions, is able to perform the function of the E. coli rpoH. However, we note that the level of purifying selection is variable and that some parts of the gene are more conserved than others. For instance, the region that codifies for the RpoH box (red dots, Figure 3)—a characteristic sequence of amino acid that is found in σ32 homologs—is under strong purifying selection and so does the region 2.4 (blue dots, Figure 3), which interacts with −10 motif of RpoH-dependent genes. On the other hand, we found clear evidence of relaxed purifying selection in the region 4.2—this region interacts with the −35 motif of the RpoH-dependent genes. This might help to explain the fact that rpoH1 and rpoH2 control—aside from a set of overlapping genes—distinct sets of genes, if one assumes that these sets of genes have different promoters. Actually, the fact that region 4.2 has accumulated more non-synonymous changes matches a recent finding by Barnett et al. (2012) in that study, when they looked at RpoH-dependent genes they found that the RpoH1-specific promoter sequence for the −35 and −10 motifs was CTTGAA-N15−16-CCTATAT, whereas that for RpoH2 was CTTGCC-N15−16-CCTATCT. If one compares the specific promoter sequences from that study, it is clear that for the −35 motif there are two differences (the last two adenines are changed to cytosines), while for the −10 motif there is only one difference (the last adenine is changed for a thymine). In other words, we found a more variable region 4.2 and comparing the −35 and −10 motifs of the RpoH-dependent genes, from Barnett et al. (2012), a more variable −35 motif is evident. Therefore, it might be that within the interaction between the region 4.2 and the −35 motif from the RpoH-dependent genes is where the differentiation to control different sets of genes lies. All in all, it seems that our selection analysis along with previous molecular studies—showing that different rpoH copies complement the E. coli rpoH mutant, but at the same time, these different copies control different sets of genes—suggest that there have been subtle protein functional changes between RpoHs encoded by different rpoH genes within a genome.
To understand how a gene evolves, it is of great significance to know how its coding sequence has changed over time, yet another important aspect is how the gene expression of this gene has changed over time. In our study as proxy for the latter aspect, we carried out an analysis to detect promoter motifs for both rpoH1 and rpoH2. Our promoter analysis suggests that rpoH1 and rpoH2 show different promoter selectivity. For instance, we note that whereas rpoH1 has promoter signals implying it is under transcriptional control of σ70 and σ32, rpoH2 only has promoter signals for σ24. We do not think that the results of our promoter analysis are spurious, as some previous experimental studies have characterized the promoters of the rpoH genes for some Rhizobiales species (Martinez-Salazar et al., 2009a; Schluter et al., 2013)—actually, that is why we chose this order to conduct our promoter analysis—and their findings agree with our promoter analysis. For instance, using 5′Rapid Amplification of cDNA Ends (RACE) to determine the transcription start sites of the rpoH genes in R. etli CFN42, it was found that rpoH1 is under transcriptional control of σ70, whereas rpoH2 shows promoter signals for σ24 (Martinez-Salazar et al., 2009a). Furthermore, another study also using 5′RACE determined that rpoH2 shows promoter signals for σ24 in S. meliloti 1021 (Schluter et al., 2013). Even experimental studies on other order (Rhodobacterales) support our findings, for example it has been shown that in R. sphaeroides rpoH2 is under the control of σ24 (Anthony et al., 2005; Dufour et al., 2008; Nuss et al., 2009) and thus further support the finding that rpoH2 has promoter signals for σ24. Interestingly, not only rpoH1 and rpoH2 have different potential promoters types but also these seem to be located at different distances in each gene. Our promoter analysis, supported by the experimental studies mentioned above, indicates that rpoH1 and rpoH2 are differentially expressed. The general picture that emerges from our analyses is that after the duplication (peppered with cases of HGT and gene loss), rather than changing substantially the protein function, selection has modified the promoter regions so that the activity of these σ32 factors is differentially controlled at the transcriptional level. Notably similar trends have been described in fungi (Wapinski et al., 2007), where it seems that duplicated genes diverge more frequently in their regulation and much less frequently in the biochemical nature of their functions.
Our analyses have dealt with long-term evolution rather than short-term evolution; however, it is important to consider how these extra copies were generated in the first place. It is important to mention that gene duplication—oftentimes also called gene amplification—could have an adaptive role in dosage response to stressful conditions over short-term evolutionary scales (Kondrashov, 2012). We note that, besides the dosage response scenario, there are other possibilities that could account for the benefits of duplications (Fares, 2015); such is the case of mutational and regulatory robustness (Fares et al., 2013; Keane et al., 2014; Fares, 2015). Remarkably, gene duplication has been often observed playing a part in heavy-metal tolerance, drug-resistance, and survival in stressful environments not only in bacteria but also in eukaryotes (Kondrashov, 2012). For instance, when six lines of E. coli were exposed to stressful high temperatures, several duplication and deletion events were identified (Riehle et al., 2001); notably, genes within the duplicated regions were related to stress and starvation conditions and the timing of these events was concurrent with an increase in the relative fitness of the strains. Hence, in order to better understand the origin of these extra copies and, therefore, have a more comprehensive view of the evolution of these sigma factors, we plan to conduct further research at much shorter evolutionary scales, such as those concerning recently emerged bacterial clones (Castillo-Ramirez et al., 2012) and recently originated species (Joseph et al., 2015).
In summary, the molecular evolution of the rpoH gene within the alphaproteobacteria appears to be shaped by an ancient duplication, with subsequent HGT and gene loss events, variable purifying selection across the gene and functional differentiation of the promoter regions. In a more general sense, by means of a phylogenomic approach, we were able to decipher some of the major evolutionary drivers of an important alternative sigma factor. This work shows that with the huge amount of genomes publicly available studies focusing on evolution of sigma factors are feasible and desirable to fully understand not only the nature of them but also, and more importantly, the complex net of bacterial gene regulation. We anticipate our study to be a point of reference for subsequent evolutionary studies of this and other sigma factor families.
Author Contributions
SC designed, supervised, and coordinated the study. GL conducted the blast searches, carried out the statistical model selection, and performed the promoter analysis. SC constructed the ML phylogenies, carried out the topology tests, conducted the molecular dating analysis, and ran the selection analysis as well as the Tajima's relative test. MC participated in the general discussion. SC and GL wrote the manuscript. MC critically revised the manuscript. All the authors reviewed and approved the manuscript.
Funding
This work was supported by “Programa de Apoyo a Proyectos de Investigación e Innovación Tecnológica PAPIIT,” grant number IA200515 to SC. Funding for SC was also provided by the Programa de Genomica Evolutiva, CCG-UNAM.
Conflict of Interest Statement
The authors declare that the research was conducted in the absence of any commercial or financial relationships that could be construed as a potential conflict of interest.
Acknowledgments
We are grateful to Bruno Contreras for helpful discussions on the promoter analyses and to Luis Lozano for his skillful bioinformatics support. We thank the three reviewers that went through our manuscript for their insightful comments. SC also thanks Timothy Read for his useful comments on the manuscript. SC thanks Canela for her advice on the graphical design of Figure 1.
Supplementary Material
The Supplementary Material for this article can be found online at: http://journal.frontiersin.org/article/10.3389/fmicb.2016.00581
References
Abascal, F., Zardoya, R., and Posada, D. (2005). ProtTest: selection of best-fit models of protein evolution. Bioinformatics 21, 2104–2105. doi: 10.1093/bioinformatics/bti263
Altschul, S. F., Gish, W., Miller, W., Myers, E. W., and Lipman, D. J. (1990). Basic local alignment search tool. J. Mol. Biol. 215, 403–410. doi: 10.1016/S0022-2836(05)80360-2
Anthony, J. R., Warczak, K. L., and Donohue, T. J. (2005). A transcriptional response to singlet oxygen, a toxic byproduct of photosynthesis. Proc. Natl. Acad. Sci. U.S.A. 102, 6502–6507. doi: 10.1073/pnas.0502225102
Barnett, M. J., Bittner, A. N., Toman, C. J., Oke, V., and Long, S. R. (2012). Dual RpoH sigma factors and transcriptional plasticity in a symbiotic bacterium. J. Bacteriol. 194, 4983–4994. doi: 10.1128/JB.00449-12
Bekker, A., Holland, H. D., Wang, P. L., Rumble, D. III, Stein, H. J., Hannah, J. L., et al. (2004). Dating the rise of atmospheric oxygen. Nature 427, 117–120. doi: 10.1038/nature02260
Bekker, A., and Kaufman, A. J. (2007). Oxidative forcing of global climate change: a biogeochemical record across the oldest Paleoproterozoic ice age in North America. Earth Planet. Sci. Lett. 258, 486–499. doi: 10.1016/j.epsl.2007.04.009
Bukau, B. (1993). Regulation of the Escherichia coli heat-shock response. Mol. Microbiol. 9, 671–680. doi: 10.1111/j.1365-2958.1993.tb01727.x
Castillo-Ramirez, S., Corander, J., Marttinen, P., Aldeljawi, M., Hanage, W. P., Westh, H., et al. (2012). Phylogeographic variation in recombination rates within a global clone of methicillin-resistant Staphylococcus aureus. Genome Biol. 13:R126. doi: 10.1186/gb-2012-13-12-r126
Castillo-Ramirez, S., and Gonzalez, V. (2008). Factors affecting the concordance between orthologous gene trees and species tree in bacteria. BMC Evol. Biol. 8:300. doi: 10.1186/1471-2148-8-300
Ciccarelli, F. D., Doerks, T., von Mering, C., Creevey, C. J., Snel, B., and Bork, P. (2006). Toward automatic reconstruction of a highly resolved tree of life. Science 311, 1283–1287. doi: 10.1126/science.1123061
Cohen, O., Gophna, U., and Pupko, T. (2011). The complexity hypothesis revisited: connectivity rather than function constitutes a barrier to horizontal gene transfer. Mol. Biol. Evol. 28, 1481–1489. doi: 10.1093/molbev/msq333
Darby, A. C., Cho, N. H., Fuxelius, H. H., Westberg, J., and Andersson, S. G. (2007). Intracellular pathogens go extreme: genome evolution in the Rickettsiales. Trends Genet. 23, 511–520. doi: 10.1016/j.tig.2007.08.002
Drummond, A. J., and Rambaut, A. (2007). BEAST: Bayesian evolutionary analysis by sampling trees. BMC Evol. Biol. 7:214. doi: 10.1186/1471-2148-7-214
Dufour, Y. S., Imam, S., Koo, B. M., Green, H. A., and Donohue, T. J. (2012). Convergence of the transcriptional responses to heat shock and singlet oxygen stresses. PLoS Genet. 8:e1002929. doi: 10.1371/journal.pgen.1002929
Dufour, Y. S., Landick, R., and Donohue, T. J. (2008). Organization and evolution of the biological response to singlet oxygen stress. J. Mol. Biol. 383, 713–730. doi: 10.1016/j.jmb.2008.08.017
Edgar, R. C. (2004). MUSCLE: a multiple sequence alignment method with reduced time and space complexity. BMC Bioinformatics 5:113. doi: 10.1186/1471-2105-5-113
Fares, M. A. (2015). The origins of mutational robustness. Trends Genet. 31, 373–381. doi: 10.1016/j.tig.2015.04.008
Fares, M. A., Keane, O. M., Toft, C., Carretero-Paulet, L., and Jones, G. W. (2013). The roles of whole-genome and small-scale duplications in the functional specialization of Saccharomyces cerevisiae genes. PLoS Genet. 9:e1003176. doi: 10.1371/journal.pgen.1003176
Feklistov, A., Sharon, B. D., Darst, S. A., and Gross, C. A. (2014). Bacterial sigma factors: a historical, structural, and genomic perspective. Annu. Rev. Microbiol. 68, 357–376. doi: 10.1146/annurev-micro-092412-155737
Galibert, F., Finan, T. M., Long, S. R., Puhler, A., Abola, P., Ampe, F., et al. (2001). The composite genome of the legume symbiont Sinorhizobium meliloti. Science 293, 668–672. doi: 10.1126/science.1060966
Gonzalez, V., Santamaria, R. I., Bustos, P., Hernandez-Gonzalez, I., Medrano-Soto, A., Moreno-Hagelsieb, G., et al. (2006). The partitioned Rhizobium etli genome: genetic and metabolic redundancy in seven interacting replicons. Proc. Natl. Acad. Sci. U.S.A. 103, 3834–3839. doi: 10.1073/pnas.0508502103
Green, H. A., and Donohue, T. J. (2006). Activity of Rhodobacter sphaeroides RpoHII, a second member of the heat shock sigma factor family. J. Bacteriol. 188, 5712–5721. doi: 10.1128/JB.00405-06
Gruber, T. M., and Gross, C. A. (2003). Multiple sigma subunits and the partitioning of bacterial transcription space. Annu. Rev. Microbiol. 57, 441–466. doi: 10.1146/annurev.micro.57.030502.090913
Guindon, S., Dufayard, J. F., Lefort, V., Anisimova, M., Hordijk, W., and Gascuel, O. (2010). New algorithms and methods to estimate maximum-likelihood phylogenies: assessing the performance of PhyML 3.0. Syst. Biol. 59, 307–321. doi: 10.1093/sysbio/syq010
Gupta, R. S., and Mok, A. (2007). Phylogenomics and signature proteins for the alpha proteobacteria and its main groups. BMC Microbiol. 7:106. doi: 10.1186/1471-2180-7-106
Hedges, S. B., Dudley, J., and Kumar, S. (2006). TimeTree: a public knowledge-base of divergence times among organisms. Bioinformatics 22, 2971–2972. doi: 10.1093/bioinformatics/btl505
Hedges, S. B., Marin, J., Suleski, M., Paymer, M., and Kumar, S. (2015). Tree of life reveals clock-like speciation and diversification. Mol. Biol. Evol. 32, 835–845. doi: 10.1093/molbev/msv037
Helmann, J. D. (2002). The extracytoplasmic function (ECF) sigma factors. Adv. Microb. Physiol. 46, 47–110. doi: 10.1016/S0065-2911(02)46002-X
Jans, A., Vercruysse, M., Gao, S., Engelen, K., Lambrichts, I., Fauvart, M., et al. (2013). Canonical and non-canonical EcfG sigma factors control the general stress response in Rhizobium etli. Microbiologyopen 2, 976–987. doi: 10.1002/mbo3.137
Joseph, S. J., Marti, H., Didelot, X., Castillo-Ramirez, S., Read, T. D., and Dean, D. (2015). Chlamydiaceae genomics reveals interspecies admixture and the recent evolution of Chlamydia abortus infecting lower mammalian species and humans. Genome Biol. Evol. 7, 3070–3084. doi: 10.1093/gbe/evv201
Kaneko, T., Nakamura, Y., Sato, S., Minamisawa, K., Uchiumi, T., Sasamoto, S., et al. (2002). Complete genomic sequence of nitrogen-fixing symbiotic bacterium Bradyrhizobium japonicum USDA110 (supplement). DNA Res. 9, 225–256. doi: 10.1093/dnares/9.6.225
Keane, O. M., Toft, C., Carretero-Paulet, L., Jones, G. W., and Fares, M. A. (2014). Preservation of genetic and regulatory robustness in ancient gene duplicates of Saccharomyces cerevisiae. Genome Res. 24, 1830–1841. doi: 10.1101/gr.176792.114
Kondrashov, F. A. (2012). Gene duplication as a mechanism of genomic adaptation to a changing environment. Proc. Biol. Sci. 279, 5048–5057. doi: 10.1098/rspb.2012.1108
MacLellan, S. R., MacLean, A. M., and Finan, T. M. (2006). Promoter prediction in the rhizobia. Microbiology 152, 1751–1763. doi: 10.1099/mic.0.28743-0
Martinez-Salazar, J. M., Salazar, E., Encarnacion, S., Ramirez-Romero, M. A., and Rivera, J. (2009b). Role of the extracytoplasmic function sigma factor RpoE4 in oxidative and osmotic stress responses in Rhizobium etli. J. Bacteriol. 191, 4122–4132. doi: 10.1128/JB.01626-08
Martinez-Salazar, J. M., Sandoval-Calderon, M., Guo, X., Castillo-Ramirez, S., Reyes, A., Loza, M. G., et al. (2009a). The Rhizobium etli RpoH1 and RpoH2 sigma factors are involved in different stress responses. Microbiology 155, 386–397. doi: 10.1099/mic.0.021428-0
Medina-Rivera, A., Defrance, M., Sand, O., Herrmann, C., Castro-Mondragon, J. A., Delerce, J., et al. (2015). RSAT 2015: regulatory sequence analysis tools. Nucleic Acids Res. 43, W50–W56. doi: 10.1093/nar/gkv362
Nakahigashi, K., Yanagi, H., and Yura, T. (1995). Isolation and sequence analysis of rpoH genes encoding sigma 32 homologs from gram negative bacteria: conserved mRNA and protein segments for heat shock regulation. Nucleic Acids Res. 23, 4383–4390.
Narberhaus, F., Krummenacher, P., Fischer, H. M., and Hennecke, H. (1997). Three disparately regulated genes for sigma 32-like transcription factors in Bradyrhizobium japonicum. Mol. Microbiol. 24, 93–104. doi: 10.1046/j.1365-2958.1997.3141685.x
Nuss, A. M., Glaeser, J., and Klug, G. (2009). RpoH(II) activates oxidative-stress defense systems and is controlled by RpoE in the singlet oxygen-dependent response in Rhodobacter sphaeroides. J. Bacteriol. 191, 220–230. doi: 10.1128/JB.00925-08
Osterberg, S., del Peso-Santos, T., and Shingler, V. (2011). Regulation of alternative sigma factor use. Annu. Rev. Microbiol. 65, 37–55. doi: 10.1146/annurev.micro.112408.134219
Paget, M. S., and Helmann, J. D. (2003). The sigma70 family of sigma factors. Genome Biol. 4, 203. doi: 10.1186/gb-2003-4-1-203
Ramirez-Romero, M. A., Masulis, I., Cevallos, M. A., Gonzalez, V., and Davila, G. (2006). The Rhizobium etli sigma70 (SigA) factor recognizes a lax consensus promoter. Nucleic Acids Res. 34, 1470–1480. doi: 10.1093/nar/gkl023
Renvoise, A., Merhej, V., Georgiades, K., and Raoult, D. (2011). Intracellular Rickettsiales: insights into manipulators of eukaryotic cells. Trends Mol. Med. 17, 573–583. doi: 10.1016/j.molmed.2011.05.009
Rice, P., Longden, I., and Bleasby, A. (2000). EMBOSS: the European Molecular Biology Open Software Suite. Trends Genet. 16, 276–277. doi: 10.1016/S0168-9525(00)02024-2
Riehle, M. M., Bennett, A. F., and Long, A. D. (2001). Genetic architecture of thermal adaptation in Escherichia coli. Proc. Natl. Acad. Sci. U.S.A. 98, 525–530. doi: 10.1073/pnas.98.2.525
Schluter, J. P., Reinkensmeier, J., Barnett, M. J., Lang, C., Krol, E., Giegerich, R., et al. (2013). Global mapping of transcription start sites and promoter motifs in the symbiotic alpha-proteobacterium Sinorhizobium meliloti 1021. BMC Genomics 14:156. doi: 10.1186/1471-2164-14-156
Tajima, F. (1993). Simple methods for testing the molecular evolutionary clock hypothesis. Genetics 135, 599–607.
Tamura, K., Stecher, G., Peterson, D., Filipski, A., and Kumar, S. (2013). MEGA6: Molecular Evolutionary Genetics Analysis version 6.0. Mol. Biol. Evol. 30, 2725–2729. doi: 10.1093/molbev/mst197
Wapinski, I., Pfeffer, A., Friedman, N., and Regev, A. (2007). Natural history and evolutionary principles of gene duplication in fungi. Nature 449, 54–61. doi: 10.1038/nature06107
Keywords: molecular evolution, sigma factors, gene duplication, horizontal gene transfer, promoter differentiation, purifying selection
Citation: López-Leal G, Cevallos MA and Castillo-Ramírez S (2016) Evolution of a Sigma Factor: An All-In-One of Gene Duplication, Horizontal Gene Transfer, Purifying Selection, and Promoter Differentiation. Front. Microbiol. 7:581. doi: 10.3389/fmicb.2016.00581
Received: 17 December 2015; Accepted: 08 April 2016;
Published: 25 April 2016.
Edited by:
M. Pilar Francino, FISABIO - Generalitat Valenciana, SpainReviewed by:
David W. Dyer, Oklahoma University Health Sciences Center, USALeonor Sánchez-Busó, Wellcome Trust Sanger Institute, UK
Mario A. Fares, Consejo Superior de Investigaciones Científicas, Spain
Copyright © 2016 López-Leal, Cevallos and Castillo-Ramírez. This is an open-access article distributed under the terms of the Creative Commons Attribution License (CC BY). The use, distribution or reproduction in other forums is permitted, provided the original author(s) or licensor are credited and that the original publication in this journal is cited, in accordance with accepted academic practice. No use, distribution or reproduction is permitted which does not comply with these terms.
*Correspondence: Santiago Castillo-Ramírez, iago@ccg.unam.mx
†These authors have contributed equally to this work.