- 1Affiliated Hangzhou Xixi Hospital, Zhejiang University School of Medicine, Hangzhou, China
- 2Key Laboratory of Image Information Processing and Intelligent Control, School of Artificial Intelligence and Automation, Huazhong University of Science and Technology, Wuhan, China
- 3Department of Orthopedics Surgery, The Second Affiliated Hospital, Zhejiang University School of Medicine, Hangzhou, China
- 4Orthopedics Research Institute of Zhejiang University, Hangzhou, China
- 5Key Laboratory of Motor System Disease Research and Precision Therapy of Zhejiang Province, Hangzhou, China
Background: The available therapeutic options of bone defects, fracture nonunion, and osteoporosis remain limited, which are closely related to the osteogenic differentiation of bone marrow–derived mesenchymal stem cells (BMSCs). Thus, there remains an urgent demand to develop a prediction method to infer osteogenic differentiation–related genes in BMSCs.
Method: We performed differential expression analysis between hBMSCs and osteogenically induced samples. Association analysis, co-expression analysis, and PPI analysis are then carried out to identify potential osteogenesis-related regulators. GO enrichment analysis and GSEA are performed to identify significantly enriched pathways associated with AOX1. qRT-PCR and Western blotting were employed to investigate the expression of genes on osteogenic differentiation, and plasmid transfection was used to overexpress the gene AOX1 in hBMSCs.
Result: We identified 25 upregulated genes and 17 downregulated genes. Association analysis and PPI network analysis among these differentially expressed genes show that AOX1 is a potential regulator of osteogenic differentiation. GO enrichment analysis and GSEA show that AOX1 is significantly associated with osteoblast-related pathways. The experiments revealed that AOX1 level was higher and increased gradually in differentiated BMSCs compared with undifferentiated BMSCs, and AOX1 overexpression significantly increased the expression of osteo-specific genes, thereby clearly indicating that AOX1 plays an important role in osteogenic differentiation. Moreover, our method has ability in discriminating genes with osteogenic differentiation properties and can facilitate the process of discovery of new osteogenic differentiation–related genes.
Conclusion: These findings collectively demonstrate that AOX1 is an osteogenic differentiation-relevant gene and provide a novel method established with a good performance for osteogenic differentiation-relevant genes prediction.
Introduction
The rate of bone defects, fracture nonunion, and osteoporosis incidence continues to rise, and available therapeutic options remain limited. These diseases, closely related to the osteogenic differentiation of bone marrow–derived mesenchymal stem cells (BMSCs), have been confirmed by numerous studies (Marongiu et al., 2020; Xiao et al., 2020; Xiong et al., 2020; Jiang et al., 2021).
BMSCs possess self-renewal capabilities and the potential to differentiate into a variety of cell types, including osteoblasts, chondrocytes, and adipocytes (Bianco et al., 2001; Liao et al., 2017). As a key contributor to the bone formation, BMSCs are regulated by genetic factors (Zhang et al., 2018). However, relying solely on the experimental identification of genes that regulate osteogenic differentiation of BMSCs is generally costly and time-consuming, which leads to an urgent demand to develop a prediction method to infer osteogenic differentiation–related genes in a short time.
High-throughput sequencing including RNA-seq and microarray is a novel technique, which plays an important role in the exploration for genome‐level differences and is providing valuable insights into the landscape of the identification of key genes and functional pathways associated with osteogenic differentiation in BMSCs (Fan et al., 2020). In our study, we performed a bioinformatics-guided methodology to identify potential regulators of osteogenic differentiation. We first identified 25 upregulated and 17 downregulated genes between hBMSCs and osteogenically induced samples. Association analysis, co-expression analysis, and PPI analysis are then carried out to infer potential regulators. As a result, AOX1 is identified as a new potential regulator. Association analysis, GO enrichment analysis, and GSEA show that AOX1 is significantly associated with osteogenic differentiation.
Aldehyde oxidase (AOX) is a complex enzyme present in the cytosol of eukaryotes (Garattini et al., 2008; Garattini et al., 2009). In humans, only one AOX1 functional gene is present (Terao et al., 2006), which is expressed predominantly in the liver but detectable amounts are also found in other organs (Garattini and Terao, 2012). Although its physiological substrates are still unclear, AOX1 is suggested to have an important role in the metabolism of drugs and xenobiotics in the liver (Pryde et al., 2010; Garattini and Terao, 2011). However, its role with respect to the regulation of osteogenic differentiation has not previously been reported.
In this study, we examined the role of AOX1 in the osteogenic differentiation of human BMSCs (hBMSCs) through bioinformatics analysis and experimental verification. The results indicate that AOX1 plays an important role in the osteogenic differentiation via multiple pathways, meanwhile providing a novel method for the further study of osteogenic differentiation of human bone marrow–derived mesenchymal stem cells.
Result
Identification of Differentially Expressed Genes That Are Associated With Osteogenic Differentiation
To characterize the process of osteogenic differentiation, we performed differential expression analysis between hBMSCs and osteogenically induced samples using the limma R package (Ritchie et al., 2015). As a result, 42 genes are identified to be differentially expressed. Among all of these differentially expressed genes, 25 genes are upregulated and the remaining 17 genes are downregulated in the osteogenically induced samples (Figure 1A). Clustering analysis of samples based on these differentially expressed genes shows that samples within the same group tend to be clustered together (Figure 1B), suggesting that these differentially expressed genes can differentiate between the control group and osteogenically induced samples. Furthermore, in the osteogenically induced group, samples in the advanced stages of the osteogenic process are found to be clustered with each other, suggesting that the osteogenic stage can indeed affect the expression of these differentially expressed genes.
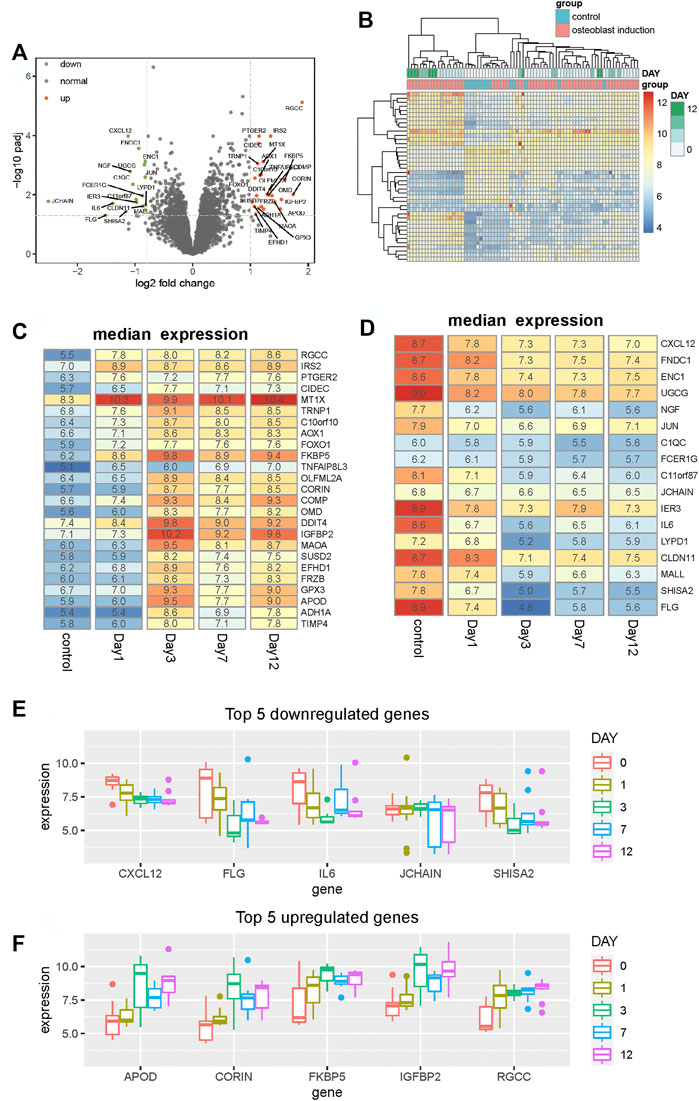
FIGURE 1. Identification of differentially expressed genes between hBMSCs and osteogenically induced samples. (A) Volcano plot shows the differentially expressed genes based on fold change and BH-adjusted p-value. Upregulated and downregulated genes are displayed using red and blue nodes, respectively. (B) Heat map shows the expression pattern of these differentially expressed genes. Samples are annotated into different groups. Day means the time of osteogenically induced samples in the differentiation process. (C,D) Heat map shows the median expression of upregulated and downregulated genes respectively for samples in different differentiation stages. (E,F) Boxplot shows the top five downregulated and upregulated genes respectively in the osteogenic process.
To analyze the expression variation of these differentially expressed genes during the osteogenic process and identify to which extent the osteogenic stage can affect the expression of these genes, we analyzed the expression of these differentially expressed genes of different osteogenic stages. The median expression of upregulated and downregulated genes is calculated respectively for samples within the same osteogenic stage. Among all upregulated genes, we found that nearly half of these genes tend to display expression variation after day 1, such as OLFML2A, CORIN, MAOA, SUSD2, FRZB, APOD, and ADH1A (Figure 1C). Moreover, in the downregulated genes, we observed similar variations. Some genes start downregulating after the osteogenic stage day 1 and then maintain stability in the following stages (Figure 1D). Among these differentially expressed genes, CXCL12, FLG, IL6, JCHAIN, and SHISA2 are the top five downregulated genes, and APOD, CORIN, FKBP5, IGFBP2, and RGCC are the top five upregulated genes. The expression profiles of these genes, presented in Figures 1E,F, show that the expression is distinct between different stages. These results collectively suggest that the osteogenic stage can indeed affect the expression of these genes, especially in the early stage of the osteogenic process.
Analysis of Osteoblast-Related Pathway in the Differentiation Process
Differentially expressed genes with osteogenic differentiation are analyzed, but the activity of osteoblast-related pathways during the osteogenic process is unknown. To analyze whether these osteoblast-related pathways are activated or inhibited, we collected 15 osteoblast-related pathways from the Molecular Signatures Database (MSigDb) (Subramanian et al., 2005). Single-sample gene set enrichment analysis is used to quantify the activity of osteoblast-related pathways and calculate an enrichment score for each pathway of individual sample. Similar with differentially expressed genes, we found that samples within the same group and stage tend to be clustered together based on the enrichment score of these osteoblast-related pathways (Figure 2A), suggesting that osteogenic stages can indeed affect the activity of these pathways.
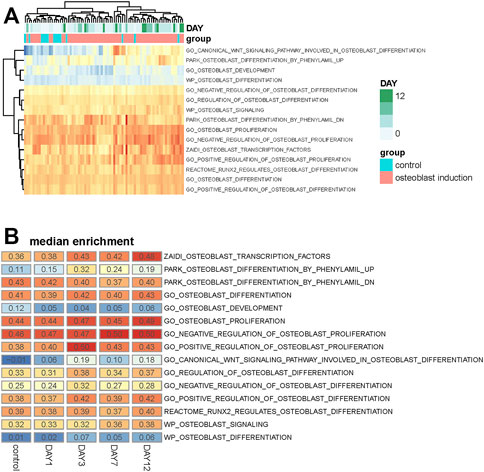
FIGURE 2. Analysis of osteoblast-related pathways in the osteogenic process. (A) Heat map shows the enrichment score of 15 osteoblast-related pathways across all samples. Samples are annotated into different groups and days. (B) Heat map shows the median value of the enrichment score of these osteoblast-related pathways across different osteogenic stages.
The median enrichment score of these osteoblast-related pathways for different osteogenic stages is calculated to identify differentially enriched pathways. We found that most of these osteoblast-related pathways tend to display upregulated trends during the osteogenic process (Figure 2B). Four representative pathways are then identified to be upregulated, in which three are associated with osteogenic differentiation and one is associated with osteoblast transcription factors (Figure 3A), suggesting that osteoblast differentiation–related pathways are specifically enhanced and activated during the osteogenic process. Interestingly, one pathway associated with osteoblast development is found to be downregulated during the osteogenic process (Figure 3B), suggesting that the differentiation and development of hBMSCs are mutually exclusive during the osteogenic process. The enrichment pattern of these pathways is presented in Figure 3C.
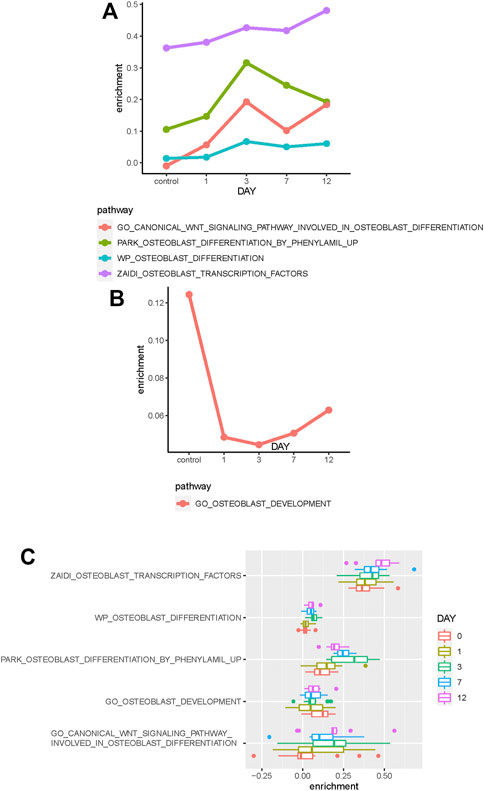
FIGURE 3. Identification of differentially enriched pathways. (A) Four osteoblast-related pathways tend to be upregulated in the osteogenic process. (B) One osteoblast-related pathway tends to be downregulated in the osteogenic process. (C) Boxplot shows these differentially enriched pathways of samples. Samples are divided into different groups based on osteogenic days.
Selection of Candidate Genes That Are Associated With Osteogenic Differentiation
To identify candidate genes that are significantly associated with the osteogenic process, we performed association analysis between these differentially expressed genes and osteoblast-related pathways. Most of these genes are found to be positively or negatively associated with osteoblast-related pathways (Figure 4A). Furthermore, analysis shows that upregulated genes tend to be positively associated with these pathways, such as C10orf10, AOX1, OLFML2A, CORIN, COMP, OMD, DDIT4, MAOA, and SUSD2, suggesting that these genes are significant determinants in promoting the osteogenic process. Analysis of downregulated genes shows that they are more likely to be negatively correlated with these osteoblast-related pathways, such as UGCG, NGF, C11orf87, LYPD1, CLDN11, and MALL, suggesting significant regulation of these genes in inhibiting the osteogenic process.
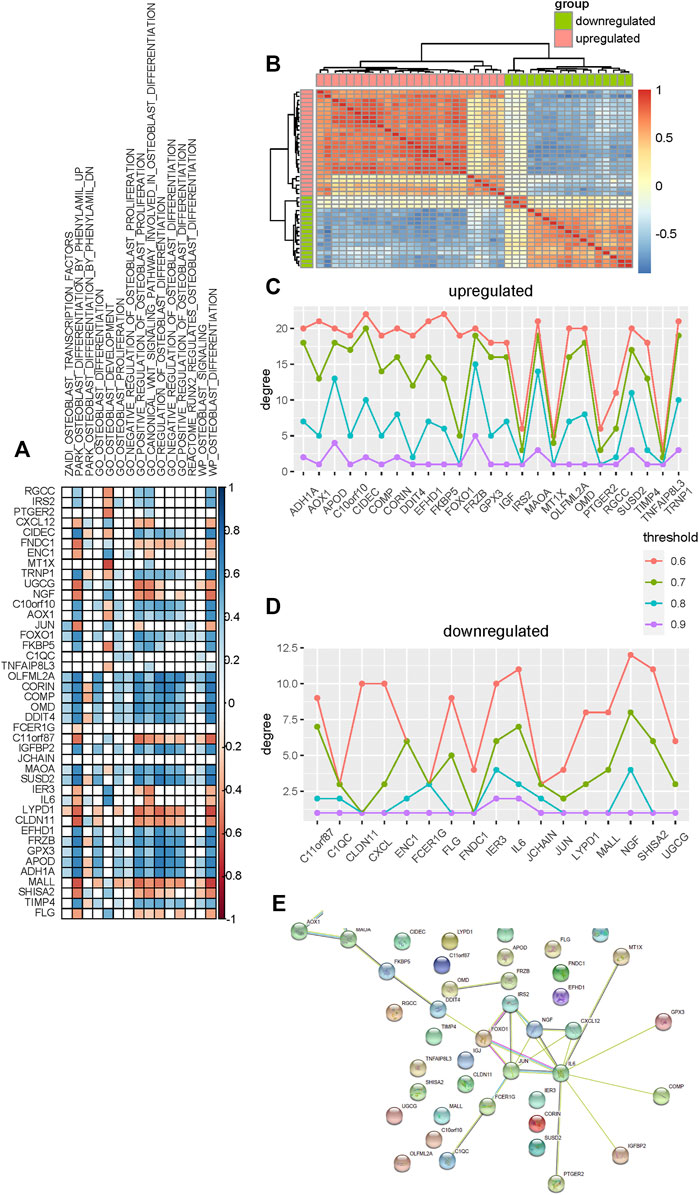
FIGURE 4. Selection of candidate genes that are significantly associated with the osteogenic process. (A) Association analysis between these differentially expressed genes and osteoblast-related pathways. Blue and red colors indicate the positive and negative correlations, respectively. Blank indicates that there is no significance. (B) Heat map shows the co-expression pattern of these differentially expressed genes. Association is evaluated using the Pearson correlation coefficient. (C,D) Degree of upregulated and downregulated genes in the co-expression network constructed using different thresholds. (E) Protein–protein interaction network of these differentially expressed genes is constructed.
Co-expression analysis is then used to identify candidate genes associated with osteogenic differentiation. We evaluated the expression association among these differentially expressed genes based on the Pearson correlation coefficient and constructed the co-expression network for upregulated and downregulated genes respectively (Figure 4B). To make sure that the co-expression network is reliable and hub genes identified indeed capture the topological structure of the network, the network is constructed based on a varied association threshold from 0.6 to 0.9. Degree analysis for these upregulated co-expression networks shows that MAOA, FRZB, CIDEC, TRNP1, and APOD are hub genes (Figure 4C). Analysis for downregulated co-expression networks shows that NGF, IL6, and IER3 are hub genes (Figure 4D).
Additionally, the protein–protein interaction network is also used to identify hub genes that may be associated with the osteogenic process. We constructed a protein–protein interaction network for these differentially expressed genes using the STRING database (Szklarczyk et al., 2019) and then rank these genes by degree. As a result, nine genes are identified as hub genes based on the degree (Figure 4E). Among these hub genes, ADH1A, AOX1, MAOA, IRS2, and FOXO1 are upregulated and JUN, NGF, IL6, and CXCL12 are downregulated.
Based on these results, a few genes are identified as potential targets that may promote or inhibit osteogenic differentiation during the osteogenic process. Among these candidate genes, some of them are involved in osteoblast-related pathways, and some genes have been previously studied to be associated with osteogenic differentiation. These genes are then removed from the candidate gene set. In the remaining candidate genes, AOX1 has not yet been studied extensively and no literature reports its relationship with osteogenic differentiation. Due to the strong statistical evidence of AOX1 in the osteogenic process, it is necessary and important to elucidate the interaction between AOX1 and osteogenic differentiation.
AOX1 Plays an Important Role in the Process of Osteogenic Differentiation
To determine the potential role of AOX1 in regulating the osteogenic process, we analyzed the association between AOX1 and osteoblast-related pathways. We found that AOX1 expression is positively associated with the enrichment of eight osteoblast-related pathways, in which two are associated with osteoblast proliferation and six are associated with osteogenic differentiation (Figure 5A), suggesting that AOX1 is a potential regulator in the osteogenic process.
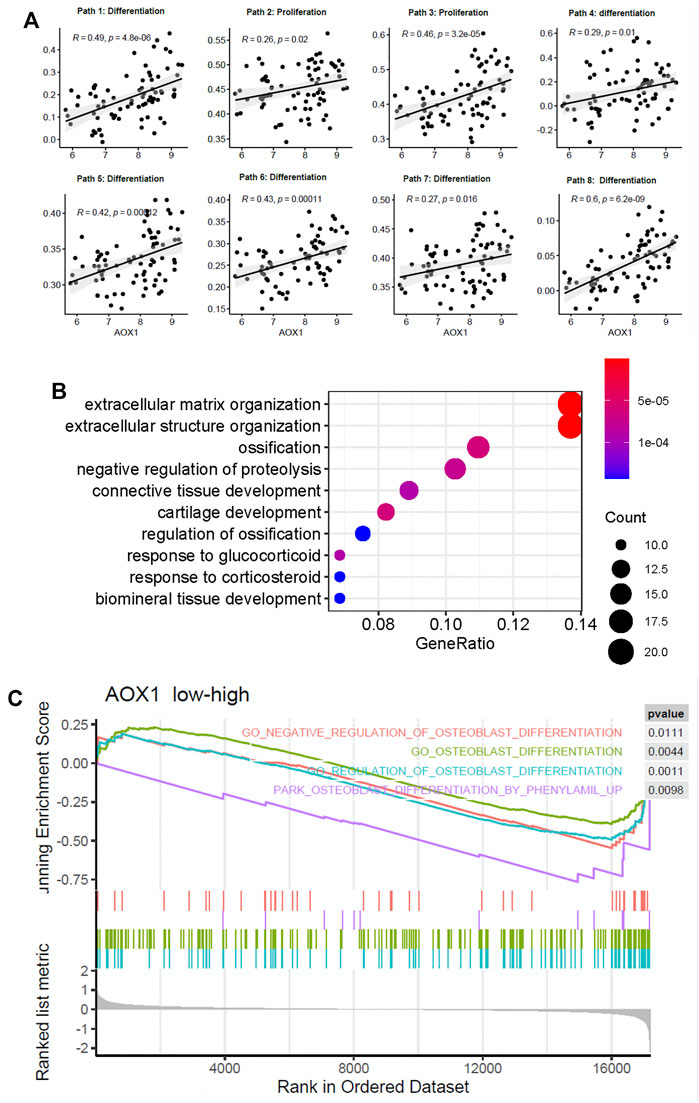
FIGURE 5. AOX1 is a potential gene positively regulating the osteogenic process. (A) Point diagram shows the positive correlation between AOX1 expression and the enrichment score of osteogenic pathways (the detailed information of osteogenic pathways are presented in Supplementary Table). (B) Gene ontology enrichment analysis of these differentially expressed genes between the high AOX1 expression group and the low expression group. (C) Gene set enrichment analysis between the high AOX1 expression group and the low expression group.
Additionally, we divided samples into high AOX1 expression and low expression groups based on the median of AOX1 expression. Differential expression analysis is applied between these two groups to identify differentially expressed genes associated with AOX1. As a result, 152 genes are identified as differentially expressed between these two groups. The GO enrichment analysis is then used to identify whether these differentially expressed genes are enriched in some specific pathways. The GO enrichment analysis shows that these genes are enriched in ossification and regulation of ossification, further demonstrating the regulation of AOX1 in the osteogenic process. The top 10 enriched pathways are presented in Figure 5B.
Apart from the GO enrichment analysis, the gene set enrichment analysis is used to determine whether some pathways are enriched in a specific AOX1 group. All genes ranked by fold change between AOX1 groups are inputted into the gene set enrichment analysis, and 15 osteoblast differentiation–related pathways are inputted to analyze whether these genes are enriched in specific pathways. As a result, four osteoblast differentiation–related pathways are found to be enriched in the high-AOX1 expression group (Figure 5C). In conclusion, all these results suggest that AOX1 is indeed a potential regulator in the process of osteogenic differentiation.
AOX1 Level Was Higher and Increased Gradually in Differentiated BMSCs Compared With Undifferentiated BMSCs
To determine the expression level of AOX1 associated with osteogenic differentiation of MSCs, we examined endogenous AOX1 expression in hBMSCs at days 0, 1, 3, and 7. Compared with undifferentiated BMSCs, both the mRNA (Figure 6A) and protein expression (Figures 6B,C) of AOX1 were higher and increased gradually in osteogenic differentiation.
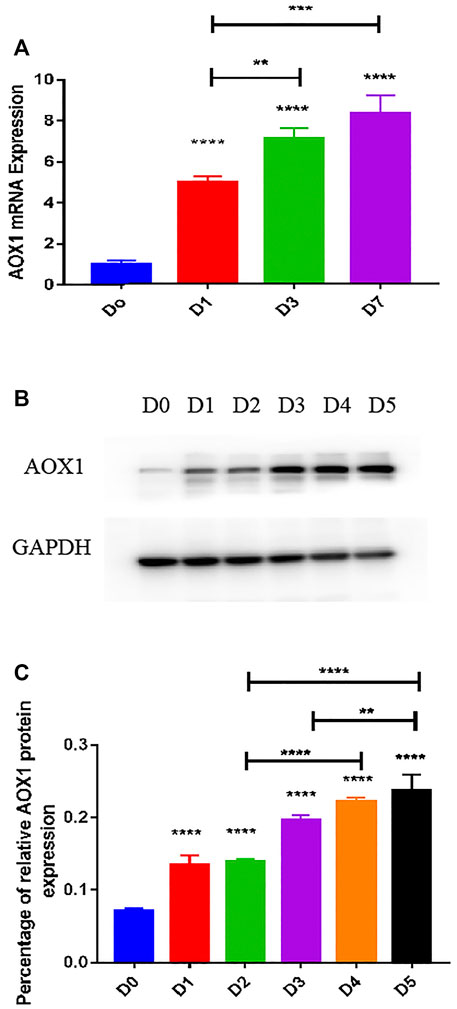
FIGURE 6. Endogenous AOX1 mRNA expression and the endogenous AOX1 protein expression on osteogenic differentiation of hBMSCs. (A) Endogenous expression of AOX1 mRNA was determined by qPCR at days 0, 1, 3, and 7 of osteogenic differentiation. (B) Endogenous expression of AOX1 protein was determined by Western blotting analysis at days 0, 1, 2, 3, 4, and 5 of osteogenic differentiation (C) Relative quantitative analysis of Western blot analyses for AOX1, data are used by percentage and expressed as the mean ± SD.
AOX1 Overexpression in hBMSCs
To clarify the role of AOX1 in osteogenic differentiation, plasmid transfection was used to efficiently overexpress AOX1 of third-generation hBMSCs. AOX1 expression was quantified by qRT-PCR after infection. Compared with the Ctrl-OE (control-overexpression) group, the mRNA expression of AOX1 was increased in the AOX1-OE (AOX1-overexpression) group (Figure 7E).
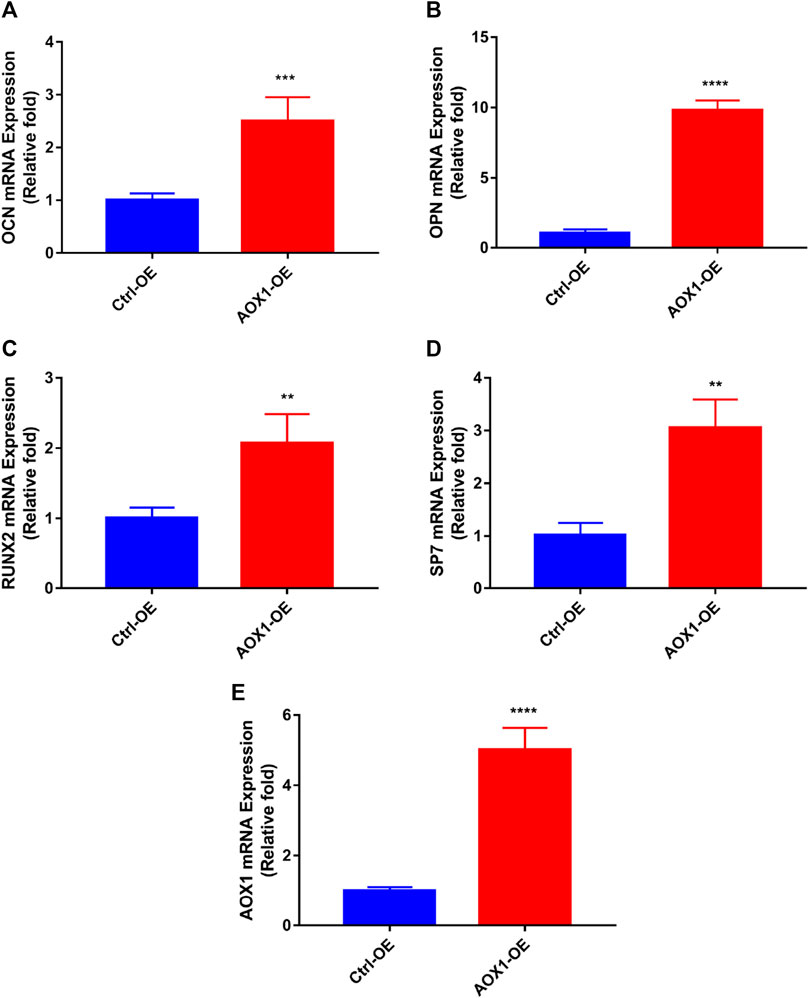
FIGURE 7. Effects of AOX1 overexpression on osteo-specific genes. (A–D) Relative mRNA expression of osteo-specific genes and (E) AOX1 on day three of osteogenesis after AOX1 overexpression. The mRNA expression levels were normalized to that of 18S ribosomal RNA.
AOX1 Overexpression Increased the Levels of Osteo-Specific Genes
To evaluate the effect of AOX1 overexpression in osteogenic differentiation, the levels of osteo-specific genes including the runt-related transcription factor 2 (RUNX2), osteocalcin (OCN), osteopontin (OPN), and osterix (specificity protein 7, SP7) were determined by quantitative real-time PCR (qRT-PCR). qRT-PCR analysis revealed that RUNX2, OCN, OPN, and SP7 mRNA levels were significantly higher in the AOX1 overexpression group than in the control-overexpression group at day three(p<0.05, Figures 7A–D).
Discussion
Mesenchymal stem cells have been proved to be ideal seed cells for bone tissue engineering and are closely associated with bone defects fracture nonunion and osteoporosis. Better understanding of the molecular mechanism in osteogenesis will enable researchers to design suitable targets for more effectively inducing bone tissue regeneration and treat related diseases. Microarray technology enables us to explore the genetic alterations and identify novel biomarkers in mesenchymal stem cells osteogenesis.
In this study, we performed differential expression analysis between hBMSCs and osteogenically induced samples and identified differentially expressed genes. These genes are found to be significantly associated with osteoblast-related pathways, suggesting a significant value of these genes in regulating osteogenic pathways. The co-expression and PPI network analyses show that AOX1 is a newly discovered potential regulator in osteogenic differentiation of hBMSCs. The GO analysis and GSEA among different AOX1 groups show that osteoblast-related pathways are significantly associated with AOX1 expression.
To verify the gene AOX1 associated with osteogenic differentiation, we performed preliminary verification in hBMSCs. We report here that significant upregulation of AOX1 during the osteogenic differentiation of hBMSCs through the results of PCR and Western blotting. This study revealed that AOX1 overexpression significantly promotes osteogenesis and increases the expression of key genes that regulate the induction of osteogenesis, thereby clearly indicating that AOX1 plays an important role in osteogenic differentiation. As part of a study that was directed toward elucidating the mechanism of action of AOX1, expression of ROS, involved in the osteogenic differentiation (Tao et al., 2020), thereby clearly indicating that AOX1 plays an important role in osteogenic differentiation.
AOX1 is a molybdo-flavoenzyme with complex evolutionary profile, catalyzing the oxidation of a wide variety of substrates (Garattini and Terao, 2012), which is involved in electron transfer (Coelho et al., 2015). The important aspect of AOX1 is that it contributes to the endogenous production of H2O2 as reactive oxygen species (ROS), thereby regulating intracellular signaling pathways and determining cell proliferation, apoptosis, migration, and differentiation (Kundu et al., 2007; Gough and Cotter, 2011). It is currently believed that absolute levels of ROS is harmful under pathological conditions, whereas moderately elevated ROS have beneficial effects on osteogenic differentiation (Guzik and Harrison, 2006; Mandal et al., 2011; Li et al., 2017; Rossi et al., 2017; Zhu et al., 2018; Gardin et al., 2020; Khalid et al., 2020; Tao et al., 2020; Li et al., 2021). ROS enables induction of FOXO activation, which promotes bone formation in part by modulating the differentiation of osteoblastic cells (Ambrogini et al., 2010) and is also known to suppress the expression and transcriptional activity of PPARγ (a potent repressor of osteoblastogenesis) (Armoni et al., 2006). FOXO1 acts upstream of RUNX2 induction and plays an important role in promoting osteogenic differentiation and suppressing proliferation in differentiating cells (Siqueira et al., 2011).
AOX1 is one of the key enzymes of tryptophan catabolism and loss of AOX1 may lead to the accumulation of kynurenine and NADP (Vantaku et al., 2020). Meanwhile, kynurenine impacts MSCs and causes age-related bone loss (Anaya et al., 2020). AOX1 also contributes to ATRA biosynthesis (Zhong et al., 2021). ATRA-induced osteogenic differentiation of mesenchymal stem cells and its ability to influence osteogenic differentiation has been observed in numerous cell systems (Zhang et al., 2016; Ahmed et al., 2019; Weng et al., 2019).
However, the underlying molecular mechanisms accounting for the role of AOX1 in regulating osteogenic differentiation in hBMSCs need further research. Moreover, these results also suggest that our method has the ability in discriminating genes with osteogenic differentiation properties and can facilitate the process of discovery of new osteogenic differentiation-related genes.
Although more research is necessary to confirm the detailed mechanism during osteogenic differentiation, the present findings indicate that the gene AOX1 plays an important role in the osteogenic differentiation of stem cells and are thus worthy of further exploration. Furthermore, we developed a novel way for the prediction of osteogenic differentiation-relevant genes, and can thus provide potential biomarkers and targets for osteogenic differentiation of stem cells.
Method
Data Collection
Gene expression profiles of hBMSCs and osteogenically induced samples are collected from gene expression omnibus (GEO). Four datasets GSE12266, GSE18043, GSE37558, and GSE80614 are downloaded to identify osteogenic differentiation–related genes. GSE12266 contains four hBMSCs and 12 osteogenically induced samples. GSE18043 contains three hBMSCs and nine osteogenically induced samples (Granchi et al., 2010). GSE37558 contains four hBMSCs and 12 osteogenically induced samples (Alves et al., 2014). GSE80614 contains three hBMSCs and 30 osteogenically induced samples. For probes that did not match any gene, we removed them from the expression profiling matrix. For genes that match multiple probes, we averaged the signal intensity of these probes as the expression of the matched gene. To eliminate the platform-based bias and create an integrated dataset, we combined these four datasets using the combat algorithm, which is implemented using the sva R package. In total, 14 controls and 63 osteogenically induced samples are included in our analysis.
MSigDb is an integrated database of annotated gene sets from different sources. Osteoblast-related pathways are retrieved from this database. To identify gene sets that are associated with osteoblast, we search for related gene sets from all of these annotated names using the keyword osteoblast. As a result, 15 gene sets whose name contains osteoblast are identified as osteoblast-related pathways. Among these pathways, one is associated with the osteoblast transcription factor, nine are associated with osteogenic differentiation, one is associated with osteoblast development, three are associated with osteoblast proliferation, and one is associated with osteoblast signaling.
Single-Sample Gene Set Enrichment Analysis
Single-sample gene set enrichment analysis (ssGSEA) is used to evaluate the activity of a specific pathway in an individual sample. It calculates an enrichment score of a pathway based on the relative expression of these genes involved in the pathway. In this study, we calculated the enrichment score of these 15 osteoblast-related pathways for all samples. The ssGSEA is conducted using gsva R package (Hänzelmann et al., 2013).
Differential Expression Analysis
Combined expression profiling dataset is used to identify differentially expressed genes between hBMSCs and osteogenically induced samples. limma R package is then applied to identify these genes differentially expressed between the control and osteogenic induction groups based on BH-adjusted p-value and fold change. A gene is identified as upregulated if BH-adjusted p-value <0.05 and log-transformed fold change >1, and downregulated if BH-adjusted p-value <0.05 and log-transformed fold change <−0.8. The reason why we use −0.8 as the threshold for the identification of downregulated genes is that the threshold −1 will lead to a limited number of downregulated genes. As a result, 25 genes are identified as upregulated and 17 genes are identified as downregulated.
Construction of the Co-Expressed Network
The Pearson correlation coefficient is used to evaluate the co-expression pattern for these differentially expressed genes. Upregulated genes and downregulated genes are analyzed separately to identify hub genes in the co-expressed network. The correlation matrix is first calculated for upregulated genes. Due to the uncertainty of the threshold to construct a co-expressed network, we construct multiple networks based on the varied correlation threshold from 0.6 to 0.9. After analyzing the hub genes of each co-expressed network, we can create a final set of hub genes based on the frequency of hub identified in a single network. We note that the hub genes identified based on this method are reliable and can indeed capture the topological structure of multiple networks compared with a single network. This method is then applied in downregulated genes to identify hub genes that are associated with the osteogenic process.
Functional Enrichment Analysis
GO enrichment analysis is used to identify pathways that are associated with AOX1. We first divided samples into high AOX1 expression group and low expression group based on the median expression of AOX1. Differentially expressed genes are then identified between these two groups. The GO enrichment analysis is used to identify pathways that are presented by these differentially expressed genes. BH-adjusted p-value <0.05 is considered to be statistically significant. The GO enrichment analysis is conducted using the clusterProfiler R package (Yu et al., 2012).
Gene Set Enrichment Analysis Between Different AOX1 Groups
Gene set enrichment analysis is used to identify pathways that are associated with the AOX1 group. Differential expression analysis between AOX1 groups has been performed earlier. All genes are ranked based on the fold change between AOX1 groups. The ranked gene list and osteoblast-related pathways are then inputted into the gene set enrichment analysis to identify whether osteoblast-related pathways are enriched in the top or the bottom of the ranked gene list. This analysis is conducted using the clusterProfiler R package.
Cell Culture, Reagents, and Antibodies
hBMSCs were provided by Cyagen Biosciences (Guangzhou, China), which can differentiate into osteoblasts, adipocytes, and chondrocytes under specific inductive conditions. Adherent hBMSCs were incubated in culture flasks in the hBMSC special growth medium (Cyagen Biosciences, Inc., Guangzhou, China) in a cell incubator contained at 37 °C with 5% CO2 and were passaged at nearly 80–90% confluence. Cells from passages 2–6 were used in subsequent experiments. Specific antibodies against glyceraldehyde-3-phosphate dehydrogenase (GAPDH) and AOX1 were purchased from Proteintech Group. Inc. (Chicago, United States).
Osteogenic Differentiation Protocol
hBMSCs were cultured in the special growth medium (Cyagen Biosciences, Guangzhou, China) and 100 IU/ml penicillin/streptomycin) in six- or twelve-well cell culture plates at a density of 3 × 104/cm2 and incubated for 48 h at 37 °C under 5% CO2. Subsequently, the cells were cultured in osteogenic induction medium (L-DMEM with 10% FBS, 100 IU/ml penicillin/streptomycin, 100 nM dexamethasone, 0.2 mM ascorbic acid, and 10 mM β-glycerophosphate). The cells were maintained by the replacement of fresh osteogenic induction medium every 2–3 days.
Plasmid Transfection
AOX1 plasmid transfection and negative control plasmid were used for overexpression experiments (Genomeditech). hBMSCs were prepared at 50–60% confluence in six-well plates. The transfection mixture was prepared by adding 200 μL Opti-MEM containing 4 μL TransMate reagent (GenePharma) to 200 μL Opti-MEM containing 0.66ug AOX1 plasmid and incubating for 10 min at room temperature. The transfection mixture was added in six-well plates containing 800ul α-MEM with FBS. After transfection for 4 h, the medium was changed to the growth medium.
RNA Extraction and Quantitative RT-PCR
Levels of the osteoblast formation gene expression were measured using quantitative RT-PCR (qRT-PCR). hBMSCs (3 × 104 cells/cm2) were cultured in six-well plates with the medium. Total cellular RNA was isolated and measured using the RNAiso reagent (Takara Bio, Kusatsu, Japan) and NanoDrop 2000. The absorbance of the samples at 260 nm was calculated in accordance with the manufacturer’s instructions (Thermo Fisher Scientific, MA, United States). Total RNA was reverse-transcribed into complementary DNA (cDNA) in a 20 μL reaction volume (Takara). A total of 2 μL cDNA was used as the template with Power SYBR® Green PCR Master Mix (Takara), and qRT-PCR was performed in triplicate by the ABI 7500 system (Thermo Fisher Scientific); 18S was used as housekeeping genes and each reaction was repeated three times independently. GenePharma synthesized the primer AOX1 and Sangon Biotech (Shanghai, China) synthesized the other primers used in this experiment. Primer sequences are listed in Table 1. The qRT-PCR reaction was at 95°C for 30 s, followed by 45 cycles at 95°C for 5 s, and 60°C for 30 s. The expression levels of all of the genes were evaluated by the 2−△△Ct method.
Western Blotting Analysis
Cells were lysed for 30 min on ice in a RIPA buffer containing phosphatase and protease inhibitor cocktails (Beyotime, Shanghai, China). The centrifugation to clear the lysates and collect the supernatants was set at 14,000 rpm for 10 min at 4°C. Equal amounts of proteins were separated by 10% sodium dodecyl sulfate polyacrylamide gel electrophoresis and then transferred to a polyvinylidene fluoride membrane (Millipore, Shanghai, China) which was then probed with the primary antibodies. The membranes were then blocked for 1 h at room temperature in Tris-buffered saline containing 10% non-fat milk and 0.1% Tween. Subsequently, the membranes were incubated with primary antibodies overnight at 4°C. After washing with 0.1% Tween in Tris-buffered saline for three times and incubation with horseradish peroxidase–conjugated secondary antibodies (anti-rabbit; Beyotime) for 1 h at room temperature, proteins were visualized by an enhanced chemiluminescent detection reagent (MilliporeSigma) and an XRS chemiluminescence detection system (Bio-Rad Laboratories, Hercules, CA, United States).
Statistical Analysis
Statistical analysis was performed using GraphPad Prism v.7.0 (GraphPad Software, San Diego, CA, United States). All experiments were performed at least in triplicate. Data are presented as the mean ± SD. Statistical significance was determined using a two-tailed Student’s t test when comparing two groups, and one-way ANOVA followed by Tukey’s post hoc test was used when comparing more than two groups. A value of p- ≤ 0.05 was considered to indicate statistical significance.
Data Availability Statement
The datasets presented in this study can be found in online repositories. The names of the repository/repositories and accession number(s) can be found in the article/Supplementary Material.
Author Contributions
Conception: LS and LL interpretation or analysis of data: JM and JC preparation of the manuscript: LS and LL, JM experiment: LL and LS revision for important intellectual content: ZP, LL and LS supervision: LL and ZP.
Funding
The National Natural Science Foundation of China (Grant No. 81672147 and 81874007), Zhejiang Provincial Natural Science Foundation (Y21H070011) and Medical Health Science and Technology Project of Zhejiang Provincial Health Commission (Grant No.2021KY939) funds were received in support of this work. The authors declare no relevant financial activities outside the submitted work.
Conflict of Interest
The authors declare that the research was conducted in the absence of any commercial or financial relationships that could be construed as a potential conflict of interest.
Publisher’s Note
All claims expressed in this article are solely those of the authors and do not necessarily represent those of their affiliated organizations, or those of the publisher, the editors, and the reviewers. Any product that may be evaluated in this article, or claim that may be made by its manufacturer, is not guaranteed or endorsed by the publisher.
Acknowledgments
We thank all clients from the Clinical Research Center of the Second Affiliated Hospital, Zhejiang University. We also appreciate the general help of Lingl Zhang and Sm Feng from the Orthopedic Research Center of the Second Affiliated Hospital, Zhejiang University.
References
Ahmed, M. F., El-Sayed, A. K., Chen, H., Zhao, R., Yusuf, M. S., Zuo, Q., et al. (2019). Comparison between Curcumin and All-Trans Retinoic Acid in the Osteogenic Differentiation of Mouse Bone Marrow Mesenchymal Stem Cells. Exp. Ther. Med. 17, 4154–4166. doi:10.3892/etm.2019.7414
Alves, R. D., Eijken, M., van de Peppel, J., and van Leeuwen, J. P. (2014). Calcifying Vascular Smooth Muscle Cells and Osteoblasts: Independent Cell Types Exhibiting Extracellular Matrix and Biomineralization-Related Mimicries. BMC Genomics 15, 965. doi:10.1186/1471-2164-15-965
Ambrogini, E., Almeida, M., Martin-Millan, M., Paik, J.-H., Depinho, R. A., Han, L., et al. (2010). FoxO-mediated Defense against Oxidative Stress in Osteoblasts Is Indispensable for Skeletal Homeostasis in Mice. Cel Metab. 11, 136–146. doi:10.1016/j.cmet.2009.12.009
Anaya, J. M., Bollag, W. B., Hamrick, M. W., and Isales, C. M. (2020). The Role of Tryptophan Metabolites in Musculoskeletal Stem Cell Aging. Int. J. Mol. Sci. 21, 6670. doi:10.3390/ijms21186670
Armoni, M., Harel, C., Karni, S., Chen, H., Bar-Yoseph, F., Ver, M. R., et al. (2006). FOXO1 Represses Peroxisome Proliferator-Activated Receptor-Γ1 and -γ2 Gene Promoters in Primary Adipocytes: A Novel Paradigm To Increase Insulin Sensitivity. J. Biol. Chem. 281, 19881–19891. doi:10.1074/jbc.m600320200
Bianco, P., Riminucci, M., Gronthos, S., and Robey, P. G. (2001). Bone Marrow Stromal Stem Cells: Nature, Biology, and Potential Applications. Stem Cells 19, 180–192. doi:10.1634/stemcells.19-3-180
Coelho, C., Foti, A., Hartmann, T., Santos-Silva, T., Leimkühler, S., and Romão, M. J. (2015). Structural Insights into Xenobiotic and Inhibitor Binding to Human Aldehyde Oxidase. Nat. Chem. Biol. 11, 779–783. doi:10.1038/nchembio.1895
Fan, T., Qu, R., Yu, Q., Sun, B., Jiang, X., Yang, Y., et al. (2020). Bioinformatics Analysis of the Biological Changes Involved in the Osteogenic Differentiation of Human Mesenchymal Stem Cells. J. Cel Mol Med 24, 7968–7978. doi:10.1111/jcmm.15429
Garattini, E., Fratelli, M., and Terao, M. (2008). Mammalian Aldehyde Oxidases: Genetics, Evolution and Biochemistry. Cell. Mol. Life Sci. 65, 1019–1048. doi:10.1007/s00018-007-7398-y
Garattini, E., Fratelli, M., and Terao, M. (2009). The Mammalian Aldehyde Oxidase Gene Family. Hum. Genomics 4, 119–130. doi:10.1186/1479-7364-4-2-119
Garattini, E., and Terao, M. (2011). Increasing Recognition of the Importance of Aldehyde Oxidase in Drug Development and Discovery. Drug Metab. Rev. 43, 374–386. doi:10.3109/03602532.2011.560606
Garattini, E., and Terao, M. (2012). The Role of Aldehyde Oxidase in Drug Metabolism. Expert Opin. Drug Metab. Toxicol. 8, 487–503. doi:10.1517/17425255.2012.663352
Gardin, C., Bosco, G., Ferroni, L., Quartesan, S., Rizzato, A., Tatullo, M., et al. (2020). Hyperbaric Oxygen Therapy Improves the Osteogenic and Vasculogenic Properties of Mesenchymal Stem Cells in the Presence of Inflammation In Vitro. Int. J. Mol. Sci. 21, 1452. doi:10.3390/ijms21041452
Gough, D. R., and Cotter, T. G. (2011). Hydrogen Peroxide: a Jekyll and Hyde Signalling Molecule. Cell Death Dis 2, e213. doi:10.1038/cddis.2011.96
Granchi, D., Ochoa, G., Leonardi, E., Devescovi, V., Baglìo, S. R., Osaba, L., et al. (2010). Gene Expression Patterns Related to Osteogenic Differentiation of Bone Marrow-Derived Mesenchymal Stem Cells DuringEx VivoExpansion. Tissue Eng. C: Methods 16, 511–524. doi:10.1089/ten.tec.2009.0405
Guzik, T. J., and Harrison, D. G. (2006). Vascular NADPH Oxidases as Drug Targets for Novel Antioxidant Strategies. Drug Discov. Today 11, 524–533. doi:10.1016/j.drudis.2006.04.003
Hänzelmann, S., Castelo, R., and Guinney, J. (2013). GSVA: Gene Set Variation Analysis for Microarray and RNA-Seq Data. BMC Bioinformatics 14, 7. doi:10.1186/1471-2105-14-7
Jiang, Y., Zhang, P., Zhang, X., Lv, L., and Zhou, Y. (2021). Advances in Mesenchymal Stem Cell Transplantation for the Treatment of Osteoporosis. Cell Prolif 54, e12956. doi:10.1111/cpr.12956
Khalid, S., Yamazaki, H., Socorro, M., Monier, D., Beniash, E., and Napierala, D. (2020). Reactive Oxygen Species (ROS) Generation as an Underlying Mechanism of Inorganic Phosphate (Pi)-Induced Mineralization of Osteogenic Cells. Free Radic. Biol. Med. 153, 103–111. doi:10.1016/j.freeradbiomed.2020.04.008
Kundu, T. K., Hille, R., Velayutham, M., and Zweier, J. L. (2007). Characterization of Superoxide Production from Aldehyde Oxidase: an Important Source of Oxidants in Biological Tissues. Arch. Biochem. Biophys. 460, 113–121. doi:10.1016/j.abb.2006.12.032
Li, Q., Gao, Z., Chen, Y., and Guan, M.-X. (2017). The Role of Mitochondria in Osteogenic, Adipogenic and Chondrogenic Differentiation of Mesenchymal Stem Cells. Protein Cell 8, 439–445. doi:10.1007/s13238-017-0385-7
Li, X., Li, B., Shi, Y., Wang, C., and Ye, L. (2021). Targeting Reactive Oxygen Species in Stem Cells for Bone Therapy. Drug Discov. Today 26, 1226–1244. doi:10.1016/j.drudis.2021.03.002
Liao, J., Tian, T., Shi, S., Xie, X., Ma, Q., Li, G., et al. (2017). The Fabrication of Biomimetic Biphasic CAN-PAC Hydrogel with a Seamless Interfacial Layer Applied in Osteochondral Defect Repair. Bone Res. 5, 17018. doi:10.1038/boneres.2017.18
Mandal, C. C., Ganapathy, S., Gorin, Y., Mahadev, K., Block, K., Abboud, H. E., et al. (2011). Reactive Oxygen Species Derived from Nox4 Mediate BMP2 Gene Transcription and Osteoblast Differentiation. Biochem. J. 433, 393–402. doi:10.1042/bj20100357
Marongiu, G., Dolci, A., Verona, M., and Capone, A. (2020). The Biology and Treatment of Acute Long-Bones Diaphyseal Fractures: Overview of the Current Options for Bone Healing Enhancement. Bone Rep. 12, 100249. doi:10.1016/j.bonr.2020.100249
Pryde, D. C., Dalvie, D., Hu, Q., Jones, P., Obach, R. S., and Tran, T.-D. (2010). Aldehyde Oxidase: an Enzyme of Emerging Importance in Drug Discovery. J. Med. Chem. 53, 8441–8460. doi:10.1021/jm100888d
Ritchie, M. E., Phipson, B., Wu, D., Hu, Y., Law, C. W., Shi, W., et al. (2015). Limma powers Differential Expression Analyses for RNA-Sequencing and Microarray Studies. Nucleic Acids Res. 43, e47. doi:10.1093/nar/gkv007
Rossi, M. C., Bezerra, F. J. B., Silva, R. A., Crulhas, B. P., Fernandes, C. J. C., Nascimento, A. S., et al. (2017). Titanium-released from Dental Implant Enhances Pre-osteoblast Adhesion by ROS Modulating Crucial Intracellular Pathways. J. Biomed. Mater. Res. 105, 2968–2976. doi:10.1002/jbm.a.36150
Siqueira, M. F., Flowers, S., Bhattacharya, R., Faibish, D., Behl, Y., Kotton, D. N., et al. (2011). FOXO1 Modulates Osteoblast Differentiation. Bone 48, 1043–1051. doi:10.1016/j.bone.2011.01.019
Subramanian, A., Tamayo, P., Mootha, V. K., Mukherjee, S., Ebert, B. L., Gillette, M. A., et al. (2005). Gene Set Enrichment Analysis: a Knowledge-Based Approach for Interpreting Genome-wide Expression Profiles. Proc. Natl. Acad. Sci. 102, 15545–15550. doi:10.1073/pnas.0506580102
Szklarczyk, D., Gable, A. L., Lyon, D., Junge, A., Wyder, S., Huerta-Cepas, J., et al. (2019). STRING V11: Protein-Protein Association Networks with Increased Coverage, Supporting Functional Discovery in Genome-wide Experimental Datasets. Nucleic Acids Res. 47, D607–D613. doi:10.1093/nar/gky1131
Tao, H., Ge, G., Liang, X., Zhang, W., Sun, H., Li, M., et al. (2020). ROS Signaling Cascades: Dual Regulations for Osteoclast and Osteoblast. Acta Biochim. Biophys. Sin (Shanghai) 52, 1055–1062. doi:10.1093/abbs/gmaa098
Terao, M., Kurosaki, M., Barzago, M. M., Varasano, E., Boldetti, A., Bastone, A., et al. (2006). Avian and Canine Aldehyde Oxidases. J. Biol. Chem. 281, 19748–19761. doi:10.1074/jbc.m600850200
Vantaku, V., Putluri, V., Bader, D. A., Maity, S., Ma, J., Arnold, J. M., et al. (2020). Epigenetic Loss of AOX1 Expression via EZH2 Leads to Metabolic Deregulations and Promotes Bladder Cancer Progression. Oncogene 39, 6265–6285. doi:10.1038/s41388-019-0902-7
Weng, Z., Wang, C., Zhang, C., Xu, J., Chai, Y., Jia, Y., et al. (2019). All-Trans Retinoic Acid Promotes Osteogenic Differentiation and Bone Consolidation in a Rat Distraction Osteogenesis Model. Calcif Tissue Int. 104, 320–330. doi:10.1007/s00223-018-0501-6
Xiao, B., Wang, G., and Li, W. (2020). Weighted Gene Correlation Network Analysis Reveals Novel Biomarkers Associated with Mesenchymal Stromal Cell Differentiation in Early Phase. PeerJ 8, e8907. doi:10.7717/peerj.8907
Xiong, Y., Cao, F., Chen, L., Yan, C., Zhou, W., Chen, Y., et al. (2020). Identification of Key microRNAs and Target Genes for the Diagnosis of Bone Nonunion. Mol. Med. Rep. 21, 1921–1933. doi:10.3892/mmr.2020.10996
Yu, G., Wang, L.-G., Han, Y., and He, Q.-Y. (2012). clusterProfiler: an R Package for Comparing Biological Themes Among Gene Clusters. OMICS: A J. Integr. Biol. 16, 284–287. doi:10.1089/omi.2011.0118
Zhang, S., Chen, X., Hu, Y., Wu, J., Cao, Q., Chen, S., et al. (2016). All-trans Retinoic Acid Modulates Wnt3A-Induced Osteogenic Differentiation of Mesenchymal Stem Cells via Activating the PI3K/AKT/GSK3β Signalling Pathway. Mol. Cell Endocrinol. 422, 243–253. doi:10.1016/j.mce.2015.12.018
Zhang, W., Chen, E., Chen, M., Ye, C., Qi, Y., Ding, Q., et al. (2018). IGFBP7 Regulates the Osteogenic Differentiation of Bone Marrow‐derived Mesenchymal Stem cellsviaWnt/β‐catenin Signaling Pathway. FASEB j. 32, 2280–2291. doi:10.1096/fj.201700998rr
Zhong, G., Seaman, C. J., Paragas, E. M., Xi, H., Herpoldt, K.-L., King, N. P., et al. (2021). Aldehyde Oxidase Contributes to All-Trans-Retinoic Acid Biosynthesis in Human Liver. Drug Metab. Dispos 49, 202–211. doi:10.1124/dmd.120.000296
Keywords: bioinformatics, human bone marrow mesenchymal stem cells, osteogenic differentiation, novel, prediction
Citation: Sun L, Ma J, Chen J, Pan Z and Li L (2022) Bioinformatics-Guided Analysis Uncovers AOX1 as an Osteogenic Differentiation-Relevant Gene of Human Mesenchymal Stem Cells. Front. Mol. Biosci. 9:800288. doi: 10.3389/fmolb.2022.800288
Received: 22 October 2021; Accepted: 04 January 2022;
Published: 23 February 2022.
Edited by:
Ce Dou, Army Medical University, ChinaCopyright © 2022 Sun, Ma, Chen, Pan and Li. This is an open-access article distributed under the terms of the Creative Commons Attribution License (CC BY). The use, distribution or reproduction in other forums is permitted, provided the original author(s) and the copyright owner(s) are credited and that the original publication in this journal is cited, in accordance with accepted academic practice. No use, distribution or reproduction is permitted which does not comply with these terms.
*Correspondence: Zhijun Pan, enJwempAemp1LmVkdS5jbg==; Lijun Li, bGlsaWp1bkB6anUuZWR1LmNu
†These authors have contributed equally to this work and share first authorship