- 1Department of Sport Science, University of Innsbruck, Innsbruck, Austria
- 2Human Performance Laboratory, Faculty of Kinesiology, University of Calgary, Calgary, AL, Canada
Optimal feedback control theory suggests that control of movement is focused on movement dimensions that are important for the task's success. The current study tested the hypotheses that age effects would emerge in the control of only specific movement components and that these components would be linked to the task relevance. Fifty healthy volunteers, 25 young and 25 older adults, performed a 80s-tandem stance while their postural movements were recorded using a standard motion capture system. The postural movements were decomposed by a principal component analysis into one-dimensional movement components, PMk, whose control was assessed through two variables, Nk and σk, which characterized the tightness and the regularity of the neuro-muscular control, respectively. The older volunteers showed less tight and more irregular control in PM2 (N2: −9.2%, p = 0.007; σ2: +14.3.0%, p = 0.017) but tighter control in PM8 and PM9 (N8: +4.7%, p = 0.020; N9: +2.5%, p = 0.043; σ9: −8.8%, p = 0.025). These results suggest that aging effects alter the postural control system not as a whole, but emerge in specific, task relevant components. The findings of the current study thus support the hypothesis that the minimal intervention principle, as described in the context of optimal feedback control (OFC), may be relevant when assessing aging effects on postural control.
Introduction
In the USA around 20% of people aged 65 and over are diagnosed with only fair or poor health and long-term care services alone require between 210 and 317 billion USD annually (Rothwell, 2016). Healthy aging and with it the improvement of the quality of life of the elderly population is a major challenge for society. Key aspects of health and the ability to accomplish daily tasks are balance and stability (Alexander, 1994) which have been focus of extensive research. Especially healthy “golden agers”—senior individuals in the age range 55–70—are of interest since, on the one hand, deterioration in balance function accelerates after reaching the approximate age of 60 (Era et al., 2006) and, on the other hand, this age group is usually still active and thus a suitable target for preventive programs. In this context gender effects or interactions between gender and aging on postural control are also interesting, because literature is somewhat incoherent on this issue: there is evidence that the two genders are unequally influenced by aging (Evans and Hurley, 1995) and postural control may be affected differently (Wolfson et al., 1994). Other authors found that some of the gender effects on balance performance disappear when normalizing, for example, to body height (Bryant et al., 2005).
Balancing abilities and the progress of fall prevention programs are assessed with variables that quantify postural control through measures of body sway, for instance the total amount of sway, sway velocity, sway range, amongst others. These variables typically reveal age effects when comparing young healthy to frail old subjects, particularly if the sample size is very large (Era et al., 2006), if task difficulty is increased or instabilities are artificially created (eyes-closed, unstable surfaces, moving surfaces, waist-pulls, etc.; Rogers and Mille, 2003; Cavalheiro et al., 2009; Tardieu et al., 2009), or in dual-task situations with an additional cognitive task (Bernard-Demanze et al., 2009; Manor et al., 2010). Nevertheless, body sway variables are often unable to detect age effects when performing tasks that involve postural control and when comparing healthy young to older, but healthy, non-frail participants (Bernard-Demanze et al., 2009).
The body sway variables are usually derived from center of pressure (COP) trajectories which integrate all of the postural movements of the whole body into one 2-dimensional variable. Hence, to some degree, studies that assess such sway variables and interpret the results on a physiological basis interpret the control system as a whole. Implicitly it is assumed that physiological changes and adaptations that accompany aging influence the postural movements as a whole. This assumption, however, is not obvious when taking recent theories of motor control into consideration.
Currently one of the most successful models for motor control is the optimal feedback control (OFC) theory (Todorov and Jordan, 2002). This theory predicts that when performing a task, the biomechanical degrees of freedom are controlled depending on their relevance to the task. The more relevant a movement dimension is to the task, the tighter it is controlled. Movement control in this context is usually assessed by quantifying movement variability, with low variability indicating tight and high variability suggesting less tight control. This prediction of the OFC is referred to as “minimal intervention principle” (Todorov and Jordan, 2002) and corresponds to earlier concepts in motor control theory such as the uncontrolled manifold hypothesis (Scholz and Schöner, 1999; Latash et al., 2001; Friedman et al., 2009; Arpinar-Avsar et al., 2013; Park and Xu, 2017). However, if different movement dimensions are controlled differently, then one could hypothesize that aging effects may emerge differently in different dimensions or that only specific dimensions, for instance only the most task-relevant ones, reveal aging effects.
The term “movement dimension” relates to the mechanical degrees of freedom within the moving system (Todorov and Jordan, 2002). However, when analyzing whole-body postural control movements, it seems more appropriate to investigate synergistic, functional movement components such as the ankle or hip strategies (Nashner and McCollum, 1985; Woollacott et al., 1986; Winter, 1995; Ting and Macpherson, 2005). Principal component analysis (PCA) has been applied in previous research studies as a method for determining synergistic postural movement components (Troje, 2002; Daffertshofer et al., 2004; Verrel et al., 2009; Federolf et al., 2012a,b, 2013; Federolf, 2016). The movement components resulting from a PCA (called principal movements PMs) are one-dimensional whole body movement components that together form the original movement. Applying a PCA allows to assess the coordinative structure on the one hand and the control of individual postural movements on the other. The coordinative structure is represented by the amount of activity of the individual PMs. Differences in the relative contribution of the PMs show that the interplay of PMs and hence the coordinative structure of this movement is different (Zago et al., 2017). The control of individual postural movements can by analyzed using the PM-accelerations. The PM-accelerations are a direct result of the control system, since accelerations are directly proportional to the acting forces, which, in the case of postural control, are directly produced or allowed for by the neuromuscular control system (Federolf, 2016).
The purpose of the current study was to investigate age effects in the postural movements observed in healthy volunteers standing in a tandem stance. Specifically, the hypotheses were tested that (i) age effects would manifest in the control of only specific movement components; that (ii) the characteristics of how age groups control specific movement components would differ between different movement components; and we speculated that (iii) the emergence of aging effects or of how the age groups control specific movement components would be linked to the how task-relevant the movement components are. Furthermore, we tested for gender effects and age-by-gender interactions.
Methods
Subjects
The current paper analyzes data from a previous, unpublished study in which 106 subjects had volunteered for several balance and walking measurements. The study was conducted in agreement with the Declaration of Helsinki, particularly, an institutionalized ethics review board had approved the study design and informed written consent was obtained from all volunteers prior to any measurements. The inclusion criteria of the original study were (i) the age of the volunteer ranged either between 20 and 35 (young group) or 55 and 70 (older group); (ii) subjects were in good health with neither cardiovascular nor neurophysiological issues (this criterion was self-reported); (iii) subjects had an occupation that required them to be on their feet (standing, walking) roughly 50% of their time; (iv) we accepted volunteers who conducted occasional recreational sports activities, but no athletes who regularly trained for a specific sport. For the current study, a sub-set of 25 young and 25 older subjects were selected based on the following additional criterion: (v) the volunteers completed one of two trials of the tandem stance without visual instabilities, specifically, without taking a step, removing the hands from the hip, or extensive balance movements. Fulfillment of this criterion was evaluated during post-processing based on the datasets. Three investigators performed the evaluation for criterion (v) independently and the inclusion decision was based on the majority vote. This additional criterion was introduced since the goal of the current study was to compare postural control between age groups unaffected by occurrences of instability events. Of the two resulting test groups, the young group contained 17 women and 8 men aged 25 ± 3 years, height 1.69 ± 0.09 m, and weight 73 ± 18 kg (mean ± standard deviation). The older group also contained 17 women and 8 men, aged 59 ± 4 years, height 1.64 ± 0.09 m and weight 72 ± 12 kg.
Measurement Procedures
The volunteers performed 80-second tandem stances with their feet placed in one line such that the toe of the rear foot barely touched the heel of the front foot. The tandem stance is a task of medium difficulty level that is often used in balance assessments and can be easily performed by both, the older and the younger participants. The tandem stance position is well-suited to test the minimal intervention principle. The participants could test both positions and then choose whether they preferred the right or the left foot in front. The subjects were further instructed to keep their hands on the hip and to “stand as steady as possible.”
The volunteers were equipped with 37 reflective markers (standard plug-in gait marker set without markers on the hands; see Figure 1), whose positions in space were recorded at 240 frames per second by 8 synchronized video cameras (Motion Analysis Corp., Santa Rosa, CA, USA). The 3D marker trajectories were reconstructed using the software EvaReal-Time (“EvaRT”; MotionAnalysisCorporation, Santa Rosa, CA, USA). Gaps in marker trajectories were filled using a PCA-based reconstruction technique (Gløersen and Federolf, 2016).
Data Analysis—Principal Component Analysis (PCA)
The data analysis procedures were implemented in Matlab® (The MathWorks Inc., Natick, MA, USA). Non-symmetric mid-segment markers were omitted for the analysis. If subjects stood with the left foot in front, then their data were mirrored and relabeled such that all datasets for the analysis described tandem stances with the right foot in front. To address anthropometric differences, the dataset of each subject was centered by subtracting the mean posture and normalized by dividing by the subject's height. Then each marker was weighed with the relative segment mass it represents (Federolf, 2016; Gløersen et al., 2018). Finally, the normalized data of all subjects were concatenated into one input matrix for the PCA.
The underlying idea for the PCA conducted in the current study was that all marker coordinates at a given time are interpreted as a posture vector, i.e., as a position in posture space (Troje, 2002; Daffertshofer et al., 2004). By performing the PCA on the observed posture vectors variables were obtained that quantify postural variability (Troje, 2002; Daffertshofer et al., 2004; Verrel et al., 2009; Bockemühl et al., 2010). Specifically, a PCA yields eigenvalues EVk, eigenvectors PCk, and scores, where k denotes the order of the eigenvector. With the normalization used in the current study, the eigenvectors form the basis of a coordinate system in which postural movements can be compared between subjects. For an intuitive interpretation of the PCk it has been suggested to regard the postural movements they resemble as principal movements PMk. (Federolf et al., 2012a).
The scores, which are obtained by projecting the individual normalized posture vectors onto each PCk, can be interpreted as the positions in posture space, i.e., as principal positions PPk(t). The PPk(t) are therefore time series that describe the temporal evolution of a specific PMk.
The eigenvalues EVk quantify the contribution of the associated PMk to the overall variance. The EVk were therefore not subject specific in the current study. It has been proposed to calculate relative variances rVARk of the scores as subject-specific variables that directly correspond to the EVk and quantify how much each PMk contributed to this subject's overall postural variance (Federolf et al., 2013). However, as both rVARs and EVs quantify the variance in the data, they are proportional to the square of the postural movement amplitude. Therefore, in the current study the square roots of the subject specific variances were calculated to compute relative standard deviations rSTDk, to obtain variables that scale directly to postural movement amplitudes. For each subject, the rSTDk quantify the percentage of the subject-specific overall postural motion that is explained by each PMk. If systematic differences exist in the rSTDs between groups, then this indicates a difference in the coordinative structure of the postural movements in the sense that specific PMs are more or less important for the subjects' overall postural sway.
Kinematics in Posture Space and Variables Characterizing the Control of Postural Movements
Score-time series PPk(t) quantify a subject's position in posture space, which is spanned by the orthonormal basis {PCk}. Hence, their first derivatives quantify the principal velocities PVk(t) := d/dt PPk(t), and their second derivatives the principal accelerations PAk(t) := d2/dt2 PPk(t) of the postural movements PMk (Federolf, 2016).
In static balance exercises only two types of forces produce accelerations that cause postural changes: gravity—which is constant—and muscle action. Therefore the PAk(t) can be regarded as time-series that quantify the neuro-muscular system's control of the PMs (Federolf, 2016). In the current study two new variables were used to characterize the control of PMs. First, the number of zero-crossings Nk of the PAk(t) time-series were calculated. The underlying rational was that PAk(t) cross zero whenever the direction of the acceleration changes, i.e., when the postural control system counteracts the current postural acceleration. The Nk thus serve as a measure for how tightly the neuro-muscular system controls the motion of a postural movement component. Second, the standard deviations of the time between zero crossings σk were calculated in each subject's PAk-time series. The σk might be interpreted as a measure of the regularity or irregularity of the neuromuscular interventions in the control of a PM. A schematic illustration of the data analysis steps is shown in Figure 2.
Validity Considerations and Cross-Validation
A Fourier analysis was conducted on the PPk(t) time series, which revealed that the highest power resided in frequencies around 2–3 Hz, but visible power was still found in the frequency range between 4 to 7 Hz. The PPk(t) were therefore filtered with a 5th-order Butterworth lowpass filter using a cut-off frequency of 7 Hz. To calculate the PAk(t), a lowpass FIR-differentiator of order 50 was used with a passing frequency of 5 Hz and a stopping frequency of 7 Hz to avoid phase-shifts across frequency bands. Despite the filtering, Nk and σk are both highly susceptible to noise, as they are both calculated from PAk(t), a variable obtained through a double differentiation. Therefore, a detailed discussion on the effects of filtering on the magnitude of these variables and on the statistics is included in the Supplementary Materials.
A leave-one-out cross-validation was conducted to evaluate the vulnerability of the PMs and the dependent variables to changes in the input data. The first nine PMs were found to be robust to changes in the input, i.e., the PC-vector did not change its orientation in posture space by more than 15° in the cross-validation analysis. The statistical analysis was therefore limited to the dependent variables derived from these 9 PMs.
Statistics
All statistics were computed with IBM SPSS Version 24. Kolmogorov-Smirnov and Shapiro–Wilk tests were conducted to test for normality and Levene's tests were applied to assess equality of variances. To test for age and gender effects in the rSTDk, Nk, and σk, two-way ANOVAs and post-hoc comparisons with Sidak-correction were computed. The significance level was set to α = 0.05. For each statistically significant result we report the relative change of the mean value (younger or male group = 100%), p-value, partial eta squared ηp2 and the observed power π. An overview of the results for all variables, groups, and PMs (means ± standard deviation) is included as Supplementary Materials.
Results
The first nine PMs explained 98.2% of the overall postural variance. The aspects of postural control that each PM resembled are listed in Table 1 and visualized in Figure 3 and the videos submitted as Supplementary Materials.
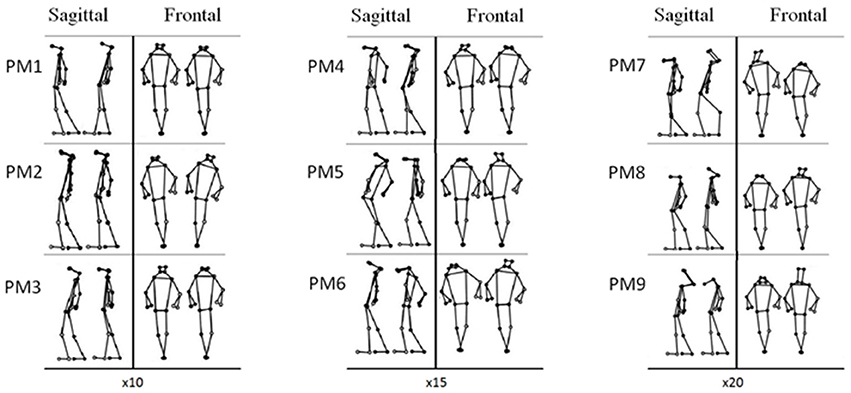
Figure 3. Each graph shows the two opposing extreme positions of the PMs of the first subject of the younger age group in the frontal and sagittal planes. The postural changes were amplified by a factor 10, 15, 20, in the first, second and third column, respectively.
In PM2, which predominantly represented medio-lateral ankle sway, significant age effects were found in both the coordinative structure {rSTD2: +13.1% [old vs. young], F(1, 46) = 6.28, p < 0.016, ηp2 = 0.12, π = 0.69} and the control variables [N2: −9.2%, F(1, 46) = 8.05, p = 0.007, ηp2 = 0.15, π = 0.79; and σ2: +13.6%, F(1, 46) = 7.4, p = 0.009, ηp2 = 0.14, π = 0.76]. The control variables showed no age-by-gender interaction, however, rSTD2 showed a significant interaction effect [rSTD2: F(1, 46) = 6.45, p = 0.015, ηp2 = 0.12, π = 0.70]. The post-hoc analysis revealed that the increase with age in rSTD2 originated only from the male group, which showed a 46% increase with age (p = 0.004, ηp2 = 0.17, π = 0.85), while the female group showed no changes (−0.3%, n.s.).
In PM3, which coupled an upper body retraction with a flexion of the front knee, no main effects were found. The age-by-gender interaction was significant in rSTD3 [F(1, 46) = 5.38, p = 0.025, ηp2 = 0.10, π = 0.62] but the post-hoc comparisons between sub-groups were not significant.
Women exhibited a different coordinative structure as they displayed more relative sway in PM4 [rSTD4: +23.9%, F(1, 46) = 4.43, p = 0.041, ηp2 = 0.09, π = 0.54] and less in PM6 [rSTD6: −17.8%, F(1, 46) = 4.31, p = 0.043, ηp2 = 0.09, π = 0.53] than men. The variable quantifying the amount of control intervention suggested that women controlled PM4 less tightly than men [N4: −5.7%, F(1, 46) = 4.39, p = 0.042, ηp2 = 0.09, π = 0.54] but exerted similar control in PM6.
The variables that quantify the control of PM8 and PM9 displayed three age effects [N8: +4.7%, F(1, 46) = 5.82, p = 0.020, ηp2 = 0.11, π = 0.66; N9: +2.5%, F(1, 46) = 4.34, p = 0.043, ηp2 = 0.09, π = 0.53 and σ9: −8.8%, F(1, 46) = 5.37, p = 0.025, ηp2 = 0.10, π = 0.62]. The relative sway did not show age effects in these PMs.
Discussion
Main Result
The current study investigated age related differences in postural control between young adults and healthy, active golden agers. Based on considerations deducted from the OFC theory (Todorov and Jordan, 2002), it was predicted that (i) age effects would manifest in the control of only specific movement components; that (ii) the characteristics of the control of these movement components may differ between different movement components; and we speculated that (iii) the emergence of aging effects would be linked to the task-relevance of the movement components.
The first two hypotheses were confirmed in the present study: age main effects were observed specifically in the control characteristics of PM2, PM8, and PM9, but not in other movement components. How age affected the control of these movement components differed between PM2 compared to PM8 and PM9: In PM2 the younger group exerted a tighter (N2 young > N2 old) and more regular (σ2 young < σ2 old) movement control while in PM8 and PM9 the older group exerted a tighter and more regular (only PM9) control. This is a remarkable result since the various sensorimotor and physiological changes that accompany aging are usually considered to affect the whole system (Bernard-Demanze et al., 2009; Jiménez-Jiménez et al., 2011; Papegaaij et al., 2014). Thus, a priori, it seems counterintuitive that aging effects in postural control would emerge only in specific postural movement components and that different components may even exhibit contrasting control characteristics.
The third hypothesis was supported by the findings in PM1 and PM2. While PM1 contributed more to the overall postural variations than PM2 (EV1 > EV2), PM1 represented an anteroposterior sway and PM2 a lateral sway. In the tandem stance it seems obvious that PM2 is a critical movement to control for maintaining postural stability in both age groups, while the different PM1 states are task-redundant. The age effect observed in PM2 suggests that the older age groups were not able to control this critical movement component as well as the younger groups. This seems plausible from a physiological point of view: aging is generally associated with deleterious changes of the sensorimotor system (Papegaaij et al., 2014). These changes arise at different levels of the sensorimotor pathway. The muscle fibers' structures degenerate (Hepple, 2003) and motor-units get enlarged (Campbell et al., 1973). Their activation is driven by the nervous system that undergoes significant changes associated with aging as well. Overall, both the motor and the proprioceptive sensory neurons deteriorate (Papegaaij et al., 2014; Vaughan et al., 2017), modifying cortical and subcortical structures, thus leading to a reorganization of the control mechanisms (Papegaaij et al., 2014). It is very likely that the slowing down and reorganization of the information conduction and processing contribute to the findings in PM2 of the older age group showing less frequent (N2) and more variable (σ2) changes in postural accelerations compared to the young volunteers.
A notable finding in the PM2 motion was that the increase of rSTD2 due to aging originated from the male population only, while the movement control variables N2 and σ2 changed equally in the two genders. An increase in rSTD2 could be a sign that the older men were not able to control the overall PM2 sway amplitude. There are indications in the literature that in compromised postural control, e.g. after a concussion, sway amplitudes regenerate faster, while differences in how postural movements are controlled are detectable for a longer period (Cavanaugh et al., 2006). The current study's finding might indicate the inverse process: aging seemed to have led to changes in the control characteristics, however, it might be speculated that the female participants were still able to control the sway amplitudes similarly to their younger counterparts, while in the male group an effect on the sway amplitude was already detectable. In general, the overall body sway increases as a function of height (Gage et al., 2004). Nevertheless, we expect that height differences can be excluded, since the data was normalized to height. Since men show more relative sway in the dimension that is harder to control, it is possible that woman suffer less from aging impairments than men. It has been shown that strength levels in woman decline at slower rates (Evans and Hurley, 1995). Speculatively, it might be harder for men to adapt to the loss of strength, which could also affect balance capabilities.
The age effects found in N8, N9, and σ9 suggested tighter and more regular control of the PM8 and PM9 components in the older group compared to the younger group. The reason why age effects were only evident in the control but not the relative sway variables of these components might be due to the fact that both components contributed <1% to the overall variance. Variability within the subjects might be of the same magnitude as the contribution itself, causing differences to remain undetected. Nevertheless, if interpreted within the paradigm of the OFC, then this finding suggests that for the older group the control of these two movement components is more task-relevant than for the younger group. PM8 and PM9 are both characterized by large head movements. Several previous studies found that the postural control in older volunteers relied more on visual information compared to younger subjects (Woollacott et al., 1986; Prioli et al., 2005). Since head movements could affect the visual cuing it seems plausible that older volunteers need to control head movements more tightly. In fact, aging effects in the head-trunk coordination have already been reported in standing (Paquette et al., 2006) and in walking (Kavanagh et al., 2005; Paquette et al., 2006).
Gender Effects
Hip flexion and upper body rotations (PM4) contributed more to the overall postural movements and seemed less tightly controlled in the women groups compared to the men groups, while upper body medio-lateral sway and hip ab-adduction movements (PM6) contributed less. These effects must be interpreted with caution since the effect in N4 is only significant for the chosen filter (see “FilteringSelection_Statistics.docx,” section 2. Gender comparisons). Nevertheless, differences were observed despite normalizing the current data to body height, contrary to previous research suggesting that gender differences in total sway disappear when normalizing to body height (Bryant et al., 2005). The anatomical differences or differences in relative strength (Claiborne et al., 2006) between men and women are the most likely explanations for these differences in the movement structure. Another possibility is that men and woman relied on slightly different hip strategies while performing the tandem stance.
Methodological Aspects of the Current Paper
The current study built on the concepts from the OFC theory (Todorov and Jordan, 2002) and the uncontrolled manifold hypothesis (Scholz and Schöner, 1999; Latash et al., 2001; Friedman et al., 2009; Arpinar-Avsar et al., 2013; Park and Xu, 2017), however, contrary to these concepts, the approach taken in the current study is fully data-driven and does not require the a priori postulation of a cost function or a task variable. Further, the current study is also based on the concept of kinematics in posture space, a novel concept that has recently been validated biomechanically by demonstrating that the PPk(t) and PAk(t) predict the center of pressure COP trajectory (Federolf, 2016). The two new variables Nk and σk introduced in the current study offer an alternative approach to characterizing how tightly and how regularly a movement component is controlled by the neuro-muscular system, while most previous studies characterized these aspects of motor control through an analysis of the observed variability in repeated tasks (Friedman et al., 2009; Verrel et al., 2009; Bockemühl et al., 2010; Kobayashi et al., 2014) or use more complex, non-linear variables, such as entropy (Cavanaugh et al., 2006; Manor et al., 2010; Zhou et al., 2015), to characterize regularity.
Limitations
Although inclusion criterion (v) was assessed by three investigators independently, this criterion for subject selection remains subjective. Furthermore, the number of male volunteers was considerably smaller than the number of female participants, leading to unevenly distributed gender groups.
PCA is a linear decomposition method. The PMs should be seen as a coordinate system for postural movements. Interpreting individual PMs independently from another should be done with caution, since any original postural movement is always a combination of all PMs.
The Nk and σk were computed on acceleration data obtained from dual differentiation of kinematic data. Therefore, the filtering of the data influences the nominal values for Nk and σk. This limitation was addressed by repeating the whole analysis with a wide range of filter settings. The results of this additional analysis are attached as Supplementary Data File “FilteringSelection _Statistics.docx.”
Conclusions and Implications
The current study supports the hypothesis that aging effects in postural control emerge in specific movement components, not in all postural movements. This finding should be considered when designing balance training programs for fall prevention or tests for monitoring the progress of participants in such programs.
Author Contributions
TH processed the data and wrote the paper; A-CD provided valuable insights for the discussion and reviewed the paper; BN was the leader of the data collection and approved the manuscript; PF was involved in the data collection. He supervised the data processing and assisted the writing process.
Conflict of Interest Statement
The authors declare that the research was conducted in the absence of any commercial or financial relationships that could be construed as a potential conflict of interest.
The reviewer BS declared a past co-authorship with the author BN to the handling Editor.
Supplementary Material
The Supplementary Material for this article can be found online at: https://www.frontiersin.org/articles/10.3389/fnagi.2018.00022/full#supplementary-material
Video S1. Visualization of PM1–PM3.
Video S2. Visualization of PM4–PM6.
Video S3. Visualization of PM7–PM9.
Video S4. Exemplary realization of PM1–PM3 of the first subject of the younger age group.
Video S5. Exemplary realization of PM4–PM6 of the first subject of the younger age group.
Video S6. Exemplary realization of PM7–PM9 of the first subject of the younger age group.
References
Alexander, N. B. (1994). Postural control in older adults. J. Am. Geriatr. Soc. 42, 93–108. doi: 10.1111/j.1532-5415.1994.tb06081.x
Arpinar-Avsar, P., Park, J., Zatsiorsky, V. M., and Latash, M. L. (2013). Effects of muscle vibration on multi-finger interaction and coordination. Exp. Brain Res. Exp. Hirnforsch. Exp. Cerebrale 229, 103–111. doi: 10.1007/s00221-013-3597-y
Bernard-Demanze, L., Dumitrescu, M., Jimeno, P., Borel, L., and Lacour, M. (2009). Age-related changes in posture control are differentially affected by postural and cognitive task complexity. Curr. Aging Sci. 2, 139–149. doi: 10.2174/1874609810902020135
Bockemühl, T., Troje, N. F., and Dürr, V. (2010). Inter-joint coupling and joint angle synergies of human catching movements. Hum. Mov. Sci. 29, 73–93. doi: 10.1016/j.humov.2009.03.003
Bryant, E. C., Trew, M. E., Bruce, A. M., Kuisma, R. M. E., and Smith, A. W. (2005). Gender differences in balance performance at the time of retirement. Clin. Biomech. 20, 330–335. doi: 10.1016/j.clinbiomech.2004.11.006
Campbell, M. J., McComas, A. J., and Petito, F. (1973). Physiological changes in ageing muscles. J. Neurol. Neurosurg. Psychiatr. 36, 174–182. doi: 10.1136/jnnp.36.2.174
Cavalheiro, G. L., Almeida, M. F. S., Pereira, A. A., and Andrade, A. O. (2009). Study of age-related changes in postural control during quiet standing through linear discriminant analysis. Biomed. Eng. 8:35. doi: 10.1186/1475-925X-8-35
Cavanaugh, J. T., Guskiewicz, K. M., Giuliani, C., Marshall, S., Mercer, V. S., and Stergiou, N. (2006). Recovery of postural control after cerebral concussion: new insights using approximate entropy. J. Athl. Train. 41, 305–313.
Claiborne, T. L., Armstrong, C. W., Gandhi, V., and Pincivero, D. M. (2006). Relationship between hip and knee strength and knee valgus during a single leg squat. J. Appl. Biomech. 22, 41–50. doi: 10.1123/jab.22.1.41
Daffertshofer, A., Lamoth, C. J. C., Meijer, O. G., and Beek, P. J. (2004). PCA in studying coordination and variability: a tutorial. Clin. Biomech. 19, 415–428. doi: 10.1016/j.clinbiomech.2004.01.005
Era, P., Sainio, P., Koskinen, S., Haavisto, P., Vaara, M., and Aromaa, A. (2006). Postural balance in a random sample of 7, 979 subjects aged 30 years and over. Gerontology 52, 204–213. doi: 10.1159/000093652
Evans, W. J., and Hurley, B. F. (1995). Age, gender, and muscular strength. J. Gerontol. A Biol. Sci. Med. Sci. 50A, 41–44. doi: 10.1093/gerona/50A.Special_Issue.41
Federolf, P. A. (2016). A novel approach to study human posture control: “Principal movements” obtained from a principal component analysis of kinematic marker data. J. Biomech. 49, 364–370. doi: 10.1016/j.jbiomech.2015.12.030
Federolf, P., Roos, L., and Nigg, B. (2012a). The effect of footwear on postural control in bipedal quiet stance. Footwear Sci. 4, 115–122. doi: 10.1080/19424280.2012.666270
Federolf, P., Roos, L., and Nigg, B. M. (2013). Analysis of the multi-segmental postural movement strategies utilized in bipedal, tandem and one-leg stance as quantified by a principal component decomposition of marker coordinates. J. Biomech. 46, 2626–2633. doi: 10.1016/j.jbiomech.2013.08.008
Federolf, P., Tecante, K., and Nigg, B. (2012b). A holistic approach to study the temporal variability in gait. J. Biomech. 45, 1127–1132. doi: 10.1016/j.jbiomech.2012.02.008
Friedman, J., Skm, V., Zatsiorsky, V. M., and Latash, M. L. (2009). The sources of two components of variance: an example of multifinger cyclic force production tasks at different frequencies. Exp. Brain Res. Exp. Hirnforsch. Exp. Cerebrale 196, 263–277. doi: 10.1007/s00221-009-1846-x
Gage, W. H., Winter, D. A., Frank, J. S., and Adkin, A. L. (2004). Kinematic and kinetic validity of the inverted pendulum model in quiet standing. Gait Posture 19, 124–132. doi: 10.1016/S0966-6362(03)00037-7
Gløersen, Ø., and Federolf, P. (2016). Predicting missing marker trajectories in human motion data using marker intercorrelations. PLoS ONE 11:e0152616. doi: 10.1371/journal.pone.0152616
Gløersen, Ø., Myklebust, H., Hallén, J., and Federolf, P. (2018). Technique analysis in elite athletes using principal component analysis. J. Sports Sci. 36, 229–237. doi: 10.1080/02640414.2017.1298826
Hepple, R. T. (2003). Sarcopenia–a critical perspective. Sci. Aging Knowl. Environ. 2003:pe31. doi: 10.1126/sageke.2003.46.pe31
Jiménez-Jiménez, F. J., Calleja, M., Alonso-Navarro, H., Rubio, L., Navacerrada, F., Pilo-de-la-Fuente, B., et al. (2011). Influence of age and gender in motor performance in healthy subjects. J. Neurol. Sci. 302, 72–80. doi: 10.1016/j.jns.2010.11.021
Kavanagh, J. J., Barrett, R. S., and Morrison, S. (2005). Age-related differences in head and trunk coordination during walking. Hum. Mov. Sci. 24, 574–587. doi: 10.1016/j.humov.2005.07.003
Kobayashi, H., Kakihana, W., and Kimura, T. (2014). Combined effects of age and gender on gait symmetry and regularity assessed by autocorrelation of trunk acceleration. J. Neuroeng. Rehabil. 11:109. doi: 10.1186/1743-0003-11-109
Latash, M. L., Scholz, J. F., Danion, F., and Schöner, G. (2001). Structure of motor variability in marginally redundant multifinger force production tasks. Exp. Brain Res. 141, 153–165. doi: 10.1007/s002210100861
Manor, B., Costa, M. D., Hu, K., Newton, E., Starobinets, O., Kang, H. G., et al. (2010). Physiological complexity and system adaptability: evidence from postural control dynamics of older adults. J. Appl. Physiol. 109, 1786–1791. doi: 10.1152/japplphysiol.00390.2010
Nashner, L. M., and McCollum, G. (1985). The organization of human postural movements: a formal basis and experimental synthesis. Behav. Brain Sci. 8, 135–150. doi: 10.1017/S0140525X00020008
Papegaaij, S., Taube, W., Baudry, S., Otten, E., and Hortobágyi, T. (2014). Aging causes a reorganization of cortical and spinal control of posture. Front. Aging Neurosci. 6:28. doi: 10.3389/fnagi.2014.00028
Paquette, C., Paquet, N., and Fung, J. (2006). Aging affects coordination of rapid head motions with trunk and pelvis movements during standing and walking. Gait Posture 24, 62–69. doi: 10.1016/j.gaitpost.2005.07.001
Park, J., and Xu, D. (2017). Multi-finger interaction and synergies in finger flexion and extension force production. Front. Hum. Neurosci. 11:318. doi: 10.3389/fnhum.2017.00318
Prioli, A. C., Freitas, P. B. Jr., and Barela, J. A. (2005). Physical activity and postural control in the elderly: coupling between visual information and body sway. Gerontology 51, 145–148. doi: 10.1159/000083984
Rogers, M. W., and Mille, M. L. (2003). Lateral stability and falls in older people. Exerc. Sport Sci. Rev. 31, 182–187. doi: 10.1097/00003677-200310000-00005
Rothwell, C. (2016). Health, United States, 2015: With Special Feature on Racial and Ethnic Health Disparities. National Center for Health Statistics. Available online at: https://www.cdc.gov/nchs/data/hus/hus15.pdf#045
Scholz, J. P., and Schöner, G. (1999). The uncontrolled manifold concept: identifying control variables for a functional task. Exp. Brain Res. 126, 289–306. doi: 10.1007/s002210050738
Tardieu, C., Dumitrescu, M., Giraudeau, A., Blanc, J. L., Cheynet, F., and Borel, L. (2009). Dental occlusion and postural control in adults. Neurosci. Lett. 450, 221–224. doi: 10.1016/j.neulet.2008.12.005
Ting, L. H., and Macpherson, J. M. (2005). A limited set of muscle synergies for force control during a postural task. J. Neurophysiol. 93, 609–613. doi: 10.1152/jn.00681.2004
Todorov, E., and Jordan, M. I. (2002). Optimal feedback control as a theory of motor coordination. Nat. Neurosci. 5, 1226–1235. doi: 10.1038/nn963
Troje, N. F. (2002). Decomposing biological motion: a framework for analysis and synthesis of human gait patterns. J. Vis. 2, 371–387. doi: 10.1167/2.5.2
Vaughan, S. K., Stanley, O. L., and Valdez, G. (2017). Impact of aging on proprioceptive sensory neurons and intrafusal muscle fibers in mice. J. Gerontol. A Biol. Sci. Med. Sci. 72, 771–779. doi: 10.1093/gerona/glw175
Verrel, J., Lövdén, M., Schellenbach, M., Schaefer, S., and Lindenberger, U. (2009). Interacting effects of cognitive load and adult age on the regularity of whole-body motion during treadmill walking. Psychol. Aging 24, 75–81. doi: 10.1037/a0014272
Winter, D. (1995). Human balance and posture control during standing and walking. Gait Posture 3, 193–214. doi: 10.1016/0966-6362(96)82849-9
Wolfson, L., Whipple, R., Derby, C. A., Amerman, P., and Nashner, L. (1994). Gender differences in the balance of healthy elderly as demonstrated by dynamic posturography. J. Gerontol. 49, M160–M167. doi: 10.1093/geronj/49.4.M160
Woollacott, M. H., Shumway-Cook, A., and Nashner, L. M. (1986). Aging and posture control: changes in sensory organization and muscular coordination. Int. J. Aging Hum. Dev. 23, 97–114. doi: 10.2190/VXN3-N3RT-54JB-X16X
Zago, M., Pacifici, I., Lovecchio, N., Galli, M., Federolf, P. A., and Sforza, C. (2017). Multi-segmental movement patterns reflect juggling complexity and skill level. Hum. Mov. Sci. 54, 144–153. doi: 10.1016/j.humov.2017.04.013
Keywords: healthy aging, postural control, principal component analysis on kinematic data, minimum intervention principle, optimal feedback control, balance control, tandem stance
Citation: Haid TH, Doix A-CM, Nigg BM and Federolf PA (2018) Age Effects in Postural Control Analyzed via a Principal Component Analysis of Kinematic Data and Interpreted in Relation to Predictions of the Optimal Feedback Control Theory. Front. Aging Neurosci. 10:22. doi: 10.3389/fnagi.2018.00022
Received: 11 September 2017; Accepted: 18 January 2018;
Published: 05 February 2018.
Edited by:
Filippo Tempia, Università degli Studi di Torino, ItalyReviewed by:
Francis Degache, University of Applied Sciences and Arts of Western Switzerland, SwitzerlandBernd Stetter, Karlsruhe Institute of Technology, Germany
Copyright © 2018 Haid, Doix, Nigg and Federolf. This is an open-access article distributed under the terms of the Creative Commons Attribution License (CC BY). The use, distribution or reproduction in other forums is permitted, provided the original author(s) and the copyright owner are credited and that the original publication in this journal is cited, in accordance with accepted academic practice. No use, distribution or reproduction is permitted which does not comply with these terms.
*Correspondence: Thomas H. Haid, dGhvbWFzLmhhaWRAdWliay5hYy5hdA==
†Co-authors.