- 1Department of Neurology, The Affiliated Brain Hospital of Nanjing Medical University, Nanjing, China
- 2Department of Radiology, The Affiliated Brain Hospital of Nanjing Medical University, Nanjing, China
- 3Institute of Brain Functional Imaging, Nanjing Medical University, Nanjing, China
- 4Institute of Neuropsychiatry, The Affiliated Brain Hospital of Nanjing Medical University, Nanjing, China
Background: Mild cognitive impairment (MCI) is regarded as a transitional stage between normal aging and Alzheimer's disease (AD) dementia. MCI individuals with deficits in executive function are at higher risk for progressing to AD dementia. Currently, there is no consistent result for alterations in the executive control network (ECN) in MCI, which makes early prediction of AD conversion difficult. The aim of the study was to find functional MRI-specific alterations in ECN in MCI patients by expounding on the convergence of brain regions with functional abnormalities in ECN.
Methods: We searched PubMed, Embase, and Web of Science to identify neuroimaging studies using methods including the amplitude of low frequency fluctuation/fractional amplitude of low-frequency fluctuation, regional homogeneity, and functional connectivity in MCI patients. Based on the Activation Likelihood Estimation algorithm, the coordinate-based meta-analysis and functional meta-analytic connectivity modeling were conducted.
Results: A total of 25 functional imaging studies with MCI patients were included in a quantitative meta-analysis. By summarizing the included articles, we obtained specific brain region changes, mainly including precuneus, cuneus, lingual gyrus, middle frontal gyrus, posterior cingulate cortex, and cerebellum posterior lobe, in the ECN based on these three methods. The specific abnormal brain regions indicated that there were interactions between the ECN and other networks.
Conclusions: This study confirms functional imaging specific abnormal markers in ECN and its interaction with other networks in MCI. It provides novel targets and pathways for individualized and precise interventions to delay the progression of MCI to AD.
Introduction
Mild cognitive impairment (MCI) is regarded as a transitional stage between normal aging and Alzheimer's disease (AD) dementia (Jia et al., 2015). Executive function is an important high-level cognitive process and a function of flexible integration and cooperative operation of different cognitive processes to achieve a specific goal (Trossman et al., 2020). The executive function of MCI patients is significantly impaired, which is mainly reflected in planning ability, continuous attention, and other aspects (Trossman et al., 2020). Hence, executive function impairment is associated with severe and lasting progression to AD in MCI individuals compared to other cognitive impairment. As is well-known, the executive control network (ECN) in MCI, which is a wide range of brain regions responsible for executive function shows different changes in brain regions in different studies (Joshi et al., 2019). There is no doubt that studying the specific abnormal biomarkers of executive function is a necessity due to a lack of consistent results.
Resting state functional MRI (rsfMRI) has superiority in detecting several neuropsychiatric disorders in which the changes in blood oxygen level signal intensity are considered an indirect tool associated with function in specific regions of the human brain (Wee et al., 2016). Current studies usually evaluate functional alterations in the ECN by the following methods in rsfMRI: (1) the amplitude of low-frequency fluctuation (ALFF)/fractional amplitude of low-frequency fluctuation (fALFF), (2) regional homogeneity (ReHo), and (3) functional connectivity (FC). The ALFF and fALFF are methods that measure the amplitude of spontaneous regional brain activity by calculating the square root of the power spectrum in the low-frequency range. The fALFF method has been confirmed to be more sensitive compared with ALFF (Yang et al., 2019). Numerous studies indicate that the specific patterns of ALFF and fALFF provide insights into the mechanism in AD and MCI patients (Yang et al., 2018). ReHo is widely used in the study of various mental disorders and has been demonstrated to have a high test–retest reliability in the study of the consistency of brain activity. A large amount of evidence shows a correlation between the ReHo value of abnormal brain areas and clinical symptoms (Luo et al., 2018). FC usually reflects the connectivity between brain regions to reveal whether there is connectivity disruption or compensation based on independent component analysis and seed point (Liu et al., 2020). All three methods have their own advantages in studying ECN in patients with MCI, but the results are unanimous.
ECN, mainly anchored in the bilateral dorsolateral prefrontal lobe, ventral prefrontal lobe, frontal insular cortex, and parietal lobe, has extensive connections with other regions (Wu et al., 2014). ECN plays an important role in the integration of sensory and memory information, the regulation of cognition and behavior, and the working memory process with the prefrontal lobe as its core (Petersen et al., 2019). A combined arterial spin labeling (ASL), perfusion, and rsfMRI study confirms that the brain regions in AD where cerebral blood flow and ALFF decrease predict a disruption in the ECN (Zheng et al., 2019). However, the lack of consistent results for specific alterations in ECN fail to provide specific imaging markers in predicting the transition from MCI to AD. Therefore, to explore the specific imaging markers in ECN is essential to determine if these early alterations will serve as sensitive predictors of clinical decline and, eventually, as markers of MCI progress to AD by summarizing previous studies.
Currently, the widely recognized resting state brain networks include the default mode network (DMN), ECN, salience network (SAL), dorsal attention network (DAN), fronto-parietal network (FPN), and sensory-motor network (SMN, a combination of three originally separate RSNs that correspond to the primary auditory, primary visual, and somatomotor cortices) (Chen et al., 2016). The components of each network are partially overlapped with other networks, forming dynamic interactions, which is considered to be of great significance in behavioral function (Brier et al., 2012). There has been evidence of possible interactions between the ECN and other networks (Yuan B. et al., 2016). Cognitive decline or disease would result from the destruction of certain aspects of this connectivity network (Leech and Sharp, 2014). As a result, it is necessary to investigate the mechanisms underlying the interaction between the known networks in MCI patients.
Anatomical/activation likelihood estimation (ALE) is an effective coordinate-based meta-analysis (CBMA), which avoids laboratory bias in quantifying consistent imaging findings across studies (Robinson et al., 2012). ALE has been used extensively in rsfMRI studies, and it is suggested that ALE may provide image-specific markers (Robinson et al., 2010). In total, each individual coordinate from each study that is presented as a 3-D Gaussian probability distribution. ALE maps were produced by combining the distributions from all the studies in which significance was determined by using the threshold of p < 0.05 (Doucet et al., 2020). This method was applied in the quantification of the location and extent of cerebellar changes across the main frontotemporal dementia and neuroimaging in Parkinson's disease dementia (Chen et al., 2019; Weil et al., 2019). Because it has been used extensively in degenerative diseases, it is reasonable to use it in MCI patients. To our knowledge, so far, it is rare that a meta-analysis is made on the functional specific alterations of the ECN in MCI patients. As a result, a quantitative meta-analysis of published neuroimaging studies using the ALE algorithm were conducted to provide an overview of the current literature on specific alterations of the ECN.
Hence, the aim of the present study was to evaluate the specific functional alterations in the ECN more comprehensively and discuss the functional imaging-specific abnormal markers in ECN and its interaction with other networks in MCI by using three techniques. It was hypothesized that, in MCI, (1) the three indexes of the ECN would show the special imaging abnormal markers, and (2) these specific brain regions would exist abnormal interactions with other networks.
Methods
Literature Search and Study Selection
The meta-analysis of neuroimaging studies was conducted according to the PRISMA statement and recorded using the suggested checklist.
Search Strategy
PubMed, Embase, and Web of science were thoroughly and systematically searched. Search keywords were as follows: (1) (“functional magnetic resonance imaging” [MeSH] OR “RESTING STATE” [MeSH]) AND [“mild cognitive impairment” [MeSH] AND “Executive Control” [MeSH] AND “Functional connectivity”]; (2) (“functional magnetic resonance imaging” [MeSH] OR “RESTING STATE” [MeSH]) AND (“mild cognitive impairment” [MeSH] AND “regional homogeneity”); and (3) (“functional magnetic resonance imaging” [MeSH] OR “RESTING STATE” [MeSH]) AND “mild cognitive impairment” [MeSH] AND (“fractional amplitude of low frequency fluctuation” OR “amplitude of low frequency fluctuation”) (Supplementary Table 1).
Only publications in English were selected. A total of 271 specific articles were screened. The flowchart of the literature search and selection strategy is shown in Figure 1.
Inclusion and Exclusion Criteria
All articles related to rsfMRI investigations on MCI were included in our study. To make comparisons between healthy controls (HCs) and MCI, information about Talairach or the Montreal Neurologic Institute (MNI) were required in these investigations.
If articles were based on other diseases, such as schizophrenia, depression, et al. they were eliminated. We dropped secondary processing of literature, such as reviews and meta-analysis articles. Case studies without group-level statistics were excluded. Articles whose necessary data could not be obtained even after contacting the author/s were excluded. Articles based on region of interest analysis were excluded.
Data Extraction and Quality Assessment
Two researchers conducted selection, data extraction, and cross-checks independently. A third reviewer participated in judgment when disagreements appeared of any kind. First, we selected articles related to ECN. Second, articles with respect to the abnormal functional network in the use of ALFF/fALFF and ReHo were included. All the abnormal brain regions extracted from the articles were based on brain regions in the ECN included in the articles.
Data Analysis Procedures
Comparing MCI with HCs, we separated the three different methods by decreasing and increasing and then calculated them on the software: increased ALFF/ fALFF (n = 251; 19 foci); decreased ALFF/fALFF (n = 414; 36 foci); increased ReHo (n = 222; 17 foci); decreased ReHo (n = 202; 18 foci); increased FC (n = 136; 19 foci); decreased FC (n = 76; 17 foci).
An ALE meta-analysis was conducted with a Java-based version of Ginger ALE 2.3.6 (http://www.brainmap.org/ale) (Eickhoff et al., 2012). The aim of ALE was to assess the convergence of the difference between MCI and HC groups in terms of foci across studies. First, we used a text file to read foci data that are imported into the software (Eickhoff et al., 2012). A threshold at p < 0.05 using the false discovery rate (FDR) was set in ALE maps (Eickhoff et al., 2012). Last, the maps were covered into the MNI 152 template and viewed with Dpabi software (http://fmri.org/dpabi).
Results
Search Results
The study characteristics and results are summarized in Table 1.
Meta-Analysis Results
Abnormal ALFF/fALFF in MCI
Compared with HC, MCI patients showed increased ALFF/fALFF in the right lingual gyrus (LING), right cerebellum posterior lobe (CPL), right cerebellum anterior lobe (CAL), right precuneus (PCUN), right inferior parietal lobule (IPL), and left inferior temporal gyrus (ITG) (Table 2, Figure 2). MCI patients showed decreased ALFF/fALFF in right PCUN, right posterior cingulate cortex (PCC), and right parahippocampal gyrus (PHG) (Table 2, Figure 2).
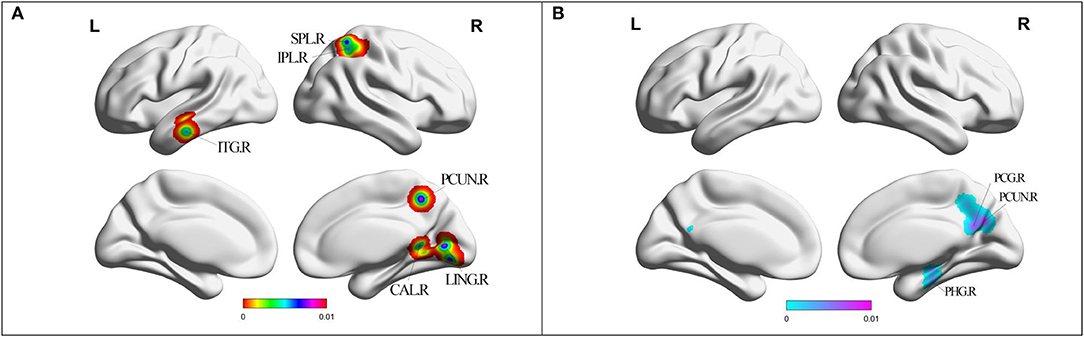
Figure 2. (A) Brain regions showing increased ALFF/fALFF in MCI patients compared with HCs. (B) Brain regions showing decreased ALFF/fALFF in MCI patients compared with HCs. MCI, amnestic mild cognitive impairment; HCs, healthy controls; ALFF/fALFF, the amplitude of low frequency fluctuation/fractional amplitude of low-frequency fluctuation; ITG, inferior temporal gyrus; CAL, cerebellum anterior lobe; LING, lingual gyrus; PCUN, precuneus; IPL, inferior parietal lobule; SPL, superior parietal lobule; PHG, parahippocampal gyrus; PCG, posterior cingulate; R, right; L, left.
Altered ReHo in MCI
Compared with HC, MCI patients showed increased ReHo in left PCUN, right precentral gyrus (PreCG), left cuneus, bilateral LING, left inferior frontal gyrus (IFG), right paracentral lobule (PCL), and right PreCG (Table 2, Figure 3). MCI patients showed increased ReHo in bilateral PCUN, left cuneus, right superior parietal lobule (SPL), and right middle temporal gyrus (MTG) (Table 2, Figure 3).
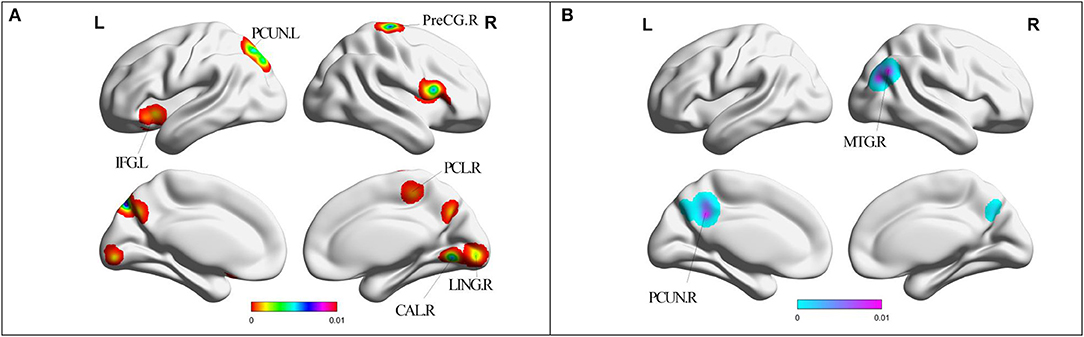
Figure 3. (A) Brain regions showing increased ReHo in MCI patients compared with HCs. (B) Brain regions showing decreased ReHo in MCI patients compared with HCs. MCI, amnestic mild cognitive impairment; ReHo, regional homogeneity; HCs, healthy controls; PHG, parahippocampal gyrus; PCG, posterior cingulate; PCUN, precuneus; MTG, middle temporal gyrus; LING, lingual gyrus; CAL, cerebellum anterior lobe; IFG, inferior frontal gyrus; PCL, paracentral lobule; PreCG, precentral gyrus; R, right; L, left. Cerebellum posterior lobe cannot be displayed in this template.
Altered FC in MCI
Compared with HC, MCI patients showed increased FC in right PreCG and right middle frontal gyrus (MFG) (Table 2, Figure 4). MCI patients showed decreased FC in right MFG, right IFG, bilateral cingulate gyrus, bilateral medial frontal gyrus (mFG), and bilateral superior frontal gyrus (SFG) (Table 2, Figure 4).
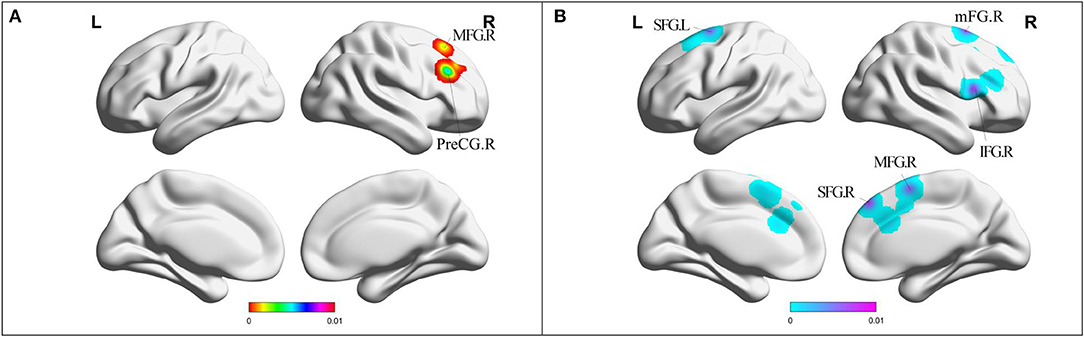
Figure 4. (A) Brain regions showing increased FC in MCI patients compared with HCs. (B) Brain regions showing decreased FC in MCI patients compared with HCs. MCI, amnestic mild cognitive impairment; FC, functional connectivity; HCs, healthy controls; PreCG, precentral gyrus; SFG, superior frontal gyrus; MFG, middle frontal gyrus; IFG, inferior frontal gyrus; mFG, medial frontal gyrus; R, right; L, left.
Discussion
Special Imaging Abnormal Markers
Our study was the first meta-analysis to identify specific brain region changes in the ECN and explore the interactions between the ECN and other networks. As expected, compared with HCs, the brain functional differences of MCI patients were observed in frontal regions, including mFG, IFG, SFG, and MFG, in addition to temporal regions, such as ITG, PHG, and MTG, as well as parietal regions, such as the PCC/PCUN, IPL, PCL, and SPL. There was little difference in the occipital lobes among the groups except for a small cluster of LING. Additionally, differences were also found in several subcortical regions, such as the lentiform nucleus (LN) and anterior and posterior lobe of the cerebellum.
In summary, reviewing the results of this paper, the ECN involved in frontal and parietal regions showed a downward trend in MCI patients. Areas of the ECN that showed an upward trend are mainly in the occipital, temporal, and subcortical regions with a small portion also rising in the frontal and parietal regions, which may be the compensatory mechanism of the disruption of frontal and parietal lobes in MCI patients (Valera-Bermejo et al., 2020). It is also well-established that the frontal and parietal regions are crucial aspects of the ECN, which send rich sensory information not only for movement controls, but also for other cognitive abilities, especially in executive function (Roh et al., 2020). Although the results reveal an increase in the frontal and parietal regions, these discrepant findings may be related to the different stages of MCI (Cosentino et al., 2020).
Interactive Neural Network
The neural networks interacting with ECN involve DMN, VN, and FPN. The cerebro-cerebellar loops also were affected. Further analyses were performed to estimate whether the co-activation patterns of these regions could be fully attributed to some recognized neural networks.
The co-activation patterns, driven by differences between MCI and HCs, involved bilateral PCC/PCUN, right IPL, right PHG, and right MTG, which were mainly located in the DMN. The DMN is usually active when the individual is awake and resting, not focused on the outside world (Zhang et al., 2019). In contrast, when the individual is in the routine task experiment with external stimuli, the network was in the deactivated state (Zhang et al., 2019). Converging lines of evidence indicate that DMN, where β-amyloid (Aβ) protein deposition tends to occur, is considered to play an important role in various cognitive functions (Esposito et al., 2018). It is well-known that DMN contributes to information processing related to motivation, emotion, learning, cognition, and memory (Nicholson et al., 2020). In the present results, decreased ALFF/fALFF and ReHo in MCI were all jointly located in bilateral PCUN, which engage in executive function. In addition to the bilateral PCUN, the PCC, right PHG, and right MTG exhibit a significant decreased group difference. Recent research revealed that PCC and PCUN appeared to have the most structural and functional alterations in MCI patients (Fuchs et al., 2020). From the structural point of view, a study highlighted the association between PHG and gait parameters in MCI patients. There was a linear correlation between the decrease of hippocampal gray matter volume and the gait parameters representing executive function (Cosentino et al., 2020). The reason why most of the abnormal areas are in the right hemisphere is that adults' cognitive networks are biased (Katsel et al., 2018). However, the increased ALFF value in the PCUN and IPL, which may be evidence of a compensatory mechanism at different stages of disease (Simo et al., 2018). As reported, a compensatory mechanism in brain networks is common (Simo et al., 2018).
DMN is involved with episodic memory (EM) in which deficits are a typical clinical symptom of MCI (Yuan B. et al., 2016). Previous studies demonstrate that overlapping brain regions between the EF and EM networks indeed exist and elaborate that the DMN and ECN are dynamically interactive and closely linked (Yuan B. et al., 2016). Additionally, patients with EF impairment can also simultaneously suffer from impaired EM. It has been proven that dysfunctional connectivity in the DMN may reflect the gradual decline from MCI to AD (Hojjati et al., 2018). As a result, the present findings in the DMN show the neural mechanisms related to the interaction between impaired EF and the DMN network in MCI patients in new sights. Focusing on these brain areas is beneficial to the later individualized intervention for AD progression.
LING has the most significantly increased difference in terms of ALFF/fALFF and ReHo. Alteration of ALFF/fALFF also indicated an increase in ITG, and alteration of ReHo also indicated an increase in cuneus. However, decreased ReHo of cuneus was still revealed. If disturbed, LING, which is the important region of VN, fails to process visual function (Li et al., 2020). VN anatomy areas include LING, CUN, PCUN, middle occipital gyrus, and ITG (Li et al., 2020). Previous studies have elucidated that multiple integrations of SMN, VN, and the cognitive network coordinate with each other to provide the organism with the signals to perceive and respond to its surrounding environment (Xu et al., 2020). That is to say, impairments in any of these components will lead to cognitive impairment at the clinical level.
In the early stages of the disease, when defects occur in any of the DMN and ECN, the VN connection may increase as a compensation owing to the whole collaborative brain system. However, studies have shown that visual impairment does not necessarily occur when a pathway in the visual network is interrupted with the disease progressing (He et al., 2020). With multiple pathways impaired, patients may have problems with space, orientation, color, and size in visual areas, which further affect the executive function (He et al., 2020). Although our results show both a decrease and increase in cuneus, this suggests that cuneus is an area that is easily affected in cognitive impairment. Compensation and impairment are manifested at different stages of the disease (Simo et al., 2018).
Compared with HCs, MCI groups exhibit decreased functional connectivity in SFG, mFG, MFG, IFG, and cingulate gyrus. The area of decreased ALFF/fALFF in patients was as well as in cingulate gyrus. Increased key areas with ECN include MFG, SPL, PreCG, and IFG. These specific regions are located in the frontal-parietal cognitive/attention network (involved in many cognitive tasks, cognitive control, learning and planning, etc.) (Margolis et al., 2019). Based on resting-state functional connectivity and graph theory analysis, a two-system model for cognitive control, including FPN and the cingulo-opercular network, is proposed as the commanders of the brain (Cignetti et al., 2018). More specifically, the two networks that are hypothesized to be responsible for a dual-system of top-down control involve the cingulate cortex, dorsolateral prefrontal cortex (DLPFC), premotor cortex, anterior prefrontal cortex, IPL, medial frontal cortex, and superior portions of the parietal cortex (Cignetti et al., 2018).
A randomized controlled trial pointed out that executive functions, including control of thoughts and actions, fine-tuning attention, and information acquisition and analysis, are regarded to be involved in the attentional network model (van Houdt et al., 2019). As the important node of FPN, SPL is connected with visual attention. Our results show a functional disconnection within a distributed FPN network and confirm that disconnection of the prefrontal cortex and cingulate gyrus can also be detected in MCI, the early stage of AD. As we know, the frontal lobe is particularly advanced, involved in processing memory and emotion (Zhang et al., 2020). The anterior prefrontal cortex is a component gathered by IFG, SFG, MFG, and PreCG (Zhang et al., 2020). Current research confirms that the completion of executive function depends on the dynamic interaction between the prefrontal cortex and other cortical regions (Liang et al., 2012). In contrast, compensation coexists in MCI patients, which is the reason for the appearance of increasing frontal regions, especially in the early stage of AD.
In recent years, the role of the cerebellum in cognition has been gradually studied, especially in the collaborative management of cognitive functions within the brain. Posterior lobe lesions lead to cerebellar cognitive affective syndrome (CCAS), the typical characteristics of which involve deficits in executive function, linguistic learning, visual spatial processing, and regulation of affect (Wang et al., 2019). Previous studies demonstrate that the underlying mechanisms of cognitive impairment with cerebellar lesions might include abnormal fiber connections observed between the cerebellum and the functional areas of the cerebrum, particularly in the precuneus, cingulate gyrus, and frontal and temporal lobes (Schmahmann, 2019). In the present study, increased ALFF/fALFF in CPL and CAL were of certain important significance in the ECN. Considering the cerebro-cerebellar loops, it is reasonable to speculate that the increase of signal in the posterior cerebellum is a compensatory mechanism after the impairment of executive function in the MCI group (Schmahmann, 2019).
Limitations
Although we have achieved valuable results, some shortcomings still need mentioning. First, the study is limited due to the existence of heterogeneity, including different data sources, preprocessing protocols, statistical or imaging methods, and threshold settings, which may have affected our results in this study. However, heterogeneity differences in study characteristics are negligible with respect to the changes in neuroimaging, which is a result from multicenter studies (Pardoe et al., 2008). Second, the subgroup analysis based on the MCI refinement classification cannot be carried out because the number of articles is inadequate. Third, confounding factors, such as age and gender, are as well as the unavoidable limitations.
Clinical Implications
Although valuable clinical information is provided by a single study, it is essential to make quantitative neuroimaging analysis by summarizing the existing research results, which is helpful to consolidate our understanding of the pathophysiology of MCI. In terms of the significant overlapping regions, such as precuneus, cingulate gyrus, and lingual gyrus in ECN, it is no doubt that the findings of our article will provide new insight into cooperative interaction between ECN and other networks, which can be used as neural markers for disease improvement in different pathological states. TMS therapeutic target design and drug treatment will obtain a more objective basis. In a word, our results strongly reveal the imaging features of disease-specific brain area damage and lay the foundation for in-depth study of the pathogenesis of MCI.
Conclusion
In the present study, we elucidate the evidence of special imaging biomarkers in the ECN and three abnormal neural networks whose core regions overlap in the ECN, including DMN, FPN network, cerebro-cerebellar loops, and interact with ECN with various methods of rsfMRI analysis. These findings provide evidence for further understanding the potential cerebral alterations in MCI. These meaningfully overlapping networks provide a new perspective for future selection of specific brain regions. However, further studies for classification of MCI among these networks are essential to explore the potential role of imaging as a biomarker for MCI.
Author Contributions
WX guided by XL and JC designed the study. WX and SC performed the meta-analysis and drafted the manuscript. CX, GH, WM, and WQ helped in literature extraction and data analyses.
Funding
This study was supported by the National Natural Science Foundation of China (No. 81701675), the Key Project supported by the Medical Science and technology development Foundation, Nanjing Department of Health (No. JQX18005), the Cooperative Research Project of Southeast University-Nanjing Medical University (No. 2018DN0031), the Key Research and Development Plan (Social Development) Project of Jiangsu Province (No. BE2018608), the Innovation and Entrepreneurship Training Program for College Students in Jiangsu Province (Nos. 201810312061X and 201910312035Z), and Key Scientific Research Projects of Colleges and Universities in Henan Province (No. 18A190003).
Conflict of Interest
The authors declare that the research was conducted in the absence of any commercial or financial relationships that could be construed as a potential conflict of interest.
Supplementary Material
The Supplementary Material for this article can be found online at: https://www.frontiersin.org/articles/10.3389/fnagi.2020.578863/full#supplementary-material
References
Brier, M. R., Thomas, J. B., Snyder, A. Z., Benzinger, T. L., Zhang, D., Raichle, M. E., et al. (2012). Loss of intranetwork and internetwork resting state functional connections with Alzheimer's disease progression. J. Neurosci. 32, 8890–8899. doi: 10.1523/JNEUROSCI.5698-11.2012
Cai, S., Chong, T., Peng, Y., Shen, W., Li, J., von Deneen, K. M., et al. (2017). Altered functional brain networks in amnestic mild cognitive impairment: a resting-state fMRI study. Brain Imaging Behav. 11, 619–631. doi: 10.1007/s11682-016-9539-0
Cai, S., Wang, Y., Kang, Y., Wang, H., Kim, H., von Deneen, K. M., et al. (2018). Differentiated regional homogeneity in progressive mild cognitive impairment: a study with post hoc label. Am. J. Alzheimers Dis. Other Demen. 33, 373–384. doi: 10.1177/1533317518778513
Chen, J., Shu, H., Wang, Z., Zhan, Y., Liu, D., Liao, W., et al. (2016). Convergent and divergent intranetwork and internetwork connectivity patterns in patients with remitted late-life depression and amnestic mild cognitive impairment. Cortex 83, 194–211. doi: 10.1016/j.cortex.2016.08.001
Chen, Y., Kumfor, F., Landin-Romero, R., Irish, M., and Piguet, O. (2019). The cerebellum in frontotemporal dementia: a meta-analysis of neuroimaging studies. Neuropsychol. Rev. 29, 450–464. doi: 10.1007/s11065-019-09414-7
Cignetti, F., Vaugoyeau, M., Decker, L. M., Grosbras, M. H., Girard, N., Chaix, Y., et al. (2018). Brain network connectivity associated with anticipatory postural control in children and adults. Cortex 108, 210–221. doi: 10.1016/j.cortex.2018.08.013
Cosentino, E., Palmer, K., Della Pieta, C., Mitolo, M., Meneghello, F., Levedianos, G., et al. (2020). Association between gait, cognition, and gray matter volumes in mild cognitive impairment and healthy controls. Alzheimer Dis. Assoc. Disord. 34, 231–237. doi: 10.1097/WAD.0000000000000371
Doucet, G. E., Janiri, D., Howard, R., O'Brien, M., Andrews-Hanna, J. R., and Frangou, S. (2020). Transdiagnostic and disease-specific abnormalities in the default-mode network hubs in psychiatric disorders: a meta-analysis of resting-state functional imaging studies. Eur. Psychiatry 63:e57. doi: 10.1192/j.eurpsy.2020.57
Eickhoff, S. B., Bzdok, D., Laird, A. R., Kurth, F., and Fox, P. T. (2012). Activation likelihood estimation meta-analysis revisited. Neuroimage 59, 2349–2361. doi: 10.1016/j.neuroimage.2011.09.017
Esposito, R., Cieri, F., Chiacchiaretta, P., Cera, N., Lauriola, M., Di Giannantonio, M., et al. (2018). Modifications in resting state functional anticorrelation between default mode network and dorsal attention network: comparison among young adults, healthy elders and mild cognitive impairment patients. Brain Imaging Behav. 12, 127–141. doi: 10.1007/s11682-017-9686-y
Fuchs, T. A., Ziccardi, S., Benedict, R. H. B., Bartnik, A., Kuceyeski, A., Charvet, L. E., et al. (2020). Functional connectivity and structural disruption in the default-mode network predicts cognitive rehabilitation outcomes in multiple sclerosis. J. Neuroimaging 30, 523–530. doi: 10.1111/jon.12723
He, L., Wang, X., Zhuang, K., and Qiu, J. (2020). Decreased dynamic segregation but increased dynamic integration of the resting-state functional networks during normal aging. Neuroscience 437, 54–63. doi: 10.1016/j.neuroscience.2020.04.030
Hojjati, S. H., Ebrahimzadeh, A., Khazaee, A., and Babajani-Feremi, A. Alzheimer's Disease Neuroimaging (2018). Predicting conversion from MCI to AD by integrating rs-fMRI and structural MRI. Comput. Biol. Med. 102, 30–39. doi: 10.1016/j.compbiomed.2018.09.004
Jia, B., Liu, Z., Min, B., Wang, Z., Zhou, A., Li, Y., et al. (2015). The effects of acupuncture at real or sham acupoints on the intrinsic brain activity in mild cognitive impairment patients. Evid. Based Complement Alternat. Med. 2015:529675. doi: 10.1155/2015/529675
Joshi, H., Bharath, S., Balachandar, R., Sadanand, S., Vishwakarma, H. V., Aiyappan, S., et al. (2019). Differentiation of early alzheimer's disease, mild cognitive impairment, and cognitively healthy elderly samples using multimodal neuroimaging indices. Brain Connect. 9, 730–741. doi: 10.1089/brain.2019.0676
Katsel, P., Roussos, P., Beeri, M. S., Gama-Sosa, M. A., Gandy, S., Khan, S., et al. (2018). Parahippocampal gyrus expression of endothelial and insulin receptor signaling pathway genes is modulated by Alzheimer's disease and normalized by treatment with anti-diabetic agents. PLoS ONE 13:e0206547. doi: 10.1371/journal.pone.0206547
Leech, R., and Sharp, D. J. (2014). The role of the posterior cingulate cortex in cognition and disease. Brain 137, 12–32. doi: 10.1093/brain/awt162
Li, S., Lv, P., He, M., Zhang, W., Liu, J., Gong, Y., et al. (2020). Cerebral regional and network characteristics in asthma patients: a resting-state fMRI study. Front. Med. doi: 10.1007/s11684-020-0745-1
Liang, P., Wang, Z., Yang, Y., and Li, K. (2012). Three subsystems of the inferior parietal cortex are differently affected in mild cognitive impairment. J. Alzheimers Dis. 30, 475–487. doi: 10.3233/JAD-2012-111721
Liang, P. P., Wang, Z. Q., Yang, Y. H., Jia, X. Q., and Li, K. C. (2011). Functional disconnection and compensation in mild cognitive impairment: evidence from DLPFC connectivity using resting-state fMRI. PLoS ONE 6:e22153. doi: 10.1371/journal.pone.0022153
Liu, R., Hu, B., Yao, Z., Ratcliffe, M., Wang, W., Liang, C., et al. (2013). “Abnormal neural activity and functional connectivity in amnestic Mild cognitive impairmet: a resting state fMRI study,” in International IEEE/EMBS Conference on Neural Engineering (Piscataway, NJ). doi: 10.1109/NER.2013.6696047
Liu, X., Bai, F., Yue, C., Shi, Y., Yu, H., Luo, B., et al. (2014). The association between TOMM40 gene polymorphism and spontaneous brain activity in amnestic mild cognitive impairment. J. Neurol. 261, 1499–1507. doi: 10.1007/s00415-014-7368-x
Liu, Y., Chen, Y., Liang, X., Li, D., Zheng, Y., Zhang, H., et al. (2020). Altered resting-state functional connectivity of multiple networks and disrupted correlation with executive function in major depressive disorder. Front. Neurol. 11:272. doi: 10.3389/fneur.2020.00272
Liu, Z., Wei, W., Bai, L., Dai, R., You, Y., Chen, S., et al. (2014). Exploring the patterns of acupuncture on mild cognitive impairment patients using regional homogeneity. PLoS ONE 9:e99335. doi: 10.1371/journal.pone.0099335
Luo, X., Jiaerken, Y., Huang, P. Y., Xu, X. J., Qiu, T. T., Jia, Y. L., et al. (2018). Alteration of regional homogeneity and white matter hyperintensities in amnestic mild cognitive impairment subtypes are related to cognition and CSF biomarkers. Brain Imaging Behav. 12, 188–200. doi: 10.1007/s11682-017-9680-4
Margolis, A. E., Pagliaccio, D., Davis, K. S., Thomas, L., Banker, S. M., Cyr, M., et al. (2019). Neural correlates of cognitive control deficits in children with reading disorder. Brain Imaging Behav. doi: 10.1007/s11682-019-00083-x
Min, J., Zhou, X. X., Zhou, F., Tan, Y., and Wang, W. D. (2019). A study on changes of the resting-state brain function network in patients with amnestic mild cognitive impairment. Braz. J. Med. Biol. Res. 52:e8244. doi: 10.1590/1414-431x20198244
Nicholson, A. A., Harricharan, S., Densmore, M., Neufeld, R. W. J., Ros, T., McKinnon, M. C., et al. (2020). Classifying heterogeneous presentations of PTSD via the default mode, central executive, and salience networks with machine learning. Neuroimage Clin. 27:102262. doi: 10.1016/j.nicl.2020.102262
Pardoe, H., Pell, G. S., Abbott, D. F., Berg, A. T., and Jackson, G. D. (2008). Multi-site voxel-based morphometry: methods and a feasibility demonstration with childhood absence epilepsy. Neuroimage 42, 611–616. doi: 10.1016/j.neuroimage.2008.05.007
Petersen, N., Ghahremani, D. G., Rapkin, A. J., Berman, S. M., Wijker, N., Liang, L., et al. (2019). Resting-state functional connectivity in women with PMDD. Transl. Psychiatry 9:339. doi: 10.1038/s41398-019-0670-8
Robinson, J. L., Laird, A. R., Glahn, D. C., Blangero, J., Sanghera, M. K., Pessoa, L., et al. (2012). The functional connectivity of the human caudate: an application of meta-analytic connectivity modeling with behavioral filtering. Neuroimage 60, 117–129. doi: 10.1016/j.neuroimage.2011.12.010
Robinson, J. L., Laird, A. R., Glahn, D. C., Lovallo, W. R., and Fox, P. T. (2010). Metaanalytic connectivity modeling: delineating the functional connectivity of the human amygdala. Hum. Brain Mapp. 31, 173–184. doi: 10.1002/hbm.20854
Roh, H. W., Choi, J. G., Kim, N. R., Choe, Y. S., Choi, J. W., Cho, S. M., et al. (2020). Associations of rest-activity patterns with amyloid burden, medial temporal lobe atrophy, and cognitive impairment. EBioMedicine 58:102881. doi: 10.1016/j.ebiom.2020.102881
Schmahmann, J. D. (2019). The cerebellum and cognition. Neurosci Lett. 688, 62–75. doi: 10.1016/j.neulet.2018.07.005
Simo, M., Rifa-Ros, X., Vaquero, L., Ripolles, P., Cayuela, N., Jove, J., et al. (2018). Brain functional connectivity in lung cancer population: an exploratory study. Brain Imaging Behav. 12, 369–382. doi: 10.1007/s11682-017-9697-8
Trossman, R., Mielke, J. G., and McAuley, T. (2020). Global executive dysfunction, not core executive skills, mediate the relationship between adversity exposure and later health in undergraduate students. Appl. Neuropsychol. Adult. 23, 1–7. doi: 10.1080/23279095.2020.1764561
Valera-Bermejo, J. M., De Marco, M., Mitolo, M., McGeown, W. J., and Venneri, A. (2020). Neuroanatomical and cognitive correlates of domain-specific anosognosia in early Alzheimer's disease. Cortex 129, 236–246. doi: 10.1016/j.cortex.2020.04.026
van Houdt, C. A., Aarnoudse-Moens, C. S. H., van Wassenaer-Leemhuis, A. G., Laarman, A. R. C., Koopman-Esseboom, C., van Kaam, A. H., et al. (2019). Effects of executive function training on attentional, behavioral and emotional functioning and self-perceived competence in very preterm children: a randomized controlled trial. Front. Psychol. 10:2100. doi: 10.3389/fpsyg.2019.02100
Wang, D., Yao, Q., Yu, M., Xiao, C., Fan, L., Lin, X., et al. (2019). Topological disruption of structural brain networks in patients with cognitive impairment following cerebellar infarction. Front. Neurol. 10:759. doi: 10.3389/fneur.2019.00759
Wang, Y. M., Zhao, X. C., Xu, S. J., Yu, L. L., Wang, L., Song, M., et al. (2015). Using regional homogeneity to reveal altered spontaneous activity in patients with mild cognitive impairment. Biomed Res. Int. 2015:807093. doi: 10.1155/2015/807093
Wang, Z., Yan, C., Zhao, C., Qi, Z., Zhou, W., Lu, J., et al. (2011). Spatial patterns of intrinsic brain activity in mild cognitive impairment and Alzheimer's disease: a resting-state functional MRI study. Hum Brain Mapp. 32, 1720–1740. doi: 10.1002/hbm.21140
Wee, C. Y., Yang, S., Yap, P. T., Shen, D., and Alzheimer's Disease Neuroimaging, I. (2016). Sparse temporally dynamic resting-state functional connectivity networks for early MCI identification. Brain Imaging Behav. 10, 342–356. doi: 10.1007/s11682-015-9408-2
Weil, R. S., Hsu, J. K., Darby, R. R., Soussand, L., and Fox, M. D. (2019). Neuroimaging in Parkinson's disease dementia: connecting the dots. Brain Commun. 1:fcz006. doi: 10.1093/braincomms/fcz006
Wu, L., Soder, R. B., Schoemaker, D., Carbonnell, F., Sziklas, V., Rowley, J., et al. (2014). Resting state executive control network adaptations in amnestic mild cognitive impairment. J Alzheime's Dis. 40, 993–1004. doi: 10.3233/JAD-131574
Xi, Q., Zhao, X. H., Wang, P. J., Guo, Q. H., and He, Y. (2013). Abnormal intrinsic brain activity in amnestic mild cognitive impairment revealed by amplitude of low-frequency fluctuation: a resting-state functional magnetic resonance imaging study. Chin. Med. J. 126, 2912–2917. doi: 10.3760/cma.j.issn.0366-6999.20130431
Xu, X., Li, W., Mei, J., Tao, M., Wang, X., Zhao, Q., et al. (2020). Feature selection and combination of information in the functional brain connectome for discrimination of mild cognitive impairment and analyses of altered brain patterns. Front. Aging Neurosci. 12:28. doi: 10.3389/fnagi.2020.00028
Yang, L., Yan, Y., Li, Y., Hu, X., Lu, J., Chan, P., et al. (2019). Frequency-dependent changes in fractional amplitude of low-frequency oscillations in Alzheimer's disease: a resting-state fMRI study. Brain Imaging Behav. doi: 10.1007/s11682-019-00169-6
Yang, L., Yan, Y., Wang, Y., Hu, X., Lu, J., Chan, P., et al. (2018). Gradual disturbances of the amplitude of low-frequency fluctuations (ALFF) and fractional ALFF in alzheimer spectrum. Front. Neurosci. 12:975. doi: 10.3389/fnins.2018.00975
Yin, C. H., Yi, L. Y., Jia, L. F., Wang, J. H., Liu, P. F., Guo, Y. Q., et al. (2014). Early morphological brain abnormalities in patients with amnestic mild cognitive impairment. Transl. Neurosci. 5, 253–259. doi: 10.2478/s13380-014-0234-6
Yu, E., Liao, Z., Tan, Y., Qiu, Y., Zhu, J., Han, Z., et al. (2019). High-sensitivity neuroimaging biomarkers for the identification of amnestic mild cognitive impairment based on resting-state fMRI and a triple network model. Brain Imaging Behav. 13, 1–14. doi: 10.1007/s11682-017-9727-6
Yuan, B., Chen, J., Gong, L., Shu, H., Liao, W., Wang, Z., et al. (2016). Mediation of episodic memory performance by the executive function network in patients with amnestic mild cognitive impairment: a resting-state functional MRI study. Oncotarget 7, 64711–64725. doi: 10.18632/oncotarget.11775
Yuan, X., Han, Y., Wei, Y., Xia, M., Sheng, C., Jia, J., et al. (2016). Regional homogeneity changes in amnestic mild cognitive impairment patients. Neurosci. Lett. 629, 1–8. doi: 10.1016/j.neulet.2016.06.047
Zhang, L., Li, W., Wang, L., Bai, T., Ji, G. J., Wang, K., et al. (2020). Altered functional connectivity of right inferior frontal gyrus subregions in bipolar disorder: a resting state fMRI study. J. Affect. Disord. 272, 58–65. doi: 10.1016/j.jad.2020.03.122
Zhang, Z., Zhou, X., Liu, J., Qin, L., Ye, W., and Zheng, J. (2019). Aberrant executive control networks and default mode network in patients with right-sided temporal lobe epilepsy: a functional and effective connectivity study. Int. J. Neurosci. 130, 683–693. doi: 10.1080/00207454.2019.1702545
Zhao, Z., Lu, J., Jia, X., Chao, W., Han, Y., Jia, J., et al. (2014). Selective changes of resting-state brain oscillations in aMCI: An fMRI study using ALFF. Biomed. Res. Int. 2014:920902. doi: 10.1155/2014/920902
Zheng, W., Cui, B., Han, Y., Song, H., Li, K., He, Y., et al. (2019). Disrupted regional cerebral blood flow, functional activity and connectivity in alzheimer's disease: a combined ASL perfusion and resting state fMRI study. Front. Neurosci. 13:738. doi: 10.3389/fnins.2019.00738
Zhou, Q. H., Wang, K., Zhang, X. M., Wang, L., and Liu, J. H. (2020). differential regional brain spontaneous activity in subgroups of mild cognitive impairment. Front. Hum. Neurosci. 14:2. doi: 10.3389/fnhum.2020.00002
Zhu, H., Zhou, P., Alcauter, S., Chen, Y., Cao, H., Tian, M., et al. (2016). Changes of intranetwork and internetwork functional connectivity in Alzheimer's disease and mild cognitive impairment. J. Neural Eng. 13:046008. doi: 10.1088/1741-2560/13/4/046008
Zhuang, L., Liu, X., Shi, Y., Liu, X., and Luo, B. (2019). Genetic variants of PICALM rs541458 modulate brain spontaneous activity in older adults with amnestic mild cognitive impairment. Front. Neurol. 10:494. doi: 10.3389/fneur.2019.00494
Keywords: mild cognitive impairment, resting state, executive control network, the amplitude of low frequency fluctuation, regional homogeneity, functional connectivity
Citation: Xu W, Chen S, Xue C, Hu G, Ma W, Qi W, Lin X and Chen J (2020) Functional MRI-Specific Alterations in Executive Control Network in Mild Cognitive Impairment: An ALE Meta-Analysis. Front. Aging Neurosci. 12:578863. doi: 10.3389/fnagi.2020.578863
Received: 01 July 2020; Accepted: 07 September 2020;
Published: 09 October 2020.
Edited by:
Jolanta Dorszewska, Poznan University of Medical Sciences, PolandReviewed by:
Zhiqun Wang, Aerospace Center Hospital, ChinaFeng Bai, Nanjing Drum Tower Hospital, China
Copyright © 2020 Xu, Chen, Xue, Hu, Ma, Qi, Lin and Chen. This is an open-access article distributed under the terms of the Creative Commons Attribution License (CC BY). The use, distribution or reproduction in other forums is permitted, provided the original author(s) and the copyright owner(s) are credited and that the original publication in this journal is cited, in accordance with accepted academic practice. No use, distribution or reproduction is permitted which does not comply with these terms.
*Correspondence: Xingjian Lin, bGlueGluZ2ppYW5AbmptdS5lZHUuY24=; Jiu Chen, ZXJpY2NzdEBhbGl5dW4uY29t
†These authors have contributed equally to this work and share first authorship