- 1Department of Neurology, Affiliated ZhongDa Hospital, School of Medicine, Southeast University, Nanjing, China
- 2Neuropsychiatric Institute, Affiliated ZhongDa Hospital, School of Medicine, Southeast University, Nanjing, China
- 3The Key Laboratory of Developmental Genes and Human Disease, Southeast University, Nanjing, China
- 4Department of Nursing, Affiliated ZhongDa Hospital, School of Medicine, Southeast University, Nanjing, China
Objective: To investigate variation in the characteristics of regional cerebral blood flow (rCBF), brain activity, and intrinsic functional connectivity (FC) across the Alzheimer's disease spectrum (ADS).
Methods: The study recruited 20 individuals in each of the following categories: Alzheimer's disease (AD), mild cognitive impairment (MCI), subjective cognitive decline (SCD), and healthy control (HC). All participants completed the 3.0T resting-state functional MRI (rs-fMRI) and arterial spin labeling scans in addition to neuropsychological tests. Additionally, the normalized CBF, regional homogeneity (ReHo), and amplitude of low-frequency fluctuation (ALFF) of individual subjects were compared in the ADS. Moreover, the changes in intrinsic FC were investigated across the ADS using the abnormal rCBF regions as seeds and behavioral correlations. Finally, a support-vector classifier model of machine learning was used to distinguish individuals with ADS from HC.
Results: Compared to the HC subjects, patients with AD showed the poorest level of rCBF in the left precuneus (LPCUN) and right middle frontal gyrus (RMFG) among all participants. In addition, there was a significant decrease in the ALFF in the bilateral posterior cingulate cortex (PCC) and ReHo in the right PCC. Moreover, RMFG- and LPCUN-based FC analysis revealed that the altered FCs were primarily located in the posterior brain regions. Finally, a combination of altered rCBF, ALFF, and ReHo in posterior cingulate cortex/precuneus (PCC/PCUN) showed a better ability to differentiate ADS from HC, AD from SCD and MCI, but not MCI from SCD.
Conclusions: The study demonstrated the significance of an altered rCBF and brain activity in the early stages of ADS. These findings, therefore, present a potential diagnostic neuroimaging-based biomarker in ADS. Additionally, the study provides a better understanding of the pathophysiology of AD.
Introduction
Alzheimer's disease (AD) is one of the most important public health burdens worldwide. Notably, constant frustrations in drug development against the disease revealed the complexity of the pathogenic mechanism of AD. This, therefore, suggested that a more comprehensive study of specific neurobiological changes should be performed across the Alzheimer's disease spectrum (ADS), at both the preclinical and clinical stages. Moreover, the search for effective biomarkers is essential for the implementation of effective interventions before the development of significant neuronal damage.
Multimodal MRI has extensively been applied to investigate the abnormalities in brain structure and function in the ADS. In addition, the estimation of medial temporal lobe atrophy (MTA) by structural MRI (sMRI) is used as a neuroimaging biomarker in the diagnosis of AD (Ten et al., 2017). However, the obvious brain structure atrophy might imply the appearance of irreversible neuronal damage in the process of AD pathophysiology. In contrast, the resting-state functional MRI (rs-fMRI) can detect early functional changes in the brain reflected by the intrinsic blood-oxygen-level-dependent (BOLD) signals before the appearance of cognitive decline and brain structural atrophy (Galvin et al., 2011; Habib et al., 2017; Kawagoe et al., 2019). Moreover, several brain regions, including the hippocampus (HIP), posterior cingulate cortex (PCC), precuneus (PCUN), prefrontal cortex (PFC), temporal lobe, and angular gyrus (ANG) (Kawagoe et al., 2019; Xue et al., 2019; Zheng et al., 2019), have been reported as the core hub of brain networks involved in the pathophysiology of AD. Importantly, functional changes in the brain are independent of and even more sensitive than brain structure atrophy during the early stages of AD (Galvin et al., 2011; Xie et al., 2015; Kawagoe et al., 2019).
In addition, arterial spin labeling (ASL), utilizing intravascular water as an endogenous contrast agent, can measure regional cerebral blood flow (rCBF) (Ma et al., 2017). Notably, several studies consistently reported that rCBF displayed a decreasing trend with the progression of cognitive impairment in ADS (Binnewijzend et al., 2013; Ding et al., 2014; Trebeschi et al., 2016; Ma et al., 2017; Li et al., 2020). Moreover, compared to the age-matched subjects of health control (HC) and subjects of subjective cognitive decline (SCD), subjects of the mild cognitive impairment (MCI) and patients with AD presented decreased perfusion in the parietal lobe, PCC/PCUN, and occipital lobe (Binnewijzend et al., 2013; Ding et al., 2014; Trebeschi et al., 2016; Ma et al., 2017; Duan et al., 2020). Furthermore, the perfusion patterns identified by ASL were highly congruent with that provided by PET (Schroeter et al., 2009; Riederer et al., 2018; Dolui et al., 2020). PCC/PCUN, as one of the core regions in the default mode network (DMN), has been widely reported to be associated with a decreased rCBF, disrupted activity, and a destructive brain network in subjects with MCI and subjects with AD (Yoshiura et al., 2009; Sierra-Marcos, 2017; Xue et al., 2019). Notably, functional abnormalities of PCC/PCUN were also associated with the increased amyloid burden and decreased hippocampal volume (Khan et al., 2020). Additionally, a recent study, which explored the correlation between an altered rCBF and brain function in AD, revealed that the combination of ASL and the amplitude of low-frequency fluctuations (ALFF) in the PCC/PCUN could be used as a potential biomarker for the diagnosis of AD (Zheng et al., 2019). However, whether the integration of an altered rCBF and functional parameters in PCC/PCUN is capable of predicting various stages of AD is still unclear.
Therefore, the present study aimed to investigate the altered brain perfusion and function in all phases of ADS. First, the study measured whole-brain rCBF, regional homogeneity (ReHo), and the ALFF in each subject through the ASL and rs-fMRI approaches. Second, a partial correlation analysis was performed between the altered regions of each modality image and neuropsychological tests in ADS to obtain the behavioral significance of these altered brain functions. Third, the study investigated the changes in whole-brain functional connectivity of the identified rCBF regions as a seed in the ADS. Finally, the altered rCBF, ALFF, and ReHo in PCC/PCUN were integrated to get an imaging biomarker for the prediction of the ADS using a linear support vector machine (SVM) based on the machine learning approach.
Materials and Methods
Participants
Participants were recruited from media advertisements and neurology outpatient clinics of the Affiliated Zhongda Hospital, Southeast University (Nanjing, China). All the subjects and their relatives were then provided with all relevant details before signing a written informed consent to participate in the study. A total of 80 Han Chinese individuals from eastern China were included in the study. Additionally, the participants underwent a full neuropsychological test battery, physical examination, blood tests, and a multi-modal MRI brain scan. This study was approved by the Research Ethics Committee of the Affiliated Zhongda Hospital, Southeast University (Nanjing, China).
Neuropsychological Assessments
Comprehensive cognitive function assessment and neurological examination were conducted on all the participants by two experienced neuropsychiatrists. The items specifically included activities of daily living (ADL), the Hamilton Depression Scale (HAMD), the Hachinski Ischemic Scale (HIS), the Mini-Mental State Examination (MMSE), and additional tests that covered the four previously characterized cognitive domains, namely: memory (episodic memory), information processing speed, visuospatial function, and executive function. In addition, memory tests included the Auditory Verbal Learning Test-20-min-Delayed Recall (AVLT-20-min-DR), Logical Memory Test-20-min-Delayed Recall (LMT-20-min-DR), and the Rey–Osterrieth Complex Figure Test-20-min-Delayed Recall (ROCFT-20-min-DR). On the other hand, the information processing speed was measured using the Symbol Digit Modalities Test (DSST), Trail Making Tests-A (TMT-A), and the Stroop Color and Word Test A and B. Moreover, the visuospatial function domain included the Clock Drawing Test (CDT) and the ROCFT. Additionally, the executive function domain was measured using the Stroop Color and Word Test C, the Digit Span Test (DST), the Verbal Fluency Test (VFT), the Trail Making Tests-B (TMT-B), and the Semantic Similarity Test (Shi et al., 2019). Finally, the raw scores of each test were transformed into z-scores using the mean and SD in order to calculate the composite score of each cognitive domain (Xie et al., 2012).
Inclusion and Exclusion Criteria
All subjects were independently evaluated and diagnosed by two experienced neuropsychiatrists. Participants were required to meet the following criteria: (1) 55–85 years old, (2) educational years ≥8, (3) right-handed, and (4) should have been from the Han Chinese population. In addition, the inclusion criteria for HC contained: (1) no memory complaints and normal in ADL, (2) all neuropsychological tests were within the normal range, and (3) no abnormal findings in routine brain MRI (Dubois et al., 2014; Yan et al., 2018). On the other hand, the eligibility criteria for SCD contained: (1) frequent complaints of memory problems; (2) normal neuropsychological performance of age- and education-matched norms; and (3) lack of impairments in the ADL (Dubois et al., 2014; Yan et al., 2018). Moreover, the inclusion criteria for MCI contained: (1) complaints of memory impairment for more than 3 months; (2) MMSE score ≥24 and HAMD ≤ 7; (3) objective impairment in at least one cognitive domain, AVLT-20-min-DR score within ≤ 1.5 SD of the same age- and education-adjusted norms (cut-off of ≤ 4 correct responses on 12 items for subjects); and (4) no dementia (Dunn et al., 2014; Shi et al., 2019). The clinical diagnosis of AD was based on the criteria by the National Institute of Neurological and Communicative Disorders and Stroke and the Alzheimer's Disease and Related Disorders Association (NINCDS-ADRDA). They included (1) a clear-cut history of worsening cognition over 6 months; (2) MMSE score <24; (3) impairments in the ADL; and (4) dementia (McKhann et al., 2011; Arevalo-Rodriguez et al., 2015).
On the other hand, the exclusion criteria were as follows: (1) history of serious neurological and psychiatric diseases including major depressive disorders, schizophrenia, hydrocephalus, significant cerebrovascular disorders, and brain trauma; (2) systemic illnesses, such as uncontrolled hypertension, diabetes, abnormalities in the thyroid hormone, folic acid levels, vitamin B12, or significant liver and kidney diseases; and (3) the inability to undergo an MRI scan (Xie et al., 2012; Shi et al., 2019).
Acquisition of MRI Data
All MRI data was obtained using the Siemens Verio 3Tesla MRI Scanner with an 8-channel head-coil. In addition, the rs-fMRI images were obtained using the following parameters: 240 time points, repetition time (TR) = 2,000 ms, echo time (TE) = 25 ms, flip angle = 90°, number of slices = 36, slice thickness = 4 mm, spatial resolution = 3.75 × 3.75 × 4 mm3, acquisition matrix = 64 × 64, and field of view (FOV) = 240 × 240 mm2. Additionally, the 3D magnetization-prepared rapid gradient echo (MP-RAGE) were acquired to get the T1-weighted images with the following data parameters: TR = 1,900 ms, TE = 2.48 ms, slice thickness = 1 mm, FA = 90°, FOV = 256 × 256 mm, gap = 0 mm, and number of slices = 176. Moreover, the ASL data was obtained using the following parameters: TI1 = 600 ms, TI2 = 1.6 s, flip angle = 90°, number of slices = 27, slice thickness = 4.0 mm, TR = 4 s, TE = 12 ms, FOV = 220 × 220 mm2, and matrix size = 64 × 64.
Data Pre-processing
SPM8 software (http://www.fil.ion.ucl.ac.uk/spm) was used to analyze the T1-weighted and ASL images. First, the T1-weighted images were segmented into three parts [cerebrospinal fluid (CSF), gray matter (GM), and white matter] using the VBM8 toolbox (http://dbm.neuro.uni-jena.de/wordpress/vbm/). Out of these, the segmented GM volume was normalized and regressed out as a covariate to control the effects of GM volume on the analysis of rCBF, ALFF, ReHo, and FC. Thereafter, deformation matrices were used to co-register the ASL images to the corresponding native GM images, which were spatially normalized to the Montreal Neurological Institute (MNI) space. During spatial normalization, the ASL images were resampled into 2 × 2 × 2 mm3 voxel size. Finally, the resulting ASL images were smoothened using an isotropic 6 mm Gaussian filter for subsequent multiple comparison analysis.
Additionally, Data Processing & Analysis for Brain Imaging (DPABI, http://www.rfmri.org) was used to perform ALFF, ReHo, and seed-based FC analysis on the rs-fMRI images (Yan et al., 2016). Briefly, the first 10 volumes for each subject were removed in case of possible instability in the rs-fMRI signal. The remaining 230 points in time were then corrected for timing differences before adopting the Friston 24-parameter model to regress out the effects of head motion from realignment (Qi et al., 2020). All subjects with cumulative translation and rotation of head motion were <2 mm or 2°. Thereafter, the original space was registered to the MNI space with a resampled voxel size of 3 mm isotropic by using the DARTEL templates created during the preprocessing of T1-weighted images (Ashburner, 2007), which could alleviate the interference from different brain structures between subjects. Following this, the normalized images were smoothed with a 6 × 6 × 6 mm Gaussian kernel to reduce variation. The effects of confounding factors were then removed and they included the global mean signal, CSF signal, and white matter signal (Zheng et al., 2019). Finally, the previously generated images were filtered between 0.01 and 0.08 Hz so as to control noise interferences.
Moreover, a seed-based connectivity analysis was adopted to investigate FC changes in the ADS. Regions showing significant differences in rCBF among the groups were selected as regions of interest (ROIs). The mean time series of the seed regions was extracted for each participant and correlated with each voxel of the whole brain to obtain the seed-based FC maps, which were then transformed to z-maps based on the Fisher z-transformation (Waltz et al., 2013; Wang et al., 2019).
Statistical Analyses
The analysis of variance (ANOVA) and chi-square tests were used for demographic characteristics. Additionally, the mixed analysis of covariance (ANCOVA) was used to calculate differences in clinical scores and images among subjects (p < 0.05, SPSS 20.0) after controlling for age, gender, and education. For image data analysis (voxel-wised ANCOVA), GM volume, age, and education were controlled as covariates of no interest, and the Gaussian random field (GRF) theory was used for multiple comparison correction (cluster level p < 0.05, voxel-level p < 0.001). Post hoc analysis was also performed with the Bonferroni correction to evaluate differences between the four groups (p < 0.05).
Thereafter, a partial correlation analysis was used to investigate relationships between the behavioral scores and the altered rCBF, functional activity, and connectivity in all the subjects, after controlling for age, gender, and education as nuisance covariates (p < 0.05/5 = 0.01).
Finally, an SVM model based on the machine learning approach was adopted to obtain an imaging biomarker for the classification of the ADS by integrating the altered rCBF, ALFF, and ReHo in specific regions. Briefly, we used an SVM package, which was built in MATLAB, the LIBSVM toolbox to get optimal classifiers and test the power of classification (Pirooznia and Deng, 2006). The mean values of PCC/PCUN that showed significant group differences in rCBF, ALFF, and ReHo were employed as input features, which were all based on voxel-wise measures. Due to our limited sample size, the leave-one-out cross-validation (LOOCV) was used to quantify the power of classification (Wee et al., 2011). At last, receiver operating characteristic (ROC) curves were utilized to assess the performance of the classifier using the results from the LOOCV data. The classification performance was manifested at the area under the ROC curve (AUC), and the larger the AUC, the better the performance. Detailed information can be found in the Supplementary Material.
Results
Demographic and Neuropsychological Tests
Table 1, Supplementary Table 1, and Supplementary Figure 1 show the main demographic and clinical scores of all the subjects. There were no significant differences in age, gender, and education, as well as HAMD and HIS scores (p > 0.05), among the subjects. However, there were obvious differences in the MMSE, ADL, and composite z-scores of each cognitive domain between AD and the other three groups. Post hoc analysis revealed that the AD groups showed the worst behavioral performance when compared with the other three groups. In addition, more importantly, the four groups showed significant differences with each other in the episodic memory scores which decreased with disease severity (i.e., HC > SCD > MCI > AD).
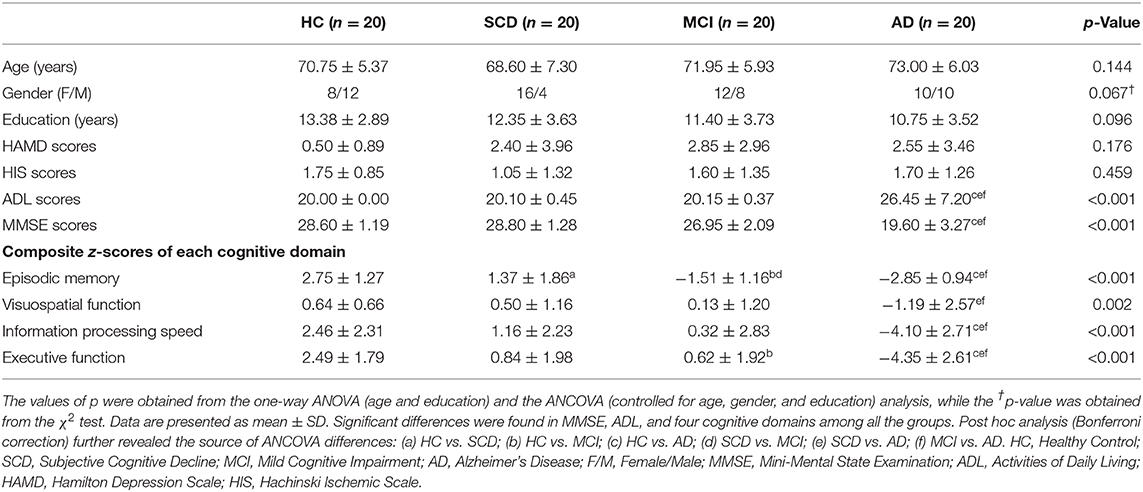
Table 1. Comparison of demographic, clinical characteristics, and cognitive function in all subjects.
Differences in Brain rCBF in the Four Groups
The voxel-wise ASL analysis showed that the altered rCBF was primarily located in the left PCUN (LPCUN) and the right middle frontal gyrus (MFG) in the four groups (Figure 1A). In addition, post hoc analysis indicated that patients with AD suffered the most severe CBF loss in both altered brain regions compared to the other three groups (Figure 1B). Figure 1C shows the correlations between the different brain rCBF regions and clinical tests in the SCD, MCI, and AD groups. The rCBF in the LPCUN showed a clear positive association with MMSE (R2 = 0.298, p < 0.001), episodic memory (R2 = 0.125, p = 0.006), information processing speed (R2 = 0.150, p = 0.002), and executive function (R2 = 0.266, p < 0.001). However, it was only related to MMSE (R2 = 0.160, p = 0.002) and episodic memory (R2 = 0.122, p = 0.006) (adjusted p-values were < 0.01) in the right MFG. Notably, the higher the neuropsychological scores, the higher the rCBF value was in these regions of the brain.
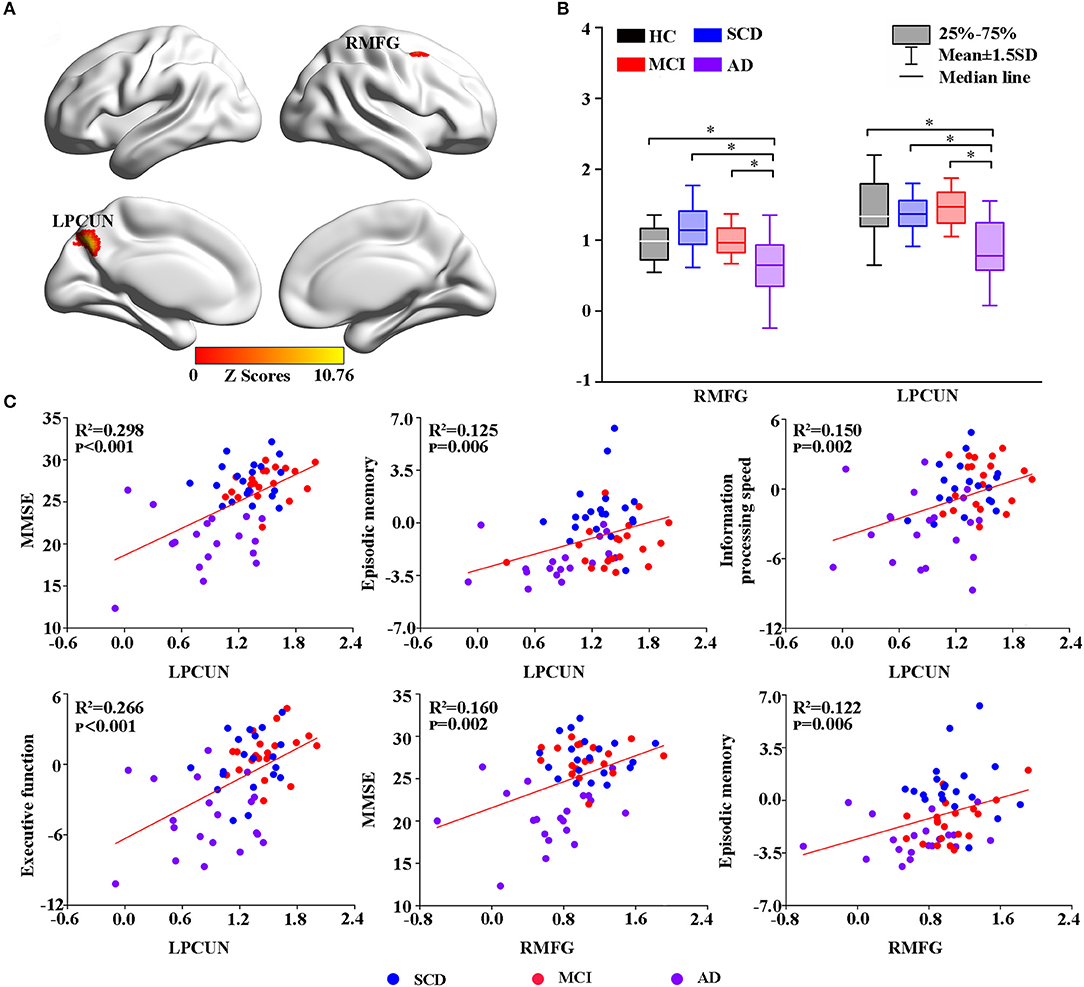
Figure 1. Group-level differences in rCBF among all subjects and behavioral significance. (A) The result revealed altered rCBF regions among the groups including LPCUN and RMFG (GRF-corrected, cluster level p < 0.05, voxel-level p < 0.001). The color bar represents the z-scores. (B) Post hoc analysis of the altered rCBF in the LPCUN and RMFG regions in all the groups (Bonferroni correction, p < 0.05). Box plots show the 25 percentile, the median, and the 75 percentile, and whisker plots are in mean ± SD. *Represents statistical difference (p < 0.05). (C) Significant correlations between the altered rCBF and cognitive performance in the SCD, MCI, and AD groups after controlling the effects of age, gender, education, and GM volumes as covariates of no interest (p < 0.01). AD, Alzheimer's Disease; MCI, Mild Cognitive Impairment; SCD, Subjective Cognitive Decline; HC, Healthy Control; MMSE, Mini-Mental State Examination; rCBF, Regional Cerebral Blood Flow; LPCUN, Left Precuneus; RMFG, Right Middle Frontal Gyrus; GM, Gray Matter; GRF, Gaussian Random Field.
Altered Brain Activities Among Groups
Thereafter, the study measured intrinsic brain activity in each region using ALFF and ReHo (Figures 2, 3). Significant changes in ALFF were shown in the bilateral PCC, LPCUN, and left paracentral lobule (PCL) as shown in Figure 2A. Additionally, ReHo was significantly altered in the bilateral PCUN, left inferior parietal (IPL), left middle temporal gyrus (MTG), and right superior occipital gyrus (SOG) among the groups (Figure 3A). Moreover, the groups with the disease showed decreased ALFF and ReHo compared to the HC group (Figures 2B, 3B). It is also worth noting that ReHo significantly increased in the MCI group in most of the altered brain regions except for the right PCUN (Figure 3B). Furthermore, partial correlation analysis revealed the relationship between brain activity and the neuropsychological scores (adjusted p-values were < 0.01) as shown in Figures 2C, 3C. The results revealed a negative correlation between the ALFF in the LPCL and MMSE (R2 = 0.121, p = 0.006), as indicated in Figure 2C. In contrast, ReHo was positively associated with MMSE (LPCUN: R2 = 0.229, p < 0.001; LMTG: R2 = 0.191, p = 0.001), information processing speed (LPCUN: R2 = 0.254, p < 0.001; LMTG: R2 = 0.147, p = 0.002), and executive function (LPCUN: R2 = 0.266, p < 0.001; LMTG: R2 = 0.185, p = 0.001) in the LPCUN and left MTG as shown in Figure 3C. Moreover, there was a significant positive correlation between the ReHo of the LPCUN and the episodic memory (R2 = 0.118, p = 0.007), as highlighted in Figure 3C.
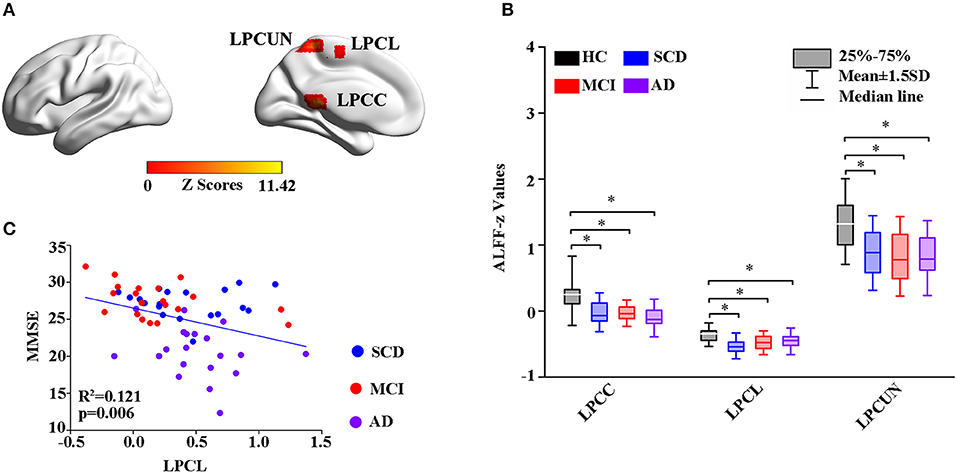
Figure 2. Group-level differences in ALFF among the subjects and behavioral significance. (A) The results showed the altered ALFF regions among the groups including LPCUN, LPCL, and LPCG (GRF-corrected, cluster level p < 0.05, voxel-level p < 0.001). Color bars represent the z-scores. (B) Post hoc analysis for the altered ALFF regions in all the groups (Bonferroni correction, p < 0.05). Box plots show the 25 percentile, the median, and the 75 percentile, and whisker plots are in mean ± SD. *Represents statistical difference (p < 0.05). (C) Significant correlations between the altered ALFF and neuropsychological tests in the SCD, MCI, and AD groups after controlling the effects of age, gender, education, and GM volumes as covariates of no interest (p < 0.01). AD, Alzheimer's Disease; MCI, Mild Cognitive Impairment; SCD, Subjective Cognitive Decline; HC, Healthy Control; MMSE, Mini-Mental State Examination; ALFF, Amplitude of Low-Frequency Fluctuation; LPCUN, Left Precuneus; LPCL, Left Paracentral Lobule; BPCG, Bilateral Posterior Cingulate Gyrus; GM, Gray Matter; GRF, Gaussian Random Field.
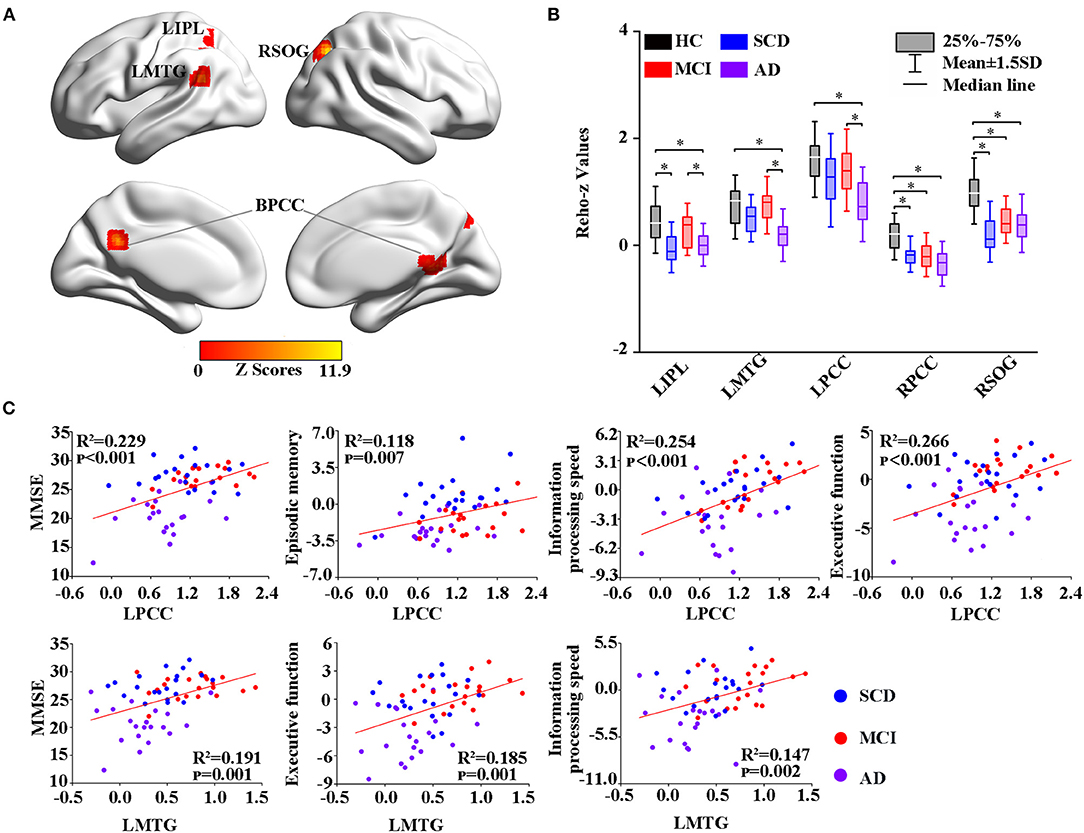
Figure 3. Group-level differences in ReHo among all the subjects and behavioral significance. (A) The results showed the altered ReHo regions among the groups, including BPCG, LIPL, LMTG, and RSOG (GRF-corrected, cluster level p < 0.05, voxel-level p < 0.001). (B) Post hoc analysis of the altered ReHo regions in all the groups (Bonferroni correction, p < 0.05). Box plots show the 25 percentile, the median, and the 75 percentile, and whisker plots are in mean ± SD. *Represents statistical difference (p < 0.05). (C) Significant correlations between the altered ReHo and neuropsychological tests in the SCD, MCI, and AD groups after controlling the effects of age, gender, education, and GM volumes as covariates of no interest (p < 0.01). AD, Alzheimer's Disease; MCI, Mild Cognitive Impairment; SCD, Subjective Cognitive Decline; HC, Healthy Control; MMSE, Mini-Mental State Examination; ReHo, Regional Homogeneity; BPCG, Bilateral Posterior Cingulate Gyrus; LIPL, Left Inferior Parietal; LMTG, Left middle Temporal gyrus; RSOG, Right Superior Occipital Gyrus; GM, Gray Matter; GRF, Gaussian Random Field.
Functional Connectivity Changes in the Resting State
Using the altered rCBF regions as ROIs, the study then performed a seed-based FC analysis (Figures 4, 5). The results showed significant differences in the FC of RMFG with bilateral SOG, right supramarginal gyrus (SMG), and right fusiform gyrus (RFFG) among the groups (Figure 4A). Notably, the bilateral SOG and right SMG showed decreased FC strength in MCI compared to SCD, while the maximum reduction was only observed in the AD group across all regions (Figure 4B). After adjusting the values of p, the partial correlation analysis also revealed that the FC strength of RMFG-RSOG significantly affected the visuospatial function in the disease groups (R2 = 0.109, p = 0.009) (adjusted p-values are < 0.01) as shown in Figure 4C.
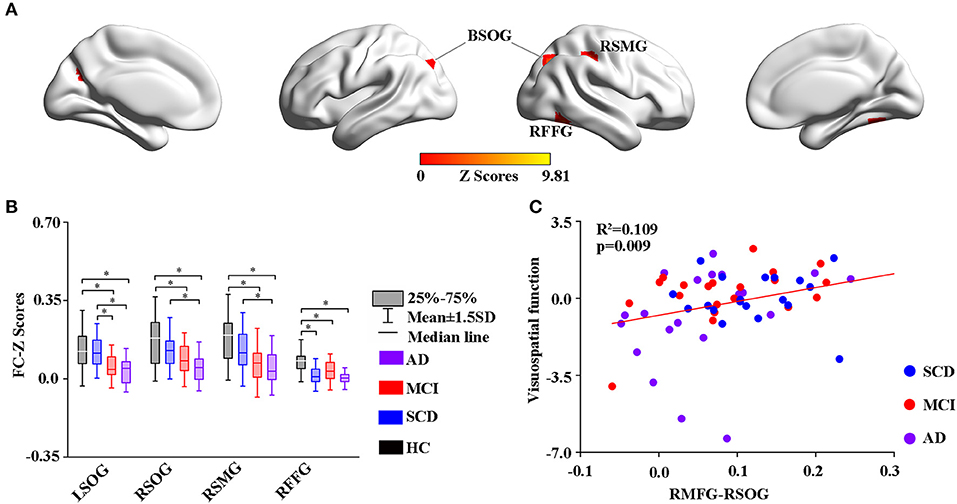
Figure 4. Group-level differences in RMFG-based functional connectivity among the subjects and behavioral significance. (A) The results showed the altered FC brain regions among the groups including BSOG, RITG, and RSMG (GRF-corrected, cluster level p < 0.05, voxel-level p < 0.001). (B) Post hoc analysis of the altered FC regions in all the groups (Bonferroni correction, p < 0.05). Box plots show the 25 percentile, the median, and the 75 percentile, whisker plots are in mean ± SD. *Indicates statistical difference (p < 0.05). (C) Significant correlations between the altered FC and cognitive performance in the SCD, MCI, and AD groups (p < 0.01) after controlling the effects of age, gender, education, and GM volumes as covariates of no interest (p < 0.01). AD, Alzheimer's Disease; MCI, Mild Cognitive Impairment; SCD, Subjective Cognitive Decline; HC, Healthy Control; FC, Function Connectivity; BSOG, Bilateral Superior Occipital Gyrus; RFFG, Right Fusiform Gyrus; RSMG, Right Supramarginal Gyrus; GM, Gray Matter; GRF, Gaussian Random Field.
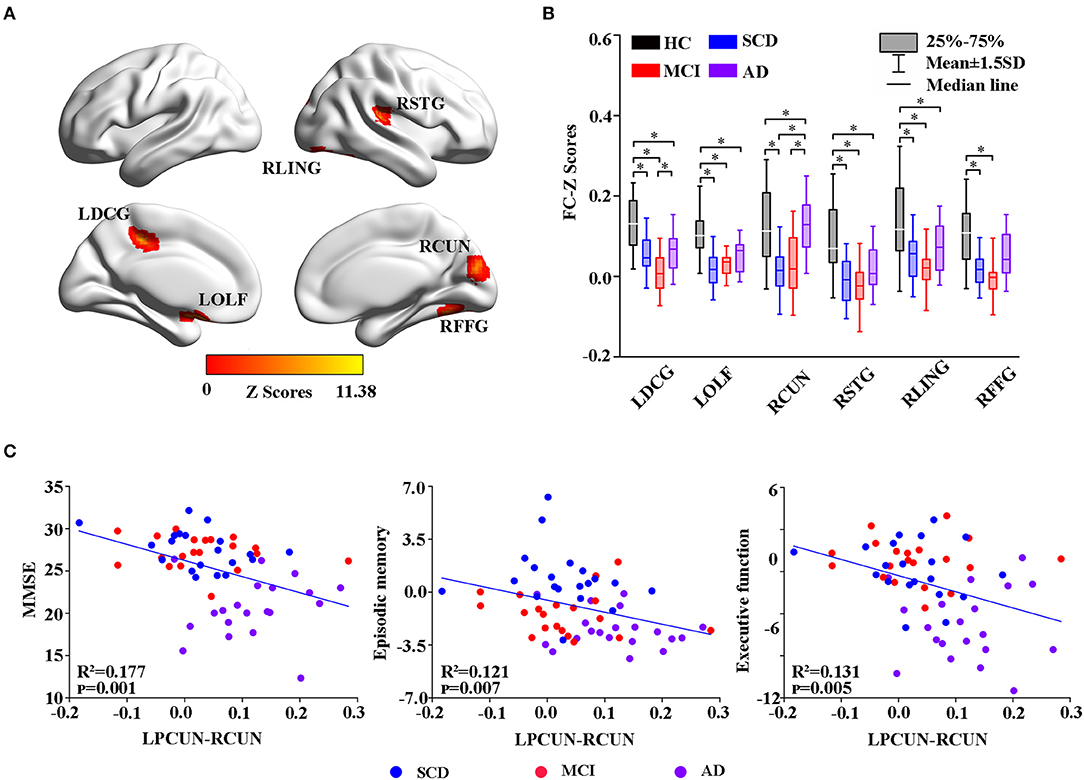
Figure 5. Group-level differences in the LPCUN-based functional connectivity across the subjects and behavioral significance. (A) The results showed the altered FC brain regions among the groups including LDCG, LOLF, RCUN, RSTG, RLING, and RFFG (GRF-corrected, cluster level p < 0.05, voxel-level p < 0.001). (B) Post hoc analysis of the altered FC regions in all the groups (Bonferroni correction, p < 0.05). Box plots show the 25 percentile, the median, and the 75 percentile, whisker plots are in mean ± SD. *Indicates statistical difference (p < 0.05). (C) Significant correlations between the altered FC and neuropsychological tests in the SCD, MCI, and AD groups after controlling the effects of age, gender, education, and GM volumes as covariates of no interest (p < 0.01). AD, Alzheimer's Disease; MCI, Mild Cognitive Impairment; SCD, Subjective Cognitive Decline; HC, Healthy Control; FC, Function Connectivity; LDCG, Left Median Cingulate and Paracingulate Gyri; LOLF, Left Olfactory Cortex; RCUN, Right Cuneus; RSTG, Right Superior Temporal Gyrus; RLING, Right Lingual Gyrus; RFFG, Right Fusiform Gyrus; GM, Gray Matter; GRF, Gaussian Random Field.
Moreover, group changes were widely observed between LPCUN and the left median cingulate, between paracingulate gyrus (DCG) and olfactory cortex (OLF), right cuneus (RCUN), superior temporal gyrus (STG), lingual gyrus (LING), and FFG (Figure 5A). Interestingly, although FC was significantly decreased in ADS compared to the HC group, patients with AD displayed an obvious increase in all the different regions compared to the SCD and MCI groups (Figure 5B). Furthermore, the partial correlation analysis revealed significant negative correlations between the FC strength of LPCUN-RCUN and MMSE (R2 = 0.177, p = 0.001), episodic memory (R2 = 0.121, p = 0.007), and executive function (R2 = 0.131, p = 0.005) (adjusted p-values are < 0.01) as shown in Figure 5C.
Analysis of the Altered rCBF, ALFF, and ReHo as Biomarkers in PCC/PCUN
Finally, the altered rCBF, ALFF, and ReHo in PCC/PCUN were used to conduct a ROC analysis. The results in Supplementary Figure 2 show that the study was able to differentiate all the disease groups from HC through the classification of altered ReHo (Supplementary Figure 2B) or ALFF (Supplementary Figure 2C) but not altered rCBF, which could only differentiate AD from HC (Supplementary Figure 2A). Within the disease groups, the altered rCBF (Supplementary Figure 2D) and ReHo (Supplementary Figure 2E) were satisfactory in their ability to classify AD and MCI, AD, and SCD but not MCI and SCD. However, the altered ALFF showed the worst performance with regard to classification (Supplementary Figure 2F). Nonetheless, a combination of altered rCBF, ALFF, and ReHo in PCC/PCUN showed a better differentiating ability across the AD spectrum (Figure 6). Therefore, using this classification, the AUC was 0.978 (95% confidence intervals from 0.942 to 1.000, p < 0.001), 0.958 (95% confidence intervals from 0.897 to 1, p < 0.001), and 0.915 (95% confidence intervals from 0.82 to 1, p < 0.001) in the distinction of AD, MCI, and SCD from HC, respectively (Figure 6A). Moreover, the AUC of the difference between MCI and AD was 0.933 (95% confidence intervals from 0.855 to 1, p < 0.001) while that of SCD and AD was 0.86 (95% confidence intervals from 0.744 to 0.977, p < 0.001). However, the combination displayed a poor ability to differentiate MCI from SCD (AUC value = 0.623, 95% confidence intervals from 0.445 to 0.8, p = 0.224) as shown in Figure 6B.
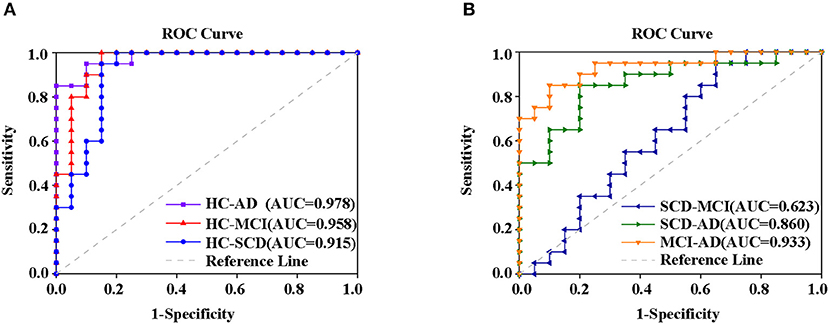
Figure 6. ROC curve from the support vector machine classifier and the general ROC model for classification of the AD spectrum. The results revealed that the combination of altered rCBF, ALFF, and ReHo in PCC/PCUN had more power in differentiating subjects with ADS from HC (A) MCI and SCD from patients with AD but not (B) SCD from MCI. The classification was able to differentiate disease groups from HC. Specifically, the AUC for the AD patients was 0.978 (95% confidence intervals from 0.942 to 1.000, p < 0.001), the MCI had 0.958 (95% confidence intervals from 0.897 to 1, p < 0.001), and SCD had 0.915 (95% confidence intervals from 0.82 to 1, p < 0.001) (A). Within disease groups, the combination showed a good ability to distinguish AD from MCI (AUC value = 0.933, 95% confidence intervals from 0.855 to 1, p < 0.001) as well as AD from SCD (AUC value = 0.86, 95% confidence intervals from 0.744 to 0.977, p < 0.001), but not MCI from SCD (AUC value = 0.623, 95% confidence intervals from 0.445 to 0.8, p = 0.224) (B). The blue line represents the HC and AD group, the red line represents the HC and MCI group, the purple line represents the HC and AD group, the orange line represents the AD and MCI group, the green line represents the AD and SCD group, the dark blue line represents the MCI and SCD group, and the gray line represents the reference. AD, Alzheimer's Disease; MCI, Mild Cognitive Impairment; SCD, Subjective Cognitive Decline; HC, Healthy Normal; ROC, Receiver Operating Characteristic, AUC, Area Under the Curve; ALFF, Amplitude of Low-Frequency Fluctuation; ReHo, Regional Homogeneity.
Discussion
This study aimed to explore the changing patterns in rCBF, brain function, and the behavioral significance across the ADS. First, the results showed that the rCBF in LPCUN and RMFG of the AD group decreased significantly compared to the other three groups. It also had a significant positive association with most cognitive tests except for visuospatial function. Second, the results revealed that the aberrant activity and function were mainly in the posterior brain regions. Compared to the HC group, the ALFF in LPCC/PCUN and the LPCL as well as ReHo in RPCC and RSOG showed an obvious decrease across the disease groups. In addition, ReHo in LMTG, LPCC, and LIPL of the MCI group was higher than that of the AD group. Furthermore, the partial correlation analysis revealed that there was a negative association between ALFF in the LPCL and the MMSE scores, while ReHo in LPCC and LMTG had a positive association with most of the behavioral tests. Additionally, it was shown that the identified regions of the brain had a significant dysfunction in FC and were closely related to cognitive performance. Finally, a combination of altered rCBF, ALFF, and ReHo in PCC/PCUN showed a better differentiating ability across the ADS.
Differences in rCBF in the ADS
In this study, there was a significant decrease in rCBF in the LPCUN and the RMFC of the AD group. In addition, rCBF was positively associated with cognitive function, consistent with the previous studies (Johnson et al., 2005; Alexopoulos et al., 2012; Hays et al., 2016; Kawagoe et al., 2019; Thomas et al., 2019; Duan et al., 2020). Additionally, a community-based cohort study showed that higher levels of rCBF were associated with better attention, executive function, and memory (Leeuwis et al., 2018). A previous study also showed that the lower the level of rCBF, the worse the cognitive performance was in patients with AD (Leeuwis et al., 2017). Notably, the DMN has two core regions, namely the MFG and the PCUN. The MFG is mainly related to attention, working memory, and regulation of emotions (Seminowicz and Moayedi, 2017), while PCUN is primarily involved in the retrieval of episodic memory, self-consciousness, and processing of the self-relevant effect (Zhang and Li, 2012). Moreover, necropsy revealed that MFG and PCUN were susceptible to Aβ deposition and hypoperfusion in the early stages of AD (Thomas et al., 2015; Miners et al., 2016). According to a previous report, a decrease in CBF starts from the PCUN and propagates along the PCC to other regions of the brain. More importantly, hypoperfusion in these regions showed no significant association with the distribution of brain atrophy in the early onset of familial AD (Benzinger et al., 2013). However, similar hypoperfusion was identified in the late-onset sporadic AD and showed the most pronounced decrease of CBF in PCUN and PCC, as well as in the prefrontal, parietal, and occipital cortices (Binnewijzend et al., 2013). Moreover, CBF in the prefrontal cortex was shown to be highly sensitive in the prediction of future cognitive performance (De Vis et al., 2018), while decreased CBF in the PCUN was considered to be a marker of severity in cognitive impairment (Binnewijzend et al., 2013). Intriguingly, aberrant local perfusion in the brain revealed that neurovascular dysfunction is commonly present in ADS. This also implied that the reduction in rCBF may be closely related to the progression of pathological processes in AD (Leeuwis et al., 2017), forming a vicious circle. Notably, decreased brain perfusion reduces the clearance of Aβ, leading to the accumulation of amyloid plaques and neurofibrillary tangles, which further impair vascular function and exacerbate the reduction of CBF (Popa-Wagner et al., 2015). As such, the altered rCBF may interfere with brain function and aggravate cognitive decline in the ADS.
Changes in Brain Activity and FC Among the Subjects
The study identified multiple areas of the brain with decreased activity and disrupted FC (Liu et al., 2008; Han et al., 2012; Zhang et al., 2012; Pan et al., 2017; Min et al., 2019). Additionally, two recent meta-analyses demonstrated that the decreased ALFF and ReHo in patients with MCI were primarily located in the BPCC/PCUN, bilateral frontal, left occipitotemporal cortex, and parietal lobule compared to HC (Pan et al., 2017; Zhen et al., 2018). In the present study, the findings showed that the altered regions were mainly located in the posterior areas of the brain, including the PCC/PCUN, LMTG, IPL, SOG, FFG, and LING. These constitute parts of the DMN, the executive control network (ECN), and the visual network (VN) (Pan et al., 2017; Zhen et al., 2018). Moreover, numerous studies reported on the interruption of the connectivity of DMN, ECN, and VN in MCI/AD (Bokde et al., 2006; Sorg et al., 2007; Brier et al., 2012; Wang et al., 2015; Joo et al., 2016; Eyler et al., 2019). It is also well-known that visual impairment is one of the most important clinical signs of AD and accounts for about 30% in MCI (Mapstone et al., 2003) and up to 50% in AD (Mendola et al., 1995). Additionally, a marked decrease in glucose metabolism was reported in the parietal and occipital cortices of patients with AD (Pietrini et al., 1996). Existing evidence suggests that visual impairment might arise from the abnormal connectivity of the VN and other regions of the brain (Bokde et al., 2006; Vannini et al., 2008; Zheng et al., 2019)w. However, it is important to note that both brain activity and FC significantly decreased as early as in SCD. This suggests that dysregulation of brain neuronal excitability appears before objective impairment upon formal testing and that might be a potential biomarker (Mattson and Arumugam, 2018; Si et al., 2020). Interestingly, the FC strength between LPCUN and the altered regions of the brain in the AD group showed an increasing trend compared to the SCD and MCI groups. Moreover, there was a significant negative correlation between the FC of LPCUN-RCUN and MMSE, episodic memory, and executive functioning. This may have been due to the slight global cognitive impairment in the preclinical stage of AD, which only manifested as decreased FC. However, the FC in the core hub of DMN led to a compensatory rise in order to maintain cognitive function during the progression of the disease (Qi et al., 2010). It is noteworthy that with continued amyloid deposition and substantial loss of neurons in the late stages of AD, DMN gradually falls out of the compensatory mode, leading to a severe decrease in FC (Tuovinen et al., 2016; Scherr et al., 2019). Furthermore, the results showed that the ALFF in LPCL had a negative association with the MMSE score. The PCL is located in the posterior ventral region of the inferior frontal gyrus (IFG), which is a crucial cortical node for the cognitive control in the circuits. Additionally, with the aggravation of cognitive impairment, the cortical motor regions in the circuits compensate for the damaged brain function by part activation (Zhang et al., 2020). In summary, the results suggested that there is a significant difference in brain activity and FC across the ADS, and the difference appears as early as in SCD. Moreover, the difference is closely correlated with cognitive performance and can be used as a potential imaging biomarker for monitoring disease progression in AD.
Altered rCBF, ALFF, and ReHo as Biomarkers in PCC/PCUN
Numerous studies have reported on the altered rCBF, the deposition of AD pathology biomarkers, and decreased brain function in the PCC/PCUN of AD (Benzinger et al., 2013; Aghakhanyan et al., 2018; Zhu et al., 2019). In addition, the rCBF was validated in a previous study as a diagnostic marker for AD but not for preclinical AD (Zheng et al., 2019). It is well-known that the machine learning method is widely employed for classification in clinical research and has been used for the prediction of AD and preclinical AD (Liu et al., 2013; Xu et al., 2018). Therefore, using SVM, the study performed an integrated analysis of altered rCBF, ALFF, and ReHo in PCC/PCUN as biomarkers to uncover the differentiating power across the AD spectrum. The results revealed that a combination of the three measured brain functional changes in PCC/PCUN has a better differentiating power across the ADS compared to each parameter. Notably, the combination was able to differentiate the disease groups from HC. Moreover, the combination displayed a good ability to distinguish between AD and MCI, AD and SCD, but not MCI and SCD in the disease groups. Therefore, the study demonstrated that a combination of multimodal neuroimaging in PCC/PCUN might be an effective biomarker for differentiating the ADS.
Limitations
Although the study supplemented and refined similar reports from the past, it had a number of limitations. First, there was no evidence of a biomarker for amyloid pathology and genetic data. Secondly, the study was a single-center cross-sectional study and might therefore have presented insufficient data. Thirdly, the sample size was relatively small and some analyses may have had insufficient power. Moving forward, more volunteers will be recruited to participate in the study. In addition, the study will refine the detection of pathological markers of AD and obtain genetic data. Finally, regular follow-up will be conducted in the future in order to further confirm the results.
Conclusion
This study demonstrated obvious changes in CBF, brain activity, and FC in the ADS, and these could appear in the early stages of the disease. In addition, a disrupted FC, decreased CBF, and brain activity were associated with more serious cognitive impairment, reflecting brain neurovascular dysfunction in ADS. Finally, the combination of altered rCBF, ALFF, and ReHo in PCC/PCUN proved to be a powerful tool in differentiating the ADS and is therefore a potential neuroimaging biomarker.
Data Availability Statement
The raw and processed data in this study cannot be shared at this time due to the ethics and the protection of privacy issues of the participants. And these data also forms part of an ongoing study.
Ethics Statement
The studies involving human participants were reviewed and approved by Research Ethics Committee of the Affiliated ZhongDa Hospital, Southeast University (Nanjing, China). The patients/participants provided their written informed consent to participate in this study.
Author Contributions
ZZ, ZW, and CX were responsible for coordinating the entire data collection process and checking the quality of data during the collection period. YZ, FZ, CT, SZ, and HS were responsible for data collection. QW, CH, and DF were responsible for cognitive function assessment and neurological examination. QZ made a draft of the manuscript. HF polished the manuscript and language revision. All authors read and approved the final manuscript.
Funding
This research was supported by the National Key Projects for Research and Development Program of China (2016YFC1305800, 2016YFC1305802, CX); the National Natural Science Foundation of China (81671256, 81871069, 81271204, CX); the Key Project for Research and Development Program of Jiangsu Province (BE2018741); the social science fund of Jiangsu Province (19GLB025); and the Nanjing International Joint Research and Development Project (201715013).
Conflict of Interest
The authors declare that the research was conducted in the absence of any commercial or financial relationships that could be construed as a potential conflict of interest.
Supplementary Material
The Supplementary Material for this article can be found online at: https://www.frontiersin.org/articles/10.3389/fnagi.2021.630382/full#supplementary-material
References
Aghakhanyan, G., Vergallo, A., Gennaro, M., Mazzarri, S., Guidoccio, F., Radicchi, C., et al. (2018). The precuneus–a witness for excessive abeta gathering in Alzheimer's disease pathology. Neurodegener. Dis. 18, 302–309. doi: 10.1159/000492945
Alexopoulos, P., Sorg, C., Forschler, A., Grimmer, T., Skokou, M., Wohlschlager, A., et al. (2012). Perfusion abnormalities in mild cognitive impairment and mild dementia in Alzheimer's disease measured by pulsed arterial spin labeling MRI. Eur. Arch. Psychiatry Clin. Neurosci. 262, 69–77. doi: 10.1007/s00406-011-0226-2
Arevalo-Rodriguez, I., Smailagic, N., Roque, I. F. M., Ciapponi, A., Sanchez-Perez, E., Giannakou, A., et al. (2015). Mini-Mental State Examination (MMSE) for the detection of Alzheimer's disease and other dementias in people with mild cognitive impairment (MCI). Cochrane Database Syst. Rev. 2015:D10783. doi: 10.1002/14651858.CD010783.pub2
Ashburner, J. (2007). A fast diffeomorphic image registration algorithm. Neuroimage 38, 95–113. doi: 10.1016/j.neuroimage.2007.07.007
Benzinger, T. L., Blazey, T., Jack, C. J., Koeppe, R. A., Su, Y., Xiong, C., et al. (2013). Regional variability of imaging biomarkers in autosomal dominant Alzheimer's disease. Proc. Natl. Acad. Sci. U.S.A. 110, E4502–E4509. doi: 10.1073/pnas.1317918110
Binnewijzend, M. A., Kuijer, J. P., Benedictus, M. R., van der Flier, W. M., Wink, A. M., Wattjes, M. P., et al. (2013). Cerebral blood flow measured with 3D pseudocontinuous arterial spin-labeling MR imaging in Alzheimer disease and mild cognitive impairment: a marker for disease severity. Radiology 267, 221–230. doi: 10.1148/radiol.12120928
Bokde, A. L., Lopez-Bayo, P., Meindl, T., Pechler, S., Born, C., Faltraco, F., et al. (2006). Functional connectivity of the fusiform gyrus during a face-matching task in subjects with mild cognitive impairment. Brain 129, 1113–1124. doi: 10.1093/brain/awl051
Brier, M. R., Thomas, J. B., Snyder, A. Z., Benzinger, T. L., Zhang, D., Raichle, M. E., et al. (2012). Loss of intranetwork and internetwork resting state functional connections with Alzheimer's disease progression. J. Neurosci. 32, 8890–8899. doi: 10.1523/JNEUROSCI.5698-11.2012
De Vis, J. B., Peng, S. L., Chen, X., Li, Y., Liu, P., Sur, S., et al. (2018). Arterial-spin-labeling (ASL) perfusion MRI predicts cognitive function in elderly individuals: a 4-year longitudinal study. J. Magn. Reson. Imaging 48, 449–458. doi: 10.1002/jmri.25938
Ding, B., Ling, H. W., Huang, J., Zhang, H., Wang, T., Yan, F. H., et al. (2014). Pattern of cerebral hyperperfusion in Alzheimer's disease and amnestic mild cognitive impairment using voxel-based analysis of 3D arterial spin-labeling imaging: initial experience. Clin. Interv. Aging 9, 493–500. doi: 10.2147/CIA.S58879
Dolui, S., Li, Z., Nasrallah, I. M., Detre, J. A., and Wolk, D. A. (2020). Arterial spin labeling versus 18F-FDG-PET to identify mild cognitive impairment. Neuroimage Clin. 25:102146. doi: 10.1016/j.nicl.2019.102146
Duan, W., Sehrawat, P., Balachandrasekaran, A., Bhumkar, A. B., Boraste, P. B., Becker, J. T., et al. (2020). Cerebral blood flow is associated with diagnostic class and cognitive decline in Alzheimer's disease. J. Alzheimers Dis. 76, 1103–1120. doi: 10.3233/JAD-200034
Dubois, B., Feldman, H. H., Jacova, C., Hampel, H., Molinuevo, J. L., Blennow, K., et al. (2014). Advancing research diagnostic criteria for Alzheimer's disease: the IWG-2 criteria. Lancet Neurol. 13, 614–629. doi: 10.1016/S1474-4422(14)70090-0
Dunn, C. J., Duffy, S. L., Hickie, I. B., Lagopoulos, J., Lewis, S. J. G., Naismith, S. L., et al. (2014). Deficits in episodic memory retrieval reveal impaired default mode network connectivity in amnestic mild cognitive impairment. Neuroimage Clin. 4, 473–480. doi: 10.1016/j.nicl.2014.02.010
Eyler, L. T., Elman, J. A., Hatton, S. N., Gough, S., Mischel, A. K., Hagler, D. J., et al. (2019). Resting state abnormalities of the default mode network in mild cognitive impairment: a systematic review and meta-analysis. J. Alzheimers Dis. 70, 107–120. doi: 10.3233/JAD-180847
Galvin, J. E., Price, J. L., Yan, Z., Morris, J. C., and Sheline, Y. I. (2011). Resting bold fMRI differentiates dementia with Lewy bodies vs Alzheimer disease. Neurology 76, 1797–1803. doi: 10.1212/WNL.0b013e31821ccc83
Habib, M., Mak, E., Gabel, S., Su, L., Williams, G., Waldman, A., et al. (2017). Functional neuroimaging findings in healthy middle-aged adults at risk of Alzheimer's disease. Ageing Res. Rev. 36, 88–104. doi: 10.1016/j.arr.2017.03.004
Han, Y., Lui, S., Kuang, W., Lang, Q., Zou, L., and Jia, J. (2012). Anatomical and functional deficits in patients with amnestic mild cognitive impairment. PLoS ONE 7:e28664. doi: 10.1371/journal.pone.0028664
Hays, C. C., Zlatar, Z. Z., and Wierenga, C. E. (2016). The utility of cerebral blood flow as a biomarker of preclinical Alzheimer's disease. Cell. Mol. Neurobiol. 36, 167–179. doi: 10.1007/s10571-015-0261-z
Johnson, N. A., Jahng, G. H., Weiner, M. W., Miller, B. L., Chui, H. C., Jagust, W. J., et al. (2005). Pattern of cerebral hypoperfusion in Alzheimer disease and mild cognitive impairment measured with arterial spin-labeling MR imaging: initial experience. Radiology 234, 851–859. doi: 10.1148/radiol.2343040197
Joo, S. H., Lim, H. K., and Lee, C. U. (2016). Three large-scale functional brain networks from resting-state functional MRI in subjects with different levels of cognitive impairment. Psychiatry Investig. 13, 1–7. doi: 10.4306/pi.2016.13.1.1
Kawagoe, T., Onoda, K., and Yamaguchi, S. (2019). Subjective memory complaints are associated with altered resting-state functional connectivity but not structural atrophy. Neuroimage Clin. 21:101675. doi: 10.1016/j.nicl.2019.101675
Khan, W., Amad, A., Giampietro, V., Werden, E., De Simoni, S., O'Muircheartaigh, J., et al. (2020). The heterogeneous functional architecture of the posteromedial cortex is associated with selective functional connectivity differences in Alzheimer's disease. Hum. Brain Mapp. 41, 1557–1572. doi: 10.1002/hbm.24894
Leeuwis, A. E., Benedictus, M. R., Kuijer, J., Binnewijzend, M., Hooghiemstra, A. M., Verfaillie, S., et al. (2017). Lower cerebral blood flow is associated with impairment in multiple cognitive domains in Alzheimer's disease. Alzheimers Dement. 13, 531–540. doi: 10.1016/j.jalz.2016.08.013
Leeuwis, A. E., Smith, L. A., Melbourne, A., Hughes, A. D., Richards, M., Prins, N. D., et al. (2018). Cerebral blood flow and cognitive functioning in a community-based, multi-ethnic cohort: the SABRE study. Front. Aging Neurosci. 10:279. doi: 10.3389/fnagi.2018.00279
Li, D., Liu, Y., Zeng, X., Xiong, Z., Yao, Y., Liang, D., et al. (2020). Quantitative study of the changes in cerebral blood flow and iron deposition during progression of Alzheimer's disease. J. Alzheimers Dis. 78, 439–452. doi: 10.3233/JAD-200843
Liu, X., Tosun, D., Weiner, M. W., and Schuff, N. (2013). Locally linear embedding (LLE) for MRI based Alzheimer's disease classification. Neuroimage 83, 148–157. doi: 10.1016/j.neuroimage.2013.06.033
Liu, Y., Wang, K., Yu, C., He, Y., Zhou, Y., Liang, M., et al. (2008). Regional homogeneity, functional connectivity and imaging markers of Alzheimer's disease: a review of resting-state fMRI studies. Neuropsychologia 46, 1648–1656. doi: 10.1016/j.neuropsychologia.2008.01.027
Ma, H. R., Pan, P. L., Sheng, L. Q., Dai, Z. Y., Wang, G. D., Luo, R., et al. (2017). Aberrant pattern of regional cerebral blood flow in Alzheimer's disease: a voxel-wise meta-analysis of arterial spin labeling MR imaging studies. Oncotarget 8, 93196–93208. doi: 10.18632/oncotarget.21475
Mapstone, M., Steffenella, T. M., and Duffy, C. J. (2003). A visuospatial variant of mild cognitive impairment: getting lost between aging and AD. Neurology 60, 802–808. doi: 10.1212/01.WNL.0000049471.76799.DE
Mattson, M. P., and Arumugam, T. V. (2018). Hallmarks of brain aging: adaptive and pathological modification by metabolic states. Cell Metab. 27, 1176–1199. doi: 10.1016/j.cmet.2018.05.011
McKhann, G. M., Knopman, D. S., Chertkow, H., Hyman, B. T., Jack, C. R. J., Kawas, C. H., et al. (2011). The diagnosis of dementia due to Alzheimer's disease: recommendations from the National Institute on Aging-Alzheimer's Association workgroups on diagnostic guidelines for Alzheimer's disease. Alzheimers Dement. 7, 263–269. doi: 10.1016/j.jalz.2011.03.005
Mendola, J. D., Cronin-Golomb, A., Corkin, S., and Growdon, J. H. (1995). Prevalence of visual deficits in Alzheimer's disease. Optom Vis. Sci. 72, 155–167. doi: 10.1097/00006324-199503000-00003
Min, J., Zhou, X. X., Zhou, F., Tan, Y., and Wang, W. D. (2019). A study on changes of the resting-state brain function network in patients with amnestic mild cognitive impairment. Braz. J. Med. Biol. Res. 52:e8244. doi: 10.1590/1414-431x20198244
Miners, J. S., Palmer, J. C., and Love, S. (2016). Pathophysiology of hypoperfusion of the precuneus in early Alzheimer's disease. Brain Pathol. 26, 533–541. doi: 10.1111/bpa.12331
Pan, P., Zhu, L., Yu, T., Shi, H., Zhang, B., Qin, R., et al. (2017). Aberrant spontaneous low-frequency brain activity in amnestic mild cognitive impairment: a meta-analysis of resting-state fMRI studies. Ageing Res. Rev. 35, 12–21. doi: 10.1016/j.arr.2016.12.001
Pietrini, P., Furey, M. L., Graff-Radford, N., Freo, U., Alexander, G. E., Grady, C. L., et al. (1996). Preferential metabolic involvement of visual cortical areas in a subtype of Alzheimer's disease: clinical implications. Am. J. Psychiatry 153, 1261–1268. doi: 10.1176/ajp.153.10.1261
Pirooznia, M., and Deng, Y. (2006). SVM classifier–a comprehensive java interface for support vector machine classification of microarray data. BMC Bioinformatics 7:S25. doi: 10.1186/1471-2105-7-S4-S25
Popa-Wagner, A., Buga, A. M., Popescu, B., and Muresanu, D. (2015). Vascular cognitive impairment, dementia, aging and energy demand. A vicious cycle. J. Neural Transm. (Vienna) 122, S47–S54. doi: 10.1007/s00702-013-1129-3
Qi, R., Luo, Y., Zhang, L., Weng, Y., Surento, W., Li, L., et al. (2020). Effects of COMT rs4680 and BDNF rs6265 polymorphisms on brain degree centrality in Han Chinese adults who lost their only child. Transl. Psychiatry 10:46. doi: 10.1038/s41398-020-0728-7
Qi, Z., Wu, X., Wang, Z., Zhang, N., Dong, H., Yao, L., et al. (2010). Impairment and compensation coexist in amnestic MCI default mode network. Neuroimage 50, 48–55. doi: 10.1016/j.neuroimage.2009.12.025
Riederer, I., Bohn, K. P., Preibisch, C., Wiedemann, E., Zimmer, C., Alexopoulos, P., et al. (2018). Alzheimer disease and mild cognitive impairment: integrated pulsed arterial spin-labeling MRI and (18)F-FDG PET. Radiology 288, 198–206. doi: 10.1148/radiol.2018170575
Scherr, M., Utz, L., Tahmasian, M., Pasquini, L., Grothe, M. J., Rauschecker, J. P., et al. (2019). Effective connectivity in the default mode network is distinctively disrupted in Alzheimer's disease-A simultaneous resting-state FDG-PET/fMRI study. Hum Brain Mapp. doi: 10.1002/hbm.24517. [Epub ahead of print].
Schroeter, M. L., Stein, T., Maslowski, N., and Neumann, J. (2009). Neural correlates of Alzheimer's disease and mild cognitive impairment: a systematic and quantitative meta-analysis involving 1351 patients. Neuroimage 47, 1196–1206. doi: 10.1016/j.neuroimage.2009.05.037
Seminowicz, D. A., and Moayedi, M. (2017). The dorsolateral prefrontal cortex in acute and chronic pain. J. Pain 18, 1027–1035. doi: 10.1016/j.jpain.2017.03.008
Shi, Y., Gu, L., Wang, Q., Gao, L., Zhu, J., Lu, X., et al. (2019). Platelet amyloid-β protein precursor (AβPP) ratio and phosphorylated tau as promising indicators for early Alzheimer's disease. J. Gerontol. A 75, 664–670. doi: 10.1093/gerona/glz005
Si, T., Xing, G., and Han, Y. (2020). Subjective cognitive decline and related cognitive deficits. Front. Neurol. 11:247. doi: 10.3389/fneur.2020.00247
Sierra-Marcos, A. (2017). Regional cerebral blood flow in mild cognitive impairment and Alzheimer's disease measured with arterial spin labeling magnetic resonance imaging. Int. J. Alzheimers Dis. 2017:5479597. doi: 10.1155/2017/5479597
Sorg, C., Riedl, V., Muhlau, M., Calhoun, V. D., Eichele, T., Laer, L., et al. (2007). Selective changes of resting-state networks in individuals at risk for Alzheimer's disease. Proc. Natl. Acad. Sci. U.S.A. 104, 18760–18765. doi: 10.1073/pnas.0708803104
Ten, K. M., Barkhof, F., Boccardi, M., Visser, P. J., Jack, C. J., Lovblad, K. O., et al. (2017). Clinical validity of medial temporal atrophy as a biomarker for Alzheimer's disease in the context of a structured 5-phase development framework. Neurobiol. Aging 52, 167–182. doi: 10.1016/j.neurobiolaging.2016.05.024
Thomas, B., Sheelakumari, R., Kannath, S., Sarma, S., and Menon, R. N. (2019). Regional cerebral blood flow in the posterior cingulate and precuneus and the entorhinal cortical atrophy score differentiate mild cognitive impairment and dementia due to Alzheimer disease. Am. J. Neuroradiol. 40, 1658–1664. doi: 10.3174/ajnr.P0057
Thomas, T., Miners, S., and Love, S. (2015). Post-mortem assessment of hypoperfusion of cerebral cortex in Alzheimer's disease and vascular dementia. Brain 138, 1059–1069. doi: 10.1093/brain/awv025
Trebeschi, S., Riederer, I., Preibisch, C., Bohn, K. P., Forster, S., Alexopoulos, P., et al. (2016). Diagnostic potential of pulsed arterial spin labeling in Alzheimer's disease. Front. Neurosci. 10:154. doi: 10.3389/fnins.2016.00154
Tuovinen, T., Rytty, R., Moilanen, V., Abou, E. A., Veijola, J., Remes, A. M., et al. (2016). The effect of gray matter ICA and coefficient of variation mapping of BOLD data on the detection of functional connectivity changes in Alzheimer's disease and bvFTD. Front. Hum. Neurosci. 10:680. doi: 10.3389/fnhum.2016.00680
Vannini, P., Lehmann, C., Dierks, T., Jann, K., Viitanen, M., Wahlund, L. O., et al. (2008). Failure to modulate neural response to increased task demand in mild Alzheimer's disease: fMRI study of visuospatial processing. Neurobiol. Dis. 31, 287–297. doi: 10.1016/j.nbd.2008.04.013
Waltz, J. A., Kasanova, Z., Ross, T. J., Salmeron, B. J., McMahon, R. P., Gold, J. M., et al. (2013). The roles of reward, default, and executive control networks in set-shifting impairments in schizophrenia. PLoS ONE 8:e57257. doi: 10.1371/journal.pone.0057257
Wang, Z., Wang, Y., Sweeney, J. A., Gong, Q., Lui, S., and Mosconi, M. W. (2019). Resting-state brain network dysfunctions associated with visuomotor impairments in autism spectrum disorder. Front. Integr. Neurosci. 13:17. doi: 10.3389/fnint.2019.00017
Wang, Z., Xia, M., Dai, Z., Liang, X., Song, H., He, Y., et al. (2015). Differentially disrupted functional connectivity of the subregions of the inferior parietal lobule in Alzheimer's disease. Brain Struct. Funct. 220, 745–762. doi: 10.1007/s00429-013-0681-9
Wee, C. Y., Yap, P. T., Li, W., Denny, K., Browndyke, J. N., Potter, G. G., et al. (2011). Enriched white matter connectivity networks for accurate identification of MCI patients. Neuroimage 54, 1812–1822. doi: 10.1016/j.neuroimage.2010.10.026
Xie, C., Bai, F., Yu, H., Shi, Y., Yuan, Y., Chen, G., et al. (2012). Abnormal insula functional network is associated with episodic memory decline in amnestic mild cognitive impairment. Neuroimage 63, 320–327. doi: 10.1016/j.neuroimage.2012.06.062
Xie, C., Bai, F., Yuan, B., Yu, H., Shi, Y., Yuan, Y., et al. (2015). Joint effects of gray matter atrophy and altered functional connectivity on cognitive deficits in amnestic mild cognitive impairment patients. Psychol. Med. 45, 1799–1810. doi: 10.1017/S0033291714002876
Xu, L., Liang, G., Liao, C., Chen, G. D., and Chang, C. C. (2018). An efficient classifier for Alzheimer's disease genes identification. Molecules 23:3140. doi: 10.3390/molecules23123140
Xue, C., Yuan, B., Yue, Y., Xu, J., Wang, S., Wu, M., et al. (2019). Distinct disruptive patterns of default mode subnetwork connectivity across the spectrum of preclinical Alzheimer's disease. Front. Aging Neurosci. 11:307. doi: 10.3389/fnagi.2019.00307
Yan, C., Wang, X., Zuo, X., and Zang, Y. (2016). DPABI: Data processing & analysis for (resting-state) brain imaging. Neuroinformatics 14, 339–351. doi: 10.1007/s12021-016-9299-4
Yan, T., Wang, W., Yang, L., Chen, K., Chen, R., and Han, Y. (2018). Rich club disturbances of the human connectome from subjective cognitive decline to Alzheimer's disease. Theranostics 8, 3237–3255. doi: 10.7150/thno.23772
Yoshiura, T., Hiwatashi, A., Noguchi, T., Yamashita, K., Ohyagi, Y., Monji, A., et al. (2009). Arterial spin labelling at 3-T MR imaging for detection of individuals with Alzheimer's disease. Eur Radiol 19, 2819–2825. doi: 10.1007/s00330-009-1511-6
Zhang, L., Li, W., Wang, L., Bai, T., Ji, G. J., Wang, K., et al. (2020). Altered functional connectivity of right inferior frontal gyrus subregions in bipolar disorder: a resting state fMRI study. J. Affect. Disord. 272, 58–65. doi: 10.1016/j.jad.2020.03.122
Zhang, S., and Li, C. S. (2012). Functional connectivity mapping of the human precuneus by resting state fMRI. Neuroimage 59, 3548–3562. doi: 10.1016/j.neuroimage.2011.11.023
Zhang, Z., Liu, Y., Jiang, T., Zhou, B., An, N., Dai, H., et al. (2012). Altered spontaneous activity in Alzheimer's disease and mild cognitive impairment revealed by regional homogeneity. Neuroimage 59, 1429–1440. doi: 10.1016/j.neuroimage.2011.08.049
Zhen, D., Xia, W., Yi, Z. Q., Zhao, P. W., Zhong, J. G., Shi, H. C., et al. (2018). Alterations of brain local functional connectivity in amnestic mild cognitive impairment. Transl. Neurodegener. 7:26. doi: 10.1186/s40035-018-0140-x
Zheng, W., Cui, B., Han, Y., Song, H., Li, K., He, Y., et al. (2019). Disrupted regional cerebral blood flow, functional activity and connectivity in Alzheimer's disease: a combined ASL perfusion and resting state fMRI study. Front. Neurosci. 13:738. doi: 10.3389/fnins.2019.00738
Keywords: Alzheimer's disease, arterial spin labeling, resting-state functional MRI, regional homogeneity, cerebral blood flow, amplitude of low frequency fluctuation
Citation: Zhang Q, Wang Q, He C, Fan D, Zhu Y, Zang F, Tan C, Zhang S, Shu H, Zhang Z, Feng H, Wang Z and Xie C (2021) Altered Regional Cerebral Blood Flow and Brain Function Across the Alzheimer's Disease Spectrum: A Potential Biomarker. Front. Aging Neurosci. 13:630382. doi: 10.3389/fnagi.2021.630382
Received: 17 November 2020; Accepted: 20 January 2021;
Published: 22 February 2021.
Edited by:
Rong Chen, University of Maryland, Baltimore, United StatesReviewed by:
Xun-Heng Wang, Hangzhou Dianzi University, ChinaCarlos Ayala Grosso, Instituto Venezolano de Investigaciones Científicas (IVIC), Venezuela
Feng Bai, Nanjing Drum Tower Hospital, China
Copyright © 2021 Zhang, Wang, He, Fan, Zhu, Zang, Tan, Zhang, Shu, Zhang, Feng, Wang and Xie. This is an open-access article distributed under the terms of the Creative Commons Attribution License (CC BY). The use, distribution or reproduction in other forums is permitted, provided the original author(s) and the copyright owner(s) are credited and that the original publication in this journal is cited, in accordance with accepted academic practice. No use, distribution or reproduction is permitted which does not comply with these terms.
*Correspondence: Chunming Xie, Y2hteGllQDE2My5jb20=; Zan Wang, dWpzX3lpbmd4aWFuZzA0MDFAMTI2LmNvbQ==