- Pharmacogenetics Laboratory, Institute of Biochemistry and Molecular Genetics, Faculty of Medicine, University of Ljubljana, Ljubljana, Slovenia
Alzheimer's disease (AD) is a complex neurodegenerative disease, affecting a significant part of the population. The majority of AD cases occur in the elderly with a typical age of onset of the disease above 65 years. AD presents a major burden for the healthcare system and since population is rapidly aging, the burden of the disease will increase in the future. However, no effective drug treatment for a full-blown disease has been developed to date. The genetic background of AD is extensively studied; numerous genome-wide association studies (GWAS) identified significant genes associated with increased risk of AD development. This review summarizes more than 100 risk loci. Many of them may serve as biomarkers of AD progression, even in the preclinical stage of the disease. Furthermore, we used GWAS data to identify key pathways of AD pathogenesis: cellular processes, metabolic processes, biological regulation, localization, transport, regulation of cellular processes, and neurological system processes. Gene clustering into molecular pathways can provide background for identification of novel molecular targets and may support the development of tailored and personalized treatment of AD.
Introduction
Alzheimer's disease (AD) is a progressive neurodegenerative disorder, affecting the cerebral cortex and hippocampus in human brain (Masters et al., 2015). The mechanisms of disease pathogenesis are still not entirely elucidated (Kocahan and Doğan, 2017). The accumulation of amyloid-β (Aβ) in form of insoluble plaques and aggregation of protein tau in neuronal neurofibrillary tangles (NFT) are considered as two important hallmarks of AD (Masters et al., 2015).
AD is the most common neurodegenerative brain disease and a significant part of worldwide population is affected. AD, as the leading cause of dementia, contributes to 60–65% of all cognitive decline cases (Rizzi et al., 2014). Reports suggest that roughly 47 million people suffered from dementia in 2015 (Prince, 2015). The mean incidence of AD is estimated to 1–3%, with a prevalence of 10–30% in population above 65 years of age (Kawas et al., 2000; 2020 Alzheimer's disease facts figures, 2020). As population is aging, the prevalence will increase, making dementia one of the most important health issues in the future. Projections suggest that more than 13 million people will suffer from AD in the United States alone and 11.8% of all people globally will be affected by the 2050 (Brookmeyer et al., 2007; Hebert et al., 2013).
A small proportion of AD cases show familial, highly inheritable form of AD, contributing to <1% of AD. Early age of onset is associated with this type of AD, that is also known as dominant inherited Alzheimer's disease (Masters et al., 2015). Furthermore, mutations in three common genes—amyloid precursor protein (APP), presenilin-1 (PSEN1), and presenilin-2 (PSEN2) are associated with early-onset AD (EOAD), developing in fourth or fifth decade of life (Mayeux and Stern, 2012; Naj and Schellenberg, 2017). However, not all EOAD cases can be explained with these mutations. Late-onset AD (LOAD) cases comprise the vast majority of all AD patients (>90%), with the typical age of onset above 65 years (Bekris et al., 2010). Complex genetic and environmental interactions have been associated with risk for sporadic LOAD (Miyashita et al., 2013; Masters et al., 2015). Studies suggest LOAD is not as strongly linked to familial background as EOAD, but genetic factors can contribute importantly to AD risk even late in life (Pedersen et al., 2004; Gatz et al., 2005). Contrary to EOAD, there are no highly penetrant mutations in a set of known genes; instead multiple low penetrance genetic variants can confer risk for LOAD (Naj and Schellenberg, 2017).
Although AD generally manifests in older population, first changes in biomarkers levels, such as Aβ42 and phosphorylated tau (ptau181) can be observed already 15–20 years prior to the onset of the clinical symptoms (Blennow et al., 2010; Efthymiou and Goate, 2017). Furthermore, functional and molecular imaging of brain with single-photon emission computed tomography and positron emission tomography (PET) provides valuable early information about the underlying pathological processes such as glucose metabolism, accumulation of tau and Aβ or neuroinflammation (Valotassiou et al., 2018). Biomarkers are usually used to inform and support the diagnostic of the disease when cognitive decline has already become apparent (Efthymiou and Goate, 2017). Using biomarkers for improved diagnostic in non-demented individuals could contribute to better understanding of neurodegenerative changes late in life and support development and implementation of novel therapeutic approaches.
Some asymptomatic changes that precede typical AD cognitive symptoms can be observed in patients before the clinical diagnosis. For instance, increased biomarkers levels in adults without symptoms of cognitive impairment are typical for preclinical AD, whereas the earliest symptomatic stage when cognitive symptoms are present, but not reaching the threshold for AD dementia diagnosis, is known as prodromal AD (Dubois et al., 2016). Another clinical stage associated with AD is mild cognitive impairment (MCI). MCI is a clinical stage of progressive cognitive impairment exceeding the expected cognitive decline for age and education status (Petersen et al., 1999; Lee et al., 2017). Since around 50% of MCI patients develop AD in 5 years from diagnosis, MCI is often considered as an intermediate stage between normal aging and AD (Petersen et al., 1999). Adults with diagnosed MCI show milder cognitive decline and higher degree of independence in functional status than patients with AD (Langa and Levine, 2014). There are several studies trying to detect or predict the conversion from MCI to AD (Davatzikos et al., 2011; Sun et al., 2017; Hojjati et al., 2018). Since therapeutic interventions are more efficient during the MCI or in early stage of AD, sensitive and reliable methods for identification of cognitive decline should be used in clinical practice (Olazaran et al., 2004; Cummings et al., 2007; Buschert et al., 2011).
Common genetic polymorphisms in genes that encode proteins involved in different biological pathways implicated in the pathogenesis of LOAD could influence its development and progression. This review summarizes the latest knowledge on genetics and genomics of AD susceptibility, compiled by GWASs and their meta-analyses. In addition we have performed gene clustering of the genomic loci and molecular pathways in development and progression of MCI and AD with the aim of facilitating identification of novel biomarkers or treatment targets.
Methods
A literature search was performed in NHGRI-EBI platform “GWAS catalog,” aiming to systematically gather vast dataset of genome-wide studies and meta-analysis of complex diseases (Buniello et al., 2019). A total of 96 GWAS and meta-analyses were included in the database until the end of December 2019. For each loci, identified by GWAS, a PubMed literature search was performed with the help of the following words: “Alzheimer's disease and gene name” or “Alzheimer's disease and polymorphisms and gene name.” Novel references, assessing the risk for the disease on the genome-wide level, were included in the review. Applied exclusion criteria were expression studies, studies not implementing case-control design, studies overlapping with other diseases and studies performed on a defined set of genes—not genome-wide design (Supplementary Figure 1). In total, GWAS (n = 54) and meta-analyses combining multiple GWAS dataset (n = 21) in AD risk evaluation were included (Supplementary Tables 1, 2). Nine studies combined GWAS and meta-analysis approach in identifying AD risk loci. Studies evaluating the association of GWAS and meta-analysis dataset with disease biomarkers (n = 16) were analyzed separately (Figure 1). Multiple studies (n = 13) combined identified genotype alterations in GWAS and meta-analyses with changes in AD-related biomarkers (Figure 1). A total of 105 AD risk loci were identified with additional 30 loci related to biomarker oscillations.
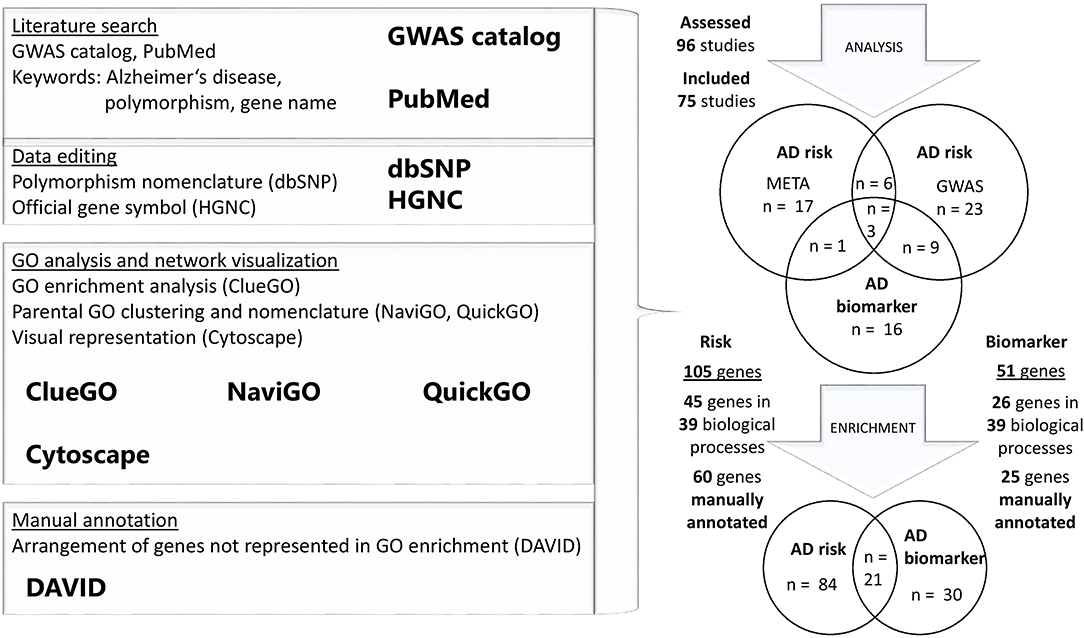
Figure 1. Flowchart of the study design and GO analysis. Literature search of GWAS and meta-analyses was performed in “GWAS catalog,” to obtain a list of AD-related genes. All of the studies were manually reviewed and some further literature search of identified gene loci was performed in PubMed. One hundred and five AD risk loci and 30 loci related to biomarker oscillations were used for GO enrichment analysis in two separate gene sets. Genes that were not enriched in performed GO analysis, were manually annotated to corresponding categories.
For the obtained gene loci, Gene Ontology (GO) enrichment analysis was performed, using Cytoscape plug-in ClueGO (Figure 1). This tool enables to find statistically overrepresented GO pathways in a set of genes and their visual representation in a functional network (Bindea et al., 2009). We focused on GO—biological process only. Analysis for AD risk and biomarker set of genes was performed separately (Supplementary Tables 3–6). Next, list of GO overrepresented pathways was visualized with NaviGO analytic tool, to find common GO parental pathways (Wei et al., 2017). Terms were manually curated with QuickGO web browser (Binns et al., 2009). Genes that did not reach significant threshold in GO analysis, were manually annotated in one of the identified categories, using DAVID functional annotation tool (Figure 1) (Huang et al., 2009).
Genes and Molecular Pathways Implicated in MCI and AD Risk
In our dataset of genes related to AD risk, we observed significant enrichment for four major GO biological process categories: cellular process, metabolic process, biological regulation, and localization (Figure 2).
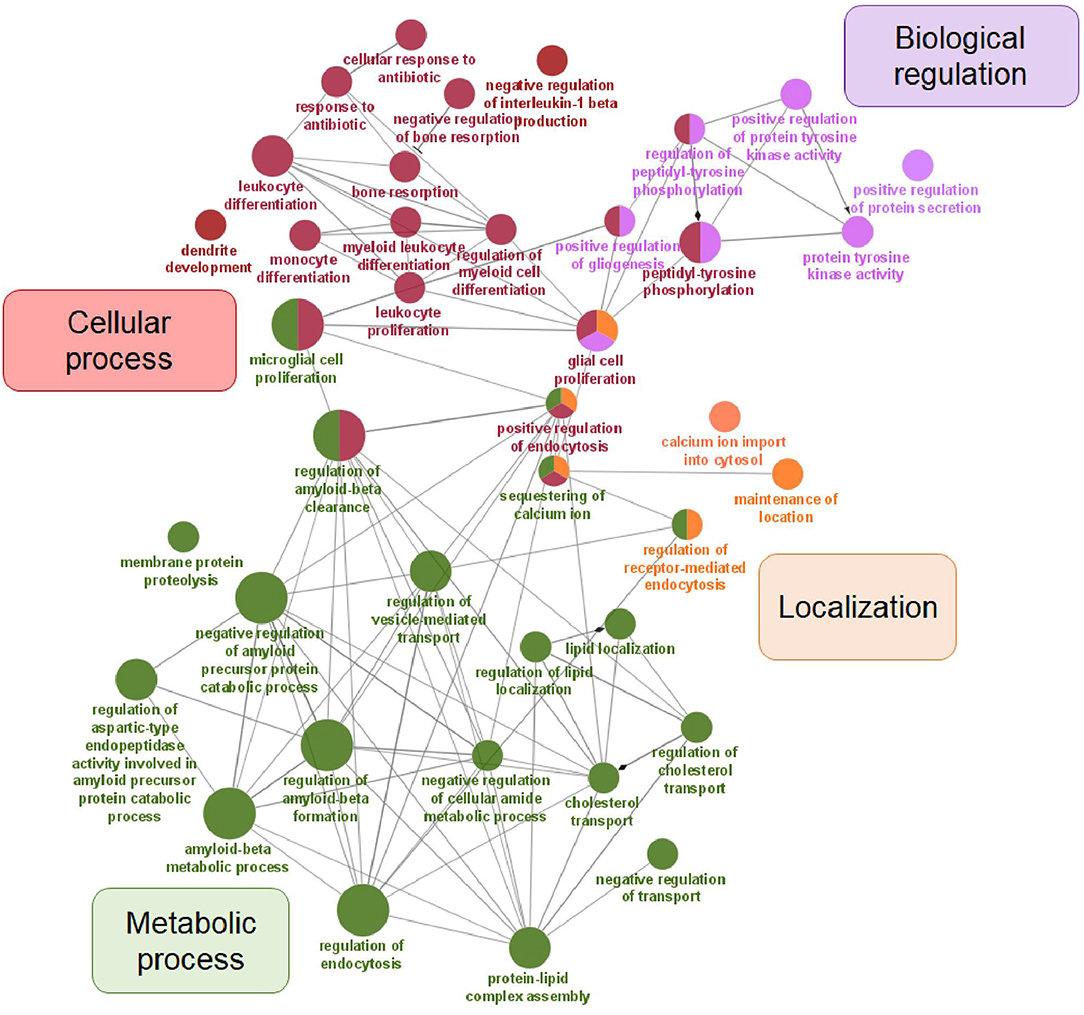
Figure 2. Visualization of GO analysis in AD risk gene set. Genes associated with AD risk were stratified according to GO – biological process. They are clustered in four parental categories and represented with specific color of the node. Biological processes that can be assigned to multiple parental categories, are represented with multiple color-pie chart.
Metabolic Processes
Since accumulation of insoluble proteins like Aβ is one of the hallmarks in neuropathology of AD, different metabolic processes are involved in their processing. Aβ is proteolytic product of APP cleavage by enzymes of the γ- and β-secretase (BACE) family that includes PS1 and PS2 (Masters et al., 2015). Studies of inherited form of EOAD suggest that mutations in APP, PSEN1, and PSEN2 genes result in overproduction of the hydrophobic Aβ40 and Aβ42 peptides, leading to aggregation and formation of insoluble plaques (Golde et al., 2000; Pimplikar, 2009; Masters et al., 2015). Normally, Aβ plaques are being degraded and cleared in processes driven by glial cells (Ries and Sastre, 2016). Insufficient clearance, due to the excessive aggregation of plaques, can affect surrounding synapses (Masters et al., 2015).
Several lines of evidence support the genetic basis of amyloid cascade hypothesis. Firstly, known mutations in APP, PSEN1, and PSEN2 genes associated with familial AD or EOAD affect the generation or aggregation propensity of Aβ (Heppner et al., 2015). Secondly, the APP gene is located on 21th chromosome and patients with Down's syndrome (the trisomy of the 21 chromosome) have increased risk for early development of memory impairment (García-Alba et al., 2019). Thirdly, apolipoprotein E (APOE) E4 allele (APOE4), which is associated with more extensive Aβ deposition is considered a major risk factor for LOAD (Amemori et al., 2015). It is estimated that 40–65% of AD patients have at least one copy of this allele (Namba and Ikeda, 1991; Olgiati et al., 2011). However, no successful therapies targeting amyloid accumulation have been implemented to date, suggesting the importance of other pathways that are also disrupted in AD (Efthymiou and Goate, 2017).
Genes and key SNPs included in metabolic processes, associated with AD risk in GWAS and meta-analyses, are summarized in Table 1.
Among all AD-related genetic risk factors, APOE on chromosome 19 is considered the strongest one and is also the most investigated in the literature. Two common APOE polymorphisms, rs429358 (p.Cys112Arg) and rs7412 (p.Arg158Cys) define polymorphic alleles APOE2, APOE3, and APOE4 that encode three respective protein variants: apoE2 (Cys112, Cys158), apoE3 (Cys112, Arg158), and apoE4 (Arg112, Arg158) (Zannis et al., 1982). Substitution of one or two amino acids affects the total charge and structure of APOE, leading to alteration in binding to cellular receptors and lipoprotein particles and possibly changing the stability and rate of production and clearance (Masters et al., 2015). Among all populations, APOE3 allele is the most frequent (50–90%), followed by APOE4 (5–35%) and APOE2 allele (1–5%) (Mahley and Rall, 2000). The association of APOE4 with increased AD risk and an earlier age of onset of AD was confirmed (Corder et al., 1993; Saunders et al., 1993). One or two copies of the APOE4 allele increases LOAD risk for 3- or 12-fold and contribute to ~50% LOAD (Ashford, 2004; Williams et al., 2020). Although APOE4 allele is widely considered as a major genetic risk factor for AD, it is neither necessary nor sufficient for the development of the disease (Meyer et al., 1998). On the other hand, a protective effect of APOE2 was shown (Corder et al., 1994). GWAS studies confirmed APOE rs429358 was associated with increased AD risk, while rs7412 was associated with decreased AD risk (Bertram et al., 2008; Shen et al., 2010; Beecham et al., 2014; Davies et al., 2014). Furthermore, APOE rs429358 showed increased risk for AD, while a protective role of APOE rs405509 was reported (Harold et al., 2009; Kunkle et al., 2019). Role of APOE in catabolism of triglyceride-rich lipoproteins is well-studied (Masters et al., 2015). APOE regulates their metabolism through binding to ApoE receptors, directing the transport, delivery, and distribution of lipoproteins (Mahley, 1988; Mahley and Rall, 2000). Discovery of APOE immunoreactivity in Aβ deposits and NFT, hallmarks of AD pathology, was an important research milestone in AD (Namba and Ikeda, 1991).
Besides APOE, a lot of other LOAD susceptibility loci involved in different metabolic processes have been reported to date (Table 1). Several genes play an important role in APP and tau processing, vesicle mediated transport or endocytosis. Multiple single nucleotide polymorphisms within and near phosphatidylinositol binding clathrin assembly protein (PICALM) gene were associated with AD. PICALM rs3851179 was associated with decreased AD risk (Seshadri et al., 2010; Kunkle et al., 2019). PICALM rs561655 showed decreased risk for LOAD and was subsequently associated with earlier age-of-onset of the disease (Naj et al., 2011, 2014). International Genomics of Alzheimer's disease project (IGAP) demonstrated an increased risk of AD associated with PICALM rs10792832 (Lambert et al., 2013b). Another polymorphism, PICALM rs867611, was confirmed as AD-related (Jansen et al., 2019). PICALM is an accessory protein in the endocytic pathway. It binds to clathrin and its adaptor proteins. Clathrin-mediated endocytosis is necessary for γ-secretase to cleave APP and form β-amyloid (Tanzi, 2012). Rs117618017 near APH1B, aph-1 homolog B, gamma-secretase subunit, coding for anterior pharynx defective-1 protein, another crucial part of γ-secretase complex important in APP cleaving, was also associated with AD risk (Acx et al., 2017; Jansen et al., 2019). BIN1 (bridging integrator 1) rs744373 SNP was associated with risk for LOAD (Seshadri et al., 2010). Naj et al. confirmed association of BIN1 rs7561528 with LOAD, while IGAP showed positive association for rs6733839 (Naj et al., 2011; Lambert et al., 2013b). Moreover, BIN1 rs6733839 was also associated with increased AD risk (Kunkle et al., 2019). Another BIN1 AD-related polymorphism was rs4663105 (Broce et al., 2019). BIN1 is a widely expressed adaptor protein that is part of the Bin1/amphiphysin/RVS167 (BAR) family. BIN1 functions in clathrin-mediated endocytosis and endocytic recycling (Wigge et al., 1997). It is also known as a tumor suppressor gene (Rosenthal and Kamboh, 2014). ADAM10 rs593742 was identified as a novel AD risk locus (Marioni et al., 2018). The protective function was observed in additional study (Kunkle et al., 2019). ADAM10 rs442495 was also associated with AD (Jansen et al., 2019). ADAM10 is as a member of ADAM family involved in the cleavage of APP in thereby influencing deposition of amyloid beta (Suh et al., 2013). Recent evidence indicated primary α-secretase function of ADAM10 in mouse models (Postina et al., 2004; Jorissen et al., 2010; Kuhn et al., 2010).
Various AD risk genes were associated with lipid metabolism. APOC1 rs4420638 was a strongly associated risk factor for AD (Coon et al., 2007). This association was confirmed in other studies (Webster et al., 2010; De Jager et al., 2012). APOC1 is involved in lipoprotein metabolism, but is interfering with fatty acids and reducing their intracellular esterification (Westerterp et al., 2007). Two ABCA7 SNP were associated with risk for LOAD (Hollingworth et al., 2011). Rs3752246 is the only coding non-synonymous missense SNP that may alter the function of ABCA7 protein in AD, while rs3764650 minor allele confers increased risk (Hollingworth et al., 2011; Pahnke et al., 2014; Kunkle et al., 2019). Another SNP, ABCA7 rs4147929, was associated with increased LOAD risk (Lambert et al., 2013b). A strong association of ABCA7 rs115550680 with increased LOAD risk was shown (Reitz et al., 2013). Furthermore, ABCA7 rs111278892 was recently associated with AD (Jansen et al., 2019). ABCA7 encodes an ATP-binding cassette transporter A7, which belongs to the A subfamily of ABC transporters (Hollingworth et al., 2011; Steinberg et al., 2015). Other than its role in cholesterol metabolism, recent data from mouse models suggest its role in the regulation of phagocytosis (Steinberg et al., 2015). It modulates the phagocytosis of apoptotic cells by macrophages mediated through the complement component C1q and it also participates in macrophage uptake of Aβ (Hollingworth et al., 2011; Rosenthal and Kamboh, 2014). ABCA7 is highly expressed in hippocampal CA1 neurons and microglia (Hollingworth et al., 2011; Rosenthal and Kamboh, 2014). A reduction in ABCA7 expression or loss of function could increase amyloid production and may contribute to AD susceptibility (Satoh et al., 2015). SLC10A2 rs16961023 showed a protective association with LOAD (Mez et al., 2017). Na+/bile acid cotransporter, encoded by SLC10A2, is a mediator in initial bile acid adsorption and is important for cholesterol homeostasis (Love et al., 2001). OSBPL6 rs1347297 was associated with LOAD (Herold et al., 2016). OSBPL6 is coding for oxysterol binding protein-like-6 receptor (Assou et al., 2013). Oxysterols are oxidized form of cholesterol that are able to cross the blood-brain-barrier (Testa et al., 2018). This process prevents excessive cholesterol accumulation in brain and may have an important role in AD pathogenesis.
The communication between different regions of the cell is mediated through dynamic networks of signaling cascades (Horbinski and Chu, 2005). This process is driven by enzymes like signaling kinases that alter the expression, activity or localization of proteins through phosphorylation mechanisms (Lash and Cummings, 2010). SPPL2A rs59685680 was associated with AD (Marioni et al., 2018). Signal peptide SPPL2A is part of aspartic intramembrane proteases, which cleave type II transmembrane proteins (Zhang et al., 2017). Interaction with immune system components, such as TNF, were previously reported (Friedmann et al., 2006). Three polymorphisms in FRMD4A—rs7081208, rs2446581, rs17314229—were associated with increased AD risk (Lambert et al., 2013a). FRMD4A is involved in Par protein binding and regulates epithelial cell polarity through cytohesins (Ikenouchi and Umeda, 2010). Par-related signaling pathway plays a crucial role in neuronal polarization (Insolera et al., 2011). A protective VSNL1 rs4038131 association with AD and psychosis was reported (Hollingworth et al., 2012). Calcium modulated VSNL1 utilizes a calcium-myristoyl switch phosphorylation, translocating the VSNL1 to cell membrane for induction of numerous cell signaling pathways (Braunewell and Szanto, 2009).
Several genes were associated with mRNA processing and transcriptional regulation. Rs10838725 in CELF1 region was associated with increased risk for AD in IGAP (Lambert et al., 2013b). Association with both AD and obesity was shown for CELF1 rs10838725 (Hinney et al., 2014). CELF1, also called CUG-BP, is a member of a family of proteins involved in the regulation of pre-mRNA alternative splicing (Gallo and Spickett, 2010). CRY2 rs12805422 was associated with AD and fasting glucose (Zhu et al., 2019). Flavin adenine dinucleotide-binding protein, encoded by CRY2 gene is important transcriptional repressor of circadian clock (Kriebs et al., 2017).
Rs10519262 near ATP8B4 was proposed as novel risk locus in AD (Li et al., 2008b). Implicated in energy metabolism, ATP8B4 is part of P4-ATPase flippase complex, potentially involved in ATP biosynthesis and phospholipid transport (Gao et al., 2016). RAB20 rs56378310 was linked to MCI-AD conversion (Lee et al., 2017). RAB20 is a member of GTPase family, involved in apical endocytosis, that negatively regulates neurite outgrowth (Oguchi et al., 2018).
Among all AD risk loci, obtained from GWAS and meta-analyses that were not enriched in GO analysis, additional 18 were manually annotated to a corresponding metabolic process and are also summarized in Table 1. ADAMTS4 rs4575098 was associated with AD (Jansen et al., 2019). A primary α-secretase function in APP processing was shown for ADAMTS4, Zn2+ metalloprotease with proteoglycan cleavage activity (Apte, 2009; Walter et al., 2019). Recently, ECHDC3 rs11257238 was associated with AD (Jansen et al., 2019). ECHDC3 (enoyl-CoA hydratase domain-containing protein 3) is a mitochondrial protein, important in fatty acid biosynthesis and possible insulin sensing mediator (Sharma et al., 2019). BDH1 rs2484 showed genome-wide significant association with conversion of MCI to AD (Lee et al., 2017). BDH1 (3-hydroxybutyrate dehydrogenase 1) is important as the initiator of β-hydroxybutyrate catabolism (Wang et al., 2019a). BCKDK rs889555 was associated with decreased AD risk (Marioni et al., 2018). BCKDK is a kinase, phosphorylating the enzyme complex of branched amino acid metabolism (Cook et al., 1984; Zigler et al., 2016). PCK1 rs8192708 was identified as AD risk allele (Grupe et al., 2007). PCK1—phosphoenolpyruvate carboxykinase 1—is a key enzyme in gluconeogenesis (Xia et al., 2010). It catalyzes decarboxylation and phosphorylation of oxaloacetate to phosphoenolpyruvate. CRYL1 rs7989332 interaction with another gene (KHDRBS2) was associated with AD (Gusareva et al., 2014). Crystallin, lambda 1 protein (CRYL1) is more known as a structural protein in lens, however it is also involved in dehydrogenation of L-gulonate in the uronate cycle, alternative pathway to metabolism of glucose (Huang et al., 2017b). ATP5MC2 rs1800634 was associated with LOAD (Meda et al., 2012). A subunit of mitochondrial ATP synthase, important for synthesis of ATP, is encoded by ATP5MC2 (Chen et al., 2006).
ADARB2 rs10903488 was associated with LOAD in MCI conversion patients (Lee et al., 2017). ADARB2 encodes a member of the double-stranded RNA adenosine deaminase family, important RNA-editing enzymes (Gentilini et al., 2017). CELF2 rs201119 was associated with AD driven neurodegeneration in APOE4 homozygotes (Wijsman et al., 2011). Besides CELF1, another member of CELF family is CELF2, implicated in several post-transcriptional events (Gallo and Spickett, 2010). KHDRBS2 rs6455128 interaction with CRYL1 was associated with AD (Gusareva et al., 2014). KHDRBS2 is involved in RNA splicing (Malouf et al., 2014). Interaction of rs9749589 with APOE4 status suggested NFIC as a novel protective locus in AD susceptibility (Jun et al., 2017). Transcriptional regulator NFIC is a member of Nuclear Factor-I (NF-I) family (Gronostajski, 2000). KAT8 rs59735493 showed a genome-wide significant association with AD (Jansen et al., 2019). KAT8 is histone acetyltransferase, part of MSL complex involved in acetylation of nucleosomal histone H4 (Smith et al., 2006; Yuan et al., 2012). An AD protective function of GALNT7 rs62341097 was observed (Beecham et al., 2014). GALNT is a member of N-acetylgalactosaminyltransferases, known for oncogenic role in cancer development (Hussain et al., 2016). It is involved in mucin-type O-glycosylation, post-translational modification, that stimulates intensive proliferation and metastasis of neoplastic cells (Kudryavtseva et al., 2019). A genome-wide association with MCI to AD conversion was observed in rs3936289 in the STG6AL1 region (Lee et al., 2017). ST6GAL1 is also involved in protein glycosylation. Interactions with BACE1 were investigated and an effect on APP secretion was shown (Kitazume et al., 2001; Nakagawa et al., 2006). Through this mode of action, BACE1 is also directly linked to synaptic function (Das and Yan, 2017). FBXL7 rs75002042 was associated with decreased LOAD risk (Tosto et al., 2015). FBXL7 is one of the F-box proteins, important subunits of E3 ubiquitin protein ligases, enzymes involved in phosphorylation-dependent ubiquitination of proteins (Rodrigues-Campos and Thompson, 2014). SPSB1 rs11121365 was associated with AD (Lee et al., 2017). SPSB1 is another regulator of ubiquitination and proteasomal degradation of NO synthase, important in AD (Nishiya et al., 2011). ALPK2 rs76726049 was reported as novel AD risk locus (Jansen et al., 2019). Protein alpha-kinase 2, encoded by ALPK2 is a serine/threonine kinase, previously associated with leukemia progression (Smirnikhina et al., 2016). GLIS3 rs514716 protective function in AD was reported (Deming et al., 2017). Involved in gene transcription, GLIS3 is a component of Krüppel-like zinc finger transcriptional regulators (Calderari et al., 2018). Through Glis3-binding sites (G3BS), target gene transcription is regulated (Kim et al., 2003).
Cellular Processes
Genes from different levels of cellular process are also highly enriched in AD pathology. Comprehensive list of genes and key SNPs, involved in cellular processes, associated with AD risk in GWAS and their meta-analyses, are presented in Table 2.
Numerous SNPs in clusterin (CLU) were linked to AD. Rs11136000 was associated with decreased risk for AD (Harold et al., 2009; Lambert et al., 2009; Seshadri et al., 2010). Furthermore, CLU rs1532278 and rs9331896 were associated with decreased LOAD risk (Naj et al., 2011; Lambert et al., 2013b; Kunkle et al., 2019). Two other polymorphisms in CLU were associated with decreased (rs2279590) or increased (rs9331888) risk for AD (Lambert et al., 2009). Another novel SNP in this region, rs4236673, was also associated with AD risk (Jansen et al., 2019). CLU is a chaperone molecule that may be involved in membrane recycling and apoptosis. It interacts with soluble form of Aβ, forming complexes that cross the blood-brain barrier (Olgiati et al., 2011). It is one of the primary chaperones for removal of Aβ from the brain (Rosenthal and Kamboh, 2014). Association of PTK2B rs28834970 with increased AD risk was observed in IGAP (Lambert et al., 2013b). In another study, the same effect was observed for PTK2B rs73223431 (Kunkle et al., 2019). PTK2B is protein-tyrosine kinase, involved in multiple cellular processes. Importance of mouse homolog in Aβ signaling and therefore a potential risk for AD was proposed (Salazar et al., 2019). CLDN18 rs16847609 was associated with increased AD risk (Jun et al., 2016). Although not expressed in nervous tissue, claudins are protein components of epithelial and endothelial tight junctions of multiple tissues, regulating cell permeability and maintaining polarity (Luo et al., 2018). Two SNPs in TP53INP1 (rs4734295, rs6982393) were associated with AD and type 2 diabetes (T2D), indicating potential shared molecular pathways between the diseases (Wang et al., 2017). TP53INP1 encodes a protein, involved in apoptosis and regulating cellular-extracellular matrix adhesion and cell migration (Seux et al., 2011). Mez et al. reported COBL rs112404845 as a novel protective locus for AD (Mez et al., 2017). COBL, a recently discovered protein, plays a role in cellular morphogenesis by regulating cytoskeletal dynamics (Ahuja et al., 2007; Hou et al., 2015). In neurons, COBL-induced actin nucleation plays a crucial role in neuritogenesis and dendritic branching (Ahuja et al., 2007).
SLC9A7 rs1883255 was associated with LOAD (Meda et al., 2012). SLC9A7 encodes for (Na+, K+)/proton (H+) exchanger in the Golgi, important in maintenance of homeostasis (Numata and Orlowski, 2001). Polymorphism rs8070572 in MINK1 region showed higher risk for AD (Broce et al., 2019). MINK1 encodes a serine-threonine kinase, involved in different cell processes, including dendrite development (Yu et al., 2020). A novel significant association with both AD and T2D in PLEKHA1 rs2421016 was observed (Wang et al., 2017). Pleckstrin homology domain-containing family A member 1 protein is another mediator in cellular signaling, encoded by PLEKHA1 (Huang and Xiang, 2018). In MS4A region, MS4A2 rs7933202 was associated with AD (Kunkle et al., 2019). MS4A (membrane spanning 4A) cluster encodes a family of cell surface proteins that participate in the regulation of calcium signaling (Hollingworth et al., 2011; Ma et al., 2015). Their function in neurodegeneration and AD has been discussed lately (LaFerla, 2002; Marambaud et al., 2009; Hermes et al., 2010; Seaton et al., 2011; Zündorf and Reiser, 2011).
There is growing evidence that immune system is involved in the early stages of AD pathogenesis. Immune processes may drive AD pathology independently of Aβ deposition and thus sustain increased Aβ levels (Heppner et al., 2015). Immune system processes are characterized by the activation of glial cells and release of pro-inflammatory cytokines and chemokines (Liu and Chan, 2014). Recently, a rare variant with comparable effects to those of APOE4 was identified in TREM2 association study (Rosenthal et al., 2015). TREM2 rs75932628 results in the substitution of a histidine for arginine at amino acid residue 47 (p.His47Arg) and was shown to considerably increase AD risk (Jonsson et al., 2013). Interestingly, this variant was associated with a significantly younger age at symptom onset compared to individuals with no TREM2 variants (Slattery et al., 2014). The positive association of TREM2 rs75932628 with LOAD was also replicated (Kunkle et al., 2019). TREM2 rs143332484 conferred greater AD risk, while TREM2 rs187370608 was significantly associated with AD (Sims et al., 2017; Jansen et al., 2019). TREM2 is a surface receptor in the plasma membrane of brain microglia, forming an immune-signaling complex with DAP12 (Sims et al., 2017). It has an important function in innate immunity and also anti-inflammatory properties (Yaghmoor et al., 2014). It is also involved in the clearance of neural debris from CNS in phagocytosis mediated mechanism, leading to the production of reactive oxygen species (Neumann and Daly, 2013). In the human brain, TREM2 is found at high concentrations in white matter, the hippocampus and the neocortex, but at very low concentrations in the cerebellum. These regions are consistent with the distribution of pathology in AD (Yaghmoor et al., 2014). IL34 rs4985556 was recently recognized as a susceptibility locus (Marioni et al., 2018). IL34 is a homodimeric cytokine, stimulating proliferation of monocytes and macrophages through the colony-stimulating factor 1 receptor (CSF1R) (Lin et al., 2008). The effect of IL34 on microglia in AD pathogenesis was shown (Mizuno et al., 2011). Recent GWASs have shown that CR1 genetic variations are associated with global cognitive decline and higher burden of AD brain pathology. Association between CR1 rs6656401 and increased AD risk was observed (Lambert et al., 2009). The effect was later confirmed in IGAP study (Lambert et al., 2013b). Naj et al. confirmed the association between CR1 rs6701713 and LOAD risk and later showed that rs6701713 was also associated with age-at-onset (Naj et al., 2011, 2014). Additionally, CR1 rs2093760 was associated with AD and rs4844610 with increased LOAD risk (Jansen et al., 2019; Kunkle et al., 2019). Complement component receptor (CR1) is a receptor for complement fragments C3b and C4b (Madeo and Frieri, 2013) and it regulates complement cascade via the inhibition of both classical and alternative pathway C3 and C5 convertases (Zhu et al., 2015). Complement inhibition can reduce the clearance of Aβ in animal models (Wyss-Coray et al., 2002). Increased LOAD risk for INPP5D rs35349669 was identified in IGAP and confirmed in a follow-up study (Lambert et al., 2013b; Ruiz et al., 2014). The genome-wide significant association of INPP5D rs10933431 was observed, suggesting protective function (Jansen et al., 2019; Kunkle et al., 2019). INPP5D (Inositol Polyphospate-5 Phosphatase) regulates cytokine signaling and inhibition of PI3K-driven oncogenic pathway. It controls degradation of IgE receptor complex together with CD2AP (Rosenthal and Kamboh, 2014).
MEF2C rs190982 polymorphism was associated with decreased LOAD in IGAP (Lambert et al., 2013b). MEF2C is an important transcription factor, involved in the control of inflammation in vascular endothelial cells, inhibition of leukocyte transport, regulation of NF-κB activity and expression of pro-inflammatory genes (Xu et al., 2015). SERPINB1 rs316341 was identified as novel risk locus (Deming et al., 2017). Serpins are a family of protease inhibitors. Their potential role in inhibition of Aβ toxicity has been proposed, potentially through regulation of neutrophil infiltration in immune system (Schubert, 1997; Farley et al., 2012). The protective function of SPI1 rs1057233 and rs3740688 in AD development was proposed (Huang et al., 2017a; Kunkle et al., 2019). SPI1 is another important gene, involved in immune system processes. It encodes PU.1, a transcription factor essential for myeloid and B-lymphoid cell development and a major regulator of cellular communication in the immune system (Huang et al., 2017a; Broce et al., 2019).
Additional 23 of the risk loci, obtained from GWAS and meta-analyses that were not enriched in GO analysis, were manually annotated to cellular processes and are also summarized in Table 2.
Two important CDK5RAP2 SNPs were identified; rs10984186 was associated with an increased risk of developing AD while rs4837766 with opposite effect in MCI/AD risk and conversion rate was specific for women only (Miron et al., 2018). The cyclin-dependent kinase 5 regulatory subunit–associated protein 2 (CDK5RAP2) regulates cyclin-dependent kinase 5 (CDK5), important for tau phosphorylation and NFT formation in CNS (Arioka et al., 1993; Patrick et al., 1999). Tau protein is another important pathophysiological hallmark of AD. Under normal conditions tau binding stabilizes microtubules in axons, however, specific pathological conditions induce tau hyperphosphorylation (Di Paolo and Kim, 2011). Besides abnormal phosphorylation, tau protein aggregation in neurons can be induced, but the mechanism is not yet known (Jouanne et al., 2017). The formation of NFT due to tau aggregation can damage the neurons. The association between NFT and progression of AD has been widely studied; concentration and distribution of tangles correlated with severity and duration of dementia (Bierer et al., 1995; Gomez-Isla et al., 1997; Giannakopoulos et al., 2003).
PFDN1 rs11168036 was associated with increased LOAD risk (Jun et al., 2017). PFDN1 is encoding for chaperone protein that binds specifically to cytosolic chaperonin and transfers target proteins to it (Wang et al., 2015). Rs2405940 in SHROOM2 region was associated with LOAD (Meda et al., 2012). SHROOM2 encodes for a SHROOM family protein, an actin binding protein (Dietz et al., 2006). NME8 rs2718058 was reported as a protective locus for AD (Lambert et al., 2013b). NME/NM23 family member 8 (NME8), is involved in numerous physiological and pathological processes, including cellular differentiation (Desvignes et al., 2010). A role of NME8 in the cytoskeletal function, axonal transport and antioxidant action has also been discussed (Liu et al., 2016). CDC42SE2 rs382216 showed decreased risk for AD (Jun et al., 2016). CDC42SE2 is another potential actin cytoskeleton modulator, acting downstream of CDC42 (Pirone et al., 2000). Association of FERMT2 rs17125944 with increased AD susceptibility was observed in IGAP and confirmed in a follow-up study (Lambert et al., 2013b; Ruiz et al., 2014). Additionally, FERMT2 rs17125924 was associated with increased AD risk (Kunkle et al., 2019). FERMT2, also known as KIND2, is a gene encoding proteins from kindlin family (Lai-Cheong et al., 2010). Kindlin-2 is an integrin-interacting protein, mediating activation of integrin and cell-extracellular matrix interactions (Lai-Cheong et al., 2010; Wei et al., 2014). Carrasquillo et al. showed strong association of PCDH11X rs5984894 with LOAD susceptibility (Carrasquillo et al., 2009). PCDH11X belongs to the protocadherin gene subfamily of the cadherin superfamily of cell surface receptor molecules. The cadherins mediate cell-cell adhesion and play a role in cell signaling that is critical in the development of the central nervous system (CNS). Rs1466662 within this DCHS2 was associated with AD (Kamboh et al., 2012a). Protocadherin-23 is another protein from the cadherin superfamily that is expressed in the cerebral cortex and is encoded by DCHS2 (Höng et al., 2004).
SHE rs4474240 was associated with increased LOAD risk (Haddick et al., 2017). Src homology 2 (SH2) domains in SHE are phosphotyrosine binding motifs important in protein-protein interactions in various signaling pathways (Oda et al., 1997). SORCS1 was identified as potential AD risk locus, but rs2245123 did not show genome-wide significant association (Laumet et al., 2010). SORCS1 is involved in insulin signaling and APP processing (Olgiati et al., 2011). The association of rs7225151 in SCIMP region with increased risk for AD was proposed (Moreno-Grau et al., 2019). Another SCIMP polymorphism, rs113260531, was recently associated with AD (Jansen et al., 2019). A Src-kinase family mediator SCIMP is palmitoylated transmembrane adaptor, important for immune cell signaling (Draber et al., 2011). PTPRG rs7609954 was associated with AD risk (Herold et al., 2016). A member of heterogeneous protein tyrosine phosphatase (PTP) family, PTPRG is a type γ receptor, involved in cell growth, differentiation, mitotic cycle and other processes (Tonks, 2006; Herold et al., 2016). Two novel risk variants for AD were reported for ABI3 rs616338 and rs28394864 (Sims et al., 2017; Jansen et al., 2019). ABI3 belongs to ABL-interactor (ABI) family proteins, binding partners for the ABL kinases (Moraes et al., 2017). Their activation induces cell growth, transformation, and cytoskeletal organization (Satoh et al., 2017). Rs802571 in CNTNAP2 region was proposed as a novel AD-related protective locus (Hirano et al., 2015). Furthermore, rs114360492 was associated with AD (Jansen et al., 2019). CNTNAP2 encodes a contactin-associated protein-like 2 transmembrane neurexin, functioning as cell adhesion molecules and receptors in nervous system (Hirano et al., 2015; Saint-Martin et al., 2018). GOLM1 rs10868366 and rs7019241 showed decreased risk for AD (Li et al., 2008b). GOLM1 is a type II Golgi membrane glycoprotein (Wei et al., 2008). The potential function of GOLM1 in Ras signaling in adenocarcinoma has been recently proposed (Duan et al., 2018). OR4S1 rs1483121 was associated with AD risk (Zhu et al., 2019). OR4S1 is one of olfactory receptor proteins, G-protein-coupled receptors which represent the largest gene family in the human genome (Milardi et al., 2018). NYAP1 rs12539172 was associated with decreased LOAD risk (Kunkle et al., 2019). Neuronal tyrosine-phosphorylated phosphoinositide-3-kinase adapter 1 (NYAP1) is involved in the activation of PI3K and the recruitment of the nearby WAVE complex, that regulates brain size and neurite outgrowth in mice (Yokoyama et al., 2011). TSPOAP1-AS1 rs2632516 and rs2526378 were associated with decreased AD risk (Jun et al., 2017; Witoelar et al., 2018). Benzodiazapine receptor associated protein 1 (TSPOAP1), is another adaptor molecule interacting with Ca2+ channels to regulate synaptic transmission (Wang et al., 2000b).
TREML2 rs9381040 was associated with AD (Marioni et al., 2018). In contrast to TREM2, TREML2 does not interact with DAP12 (Zheng et al., 2016), but it is important in inflammation as a single-pass type I membrane protein expressing an Ig-like V-type domain (Klesney-Tait et al., 2006). HLA-DRB1 rs9271058 was identified as risk factor (Kunkle et al., 2019). Rs9271192 near HLA-DRB1 was associated with increased AD risk in IGAP, while intergenic rs6931277 was associated with AD progression (Lambert et al., 2013b; Jansen et al., 2019). HLA-DRB1/HLA-DRB5 locus within the major histocompatibility complex is responsible for numerous immune responses (Rosenthal and Kamboh, 2014). NECTIN2 rs6859 was associated with LOAD (Abraham et al., 2008; Harold et al., 2009). NECTIN2 rs6857 and rs41289512 were also associated with AD (Seshadri et al., 2010; Jansen et al., 2019). NECTIN2 (also known as PVRL2) is a gene, encoding for poliovirus receptor 2, immunoglobulin expressed in neuronal cell tissues, that is important in T-cell activation (Whelan et al., 2019).
Two other proteins are important in regulation of cellular processes. A novel MADD loci, rs10501320, was recently associated with AD and fasting glucose (Zhu et al., 2019). MADD may play a role in regulating cell proliferation, survival and death through alternative mRNA splicing (Efimova et al., 2004). PDS5B rs192470679 was associated with MCI to AD conversion (Lee et al., 2017). PDS5B is part of the cohesin complex, involved in transcriptional regulation, chromosomal compaction and sister chromatid cohesion (Blind, 2020). Stimulating the release of cohesion from chromosomes, PDS5B is considered negative regulator of cohesin DNA-binding function (Carretero et al., 2013; Blind, 2020).
Biological Regulation
Regulation is a common feature of all living organisms, however complexity of biological regulation is in domain of evolutionary progress. Biological regulation can be addressed as a network of functional relationships, allowing organism to modulate response to changes in internal and external conditions (Bich et al., 2016). Genes and key SNPs, implemented in biological regulation, associated with AD risk in GWAS and meta-analyses, are summarized in Table 3.
Several AD related genes are involved in regulation of the immune system. Specific interleukin gene polymorphisms confer greater risk for AD (Du et al., 2000; Grimaldi et al., 2000; Nicoll et al., 2000). IL6R rs2228145 was associated with increased AD risk (Haddick et al., 2017). IL6, a multifunctional cytokine is involved in the regulation of acute inflammatory response and modulation of specific immune response (Akira et al., 1993; Papassotiropoulos et al., 2001). It interacts with IL6 receptor (IL6R). In the nervous system, IL6 has a role in neuronal cell growth and differentiation, as well as neuronal degradation. Multiple evidence suggest the importance of IL6 in AD pathogenesis (Breitner et al., 1986; Peter and Walter, 1991; Campbell et al., 1994). Identifying CD2AP as a LOAD risk locus, rs9349407 association with AD was observed (Naj et al., 2011). Increased AD risk effect for rs9349407 was later confirmed (Hollingworth et al., 2011). In IGAP the greatest association with increased AD risk was observed for CD2AP rs10948363 (Lambert et al., 2013b). Another two CD2AP polymorphisms, rs9381563 and rs9473117, were also associated with AD (Jansen et al., 2019; Kunkle et al., 2019). CD2AP has an important part in the immune system as it binds and clusters CD2 to facilitate junction between T-cells and antigen presenting cells (Rosenthal and Kamboh, 2014). The minor allele of CD33 rs3865444 was proposed as a protective LOAD locus (Naj et al., 2011). This effect was also confirmed in two other studies (Hollingworth et al., 2011; Jansen et al., 2019). CD33 is a member of the sialic-acid-binding immunoglobulin-like lectins family. It acts as an endocytotic receptor, mediating endocytosis through a mechanism independent of clathrin (Hollingworth et al., 2011; Rosenthal and Kamboh, 2014). It also promotes cell-cell interactions that regulates the innate immune system (Crocker et al., 2007; Tanzi, 2012; Jiang et al., 2014). The level of CD33 was found to be increased in the AD brain and it was in positive correlation with amyloid plaque burden and disease severity (Jiang et al., 2014).
CASS4 rs7274581 and rs6024870 showed protective function (Lambert et al., 2013b; Kunkle et al., 2019). Furthermore, rs6014724 was associated with AD risk (Jansen et al., 2019). As a member of CAS family, CASS4 directly regulates FAK (focal adhesion kinase) (Deneka et al., 2015). CASS4 is also involved in cytoskeletal function and important in APP metabolism (Karch and Goate, 2015). Association with AD risk was reported for PDCL3 rs1513625 (Herold et al., 2016). PDCL3 encodes phosphoducin-like 3, potential modulator of heterotrimeric G-proteins and a chaperone for the VEGF receptor, regulating its ubiquitination and degradation (Srinivasan et al., 2013). TNK1 rs1554948 association with increased LOAD risk was reported (Grupe et al., 2007). TNK1, a non-receptor protein tyrosine kinase is important in intracellular transduction pathways, involved in THFα induced apoptosis and proliferation of cancer cells (Azoitei et al., 2007; Henderson et al., 2011; Seripa et al., 2018). Rs11767557, located in EPHA1 promoter region was identified as AD protective locus in multiple studies (Hollingworth et al., 2011; Naj et al., 2011; Kamboh et al., 2012b). Similarly, EPHA1 rs11771145, rs10808026, and rs6973770 were all associated with decreased AD risk (Seshadri et al., 2010; Lambert et al., 2013b; Reitz et al., 2013; Kunkle et al., 2019). Recently, EPHA1 rs7810606 was also associated with AD (Jansen et al., 2019). EPHA1 is a member of the ephrin receptor subfamily. Ephrins and Eph receptors are membrane bound proteins involved in cell and axon guidance and in synaptic development and plasticity (Rosenberg et al., 2016). EPHA1 is expressed mainly in epithelial tissues where it regulates cell morphology and motility and it may also have a role in apoptosis and inflammation (Hollingworth et al., 2011).
Two genes, associated with AD, have important role as metabolic hormones. ACE rs4293 was identified as a risk loci for LOAD (Webster et al., 2010). Another novel polymorphism in this region was discovered recently. ACE rs138190086 association with increased LOAD risk was reported (Kunkle et al., 2019). Angiotensin II, a product of ACE gene, primarily known as vasoconstrictor, is also involved in a number of neuropathological processes in AD (Kehoe, 2018). HBEGF rs11168036 was associated with increased risk for AD (Jun et al., 2016). HBEGF is an important growth factor, involved in several biological processes like smooth muscle cell growth, skeletal muscle myogenesis, gastrointestinal tract mucosa maintenance, embryo implantation, wound healing and injury repair (Davies-Fleischer and Besner, 1998). HBEGF is widely expressed in the CNS, suggesting its important role in nervous system development (Oyagi and Hara, 2012).
Among all AD risk loci, obtained from GWAS and meta-analyses that were not enriched in GO analysis, additional three were manually annotated to biological regulation and are also summarized in Table 3. Rs6448453 near to CLNK was recently associated with AD (Jansen et al., 2019). CLNK encodes a protein with important immunomodulatory function, involved in positive regulation of immunoreceptor signaling as a SLP-76 family member (Cao et al., 1999). Since HESX1 rs184384746 was associated with AD risk, its potential role in AD pathology was discussed (Jansen et al., 2019). Homeobox transcription factor, encoded by HESX1, was primarily identified in embryonic stem cells (Thomas and Rathjen, 1992). Grupe et al. reported the association of GALP rs3745833 with increased risk for LOAD (Grupe et al., 2007). GALP is a neuropeptide, having important role in the central metabolic control of the reproductive axis (Aziz et al., 2014). It is a ligand in G-protein mediated signal transduction in CNS (Robinson et al., 2006).
Localization
Localization includes different processes, involved in transport of cells, cell organelles or protein complexes as well as their maintenance in specific location. Genes and key SNPs, implemented in localization, associated with AD risk in GWAS and meta-analyses, are presented in Table 4.
Four SNPs in SORL1 (rs2101756, rs11218313, rs626885, and rs7131432) were identified as novel AD related risk alleles (Webster et al., 2008). Twenty-five SNPs in SORL1 were identified and although none of them showed genome-wide significance for association with AD, rs2070045 was the best predictor of AD risk among them (Laumet et al., 2010). IGAP showed a protective association of SORL1 rs11218343 with AD (Lambert et al., 2013b). This association was confirmed in two other studies (Jansen et al., 2019; Kunkle et al., 2019). Another two SORL1 SNPs, rs3781834, and rs11218343 were also associated with LOAD risk (Miyashita et al., 2013). Sorting mechanisms that cause the APP and the β-secretases and γ-secretases to colocalize in the same compartment play an important role in the regulation of Aβ production in AD. APP trafficking is regulated by sortilin related receptors, including SORL1, which binds the APP in the Golgi and reduce availability of precursors for transport, cleavage and transformation in Aβ (Andersen et al., 2005). Decreased expression of SORL1 leads to overproduction of Aβ (Reitz et al., 2011).
Association of rs1981916 with LOAD could propose VPS13C as novel risk locus (Meda et al., 2012). VPS13C potential involvement in cargo selection and sorting into vesicles is consistent with its relocation from mitochondria to cytosol in response to damage. VPS13C mutations have been linked to parkinsonism, but the importance of this gene in AD has also been addressed (Lesage et al., 2016).
PLCG2 rs72824905 was associated with protection against LOAD (Sims et al., 2017). Furthermore, PLCG2 rs12444183 was associated with AD (Marioni et al., 2018). The product of PLCG2 gene is involved in the transmembrane transduction of immune signals, that determine the function of various immune cell types in CNS (Patterson et al., 2002; Kim et al., 2015). IGAP identified a protective association with AD risk in SLC24A4 rs10498633 (Lambert et al., 2013b). This association was later confirmed (Ruiz et al., 2014). Additionally, two studies identified SLC24A4 rs12590654 and rs12881735 as AD protective loci (Jansen et al., 2019; Kunkle et al., 2019). SLC24A4 is encoding NCKX4, a sodium-potassium-calcium channel, expressed in human brain (Li et al., 2002). Potential role of SLC24A4 in nervous system has been discussed, since this gene was linked to neurogenesis pathways (Larsson et al., 2011).
Another three genes, not represented in GO enrichment analysis, were manually annotated to localization and are summarized in Table 4. TOMM40 is important AD risk locus. TOMM40 rs157581 was associated with increased AD risk (Grupe et al., 2007). Two other TOMM40 polymorphisms, rs157580, and rs8106922 were associated with LOAD (Abraham et al., 2008). Both SNPs conferred decreased AD risk in several studies (Harold et al., 2009; Feulner et al., 2010; Pérez-Palma et al., 2014). On the other hand, associations with increased risk for AD were reported for TOMM40 rs2075650, rs157582, and rs10119 (Seshadri et al., 2010). Numerous studies confirmed the association for rs2075650 (Harold et al., 2009; Feulner et al., 2010; Wijsman et al., 2011). The outer mitochondrial membrane translocase pore subunit (TOMM40) forms one of the primary pores via which proteins can readily enter the mitochondria. The TOMM40 gene is the only gene identified in genetic studies to date that presumably contributes to LOAD-related mitochondria dysfunction (Gottschalk et al., 2014). It is encoded on chromosome 19, adjacent to APOE region. TOMM40 also impacts brain areas vulnerable in AD, by downstream apoptotic processes that forego extracellular Aβ aggregation. By entering and obstructing the TOMM40 pore, APP induces mechanisms for mitochondrial dysfunction (Devi et al., 2006). As APOE and TOMM40 genomic regions are in close proximity, their potentially interacting effect in mitochondrial function in AD progression is discussed (Roses et al., 2010). Seshadri et al. reported an association between EXOC3L2 rs597668 and increased LOAD risk (Seshadri et al., 2010). EXOC3L2 (exocyst complex component 3-like 2) is involved in vesicle targeting during exocytosis of proteins and lipids. SLC2A9 rs6834555 was associated with increased LOAD risk (Hollingworth et al., 2012). The SLC2A9 encodes for GLUT9, urate transporter, that was initially characterized as a glucose transporter (Vitart et al., 2008; Ebert et al., 2017).
Genes With No Known GO Function
The remaining 13 genes could not be associated with any of the four main enriched pathways, even though they were linked to risk for AD in GWAS and meta-analysis. Although some of them were linked to a specific function in the literature, they have not been annotated with any of the GO terms. Genes and key SNPs with no known function associated with AD risk in GWAS and meta-analyses are summarized in Table 5.
BTBD16 rs10510109 was identified as novel AD related polymorphism (Wang et al., 2017). CDR2L was previously associated with ovarian cancer and cerebellar degeneration (Raspotnig et al., 2017). CDR2L rs71380849 was associated with increased risk for AD (Jun et al., 2016). Increased risk for AD was reported for MBLAC1 rs35991721 (Broce et al., 2019). Binding with metals is a major function of MBLAC1, encoding for metallo-β-lactamase domain-containing protein in the brain (Fagerberg et al., 2014). It is involved in hydrolysis of different substrates and metabolic intermediates (Gibson et al., 2018). Electron transport chain in mitochondria enables proton motor force generation via redox reactions. Genome-wide significant association with T2D and AD was observed for rs7812465 in NDUFAF6 region (Wang et al., 2017). A protein involved in the assembly of mitochondrial respiratory chain complex I is encoded by NDUFAF6 (also known as C8orf38) (Zurita Rendón and Shoubridge, 2012).
Although the exact function of IGHV1-68 is not understood to date, rs79452530 in this gene showed decreased risk for AD (Witoelar et al., 2018). IGHV1-68 is a pseudogene within the immunoglobulin heavy chain (IGH) locus, contributing to the diverse and specific Ig forming in the adaptive immunity (Matsuda et al., 1998). LHFPL6 rs9315702 was associated with AD-related phenotype of hippocampal volume (Melville et al., 2012). LHFPL tetraspan subfamily member 6 protein, also known as lipoma HMGIC fusion partner is encoded by LHFPL6 gene with no confirmed function, although a recent study evaluated LHFPL6 as a bone mass regulator in mice (Mesner et al., 2019).
ARL17B rs2732703 was associated with decreased risk of AD in APOE4 negative population (Jun et al., 2016). ARL17B is localized to the Golgi apparatus and is potentially involved in modulation of vesicle budding and is a known activator of cholera toxin catalytic subunit of ADP-ribosyltransferase (Pasqualato et al., 2002).
KRBOX4 rs7876304 showed significant association with LOAD (Meda et al., 2012). KRBOX4 is a potential transcriptional regulator with no confirmed function. IGAP revealed a significant protective association of ZCWPW1 rs1476679 (Lambert et al., 2013b). Recently, rs1859788 in ZCWPW1 region was also associated with AD (Jansen et al., 2019). Zinc-finger ZCWPW1 is another gene involved in histone modification and epigenetic regulation (He et al., 2010). PPP4R3A rs2273647 showed protective effect in risk for AD (Christopher et al., 2017). It encodes a regulatory subunit PPP4R3A of serine/threonine phosphatase (Chowdhury et al., 2008). A novel susceptibility protective locus for AD was MS4A6A rs610932, while MS4A4E rs670139 showed increased risk for AD (Hollingworth et al., 2011). Tan et al. showed a significant association of MS4A6A rs610932 with the risk of LOAD (Tan et al., 2013). In IGAP MS4A6A rs983392 had a protective function (Lambert et al., 2013b). MS4A4A rs2081545 was associated with AD, while protective function of MS4A4A rs4938933 and rs10792258 in AD was also reported (Logue et al., 2011; Naj et al., 2011; Jansen et al., 2019).
Genes Associated With AD Biomarker Levels
Changes in cerebrospinal fluid (CSF) and blood plasma biomarker levels can predict neurodegenerative changes in AD progression and memory decline and are often used in clinical diagnostics. Except for their diagnostic potential, biomarkers can be applied in studies of AD molecular mechanisms and could be used to monitor the biochemical effects of potential disease intervention (Masters et al., 2015; Efthymiou and Goate, 2017). Genetic variability in different molecular pathways can contribute to differences in biomarker levels. The search for early, reliable and accurate biomarkers for AD progression exceeds genetic approach. Epigenetic factors may play an important role, and the potential of non-coding regulatory RNAs, especially miRNA as biomarkers of AD progression in body fluids has been extensively studied (Takousis et al., 2019). Furthermore, functional neuroimaging provides insight into metabolic and biochemical alterations in the brain, such as glucose metabolism, perfusion, deposition of Aβ and tau protein aggregation (Valotassiou et al., 2018). Thus, GWAS studies and meta-analyses often implement genome-wide genetic data in biomarker studies, to associate mutations or polymorphisms with measurable changes in components of body fluids and brain imaging in AD (Table 6). Apart from identifying novel genetic risk loci, Beecham et al. performed the assessment of the presence of neurofibrillary plaques and tangles, immunohistochemical detection of α-synuclein and neuropathological evaluation in most of the IGAP identified risk loci (Beecham et al., 2014). In the biomarker gene set, seven major GO categories were enriched: cellular process, metabolic process, biological regulation, localization, transport, regulation of cellular process, and neurological system process (Figure 3). Since biomarker gene set enrichment resulted in three additional major categories, some of the genes can be found in different major categories compared to AD risk gene set. Furthermore, transport can be understood as a subcategory of metabolic process, while regulation of cellular process is part of biological regulation and cellular process.
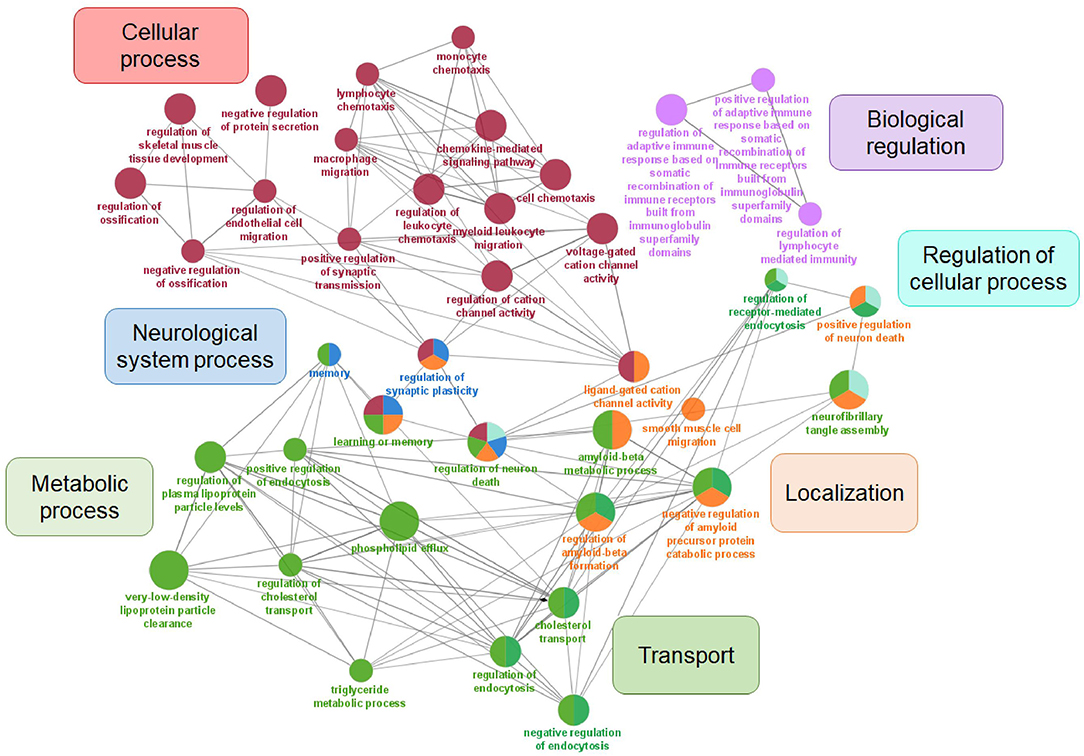
Figure 3. Visualization of GO analysis in AD biomarker gene set. Genes associated with AD biomarkers were stratified according to GO – biological process. They are clustered in seven parental categories and represented with specific color of the node. Biological processes that can be assigned to multiple parental categories, are represented with multiple color-pie chart.
Several biomarker associated loci overlap with risk-related genes (CASS4, PICALM, SORL1, APOE, CLU, CD33, CR1, MEF2C, NECTIN2, ZCWPW1, ABCA7, MS4A6A, and PTK2B) linked to molecular pathways identified in this review (Table 6).
It is not surprising that APOE locus is extensively studied not only as a risk factor, but also in genome-wide analysis of biomarkers. APOE rs429358 was associated with neuroimaging-confirmed brain changes (Shen et al., 2010), PET Aβ deposition (Ramanan et al., 2014), CSF Aβ and tau levels (Kim et al., 2011) as well as neuropathologic features (Beecham et al., 2014; Dumitrescu et al., 2019). APOE rs769449 was associated with tau/Aβ ratio (Li et al., 2017), while rs769449 and rs7259620 were associated with neuropathologic features (Dumitrescu et al., 2019). Biomarker associations were observed also for APOC1 rs10414043, rs7256200, rs483082, and rs438811 (Dumitrescu et al., 2019), while APOC1 rs4420638 was associated with tau/Aβ ratio (Li et al., 2017). CDK5RAP2 rs10984186 was associated with higher mRNA expression in hippocampus, while rs4837766 showed reduced total mRNA levels (Miron et al., 2018). Also rs157580 and rs2075650 in TOMM40, important regulator of energy metabolism were associated with AD biomarkers (Kim et al., 2011; Cruchaga et al., 2013). TOMM40 rs157581, rs11556505, rs71352238, rs184017, rs1160985, rs741780, rs1038025, rs760136, and rs1038026 were all associated with neuropathologic features of AD (Dumitrescu et al., 2019). Two additional TOMM40 polymorphisms (rs11556505, rs71352238) were associated with CSF Aβ and tau levels (Cruchaga et al., 2013). Furthermore TOMM40 rs34404554 was associated with CSF Aβ/tau levels and neuropathologic features respectively (Cruchaga et al., 2013; Dumitrescu et al., 2019). Similarly, SNPs in NECTIN2 (rs12972970, rs34342646, and rs12972156), important mediator of immune system, were associated with CSF Aβ and tau levels (Cruchaga et al., 2013). All three associations were also linked to neuropathologic features, together with rs6857 and rs283815 (Dumitrescu et al., 2019). Rs61812598, rs4845622, rs2228145, rs4129267, and rs2229238 in the IL6 receptor (IL6R), were associated with LOAD protein expression in CSF [271]. Furthermore, association of rs2228145 with CSF and serum IL6R levels revealed the effect on age of onset in AD [171]. GLIS3 rs514716 association with CSF Aβ and tau levels was observed (Cruchaga et al., 2013). SERPINB1 rs316341 and SPI1 rs1057233 were associated with CSF Aβ levels (Deming et al., 2017; Huang et al., 2017a). The association between decline in brain glucose metabolism and PPP4R3A rs2273647 was recently reported (Christopher et al., 2017). Three ACE polymorphisms (rs4968782, rs4316, rs4343) were associated with CSF levels of ACE gene product (Kauwe et al., 2014). An association with soluble TREM2 was also observed for MS4A6A rs7232 (Hou et al., 2019).
Numerous other GWAS studies investigated associations of genetic polymorphisms with biomarker level changes rather than the risk for AD and identified additional 30 genes that were not associated with AD risk (Figure 1).
Metabolic Processes
Genes and key SNPs, involved in metabolic processes, associated with biomarkers in GWAS and their meta-analyses, are summarized in Table 6.
Rs7867518, adjacent to VLDLR, was associated with CSF total tau levels (Huang et al., 2017a). VLDLR encodes for a receptor, regulating lipoprotein binding, with high affinity for very low density lipoprotein (VLDL) and APOE-containing lipoproteins (Sakai et al., 1994).
Four other genes associated with biomarker levels, were manually annotated to metabolic process and are also summarized in Table 6. Enzymes in the TCA play a crucial role in energy metabolism, and provide points of interaction between catabolic and anabolic pathways in different cells types, including neuronal tissue. Ramirez et al. found a significant association between SUCLG2 rs62256378 and CSF Aβ levels in AD subjects (Ramirez et al., 2014). SUCLG2 is a substrate-specific subunit of succinyl CoA ligase, enabling GTP formation in TCA (Johnson et al., 1998). Experiments in cell cultures indicate the absence of SUCLG2 in astrocytes, microglia, and oligodendrocytes, addressing the question of normal TCA function in brain (Dobolyi et al., 2014). Association of GCFC2 rs2298948 with AD was reported for brain magnetic resonance imaging (Melville et al., 2012). GCFC2 (GC-rich sequence DNA-binding factor 2) is a regulator of pre-mRNA splicing (Yoshimoto et al., 2014). Multiple polymorphism in EPC2 region were identified (rs2121433, rs1374441, rs4499362, and rs10171238) that were associated with CSF Aβ levels in AD subjects (Kim et al., 2011). Enhancer of polycomb homolog 2, EPC2, is associated with chromatin repressive complex (Whitton et al., 2016). F5 rs6703865 was associated with brain magnetic resonance imaging with AD (Melville et al., 2012). Specific function of coagulation factor V (F5), a glycoprotein, is important in clot formation (LaBonte, 2014).
Cellular Processes
Genes and key SNPs, involved in cellular processes, associated with biomarkers in GWAS and their meta-analyses, are summarized in Table 6.
Association with language performance was observed for GLI3 rs3801203 in AD patients (Deters et al., 2017). GLI3 is a GLI family zinc-finger protein 3 that in presence or absence of Sonic Hedgehog functions as a mediator of the Sonic Hedgehog pathway (Wang et al., 2000a). A novel HDAC9 polymorphism rs79524815 was recently associated with neuropathologic traits for AD (Chung et al., 2018b). Control of gene expression through chromatin remodeling is a function of histone deacetylase HDAC9 (Sugo et al., 2010). A novel AD-related locus RBFOX1 rs12444565 in AD driven neurodegeneration was evaluated with fluorodeoxyglucose PET scanning (Kong et al., 2018). Alternative splicing mechanism regulator, important in erythropoiesis, is encoded by RNA binding protein fox-1 homolog 1 (RBFOX1) (Ponthier et al., 2006). ANK3 rs10761514 was associated with CSF Aβ levels in AD (Huang et al., 2019). ANK3 encodes for a membrane protein AnkG, important for spectrin-based anchoring of membrane proteins to the cytoskeleton (Kordeli et al., 1995). Kauwe et al. linked CCL4 rs6808835 with CCL4 protein expression in CSF of LOAD cases (Kauwe et al., 2014). A macrophage inflammatory protein, product of CCL4 (C-C motif chemokine 4-like) gene, is important enhancer of immune response (Lien et al., 2017). MTUS1 rs55653268, identified in cognitively normal and MCI subjects, was associated with AD-related changes in hippocampal volume (Chung et al., 2018a). Findings could lead to the understanding of genetic mechanisms for conversion of normal cognitive or mild cognitive impaired individuals to AD patients. Microtubule-associated tumor suppressor 1 gene (MTUS1) encodes ATIP3, inhibitor of extracellular signal-regulated kinase 2 (ERK2) and cell proliferation. Similarly, rs3092960 within CCR2 encoding for CCL2 receptor was also associated with significant levels of CCR2 protein in CSF (Kauwe et al., 2014). Furthermore, two polymorphisms (rs6441977, rs11574428) in C-C chemokine receptor-like 2 gene (CCRL2) were associated with CCRL2 protein expression in CSF as well (Kauwe et al., 2014).
Although not enriched in GO analysis, many other genes were manually annotated cellular processes and are also summarized in Table 6. IL1RAP rs12053868 was proposed as a marker for PET Aβ deposition in MCI to AD conversion (Ramanan et al., 2015). Interleukin-1 receptor accessory protein (IL1RAP) is essential in cellular response to IL1 and involved in several other signaling pathways (Cullinan et al., 1998; Dinarello, 2009). Polymorphisms in MMP3 gene (rs573521, rs645419, rs679620, rs650108, and rs948399) were associated with biomarker levels, with rs573521 being the best predictor of MMP3 protein expression in CSF in LOAD (Kauwe et al., 2014). Matrix metalloproteinase-3 (MMP3) is important in extracellular matrix remodeling (Banik et al., 2015). The Aβ-induced expression of MMP3, as well as potential degrading function of extracellular Aβ were observed in astrocytes (Deb and Gottschall, 2002; White et al., 2006). CLUAP1 rs17794023 was associated with higher CSF α-synuclein levels, suggesting CLUAP1 (CLU-associated protein 1) as a novel AD-related locus (Zhong et al., 2019). Rs4803758 near BCAM was associated with CSF levels of phosphorylated tau181 and Aβ42 (Huang et al., 2017a). BCAM is a gene encoding Lutheran blood group glycoprotein, an immunoglobulin important in laminin recognition (Parsons et al., 1995). ARHGAP24 rs111882035 was associated with memory tests outcomes in MCI individuals (Chung et al., 2018a). A Rho GTPase-activating protein 24, encoded by ARHGAP24 is important in actin cytoskeleton remodeling and specifically suppresses Rac1 and Cdc42 activity (Lavelin and Geiger, 2005). Another SNP associated with neuropathologic features in AD was rs34331204, an intergenic SNP near basic leucine zipper and W2 domain-containing protein 2 (BZW2) (Dumitrescu et al., 2019). The rat homolog, Bdm2, is highly expressed in brain, suggesting the role of the protein in neurodevelopment (Nishinaka et al., 2000).
Biological Regulation
Genes and key SNPs, involved in biological regulation, associated with biomarkers in GWAS and their meta-analyses, are summarized in Table 6.
Three CD1A polymorphisms: rs16840041, rs2269714, and rs2269715 were associated with increased plasma neurofilament light level, a potential protein biomarker for AD (Wang et al., 2019b). CD1A proteins are another important molecules of immune system, regulating glycolipid and lipid antigen presentation of microbial origin or themselves to T-cells (Zajonc et al., 2003; Moody et al., 2004).
Additionally, one biomarker associated gene was manually annotated to biological regulation (Table 6). A polymorphism in the gene encoding for the V1H subunit of vacuolar ATPase, regulating enzyme activity (Marshansky et al., 2014; Colacurcio and Nixon, 2016), ATP6V1H rs1481950 was associated with higher CSF BACE activity (Hu et al., 2018).
Localization
Genes and key SNPs, involved in localization, associated with biomarkers in GWAS and their meta-analyses, are summarized in Table 6.
GRIN2B rs10845840 was reported as a risk loci for AD, associated with temporal lobe atrophy (Stein et al., 2010). GRIN2B encodes the NR2B subunit of NMDA receptor that mediates a Ca2+ dependent synaptic transmission in the CNS (Hu et al., 2016).
Neurological System Processes
Genes and key SNPs, involved in neurological system process, GO term specific for biomarker gene set, are summarized in Table 6. MAPT rs242557 was associated with plasma tau levels (Chen et al., 2017). MAPT encodes for tau, the prominent component of NFTs. H2 haplotype is associated with MAPT expression and LOAD risk (Allen et al., 2014). A significant association of BCHE rs509208 with PET imaging of cortical Aβ in AD subjects was revealed (Ramanan et al., 2014). Butyrylcholinesterase (BCHE) is a serine esterase, involved in organophosphate ester hydrolysis (Amitay and Shurki, 2009). It is important in neurotransmitter activation and enriched in senile plaques of AD brains (Darvesh et al., 2003).
Among all AD related biomarker loci, obtained from GWAS and meta-analyses that were not enriched in GO analysis, additional 18 were manually annotated.
Genes With No Known GO Function
The remaining seven genes could not be associated with any of the seven main enriched pathways, even though they were linked biomarker changes in AD GWAS and meta-analysis. Although some of them were linked to a specific function in the literature, they have not been annotated with any of the GO terms. Genes and key SNPs with no known function associated with AD biomarkers in GWAS and meta-analyses are summarized in Table 6.
CCDC134 rs7364180 was associated with CSF Aβ levels in AD subjects (Kim et al., 2011). Coiled-coil domain-containing protein 134, encoded by CCDC134 is a proliferation promoting molecule, driving cytokine-like activation of CD8+ T-cells (Huang et al., 2014). Association with neuropathologic traits of AD in ECRG4 rs34487851 was observed (Chung et al., 2018b). ECRG4 encodes a peptide hormone that is involved in NFT formation, age-related senescence of precursor cells in the CNS and activation of microglia and peripheral mononuclear leukocytes (Kujuro et al., 2010; Woo et al., 2010; Podvin et al., 2016). Two polymorphisms (rs10509663, rs116953792) were associated with CSF Aβ levels in AD patients, proposing FRA10AC1 as a novel risk locus (Li et al., 2015). FRA10AC1 is a protein of unknown function. The polymorphic CGG/CCG repeats in the 5'-UTR of FRA10AC1 gene are potential cause of folate-sensitive fragile site FRA10A expression (Sarafidou et al., 2004). LUZP2 was also proposed as a novel AD risk locus as rs7943454 was associated with higher plasma neurofilament light levels (Li et al., 2018). A leucine-zipper protein of unknown function, which is normally expressed only in the brain and the spinal cord, is encoded by LUZP2 gene (Stepanov et al., 2018). Although the function of a zinc finger protein, encoded by ZNF804B, is not known yet, a ZNF804B rs73705514 was associated with memory tests outcomes in MCI individuals (Chung et al., 2018a).
Conclusion and Further Perspectives
Alzheimer's disease is the most prevalent neurodegenerative disorder worldwide. A lot of research focuses on the identification of genetic factors that may contribute to the development and progression of the disease. Numerous GWASs and meta-analyses reported different genetic factors associated with AD risk or biomarker levels. A cumulative effect of small but significant contributions of numerous genetic factors can at least in part elucidate the LOAD progression. The pathogenic processes in AD may be influenced on a personalized basis by a combination of variants in key genes and pathways. Apart from serving as a hallmark of the disease, polymorphisms in various genes might help in early diagnostics and prediction of disease progression. Integration of genetic factors and biomarker status may increase the predictive value of diagnostic or prognostic models.
Through the GO analysis we compiled a list of the most enriched pathways, associated with AD pathology. Among four GO parental categories in AD risk gene set, immune response, APP metabolism, cholesterol metabolism, endocytosis and biological regulation on different levels can be exposed as important AD related biological processes. Furthermore, enrichment analysis on smaller AD biomarker gene set pinpointed three additional parental categories. Besides neurodegeneration, numerous research evidence link AD with neuroinflammation, lipid metabolism as well as receptor mediated endocytosis, supporting scientific background of our analysis. Several identified genes were associated with more than one biologic process, represented in various GO categories. The intersection of different biological processes creates a complex interconnected network, suggesting multi-pathway approach in AD genetic background evaluation is needed. Additionally, manual annotation of genes that were not associated with the most significant pathways in GO analysis, could help to elucidate their function in AD pathogenesis.
This comprehensive summary of genetic variants identified by GWAS studies and their meta-analyses can also provide background for identification of novel molecular targets, and the results may be important for development of personalized medicine. However, GWAS and meta-analyses cannot explain the molecular mechanisms of the contribution of a novel susceptibility locus to the overall genetic risk. Therefore, our compiled and annotated results may serve as a basis for the functional studies of pathophysiological mechanisms of risk genes, identified on a genome-wide scale. Furthermore, better characterization of risk genes functions could enable the stratification of AD patients according to the main molecular mechanisms of pathogenesis, supporting development of tailored and personalized treatment of the disease.
Data Availability Statement
The original contributions presented in the study are included in the article/Supplementary Material, further inquiries can be directed to the corresponding author/s.
Author Contributions
DV performed literature search and gene enrichment analysis. DV, KG, and VD participated in writing and editing of manuscript. All authors read and approved the final manuscript.
Funding
This work has received funding from the Slovenian Research Agency (ARRS Grant No. P1-0170) and from the European Union's Horizon 2020 research and innovation programme under grant agreement No. 951851. The results reflect only the authors' view. The Research Executive Agency is not responsible for any use that may be made of the information this publication contains.
Conflict of Interest
The authors declare that the research was conducted in the absence of any commercial or financial relationships that could be construed as a potential conflict of interest.
Acknowledgments
We would like to thank Katarina Esih, MD for previous work that was done on the topic.
Supplementary Material
The Supplementary Material for this article can be found online at: https://www.frontiersin.org/articles/10.3389/fnagi.2021.646901/full#supplementary-material
Supplementary Figure 1. PRISMA flow diagram of the literature search.
Supplementary Table 1. Characteristics of genome-wide association studies (GWAS) included in the review.
Supplementary Table 2. Characteristics of meta-analyses included in the review.
Supplementary Table 3. List of enriched GO biological processes in AD risk gene set.
Supplementary Table 4. List of enriched GO biological processes in AD biomarker gene set.
Supplementary Table 5. Raw data of GO enrichment analysis from ClueGO for AD risk gene set.
Supplementary Table 6. Raw data of GO enrichment analysis from ClueGO for AD risk biomarker set.
Abbreviations
Aβ, amyloid-β; AD, Alzheimer's disease; APP, amyloid precursor protein; EOAD, Early onset Alzheimer's disease; CNS, central nervous system; GO, Gene Ontology; GWAS, genome-wide association study; IGAP, International Genomics of Alzheimer's Project; LOAD, Late onset Alzheimer's disease; MCI, mild cognitive impairment; NFT, neurofibrillary tangles; PET, positron emission tomography; PSEN1, presenilin-1; PSEN2, presenilin-2; T2D, Type 2 diabetes; TCA, tricarboxylic acid cycle.
References
2020 Alzheimer's disease facts and figures (2020). 2020 Alzheimer's disease facts and figures. Alzheimer's Dement. 16, 391–460. doi: 10.1002/alz.12068
Abraham, R., Moskvina, V., Sims, R., Hollingworth, P., Morgan, A., Georgieva, L., et al. (2008). A genome-wide association study for late-onset Alzheimer's disease using DNA pooling. BMC Med. Genom. 1:44. doi: 10.1186/1755-8794-1-44
Acx, H., Serneels, L., Radaelli, E., Muyldermans, S., Vincke, C., Pepermans, E., et al. (2017). Inactivation of γ-secretases leads to accumulation of substrates and non-Alzheimer neurodegeneration. EMBO Mol. Med. 9, 1088–1099. doi: 10.15252/emmm.201707561
Ahuja, R., Pinyol, R., Reichenbach, N., Custer, L., Klingensmith, J., Kessels, M. M., et al. (2007). Cordon-bleu is an actin nucleation factor and controls neuronal morphology. Cell 131, 337–350. doi: 10.1016/j.cell.2007.08.030
Akira, S., Taga, T., and Kishimoto, T. (1993). Interleukin-6 in biology and medicine. Adv. Immunol. 54, 1–78. doi: 10.1016/S0065-2776(08)60532-5
Allen, M., Kachadoorian, M., Quicksall, Z., Zou, F., Chai, H. S., Younkin, C., et al. (2014). Association of MAPT haplotypes with Alzheimer's disease risk and MAPT brain gene expression levels. Alzheimer's Res. Ther. 6:alzrt268. doi: 10.1186/alzrt268
Amemori, T., Jendelova, P., Ruzicka, J., Urdzikova, L. M., and Sykova, E. (2015). Alzheimer's disease: mechanism and approach to cell therapy. Int. J. Mol. Sci. 16, 26417–26451. doi: 10.3390/ijms161125961
Amitay, M., and Shurki, A. (2009). The structure of G117H mutant of butyrylcholinesterase: nerve agents scavenger. Proteins Struct. Funct. Bioinform. 77, 370–377. doi: 10.1002/prot.22442
Andersen, O. M., Reiche, J., Schmidt, V., Gotthardt, M., Spoelgen, R., Behlke, J., et al. (2005). Neuronal sorting protein-related receptor sorLA/LR11 regulates processing of the amyloid precursor protein. Proc. Natl. Acad. Sci. U. S. A. 102, 13461–13466. doi: 10.1073/pnas.0503689102
Apte, S. S. (2009). A disintegrin-like and metalloprotease (reprolysin-type) with thrombospondin type 1 motif (ADAMTS) superfamily: functions and mechanisms. J. Biol. Chem. 284, 31493–31497. doi: 10.1074/jbc.R109.052340
Arioka, M., Tsukamoto, M., Ishiguro, K., Kato, R., Sato, K., Imahori, K., et al. (1993). τ protein kinase II is involved in the regulation of the normal phosphorylation state of τ protein. J. Neurochem. 60, 461–468. doi: 10.1111/j.1471-4159.1993.tb03173.x
Ashford, J. W. (2004). APOE genotype effects on Alzheimer's disease definition of AD epidemiology of AD. J. Mol. Neurosci. 23, 157–165. doi: 10.1385/JMN:23:3:157
Assou, S., Haouzi, D., Dechaud, H., Gala, A., Ferrières, A., and Hamamah, S. (2013). Comparative gene expression profiling in human cumulus cells according to ovarian gonadotropin treatments. Biomed Res. Int. 2013:354582. doi: 10.1155/2013/354582
Aziz, R., Beymer, M., Negrón, A. L., Newshan, A., Yu, G., Rosati, B., et al. (2014). Galanin-Like Peptide (GALP) neurone-specific phosphoinositide 3-kinase signalling regulates GALP mRNA levels in the hypothalamus of males and luteinising hormone levels in both sexes. J. Neuroendocrinol. 26, 426–438. doi: 10.1111/jne.12163
Azoitei, N., Brey, A., Busch, T., Fulda, S., Adler, G., and Seufferlein, T. (2007). Thirty-eight-negative kinase 1 (TNK1) facilitates TNFα-induced apoptosis by blocking NF-κB activation. Oncogene 26, 6536–6545. doi: 10.1038/sj.onc.1210476
Banik, D., Netherby, C. S., Bogner, P. N., and Abrams, S. I. (2015). MMP3-Mediated tumor progression is controlled transcriptionally by a novel IRF8-MMP3 interaction. Oncotarget 6, 15164–15179. doi: 10.18632/oncotarget.3897
Beecham, G. W., Hamilton, K., Naj, A. C., Martin, E. R., Huentelman, M., Myers, A. J., et al. (2014). Genome-wide association meta-analysis of neuropathologic features of Alzheimer's disease and related dementias. PLoS Genet. 10:e1004606. doi: 10.1371/journal.pgen.1004606
Bekris, L. M., Yu, C. E., Bird, T. D., and Tsuang, D. W. (2010). Review article: genetics of Alzheimer disease. J. Geriatr. Psychiatry Neurol. 23, 213–227. doi: 10.1177/0891988710383571
Bertram, L., Lange, C., Mullin, K., Parkinson, M., Hsiao, M., Hogan, M. F., et al. (2008). Genome-wide association analysis reveals putative Alzheimer's disease susceptibility loci in addition to APOE. Am. J. Hum. Genet. 83, 623–632. doi: 10.1016/j.ajhg.2008.10.008
Bich, L., Mossio, M., Ruiz-Mirazo, K., and Moreno, A. (2016). Biological regulation: controlling the system from within. Biol. Philos. 31, 237–265. doi: 10.1007/s10539-015-9497-8
Bierer, L. M., Patrick, R., Purohit, D. P., Carlin, L., Schmeidler, J., Davis, K. L., et al. (1995). Neocortical neurofibrillary tangles correlate with dementia severity in Alzheimer's disease. Arch. Neurol. 52, 81–88. doi: 10.1001/archneur.1995.00540250089017
Bindea, G., Mlecnik, B., Hackl, H., Charoentong, P., Tosolini, M., Kirilovsky, A., et al. (2009). ClueGO: a cytoscape plug-in to decipher functionally grouped gene ontology and pathway annotation networks. Bioinformatics 25, 1091–1093. doi: 10.1093/bioinformatics/btp101
Binns, D., Dimmer, E., Huntley, R., Barrell, D., O'Donovan, C., and Apweiler, R. (2009). QuickGO: a web-based tool for Gene Ontology searching. Bioinformatics 25, 3045–3046. doi: 10.1093/bioinformatics/btp536
Blennow, K., Hampel, H., Weiner, M., and Zetterberg, H. (2010). Cerebrospinal fluid and plasma biomarkers in Alzheimer disease. Nat. Rev. Neurol. 6, 131–144. doi: 10.1038/nrneurol.2010.4
Blind, R. D. (2020). Structural analyses of inositol phosphate second messengers bound to signaling effector proteins. Adv. Biol. Regul. 75:100667. doi: 10.1016/j.jbior.2019.100667
Braunewell, K. H., and Szanto, A. J. K. (2009). Visinin-like proteins (VSNLs): Interaction partners and emerging functions in signal transduction of a subfamily of neuronal Ca2+-sensor proteins. Cell Tissue Res. 335, 301–316. doi: 10.1007/s00441-008-0716-3
Breitner, J. C. S., Folstein, M. F., and Murphy, E. A. (1986). Familial aggregation in Alzheimer dementia-I. A model for the age-dependent expression of an autosomal dominant gene. J. Psychiatr. Res. 20, 31–43. doi: 10.1016/0022-3956(86)90021-X
Broce, I. J., Tan, C. H., Fan, C. C., Jansen, I., Savage, J. E., Witoelar, A., et al. (2019). Dissecting the genetic relationship between cardiovascular risk factors and Alzheimer's disease. Acta Neuropathol. 137, 209–226. doi: 10.1007/s00401-018-1928-6
Brookmeyer, R., Johnson, E., Ziegler-Graham, K., and Arrighi, H. M. (2007). Forecasting the global burden of Alzheimer's disease. Alzheimer's Dement. 3, 186–191. doi: 10.1016/j.jalz.2007.04.381
Buniello, A., Macarthur, J. A. L., Cerezo, M., Harris, L. W., Hayhurst, J., Malangone, C., et al. (2019). The NHGRI-EBI GWAS Catalog of published genome-wide association studies, targeted arrays and summary statistics 2019. Nucleic Acids Res. 47, D1005–D1012. doi: 10.1093/nar/gky1120
Buschert, V. C., Friese, U., Teipel, S. J., Schneider, P., Merensky, W., Rujescu, D., et al. (2011). Effects of a newly developed cognitive intervention in amnestic mild cognitive impairment and mild alzheimer's disease: A pilot study. J. Alzheimer's Dis. 25, 679–694. doi: 10.3233/JAD-2011-100999
Calderari, S., Ria, M., Gérard, C., Nogueira, T. C., Villate, O., Collins, S. C., et al. (2018). Molecular genetics of the transcription factor GLIS3 identifies its dual function in beta cells and neurons. Genomics 110, 98–111. doi: 10.1016/j.ygeno.2017.09.001
Campbell, I. L., Hobbs, M. V., Dockter, J., Oldstone, M. B. A., and Allison, J. (1994). Islet inflammation and hyperplasia induced by the pancreatic islet- specific overexpression of interleukin-6 in transgenic mice. Am. J. Pathol. 145, 157–166.
Cao, M. Y., Davidson, D., Yu, J., Latour, S., and Veillette, A. (1999). Clnk, a Novel SLP-76 – related adaptor molecule expressed in cytokine-stimulated Hemopoietic Cells. 190, 1527–1534. doi: 10.1084/jem.190.10.1527
Carrasquillo, M. M., Zou, F., Pankratz, V. S., Wilcox, S. L., Ma, L., Walker, L. P., et al. (2009). Genetic variation in PCDH11X is associated with susceptibility to late-onset Alzheimer's disease. Nat. Genet. 41, 192–198. doi: 10.1038/ng.305
Carretero, M., Ruiz-Torres, M., Rodríguez-Corsino, M., Barthelemy, I., and Losada, A. (2013). Pds5B is required for cohesion establishment and Aurora B accumulation at centromeres. EMBO J. 32, 2938–2949. doi: 10.1038/emboj.2013.230
Chen, C. S., Chen, N. J., Lin, L. W., Hsieh, C. C., Chen, G. W., and Hsieh, M. T. (2006). Effects of Scutellariae Radix on gene expression in HEK 293 cells using cDNA microarray. J. Ethnopharmacol. 105, 346–351. doi: 10.1016/j.jep.2005.11.012
Chen, J., Yu, J. T., Wojta, K., Wang, H. F., Zetterberg, H., Blennow, K., et al. (2017). Genome-wide association study identifies MAPT locus influencing human plasma tau levels. Neurology 88, 669–676. doi: 10.1212/WNL.0000000000003615
Chowdhury, D., Xu, X., Zhong, X., Ahmed, F., Zhong, J., Liao, J., et al. (2008). A PP4-phosphatase complex dephosphorylates γ-H2AX generated during DNA replication. Mol. Cell 31, 33–46. doi: 10.1016/j.molcel.2008.05.016
Christopher, L., Napolioni, V., Khan, R. R., Han, S. S., and Greicius, M. D. (2017). A variant in PPP4R3A protects against alzheimer-related metabolic decline. Ann. Neurol. 82, 900–911. doi: 10.1002/ana.25094
Chung, J., Wang, X., Maruyama, T., Ma, Y., Zhang, X., Mez, J., et al. (2018a). Genome-wide association study of Alzheimer's disease endophenotypes at prediagnosis stages. Alzheimer's Dement. 14, 623–633. doi: 10.1016/j.jalz.2017.11.006
Chung, J., Zhang, X., Allen, M., Wang, X., Ma, Y., Beecham, G., et al. (2018b). Genome-wide pleiotropy analysis of neuropathological traits related to Alzheimer's disease. Alzheimers. Res. Ther. 10. doi: 10.1186/s13195-018-0349-z
Colacurcio, D. J., and Nixon, R. A. (2016). Disorders of lysosomal acidification—the emerging role of v-ATPase in aging and neurodegenerative disease. Ageing Res. Rev. 32, 75–88. doi: 10.1016/j.arr.2016.05.004
Cook, K. G., Bradford, A. P., Yeaman, S. J., Aitken, A., Fearnley, I. M., and Walker, J. E. (1984). Regulation of bovine kidney branched-chain 2-oxoacid dehydrogenase complex by reversible phosphorylation. Eur. J. Biochem. 145, 587–591. doi: 10.1111/j.1432-1033.1984.tb08597.x
Coon, K. D., Myers, A. J., Craig, D. W., Webster, J. A., Pearson, J. V., Lince, D. H., et al. (2007). A high-density whole-genome association study reveals that APOE is the major susceptibility gene for sporadic late-onset Alzheimer's disease. J. Clin. Psychiatry 68, 613–618. doi: 10.4088/JCP.v68n0419
Corder, E. H., Saunders, A. M., Risch, N. J., Strittmatter, W. J., Schmechel, D. E., Gaskell, P. C., et al. (1994). Protective effect of apolipoprotein E type 2 allele for late onset Alzheimer disease. Nat. Genet. 7, 180–184. doi: 10.1038/ng0694-180
Corder, E. H., Saunders, A. M., Strittmatter, W. J., Schmechel, D. E., Gaskell, P. C., Small, G. W., et al. (1993). Gene dose of apolipoprotein E type 4 allele and the risk of Alzheimer's disease in late onset families. Science 261, 921–923. doi: 10.1126/science.8346443
Crocker, P. R., Paulson, J. C., and Varki, A. (2007). Siglecs and their roles in the immune system. Nat. Rev. Immunol. 7, 255–266. doi: 10.1038/nri2056
Cruchaga, C., Kauwe, J. S. K., Harari, O., Jin, S. C., Cai, Y., Karch, C. M., et al. (2013). GWAS of cerebrospinal fluid tau levels identifies risk variants for Alzheimer's disease. Neuron 78, 256–268. doi: 10.1016/j.neuron.2013.02.026
Cullinan, E. B., Kwee, L., Nunes, P., Shuster, D. J., Ju, G., McIntyre, K. W., et al. (1998). IL-1 receptor accessory protein is an essential component of the IL-1 receptor. J. Immunol. 161, 5614–5620.
Cummings, J. L., Doody, R., and Clark, C. (2007). Disease-modifying therapies for Alzheimer disease: challenges to early intervention. Neurology 69, 1622–1634. doi: 10.1212/01.wnl.0000295996.54210.69
Darvesh, S., Hopkins, D. A., and Geula, C. (2003). Neurobiology of butyrylcholinesterase. Nat. Rev. Neurosci. 4, 131–138. doi: 10.1038/nrn1035
Das, B., and Yan, R. (2017). Role of BACE1 in Alzheimer's synaptic function. Transl. Neurodegener. 6, 4–11. doi: 10.1186/s40035-017-0093-5
Davatzikos, C., Bhatt, P., Shaw, L. M., Batmanghelich, K. N., and Trojanowski, J. Q. (2011). Prediction of MCI to AD conversion, via MRI, CSF biomarkers, and pattern classification. Neurobiol. Aging 32. doi: 10.1016/j.neurobiolaging.2010.05.023
Davies, G., Harris, S. E., Reynolds, C. A., Payton, A., Knight, H. M., Liewald, D. C., et al. (2014). A genome-wide association study implicates the APOE locus in nonpathological cognitive ageing. Mol. Psychiatry 19, 76–87. doi: 10.1038/mp.2012.159
Davies-Fleischer, K.aren M., and Besner, G. E. (1998). Structure and function of Heparin-binding EGF-like growth factor (HB-EGF). Front. Biosci. 3, 288–299. doi: 10.2741/A241
De Jager, P. L., Shulman, J. M., Chibnik, L. B., Keenan, B. T., Raj, T., Wilson, R. S., et al. (2012). A genome-wide scan for common variants affecting the rate of age-related cognitive decline. Neurobiol. Aging 33, 1017.e1–15. doi: 10.1016/j.neurobiolaging.2011.09.033
Deb, S., and Gottschall, P. E. (2002). Increased production of matrix metalloproteinases in enriched astrocyte and mixed hippocampal cultures treated with β-amyloid peptides. J. Neurochem. 66, 1641–1647. doi: 10.1046/j.1471-4159.1996.66041641.x
Deming, Y., Li, Z., Kapoor, M., Harari, O., Del-Aguila, J. L., Black, K., et al. (2017). Genome-wide association study identifies four novel loci associated with Alzheimer's endophenotypes and disease modifiers. Acta Neuropathol. 133, 839–856. doi: 10.1007/s00401-017-1685-y
Deneka, A., Korobeynikov, V., and Golemis, E. A. (2015). Embryonal Fyn-associated substrate (EFS) and CASS4: the lesser-known CAS protein family members. Gene 570, 25–35. doi: 10.1016/j.gene.2015.06.062
Desvignes, T., Pontarotti, P., and Bobe, J. (2010). Nme gene family evolutionary history reveals pre-metazoan origins and high conservation between humans and the sea anemone, nematostella vectensis. PLoS ONE 5:e15506. doi: 10.1371/annotation/ad419d55-e0bd-4a2e-a79e-5265f44ae545
Deters, K. D., Nho, K., Risacher, S. L., Kim, S., Ramanan, V. K., Crane, P. K., et al. (2017). Genome-wide association study of language performance in Alzheimer's disease. Brain Lang. 172, 22–29. doi: 10.1016/j.bandl.2017.04.008
Devi, L., Prabhu, B. M., Galati, D. F., Avadhani, N. G., and Anandatheerthavarada, H. K. (2006). Accumulation of amyloid precursor protein in the mitochondrial import channels of human Alzheimer's disease brain is associated with mitochondrial dysfunction. J. Neurosci. 26, 9057–9068. doi: 10.1523/JNEUROSCI.1469-06.2006
Di Paolo, G., and Kim, T. W. (2011). Linking lipids to Alzheimer's disease: cholesterol and beyond. Nat. Rev. Neurosci. 12, 284–296. doi: 10.1038/nrn3012
Dietz, M. L., Bernaciak, T. M., Vendetti, F., Kielec, J. M., and Hildebrand, J. D. (2006). Differential actin-dependent localization modulates the evolutionarily conserved activity of Shroom family proteins. J. Biol. Chem. 281, 20542–20554. doi: 10.1074/jbc.M512463200
Dinarello, C. A. (2009). Immunological and inflammatory functions of the interleukin-1 family. Annu. Rev. Immunol. 27, 519–550. doi: 10.1146/annurev.immunol.021908.132612
Dobolyi, A., Bag,ó, A. G., Gál, A., Molnár, M. J., Palkovits, M., Adam-Vizi, V., et al. (2014). Localization of SUCLA2 and SUCLG2 subunits of succinyl CoA ligase within the cerebral cortex suggests the absence of matrix substrate-level phosphorylation in glial cells of the human brain. J. Bioenerg. Biomembr. 47, 33–41. doi: 10.1007/s10863-014-9586-4
Draber, P., Vonkova, I., Stepanek, O., Hrdinka, M., Kucova, M., Skopcova, T., et al. (2011). SCIMP, a transmembrane adaptor protein involved in major histocompatibility complex class II signaling. Mol. Cell. Biol. 31, 4550–4562. doi: 10.1128/MCB.05817-11
Du, Y., Dodel, R. C., Eastwood, B. J., Bales, K. R., Gao, F., Lohmüller, F., et al. (2000). Association of an interleukin 1α polymorphism with Alzheimer's disease. Neurology 55, 480–483. doi: 10.1212/WNL.55.4.480
Duan, J., Li, X., Huang, S., Zeng, Y., He, Y., Liu, H., et al. (2018). GOLPH2, a gene downstream of Ras signaling, promotes the progression of pancreatic ductal adenocarcinoma. Mol. Med. Rep. 17, 4187–4194. doi: 10.3892/mmr.2018.8430
Dubois, B., Hampel, H., Feldman, H. H., Scheltens, P., Aisen, P., Andrieu, S., et al. (2016). Preclinical Alzheimer's disease: definition, natural history, and diagnostic criteria. Alzheimer's Dement. 12, 292–323. doi: 10.1016/j.jalz.2016.02.002
Dumitrescu, L., Barnes, L. L., Thambisetty, M., Beecham, G., Kunkle, B., Bush, W. S., et al. (2019). Sex differences in the genetic predictors of Alzheimer's pathology. Brain 142, 2581–2589. doi: 10.1093/brain/awz206
Ebert, K., Ludwig, M., Geillinger, K. E., Schoberth, G. C., Essenwanger, J., Stolz, J., et al. (2017). Reassessment of GLUT7 and GLUT9 as putative fructose and glucose transporters. J. Membr. Biol. 250, 171–182. doi: 10.1007/s00232-016-9945-7
Efimova, E. V., Al-Zoubi, A. M., Martinez, O., Kaithamana, S., Lu, S., Arima, T., et al. (2004). IG20, in contrast to DENN-SV, (MADD splice variants) suppresses tumor cell survival, and enhances their susceptibility to apoptosis and cancer drugs. Oncogene 23, 1076–1087. doi: 10.1038/sj.onc.1207210
Efthymiou, A. G., and Goate, A. M. (2017). Late onset Alzheimer's disease genetics implicates microglial pathways in disease risk. Mol. Neurodegener. 12, 1–12. doi: 10.1186/s13024-017-0184-x
Fagerberg, L., Hallstrom, B. M., Oksvold, P., Kampf, C., Djureinovic, D., Odeberg, J., et al. (2014). Analysis of the human tissue-specific expression by genome-wide integration of transcriptomics and antibody-based proteomics. Mol. Cell. Proteom. 13, 397–406. doi: 10.1074/mcp.M113.035600
Farley, K., Stolley, J. M., Zhao, P., Cooley, J., and Remold-O'Donnell, E. (2012). A SerpinB1 regulatory mechanism is essential for restricting NETosis. J. Immunol. 189, 4574–4581. doi: 10.4049/jimmunol.1201167
Feulner, T. M., Laws, S. M., Friedrich, P., Wagenpfeil, S., Wurst, S. H. R., Riehle, C., et al. (2010). Examination of the current top candidate genes for AD in a genome-wide association study. Mol. Psychiatry 15, 756–766. doi: 10.1038/mp.2008.141
Friedmann, E., Hauben, E., Maylandt, K., Schleeger, S., Vreugde, S., Lichtenthaler, S. F., et al. (2006). SPPL2a and SPPL2b promote intramembrane proteolysis of TNFα in activated dendritic cells to trigger IL-12 production. Nat. Cell Biol. 8, 843–848. doi: 10.1038/ncb1440
Gallo, J. M., and Spickett, C. (2010). The role of CELF proteins in neurological disorders. RNA Biol. 7, 474–479. doi: 10.4161/rna.7.4.12345
Gao, L., Emond, M. J., Louie, T., Cheadle, C., Berger, A. E., Rafaels, N., et al. (2016). Identification of rare variants in ATP8B4 as a risk factor for systemic sclerosis by whole-exome sequencing. Arthritis Rheumatol. 68, 191–200. doi: 10.1002/art.39449
García-Alba, J., Ramírez-Toraño, F., Esteba-Castillo, S., Bruña, R., Moldenhauer, F., Novell, R., et al. (2019). Neuropsychological and neurophysiological characterization of mild cognitive impairment and Alzheimer's disease in Down syndrome. Neurobiol. Aging 84, 70–79. doi: 10.1016/j.neurobiolaging.2019.07.017
Gatz, M., Fratiglioni, L., Johansson, B., Berg, S., Mortimer, J. A., Reynolds, C. A., et al. (2005). Complete ascertainment of dementia in the Swedish Twin Registry: the HARMONY study. Neurobiol. Aging 26, 439–447. doi: 10.1016/j.neurobiolaging.2004.04.004
Gentilini, D., Scala, S., Gaudenzi, G., Garagnani, P., Capri, M., Cescon, M., et al. (2017). Epigenome-wide association study in hepatocellular carcinoma: identification of stochastic epigenetic mutations through an innovative statistical approach. Oncotarget 8, 41890–41902. doi: 10.18632/oncotarget.17462
Giannakopoulos, P., Herrmann, F. R., Bussière, T., Bouras, C., Kövari, E., Perl, D. P., et al. (2003). Tangle and neuron numbers, but not amyloid load, predict cognitive status in Alzheimer's disease. Neurology 60, 1495–1500. doi: 10.1212/01.WNL.0000063311.58879.01
Gibson, C. L., Codreanu, S. G., Schrimpe-Rutledge, A. C., Retzlaff, C. L., Wright, J., Mortlock, D. P., et al. (2018). Global untargeted serum metabolomic analyses nominate metabolic pathways responsive to loss of expression of the orphan metallo β-lactamase, MBLAC1. Mol. Omi. 14, 142–155. doi: 10.1039/C7MO00022G
Golde, T. E., Eckman, C. B., and Younkin, S. G. (2000). Biochemical detection of Aβ isoforms: implications for pathogenesis, diagnosis, and treatment of Alzheimer's disease. Biochim. Biophys. Acta Mol. Basis Dis. 1502, 172–187. doi: 10.1016/S0925-4439(00)00043-0
Gomez-Isla, T., Hollister, R., West, H., Mui, S., Growdon, J. H., Petersen, R. C., et al. (1997). Neuronal loss correlates with but exceeds neurofibrillary tangles in Alzheimer's disease. Ann. Neurol. 41, 17–24. doi: 10.1002/ana.410410106
Gottschalk, W. K., Lutz, M. W., He, Y. T., Saunders, A. M., Daniel, K., Roses, A. D., et al. (2014). The broad impact of TOM40 on neurodegenerative diseases in aging. J. Park. Dis. Alzheimer's Dis. 1:12. doi: 10.13188/2376-922X.1000003
Grimaldi, L. M. E., Casadei, V. M., Ferri, C., Veglia, F., Licastro, F., Annoni, G., et al. (2000). Association of early-onset Alzheimer's disease with an interleukin-1? gene polymorphism. Ann. Neurol. 47, 361–365. doi: 10.1002/1531-8249(200003)47:3<361::AID-ANA12>3.0.CO;2-N
Gronostajski, R. M. (2000). Roles of the NFI/CTF gene family in transcription and development. Gene 249, 31–45. doi: 10.1016/S0378-1119(00)00140-2
Grupe, A., Abraham, R., Li, Y., Rowland, C., Hollingworth, P., Morgan, A., et al. (2007). Evidence for novel susceptibility genes for late-onset Alzheimer's disease from a genome-wide association study of putative functional variants. Hum. Mol. Genet. 16, 865–873. doi: 10.1093/hmg/ddm031
Gusareva, E. S., Carrasquillo, M. M., Bellenguez, C., Cuyvers, E., Colon, S., Graff-Radford, N. R., et al. (2014). Genome-wide association interaction analysis for Alzheimer's disease. Neurobiol. Aging 35, 2436–2443. doi: 10.1016/j.neurobiolaging.2014.05.014
Haddick, P. C. G., Larson, J. L., Rathore, N., Bhangale, T. R., Phung, Q. T., Srinivasan, K., et al. (2017). A common variant of IL-6R is associated with elevated IL-6 pathway activity in Alzheimer's disease brains. J. Alzheimer's Dis. 56, 1037–1054. doi: 10.3233/JAD-160524
Harold, D., Abraham, R., Hollingworth, P., Sims, R., Hamshere, M., Pahwa, J. S., et al. (2009). Genome-wide association study identifies variants at CLU and PICALM associated with Alzheimer's disease, and shows evidence for additional susceptibility genes. Nat. Genet. 41, 1088–1093. doi: 10.1038/ng.440
He, F., Umehara, T., Saito, K., Harada, T., Watanabe, S., Yabuki, T., et al. (2010). Structural insight into the zinc finger CW domain as a histone modification reader. Structure 18, 1127–1139. doi: 10.1016/j.str.2010.06.012
Hebert, L. E., Weuve, J., Scherr, P. A., and Evans, D. A. (2013). Alzheimer disease in the United States (2010-2050) estimated using the 2010 census. Neurology 80, 1778–1783. doi: 10.1212/WNL.0b013e31828726f5
Henderson, M. C., Gonzales, I. M., Arora, S., Choudhary, A., Trent, J. M., Von Hoff, D. D., et al. (2011). High-throughput RNAi screening identifies a role for TNK1 in growth and survival of pancreatic cancer cells. Mol. Cancer Res. 9, 724–732. doi: 10.1158/1541-7786.MCR-10-0436
Heppner, F. L., Ransohoff, R. M., and Becher, B. (2015). Immune attack: the role of inflammation in Alzheimer disease. Nat. Rev. Neurosci. 16, 358–372. doi: 10.1038/nrn3880
Hermes, M., Eichhoff, G., and Garaschuk, O. (2010). Intracellular calcium signalling in Alzheimer's disease. J. Cell. Mol. Med. 14, 30–41. doi: 10.1111/j.1582-4934.2009.00976.x
Herold, C., Hooli, B. V., Mullin, K., Liu, T., Roehr, J. T., Mattheisen, M., et al. (2016). Family-based association analyses of imputed genotypes reveal genome-wide significant association of Alzheimer's disease with OSBPL6, PTPRG, and PDCL3. Mol. Psychiatry 21, 1608–1612. doi: 10.1038/mp.2015.218
Hinney, A., Albayrak, Ö., Antel, J., Volckmar, A. L., Sims, R., Chapman, J., et al. (2014). Genetic variation at the CELF1 (CUGBP, elav-like family member 1 gene) locus is genome-wide associated with Alzheimer's disease and obesity. Am. J. Med. Genet. B Neuropsychiatr. Genet. 165, 283–293. doi: 10.1002/ajmg.b.32234
Hirano, A., Ohara, T., Takahashi, A., Aoki, M., Fuyuno, Y., Ashikawa, K., et al. (2015). A genome-wide association study of late-onset Alzheimer's disease in a Japanese population. Psychiatr. Genet. 25, 139–146. doi: 10.1097/YPG.0000000000000090
Hojjati, S. H., Ebrahimzadeh, A., Khazaee, A., and Babajani-Feremi, A. (2018). Predicting conversion from MCI to AD by integrating rs-fMRI and structural MRI. Comput. Biol. Med. 102, 30–39. doi: 10.1016/j.compbiomed.2018.09.004
Hollingworth, P., Harold, D., Sims, R., Gerrish, A., Lambert, J. C., Carrasquillo, M. M., et al. (2011). Common variants at ABCA7, MS4A6A/MS4A4E, EPHA1, CD33 and CD2AP are associated with Alzheimer's disease. Nat. Genet. 43, 429–436. doi: 10.1038/ng.803
Hollingworth, P., Sweet, R., Sims, R., Harold, D., Russo, G., Abraham, R., et al. (2012). Genome-wide association study of Alzheimer's disease with psychotic symptoms. Mol. Psychiatry 17, 1316–1327. doi: 10.1038/mp.2011.125
Höng, J. C., Ivanov, N. V., Hodor, P., Xia, M., Wei, N., Blevins, R., et al. (2004). Identification of new human cadherin genes using a combination of protein motif search and gene finding methods. J. Mol. Biol. 337, 307–317. doi: 10.1016/j.jmb.2004.01.026
Horbinski, C., and Chu, C. T. (2005). Kinase signaling cascades in the mitochondrion: a matter of life or death. Free Radic. Biol. Med. 38, 2–11. doi: 10.1016/j.freeradbiomed.2004.09.030
Hou, W., Izadi, M., Nemitz, S., Haag, N., Kessels, M. M., and Qualmann, B. (2015). The actin nucleator cobl is controlled by calcium and calmodulin. PLoS Biol. 13:1002233. doi: 10.1371/journal.pbio.1002233
Hou, X. H., Bi, Y. L., Tan, M. S., Xu, W., Li, J. Q., Shen, X. N., et al. (2019). Genome-wide association study identifies Alzheimer's risk variant in MS4A6A influencing cerebrospinal fluid sTREM2 levels. Neurobiol. Aging 84, 241.e13–241.e20. doi: 10.1016/j.neurobiolaging.2019.05.008
Hu, C., Chen, W., Myers, S. J., Yuan, H., and Traynelis, S. F. (2016). Human GRIN2B variants in neurodevelopmental disorders. J. Pharmacol. Sci. 132, 115–121. doi: 10.1016/j.jphs.2016.10.002
Hu, H., Li, H., Li, J., Yu, J., and Tan, L. (2018). Genome-wide association study identified ATP6V1H locus influencing cerebrospinal fluid BACE activity. BMC Med. Genet. 19:75. doi: 10.1186/s12881-018-0603-z
Huang, D. W., Sherman, B. T., and Lempicki, R. A. (2009). Systematic and integrative analysis of large gene lists using DAVID bioinformatics resources. Nat. Protoc. 4, 44–57. doi: 10.1038/nprot.2008.211
Huang, J., Xiao, L., Gong, X., Shao, W., Yin, Y., Liao, Q., et al. (2014). Cytokine-like molecule CCDC134 contributes to CD8+ T-cell effector functions in cancer immunotherapy. Cancer Res. 74, 5734–5745. doi: 10.1158/0008-5472.CAN-13-3132
Huang, K. L., Marcora, E., Pimenova, A. A., Di Narzo, A. F., Kapoor, M., Jin, S. C., et al. (2017a). A common haplotype lowers PU.1 expression in myeloid cells and delays onset of Alzheimer's disease. Nat. Neurosci. 20, 1052–1061. doi: 10.1038/nn.4587
Huang, M., Deng, C., Yu, Y., Lian, T., Yang, W., and Feng, Q. (2019). Spatial correlations exploitation based on nonlocal voxel-wise GWAS for biomarker detection of AD. NeuroImage Clin. 21:101642. doi: 10.1016/j.nicl.2018.101642
Huang, Q., and Xiang, Y. (2018). Polymorphisms in selected genes and their association with age-related macular degeneration in a Chinese population. Med. Sci. Monit. 24, 1693–1700. doi: 10.12659/MSM.906298
Huang, Y., Zheng, J., Chen, D., Li, F., Wu, W., Huang, X., et al. (2017b). Transcriptome profiling identifies a recurrent CRYL1-IFT88 chimeric transcript in hepatocellular carcinoma. Oncotarget 8, 40693–40704. doi: 10.18632/oncotarget.17244
Hussain, M. R. M., Hoessli, D. C., and Fang, M. (2016). N-acetylgalactosaminyltransferases in cancer. Oncotarget 7, 54067–54081. doi: 10.18632/oncotarget.10042
Ikenouchi, J., and Umeda, M. (2010). FRMD4A regulates epithelial polarity by connecting Arf6 activation with the PAR complex. Proc. Natl. Acad. Sci. U. S. A. 107, 748–753. doi: 10.1073/pnas.0908423107
Insolera, R., Chen, S., and Shi, S. H. (2011). Par proteins and neuronal polarity. Dev. Neurobiol. 71, 483–494. doi: 10.1002/dneu.20867
Jansen, I. E., Savage, J. E., Watanabe, K., Bryois, J., Williams, D. M., Steinberg, S., et al. (2019). Genome-wide meta-analysis identifies new loci and functional pathways influencing Alzheimer's disease risk. Nat. Genet. 51, 404–413. doi: 10.1038/s41588-018-0311-9
Jiang, T., Yu, J. T., Hu, N., Tan, M. S., Zhu, X. C., and Tan, L. (2014). CD33 in alzheimer's disease. Mol. Neurobiol. 49, 529–535. doi: 10.1007/s12035-013-8536-1
Johnson, J. D., Mehus, J. G., Tews, K., Milavetz, B. I., and Lambeth, D. O. (1998). Genetic evidence for the expression of ATP- and GTP-specific succinyl- CoA synthetases in multicellular eucaryotes. J. Biol. Chem. 273, 27580–27586. doi: 10.1074/jbc.273.42.27580
Jonsson, T., Stefansson, H., Steinberg, S., Jonsdottir, I., Jonsson, P. V., Snaedal, J., et al. (2013). Variant of TREM2 associated with the risk of Alzheimer's disease. N. Engl. J. Med. 368, 107–116. doi: 10.1056/NEJMoa1211103
Jorissen, E., Prox, J., Bernreuther, C., Weber, S., Schwanbeck, R., Serneels, L., et al. (2010). The disintegrin/metalloproteinase ADAM10 is essential for the establishment of the brain cortex. J. Neurosci. 30, 4833–4844. doi: 10.1523/JNEUROSCI.5221-09.2010
Jouanne, M., Rault, S., and Voisin-Chiret, A. S. (2017). Tau protein aggregation in Alzheimer's disease: an attractive target for the development of novel therapeutic agents. Eur. J. Med. Chem. 139, 153–167. doi: 10.1016/j.ejmech.2017.07.070
Jun, G., Ibrahim-Verbaas, C. A., Vronskaya, M., Lambert, J. C., Chung, J., Naj, A. C., et al. (2016). A novel Alzheimer disease locus located near the gene encoding tau protein. Mol. Psychiatry 21, 108–117. doi: 10.1038/mp.2015.23
Jun, G. R., Chung, J., Logue, M. W., Sherva, R., Farrer, L. A., Mez, J., et al. (2017). Transethnic genome-wide scan identifies novel Alzheimer's disease loci. Alzheimer's Dement. 13, 727–738. doi: 10.1016/j.jalz.2016.12.012
Kamboh, M. I., Barmada, M. M., Demirci, F. Y., Minster, R. L., Carrasquillo, M. M., Pankratz, V. S., et al. (2012a). Genome-wide association analysis of age-at-onset in Alzheimer's disease. Mol. Psychiatry 17, 1340–1346. doi: 10.1038/mp.2011.135
Kamboh, M. I., Demirci, F. Y., Wang, X., Minster, R. L., Carrasquillo, M. M., Pankratz, V. S., et al. (2012b). Genome-wide association study of Alzheimer's disease. Transl. Psychiatry 2, 1–7. doi: 10.1038/tp.2012.45
Karch, C. M., and Goate, A. M. (2015). Alzheimer's disease risk genes and mechanisms of disease pathogenesis. Biol. Psychiatry 77, 43–51. doi: 10.1016/j.biopsych.2014.05.006
Kauwe, J. S. K., Bailey, M. H., Ridge, P. G., Perry, R., Wadsworth, M. E., Hoyt, K. L., et al. (2014). Genome-wide association study of CSF levels of 59 Alzheimer's disease candidate proteins: significant associations with proteins involved in amyloid processing and inflammation. PLoS Genet. 10:e1004758. doi: 10.1371/journal.pgen.1004758
Kawas, C., Gray, S., Brookmeyer, R., Fozard, J., and Zonderman, A. (2000). Age-specific incidence rates of Alzheimer's disease: the Baltimore longitudinal study of aging. Neurology 54, 2072–2077. doi: 10.1212/WNL.54.11.2072
Kehoe, P. G. (2018). The coming of age of the angiotensin hypothesis in Alzheimer's disease: progress toward disease prevention and treatment? J. Alzheimer's Dis. 62, 1443–1466. doi: 10.3233/JAD-171119
Kim, S., Swaminathan, S., Shen, L., Risacher, S. L., Nho, K., Foroud, T., et al. (2011). Genome-wide association study of CSF biomarkers Aβ1-42, t-tau, and p-tau181p in the ADNI cohort. Neurology 76, 69–79. doi: 10.1212/WNL.0b013e318204a397
Kim, S. W., Cho, T., and Lee, S. (2015). Phospholipase C-β1 hypofunction in the pathogenesis of schizophrenia. Front. Psychiatry 6:159. doi: 10.3389/fpsyt.2015.00159
Kim, Y. S., Nakanishi, G., Lewandoski, M., and Jetten, A. M. (2003). GLIS3, a novel member of the GLIS subfamily of Krüppel-like zinc finger proteins with repressor and activation functions. Nucleic Acids Res. 31, 5513–5525. doi: 10.1093/nar/gkg776
Kitazume, S., Tachida, Y., Oka, R., Shirotani, K., Saido, T. C., and Hashimoto, Y. (2001). Alzheimer's β-secretase, β-site amyloid precursor protein-cleaving enzyme, is responsible for cleavage secretion of a Golgi-resident sialyltransferase. Proc. Natl. Acad. Sci. U. S. A. 98, 13554–13559. doi: 10.1073/pnas.241509198
Klesney-Tait, J., Turnbull, I. R., and Colonna, M. (2006). The TREM receptor family and signal integration. Nat. Immunol. 7, 1266–1273. doi: 10.1038/ni1411
Kocahan, S., and Doğan, Z. (2017). Mechanisms of Alzheimer's disease pathogenesis and prevention: the brain, neural pathology, N-methyl-D-Aspartate receptors, tau protein and other risk factors. Clin. Psychopharmacol. Neurosci. 15, 1–8. doi: 10.9758/cpn.2017.15.1.1
Kong, L.-L., Miao, D., Tan, L., Liu, S.-L., Li, J.-Q., Cao, X.-P., et al. (2018). Genome-wide association study identifies RBFOX1 locus influencing brain glucose metabolism. Ann. Transl. Med. 6:5. doi: 10.21037/atm.2018.07.05
Kordeli, E., Lambert, S., and Bennett, V. (1995). Ankyrin(G). A new ankyrin gene with neural-specific isoforms localized at the axonal initial segment and node of Ranvier. J. Biol. Chem. 270, 2352–2359. doi: 10.1074/jbc.270.5.2352
Kriebs, A., Jordan, S. D., Soto, E., Henriksson, E., Sandate, C. R., Vaughan, M. E., et al. (2017). Circadian repressors CRY1 and CRY2 broadly interact with nuclear receptors and modulate transcriptional activity. Proc. Natl. Acad. Sci. U. S. A. 114, 8776–8781. doi: 10.1073/pnas.1704955114
Kudryavtseva, A. V., Lukyanova, E. N., Kharitonov, S. L., Nyushko, K. M., Krasheninnikov, A. A., Pudova, E. A., et al. (2019). Bioinformatic identification of differentially expressed genes associated with prognosis of locally advanced lymph node-positive prostate cancer. J. Bioinform. Comput. Biol. 17:33. doi: 10.1142/S0219720019500033
Kuhn, P. H., Wang, H., Dislich, B., Colombo, A., Zeitschel, U., Ellwart, J. W., et al. (2010). ADAM10 is the physiologically relevant, constitutive α-secretase of the amyloid precursor protein in primary neurons. EMBO J. 29, 3020–3032. doi: 10.1038/emboj.2010.167
Kujuro, Y., Suzuki, N., and Kondo, T. (2010). Esophageal cancer-related gene 4 is a secreted inducer of cell senescence expressed by aged CNS precursor cells. Proc. Natl. Acad. Sci. U. S. A. 107, 8259–8264. doi: 10.1073/pnas.0911446107
Kunkle, B. W., Grenier-Boley, B., Sims, R., Bis, J. C., Damotte, V., Naj, A. C., et al. (2019). Genetic meta-analysis of diagnosed Alzheimer's disease identifies new risk loci and implicates Aβ, tau, immunity and lipid processing. Nat. Genet. 51, 414–430. doi: 10.1038/s41588-019-0358-2
LaBonte, M. L. (2014). Anticoagulant factor V: factors affecting the integration of novel scientific discoveries into the broader framework. Stud. Hist. Philos. Sci. Part C Stud. Hist. Philos. Biol. Biomed. Sci. 47, 23–34. doi: 10.1016/j.shpsc.2014.03.007
LaFerla, F. M. (2002). Calcium dyshomeostasis and intracellular signalling in alzheimer's disease. Nat. Rev. Neurosci. 3, 862–872. doi: 10.1038/nrn960
Lai-Cheong, J. E., Parsons, M., and McGrath, J. A. (2010). The role of kindlins in cell biology and relevance to human disease. Int. J. Biochem. Cell Biol. 42, 595–603. doi: 10.1016/j.biocel.2009.10.015
Lambert, J. C., Grenier-Boley, B., Harold, D., Zelenika, D., Chouraki, V., Kamatani, Y., et al. (2013a). Genome-wide haplotype association study identifies the FRMD4A gene as a risk locus for Alzheimer's disease. Mol. Psychiatry 18, 461–470. doi: 10.1038/mp.2012.14
Lambert, J. C., Heath, S., Even, G., Campion, D., Sleegers, K., Hiltunen, M., et al. (2009). Genome-wide association study identifies variants at CLU and CR1 associated with Alzheimer's disease. Nat. Genet. 41, 1094–1099. doi: 10.1038/ng.439
Lambert, J. C., Ibrahim-Verbaas, C. A., Harold, D., Naj, A. C., Sims, R., Bellenguez, C., et al. (2013b). Meta-analysis of 74,046 individuals identifies 11 new susceptibility loci for Alzheimer's disease. Nat. Genet. 45, 1452–1458. doi: 10.1038/ng.2802
Langa, K. M., and Levine, D. A. (2014). The diagnosis and management of mild cognitive impairment: a clinical review. J. Am. Med. Assoc. 312, 2551–2561. doi: 10.1001/jama.2014.13806
Larsson, M., Duffy, D. L., Zhu, G., Liu, J. Z., MacGregor, S., McRae, A. F., et al. (2011). GWAS findings for human iris patterns: associations with variants in genes that influence normal neuronal pattern development. Am. J. Hum. Genet. 89, 334–343. doi: 10.1016/j.ajhg.2011.07.011
Lash, L. H., and Cummings, B. S. (2010). Mechanisms of toxicant-induced acute kidney injury. Compr. Toxicol. Second Ed. 7, 81–115. doi: 10.1016/B978-0-08-046884-6.00804-6
Laumet, G., Chouraki, V., Grenier-Boley, B., Legry, V., Heath, S., Zelenika, D., et al. (2010). Systematic analysis of candidate genes for Alzheimer's disease in a French, genome-wide association study. J. Alzheimer's Dis. 20, 1181–1188. doi: 10.3233/JAD-2010-100126
Lavelin, I., and Geiger, B. (2005). Characterization of a novel GTPase-activating protein associated with focal adhesions and the actin cytoskeleton. J. Biol. Chem. 280, 7178–7185. doi: 10.1074/jbc.M411990200
Lee, E., Giovanello, K. S., Saykin, A. J., Xie, F., Kong, D., Wang, Y., et al. (2017). Single-nucleotide polymorphisms are associated with cognitive decline at Alzheimer's disease conversion within mild cognitive impairment patients. Alzheimer's Dement. 8, 86–95. doi: 10.1016/j.dadm.2017.04.004
Lesage, S., Drouet, V., Majounie, E., Deramecourt, V., Jacoupy, M., Nicolas, A., et al. (2016). Loss of VPS13C function in autosomal-recessive Parkinsonism causes mitochondrial dysfunction and increases PINK1/parkin-dependent mitophagy. Am. J. Hum. Genet. 98, 500–513. doi: 10.1016/j.ajhg.2016.01.014
Li, H., Wetten, S., St. Jean, P. L., Upmanyu, R., Surh, L., Hosford, D., et al. (2008a). Candidate single-nucleotide polymorphisms from a genomewide association study of Alzheimer disease. Arch. Neurol. 65, 45–53. doi: 10.1001/archneurol.2007.3
Li, J., Zhang, Q., Chen, F., Meng, X., Liu, W., Chen, D., et al. (2017). Genome-wide association and interaction studies of CSF T-tau/Aβ 42 ratio in ADNI cohort. Neurobiol. Aging 57, 247.e1–247.e8. doi: 10.1016/j.neurobiolaging.2017.05.007
Li, J.-Q., Yuan, X.-Z., Li, H.-Y., Cao, X.-P., Yu, J.-T., Tan, L., et al. (2018). Genome-wide association study identifies two loci influencing plasma neurofilament light levels. BMC Med. Genom. 11:8. doi: 10.1186/s12920-018-0364-8
Li, Q. S., Parrado, A. R., Samtani, M. N., and Narayan, V. A. (2015). Variations in the FRA10AC1 fragile site and 15q21 are associated with cerebrospinal fluid Aβ1-42 level. PLoS ONE 10:e0134000. doi: 10.1371/journal.pone.0134000
Li, X. F., Kraev, A. S., and Lytton, J. (2002). Molecular cloning of a fourth member of the potassium-dependent sodium-calcium exchanger gene family, NCKX4. J. Biol. Chem. 277, 48410–48417. doi: 10.1074/jbc.M210011200
Li, Y., Rowland, C., Catanese, J., Morris, J., Lovestone, S., O'Donovan, M. C., et al. (2008b). SORL1 variants and risk of late-onset Alzheimer's disease. Neurobiol. Dis. 29, 293–296. doi: 10.1016/j.nbd.2007.09.001
Lien, M. Y., Lin, C. W., Tsai, H. C., Chen, Y. T., Tsai, M. H., Hua, C. H., et al. (2017). Impact of CCL4 gene polymorphisms and environmental factors on oral cancer development and clinical characteristics. Oncotarget 8, 31424–31434. doi: 10.18632/oncotarget.15615
Lin, H., Lee, E., Hestir, K., Leo, C., Huang, M., Bosch, E., et al. (2008). Discovery of a cytokine and its receptor by functional screening of the extracellular proteome. Science 707, 807–811. doi: 10.1126/science.1154370
Liu, L., and Chan, C. (2014). The role of inflammasome in Alzheimer's disease. Ageing Res. Rev. 15, 6–15. doi: 10.1016/j.arr.2013.12.007
Liu, S. L., Wang, X. C., Tan, M. S., Wang, H. F., Zhang, W., Wang, Z. X., et al. (2016). NME8 rs2718058 polymorphism with Alzheimer's disease risk: a replication and meta-analysis. Oncotarget 7, 36014–36020. doi: 10.18632/oncotarget.9086
Logue, M. W., Schu, M., Vardarajan, B. N., Buros, J., Green, R. C., Go, R. C. P., et al. (2011). A comprehensive genetic association study of Alzheimer disease in African Americans. Arch. Neurol. 68, 1569–1579. doi: 10.1001/archneurol.2011.646
Love, M. W., Craddock, A. L., Angelin, B., Brunzell, J. D., Duane, W. C., and Dawson, P. A. (2001). Analysis of the ileal bile acid transporter gene, SLC10A2, in subjects with familial hypertriglyceridemia. Arterioscler. Thromb. Vasc. Biol. 21, 2039–2045. doi: 10.1161/hq1201.100262
Luo, J., Chimge, N. O., Zhou, B., Flodby, P., Castaldi, A., Firth, A. L., et al. (2018). CLDN18.1 attenuates malignancy and related signaling pathways of lung adenocarcinoma in vivo and in vitro. Int. J. Cancer 143, 3169–3180. doi: 10.1002/ijc.31734
Ma, J., Yu, J. T., and Tan, L. (2015). MS4A cluster in Alzheimer's disease. Mol. Neurobiol. 51, 1240–1248. doi: 10.1007/s12035-014-8800-z
Madeo, J., and Frieri, M. (2013). Alzheimer's disease and immunotherapy. Aging Dis. 4, 210–220. doi: 10.2174/1567205043332126
Mahley, R. W. (1988). Apolipoprotein E : cholesterol transport protein with expanding role in cell biology. Science 240, 622–630. doi: 10.1126/science.3283935
Mahley, R. W., and Rall, S. C. (2000). A POLIPOPROTEIN E: far more than a lipid transport protein. Annu. Rev. Genomics Hum. Genet. 1, 507–537. doi: 10.1146/annurev.genom.1.1.507
Malouf, G. G., Su, X., Yao, H., Gao, J., Xiong, L., He, Q., et al. (2014). Next-generation sequencing of translocation renal cell carcinoma reveals novel RNA splicing partners and frequent mutations of chromatin-remodeling Genes. Clin. Cancer Res. 20, 4129–4140. doi: 10.1158/1078-0432.CCR-13-3036
Marambaud, P., Dreses-Werringloer, U., and Vingtdeux, V. (2009). Calcium signaling in neurodegeneration. Mol. Neurodegener. 4:20. doi: 10.1186/1750-1326-4-20
Marioni, R. E., Harris, S. E., Zhang, Q., McRae, A. F., Hagenaars, S. P., Hill, W. D., et al. (2018). GWAS on family history of Alzheimer's disease. Transl. Psychiatry 8:6. doi: 10.1038/s41398-018-0150-6
Marshansky, V., Rubinstein, J. L., and Grüber, G. (2014). Eukaryotic V-ATPase: novel structural findings and functional insights. Biochim. Biophys. Acta. 1837, 857–879. doi: 10.1016/j.bbabio.2014.01.018
Masters, C. L., Bateman, R., Blennow, K., Rowe, C. C., Sperling, R. A., and Cummings, J. L. (2015). Alzheimer's disease. Nat. Rev. Dis. Prim. 1, 1–18. doi: 10.1038/nrdp.2015.56
Matsuda, F., Ishii, K., Bourvagnet, P., Kuma, K. I., Hayashida, H., Miyata, T., et al. (1998). The complete nucleotide sequence of the human immunoglobulin heavy chain variable region locus. J. Exp. Med. 188, 2151–2162. doi: 10.1084/jem.188.11.2151
Mayeux, R., and Stern, Y. (2012). Epidemiology of Alzheimer disease. Cold Spring Harb. Perspect. Med. 2:a006239. doi: 10.1101/cshperspect.a006239
Meda, S. A., Narayanan, B., Liu, J., Perrone-Bizzozero, N. I., Stevens, M. C., Calhoun, V. D., et al. (2012). A large scale multivariate parallel ICA method reveals novel imaging-genetic relationships for Alzheimer's disease in the ADNI cohort. Neuroimage 60, 1608–1621. doi: 10.1016/j.neuroimage.2011.12.076
Melville, S. A., Buros, J., Parrado, A. R., Vardarajan, B., Logue, M. W., Shen, L., et al. (2012). Multiple loci influencing hippocampal degeneration identified by genome scan. Ann. Neurol. 72, 65–75. doi: 10.1002/ana.23644
Mesner, L. D., Calabrese, G. M., Al-Barghouthi, B., Gatti, D. M., Sundberg, J. P., Churchill, G. A., et al. (2019). Mouse genome-wide association and systems genetics identifies Lhfp as a regulator of bone mass. PLoS Genet. 15:e1008123. doi: 10.1371/journal.pgen.1008123
Meyer, M. R., Tshanz, J. T., Norton, M. C., Welsh-Bohmer, K. A., Steffens, D. C., Wyse, B. W., et al. (1998). APOE genotype predicts when — not whether — one is predisposed to develop Alzheimer disease. Nat. Genet. 19, 321–322. doi: 10.1038/1206
Mez, J., Chung, J., Jun, G., Kriegel, J., Bourlas, A. P., Sherva, R., et al. (2017). Two novel loci, COBL and SLC10A2, for Alzheimer's disease in African Americans. Alzheimer's Dement. 13, 119–129. doi: 10.1016/j.jalz.2016.09.002
Milardi, D., Colussi, C., Grande, G., Vincenzoni, F., Pierconti, F., Mancini, F., et al. (2018). Olfactory receptors in semen and in the male tract: from proteome to proteins. Front. Endocrinol. 8:379. doi: 10.3389/fendo.2017.00379
Miron, J., Picard, C., Nilsson, N., Frappier, J., Dea, D., Théroux, L., et al. (2018). CDK5RAP2 gene and tau pathophysiology in late-onset sporadic Alzheimer's disease. Alzheimer's Dement. 14, 787–796. doi: 10.1016/j.jalz.2017.12.004
Miyashita, A., Koike, A., Jun, G., Wang, L. S., Takahashi, S., Matsubara, E., et al. (2013). SORL1 is genetically associated with late-onset Alzheimer's disease in Japanese, Koreans and Caucasians. PLoS ONE 8:e58618. doi: 10.1371/annotation/fcb56ea7-d32a-4e45-818d-39cef330c731
Mizuno, T., Doi, Y., Mizoguchi, H., Jin, S., Noda, M., Sonobe, Y., et al. (2011). Interleukin-34 selectively enhances the neuroprotective effects of microglia to attenuate oligomeric amyloid-β neurotoxicity. Am. J. Pathol. 179, 2016–2027. doi: 10.1016/j.ajpath.2011.06.011
Moody, D. B., Young, D. C., Cheng, T. Y., Rosat, J. P., Roura-Mir, C., O'Connor, P. B., et al. (2004). T cell activation by lipopeptide antigens. Science 303, 527–531. doi: 10.1126/science.1089353
Moraes, L., Zanchin, N. I. T., and Cerutti, J. M. (2017). ABI3, a component of the WAVE2 complex, is potentially regulated by PI3K/AKT pathway. Oncotarget 8, 67769–67781. doi: 10.18632/oncotarget.18840
Moreno-Grau, S., de Rojas, I., Hernández, I., Quintela, I., Montrreal, L., Alegret, M., et al. (2019). Genome-wide association analysis of dementia and its clinical endophenotypes reveal novel loci associated with Alzheimer's disease and three causality networks: The GR@ACE project. Alzheimer's Dement. 15, 1333–1347. doi: 10.1016/j.jalz.2019.06.4950
Naj, A. C., Jun, G., Beecham, G. W., Wang, L., Narayan, B., Buros, J., et al. (2011). Common variants in MS4A4/MS4A6E, CD2uAP, CD33, and EPHA1 are associated with late-onset Alzheimer ' s disease. Nat. Genet. Genet. 43, 436–441. doi: 10.1038/ng.801
Naj, A. C., Jun, G., Reitz, C., Kunkle, B. W., Perry, W., Park, Y. S., et al. (2014). Effects of multiple genetic loci on age at onset in late-onset Alzheimer disease: a genome-wide association study. JAMA Neurol. 71, 1394–1404. doi: 10.1001/jamaneurol.2014.1491
Naj, A. C., and Schellenberg, G. D. (2017). Genomic variants, genes, and pathways of Alzheimer's disease: an overview. Am. J. Med. Genet. B Neuropsychiatr. Genet. 174, 5–26. doi: 10.1002/ajmg.b.32499
Nakagawa, K., Kitazume, S., Oka, R., Maruyama, K., Saido, T. C., Sato, Y., et al. (2006). Sialylation enhances the secretion of neurotoxic amyloid-β peptides. J. Neurochem. 96, 924–933. doi: 10.1111/j.1471-4159.2005.03595.x
Namba, Y., and Ikeda, K. (1991). Apolipoprotein B immunoreactivity in cerebal amyloid deposits and neurofibrillary tangles in senile dementia of Alzheimer type. Clin. Neurol. 31, 826–830.
Neumann, H., and Daly, M. J. (2013). Variant TREM2 as Risk Factor for Alzhei mer's Disease. N. Engl. J. Med. 368, 182–184. doi: 10.1056/NEJMe1213157
Nicoll, J. A. R., Mrak, R. E., Graham, D. I., Stewart, J., Wilcock, G., MacGowan, S., et al. (2000). Association of interleukin-1 gene polymorphisms with Alzheimer's disease. Ann. Neurol. 47, 365–368. doi: 10.1002/1531-8249(200003)47:3<365::AID-ANA13>3.0.CO;2-G
Nishinaka, N., Hongo, S., Zhou, C. J., Shioda, S., Takahashi, R., Yamauchi, Y., et al. (2000). Identification of the novel developmentally regulated gene, Bdm2, which is highly expressed in fetal rat brain. Dev. Brain Res. 120, 57–64. doi: 10.1016/S0165-3806(99)00191-1
Nishiya, T., Matsumoto, K., Maekawa, S., Kajita, E., Horinouchi, T., Fujimuro, M., et al. (2011). Regulation of inducible nitric-oxide synthase by the SPRY domain- and SOCS box-containing proteins. J. Biol. Chem. 286, 9009–9019. doi: 10.1074/jbc.M110.190678
Numata, M., and Orlowski, J. (2001). Molecular cloning and characterization of a novel (Na+,K +)/H+ exchanger localized to the trans-Golgi Network. J. Biol. Chem. 276, 17387–17394. doi: 10.1074/jbc.M101319200
Oda, T., Kujovich, J., Reis, M., Newman, B., and Druker, B. J. (1997). Identification and characterization of two novel SH2 domain-containing proteins from a yeast two hybrid screen with the ABL tyrosine kinase. Oncogene 15, 1255–1262. doi: 10.1038/sj.onc.1201299
Oguchi, M. E., Etoh, K., and Fukuda, M. (2018). Rab20, a novel Rab small GTPase that negatively regulates neurite outgrowth of PC12 cells. Neurosci. Lett. 662, 324–330. doi: 10.1016/j.neulet.2017.10.056
Olazaran, J., Muniz, R., Reisberg, B., Pena-Casanova, J., del Ser, T., Cruz-Jentoft, A. J., et al. (2004). Benefits of cognitive-motor intervention in MCI and mild to moderate Alzheimer disease. Neurology 63, 2348–2353. doi: 10.1212/01.WNL.0000147478.03911.28
Olgiati, P., Politis, A. M., Papadimitriou, G. N., De Ronchi, D., and Serretti, A. (2011). Genetics of late-onset Alzheimer's disease: update from the Alzgene database and analysis of shared pathways. Int. J. Alzheimers. Dis. 2011:832379. doi: 10.4061/2011/832379
Oyagi, A., and Hara, H. (2012). Essential roles of heparin-binding epidermal growth factor-like growth factor in the brain. CNS Neurosci. Ther. 18, 803–810. doi: 10.1111/j.1755-5949.2012.00371.x
Pahnke, J., Langer, O., and Krohn, M. (2014). Alzheimer's and ABC transporters - new opportunities for diagnostics and treatment. Neurobiol. Dis. 72, 54–60. doi: 10.1016/j.nbd.2014.04.001
Papassotiropoulos, A., Hock, C., and Nitsch, R. M. (2001). Genetics of interleukin 6: implications for Alzheimer's disease. Neurobiol. Aging 22, 863–871. doi: 10.1016/S0197-4580(01)00294-9
Parsons, S. F., Mallinson, G., Holmes, C. H., Houlihan, J. M., Simpson, K. L., Mawby, W. J., et al. (1995). The Lutheran blood group glycoprotein, another member of the immunoglobulin superfamily, is widely expressed in human tissues and is developmentally regulated in human liver. Proc. Natl. Acad. Sci. U. S. A. 92, 5496–5500. doi: 10.1073/pnas.92.12.5496
Pasqualato, S., Renault, L., and Cherfils, J. (2002). Arf, Arl, Arp and Sar proteins: a family of GTP-binding proteins with a structural device for “front-back” communication. EMBO Rep. 3, 1035–1041. doi: 10.1093/embo-reports/kvf221
Patrick, G. N., Zukerberg, L., Nikolic, M., De La Monte, S., Dikkes, P., and Tsai, L. H. (1999). Conversion of p35 to p25 deregulates Cdk5 activity and promotes neurodegeneration. Nature 402, 615–622. doi: 10.1038/45159
Patterson, R. L., Van Rossum, D. B., Ford, D. L., Hurt, K. J., Bae, S. S., Suh, P. G., et al. (2002). Phospholipase C-γ is required for agonist-induced Ca2+ entry. Cell 111, 529–541. doi: 10.1016/S0092-8674(02)01045-0
Pedersen, N. L., Gatz, M., Berg, S., and Johansson, B. (2004). How heritable is Alzheimer's disease late in life? Findings from Swedish Twins. Ann. Neurol. 55, 180–185. doi: 10.1002/ana.10999
Pérez-Palma, E., Bustos, B. I., Villamán, C. F., Alarcón, M. A., Avila, M. E., Ugarte, G. D., et al. (2014). Overrepresentation of glutamate signaling in Alzheimer's disease: network-based pathway enrichment using meta-analysis of genome-wide association studies. PLoS ONE 9:e95413. doi: 10.1371/journal.pone.0095413
Peter, V., and Walter, F. (1991). Is amyloidogenesis during Alzheimer's disease due to an IL-1-/IL-6-mediated “acute phase response” in the brain? Immunol. Today 12, 217–219. doi: 10.1016/0167-5699(91)90032-O
Petersen, R. C., Smith, G. E., Waring, S. C., Ivnik, R. J., Tangalos, E. G., and Kokmen, E. (1999). Mild cognitive impairment: clinical characterization and outcome. Arch. Neurol. 56, 303–308. doi: 10.1001/archneur.56.3.303
Pimplikar, S. W. (2009). Reassessing the amyloid cascade hypothesis of Alzheimer's disease. Int. J. Biochem. Cell Biol. 41, 1261–1268. doi: 10.1016/j.biocel.2008.12.015
Pirone, D. M., Fukuhara, S., Gutkind, J. S., and Burbelo, P. D. (2000). SPECs, small binding proteins for Cdc42. J. Biol. Chem. 275, 22650–22656. doi: 10.1074/jbc.M002832200
Podvin, S., Miller, M. C., Rossi, R., Chukwueke, J., Donahue, J. E., Johanson, C. E., et al. (2016). The orphan C2orf40 gene is a neuroimmune factor in Alzheimer's disease. JSM Alzheimer's Dis. Relat. Dement. 3:1020.
Ponthier, J. L., Schluepen, C., Chen, W., Lersch, R. A., Gee, S. L., Hou, V. C., et al. (2006). Fox-2 splicing factor binds to a conserved intron motif to promote inclusion of protein 4.1R alternative exon 16. J. Biol. Chem. 281, 12468–12474. doi: 10.1074/jbc.M511556200
Postina, R., Schroeder, A., Dewachter, I., Bohl, J., Schmitt, U., Kojiro, E., et al. (2004). A disintegrin-metalloproteinase prevents amyloid plaque formation and hippocampal defects in an Alzheimer disease mouse model. J. Clin. Invest. 113, 1456–1464. doi: 10.1172/JCI20864
Prince, M. (2015). World Alzheimer Report. Alzheimer's Dis. Int. Available online at: https://www.alz.co.uk/research/WorldAlzheimerReport2015.pdf (accessed November 11, 2020).
Ramanan, V. K., Risacher, S. L., Nho, K., Kim, S., Shen, L., McDonald, B. C., et al. (2015). GWAS of longitudinal amyloid accumulation on 18F-florbetapir PET in Alzheimer's disease implicates microglial activation gene IL1RAP. Brain 138, 3076–3088. doi: 10.1093/brain/awv231
Ramanan, V. K., Risacher, S. L., Nho, K., Kim, S., Swaminathan, S., Shen, L., et al. (2014). APOE and BCHE as modulators of cerebral amyloid deposition: a florbetapir PET genome-wide association study. Mol. Psychiatry 19, 351–357. doi: 10.1038/mp.2013.19
Ramirez, A., van der Flier, W. M., Herold, C., Ramonet, D., Heilmann, S., Lewczuk, P., et al. (2014). SUCLG2 identified as both a determinator of CSF Aβ1-42 levels and an attenuator of cognitive decline in Alzheimer's disease. Hum. Mol. Genet. 23, 6644–6658. doi: 10.1093/hmg/ddu372
Raspotnig, M., Haugen, M., Thorsteinsdottir, M., Stefansson, I., Salvesen, H. B., Storstein, A., et al. (2017). Cerebellar degeneration-related proteins 2 and 2-like are present in ovarian cancer in patients with and without Yo antibodies. Cancer Immunol. Immunother. 66, 1463–1471. doi: 10.1007/s00262-017-2041-8
Reitz, C., Cheng, R., Rogaeva, E., Lee, J. H., Tokuhiro, S., Zou, F., et al. (2011). Meta-analysis of the association between variants in SORL1 and Alzheimer disease. Arch. Neurol. 68, 99–106. doi: 10.1001/archneurol.2010.346
Reitz, C., Jun, G., Naj, A., Rajbhandary, R., Vardarajan, B. N., Wang, L. S., et al. (2013). Variants in the ATP-binding cassette transporter (ABCA7), apolipoprotein e ε4, and the risk of late-onset Alzheimer disease in African Americans. J. Am. Med. Assoc. 309, 1483–1492. doi: 10.1001/jama.2013.2973
Ries, M., and Sastre, M. (2016). Mechanisms of Aβ clearance and degradation by glial cells. Front. Aging Neurosci. 8:160. doi: 10.3389/fnagi.2016.00160
Rizzi, L., Rosset, I., and Roriz-Cruz, M. (2014). Global epidemiology of dementia: Alzheimer's and vascular types. Biomed Res. Int. 2014:908915. doi: 10.1155/2014/908915
Robinson, J. K., Bartfai, T., and Langel, Ü. (2006). Galanin / GALP receptors and CNS homeostatic processes. CNS Neurol. Disord. Drug Targets 5, 327–334. doi: 10.2174/187152706777452281
Rodrigues-Campos, M., and Thompson, B. J. (2014). The ubiquitin ligase fbxl7 regulates the dachsous-fat-dachs system in drosophila. Dev. 141, 4098–4103. doi: 10.1242/dev.113498
Rosenberg, R. N., Lambracht-Washington, D., Yu, G., and Xia, W. (2016). Genomics of Alzheimer disease: a review. JAMA Neurol. 73, 867–874. doi: 10.1001/jamaneurol.2016.0301
Rosenthal, S. L., Bamne, M. N., Wang, X., Berman, S., Snitz, B. E., Klunk, W. E., et al. (2015). More evidence for association of a rare TREM2 mutation (R47H) with Alzheimer's disease risk. Neurobiol. Aging 36, 2443.e21–2443.e26. doi: 10.1016/j.neurobiolaging.2015.04.012
Rosenthal, S. L., and Kamboh, M. I. (2014). Late-onset Alzheimer's disease genes and the potentially implicated pathways. Curr. Genet. Med. Rep. 2, 85–101. doi: 10.1007/s40142-014-0034-x
Roses, A. D., Lutz, M. W., Amrine-Madsen, H., Saunders, A. M., Crenshaw, D. G., Sundseth, S. S., et al. (2010). A TOMM40 variable-length polymorphism predicts the age of late-onset Alzheimer's disease. Pharmacogenomics J. 10, 375–384. doi: 10.1038/tpj.2009.69
Ruiz, A., Heilmann, S., Becker, T., Hernández, I., Wagner, H., Thelen, M., et al. (2014). Follow-up of loci from the International Genomics of Alzheimer's Disease Project identifies TRIP4 as a novel susceptibility gene. Transl. Psychiatry 4, 2–5. doi: 10.1038/tp.2014.2
Saint-Martin, M., Joubert, B., Pellier-Monnin, V., Pascual, O., Noraz, N., and Honnorat, J. (2018). Contactin-associated protein-like 2, a protein of the neurexin family involved in several human diseases. Eur. J. Neurosci. 48, 1906–1923. doi: 10.1111/ejn.14081
Sakai, J., Hoshino, A., Takahashi, S., Miura, Y., Ishii, H., Suzuki, H., et al. (1994). Structure, chromosome location, and expression of the human very low density lipoprotein receptor gene. J. Biol. Chem. 269, 2173–2182. doi: 10.1016/S0021-9258(17)42151-X
Salazar, S. V., Cox, T. O., Lee, S., Brody, A. H., Chyung, A. S., Haas, L. T., et al. (2019). Alzheimer's disease risk factor Pyk2 mediates amyloid-β-induced synaptic dysfunction and loss. J. Neurosci. 39, 758–772. doi: 10.1523/JNEUROSCI.1873-18.2018
Sarafidou, T., Kahl, C., Martinez-Garay, I., Mangelsdorf, M., Gesk, S., Baker, E., et al. (2004). Folate-sensitive fragile site FRA10A is due to an expansion of a CGG repeat in a novel gene, FRA10AC1, encoding a nuclear protein. Genomics 84, 69–81. doi: 10.1016/j.ygeno.2003.12.017
Satoh, J. I., Kino, Y., Yanaizu, M., Tosaki, Y., Sakai, K., Ishida, T., et al. (2017). Microglia express ABI3 in the brains of Alzheimer's disease and Nasu-Hakola disease. Intractable Rare Dis. Res. 6, 262–268. doi: 10.5582/irdr.2017.01073
Satoh, K., Abe-Dohmae, S., Yokoyama, S., St. George-Hyslop, P., and Fraser, P. E. (2015). ATP-binding cassette transporter A7 (ABCA7) loss of function alters Alzheimer amyloid processing. J. Biol. Chem. 290, 24152–24165. doi: 10.1074/jbc.M115.655076
Saunders, A. M., Strittmatter, W. J., Schmechel, D., St George-Hyslop, P. H., Pericak-Vance, M. A., Joo, S. H., et al. (1993). Association of apolipoprotein E allele ε4 with late-onset sporadic Alzheimer's disease. Neurology 43, 1467–1472. doi: 10.1212/WNL.43.8.1467
Schubert, D. (1997). Serpins inhibit the toxicity of amyloid peptides. Eur. J. Neurosci. 9, 770–777. doi: 10.1111/j.1460-9568.1997.tb01425.x
Seaton, G., Hogg, E. L., Jo, J., Whitcomb, D. J., and Cho, K. (2011). Sensing change: the emerging role of calcium sensors in neuronal disease. Semin. Cell Dev. Biol. 22, 530–535. doi: 10.1016/j.semcdb.2011.07.014
Seripa, D., Panza, F., Paroni, G., D'Onofrio, G., Bisceglia, P., Gravina, C., et al. (2018). Role of CLU, PICALM, and TNK1 genotypes in aging with and without Alzheimer's disease. Mol. Neurobiol. 55, 4333–4344. doi: 10.1007/s12035-017-0547-x
Seshadri, S., Fitzpatrick, A. L., Ikram, M. A., DeStefano, A. L., Gudnason, V., Boada, M., et al. (2010). Genome-wide analysis of genetic loci associated with Alzheimer disease. J. Am. Med. Assoc. 303, 1832–1840. doi: 10.1001/jama.2010.574
Seux, M., Peuget, S., Montero, M. P., Siret, C., Rigot, V., Clerc, P., et al. (2011). TP53INP1 decreases pancreatic cancer cell migration by regulating SPARC expression. Oncogene 30, 3049–3061. doi: 10.1038/onc.2011.25
Sharma, N. K., Key, C. C. C., Civelek, M., Wabitsch, M., Comeau, M. E., Langefeld, C. D., et al. (2019). Genetic regulation of Enoyl-CoA hydratase domain-containing 3 in adipose tissue determines insulin sensitivity in African Americans and Europeans. Diabetes 68, 1508–1522. doi: 10.2337/db18-1229
Shen, L., Kim, S., Risacher, S. L., Nho, K., Swaminathan, S., West, J. D., et al. (2010). Whole genome association study of brain-wide imaging phenotypes for identifying quantitative trait loci in MCI and AD: a study of the ADNI cohort. Neuroimage 53, 1051–1063. doi: 10.1016/j.neuroimage.2010.01.042
Sims, R., Van Der Lee, S. J., Naj, A. C., Bellenguez, C., Badarinarayan, N., Jakobsdottir, J., et al. (2017). Rare coding variants in PLCG2, ABI3, and TREM2 implicate microglial-mediated innate immunity in Alzheimer's disease. Nat. Genet. 49, 1373–1384. doi: 10.1038/ng.3916
Slattery, C. F., Beck, J. A., Harper, L., Adamson, G., Abdi, Z., Uphill, J., et al. (2014). R47H TREM2 variant increases risk of typical early-onset Alzheimer's disease but not of prion or frontotemporal dementia. Alzheimer's Dement. 10, 602–608.e4. doi: 10.1016/j.jalz.2014.05.1751
Smirnikhina, S. A., Lavrov, A. V., Chelysheva, E. Y., Adilgereeva, E. P., Shukhov, O. A., Turkina, A., et al. (2016). Whole-exome sequencing reveals potential molecular predictors of relapse after discontinuation of the targeted therapy in chronic myeloid leukemia patients. Leuk. Lymphoma 57, 1669–1676. doi: 10.3109/10428194.2015.1132420
Smith, E. R., Cayrou, C., Huang, R., Lane, W. S., Cote, J., and Lucchesi, J. C. (2006). A human protein complex homologous to the Drosophila MSL complex is responsible for the majority of histone H4 acetylation at lysine 16. Mol. Cell. Biol. 25, 9175–9188. doi: 10.1128/MCB.25.21.9175-9188.2005
Srinivasan, S., Meyer, R. D., Lugo, R., and Rahimi, N. (2013). Identification of PDCL3 as a novel chaperone protein involved in the generation of functional VEGF receptor 2. J. Biol. Chem. 288, 23171–23181. doi: 10.1074/jbc.M113.473173
Stein, J. L., Hua, X., Morra, J. H., Lee, S., Hibar, D. P., Ho, A. J., et al. (2010). Genome-wide analysis reveals novel genes influencing temporal lobe structure with relevance to neurodegeneration in Alzheimer's disease. Neuroimage 51, 542–554. doi: 10.1016/j.neuroimage.2010.02.068
Steinberg, S., Stefansson, H., Jonsson, T., Johannsdottir, H., Ingason, A., Helgason, H., et al. (2015). Loss-of-function variants in ABCA7 confer risk of Alzheimer's disease. Nat. Genet. 47, 445–447. doi: 10.1038/ng.3246
Stepanov, V., Vagaitseva, K., Bocharova, A., Marusin, A., Markova, V., Minaycheva, L., et al. (2018). Analysis of association of genetic markers in the LUZP2 and FBXO40 genes with the normal variability in cognitive performance in the elderly. Int. J. Alzheimers. Dis. 2018:2686045. doi: 10.1155/2018/2686045
Sugo, N., Oshiro, H., Takemura, M., Kobayashi, T., Kohno, Y., Uesaka, N., et al. (2010). Nucleocytoplasmic translocation of HDAC9 regulates gene expression and dendritic growth in developing cortical neurons. Eur. J. Neurosci. 31, 1521–1532. doi: 10.1111/j.1460-9568.2010.07218.x
Suh, J., Choi, S. H., Romano, D. M., Gannon, M. A., Lesinski, A. N., Kim, D. Y., et al. (2013). ADAM10 missense mutations potentiate β-amyloid accumulation by impairing prodomain chaperone function. Neuron 80, 385–401. doi: 10.1016/j.neuron.2013.08.035
Sun, Z., Van De Giessen, M., Lelieveldt, B. P. F., and Staring, M. (2017). Detection of conversion from mild cognitive impairment to Alzheimer's disease using longitudinal brain MRI. Front. Neuroinform. 11, 1–16. doi: 10.3389/fninf.2017.00016
Takousis, P., Sadlon, A., Schulz, J., Wohlers, I., Dobricic, V., Middleton, L., et al. (2019). Differential expression of microRNAs in Alzheimer's disease brain, blood, and cerebrospinal fluid. Alzheimer's Dement. 15, 1468–1477. doi: 10.1016/j.jalz.2019.06.4952
Tan, L., Yu, J. T., Zhang, W., Wu, Z. C., Zhang, Q., Liu, Q. Y., et al. (2013). Association of GWAS-linked loci with late-onset Alzheimer's disease in a northern Han Chinese population. Alzheimer's Dement. 9, 546–553. doi: 10.1016/j.jalz.2012.08.007
Tanzi, R. E. (2012). The genetics of Alzheimer disease. Cold Spring Harb. Perspect. Med. 2:a006296. doi: 10.1101/cshperspect.a006296
Testa, G., Rossin, D., Poli, G., Biasi, F., and Leonarduzzi, G. (2018). Implication of oxysterols in chronic inflammatory human diseases. Biochimie 153, 220–231. doi: 10.1016/j.biochi.2018.06.006
Thomas, P. Q., and Rathjen, P. D. (1992). HES-1, a novel homeobox gene expressed by murine embryonic stem cells, identifies a new class of homeobox genes. Nucleic Acids Res. 20:5840. doi: 10.1093/nar/20.21.5840
Tonks, N. K. (2006). Protein tyrosine phosphatases: from genes, to function, to disease. Nat. Rev. Mol. Cell Biol. 7, 833–846. doi: 10.1038/nrm2039
Tosto, G., Fu, H., Vardarajan, B. N., Lee, J. H., Cheng, R., Reyes-Dumeyer, D., et al. (2015). F-box/LRR-repeat protein 7 is genetically associated with Alzheimer's disease. Ann. Clin. Transl. Neurol. 2, 810–820. doi: 10.1002/acn3.223
Valotassiou, V., Malamitsi, J., Papatriantafyllou, J., Dardiotis, E., Tsougos, I., Psimadas, D., et al. (2018). SPECT and PET imaging in Alzheimer's disease. Ann. Nucl. Med. 32, 583–593. doi: 10.1007/s12149-018-1292-6
Vitart, V., Rudan, I., Hayward, C., Gray, N. K., Floyd, J., Palmer, C. N. A., et al. (2008). SLC2A9 is a newly identified urate transporter influencing serum urate concentration, urate excretion and gout. Nat. Genet. 40, 437–442. doi: 10.1038/ng.106
Walter, S., Jumpertz, T., Hüttenrauch, M., Ogorek, I., Gerber, H., Storck, S. E., et al. (2019). The metalloprotease ADAMTS4 generates N-truncated Aβ4–x species and marks oligodendrocytes as a source of amyloidogenic peptides in Alzheimer's disease. Acta Neuropathol. 137, 239–257. doi: 10.1007/s00401-018-1929-5
Wang, B., Fallon, J. F., and Beachy, P. A. (2000a). Hedgehog-regulated processing of Gli3 produces an anterior / posterior repressor gradient in the developing vertebrate limb. Cell 100, 423–434. doi: 10.1016/S0092-8674(00)80678-9
Wang, P., Zhao, J., Yang, X., Guan, S., Feng, H., Han, D., et al. (2015). PFDN1, an indicator for colorectal cancer prognosis, enhances tumor cell proliferation and motility through cytoskeletal reorganization. Med. Oncol. 32:710. doi: 10.1007/s12032-015-0710-z
Wang, W., Ishibashi, J., Trefely, S., Shao, M., Cowan, A. J., Sakers, A., et al. (2019a). A PRDM16-driven metabolic signal from adipocytes regulates precursor cell fate. Cell Metab. 30, 174–189.e5. doi: 10.1016/j.cmet.2019.05.005
Wang, X. F., Lin, X., Li, D. Y., Zhou, R., Greenbaum, J., Chen, Y. C., et al. (2017). Linking Alzheimer's disease and type 2 diabetes: novel shared susceptibility genes detected by cFDR approach. J. Neurol. Sci. 380, 262–272. doi: 10.1016/j.jns.2017.07.044
Wang, Y., Sugita, S., and Südhof, T. C. (2000b). The RIM/NIM family of neuronal C2 domain proteins: interactions with Rab3 and a new class of Src homology 3 domain proteins. J. Biol. Chem. 275, 20033–20044. doi: 10.1074/jbc.M909008199
Wang, Z. T., Chen, S. D., Xu, W., Chen, K. L., Wang, H. F., Tan, C. C., et al. (2019b). Genome-wide association study identifies CD1A associated with rate of increase in plasma neurofilament light in non-demented elders. Aging 11, 4521–4535. doi: 10.18632/aging.102066
Webster, J., Reiman, E. M., Zismann, V. L., Joshipura, K. D., Pearson, J. V., Hu-Lince, D., et al. (2010). Whole genome association analysis shows that ACE is a risk factor for Alzheimer's disease and fails to replicate most candidates from meta-analysis. Int. J. Mol. Epidemiol. Genet. 1, 19–30.
Webster, J. A., Myers, A. J., Pearson, J. V., Craig, D. W., Hu-Lince, D., Coon, K. D., et al. (2008). Sorl1 as an Alzheimer's disease predisposition gene? Neurodegener. Dis. 5, 60–64. doi: 10.1159/000110789
Wei, Q., Khan, I. K., Ding, Z., Yerneni, S., and Kihara, D. (2017). NaviGO: interactive tool for visualization and functional similarity and coherence analysis with gene ontology. BMC Bioinform. 18, 1–13. doi: 10.1186/s12859-017-1600-5
Wei, S., Dunn, T. A., Isaacs, W. B., De Marzo, A. M., and Luo, J. (2008). GOLPH2 and MYO6: putative prostate cancer markers localized to the golgi apparatus. Prostate 68, 1387–1395. doi: 10.1002/pros.20806
Wei, X., Wang, X., Xia, Y., Tang, Y., Li, F., Fang, W., et al. (2014). Kindlin-2 regulates renal tubular cell plasticity by activation of Ras and its downstream signaling. Am. J. Physiol. Ren. Physiol. 306, 271–278. doi: 10.1152/ajprenal.00499.2013
Westerterp, M., Berbée, J. F. P., Delsing, D. J. M., Jong, M. C., Gijbels, M. J. J., Dahlmans, V. E. H., et al. (2007). Apolipoprotein C-I binds free fatty acids and reduces their intracellular esterification. J. Lipid Res. 48, 1353–1361. doi: 10.1194/jlr.M700024-JLR200
Whelan, S., Ophir, E., Kotturi, M. F., Levy, O., Ganguly, S., Leung, L., et al. (2019). PVRIG and PVRL2 are induced in cancer and inhibit CD8 + T-cell function. Cancer Immunol. Res. 7, 257–268. doi: 10.1158/2326-6066.CIR-18-0442
White, A. R., Du, T., Laughton, K. M., Volitakis, I., Sharples, R. A., Xilinas, M. E., et al. (2006). Degradation of the Alzheimer disease amyloid β-peptide by metal-dependent up-regulation of metalloprotease activity. J. Biol. Chem. 281, 17670–17680. doi: 10.1074/jbc.M602487200
Whitton, L., Cosgrove, D., Clarkson, C., Harold, D., Kendall, K., Richards, A., et al. (2016). Cognitive analysis of schizophrenia risk genes that function as epigenetic regulators of gene expression. Am. J. Med. Genet. B Neuropsychiatr. Genet. 171, 1170–1179. doi: 10.1002/ajmg.b.32503
Wigge, P., Köhler, K., Vallis, Y., Doyle, C. A., Owen, D., Hunt, S. P., et al. (1997). Amphiphysin heterodimers: potential role in clathrin-mediated endocytosis. Mol. Biol. Cell 8, 2003–2015. doi: 10.1091/mbc.8.10.2003
Wijsman, E. M., Pankratz, N. D., Choi, Y., Rothstein, J. H., Faber, K. M., Cheng, R., et al. (2011). Genome-wide association of familial late-onset Alzheimer's disease replicates BIN1 and CLU and nominates CUGBP2 in interaction with APOE. PLoS Genet. 7:e1001308. doi: 10.1371/journal.pgen.1001308
Williams, T., Borchelt, D. R., and Chakrabarty, P. (2020). Therapeutic approaches targeting Apolipoprotein e function in Alzheimer's disease. Mol. Neurodegener. 15:9. doi: 10.1186/s13024-020-0358-9
Witoelar, A., Rongve, A., Almdahl, I. S., Ulstein, I. D., Engvig, A., White, L. R., et al. (2018). Meta-analysis of Alzheimer's disease on 9,751 samples from Norway and IGAP study identifies four risk loci. Sci. Rep. 8:6. doi: 10.1038/s41598-018-36429-6
Woo, J.-M., Park, S. J., Kang, H., Il Kim, B. G., Shim, S. B., Jee, S. W., et al. (2010). Characterization of changes in global gene expression in the brain of neuron-specific enolase/human Tau23 transgenic mice in response to overexpression of Tau protein. Int. J. Mol. Med. 25, 667–675. doi: 10.3892/ijmm_00000390
Wyss-Coray, T., Yan, F., Lin, A. H.-T., Lambris, J. D., Alexander, J. J., Quigg, R. J., et al. (2002). Prominent neurodegeneration and increased plaque formation in complement-inhibited Alzheimer's mice. Proc. Natl. Acad. Sci. U. S. A. 99, 10837–10842. doi: 10.1073/pnas.162350199
Xia, Z., Chibnik, L. B., Glanz, B. I., Liguori, M., Shulman, J. M., Tran, D., et al. (2010). A putative Alzheimer's disease risk allele in PCK1 influences brain atrophy in multiple sclerosis. PLoS ONE 5:e14169. doi: 10.1371/journal.pone.0014169
Xu, Z., Yoshida, T., Wu, L., Maiti, D., Cebotaru, L., and Duh, E. J. (2015). Transcription factor MEF2C suppresses endothelial cell inflammation via regulation of NF-κB and KLF2. J. Cell. Physiol. 230, 1310–1320. doi: 10.1002/jcp.24870
Yaghmoor, F., Noorsaeed, A., Alsaggaf, S., Aljohani, W., Scholtzova, H., Boutajangout, A., et al. (2014). The role of TREM2 in Alzheimer's disease and other neurological disorders. J. Alzheimer's Dis. Park. 4:160. doi: 10.4172/2161-0460.1000160
Yokoyama, K., Tezuka, T., Kotani, M., Nakazawa, T., Hoshina, N., Shimoda, Y., et al. (2011). NYAP: A phosphoprotein family that links PI3K to WAVE1 signalling in neurons. EMBO J. 30, 4739–4754. doi: 10.1038/emboj.2011.348
Yoshimoto, R., Okawa, K., Yoshida, M., Ohno, M., and Kataoka, N. (2014). Identification of a novel component C2ORF3 in the lariat-intron complex: Lack of C2ORF3 interferes with pre-mRNA splicing via intron turnover pathway. Genes to Cells 19, 78–87. doi: 10.1111/gtc.12114
Yu, D., Hu, J., Sheng, Z., Fu, G., Wang, Y., Chen, Y., et al. (2020). Dual roles of misshapen/NIK-related kinase (MINK1) in osteoarthritis subtypes through the activation of TGFβ signaling. Osteoarthr. Cartil. 28, 112–121. doi: 10.1016/j.joca.2019.09.009
Yuan, H., Rossetto, D., Mellert, H., Dang, W., Srinivasan, M., Johnson, J., et al. (2012). MYST protein acetyltransferase activity requires active site lysine autoacetylation. EMBO J. 31, 58–70. doi: 10.1038/emboj.2011.382
Zajonc, D. M., Elsliger, M. A., Teyton, L., and Wilson, I. A. (2003). Crystal structure of CD1a in complex with a sulfatide self antigen at a resolution of 2.15 Å. Nat. Immunol. 4, 808–815. doi: 10.1038/ni948
Zannis, V. I., Breslow, J. L., Utermann, G., Mahley, R. W., Weisgraber, K. H., Havel, R. J., et al. (1982). Proposed nomenclature of apoE isoproteins, apoE genotypes, and phenotypes. J Lipid Res 23, 911–914. doi: 10.1016/S0022-2275(20)38094-9
Zhang, X., Götte, M., Ibig-Rehm, Y., Schuffenhauer, A., Kamke, M., Beisner, D., et al. (2017). Identification of SPPL2a inhibitors by multiparametric analysis of a high-content ultra-high-throughput screen. SLAS Discov. 22, 1106–1119. doi: 10.1177/2472555217719834
Zheng, H., Liu, C. C., Atagi, Y., Chen, X. F., Jia, L., Yang, L., et al. (2016). Opposing roles of the triggering receptor expressed on myeloid cells 2 and triggering receptor expressed on myeloid cells-like transcript 2 in microglia activation. Neurobiol. Aging 42, 132–141. doi: 10.1016/j.neurobiolaging.2016.03.004
Zhong, X., ling Li, J. Q., Sun, L., Li, Y. Q., Wang, H. F., Cao, X. P., et al. (2019). A genome-wide association study of α-synuclein levels in cerebrospinal fluid. Neurotox. Res. 35, 41–48. doi: 10.1007/s12640-018-9922-2
Zhu, X. C., Yu, J. T., Jiang, T., Wang, P., Cao, L., and Tan, L. (2015). CR1 in Alzheimer's disease. Mol. Neurobiol. 51, 753–765. doi: 10.1007/s12035-014-8723-8
Zhu, Z., Lin, Y., Li, X., Driver, J. A., and Liang, L. (2019). Shared genetic architecture between metabolic traits and Alzheimer's disease: a large-scale genome-wide cross-trait analysis. Hum. Genet. 138, 271–285. doi: 10.1007/s00439-019-01988-9
Zigler, J. S., Hodgkinson, C. A., Wright, M., Klise, A., Sundin, O., Broman, K. W., et al. (2016). A spontaneous missense mutation in branched chain keto acid dehydrogenase kinase in the rat affects both the central and peripheral nervous systems. PLoS ONE 11:e0160447. doi: 10.1371/journal.pone.0160447
Zündorf, G., and Reiser, G. (2011). Calcium dysregulation and homeostasis of neural calcium in the molecular mechanisms of neurodegenerative diseases provide multiple targets for neuroprotection. Antioxidants Redox Signal. 14, 1275–1288. doi: 10.1089/ars.2010.3359
Keywords: Alzheimer's disease, genetics, biomarker, molecular pathways, gene ontology
Citation: Vogrinc D, Goričar K and Dolžan V (2021) Genetic Variability in Molecular Pathways Implicated in Alzheimer's Disease: A Comprehensive Review. Front. Aging Neurosci. 13:646901. doi: 10.3389/fnagi.2021.646901
Received: 28 December 2020; Accepted: 16 February 2021;
Published: 18 March 2021.
Edited by:
Can Martin Zhang, Massachusetts General Hospital and Harvard Medical School, United StatesReviewed by:
Murali Vijayan, Texas Tech University Health Sciences Center, United StatesLiu Shi, University of Oxford, United Kingdom
Dafin F. Muresanu, Iuliu Haţieganu University of Medicine and Pharmacy, Romania
Copyright © 2021 Vogrinc, Goričar and Dolžan. This is an open-access article distributed under the terms of the Creative Commons Attribution License (CC BY). The use, distribution or reproduction in other forums is permitted, provided the original author(s) and the copyright owner(s) are credited and that the original publication in this journal is cited, in accordance with accepted academic practice. No use, distribution or reproduction is permitted which does not comply with these terms.
*Correspondence: Vita Dolžan, dml0YS5kb2x6YW5AbWYudW5pLWxqLnNp