- 1Department of Neurobiology, Care Sciences, and Society, Division of Clinical Geriatrics, Center for Alzheimer Research, Karolinska Institutet (KI), Stockholm, Sweden
- 2Department of Radiology, Mayo Clinic, Rochester, MN, United States
- 3Department of Clinical Psychology, Psychobiology, and Methodology, Faculty of Psychology, University of La Laguna, San Cristóbal de La Laguna, Tenerife, Spain
- 4Department of Neuroimaging, Center for Neuroimaging Sciences, Institute of Psychiatry, Psychology and Neuroscience, King's College London, London, United Kingdom
Subjective cognitive decline (SCD) may be the first sign of Alzheimer's disease (AD), but it can also reflect other pathologies such as cerebrovascular disease or conditions like depressive symptomatology. The role of depressive symptomatology in SCD is controversial. We investigated the association between depressive symptomatology, cerebrovascular disease, and SCD. We recruited 225 cognitively unimpaired individuals from a prospective community-based study [mean age (SD) = 54.64 (10.18); age range 35–77 years; 55% women; 123 individuals with one or more subjective cognitive complaints, 102 individuals with zero complaints]. SCD was assessed with a scale of 9 memory and non-memory subjective complaints. Depressive symptomatology was assessed with established questionnaires. Cerebrovascular disease was assessed with magnetic resonance imaging markers of white matter signal abnormalities (WMSA) and mean diffusivity (MD). We combined correlation, multiple regression, and mediation analyses to investigate the association between depressive symptomatology, cerebrovascular disease, and SCD. We found that SCD was associated with more cerebrovascular disease, older age, and increased depressive symptomatology. In turn, depressive symptomatology was not associated with cerebrovascular disease. Variability in MD was mediated by WMSA burden, presumably reflecting cerebrovascular disease. We conclude that, in our community-based cohort, depressive symptomatology is associated with SCD but not with cerebrovascular disease. In addition, depressive symptomatology did not influence the association between cerebrovascular disease and SCD. We suggest that therapeutic interventions for depressive symptomatology could alleviate the psychological burden of negative emotions in people with SCD, and intervening on vascular risk factors to reduce cerebrovascular disease should be tested as an opportunity to minimize neurodegeneration in SCD individuals from the community.
Introduction
It has been postulated that subjective cognitive decline (SCD) may be the first sign of Alzheimer's disease (AD) (Jessen et al., 2014). However, SCD has also been associated with other pathologies such as cerebrovascular disease (Diniz et al., 2013), especially in community-based studies (Slot et al., 2018). SCD has also been associated with other conditions like depressive symptomatology (Ginó et al., 2010; Zlatar et al., 2014; Cedres et al., 2019). Indeed, the role of depressive symptomatology in current diagnostic criteria of SCD is controversial (Jessen et al., 2014), and it is intensively discussed at the moment (Molinuevo et al., 2017; Rabin et al., 2017; Jessen et al., 2020).
Part of the discussion about the role of depressive symptomatology in SCD stems from the well-known association between depressive symptomatology and SCD (Clarnette et al., 2001; Reid and Maclullich, 2006; Ginó et al., 2010; Zlatar et al., 2014; Cedres et al., 2019). Due to this association, it was traditionally believed that SCD could merely reflect emotional factors (Apolinario et al., 2013; Yates et al., 2015; Burmester et al., 2016). However, there is convincing data showing that depressive symptomatology is a risk factor for future cognitive decline (Butters et al., 2008), or an early symptom of an underlying neurodegenerative disease (Alexopoulos et al., 2013). For example, late-life depression exacerbates the cognitive decline associated with both AD and cerebrovascular disease (Da Silva et al., 2013; Diniz et al., 2013). Also, cerebrovascular disease affects brain networks and causes early depressive symptoms (Murphy et al., 2007; Alexopoulos et al., 2013).
Cerebrovascular disease can be measured through markers assessed on magnetic resonance imaging (MRI) (Wardlaw et al., 2013). A common MRI marker of cerebrovascular disease is white matter signal abnormalities (WMSA), which can be assessed both on T1-weigthed images (white matter hypointensities) and T2-weigthed or fluid-attenuated inversion recovery (FLAIR) images (white matter hyperintensities). Another promising yet unspecific MRI marker is diffusion tensor imaging (DTI), which assesses microstructural alterations in the white matter that might be due to cerebrovascular disease (Zhou et al., 2008; Black et al., 2009; Kennedy and Raz, 2009; Salat et al., 2012). For example, DTI has been proposed as a marker to monitor the progression of cerebrovascular disease (Fu et al., 2012). Both WMSA and DTI alterations have been separately associated with depression (Murphy et al., 2007; Allan et al., 2016) and SCD (Wang et al., 2012; Selnes et al., 2013; Li et al., 2016; Cedres et al., 2019, 2020a; Ohlhauser et al., 2019). However, little is known about the association between cerebrovascular disease, depressive symptomatology, and SCD. This association is especially relevant in SCD individuals from the community, since the prevalence of cerebrovascular disease is significantly higher in community-based cohorts than in clinical cohorts of SCD individuals who seek medical help (Buckley et al., 2017; Slot et al., 2018).
In keeping with the recent contribution from the international working group on SCD (Jessen et al., 2020), the role of depressive symptomatology in SCD still needs to be elucidated (Molinuevo et al., 2017; Rabin et al., 2017). Therefore, the first aim of this study was to investigate the role of depressive symptomatology in SCD in a community-based cohort. We hypothesized three possible scenarios where depressive symptomatology would (A) co-exist with SCD, (B) influence SCD, or (C) reflect SCD (Figure 1). We addressed these hypotheses by combining correlation, multiple regression, and mediation analyses. We wanted to: (A) prove that depressive symptomatology and cerebrovascular disease are independently associated with SCD, but there is no association between depressive symptomatology and cerebrovascular disease (hypothesis: depressive symptomatology co-exists with SCD); (B) depressive symptomatology is associated with cerebrovascular disease and it mediates the association between cerebrovascular disease and SCD (hypothesis: depressive symptomatology influences SCD by mediating the association between cerebrovascular disease and SCD); and (C) SCD mediates the association between cerebrovascular disease and depressive symptomatology (hypothesis: depressive symptomatology reflects SCD). The second aim of this study was to test the hypothesis that variability in the unspecific DTI marker of neurodegeneration would be associated with cerebrovascular disease in our community-based SCD cohort. In addition to correlation analysis, we also used mediation analysis to demonstrate that T1 WMSA burden would mediate the association between DTI abnormalities and SCD. Further, older individuals in our cohort have an increased WMSA burden (Nemy et al., 2020), a higher frequency of SCD (Cedres et al., 2019), and higher levels of depressive symptomatology (Machado et al., 2018). Hence, our third aim was to investigate the effect of aging in our analyses. We hypothesized that DTI abnormalities in SCD are associated with increased WMSA burden and older age.
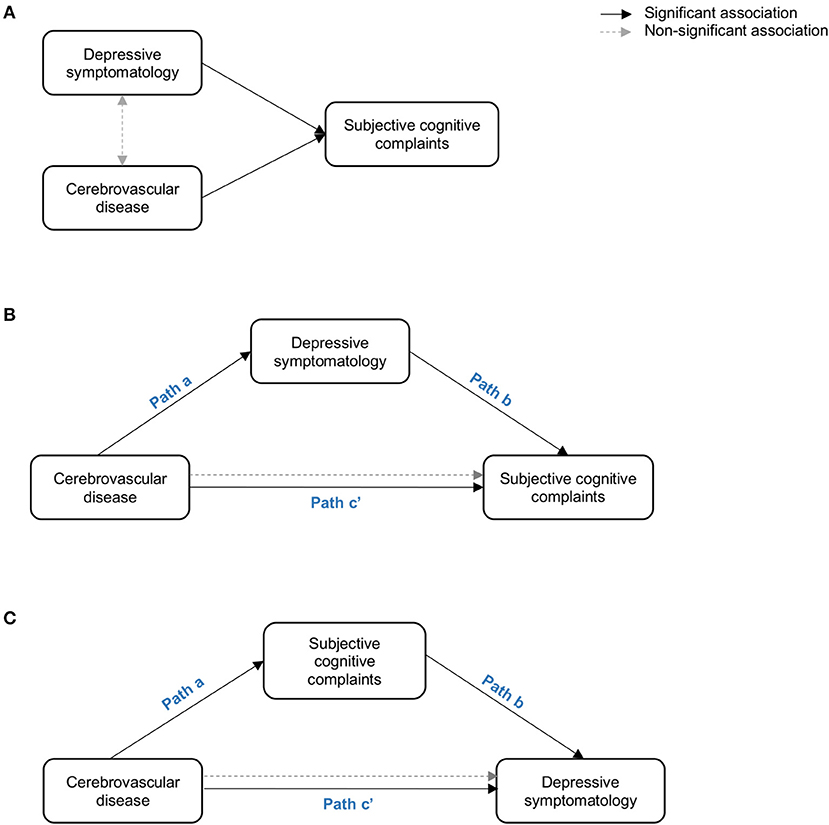
Figure 1. Representation of the three hypothetical scenarios: (A) depressive symptomatology co-exist with SCD (i.e. depressive symptomatology and cerebrovascular disease are independently associated with SCD; (B) depressive symptomatology influeces SCD by mediating the association between cerebrovascular disease and SCD; (C) depressive symptomatology reflects SCD (i.e. SCD mediates the association between cerebrovascular disease and depressive symptomatology. Paths in the figure refer to the associations betwen the independent variable, the mediator, and the dependent variable as described in Baron and Kenny' mediation framework (Baron and Kenny, 1986).
Methods
Participants
A total of 225 cognitively unimpaired individuals from the GENIC-database (Machado et al., 2018) were included in the current study. The GENIC is a prospective community-based study from the Canary Islands (Spain). Recruitment was performed through primary care health centers, advertisements in local schools, and relatives, as well as acquaintances of the research staff. A more detailed description of the cohort is provided in previous publications (Ferreira et al., 2015; Machado et al., 2018; Gonzalez-Burgos et al., 2019).
All the individuals who received an MRI scan including both T1 and DTI sequences (see further down) were candidate cases for the current study. Inclusion criteria were in concordance with the SCD initiative (SCD-I) working group (Jessen et al., 2014): (1) Normal cognition, which was established in a two-step diagnostic procedure: Firstly, in a screening phase dementia was excluded based on altered activities of daily living and global cognition operationalized as a Blessed Rating Dementia Scale (BRDS) score >4 (Blessed et al., 1968), a Functional Activity Questionnaire (FAQ) score >5 (Pfeffer et al., 1982), and a Mini-Mental State Examination (MMSE) score <24 (Folstein et al., 1975); Secondly, mild cognitive impairment was excluded based on comprehensive neuropsychological assessment and age-, sex-, and education-adjusted normative data following current clinical criteria (Winblad et al., 2004). The neuropsychological protocol is summarized in the Supplementary Table 1. Briefly, the neuropsychological protocol was applied in two sessions and MRI scanning was conducted in a third session. In all participants, the time between neuropsychological assessment (first session) and MRI scanning was within 6 months (mean = 1.5 months, SD = 2.5); (2) No abnormal findings such as stroke, tumors, hippocampal sclerosis, etc., in MRI according to an experienced neuroradiologist; (3) no medical history of neurological and psychiatric disorders (including a diagnosis of major depression and/or individuals under treatment for depression), systemic diseases or head trauma; and (4) no history of substance abuse.
This study was approved by the ethics committee from the University of La Laguna (Spain). Participation was completely voluntarily, and all the individuals gave their written informed consent.
Subjective Cognitive Decline
SCD was assessed with a questionnaire that covers subjective cognitive complaints (SCC) in different cognitive domains, including memory, orientation, executive functions, face recognition, language production, language comprehension, word-finding, reading and writing (Cedres et al., 2019). Participants answered nine yes/no questions referred to cognitive changes occurring during approximately the last 6 months. Answers were codified as 0 (absence of complaints) or 1 (presence of complaints). Answers were summed up and the total of complaints was obtained ranging from 0 to 9. In the current study, we use SCD when we refer to the clinical entity or concept of SCD, and we use SCC when we refer to the variable we used in our statistical analyses. The continuous variable of SCC was preferred to the dichotomous variable of SCD due to the nature of our statistical models and to avoid arbitrary clinical thresholds.
Depressive Symptomatology
Depressive symptomatology was assessed with two validated scales. The Beck Depression Inventory (BDI, 21-items version) (Beck et al., 1961) was used for participants younger than 63 years of age, and the Geriatric Depression Scale (GDS, 15-items version) (Yesavage et al., 1982) was used for participants 63 years old or older. Following previous publications, BDI and GDS scores were transformed into z-scores and combined into one single variable for statistical analysis (BDI-GDS composite) (Ferreira et al., 2017; Cedres et al., 2019).
MRI Data Acquisition and Image Processing
Participants were scanned using a 3.0T GE imaging system (General Electric, Milwaukee, WI, USA), located at the Hospital Universitario de Canarias in Tenerife, Spain. A three-dimensional T1-weighted Fast Spoiled Gradient Echo (FSPGR) sequence was acquired in sagittal plane: repetition time/echo time/inversion time = 8.73/1.74/650 ms., field of view = 250 × 250 mm, matrix = 250 × 250 mm, flip angle = 12°, slice thickness = 1 mm, voxel resolution = 1 × 1 × 1 mm. Also, a DTI sequence was acquired in axial plane: repetition time/echo time = 15.000/≈72 ms., field of view = 256 × 256 mm, matrix: 128 × 128 mm, directions = 31, B-value = 1,000, flip angle = 90°, slice thickness = 2.4 mm, voxel resolution = 2 × 2 × 2.4 mm. Full brain and skull coverage was required for the MRI datasets and detailed quality control was carried out on all MR images according to previously published criteria (Simmons et al., 2011).
T1-weighted images were processed and analyzed with the FreeSurfer 6.0.0 image analysis suite (http://surfer.nmr.mgh.harvard.edu/). The FreeSurfer measure of white matter hypointensities was used as a surrogate marker of cerebrovascular disease, and referred to as WMSA in the current study. Briefly, FreeSurfer uses a probabilistic procedure to detect hypointensities in the white matter and labels them as WMSA (Fischl et al., 2002). This procedure has previously demonstrated sensitivity in measuring white matter damage both in healthy individuals and in patients with Alzheimer's disease (Salat et al., 2010; Leritz et al., 2014). These T1-weighted WMSA correlate with hyperintensity volumes measured on T2/FLAIR, as well as with microstructural white matter changes as measured on diffusion tensor imaging data (Leritz et al., 2014; Riphagen et al., 2018; Cedres et al., 2020b; Nemy et al., 2020). The estimated total intracranial volume (TIV) was also obtained from FreeSurfer in order to adjust the WMSA volume by the TIV. This adjustment was performed by dividing the WMSA volume by the TIV of each individual (Voevodskaya, 2014). The TIV-adjusted WMSA measure was used for statistical analyses.
The DTI images were pre-processed and analyzed with the FSL software (http://www.fmrib.ox.ac.uk/fsl/index.html), using the FDT and tract-based spatial statistics (TBSS) tools. The mean diffusivity (MD) index was selected as our measure of interest in this study because MD has shown to be an earlier indicator of neurodegeneration compared to other diffusivity measures (Liu et al., 2013; Li et al., 2015).
All the data were processed through theHiveDB system (Muehlboeck et al., 2014). Careful visual quality control was performed on all output data obtained from both FreeSurfer and FSL, and manual edits were applied when needed.
Statistical Analysis
The DTI data was analyzed through a voxel-based approach on the white matter skeleton, using the FSL software (Smith et al., 2006). Separate general linear models were fitted at the voxel level with MD as the dependent variable and SCC, depressive symptomatology, WMSA, or age as independent variables. Permutation-based non-parametric testing with 5,000 iterations was used followed by threshold-free cluster enhancement (TFCE) and the family-wise error (FWE) correction for multiple testing (p ≤ 0.01, two-sided). Average MD values of significant clusters in individual's native space were extracted as new measures for further analysis (e.g., SCC-related MD and WMSA-related MD, please see the results section below). In addition, the global MD was extracted as a measure of mean MD values in the whole white matter skeleton.
We designed an approach based on correlation, multiple regression, and mediation analyses to address our first aim: to investigate de role of the depressive symptomatology in SCD (Figure 1). Firstly, bivariate Pearson correlations were used to study relationships between SCC and depressive symptomatology, WMSA, and MD measures. Secondly, multiple linear regression models were used to further investigate partial associations of depressive symptomatology, WMSA, and MD measures (predictors) with SCC (outcome variable). Thirdly, these analyses were complemented with mediation models when the three basic conditions of mediation analysis were satisfied (Baron and Kenny, 1986): (1) there is a significant association between the mediator and the independent variable; (2) there is a significant association between the independent variable and the dependent variable; and (3) there is a significant association between the mediator and the dependent variable when the independent variable is also included in the model. For an illustration of the mediation models please see Figure 1.
Mediation analysis was also used to investigate our second aim: to investigate whether WMSA mediates the association between MD and SCC. Mediation was based on the average direct effect (ADE), the average causal mediation effect (ACME), and the total effect. Briefly, the ADE represents the direct effect of the independent variable on the dependent variable, while the ACME represents the indirect effect of the independent variable on the dependent variable, through the mediator variable. The total effect represents the sum of the ACME and the ADE. When the ACME is statistically significant (in conjunction with a significant total effect) there is a mediation effect that can be of two types: full mediation, when the ACME is significant but the ADE is non-significant; and partial mediation, when both the ACME and the ADE are significant (Tingley et al., 2014). The ACME and the ADE were calculated by using confidence intervals based on non-parametric bootstrap sampling (1,s000 simulations).
To address our third aim—to investigate the effect of aging in our analyses—we repeated the above-mentioned regression models including age as a covariate, and we tested for bivariate Pearson's correlations for age with SCC, depressive symptomatology, WMSA, and MD.
Statistical analyses were conducted using the R statistical software (http://www.r-project.org). A p < 0.05 (two-tailed) was deemed significant in all these analyses.
Results
The demographic and clinical characteristics of the cohort are described in Table 1. A total of 123 (55%) participants endorsed one or more SCC, while 102 (45%) participants reported zero SCC (number of complaints: mean = 0.92; SD = 1.1, range = 0–6). There were significantly more women in the subgroup of individuals with one or more SCC compared with those individuals with zero SCC (Table 1). Individuals with one or more SCC also showed significantly lower scores in the WAIS-III Information subtest after correcting for sex. Individuals with one or more SCC also had significantly lower scores in the MMSE; higher scores in the BDRS; and more depressive symptoms. These differences remained significant after controlling for the effect of sex and WAIS-III Information subtest. The proportion of individuals with high cholesterol and blood pressure was higher in the subgroup with one or more SCC than the group with zero SCC. Individuals with one or more SCC had a higher WMSA burden and worse white matter integrity (i.e., higher MD values) than individuals with zero SCC. Regarding depressive symptomatology irrespective of SCC, participants younger than 63 years scored between 0 and 23 in the BDI (mean = 5.6; SD = 4.6), and participants 63 years old or older scored between 0 and 9 in the GDS (mean = 2.3; SD = 2.1). The distributions of BDI, GDS, and the BDI-GDS composite variable are shown in Figure 2.
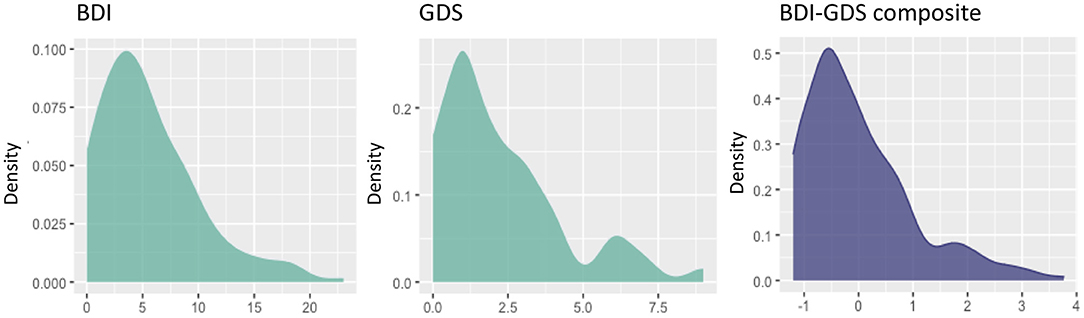
Figure 2. Distribution of the variables of depressive symptomatology. Scores on the x-axis (original scores from the BDI and the GDS, or z-scores from the BDI-GDS composite measure), and densities on the y-axis. BDI, Beck Depression Inventory; GDS, Geriatric Depression Scale.
First Aim: The Role of Depressive Symptomatology
The first aim of this study was to investigate the role of depressive symptomatology in SCD, under the hypotheses that depressive symptomatology would (A) co-exist with SCD, (B) influence SCD, or (C) reflect SCD (Figure 1). Correlation analyses showed that higher scores in depressive symptomatology were associated with a higher number of SCC (r = 0.340, p < 0.001). In contrast, depressive symptomatology did not correlate with the global MD (r = 0.076, p = 0.321) or WMSA (r = 0.003, p = 0.961). Depressive symptomatology did not correlate with MD values at the voxel level either (Figure 3A). Based on these results, we could not satisfy some of the three basic conditions for mediation analysis proposed by Baron and Kenny's (1986) (i.e., the association between depressive symptomatology and MD or WMSA). Hence, we could not test for mediation models including depressive symptomatology together with WMSA or global MD (Figures 1B,C). We next conducted a multiple linear regression model to investigate the partial association of depressive symptomatology, WMSA, and MD with SCC. We included SCC as the criterion variable, and WMSA, global MD, and depressive symptomatology as the predictors (Table 2; model 1). This model was significant [F(3, 221) = 15.655, p < 0.001, R2 adj. = 0.16], indicating that depressive symptomatology and WMSA were independently associated with SCC. In contrast, global MD was not significant (Table 2). However, the lack of a significant effect for global MD may due to the fact that the measure of global MD may include areas that are not involved in SCC. Hence, in a second model, we restricted MD values to those voxels that were significantly related with SCC (“average SCC-related MD,” see below). We observed that depressive symptomatology continued to be a significant predictor of SCC, but WMSA was no longer a significant predictor when the average SCC-related MD was also in the model [Table 2; model 2, full model: F(3, 221) = 20.987, p < 0.001, R2 adj. = 0.211]. In correlation analysis, depressive symptomatology was not correlated with the average SCC-related MD either (r = 0.117, p = 0.079).
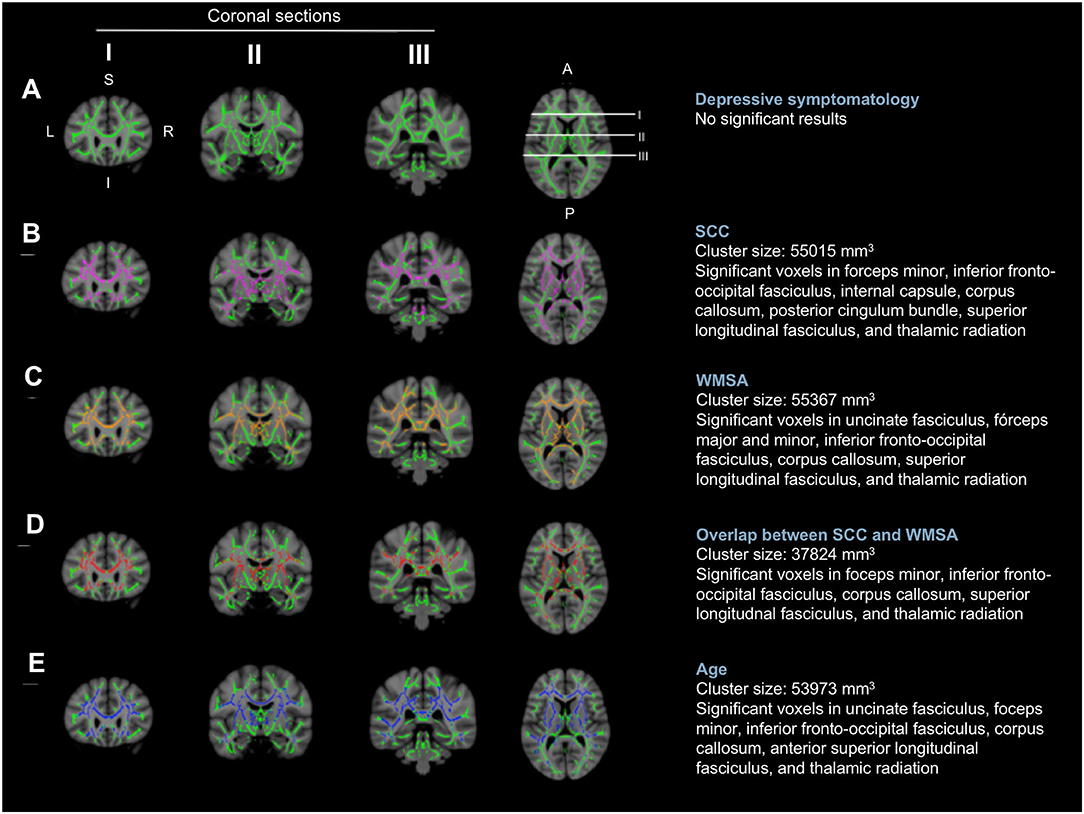
Figure 3. Voxel-wise correlations of MD values with depressive symptomatology, SCC, WMSA, and age. The white matter skeleton is depicted in green. Significant voxels are colored in pink [(B) the association between MD values and SCC], orange [(C) the association between MD values and WMSA], red [(D) overlap of the association between MD values and SCC, and MD values and WMSA], and blue [(E) the association between MD values and age]. No significant voxels were obtained for the association between MD values and depressive symptomatology (A). L, left; R, right; S, superior; I, inferior; A, anterior; P, posterior; MD, mean diffusivity; SCC, subjective cognitive complaints; WMSA, white matter signal abnormalities; mm, millimeters.
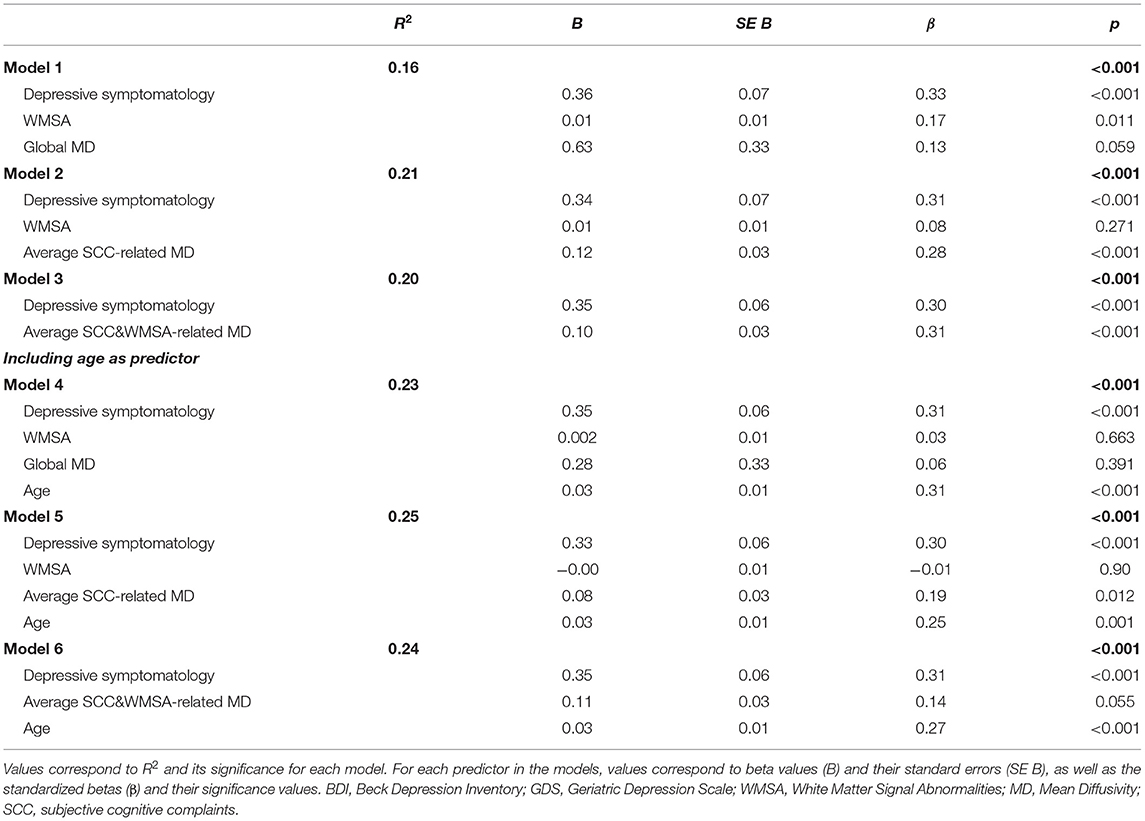
Table 2. Partial association of depressive symptomatology, WMSA, MD, and age with SCC (multiple regression models).
These results suggest that depressive symptomatology may co-exist with SCC (Figure 1A). To fully prove that hypothesis we had to demonstrate that cerebrovascular disease is also associated with SCC. Hence, we conducted complementary analyses to further characterize the association of WMSA and MD with SCC. A higher burden of WMSA and a higher global MD correlated with a higher number of SCC (r = 0.216, p = 0.001, and r = 0.210, p = 0.002, respectively). The voxel-based analysis showed that the association between higher MD values and a higher number of SCC involved most of the white matter skeleton, with a tendency to spare the occipital white matter and the anterior part of the cingulum bundle (Figure 3B). The average MD value of these SCC related areas was extracted in a new variable (“average SCC-related MD”) for further analysis.
Second Aim: The Contribution of WMSA to Variability in MD
The second aim of this study was to test the hypothesis that variability in MD, an unspecific DTI biomarker of neurodegeneration, would mediate cerebrovascular disease as measured by WMSA. Correlation analyses showed that a higher global MD correlated with a higher burden of WMSA (r = 0.370, p < 0.001), and a higher MD in areas specifically associated with SCC (“average SCC-related MD”) showed an even stronger correlation with a higher burden of WMSA (r = 0.492, p < 0.001). At the voxel level, the association between higher MD levels and higher WMSA burden involved most of the white matter skeleton, with a tendency to spare the internal capsule, the occipital white matter, and the cingulum bundle (Figure 3C). The average MD value of WMSA-related areas was extracted in a new variable (“average WMSA-related MD”) for further analysis. Results showed that the correlation coefficient of the association between SCC and the average WMSA-related MD (r = 0.267) was larger than the correlation coefficient of the association between SCC and global MD (r = 0.210). We also assessed the conjunction between the association of MD with SCC and WMSA. When we overlapped these two maps, MD values in forceps minor, corpus callosum, superior longitudinal fasciculus, inferior fronto-occipital fasciculus, and thalamic radiation were associated with both SCC and WMSA burden (Figure 3D). The measure “average SCC&WMSA-related MD” was calculated as the conjunction between these two maps. A new multiple regression model was conducted to investigate the partial association of depressive symptomatology and the MD voxels that were associated with both SCC and WMSA burden (“average SCC&WMSA-related MD”) with SCC. The model included depressive symptomatology and “average SCC&WMSA-related MD” as predictors, and SCC as the criterion (Table 2; model 3). This model was significant [F(2, 222) = 28.534, p < 0.001, R2 adj. = 0.197], indicating that both the average SCC&WMSA-related MD (β = 0.300, p < 0.001) and depressive symptomatology (β = 0.309 p < 0.001) were independently associated with SCC.
Finally, we used mediation analysis to investigate whether WMSA mediates the association between global MD and SCC. We found that WMSA significantly mediated the association between global MD and SCC (ACME = 2960.825; p = 0.026). This mediation effect was partial because the direct effect of global MD on SCC was also significant (ADE = 7590.678, p = 0.032).
Third Aim: The Effect of Aging
The third aim of this study was to investigate the effect of aging in our data. Correlation analyses showed that an older age correlated with a higher volume of WMSA (r = 0.521, p < 0.001), a higher global MD (r = 0.387, p < 0.001), a higher number of SCC (r = 0.373, p < 0.001), and higher scores in depressive symptomatology (r = 0.069, p = 0.030). The voxel-based analysis showed that the association between an older age and higher MD values involved most of the white matter skeleton, with a tendency to spare the occipital and parietal white matter and tracts going through the internal capsule and the cingulum bundle (Figure 3E). Next, we added age as an extra predictor to the multiple regression models reported for the first and second aims. The model for global MD (model 4 in Table 2) was significant [F(4, 222) = 17.581, p < 0.001, R2 adj. = 0.228], showing that age was the main predictor of SCC, followed by depressive symptomatology. In contrast, WMSA and global MD were not significant as predictors. The model specific for MD areas involved in SCC (“average SCC-related MD,” model 5 in Table 2) was significant [F(4, 220) = 19.466, p < 0.001, R2 adj. = 0.248], showing that age, depressive symptomatology, and the average SCC-related MD were significant predictors of SCC, while WMSA was not significant (p = 0.900). Finally, the model for the average SCC&WMSA-related MD (model 6 in Table 2) was significant [F(3, 221) = 24.676, p < 0.001, R2 adj. = 0.241], showing that both depressive symptomatology and age (β = 0.268, p < 0.001) were independently associated with SCC, with a trend to significance for the average SCC&WMSA-related MD to predict SCC (β = 0.140, p = 0.055).
Discussion
In this study, we tested the role of depressive symptomatology in the context of SCD and cerebrovascular disease using cross-sectional data from a community-based cohort. We also investigated whether DTI abnormalities (increased MD values) in SCD are associated with increased WMSA burden and older age. We operationalized SCD following the diagnostic criteria of the international working group on SCD (Jessen et al., 2014), and used the number of SCC (i.e., subjective cognitive complaints) in our statistical analyses. While our results showed that an increased depressive symptomatology is significantly associated with more SCC, we could not find a significant association of depressive symptomatology with WMSA or MD measures. We thus accepted the hypothesis of depressive symptomatology co-existing with SCC, independently of any MRI marker of cerebrovascular disease. In addition, we demonstrated that WMSA mediated the association between MD and SCC, and age had an important contribution to our findings.
The role of depressive symptomatology in SCD is controversial. While major depression is an exclusion criterion in current diagnostic criteria of SCD (Jessen et al., 2014), individual symptoms of depression that do not reach the threshold of a disorder are not considered a criterion for exclusion (Jessen et al., 2014). However, it is not clear what should be the exact threshold to exclude depression and how this type of symptomatology should be assessed in SCD (e.g., concurrent, past, subclinical, etc.). Currently, the most urgent need is to elucidate the role of subthreshold depressive symptomatology in SCD (Molinuevo et al., 2017; Jessen et al., 2020). In our relatively large community-based cohort, we approached this question by assessing subthreshold variability in depressive symptomatology in SCD individuals who did not have a diagnosis of major depression nor were under treatment for depression. Our analyses confirmed the well-known association between depressive symptomatology and SCD (Donovan et al., 2014, 2015; Buckley et al., 2016; Burmester et al., 2016; Lebedeva et al., 2018; Cedres et al., 2019), showing that a higher number of SCC was associated with increased depressive symptomatology in our cohort. In contrast, we could not demonstrate that an increased depressive symptomatology in our cohort is related with an older age, which would reflect the concept of late life depression (Diniz et al., 2013). In addition, we did not find an association of WMSA or MD measures with depressive symptomatology, as it would be predicted by the vascular depression hypothesis (Alexopoulos et al., 2013; Taylor et al., 2013). Altogether, our results suggest that the variability in depressive symptomatology in our cohort may be related to emotional factors rather than cerebrovascular disease or age-related factors. Moreover, despite its strong association with SCC, depressive symptomatology seems to just co-exist with SCD in our cohort, without influencing associations of SCC with markers of cerebrovascular disease or, as demonstrated in previous studies using the same cohort, with markers of gray matter degeneration (Cedres et al., 2019, 2020a) or clinical-cognitive status (Diaz-Galvan et al., 2021). Hence, SCD in our cohort does not seem to merely reflect emotional factors, as traditionally postulated (Apolinario et al., 2013; Yates et al., 2015; Burmester et al., 2016), but may rather reflect neurodegeneration and subclinical cognitive decline.
We observed a strong association of SCC with both WMSA and MD. Since WMSA correlated with MD, and WMSA mediated the association between MD and SCC, we suggest that variability in our MD measure may be influenced by cerebrovascular disease. In other words, despite being an unspecific marker, our MD measure may be reflecting cerebrovascular disease in our study. Other studies also highlighted the contribution of non-AD pathologies such as cerebrovascular disease to SCD in community-based cohorts (Diniz et al., 2013). The novelty of our study is the use of DTI to investigate white matter neurodegeneration associated with cerebrovascular disease, and the analysis of its topographical distribution. The utility of DTI measures as markers of cerebrovascular disease has previously been noted (Zhou et al., 2008; Black et al., 2009; Fu et al., 2012; Salat et al., 2012). Interestingly, in our study, the association between WMSA and MD in areas related with SCC (“average SCC-related MD”) was stronger than the association between WMSA and MD in the whole white matter skeleton (“global MD”). This suggests that the brain areas in which integrity of the white matter is associated with SCC seems to be more vulnerable to the effect of cerebrovascular disease than other white matter areas. Hence, cerebrovascular disease may be a contributor to SCC in our community-based cohort. This interpretation was further supported by our result showing that the association between SCC and MD in areas related with WMSA (“average WMSA-related MD”) was stronger than the association between SCC and MD in the whole white matter skeleton (“global MD”). Another important observation is that our associations between SCC and markers of cerebrovascular disease were related with or accounted by the age, as discussed further down.
We demonstrated the strong association between an older age and increased SCC, a finding that is well-established in the SCD literature (Derouesné et al., 1993; Wang et al., 2004; Jessen et al., 2010; van Harten et al., 2018; Cedres et al., 2019). We also demonstrated the strong association between an older age and increased cerebrovascular disease, in line with previous reports (Raz et al., 2012; Habes et al., 2016; Nemy et al., 2020). Whether this cerebrovascular disease in cognitively unimpaired older individuals indicates preclinical stages of vascular cognitive impairment or is rather a feature of normal aging when not reaching the clinical threshold is currently not known. The hypothesis of a preclinical stage is attractive in the context of our study, highlighting the capacity of SCD to reflect underlying neurodegenerative processes of presumably vascular origin. In a recent study using the same cohort we demonstrated that the effect of WMSA on cholinergic white matter pathways goes beyond the effect of age (Nemy et al., 2020). In our current study, including the age in our models removed the predictive partial effect of global MD, but the effect of MD in white matter areas associated with SCC remained significant. This suggests that while the integrity of the white matter overall seems to be primarily driven by increasing age, variability in the integrity of areas specific to SCC goes beyond the effect of aging (and depressive symptomatology). This finding was attenuated when investigating the integrity of areas specific to both SCC and WMSA (p = 0.055), suggesting that the cerebrovascular component that is related to SCC in our cohort may primarily be explained by increasing age, and not to stroke or other major vascular disease. This interpretation is congruent with the current definition of SCD, where neurological diseases other than AD are a criterion for exclusion (Jessen et al., 2014).
This study has some limitations. Although we did not find a significant association between depressive symptomatology and MRI markers of cerebrovascular disease, we cannot exclude that depressive symptomatology in our cohort could be an early symptom of other brain pathologies previously reported in SCD, such as amyloid-beta or tau pathologies (Amariglio et al., 2012; Perrotin et al., 2012; Buckley et al., 2017). The lack of biomarkers for amyloid-beta and tau pathologies is thus a limitation of our study. Nonetheless, vascular risk factors are highly prevalent in community-based cohorts like the one used in our study (Buckley et al., 2017), while AD pathologies are more prevalent in clinical cohorts (Kern et al., 2018). In the same vein, variability in MD was strongly associated with WMSA, but other pathologies such as amyloid-beta and tau could also be contributing to MD. This interpretation is supported by our finding of SCC-related MD remaining in our model, while WMSA was automatically removed when including the age in the same model. We believe that SCC-related MD reflects neurodegeneration beyond that related with an older age or a higher WMSA burden. However, our measure of WMSA is global, and it would be interesting to investigate WMSA intersecting SCC-related white matter tracts in future studies. We partially circumvented this by investigating the conjunction between WMSA-related MD and SCC-related MD. We reported the frequency of some vascular risk factors but a more complete characterization of vascular risk factors, as well as their contribution to our current findings is warranted in the future. In any case, these limitations do not compromise our main interpretation of depressive symptomatology as an emotional factor co-existing but not related with neurodegenerative factors that underlie SCC, since MD and WMSA correlated with SCC but not with depressive symptomatology. We used correlation, regression, and mediation analyses to investigate associations on cross-sectional data. Longitudinal designs or clinical trials to demonstrate that treating emotional or vascular risk factors reduces SCD would help to support potential causality in our current associations. Finally, previous studies showed that individuals with SCD differed in the frequency of affective symptoms and underlying neurodegeneration depending on whether they are recruited in the community or in clinical settings (Perrotin et al., 2017; Slot et al., 2018). Therefore, our current findings could also be tested in clinical-based samples, including SCD individuals who seek medical help.
In conclusion, depressive symptomatology co-exists with SCD and reflects emotional factors but not cerebrovascular disease, in our community-based cohort. In addition, we did not find any evidence for depressive symptomatology to influence the association between cerebrovascular disease and SCD. In our cohort, SCD reflected white matter neurodegeneration in spite of its association with depressive symptomatology. This highlights the clinical usefulness of SCD, especially in older individuals who often show subjective complaints, depressive symptomatology, and positive cerebrovascular disease biomarkers. A remark is that although SCD increased with age in our cohort, the association between white matter abnormalities and SCD was beyond the effect of aging. Therapeutic interventions for depressive symptomatology could alleviate the psychological burden of negative emotions in people SCD, and intervening on vascular risk factors to reduce cerebrovascular disease should be tested as an opportunity to minimize neurodegeneration in SCD individuals from the community. Another important contribution of the current study is the data reported to help understanding the association between cerebrovascular disease, depressive symptomatology, and SCD.
Data Availability Statement
The raw data supporting the conclusions of this article will be available upon reasonable request from qualified researchers.
Ethics Statement
The studies involving human participants were reviewed and approved by the ethics committee from the University of La Laguna (Spain). The patients/participants provided their written informed consent to participate in this study.
Author Contributions
PD-G: data acquisitions, interpretation of results, writing of portions of the manuscript, and preparing figures. NC: data acquisitions, analysis and interpretation of results, writing of portions of the manuscript, and preparing figures. NF: study concept and design, data acquisitions, analysis and interpretation of results, and writing of portions of the manuscript. JB: supervision of the project, revision of manuscript, and funding. EW: revision of manuscript and funding. DF: study concept and design, data acquisition, interpretation of results, writing of portions of the manuscript, supervision of the study, and funding. All authors contributed to the article and approved the submitted version.
Funding
This research was funded by the Estrategia de Especialización Inteligente de Canarias RIS3 de la Consejería de Economía, Industria, Comercio y Conocimiento del Gobierno de Canarias, co-funded by the Programa Operativo FEDER Canarias 2014–2020 (ProID2020010063); the Fundación Canaria Dr. Manuel Morales (calls in 2012, 2014, and 2017); Fundación Cajacanarias; Center for Innovative Medicine (CIMED), the Swedish Foundation for Strategic Research (SSF), the Strategic Research Programme in Neuroscience at Karolinska Institutet (StratNeuro), the Swedish Research Council (VR), the Åke Wiberg foundation, Hjärnfonden, Alzheimerfonden, Demensfonden Stiftelsen, Olle Engkvist Byggmästare, Birgitta och Sten Westerberg, Demensförbundet, Loo och Hans Ostermans Foundation, Gun och Bertil Stohnes Stiftelse, Foundation for Geriatric Diseases at Karolinska Institutet, Research Funding from Karolinska Institutet, and Stiftelsen För Gamla Tjänarinnor. The funders of the study had no role in the study design nor the collection, analysis, and interpretation of data, writing of the report, or decision to submit the manuscript for publication.
Conflict of Interest
The authors declare that the research was conducted in the absence of any commercial or financial relationships that could be construed as a potential conflict of interest.
Publisher's Note
All claims expressed in this article are solely those of the authors and do not necessarily represent those of their affiliated organizations, or those of the publisher, the editors and the reviewers. Any product that may be evaluated in this article, or claim that may be made by its manufacturer, is not guaranteed or endorsed by the publisher.
Acknowledgments
The authors would like to thank Dr. Antonio Rodríguez for providing access to participants and helpful assistance; and the Servicio de Resonancia Magnética para Investigaciones Biomédicas del SEGAI (University of La Laguna, Spain). Data used in preparation of this article is part of the GENIC-database (Group of Neuropsychological Studies of the Canary Islands, University of La Laguna, Spain. Principal investigator: JB. Contact: DF, ZGFuaWVsLmZlcnJlaXJhLnBhZGlsbGFAa2kuc2U=). The following collaborators contributed to the GENIC-database but did not participate in analysis or writing of this report (in alphabetic order by family name): Rut Correia, Aida Figueroa, Eloy García, Lissett González, Teodoro González, Zaira González, Cathaysa Hernández, Edith Hernández, Nira Jiménez, Judith López, Cándida Lozano, Alejandra Machado, María Antonieta Nieto, María Sabucedo, Elena Sirumal, Marta Suárez, Manuel Urbano, and Pedro Velasco.
Supplementary Material
The Supplementary Material for this article can be found online at: https://www.frontiersin.org/articles/10.3389/fnagi.2021.656990/full#supplementary-material
Abbreviations
ACME, average causal mediation effect; AD, Alzheimer's disease; ADE, average direct effect; BDI, Beck's Depression Inventory; BDRS, Blessed Dementia Rating Scale; CVD, cerebrovascular disease; DTI, diffusion tensor imaging; FAQ, Functional Activity Questionnaire; FSPGR, Fast Spoiled Gradient Echo; GDS, Geriatric Depression Scale; GENIC, Grupo de Estudios Neuropsicológicos de las Islas Canarias; ICV, Intracranial volume; MD, Mean diffusivity; MMSE, Mini Mental State Examination; MRI, Structural magnetic resonance imaging; SCC, Subjective Cognitive Complaints; SCD, Subjective Cognitive Decline; SCD-I, Subjective Cognitive Decline initiative; TBSS, tract-based spatial statistics; WMSA, White matter signal abnormalities.
References
Alexopoulos, G. S., Young, R. C., Campbell, S., Silbersweig, D., and Charlson, M. (2013). Vascular depression' hypothesis. J. Chem. Inf. Model. 53, 1689–1699. doi: 10.1021/ci400128m
Allan, C. L., Sexton, C. E., Filippini, N., Topiwala, A., Mahmood, A., Zsoldos, E., et al. (2016). Sub-threshold depressive symptoms and brain structure: A magnetic resonance imaging study within the Whitehall II cohort. J. Affect. Disord. 204, 219–225. doi: 10.1016/j.jad.2016.06.049
Amariglio, R. E., Becker, J. A., Carmasin, J., Wadsworth, L. P., Lorius, N., Sullivan, C., et al. (2012). Subjective cognitive complaints and amyloid burden in cognitively normal older individuals. Neuropsychologia 50, 2880–2886. doi: 10.1016/j.neuropsychologia.2012.08.011
Apolinario, D., Miranda, R. B., Suemoto, C. K., Magaldi, R. M., Busse, A. L., Soares, A. T., et al. (2013). Characterizing spontaneously reported cognitive complaints: The development and reliability of a classification instrument. Int. Psychogeriatr. 25, 157–166. doi: 10.1017/S1041610212001494
Baron, R. M., and Kenny, D. A. (1986). The moderator-mediator variable distinction in social psychological research: Conceptual, strategic, and statistical considerations. J. Pers. Soc. Psychol. 51, 1173–1182. doi: 10.1037/0022-3514.51.6.1173
Beck, A. A. T., Ward, C. H. H., Mendelson, M., Mock, J., and Erbaugh, J. (1961). An inventory for measuring depression. Arch. Gen. Psychiatry 4, 561–571. doi: 10.1001/archpsyc.1961.01710120031004
Black, S., Gao, F., and Bilbao, J. (2009). Understanding white matter disease: Imaging-pathological correlations in vascular cognitive impairment. Stroke 40(Suppl. 3), S48–52. doi: 10.1161/STROKEAHA.108.537704
Blessed, G., Tomlinson, B. E., and Roth, M. (1968). The association between quantitative measures of dementia and of senile change in the cerebral grey matter of elderly subjects. Br. J. Psychiatry 114, 797–811. doi: 10.1192/bjp.114.512.797
Buckley, R. F., Hanseeuw, B., Schultz, A. P., Vannini, P., Aghjayan, S. L., Properzi, M. J., et al. (2017). Region-specific association of subjective cognitive decline with tauopathy independent of global β-amyloid burden. JAMA Neurol. 74, 1455–1463. doi: 10.1001/jamaneurol.2017.2216
Buckley, R. F., Maruff, P., Ames, D., Bourgeat, P., Martins, R. N., Masters, C. L., et al. (2016). Subjective memory decline predicts greater rates of clinical progression in preclinical Alzheimer's disease. Alzheimer's Dement. 12, 796–804. doi: 10.1016/j.jalz.2015.12.013
Burmester, B., Leathem, J., and Merrick, P. (2016). Subjective cognitive complaints and objective cognitive function in aging: a systematic review and meta-analysis of recent cross-sectional findings. Neuropsychol. Rev. 26, 376–393. doi: 10.1007/s11065-016-9332-2
Butters, M. A., Young, J. B., Lopez, O., Aizenstein, H. J., Mulsant, B. H., Reynolds, C. F., et al. (2008). Pathways linking late-life depression to persistent cognitive impairment and dementia. Dialog. Clin. Neurosci. 10, 345–357. doi: 10.31887/DCNS.2008.10.3/mabutters
Cedres, N., Ferreira, D., Machado, A., Shams, S., Sacuiu, S., Waern, M., et al. (2020b). Predicting Fazekas scores from automatic segmentations of white matter signal abnormalities. Aging. 12, 894–901. doi: 10.18632/aging.102662
Cedres, N., Institutet, K., Diaz-galvan, P., Institutet, K., Ferreira, D., and Institutet, K. (2020a). The interplay between gray matter and white matter neurodegeneration in subjective cognitive decline. ResearchSquare. doi: 10.21203/rs.3.rs-91497/v1
Cedres, N., Machado, A., Molina, Y., Diaz-Galvan, P., Hernández-Cabrera, J. A., Barroso, J., et al. (2019). Subjective cognitive decline below and above the age of 60: a multivariate study on neuroimaging, cognitive, clinical, and demographic measures. J. Alzheimer's Dis. 68, 295–309. doi: 10.3233/JAD-180720
Clarnette, R. M., Almeida, O. P., Forstl, H., Paton, A., and Martins, R. N. (2001). Clinical characteristics of individuals with subjective memory loss in Western Australia: results from a cross-sectional survey. Int. J. Geriatr. Psychiatry 16, 168–174. doi: 10.1002/1099-1166(200102)16:2<168::AID-GPS291>3.0.CO;2-D
Da Silva, J., Gonçalves-Pereira, M., Xavier, M., and Mukaetova-Ladinska, E. B. (2013). Affective disorders and risk of developing dementia: Systematic review. Br. J. Psychiatry 202, 177–186. doi: 10.1192/bjp.bp.111.101931
Derouesné, C., Dealberto, M., Boyer, P., Lubin, S., Sauron, B., Piette, F., et al. (1993). Empirical evaluation of the ‘Cognitive Difficulties Scale’ for assessment of memory complaints in general practice: a study of 1628 cognitively normal subjects aged 45–75 years. Int. J. Geriatr. Psychiatry 8, 599–607. doi: 10.1002/gps.930080712
Diaz-Galvan, P., Ferreira, D., Cedres, N., Falahati, F., Hernández-Cabrera, J. A., Ames, D., et al. (2021). Comparing different approaches for operationalizing subjective cognitive decline: impact on syndromic and biomarker profiles. Sci. Rep. 11, 1–15. doi: 10.1038/s41598-021-83428-1
Diniz, B. S., Butters, M. A., Albert, S. M., Dew, M. A., and Reynolds, C. F. (2013). Late-life depression and risk of vascular dementia and Alzheimer's disease: Systematic review and meta-analysis of community-based cohort studies. Br. J. Psychiatry 202, 329–335. doi: 10.1192/bjp.bp.112.118307
Donovan, N. J., Amariglio, R. E., Zoller, A. S., Rudel, R. K., Gomez-Isla, T., Blacker, D., et al. (2014). Subjective cognitive concerns and neuropsychiatric predictors of progression to the early clinical stages of Alzheimer's disease. Am. J. Geriatr. Psychiatry 22, 1642–1651. doi: 10.1016/j.jagp.2014.02.007
Donovan, N. J., Hsu, D. C., Dagley, A. S., Schultz, A. P., Amariglio, R. E., Mormino, E. C., et al. (2015). Depressive symptoms and biomarkers of Alzheimer's disease in cognitively normal older adults. J. Alzheimers Dis. 46, 63–73. doi: 10.3233/JAD-142940
Ferreira, D., Correia, R., Nieto, A., Machado, A., Molina, Y., and Barroso, J. (2015). Cognitive decline before the age of 50 can be detected with sensitive cognitive measures. Psicothema 27, 216–222. doi: 10.7334/psicothema2014.192
Ferreira, D., Machado, A., Molina, Y., Nieto, A., Correia, R., Westman, E., et al. (2017). Cognitive variability during middle-age: possible association with neurodegeneration and cognitive reserve. Front. Aging Neurosci. 9:188. doi: 10.3389/fnagi.2017.00188
Fischl, B., van Der Kouwe, A., Salat, D. H., Busa, E., Albert, M., Dieterich, M., et al. (2002). Whole brain segmentation: automated labeling of neuroanatomical structures in the human brain. Neuron 33, 341–355. doi: 10.1016/S0896-6273(02)00569-X
Folstein, M. F., Folstein, S. E., and McHugh, P. R. (1975). “Mini-mental state”: A practical method for grading the cognitive state of patients for the clinician. J. Psychiatr. Res. 12, 189–198. doi: 10.1016/0022-3956(75)90026-6
Fu, J. L., Zhang, T., Chang, C., Zhang, Y. Z., and Li, W. B. (2012). The value of diffusion tensor imaging in the differential diagnosis of subcortical ischemic vascular dementia and Alzheimer's disease in patients with only mild white matter alterations on T2-weighted images. Acta Radiol. 53, 312–317. doi: 10.1258/ar.2011.110272
Ginó, S., Mendes, T., Maroco, J., Ribeiro, F., Schmand, B. A., De Mendonça, A., et al. (2010). Memory complaints are frequent but qualitatively different in young and elderly healthy people. Gerontology 56, 272–277. doi: 10.1159/000240048
Gonzalez-Burgos, L., Hernández-Cabrera, J. A., Westman, E., Barroso, J., and Ferreira, D. (2019). Cognitive compensatory mechanisms in normal aging: a study on verbal fluency and the contribution of other cognitive functions. Aging 11, 4090–4106. doi: 10.18632/aging.102040
Habes, M., Erus, G., Toledo, J. B., Zhang, T., Bryan, N., Launer, L. J., et al. (2016). White matter hyperintensities and imaging patterns of brain ageing in the general population. Brain 139, 1164–1179. doi: 10.1093/brain/aww008
Jessen, F., Amariglio, R. E., Buckley, R. F., van der Flier, W. M., Han, Y., Molinuevo, J. L., et al. (2020). The characterisation of subjective cognitive decline. Lancet Neurol. 4422, 1–8. doi: 10.1016/S1474-4422(19)30368-0
Jessen, F., Amariglio, R. E., van Boxtel, M., Breteler, M., Ceccaldi, M., Chételat, G., et al. (2014). A conceptual framework for research on subjective cognitive decline in preclinical Alzheimer's disease. Alzheimers Dement. 10, 844–852. doi: 10.1016/j.jalz.2014.01.001
Jessen, F., Wiese, B., Bachmann, C., Eifflaender-Gorfer, S., Haller, F., Kölsch, H., et al. (2010). Prediction of dementia by subjective memory impairment: effects of severity and temporal association with cognitive impairment. Arch. Gen. Psychiatry 67, 414–422. doi: 10.1001/archgenpsychiatry.2010.30
Kennedy, K. M., and Raz, N. (2009). Pattern of normal age-related regional differences in white matter microstructure is modified by vascular risk. Brain Res. 1297, 41–56. doi: 10.1016/j.brainres.2009.08.058
Kern, S., Zetterberg, H., Kern, J., Zettergren, A., Waern, M., Höglund, K., et al. (2018). Prevalence of preclinical Alzheimer disease: Comparison of current classification systems. Neurology 90, e1682–e1691. doi: 10.1212/WNL.0000000000005476
Lebedeva, A., Institutet, K., Ume, A. S., Westman, E., Institutet, K., and Ume, T. O. (2018). Longitudinal relationships among depressive symptoms, cortisol, and brain atrophy in the neocortex and the hippocampus. Acta Psychiatr. Scand. 137, 491–502. doi: 10.1111/acps.12860
Leritz, E. C., Shepel, J., Williams, V. J., Lipsitz, L. A., McGlinchey, R. E., Milberg, W. P., et al. (2014). Associations between T1 white matter lesion volume and regional white matter microstructure in aging. Hum. Brain. Mapp. 35, 1085–1100. doi: 10.1002/hbm.22236
Li, X., Westman, E., Stahlbom, A. K., Thordardottir, S., Almkvist, O., Blennow, K., et al. (2015). White matter changes in familial Alzheimer's disease. J. Intern. Med. 278, 211–218. doi: 10.1111/joim.12352
Li, X.-Y., Tang, Z.-C., Sun, Y., Tian, J., Liu, Z.-Y., and Han, Y. (2016). White matter degeneration in subjective cognitive decline: a diffusion tensor imaging study. Oncotarget 7, 54405–54414. doi: 10.18632/oncotarget.10091
Liu, J., Yin, C., Xia, S., Jia, L., Guo, Y., Zhao, Z., et al. (2013). White matter changes in patients with amnestic mild cognitive impairment detected by diffusion tensor imaging. PLoS ONE 8:59440. doi: 10.1371/journal.pone.0059440
Machado, A., Barroso, J., Molina, Y., Nieto, A., Díaz-Flores, L., Westman, E., et al. (2018). Proposal for a hierarchical, multidimensional, and multivariate approach to investigate cognitive aging. Neurobiol. Aging. 71, 179–188. doi: 10.1016/j.neurobiolaging.2018.07.017
Molinuevo, J. L., Rabin, L. A., Amariglio, R., Buckley, R., Dubois, B., Ellis, K. A., et al. (2017). Implementation of subjective cognitive decline criteria in research studies. Alzheimers Dement. 13, 296–311. doi: 10.1016/j.jalz.2016.09.012
Muehlboeck, J.-S., Westman, E., and Simmons, A. (2014). TheHivedb image data management and analysis framework. Front. Neuroinform. 6:49. doi: 10.3389/fninf.2013.00049
Murphy, C. F., Gunning-Dixon, F. M., Hoptman, M. J., Lim, K. O., Ardekani, B., Shields, J. K., et al. (2007). White-matter integrity predicts stroop performance in patients with geriatric depression. Biol. Psychiatry 61, 1007–1010. doi: 10.1016/j.biopsych.2006.07.028
Nemy, M., Cedres, N., Grothe, M. J., Muehlboeck, J. S., Lindberg, O., Nedelska, Z., et al. (2020). Cholinergic white matter pathways make a stronger contribution to attention and memory in normal aging than cerebrovascular health and nucleus basalis of Meynert. Neuroimage 211:116607. doi: 10.1016/j.neuroimage.2020.116607
Ohlhauser, L., Parker, A. F., Smart, C. M., and Gawryluk, J. R. (2019). White matter and its relationship with cognition in subjective cognitive decline. Alzheimer's Dement. Diagn. Assess Dis. Monit. 11, 28–35. doi: 10.1016/j.dadm.2018.10.008
Perrotin, A., La Joie, R., de La Sayette, V., Barré, L., Mézenge, F., Mutlu, J., et al. (2017). Subjective cognitive decline in cognitively normal elders from the community or from a memory clinic: differential affective and imaging correlates. Alzheimers Dement. 13, 550–560. doi: 10.1016/j.jalz.2016.08.011
Perrotin, A., Mormino, E. C., Madison, C. M., Hayenga, A. O., and Jagust, W. J. (2012). Subjective cognition and amyloid deposition imaging: a Pittsburgh Compound B positron emission tomography study in normal elderly individuals. Arch. Neurol. 69, 223–229. doi: 10.1001/archneurol.2011.666
Pfeffer, R. I., Kurosaki, T. T., Harrah, C. H., Chance, J. M., and Filos, S. (1982). Measurement of functional activities in older adults in the community. J. Gerontol. 37, 323–329. doi: 10.1093/geronj/37.3.323
Rabin, L. A., Smart, C. M., and Amariglio, R. E. (2017). Subjective cognitive decline in preclinical Alzheimer's disease. Annu. Rev. Clin. Psychol. 13, 369–396. doi: 10.1146/annurev-clinpsy-032816-045136
Raz, N., Yang, Y., and Dahle, C. L. (2012). Volume of white matter hyperintensities in healthy adults: Contribution of age, vascular risk factors, and inflammation-related genetic variants. Biochim. Biophys. Acta. 1822, 361–369. doi: 10.1016/j.bbadis.2011.08.007
Reid, L. M., and Maclullich, A. M. J. (2006). Subjective memory complaints and cognitive impairment in older people. Dement. Geriatr. Cogn. Disord. 22, 471–85. doi: 10.1159/000096295
Riphagen, J. M., Gronenschild, E. H. B. M., Salat, D. H., Freeze, W. M., Ivanov, D., Clerx, L., et al. (2018). Shades of white : diffusion properties of T1- and FLAIR-defined white matter signal abnormalities differ in stages from cognitively normal to dementia. Neurobiol. Aging 68, 48–58. doi: 10.1016/j.neurobiolaging.2018.03.029
Salat, D., Tusch, D., van der Kouwe, A., Greve, D., Pappu, V., Lee, S., et al. (2010). White matter pathology isolates the hippocampal formation in Alzheimer's disease. Neurobiol. Aging 31, 244–256. doi: 10.1016/j.neurobiolaging.2008.03.013
Salat, D. H., Williams, V. J., Leritz, E. C., Schnyer, D. M., Rudolph, J. L., Lipsitz, L. A., et al. (2012). Inter-individual variation in blood pressure is associated with regional white matter integrity in generally healthy older adults. Neuroimage J. 59, 181–192. doi: 10.1016/j.neuroimage.2011.07.033
Selnes, P., Aarsland, D., Bjørnerud, A., Gjerstad, L., Wallin, A., Hessen, E., et al. (2013). Diffusion tensor imaging surpasses cerebrospinal fluid as predictor of cognitive decline and medial temporal lobe atrophy in subjective cognitive impairment and mild cognitive impairment. J. Alzheimers Dis. 33, 723–736. doi: 10.3233/JAD-2012-121603
Simmons, A., Westman, E., Muehlboeck, S., Mecocci, P., Vellas, B., Tsolaki, M., et al. (2011). The AddNeuroMed framework for multi-centre MRI assessment of Alzheimer's disease: experience from the first 24 months. Int. J. Geriatr. Psychiatry 26, 75–82. doi: 10.1002/gps.2491
Slot, R. E. R., Sikkes, S. A. M., Berkhof, J., Brodaty, H., Buckley, R., Cavedo, E., et al. (2018). Subjective cognitive decline and rates of incident Alzheimer's disease and non-Alzheimer's disease dementia. Alzheimer's Dement. 15, 465–476. doi: 10.1016/j.jalz.2018.10.003
Smith, S. M., Jenkinson, M., Johansen-Berg, H., Rueckert, D., Nichols, T. E., Mackay, C. E., et al. (2006). Tract-based spatial statistics: Voxelwise analysis of multi-subject diffusion data. Neuroimage 31, 1487–1505. doi: 10.1016/j.neuroimage.2006.02.024
Taylor, W. D., Aizenstein, H. J., and Alexopoulos, G. S. (2013). The vascular depression hypothesis: mechanisms linking vascular disease with depression. Mol. Psychiatry 18, 963–974. doi: 10.1038/mp.2013.20
Tingley, D., Yamamoto, T., Hirose, K., Keele, L., and Imai, K. (2014). Mediation: R Package for Causal Mediation Analysis. J. Stat. Soft. 59:1–38. doi: 10.18637/jss.v059.i05
van Harten, A. C., Mielke, M. M., Swenson-Dravis, D. M., Hagen, C. E., Edwards, K. K., Roberts, R. O., et al. (2018). Subjective cognitive decline and risk of MCI: the mayo clinic study of aging. Neurology 91, e300–e312. doi: 10.1212/WNL.0000000000005863
Voevodskaya, O. (2014). The effects of intracranial volume adjustment approaches on multiple regional MRI volumes in healthy aging and Alzheimer's disease. Front. Aging Neurosci. 6:264. doi: 10.3389/fnagi.2014.00264
Wang, L., van Belle, G., Crane, P. K., Kukull, W. A., Bowen, J. D., McCormick, W. C., et al. (2004). Subjective memory deterioration and future dementia in people aged 65 and older. J. Am. Geriatr. Soc. 52, 2045–2051. doi: 10.1111/j.1532-5415.2004.52568.x
Wang, Y., West, J. D., Flashman, L. A., Wishart, H. A., Santulli, R. B., Rabin, L. A., et al. (2012). Selective changes in white matter integrity in MCI and older adults with cognitive complaints. Biochim. Biophys. Acta 1822, 423–430. doi: 10.1016/j.bbadis.2011.08.002
Wardlaw, J. M., Smith, E. E., Biessels, G. J., Cordonnier, C., Fazekas, F., Frayne, R., et al. (2013). Neuroimaging standards for research into small vessel disease and its contribution to ageing and neurodegeneration. Lancet Neurol. 12, 822–838. doi: 10.1016/S1474-4422(13)70124-8
Winblad, B., Palmer, K., Kivipelto, M., Jelic, V., Fratiglioni, L., Wahlund, L.-O., et al. (2004). Mild cognitive impairment–beyond controversies, towards a consensus: report of the International Working Group on Mild Cognitive Impairment. J. Intern. Med. 256, 240–246. doi: 10.1111/j.1365-2796.2004.01380.x
Yates, J. A., Clare, L., Woods, R. T., Matthews, F. E., and Cognitive Function and Ageing Study Wales (2015). Subjective memory complaints are involved in the relationship between mood and mild cognitive impairment. J. Alzheimers Dis. 48(Suppl. 1), S115–S123. doi: 10.3233/JAD-150371
Yesavage, J. A., Brink, T. L., Rose, T. L., Lum, O., Huang, V., Adey, M., et al. (1982). Development and validation of a geriatric depression screening scale: a preliminary report. J. Psychiatr. Res. 17, 37–49. doi: 10.1016/0022-3956(82)90033-4
Zhou, Y., Lin, F., Zhu, J., Zhuang, Z., guo, Li, Y., sheng Tao, J., et al. (2008). Whole brain diffusion tensor imaging histogram analysis in vascular cognitive impairment. J. Neurol. Sci. 268, 60–64. doi: 10.1016/j.jns.2007.11.005
Zlatar, Z. Z., Moore, R. C., Palmer, B. W., Thompson, W. K., and Jeste, D. V. (2014). Cognitive complaints correlate with depression rather than concurrent objective cognitive impairment in the successful aging evaluation baseline sample. J. Geriatr. Psychiatry Neurol. 27, 181–187. doi: 10.1177/0891988714524628
Keywords: subjective cognitive decline, subjective cognitive complaints, DTI, mean diffusivity, cerebrovascular disease, depressive symptomatology, mediation
Citation: Diaz-Galvan P, Cedres N, Figueroa N, Barroso J, Westman E and Ferreira D (2021) Cerebrovascular Disease and Depressive Symptomatology in Individuals With Subjective Cognitive Decline: A Community-Based Study. Front. Aging Neurosci. 13:656990. doi: 10.3389/fnagi.2021.656990
Received: 21 January 2021; Accepted: 28 May 2021;
Published: 27 July 2021.
Edited by:
Jiu Chen, Nanjing Medical University, ChinaReviewed by:
Julie Suhr, Ohio University, United StatesKiyoka Kinugawa, Hôpital Charles-Foix, France
Copyright © 2021 Diaz-Galvan, Cedres, Figueroa, Barroso, Westman and Ferreira. This is an open-access article distributed under the terms of the Creative Commons Attribution License (CC BY). The use, distribution or reproduction in other forums is permitted, provided the original author(s) and the copyright owner(s) are credited and that the original publication in this journal is cited, in accordance with accepted academic practice. No use, distribution or reproduction is permitted which does not comply with these terms.
*Correspondence: Daniel Ferreira, ZGFuaWVsLmZlcnJlaXJhLnBhZGlsbGFAa2kuc2U=
†These authors share first authorship
‡These authors share last authorship