- 1School of Public Health, Capital Medical University, Beijing, China
- 2Beijing Key Laboratory of Environmental Toxicology, Beijing, China
- 3Beijing Key Laboratory of Clinical Epidemiology, Beijing, China
Vitamin A deficiency (VAD) plays an essential role in the pathogenesis of Alzheimer’s disease (AD). However, the specific mechanism by which VAD aggravates cognitive impairment is still unknown. At the intersection of microbiology and neuroscience, the gut-brain axis is undoubtedly contributing to the formation and function of neurological systems, but most of the previous studies have ignored the influence of gut microbiota on the cognitive function in VAD. Therefore, we assessed the effect of VAD on AD pathology and the decline of cognitive function in AD model mice and determined the role played by the intestinal microbiota in the process. Twenty 8-week-old male C57BL/6J amyloid precursor protein/presenilin 1 (APP/PS1) transgenic mice were randomly assigned to either a vitamin A normal (VAN) or VAD diet for 45 weeks. Our results show that VAD aggravated the behavioral learning and memory deficits, reduced the retinol concentration in the liver and the serum, decreased the transcription of vitamin A (VA)-related receptors and VA-related enzymes in the cortex, increased amyloid-β peptides (Aβ40 and Aβ42) in the brain and gut, upregulate the translation of beta-site APP-cleaving enzyme 1 (BACE1) and phosphorylated Tau in the cortex, and downregulate the expression of brain-derived neurotrophic factor (BDNF) and γ-aminobutyric acid (GABA) receptors in the cortex. In addition, VAD altered the composition and functionality of the fecal microbiota as exemplified by a decreased abundance of Lactobacillus and significantly different α- and β-diversity. Of note, the functional metagenomic prediction (PICRUSt analysis) indicated that GABAergic synapse and retinol metabolism decreased remarkably after VAD intervention, which was in line with the decreased expression of GABA receptors and the decreased liver and serum retinol. In summary, the present study provided valuable facts that VAD exacerbated the morphological, histopathological, molecular biological, microbiological, and behavioral impairment in the APP/PS1 transgenic mice, and the intestinal microbiota may play a key mediator role in this mechanism.
Introduction
Alzheimer’s disease (AD) is a devastating neurological disease characterized by a loss of cognitive function and a gradual decline in daily life activities, accompanied by behavioral changes and various neuropsychiatric symptoms (Joe and Ringman, 2019). It is predicted that there will be 131.5 million patients suffering from AD in the world by 2050 (Hsiao et al., 2018). Although the pathological process of AD is extremely complex and has not been fully elucidated so far, it is known that the typical pathological features of AD are the formation and deposition of extracellular β-amyloid (Aβ) and intracellular neurofibrillary tangles formed by the excessive phosphorylation of Tau protein in neurons, causing a series of reactions, such as the release of inflammatory factors, energy metabolism disorders, and oxidative stress in neurons, and eventually, leading to the degeneration and loss of neurons in cerebral cortex and hippocampus (Reitz et al., 2011). Therefore, preventing the formation of these pathological phenomena will be the key to solve this problem.
As an essential micronutrient, vitamin A (VA) and its derivatives are closely related to the development of the central nervous system (CNS) (Malaspina and Michael-Titus, 2008) and are essential for normal learning and memory functions (Sherwin et al., 2012). VA deficiency (VAD) is one of the most significant micronutrient deficiencies that pose a severe threat to the health of patients with AD in many countries (Lopes et al., 2014). It has been found that VA and β-carotene levels in patients with AD are significantly lower than those in normal controls, which may be partly attributed to the alterations in dietary behavior (Lopes et al., 2014).
It is of great significance to assess the effect of VAD on the pathogenesis of AD. As an active metabolite of VA, retinoic acid (RA) binds to the retinoic acid receptors (RARs) on the nuclear membrane and stimulates the transcription of target genes, participating in the regulation of organ formation and development. Nuclear retinoid X receptors (RXRs) areRA receptors activated by the 9-cis RA and potentially other endogenous retinoids in the CNS (Krezel et al., 1999). The RAR-RXR heterodimer could combine with the RA response element (RARE) in the promoter region of the target genes, thereby regulating the AD-related gene expression (Maden, 2007). In addition, retinaldehyde dehydrogenase 1 (RALDH1) has two main functions: one is to increase the synthesis of RA when retinol is less available; the other is to participate in the synthesis of neurotransmitters, such as γ-aminobutyric acid (GABA). Furthermore, RAR dysfunction can lead to Aβ deposition, impairment of the long-term synaptic plasticity, and memory in the brain of the patients with AD, which can be rescued by the VA supplementation (Misner et al., 2001; Nomoto et al., 2012). It was previously observed that VA can modulate the expression of β-amyloid precursor protein (APP) and the α-secretase to reduce the formation of the oligomerization of Aβ40/Aβ42 in vitro (Shudo et al., 2009). Moreover, Wang et al. (2015) demonstrated that VA could reduce the expression of beta-site APP-cleaving enzyme 1 (BACE1), which is a crucial enzyme involved in catalyzing the formation of Aβ polypeptide and cleavage of APP (Leng and Edison, 2020).
The gut microbiota is considered as an invisible organ, which plays a mediating role in the bidirectional signal transduction between the gut-brain axis (Petra et al., 2015). The gut-brain axis conducts bidirectional communication between the gut and the brain through the immune system, enteric nervous system, microbial metabolites, and vagus nerve, which ultimately contributes to the formation and function of neurological systems (Doifode et al., 2021). Many specific bacterial genera in the gut, such as Lactobacillus, regulate the expression of specific receptors in the brain via the vagus nerve (Bravo et al., 2011). Additionally, the intestinal microbiota is known to secrete immunogenic mixtures into the surrounding environment, such as amyloids, lipopolysaccharides, and other microbial exudates, which may regulate the signaling pathways and produce proinflammatory cytokines in the AD pathogenesis (Zhao et al., 2015). Previous research has indicated that the intestinal microbiota composition can be regulated by diet and specific nutrients, resulting in the production or aggregation of amyloid protein in the brain (Scott et al., 2013). Therefore, the gut-brain axis may play an important role in the process of VAD aggravating the cognitive function of AD.
Emerging evidence shows that VAD in the different life stages could impair gut microbiota homeostasis, leading to the imbalance of Firmicutes and Bacteroidetes in rat colonic mucosal microbiota (Chen et al., 2020). Amit-Romach et al. (2009) illustrated that VAD could change the composition of gut microbiota and damage the integrity of the gastrointestinal mucosal barrier by reducing the abundance of Lactobacillus and the total bacterial count in the gastrointestinal tract. In addition, the alterations in the gut microbial community can reduce the level of brain-derived neurotrophic factor (BDNF), which is considered a genuine molecular mediator of functional and morphological synaptic plasticity in the brain (Bercik et al., 2011). Thus, alterations in the function and composition of the intestinal microbiota can help to recognize the new mechanisms that account for the impact of VAD on the pathogenesis of AD.
To date, the specific mechanism of how VA affects the cognitive function of patients with AD has not been clarified. This research aimed to assess the effect of VAD on the cognitive function and pathological mechanism of AD and determine the intestinal microbiota changes driven by VAD in the amyloid precursor protein/presenilin 1 (APP/PS1) transgenic mice. Our study implies that VAD aggravates the decline of learning and memory function of AD, and intestinal microbiota may play an important role in this process.
Materials and Methods
Animals and Diet
In this study, 20 specific pathogen-free (SPF) male C57BL/6J APP/PS1 transgenic mice aged 8 weeks, weighing 20 ± 2 g, were purchased from Beijing Huafukang Biotechnology Co., Ltd. (Beijing, China), and were randomly separated into two parallel groups (n = 10 per group). They were raised in standard individual ventilated cages with a 12-h dark/light cycle in a room with controlled temperature (23–25°C) and humidity (45–55%). The VAD feed (VA < 120 IU/kg) and VA-normal feed (VAN; 15,000 IU/kg VA) were purchased from Keao Xieli Feed Co., Ltd. (Beijing, China). Both the feeds and drinking water were available to the mice ad libitum. Considering that the mouse species has a high VA storage capacity and needs a long time (up to 40 weeks) (Etchamendy et al., 2003) to induce VAD, we conducted a 45-week dietary intervention in the present experiment. After 45 weeks of dietary intervention, we assessed their cognitive deficits with the Morris water maze (MWM) test and the step-down passive avoidance (SDPA) test.
Reversed-Phase High-Performance Liquid Chromatography
Since the liver is the most important organ for storing VA and the serum retinol is a common indicator of VAD (WHO, 2009), we used reversed-phase high-performance liquid chromatography (RP-HPLC) to determine the concentration of retinol in the liver and the serum. The serum and liver samples were pretreated with acetonitrile to precipitate the protein. The resulting supernatant was added to 1,000 μl hexane, allowing the retinol to be extracted into hexane. The extract was evaporated to dryness under nitrogen, reconstituted in methanol, and then, separated with methanol in an isometric separation on an HPLC system (Agilent Technologies Inc., CA, United States) with a Pursuit XRs 100A C18 (4.6 mm × 250 mm) column. We set the flow rate to 0.8 ml/min and the detection wavelength to 325 nm to achieve a better detection effect. The peaks were identified by comparing the retention times with those of the standard samples.
Morris Water Maze Test
The spatial learning and memory abilities of mice were assessed by the MWM test, which includes orientation navigation and spatial probe tests. MWM was carried out in a stainless-steel circular pool with a diameter of 1.2 m and a height of 0.5 m. A round table with a diameter of 9 cm was placed in the first quadrant of the pool and placed 1 cm below the surface of the water. We added titanium dioxide to the pool water and mixed it evenly to make it milky white so that the animal cannot recognize the position of the platform in the pool.
The orientation navigation was carried out for 5 consecutive days. The mice were placed into the pool water facing the wall of the pool at different quadrant positions, and the time (the escape latency) and swimming trajectory of the mice from entering the water to finding the round platform within 90 s were recorded with an image automatic acquisition and software analysis system (Xin Ruan Information Technology Co., Ltd., Shanghai, China). Then, the spatial probe test was carried out on the sixth day. After the platform was removed, the mice were allowed to enter the pool in the quadrant opposite to the platform and search for the platform based on memory. The time spent in the target quadrant, the number of times across the platform, and the swimming trajectory were recorded.
Step-Down Passive Avoidance Test
The state-dependent learning and memory were evaluated by SDPA, which includes training sessions and testing sessions. The SPDA test was performed in a small chamber containing an insulated wooden platform at the corner and a floor covered with electrode strips. During the training session, the mice were put on a wooden platform and received electric shocks as soon as they stepped on the electrode strips on the floor. The number of times the mouse stepped down from the wooden platform (number of errors) was recorded. After 24 h, the testing session was performed. The electrode strips on the floor were no longer energized. The mice were placed on the wooden platform again, and the time of jumping from the wooden platform to the floor (step-down latency) was recorded.
Hematoxylin-Eosin Staining
The mouse brain tissue samples were stored in 10% formalin solution, dehydrated according to the conventional methods, and then embedded in paraffin. After the sections were deparaffinized with xylene and different ethanol concentrations, they were stained with hematoxylin for 15 min. Afterward, it was soaked in acidified hydrochloric acid ethanol for 5 s and stained with eosin for 4 min. Finally, each section was examined with a microscope slide scanner (3DHISTECH Co., Ltd., Budapest, Hungary).
Immunohistochemistry
The mouse brain sections were placed in a small box filled with ethylenediamine tetraacetic acid (EDTA) antigen retrieval buffer (pH 9.0) and boiled in a microwave oven for 5 min; then, placed in 3% hydrogen peroxide solution and incubated for 20 min to inactivate the endogenous peroxidase activity. Next, placed the slides in PBS (pH 7.4), and washed them three times with shaking on a decolorizing shaker, 5 min each time. Then, they were incubated overnight with the primary antibodies: anti-Aβ40-42 (1:500, Abcam, Cambridge, United Kingdom) and anti-BDNF (1:500, Abcam, Cambridge, United Kingdom). Covered the tissue with the horseradish peroxidase (HRP)-conjugated secondary antibody (1:2,000, Abcam, Cambridge, United Kingdom) and incubated for 60 min at room temperature in the dark chamber. Finally, it reacted with 3,3′-diaminobenzidine (DAB) solution for 10 min. Similar to the Hematoxylin-Eosin (H&E) sections, immunohistochemistry (IHC) sections are visualized using the Micro Slide Scanner (3DHISTECH Co, Ltd., Budapest, Hungary). Analytical software (Image J) was used to quantify the integral optical density (IOD) of Aβ and BDNF in each image.
Western Blot
The lysis buffer was prepared by adding 1 mM PMSF, 10 mM NaF, and 1 mM Na3VO3 to the RIPA buffer (50 mM Tris buffer saline, 0.5% sodium deoxycholate, 1 mM EDTA, 150 mM NaCl, and 1% NP-40). Total proteins from the mouse cortical tissues were extracted using the lysis buffer. The concentrations of the protein in the extracts were determined by using the BCA test kit (Servicebio Technology Co., Ltd., Wuhan, China) according to the instructions from the manufacturer. Then, 30 (μg of protein was added to each lane, and the protein was separated by sodium dodecyl sulfate-polyacrylamide gel electrophoresis (SDS-PAGE), and then transferred to polyvinylidene fluoride (PVDF) membranes (Merck Millipore Co., Ltd., Darmstadt, Germany). After blocking with 5% (w/v) bovine serum albumin (BSA) for 2 h at room temperature, the membranes were incubated with the following primary antibodies overnight at 4°C: anti-BACE1 (1:1,000, Abcam, Cambridge, United Kingdom), anti-Tau (1:1,000, Abcam, Cambridge, United Kingdom), anti-phosphorylated-Tau (phospho S396, 1:1,000, Abcam, Cambridge, United Kingdom), anti-BDNF (1:1,000, Abcam, Cambridge, United Kingdom), anti-GABAAα2 (1:1,000, Abcam, Cambridge, United Kingdom), anti-GABAB1b (1:1,000, Abcam, Cambridge, United Kingdom), and anti-beta actin (1:5,000, Abcam, Cambridge, United Kingdom). Afterward, the PVDF membrane was incubated with the HRP-conjugated secondary antibody (1:5,000, Abcam, Cambridge, United Kingdom) at room temperature for 1 h. After that, the membranes were stripped by the stripping buffer (Beijing Solarbio Science & Technology Co., Ltd., Beijing, China), and reprobed with β-actin antibody as a loading control. Immunoreactive bands were visualized using the ECL Plus Reagent kit (Merck Millipore Co., Ltd., Darmstadt, Germany). The protein bands were quantified by densitometry using the Image System Fusion FX (Vilber Lourmat Co., Ltd., Paris, France) and normalized to β-actin.
Real-Time Quantitative PCR
Total RNA of the hippocampus and cortex of the APP/PS1 mice was extracted with the TRIzol® reagent (Thermo Fisher Scientific Co., Ltd., MA, United States). The bacterial genomic DNA was extracted from the feces of the mice with the TIANamp Stool DNA Kit (Tiangen Biotech Co., Ltd, Beijing, China). To ensure the purity of RNA, we used a Genomic DNA pollution scavenger (Applygen Biotech Co., Ltd, Beijing, China) to remove the genomic DNA from the RNA extract. RNA and DNA quality and yield were determined by the ratio of OD260/OD280 with an ultraviolet spectrophotometer (NanoDrop R ND-1000, Thermo Fisher Scientific Co., Ltd., MA, United States). Then, 1 μg of the total RNA from each sample was reverse-transcribed using a RevertAid First Strand cDNA Synthesis kit (Thermo Fisher Scientific Co., Ltd., MA, United States) as prescribed by the supplier. Real-time quantitative PCR (RT-qPCR) was conducted with a Real-time PCR Detection System (Bio-Rad Laboratories Co., Ltd., MA, United States) using KAPA SYBR® FAST qPCR Master Mix (2X) Kit (KAPA Biosystems, Co., Ltd., MA, United States) based on the instructions from the manufacturer. The housekeeping gene β-actin served as the reference for standardization in the hippocampus and cortex, while the universal 16S rRNA gene was used as an internal reference in the fecal samples. The target genes and DNA levels relative to internal reference were quantitatively determined by the 2–ΔΔCT method. The sequences of the primers, such as the genus-specific primer for Lactobacillus and Clostridia_UCG-014 used for RT-qPCR, are listed in Table 1.
Luminex xMAP® Technology
The concentrations of Aβ40 and Aβ42 in the brain and gut of the mice were measured using the Luminex xMAP® technology and a specific multiplex plate, MILLIPLEX® MAP Mouse Amyloid Beta Magnetic Bead Panel kit (Merck Millipore, Co., Ltd., Darmstadt, Germany) following the instructions from the manufacturer. The Luminex xMAP® technology is to covalently cross-link the antibody molecules against different test substances to specifically coded microspheres and then use flow cytometry to detect the corresponding items of each coded microsphere. The multiplex plate was measured using a Luminex 200® analyzer (Luminex, Co., Ltd., TX, United States). The concentrations of Aβ40 and Aβ42 were analyzed using the ELISAcalc software with a five-parameter logistic curve-fitting method.
Bacterial 16S rRNA Gene Sequencing
As mentioned above, the fecal bacterial genomic DNA is extracted using the TIANamp Stool DNA Kit (Tiangen Biotech Co., Ltd., Beijing, China) according to the instruction from the manufacturer. The library of each sample was generated by PCR amplification using the universal specific primers 338F (5′-ACTCCTACGGGAGGCAGCAG-3′) and 806R (5′-GGACTACHVGGGTWTCTAAT-3′) targeted in the variable V3–V4 regions of 16S rRNA (Caporaso et al., 2011). The expected amplicon size is about 468 bp, and then AMPure XP beads (Beckman Coulter, Inc., CA, United States) are used to purify these amplicons to remove the unbound primers or primer dimers. The DNA concentration of the amplified sequence library was determined using the Qubit quantification system (Thermo Fisher Scientific Co., Ltd., MA, United States). Then, the paired-end sequencing was conducted on the purified libraries using the Illumina MiSeq PE 300 platform (Illumina, Inc., CA, United States).
Bioinformatic Analysis
As previously described (Edgar, 2013), the operational taxonomic units (OTUs) were clustered using UPARSE (version 7.0) with the threshold of 97% sequence similarity. The Ribosomal Database Project (RDP) Bayesian algorithm was conducted to taxonomy up to the genus level using the Greengenes 13_8 reference database with a confidence threshold of 70% (McDonald et al., 2012). After filtering out the nonaligned and chimeric OTUs and singletons, the final OTU table is generated using Quantitative Insights Into Microbial Ecology 2 (QIIME2) (Bolyen et al., 2019). The difference in abundance between the two groups was analyzed by Mann–Whitney U test and Metastats analysis. The intestinal microbiota diversity within a single sample, namely α-diversity, was assessed using the four indices, the Shannon, Chao1, Ace, and Faith’s phylogenetic diversity (PD) indices, which describe the richness and evenness of each sample. Welch’s t-test was performed to assess α-diversity between the two groups. To assess the β-diversity, the principal coordinates analysis (PCoA) plots were obtained based on the unweighted UniFrac distances (Lozupone and Knight, 2005), which were visualized using the Emperor 0.9.4 (Vazquez-Baeza et al., 2013). A permutational multivariate ANOVA (PERMANOVA) was performed to quantify the difference in the β-diversity analysis. The linear discriminant analysis (LDA) effect size (LEfSe) analysis was performed to evaluate the differentially abundant taxa and biological relevance across the groups with a score cutoff of 2. Functional potential profiling of the microbial communities was predicted from the Kyoto Encyclopedia of Gene and Genomes (KEGG) database using the phylogenetic investigation of communities by reconstruction of unobserved states (PICRUSt) (Kanehisa et al., 2012). Spearman rank correlation was performed to examine the associations of the liver retinol and the serum retinol with the abundance of the genus by using R (version 4.0.0) (R Core Team, Vienna, Austria).
Statistical Analysis
Excluding the microbiota bioinformatic analysis mentioned above, the data from other analyses were represented as mean ± SD. A two-tailed, unpaired Student’s t-test was used to compare the difference between the two groups by using the SPSS 23.0 software (IBM, NY, United States). The differences were considered statistically significant at P < 0.05, and presented by the asterisks as follows: ∗P < 0.05, ∗∗P < 0.01, and ∗∗∗P < 0.001.
Results
Vitamin A Deficiency Reduced Liver and Serum Retinol Levels in the Amyloid Precursor Protein/Presenilin 1 Transgenic Mice
Compared with the VAN-diet-fed mice, there was no significant decrease in the bodyweight of the VAD-diet-fed mice after 45 weeks of VA deprivation (n.s.; Figure 1A). However, as shown in Figure 1B, the liver-to-body weight ratio was markedly decreased in the VAD group compared with the VAN group (P < 0.05). To confirm whether the established mice model was VAD or marginal VAD, we detected the serum retinol (Figure 1C) and found that the serum concentration of VAD-diet-fed mice matched the recommended standards (WHO, 2009) for VAD (≤0.7 μmol/L). Considering that the liver is the primary organ for VA storage in the body, we measured the retinol level in the liver by RP-HPLC and found that it was significantly decreased in the VAD group compared with the VAN group (P < 0.01; Figure 1D). The representative HPLC traces of retinol in the liver and serum are shown in Supplementary Figures 1, 2.
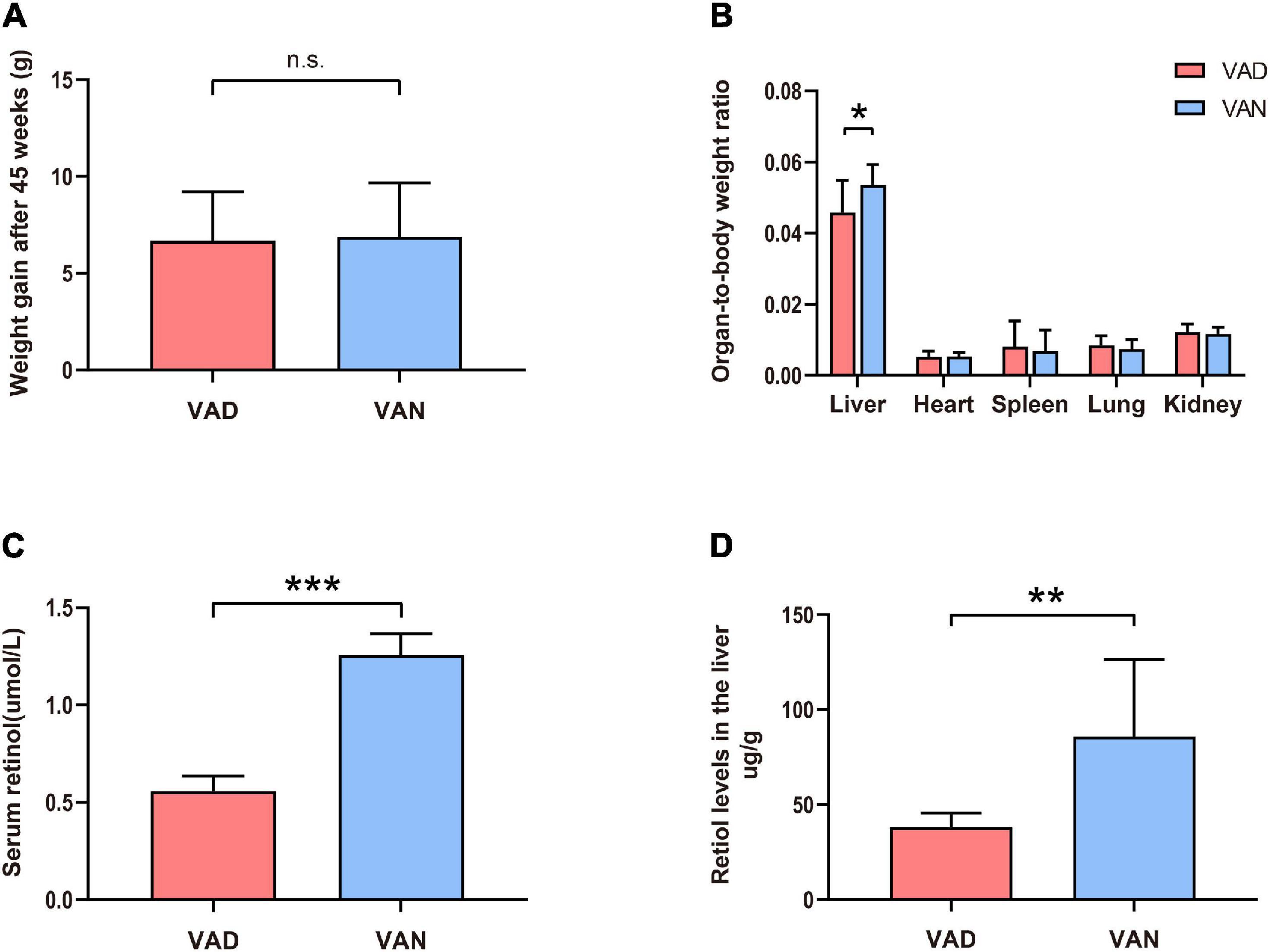
Figure 1. Consumption of a vitamin A deficiency (VAD)-diet for 45 weeks led to reductions in the serum and liver retinol levels. (A) Weight gain of the amyloid precursor protein/presenilin 1 (APP/PS1) transgenic mice after 45 weeks on VAD-diet. (B) The organ-to-body weight ratio in the APP/PS1 transgenic mice after 45 weeks on the VAD-diet. (C) The serum retinol level of the APP/PS1 transgenic mice after 45 weeks on VAD-diet. (D) The liver retinol level of the APP/PS1 transgenic mice after 45 weeks on VAD-diet. VAD, vitamin A deficiency diet; VAN, vitamin A normal diet. n = 10 per group. ∗P < 0.05, ∗∗P < 0.01, ∗∗∗P < 0.001, and n.s., non-significant.
Vitamin A Deficiency Reduced the mRNA Expression of Vitamin A-Related Receptors and Vitamin A-Related Enzymes in the Cortex
Compared with the VAN group, the transcription of the RARγ, RALDH1, RXRα, RXRβ, and RXRγ genes in the cortex were markedly decreased in the VAD group (P < 0.05; Figure 2). Furthermore, VAD decreased the expression of CYP26B1, a catabolizing enzyme that degrades the RA (Woloszynowska-Fraser et al., 2020), in the cortex of APP/PS1 mice (P < 0.05; Figure 2). Besides, the transcription of the RARα, RARβ, RALDH2, and RALDH3 genes was reduced in the VAD group compared with the VAN group, but the differences were not statistically significant (n.s.; Figure 2).
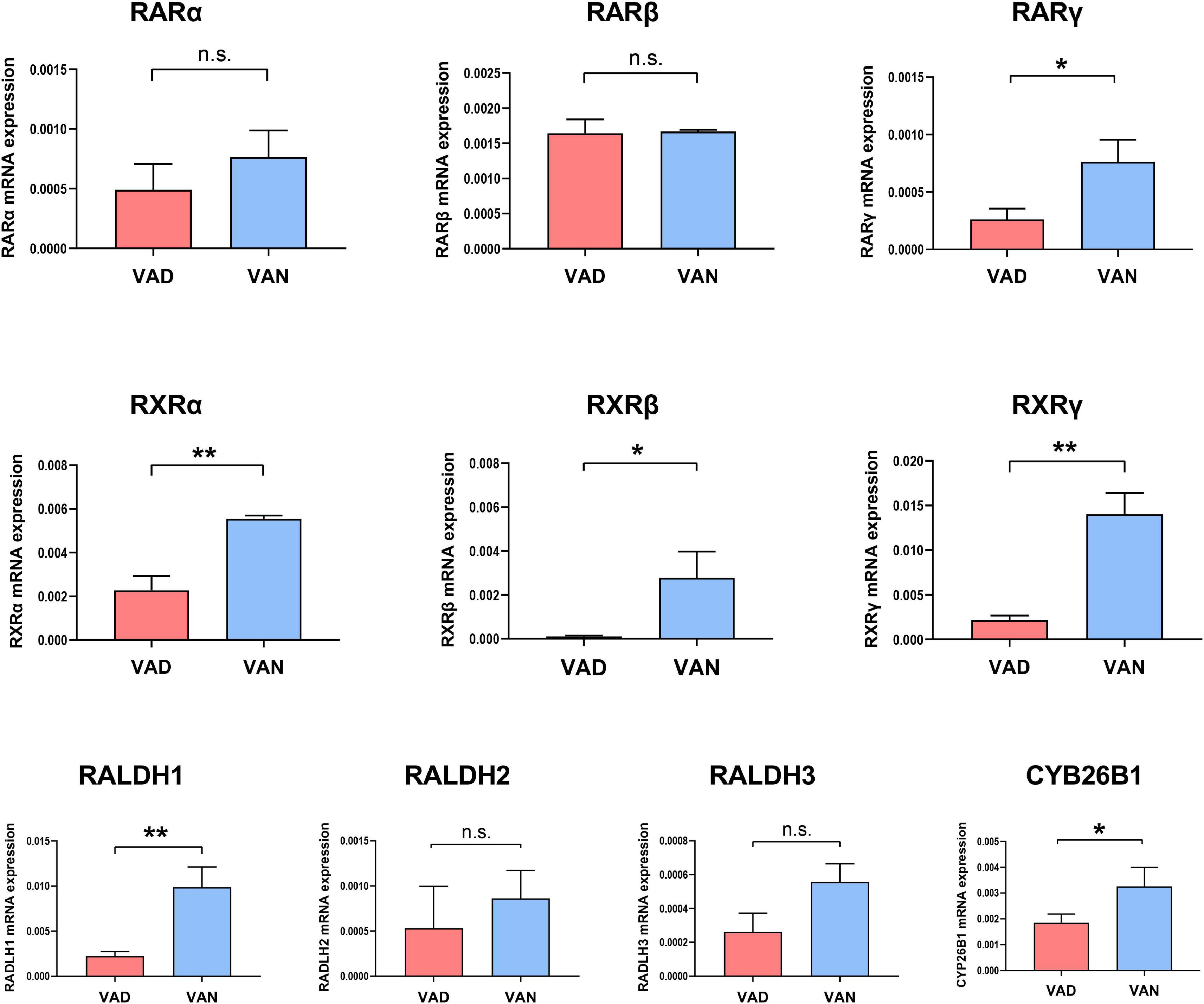
Figure 2. Impact of consumption of the VAD-diet for 45 weeks on the mRNA expression of the vitamin A (VA)-related receptors and VA-related enzymes in the cortex of the APP/PS1 transgenic mice. The expression of retinoic acid receptors (RAR-α, RAR-β, and RAR-γ), retinoid X receptors (RXR-α, RXR-β, and RXR-γ), retinaldehyde dehydrogenases (RALDH1, RALDH2, and RALDH3), and CYP26B1 were determined by real-time quantitative PCR (RT-qPCR). VAD, vitamin A deficiency diet; VAN, vitamin A normal diet. n = 3 per group. ∗P < 0.05, ∗∗P < 0.01, and n.s., non-significant.
Vitamin A Deficiency Exacerbated β-Amyloid Deposition, Tau Phosphorylation, and Pathological Degeneration
From the results of H&E staining, it can be seen that there may be some histopathological changes in the cortex and hippocampus of VAD-diet-fed mice, such as sparse and disordered neuron arrangement (Figure 3A).
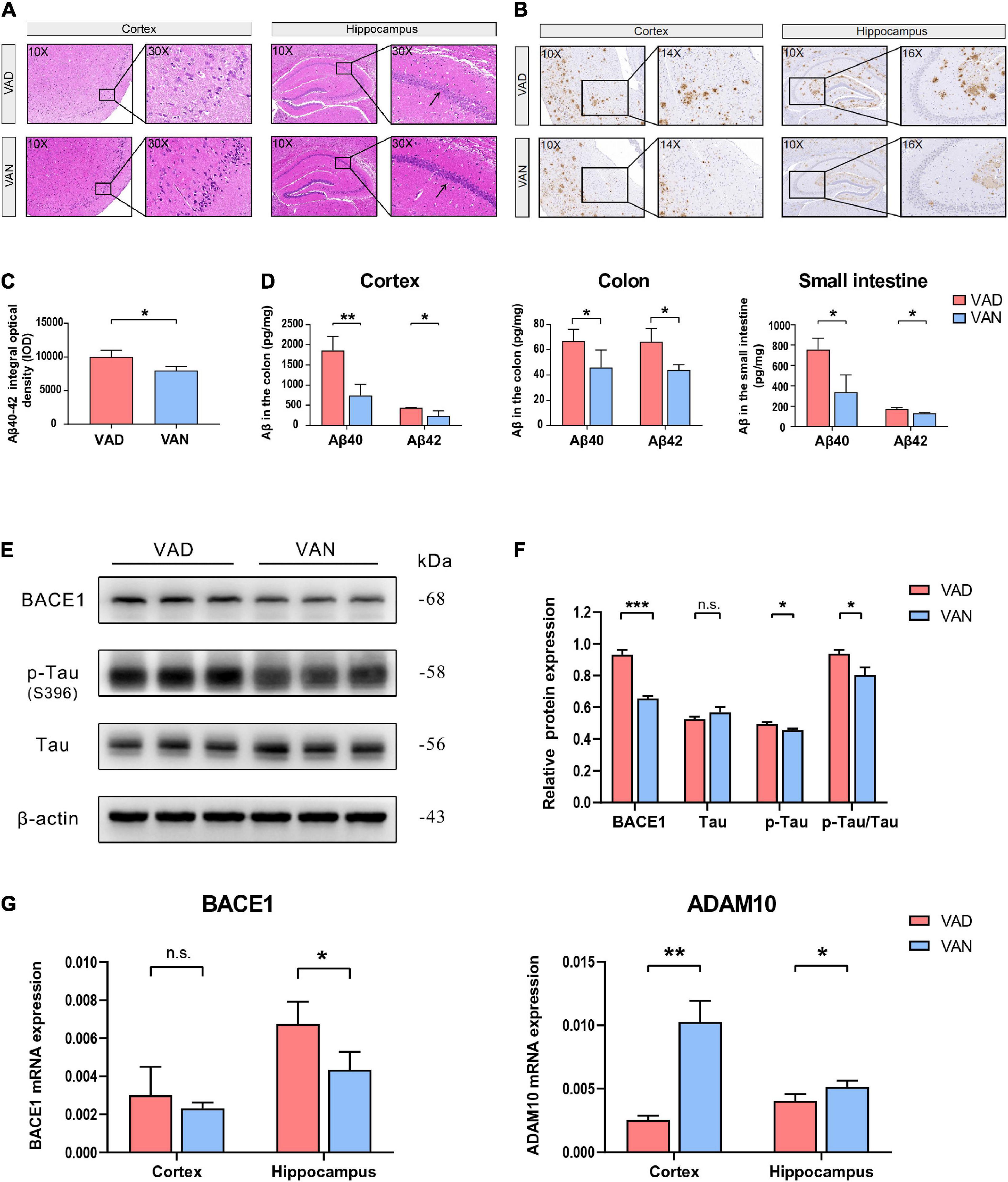
Figure 3. Consumption of the VAD diet for 45 weeks exacerbated the AD-related pathological changes in the cortex, hippocampus, colon, and small intestine of the APP/PS1 transgenic mice. (A) Hematoxylin-Eosin (H&E) staining (10× and 30×) in the brain of the APP/PS1 mice. (B) Immunohistochemistry (IHC) staining (10×, 14×, and 16×) of Aβ40 and Aβ42 depositions in the brain of the APP/PS1 mice. The primary antibody used for IHC staining could specifically bind both the Aβ40 and Aβ42. (C) Quantification of the IHC staining of the Aβ40 and Aβ42 depositions in the cortex of the APP/PS1 mice. (D) The Aβ40 and Aβ42 concentrations in the cortex, colon, and small intestine as determined by the Luminex immunoassay. The Luminex immunoassay can detect the concentration of Aβ40 and Aβ42, respectively. (E) Western blot (WB) images showing the level of BACE1, Tau proteins, and the phosphorylated Tau (p-Tau, S396) protein in the cortex of the APP/PS1 mice. (F) Quantification of the WB result showing the relative level of BACE1, Tau, p-Tau, and the ratio of p-Tau toTau. (G) The RT-qPCR result showing the mRNA level of the BACE1 and ADAM10 genes in the cortex and hippocampus of the APP/PS1 mice. VAD, vitamin A deficiency diet; VAN, vitamin A normal diet. n = 3 per group. ∗P < 0.05, ∗∗P < 0.01, ∗∗∗P < 0.001, and n.s., non-significant.
To assess the effect of VA deprivation on the Aβ depositions, IHC staining of the brain tissue with the specific Aβ antibody was carried out, and it showed a remarkable increment of Aβ plaque burden in the brain of the VAD mice as compared with the VAN group (Figure 3B). Moreover, our IHC relative quantitative results advocated that the IOD value of Aβ was greatly increased in the VAD mice compared with that in the VAN mice (P < 0.05; Figure 3C).
Consistent with the IHC staining, the Luminex results showed that Aβ40 and Aβ42 in the cortex were remarkably increased in the VAD group compared with the VAN group (P < 0.05; Figure 3D). Intriguingly, we found that the Aβ burden of the colon and small intestine increased after 45-week VAD-diet consumption (P < 0.05; Figure 3D).
As shown in Figures 3E,F, the expression of the BACE1 and p-Tau in the cortex of mice in the VAD group were notably higher than those in the VAN group (P < 0.05). Additionally, the ratio of p-Tau to Tau in the VAD group was higher than that in the VAN group (P < 0.05).
The mRNA expression of BACE1 in both the cortex and hippocampus of the VAD group was higher than that of the VAN group, but the difference was statistically significant only in the hippocampus (Figure 3G). Moreover, VAD decreased the expression of ADAM10, an enzyme that decreases the formation of Aβ from APP(Shudo et al., 2009), in the cortex and hippocampus (P < 0.05; Figure 3G).
Given the findings described above, VAD intensified Aβ generation, Tau phosphorylation, and pathological degeneration in the AD model mice.
Vitamin A Deficiency Aggravated the Learning and Memory Deficits in Amyloid Precursor Protein/Presenilin 1 Transgenic Mice
To examine the effect of VAD on spatial learning and memory ability, the MWM test was performed. During the orientation navigation, the escape latency to the target platform during the 5 consecutive days is illustrated in Figure 4A. On the third and the fifth day of the test, the escape latency of the VAD group was prolonged as compared with the VAN group (P < 0.05). Moreover, the VAD mice traveled longer distances than the VAN mice to reach the platform (P < 0.01; Figure 4B,C). In the spatial probe test, compared with the VAN mice, the VAD mice traveled more randomly in the tank without knowing the target location, which demonstrates poor performance in the spatial learning and memory tasks (Figure 4D). Furthermore, the VAD mice spent less time on the target quadrants and crossed the target platform less frequently than the VAN group, but only the difference in the time of target quadrants was statistically significant (Figures 4E,F).
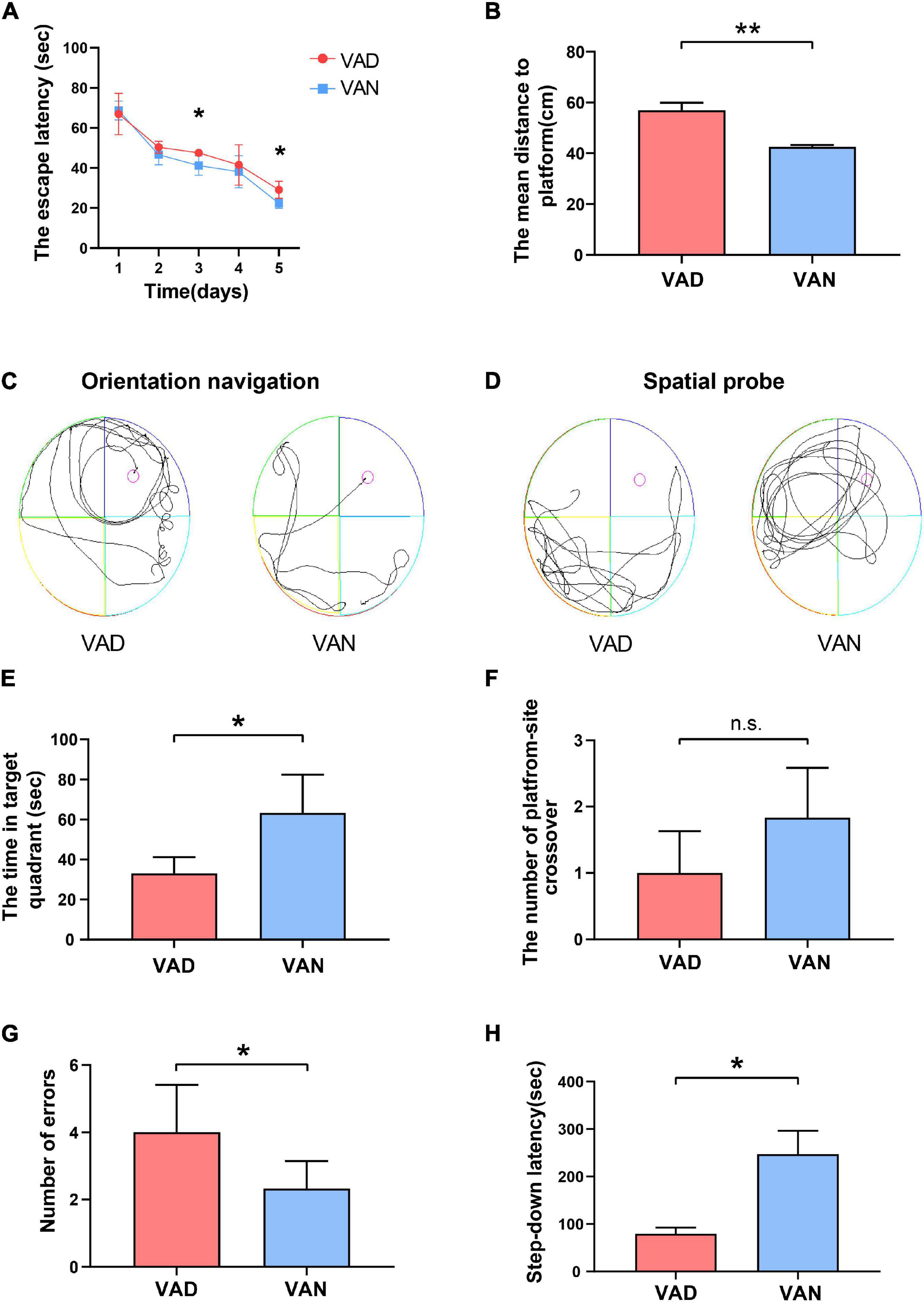
Figure 4. Consumption of the VAD diet for 45 weeks aggravated the learning and memory deficits of the APP/PS1 transgenic mice. (A) The escape latency (time to reach the escape platform over days) in the Morris water maze (MWM) test. (B) The escape distance in the MWM test. (C) The orientation navigation path in the MWM test. (D) The spatial probe path in the MWM test. (E) The target-quadrant abidance was measured in the MWM test. (F) The crossing-target number was measured in the MWM test. (G) The error frequency to step down was measured in the step-down passive avoidance (SDPA) test. (H) The latency of the step-down response was measured in the SDPA test. VAD, vitamin A deficiency diet; VAN, vitamin A normal diet. n = 10 per group. ∗P < 0.05, ∗∗P < 0.01, and n.s., non-significant.
We conducted the SDPA tests to observe the state-dependent learning and memory, 1 day after completing the MWM tests. As illustrated in Figure 4G, the average number of errors in the VAD mice was remarkably higher than in the VAN mice (P < 0.05). Moreover, compared with the VAN group, the latency to step-down was notably shortened in the VAD group (P < 0.05; Figure 4H).
Both the MWM and SDPA test indicated that VAD could aggravate the learning and memory impairment, thus exacerbating the cognitive deficits in the APP/PS1 transgenic mice.
Vitamin A Deficiency Altered the Structure and Function of the Gut Microbiota in Amyloid Precursor Protein/Presenilin 1 Transgenic Mice
To evaluate the effect of VAD on the intestinal microbiota, sequencing of the V3–V4 regions of the bacterial 16S rRNA was performed on the fecal samples. In the phylum level, the Firmicutes (F)/Bacteroidetes (B) ratio of the VAD group and the VAN group were 1.95 and 3.61, respectively, as displayed in Figure 5A. Community abundance at class, order, and family level are shown in Supplementary Figure 3. Of note, the VAD mice exhibited an enrichment of some potential pro-AD microorganisms, such as Clostridia_UCG-014, and a reduction in the abundance of other potential anti-AD microorganisms, such as Lactobacillus, compared with the VAN mice (Figures 5B,D,E; P < 0.05). The data of Mann–Whitney U test and Metastats analysis for all genera are shown in Supplementary Tables 1, 2. Venn diagram at the genus level (Figure 5C) revealed that 120 genera were shared by the VAD and the VAN group, but mice on the VAD-diet have more genus than those on the VAN-diet. After VAD intervention, three genera (Formosa, Rhodobacteraceae, and Alloprevotella) were missing, while 19 new genera (Sphingomonas, Peptoniphilus, Rodentibacter, etc.) have appeared (Supplementary Table 3). Interestingly, we found that most of the 19 genera in the VAD diet are Gram-positive microbes, while the three genera in the VAN diet are Gram-negative microbes. As a result, the α-diversity indices (Shannon, Chao1, ACE, and Faith’s PD) in the VAD group were remarkably higher than those in the VAN group (P < 0.05; Figures 6A–D). It means that, compared with the VAN-diet, the consumption of the VAD-diet for 45 weeks increased the community evenness and richness in the fecal microbiota of the APP/PS1 mice. The PCoA plot and the hierarchical clustering tree manifested that most of the samples could be separated according to their diets (Figures 6E,F). The dissimilarity of the microbiota between the two groups was further confirmed by the statistical analysis of the differences with the PERMANOVA analysis (P = 0.002).
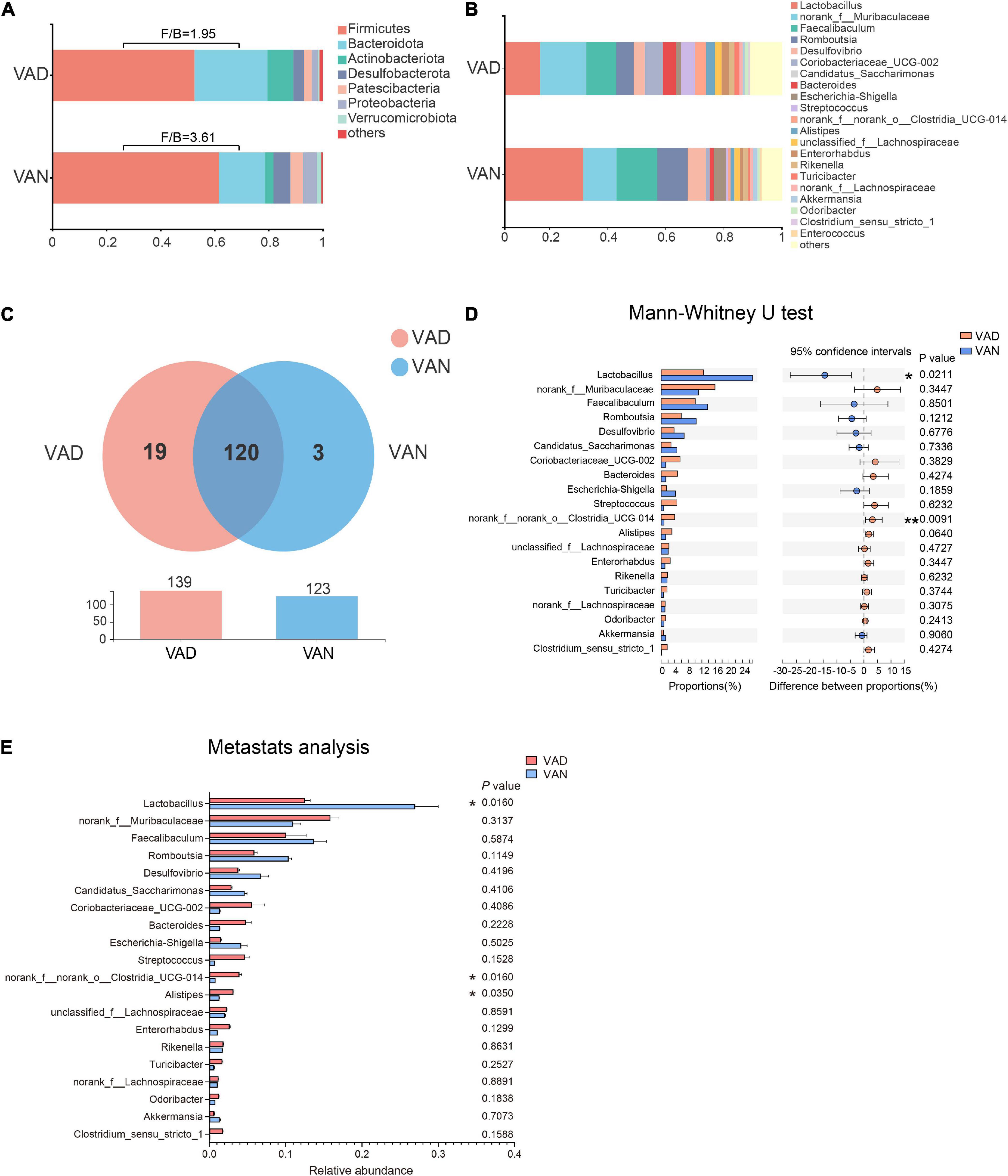
Figure 5. Consumption of the VAD diet for 45 weeks altered the gut microbiota of APP/PS1 transgenic mice. (A) The fecal microbiota composition at the phylum level. (B) The fecal microbiota composition at the genus level. (C) Venn diagram illustrated the overlap and number of the genus identified in the fecal samples obtained from the VAD- and VAN-fed mice. (D) Comparison of the differences of the top 20 genera identified in the fecal samples using the Mann–Whitney U test. (E) Comparison of the differences of the top 20 genera identified in the fecal samples using Metastats analysis. F/B, the Firmicutes/Bacteroidetes ratio; VAD, vitamin A deficiency diet; VAN, vitamin A normal diet. n = 10 per group. ∗P < 0.05 and ∗∗P < 0.01.
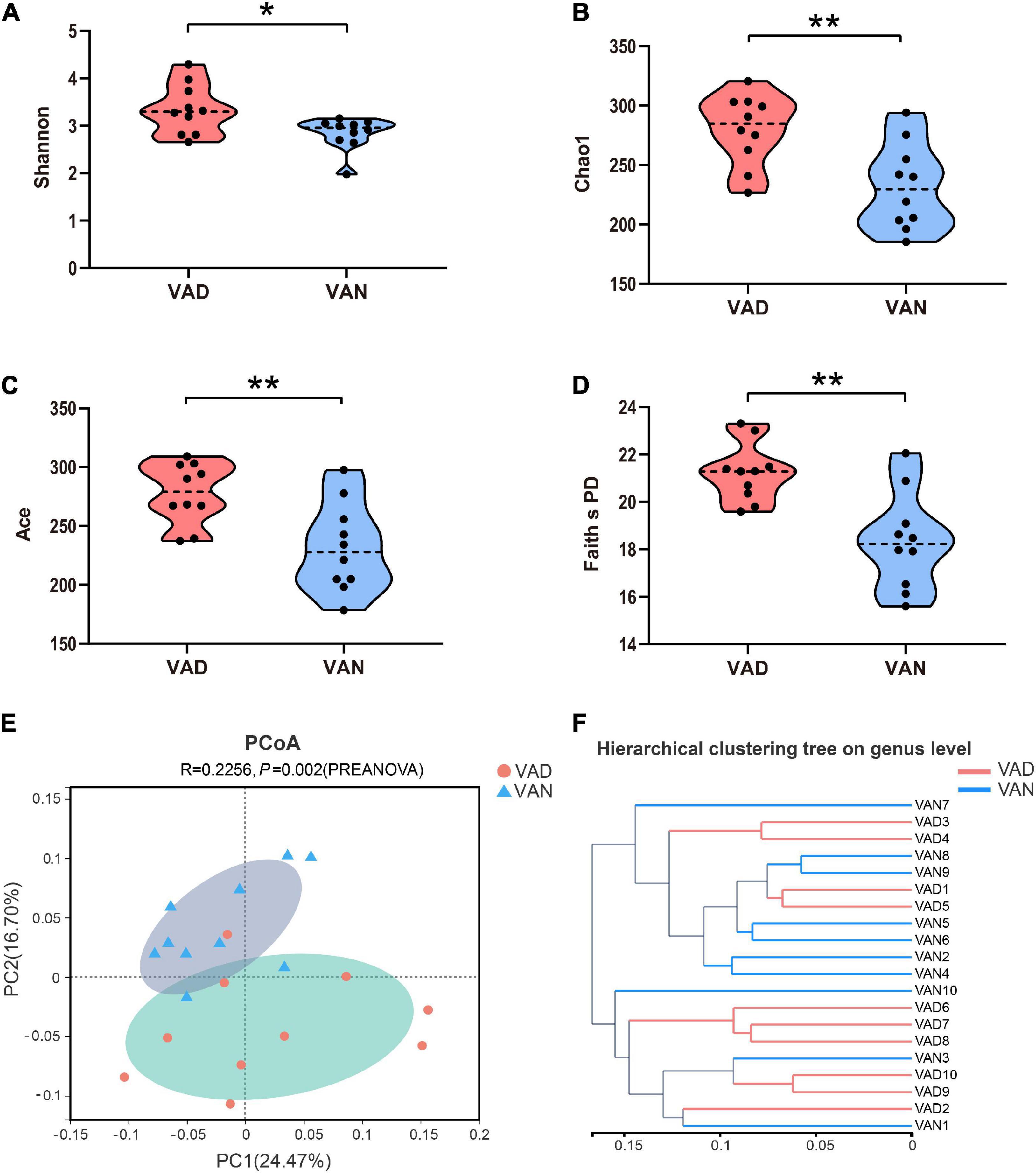
Figure 6. Alpha- and Beta-diversity indices of the fecal microbiota of the APP/PS1 transgenic mice after consumption of the VAD- or VAN-diet for 45 weeks. (A) Shannon index. (B) Chao1 index. (C) Ace index. (D) Faith’s phylogenetic diversity index. (E) Principal coordinates analysis (PCoA) plot based on the unweighted UniFrac distance. A red dot denotes samples from the VAD group, a cyan triangle denotes samples from the VAN group. (F) Hierarchical clustering of the individual samples based on the unweighted UniFrac distance. VAD, vitamin A deficiency diet; VAN, vitamin A normal diet. n = 10 per group. ∗P < 0.05 and ∗∗P < 0.01.
The LEfSe algorithm makes a supervised comparison of the microbiota to elucidate variant taxa at different taxonomic levels between the two groups. The LEfSe analysis demonstrated that, compared with the VAN group, the intestinal microbiota of the VAD group was different in 12 bacterial genera, seven bacterial families, and five bacterial orders (Figures 7A,B). Particularly, the potentially beneficial bacteria Lactobacillus in the VAD group was significantly lower than that in the VAN mice, while the potentially harmful bacteria Clostridia_UCG-014 in the VAD group was greatly higher than that in the VAN mice, which was consistent with the Mann–Whitney U test and Metastats analysis in Figures 5D,E. Moreover, the reduction in Lactobacillus and the increment in Clostridia_UCG-014 were further verified by RT-qPCR using their respective taxa-specific primers (P < 0.05; Figure 7C).
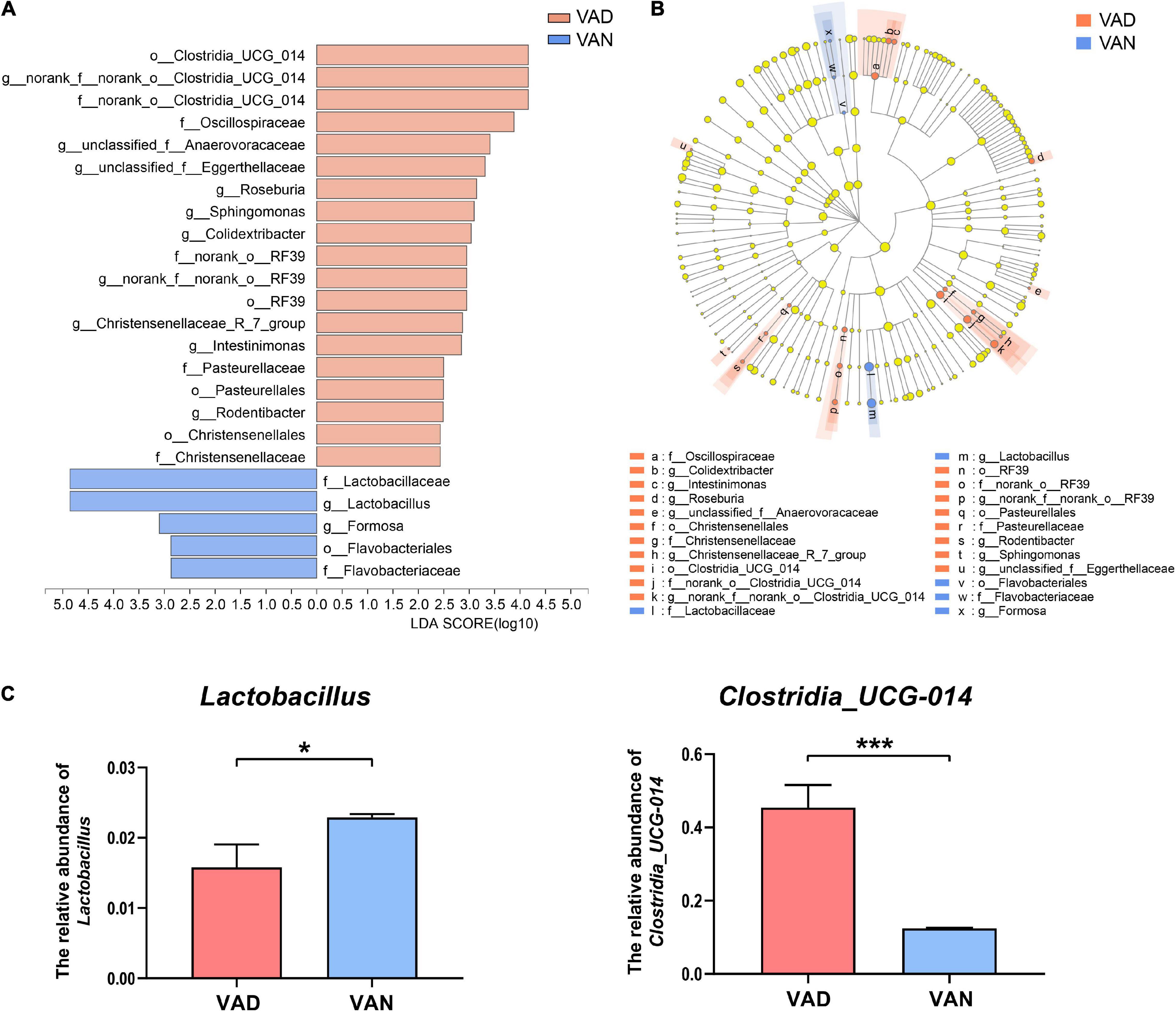
Figure 7. Linear discriminant analysis (LDA) effect size (LEfSe) analysis of the 16S rRNA gene sequencing data and the RT-qPCR verified abundance of Lactobacillus and Clostridia_UCG-014. (A) Histogram of the LDA scores computed for differentially abundant bacterial taxa between the VAD and VAN groups. Only taxa with a LDA score (log10) > 2 and P < 0.05 are listed. (B) Cladogram showing the impact of the diet on the taxonomic distribution of the bacteria. A total of 23 differentially abundant bacterial taxa were detected. Of those, seven bacterial taxa were significantly overrepresented in the VAN group (blue) and 15 bacterial taxa were overrepresented in the VAD group (red). (C) The RT-qPCR validation of the changes in the relative abundance of Lactobacillus and Clostridia_UCG-014 by using their specific primers. VAD, vitamin A deficiency diet; VAN, vitamin A normal diet. n = 10 per group. ∗P < 0.05, and ∗∗∗P < 0.001.
We used the PICRUSt analysis to predict the functional changes in the metagenome of the present experiment. In the KEGG pathway prediction results, quite a few metabolic pathways were altered in the VAD mice. Interestingly, the VAD mice significantly enriched the pathways related to aging, amino acid metabolism, biosynthesis of other secondary metabolites, digestive system, environmental adaptation, and immune system at KEGG level 2, while lowered those involved in the GABAergic synapse, glutamatergic synapse, retinol metabolism, and lipoic acid metabolism at KEGG level 3 (P < 0.05; Figures 8A,B and Supplementary Table 4). To identify whether VAD diet-driven intestinal microbiota changes are related to the VA concentration or not, we performed correlation analyses between these parameters. Spearman’s rank correlation indicated that both the liver retinol and serum retinol were positively correlated with the abundance of Lactobacillus (r = 0.51, P < 0.05; r = 0.70, P < 0.001; Figure 8C) and negatively correlated with the abundance of Clostridia_UCG-014 (r = − 0.66, P < 0.01; r = −0.49, P < 0.05; Figure 8C and Supplementary Table 5).
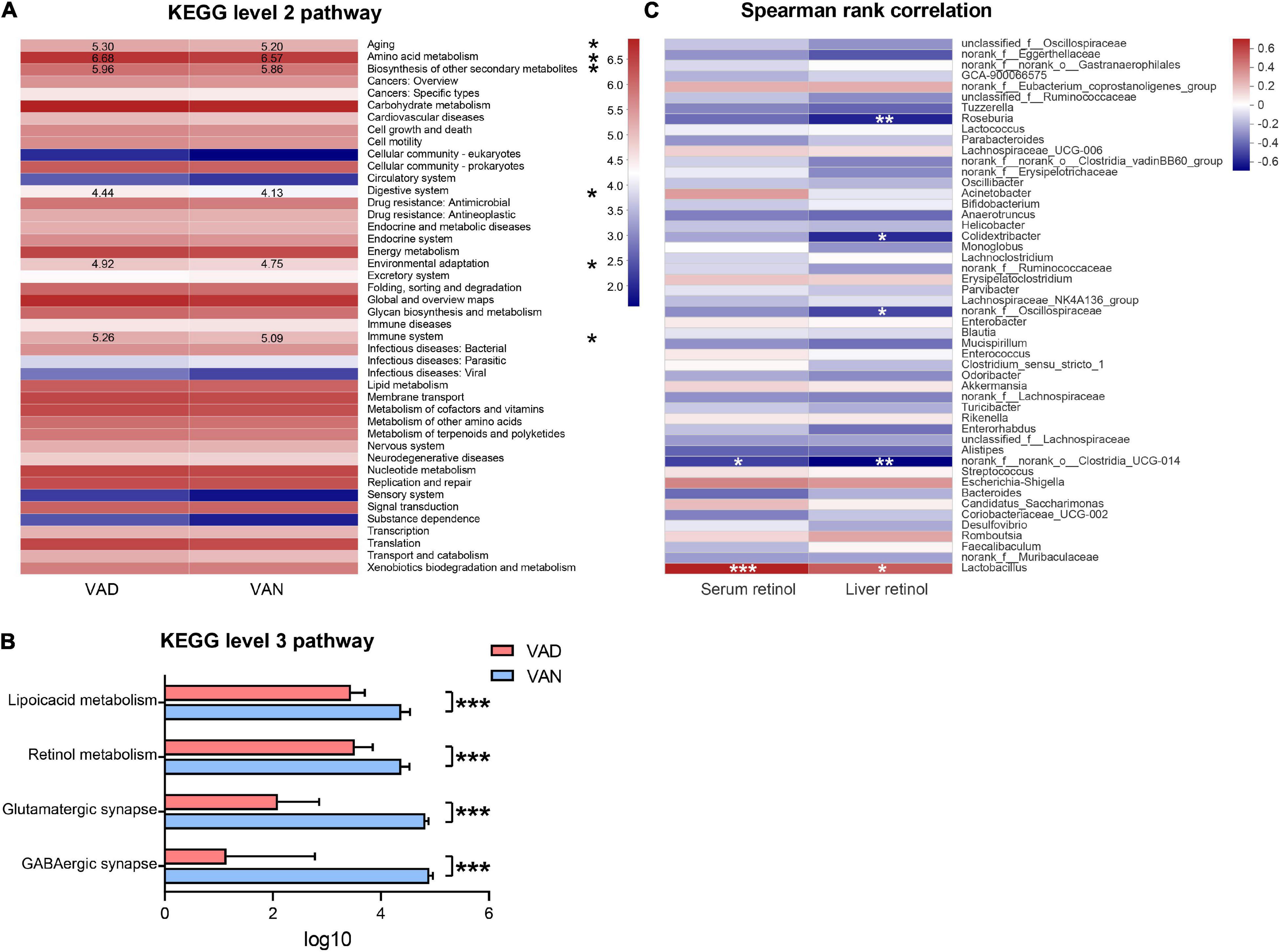
Figure 8. Predicted function of the gut microbiota and its correlation with the liver retinol and serum retinol. (A) Comparisons of the VAD- and VAN-diet impact on the predicted functions of the gut microbiota at the Kyoto Encyclopedia of Gene and Genomes (KEGG) level 2 by using the PICRUSt analysis. The number indicates the logarithmic value of enrichment to the pathways. (B) Significantly different functions of the gut microbiota at KEGG level 3 between the VAN and VAD group as predicted by the PICRUSt analysis (partial). (C) Correlation of the liver retinol and the serum retinol with the relative abundance of the top 50 bacterial genera in all mice. The red color represents a positive correlation. Blue color represents a negative correlation. VAD, vitamin A deficiency diet; VAN, vitamin A normal diet. n = 10 per group. ∗P < 0.05, ∗∗P < 0.01, and ∗∗∗P < 0.001.
Vitamin A Deficiency Reduced the Expression of Brain-Derived Neurotrophic Factor and γ-Aminobutyric Acid Receptors in the Brain of the Amyloid Precursor Protein/Presenilin 1 Mice
To assess the effect of long-term VAD on the brain BDNF expression and secretion, we measured the BDNF in the cortex and hippocampus with IHC, western blot (WB), and RT-qPCR, respectively. As shown in Figure 9, compared with the VAN mice, the BDNF gene and the protein expression were markedly downregulated in the VAD-diet APP/PS1 transgenic mice (P < 0.05).
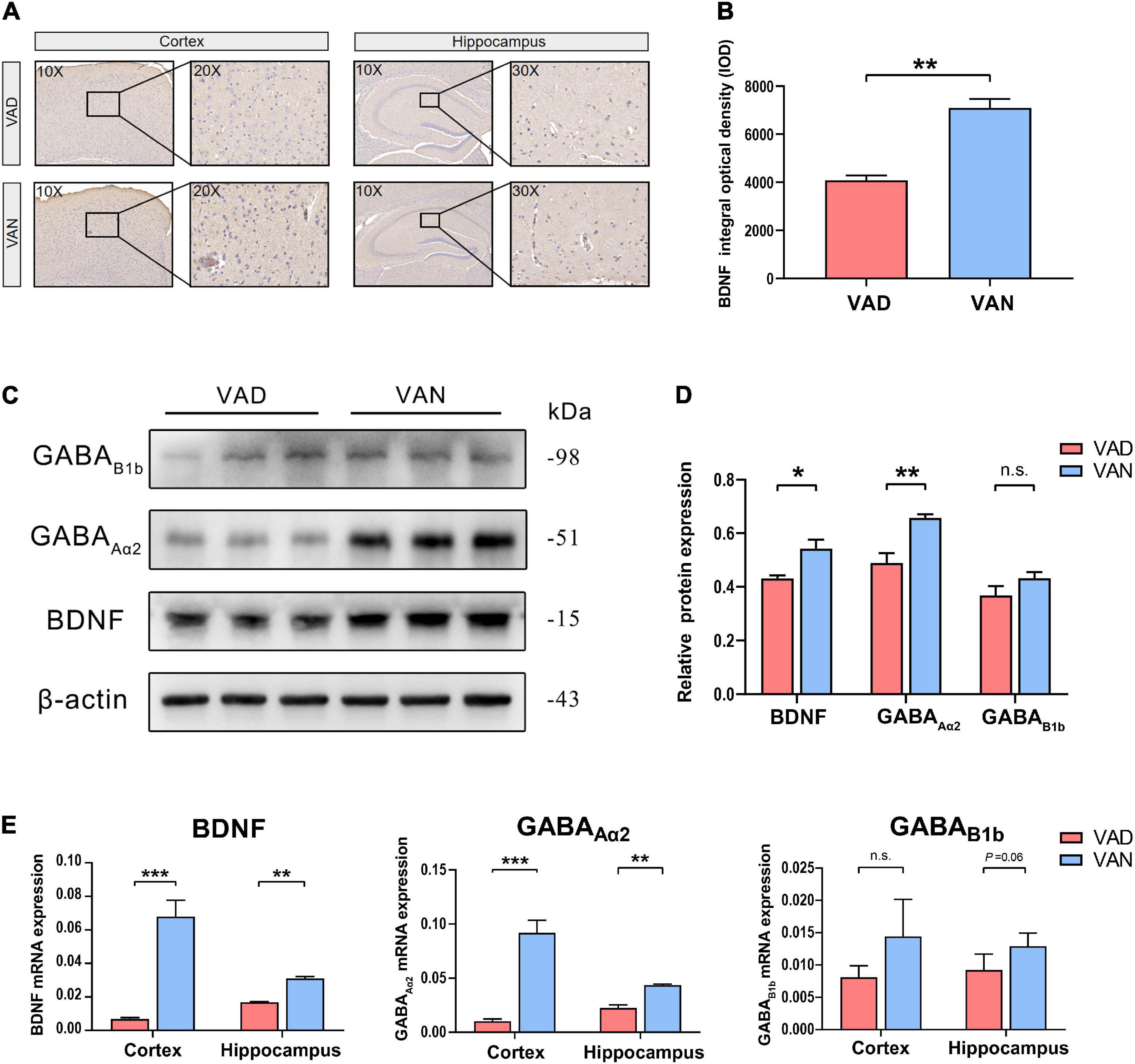
Figure 9. Consumption of the VAD diet for 45 weeks reduced the expression of brain-derived neurotrophic factor (BDNF) and the γ-aminobutyric acid (GABA) receptors in the cortex and hippocampus of the APP/PS1 transgenic mice. (A) The IHC images (10×, 20×, and 30×) showing the staining of the BDNF in the brain. (B) Quantification of the BDNF level in the brain of the mice in the IHC assay. (C) The WB images showing the expression of BDNF, GABAAα2, and GABAB1b in the cortex of the APP/PS1 mice fed with either VAD or VAN. (D) The BDNF, GABAAα2, and GABAB1b were quantitated according to the band density and normalized against the levels of β-actin. (E) RT-qPCR analysis of the expression of BDNF, GABAAα2, and GABAB1b genes in the cortex and hippocampus of the APP/PS1 mice. VAD, vitamin A deficiency diet; VAN, vitamin A normal diet. n = 3 per group. ∗P < 0.05, ∗∗P < 0.01, ∗∗∗P < 0.001, and n.s., non-significant.
To verify the results of functional metagenomic prediction about the GABAergic synapse, Western blotting and RT-qPCR were employed to determine the expression of the GABAAα2 and GABAB1b in the cortex and hippocampus of the APP/PS1 mice. As shown in Figures 9C,D, compared with the VAN group, the protein expression of GABAAα2 and GABAB1b were downregulated in the cortex of the VAD-diet-fed mice, but only the GABAAα2 was significantly changed. Moreover, the RT-qPCR results demonstrated that the transcription of GABAAα2 in the cortex and hippocampus of APP/PS1 mice in the VAD group was notably lower than those in the VAN group (P < 0.05; Figure 9E), which was consistent with the WB results.
Discussion
Vitamin A deficiency in older age is considered a modifiable risk factor for AD and other cognitive disorders (Woloszynowska-Fraser et al., 2020). However, the prevalence of VAD in developing countries, particularly among older persons with AD, demonstrates the importance of ensuring adequate VA intake for seniors. Diet-derived VA, which are mainly stored in the liver, is released in a homeostatically controlled way to provide a constant source of RA to cells of the body, including the brain cells (Pardridge et al., 1985). Retinol from the liver can also pass through the blood–brain barrier (BBB) by binding to the retinol-binding protein 1 on the choroid plexus and vascular walls of the brain (Woloszynowska-Fraser et al., 2020). After entering the BBB, retinol is sequentially transformed to retinal and RA by the retinol dehydrogenase and retinaldehyde dehydrogenase (RALDH), respectively. VAD in rodents impairs both long-term potentiation and long-term depression in the brain, and the degree of their disruption correlates with the level of VA deprivation (Misner et al., 2001). VA deficiency may further aggravate the decline in RA signaling (Zeng et al., 2017a) because such conditions lead to a decrease in the expression of RARs and RXRs. In addition, the studies in RARβ/RXRγ knockout mice have shown that lack of these receptors leads to decreased long-term declarative (conscious) memory and cognitive flexibility (Mingaud et al., 2008). This evidence is in line with our results that long-term VA deprivation resulted in a significantly decreased serum and liver retinol level and reduced the mRNA expression of the VA-related receptors (RARγ, RXRα, RXRβ, and RXRγ) and VA-related enzyme (RALDH1 and CYP26B1) in the cortex of the APP/PS1 mice.
Accumulating studies illustrate that Aβ deposition and Tau hyperphosphorylation are tightly related to the cognitive deficits and participate in the pathological mechanism of AD (Ramos-Rodriguez et al., 2013). Through IHC staining and Luminex immunoassay in the AD model mice, our research shows that the Aβ plaque load significantly increased after VA deprivation. Interestingly, we found that the Aβ levels were increased in the small intestine and colon of the mice after VA deprivation. In addition, previous studies have illustrated that enteric Aβ may translocate to the brain via axonal transportation in the vagal nerves (Sun et al., 2020). Although VAD did not affect the total Tau levels, it significantly increased the phosphorylated Tau protein, suggesting that VA deprivation facilitated Tau hyperphosphorylation in the cortex of mice. Additionally, the increased expression of BACE1 indicated that VAD might lead to Aβ plaque accumulation by dysregulating BACE1 expression, which is consistent with previous studies (Wang et al., 2015; Zeng et al., 2017b). In addition to the increase in Aβ, p-Tau, and BACE1, the decreased mRNA expression of ADAM10 suggested that VA deprivation may facilitate AD pathogenesis. These pathological manifestations are consistent with the behavioral results of the MWM test and SDPA test in our study, which are shown as aggravated learning and memory deficits in VAD mice.
Interestingly, our results demonstrate that VA deprivation decreased the mRNA expression of RALDH1 and CYP26B1, suggesting that VA deprivation may lead to a decrease in the synthesis and decomposition of RA in the brain. However, the effect of VAD on RA production and metabolism needs further study to verify.
Considering that there are amounts of undefined soluble amyloid protein secreted by symbionts, the intestinal microbiome may play an essential role in the pathogenesis of neurodegenerative diseases characterized by amyloidogenic features, such as AD (Zhao and Lukiw, 2015). The contribution of the gut microbiota to the formation and dissemination of amyloid in the elderly has become more critical because their gastrointestinal epithelial cells and the BBB have become more permeable to small molecules. Moreover, Shen et al. (2017) reported that the cognitive deficits of APP/PS1 mice are related to the specific gut microbiome states. Although individuals have different gut microbiota, it mainly contains members of four phyla (Firmicutes, Bacteroidetes, Actinobacteria, and Proteobacteria), among which Firmicutes and Bacteroidetes account for the largest proportion (Gerard, 2016). As a simple indicator of intestinal microbiota status, the Firmicutes/Bacteroidetes (F/B) ratio increased with the age before adulthood (10.9) and then decreased with age (0.6) (Doifode et al., 2021). Vogt et al. (2017) demonstrated that the F/B ratio decreased in the patients with AD. Our results also demonstrated that the F/B ratio decreased in the VAD-diet mice, implying that the intestinal homeostasis was disrupted. The Venn diagram manifested that VAD led to more new genera in the intestinal microbiota, but most were not probiotics. The α-diversity is primarily associated with two factors. One is the number of species: richness; the other is diversity, the evenness of individual distribution in the community (Koh, 2018). Interestingly, the α-diversity increased in the VAD mice, which indicated that either there were more genera in the intestines of the VAD mice or there are more similar distribution of abundances of genera in VAD mice. But that does not mean it is in a better intestinal microbial homeostasis, as it may be caused by the increase or appearance of harmful bacteria, such as the 19 unique bacterial genera in the VAD mice (Supplementary Table 3).
The β-diversity uses the evolutionary relationship and abundance information of the sequences of each sample to estimate the relative distance between the samples to reflect significant differences in the microbial communities between the groups (Soininen et al., 2007). The PCoA plot indicated that the composition, species, and differentiation of gut microbiota altered significantly after long-term VAD.
The Mann–Whitney U test, LEfSe analysis, and RT-PCR validation showed that the genus Lactobacillus decreased significantly after long-term VAD (P < 0.05). In addition, the Spearman’s rank correlation analysis showed that the liver and serum retinol level was positively associated with the abundance of Lactobacillus (r = 0.51, P < 0.05; r = 0.70, P < 0.001). Lactobacillus can enhance the intestinal mucosal barrier function by increasing the permeability resistance of HT-29 cells and Caco-2 cells, along with the increase of the phosphorylation level of tight junction proteins (Resta-Lenert and Barrett, 2003). It has been demonstrated that Lactobacillus can induce the expression of mucin in the colonic epithelial cells, which forms a protective barrier between the body and the external environment (Caballero-Franco et al., 2007). Moreover, previous studies have demonstrated that Lactobacillus could stimulate the gut-brain axis and upregulate the expression of BDNF (Ranuh et al., 2019). The study by Buchman et al. (2016) highlights the brain BDNF as a potential substantial contributor to slowing cognitive deterioration in the elderly, particularly in the context of advancing AD neuropathology. Accumulating studies have shown that BDNF levels decreased in both the brain and serum of the patients with AD (Carlino et al., 2013). In addition, Beeri and Sonnen (2016) argued that the brain BDNF expression could be regarded as a biomarker for the cognitive improvement against the AD pathological progression. In neurons, BDNF mainly binds to the tyrosine kinase receptor B (TrkB) to activate the intracellular signaling pathways, such as the PI3K-Akt signaling pathway, Ras/Raf-MEK-ERK signaling pathway, and PLC-PKC signaling pathway, thereby improving the viability and regeneration of the neurons (Hang et al., 2021) and increasing the synaptic plasticity and the learning and memory function of the brain (Zhang et al., 2012). On the contrary, the BDNF-devoid neurons often manifest neurofibrillary tangles, a characteristic of AD, which was absent in the densely BDNF-labeled neurons (Murer et al., 1999). Furthermore, Nigam et al. (2017) demonstrated that BDNF reduces the Aβ production by enhancing α-secretase processing of the APP. Therefore, BDNF may be an important mediator of the aggravated cognitive deficits after long-term VAD.
Meanwhile, we observed that the abundance of Clostridia_UCG-014 increased significantly in the VAD mice. Similar to the results of Lactobacillus, this difference was also verified by Mann–Whitney U-test, Metastats analysis, LEfSe analysis, Spearman’s rank correlation analysis, and RT-qPCR. Clostridia_UCG-014 is an obligately anaerobic bacteria, which is common in soil and the mammalian intestine (Suen et al., 2007). Previous studies have shown that the increased abundance of Clostridia_UCG-014 is related to elevated fasting blood glucose (Zhao et al., 2021) and colitis (Liu et al., 2021). Clostridia-related diseases mainly occur when the spores enter the body, colonize the host, germinate and generate toxins (Mallozzi et al., 2010). Thus, the increase in Clostridia_UCG-014 after long-term VAD may cause more serious damage to the health of the patients with AD.
Functional interpretation of the fecal metagenomes by PICRUSt revealed that the pathways, such as amino acid metabolism, GABAergic synapse, glutamatergic synapse, retinol metabolism, and lipoic acid metabolism displayed remarkable alterations after long-term VAD. The decrease in the retinol metabolism pathway is consistent with the decrease of the retinol level in the liver and the serum and the decrease of VA-related receptors in the brain. An AD microbiome cohort (Liu et al., 2019) revealed that decreased amino acid metabolism was found in the patients with cognitive impairment. In addition, the function and structure of glutamatergic synapses are affected by Aβ in the early stage of AD (Marcello et al., 2012). Holmquist et al. (2007) found that lipoic acid could be regarded as a neuroprotective and anti-inflammatory treatment for patients with AD. Besides, the GABAergic synapses play a neuroprotective role in the process of Aβ-induced neurotoxicity by releasing GABA (Cisternas et al., 2020). More interestingly, Bravo et al. (2011) highlighted that Lactobacillus could upregulate the GABA receptors in the hippocampus and cortex through the vagus nerve, which is consistent with our RT-qPCR and WB results about the GABAAα2 and GABAB1b receptors. Moreover, BDNF can promote the synaptic formation and maturation of the GABAergic synapses (Huang et al., 1999), and the excitatory action of GABA has been shown to activate the BDNF expression (Fukuchi et al., 2014). Therefore, we hypothesize that intestinal microbiota, especially Lactobacillus, may play an important role in the reduction of the BDNF and GABA receptors in the brain after long-term VAD in AD individuals, thereby increasing the production of Aβ and Tau hyperphosphorylation, and ultimately affecting the development of the cognitive dysfunction. Although the above hypothesis can be proposed from the results of our study, we do not know to what extent the AD pathological and behavioral impair can be attributed to the reduced BDNF and GABA receptors by the VAD-induced microbiota disruption. The present study is only a preliminary observation, but we provide a new perspective on the gut-brain axis that VAD exacerbates the gut microbiota dysbiosis and cognitive deficits, and we will conduct further experiments to verify this hypothesis in the future.
Conclusion
In summary, the findings of the present study provided valuable facts that long-term VAD exacerbates the gut microbiota dysbiosis and cognitive deficits, highlighting the importance of monitoring the VA levels during senescence and necessitating the timely supplementation of VA in person who is at a higher risk of developing AD. Additionally, we posit that VAD may reduce the expression of GABA receptors and downregulate BDNF in the brain by disturbing intestinal microbiota (especially Lactobacillus), thus leading to histological and cognitive impairment in AD. Further studies are warranted to verify the hypothesis and elucidate the mechanisms by which VAD exerts its effect on microecology.
Data Availability Statement
The datasets presented in this study can be found in online repositories. The names of the repository/repositories and accession number(s) can be found in the article/Supplementary Material.
Ethics Statement
The animal study was reviewed and approved by the Ethics Committee of Capital Medical University (AEEI-2018-176).
Author Contributions
P-GL and CY had primary responsibility for the final content and designed the research. B-WC and K-WZ conducted the experiment. B-WC analyzed the data and wrote the manuscript. S-JC revised the manuscript. All authors contributed to the article and approved the submitted version.
Funding
This study was supported by grants from the National Natural Science Foundation of China (Nos. 81573128 and 81703216).
Conflict of Interest
The authors declare that the research was conducted in the absence of any commercial or financial relationships that could be construed as a potential conflict of interest.
Publisher’s Note
All claims expressed in this article are solely those of the authors and do not necessarily represent those of their affiliated organizations, or those of the publisher, the editors and the reviewers. Any product that may be evaluated in this article, or claim that may be made by its manufacturer, is not guaranteed or endorsed by the publisher.
Supplementary Material
The Supplementary Material for this article can be found online at: https://www.frontiersin.org/articles/10.3389/fnagi.2021.753351/full#supplementary-material
References
Amit-Romach, E., Uni, Z., Cheled, S., Berkovich, Z., and Reifen, R. (2009). Bacterial population and innate immunity-related genes in rat gastrointestinal tract are altered by vitamin A-deficient diet. J. Nutr. Biochem. 20, 70–77. doi: 10.1016/j.jnutbio.2008.01.002
Beeri, M. S., and Sonnen, J. (2016). Brain BDNF expression as a biomarker for cognitive reserve against Alzheimer disease progression. Neurology 86, 702–703. doi: 10.1212/WNL.0000000000002389
Bercik, P., Denou, E., Collins, J., Jackson, W., Lu, J., Jury, J., et al. (2011). The intestinal microbiota affect central levels of brain-derived neurotropic factor and behavior in mice. Gastroenterology 141, 599–609, 609.e1-3. doi: 10.1053/j.gastro.2011.04.052
Bolyen, E., Rideout, J. R., Dillon, M. R., Bokulich, N. A., Abnet, C. C., Al-Ghalith, G. A., et al. (2019). Reproducible, interactive, scalable and extensible microbiome data science using QIIME 2. Nat. Biotechnol. 37, 852–857. doi: 10.1038/s41587-019-0209-209
Bravo, J. A., Forsythe, P., Chew, M. V., Escaravage, E., Savignac, H. M., Dinan, T. G., et al. (2011). Ingestion of Lactobacillus strain regulates emotional behavior and central GABA receptor expression in a mouse via the vagus nerve. Proc. Natl. Acad. Sci. U S A. 108, 16050–16055. doi: 10.1073/pnas.1102999108
Buchman, A. S., Yu, L., Boyle, P. A., Schneider, J. A., De Jager, P. L., and Bennett, D. A. (2016). Higher brain BDNF gene expression is associated with slower cognitive decline in older adults. Neurology 86, 735–741. doi: 10.1212/WNL.0000000000002387
Caballero-Franco, C., Keller, K., De Simone, C., and Chadee, K. (2007). The VSL#3 probiotic formula induces mucin gene expression and secretion in colonic epithelial cells. Am. J. Physiol. Gastrointest. Liver Physiol. 292, G315–G322. doi: 10.1152/ajpgi.00265.2006
Caporaso, J. G., Lauber, C. L., Walters, W. A., Berg-Lyons, D., Lozupone, C. A., Turnbaugh, P. J., et al. (2011). Global patterns of 16S rRNA diversity at a depth of millions of sequences per sample. Proc. Natl. Acad. Sci. U S A. 108, (Suppl. 1), 4516–4522. doi: 10.1073/pnas.1000080107
Carlino, D., De Vanna, M., and Tongiorgi, E. (2013). Is altered BDNF biosynthesis a general feature in patients with cognitive dysfunctions? Neuroscientist 19, 345–353. doi: 10.1177/1073858412469444
Chen, B., Liu, S., Feng, D., Xiao, L., Yang, T., Li, T., et al. (2020). Vitamin A deficiency in the early-life periods alters a diversity of the colonic mucosal microbiota in rats. Front. Nutr. 7:580780. doi: 10.3389/fnut.2020.580780
Cisternas, P., Taylor, X., Perkins, A., Maldonado, O., Allman, E., Cordova, R., et al. (2020). Vascular amyloid accumulation alters the gabaergic synapse and induces hyperactivity in a model of cerebral amyloid angiopathy. Aging Cell 19, e13233. doi: 10.1111/acel.13233
Doifode, T., Giridharan, V. V., Generoso, J. S., Bhatti, G., Collodel, A., Schulz, P. E., et al. (2021). The impact of the microbiota-gut-brain axis on Alzheimer’s disease pathophysiology. Pharmacol. Res. 164:105314. doi: 10.1016/j.phrs.2020.105314
Edgar, R. C. (2013). UPARSE: highly accurate OTU sequences from microbial amplicon reads. Nat. Methods 10, 996–998. doi: 10.1038/nmeth.2604
Etchamendy, N., Enderlin, V., Marighetto, A., Pallet, V., Higueret, P., and Jaffard, R. (2003). Vitamin A deficiency and relational memory deficit in adult mice: relationships with changes in brain retinoid signalling. Behav. Brain Res. 145, 37–49. doi: 10.1016/s0166-4328(03)00099-98
Fukuchi, M., Kirikoshi, Y., Mori, A., Eda, R., Ihara, D., Takasaki, I., et al. (2014). Excitatory GABA induces BDNF transcription via CRTC1 and phosphorylated CREB-related pathways in immature cortical cells. J. Neurochem. 131, 134–146. doi: 10.1111/jnc.12801
Gerard, P. (2016). Gut microbiota and obesity. Cell Mol. Life. Sci. 73, 147–162. doi: 10.1007/s00018-015-2061-2065
Hang, P. Z., Zhu, H., Li, P. F., Liu, J., Ge, F. Q., Zhao, J., et al. (2021). The emerging role of BDNF/TrkB signaling in cardiovascular diseases. Life (Basel) 11:70. doi: 10.3390/life11010070
Holmquist, L., Stuchbury, G., Berbaum, K., Muscat, S., Young, S., Hager, K., et al. (2007). Lipoic acid as a novel treatment for Alzheimer’s disease and related dementias. Pharmacol. Ther. 113, 154–164. doi: 10.1016/j.pharmthera.2006.07.001
Hsiao, Y. H., Chang, C. H., and Gean, P. W. (2018). Impact of social relationships on Alzheimer’s memory impairment: mechanistic studies. J. Biomed. Sci. 25:3. doi: 10.1186/s12929-018-0404-x
Huang, Z. J., Kirkwood, A., Pizzorusso, T., Porciatti, V., Morales, B., Bear, M. F., et al. (1999). BDNF regulates the maturation of inhibition and the critical period of plasticity in mouse visual cortex. Cell 98, 739–755. doi: 10.1016/s0092-8674(00)81509-81503
Joe, E., and Ringman, J. M. (2019). Cognitive symptoms of Alzheimer’s disease: clinical management and prevention. BMJ 367:l6217. doi: 10.1136/bmj.l6217
Kanehisa, M., Goto, S., Sato, Y., Furumichi, M., and Tanabe, M. (2012). KEGG for integration and interpretation of large-scale molecular data sets. Nucleic Acids Res. 40, D109–D114. doi: 10.1093/nar/gkr988
Koh, H. (2018). An adaptive microbiome alpha-diversity-based association analysis method. Sci. Rep. 8:18026. doi: 10.1038/s41598-018-36355-36357
Krezel, W., Kastner, P., and Chambon, P. (1999). Differential expression of retinoid receptors in the adult mouse central nervous system. Neuroscience 89, 1291–1300. doi: 10.1016/s0306-4522(98)00342-x
Leng, F., and Edison, P. (2020). Neuroinflammation and microglial activation in Alzheimer disease: where do we go from here? Nat. Rev. Neurol. 17, 157–172. doi: 10.1038/s41582-020-00435-y
Liu, L., Wu, Y., Wang, B., Jiang, Y., Lin, L., Li, X., et al. (2021). DA-DRD5 signaling controls colitis by regulating colonic M1/M2 macrophage polarization. Cell Death Dis. 12:500. doi: 10.1038/s41419-021-03778-3776
Liu, P., Wu, L., Peng, G., Han, Y., Tang, R., Ge, J., et al. (2019). Altered microbiomes distinguish Alzheimer’s disease from amnestic mild cognitive impairment and health in a Chinese cohort. Brain Behav. Immun. 80, 633–643. doi: 10.1016/j.bbi.2019.05.008
Lopes, D. S. S., Vellas, B., Elemans, S., Luchsinger, J., Kamphuis, P., Yaffe, K., et al. (2014). Plasma nutrient status of patients with Alzheimer’s disease: systematic review and meta-analysis. Alzheimers Dement 10, 485–502. doi: 10.1016/j.jalz.2013.05.1771
Lozupone, C., and Knight, R. (2005). UniFrac: a new phylogenetic method for comparing microbial communities. Appl. Environ. Microbiol. 71, 8228–8235. doi: 10.1128/AEM.71.12.8228-8235.2005
Maden, M. (2007). Retinoic acid in the development, regeneration and maintenance of the nervous system. Nat. Rev. Neurosci. 8, 755–765. doi: 10.1038/nrn2212
Malaspina, A., and Michael-Titus, A. T. (2008). Is the modulation of retinoid and retinoid-associated signaling a future therapeutic strategy in neurological trauma and neurodegeneration? J. Neurochem. 104, 584–595. doi: 10.1111/j.1471-4159.2007.05071.x
Mallozzi, M., Viswanathan, V. K., and Vedantam, G. (2010). Spore-forming Bacilli and Clostridia in human disease. Future Microbiol. 5, 1109–1123. doi: 10.2217/fmb.10.60
Marcello, E., Epis, R., Saraceno, C., and Di Luca, M. (2012). Synaptic dysfunction in Alzheimer’s disease. Adv. Exp. Med. Biol. 970, 573–601. doi: 10.1007/978-3-7091-0932-8_25
McDonald, D., Price, M. N., Goodrich, J., Nawrocki, E. P., DeSantis, T. Z., Probst, A., et al. (2012). An improved Greengenes taxonomy with explicit ranks for ecological and evolutionary analyses of bacteria and archaea. ISME J. 6, 610–618. doi: 10.1038/ismej.2011.139
Mingaud, F., Mormede, C., Etchamendy, N., Mons, N., Niedergang, B., Wietrzych, M., et al. (2008). Retinoid hyposignaling contributes to aging-related decline in hippocampal function in short-term/working memory organization and long-term declarative memory encoding in mice. J. Neurosci. 28, 279–291. doi: 10.1523/JNEUROSCI.4065-07.2008
Misner, D. L., Jacobs, S., Shimizu, Y., de Urquiza, A. M., Solomin, L., Perlmann, T., et al. (2001). Vitamin A deprivation results in reversible loss of hippocampal long-term synaptic plasticity. Proc. Natl. Acad. Sci. U S A. 98, 11714–11719. doi: 10.1073/pnas.191369798
Murer, M. G., Boissiere, F., Yan, Q., Hunot, S., Villares, J., Faucheux, B., et al. (1999). An immunohistochemical study of the distribution of brain-derived neurotrophic factor in the adult human brain, with particular reference to Alzheimer’s disease. Neuroscience 88, 1015–1032. doi: 10.1016/s0306-4522(98)00219-x
Nigam, S. M., Xu, S., Kritikou, J. S., Marosi, K., Brodin, L., and Mattson, M. P. (2017). Exercise and BDNF reduce Abeta production by enhancing alpha-secretase processing of APP. J. Neurochem. 142, 286–296. doi: 10.1111/jnc.14034
Nomoto, M., Takeda, Y., Uchida, S., Mitsuda, K., Enomoto, H., Saito, K., et al. (2012). Dysfunction of the RAR/RXR signaling pathway in the forebrain impairs hippocampal memory and synaptic plasticity. Mol. Brain 5:8. doi: 10.1186/1756-6606-5-8
Pardridge, W. M., Sakiyama, R., and Coty, W. A. (1985). Restricted transport of vitamin D and A derivatives through the rat blood-brain barrier. J. Neurochem. 44, 1138–1141. doi: 10.1111/j.1471-4159.1985.tb08735.x
Petra, A. I., Panagiotidou, S., Hatziagelaki, E., Stewart, J. M., Conti, P., and Theoharides, T. C. (2015). Gut-Microbiota-Brain axis and its effect on neuropsychiatric disorders with suspected immune dysregulation. Clin. Ther. 37, 984–995. doi: 10.1016/j.clinthera.2015.04.002
Ramos-Rodriguez, J. J., Pacheco-Herrero, M., Thyssen, D., Murillo-Carretero, M. I., Berrocoso, E., Spires-Jones, T. L., et al. (2013). Rapid beta-amyloid deposition and cognitive impairment after cholinergic denervation in APP/PS1 mice. J. Neuropathol. Exp. Neurol. 72, 272–285. doi: 10.1097/NEN.0b013e318288a8dd
Ranuh, R., Athiyyah, A. F., Darma, A., Risky, V. P., Riawan, W., Surono, I. S., et al. (2019). Effect of the probiotic Lactobacillus plantarum IS-10506 on BDNF and 5HT stimulation: role of intestinal microbiota on the gut-brain axis. Iran J. Microbiol. 11, 145–150.
Reitz, C., Brayne, C., and Mayeux, R. (2011). Epidemiology of Alzheimer disease. Nat. Rev. Neurol. 7, 137–152. doi: 10.1038/nrneurol.2011.2
Resta-Lenert, S., and Barrett, K. E. (2003). Live probiotics protect intestinal epithelial cells from the effects of infection with enteroinvasive Escherichia coli (EIEC). Gut 52, 988–997. doi: 10.1136/gut.52.7.988
Scott, K. P., Gratz, S. W., Sheridan, P. O., Flint, H. J., and Duncan, S. H. (2013). The influence of diet on the gut microbiota. Pharmacol. Res. 69, 52–60. doi: 10.1016/j.phrs.2012.10.020
Shen, L., Liu, L., and Ji, H. F. (2017). Alzheimer’s disease histological and behavioral manifestations in transgenic mice correlate with specific gut microbiome state. J. Alzheimers Dis. 56, 385–390. doi: 10.3233/JAD-160884
Sherwin, J. C., Reacher, M. H., Dean, W. H., and Ngondi, J. (2012). Epidemiology of vitamin A deficiency and xerophthalmia in at-risk populations. Trans. R. Soc. Trop. Med. Hyg. 106, 205–214. doi: 10.1016/j.trstmh.2012.01.004
Shudo, K., Fukasawa, H., Nakagomi, M., and Yamagata, N. (2009). Towards retinoid therapy for Alzheimer’s disease. Curr. Alzheimer Res. 6, 302–311. doi: 10.2174/156720509788486581
Soininen, J., Lennon, J. J., and Hillebrand, H. (2007). A multivariate analysis of beta diversity across organisms and environments. Ecology 88, 2830–2838. doi: 10.1890/06-1730.1
Suen, G., Goldman, B. S., and Welch, R. D. (2007). Predicting prokaryotic ecological niches using genome sequence analysis. PLoS One 2:e743. doi: 10.1371/journal.pone.0000743
Sun, Y., Sommerville, N. R., Liu, J., Ngan, M. P., Poon, D., Ponomarev, E. D., et al. (2020). Intra-gastrointestinal amyloid-beta1-42 oligomers perturb enteric function and induce Alzheimer’s disease pathology. J. Physiol. 598, 4209–4223. doi: 10.1113/JP279919
Vazquez-Baeza, Y., Pirrung, M., Gonzalez, A., and Knight, R. (2013). EMPeror: a tool for visualizing high-throughput microbial community data. Gigascience 2:16. doi: 10.1186/2047-217X-2-16
Vogt, N. M., Kerby, R. L., Dill-McFarland, K. A., Harding, S. J., Merluzzi, A. P., Johnson, S. C., et al. (2017). Gut microbiome alterations in Alzheimer’s disease. Sci. Rep. 7:13537. doi: 10.1038/s41598-017-13601-y
Wang, R., Chen, S., Liu, Y., Diao, S., Xue, Y., You, X., et al. (2015). All-trans-retinoic acid reduces BACE1 expression under inflammatory conditions via modulation of nuclear factor kappaB (NFkappaB) signaling. J. Biol. Chem. 290, 22532–22542. doi: 10.1074/jbc.M115.662908
WHO (2009). Global Prevalence of Vitamin A Deficiency in Populations at Risk 1995-2005. WHO Global Database on Vitamin A Deficiency. Geneva: World Health Organization.
Woloszynowska-Fraser, M. U., Kouchmeshky, A., and McCaffery, P. (2020). Vitamin A and retinoic acid in cognition and cognitive disease. Annu. Rev. Nutr. 40, 247–272. doi: 10.1146/annurev-nutr-122319-134227
Zeng, J., Chen, L., Wang, Z., Chen, Q., Fan, Z., Jiang, H., et al. (2017a). Marginal vitamin A deficiency facilitates Alzheimer’s pathogenesis. Acta Neuropathol. 133, 967–982. doi: 10.1007/s00401-017-1669-y
Zeng, J., Li, T., Gong, M., Jiang, W., Yang, T., Chen, J., et al. (2017b). Marginal Vitamin A deficiency exacerbates memory deficits following Abeta1-42 injection in rats. Curr. Alzheimer Res. 14, 562–570. doi: 10.2174/1567205013666161223162110
Zhang, F., Kang, Z., Li, W., Xiao, Z., and Zhou, X. (2012). Roles of brain-derived neurotrophic factor/tropomyosin-related kinase B (BDNF/TrkB) signalling in Alzheimer’s disease. J. Clin. Neurosci. 19, 946–949. doi: 10.1016/j.jocn.2011.12.022
Zhao, J. D., Li, Y., Sun, M., Yu, C. J., Li, J. Y., Wang, S. H., et al. (2021). Effect of berberine on hyperglycaemia and gut microbiota composition in type 2 diabetic Goto-Kakizaki rats. World J. Gastroenterol. 27, 708–724. doi: 10.3748/wjg.v27.i8.708
Zhao, Y., Dua, P., and Lukiw, W. J. (2015). Microbial sources of amyloid and relevance to amyloidogenesis and Alzheimer’s Disease (AD). J. Alzheimers Dis. Parkinsonism 5:177. doi: 10.4172/2161-0460.1000177
Keywords: vitamin A deficiency, Alzheimer’s disease, gut microbiota dysbiosis, cognitive deficits, APP/PS1 transgenic mice
Citation: Chen B-W, Zhang K-W, Chen S-J, Yang C and Li P-G (2021) Vitamin A Deficiency Exacerbates Gut Microbiota Dysbiosis and Cognitive Deficits in Amyloid Precursor Protein/Presenilin 1 Transgenic Mice. Front. Aging Neurosci. 13:753351. doi: 10.3389/fnagi.2021.753351
Received: 04 August 2021; Accepted: 04 October 2021;
Published: 01 November 2021.
Edited by:
Boon-Seng Wong, Singapore Institute of Technology, SingaporeReviewed by:
Peter McCaffery, University of Aberdeen, United KingdomJung A. Woo, Case Western Reserve University, United States
Copyright © 2021 Chen, Zhang, Chen, Yang and Li. This is an open-access article distributed under the terms of the Creative Commons Attribution License (CC BY). The use, distribution or reproduction in other forums is permitted, provided the original author(s) and the copyright owner(s) are credited and that the original publication in this journal is cited, in accordance with accepted academic practice. No use, distribution or reproduction is permitted which does not comply with these terms.
*Correspondence: Peng-Gao Li, cGVuZ2dhb0BjY211LmVkdS5jbg==; Chun Yang, bGlueWluZ3lhbmdjaHVuQDE2My5jb20=