- 1Dongfang Hospital, Beijing University of Chinese Medicine, Beijing, China
- 2Key Laboratory of Health-Cultivation, Ministry of Education of the People’s Republic of China, Beijing University of Chinese Medicine, Beijing, China
- 3Department of Tibetan Medicine, University of Tibetan Medicine, Lhasa, China
Background: Diabetes cognitive impairment (DCI) is a common diabetic central nervous system disorder that severely affects the quality of life of patients. Qishiwei Zhenzhu Pills (Ranasampel) is a valuable Tibetan medicine formula with the ability to improve cerebral blood vessels, protect nerves and improve learning and memory, which has also been widely verified in clinical and basic research. Currently, the prevention and treatment of DCI are still in the exploratory research stage, and the use of Ranasampel will provide new ideas and insights for its treatment.
Objective: This study is to explore the absorbed components in serum derived from Ranasampel using serum pharmacochemistry, then identify the potential mechanism of Ranasampel for the treatment of DCI through bioinformatics and microarray data validation.
Methods: The UPLC-Q-Exactive MS/MS-based serum pharmacochemistry method was conducted to identify the main active components in serum containing Ranasampel. Then, these components were used to predict the possible biological targets of Ranasampel and explore the potential targets in treating DCI by overlapping with differentially expressed genes (DEGs) screened from Gene Expression Omnibus datasets. Afterward, the protein–protein interaction network, enrichment analyses, hub gene identification, and co-expression analysis were used to study the potential mechanism of Ranasampel. Particularly, the hub genes and co-expression transcription factors were further validated using hippocampal expression profiles of db/db mice treated with Ranasampel, while the Morris water-maze test and H&E staining were used to assess the spatial learning and memory behaviors and histopathological changes.
Results: Totally, 40 compounds derived from Ranasampel had been identified by serum sample analysis, and 477 genes related to these identified compounds in Ranasampel, 110 overlapping genes were collected by the intersection of Ranasampel target genes and DEGs. Further comprehensive analysis and verification emphasized that the mechanism of Ranasampel treatment of DCI may be related to the improvement of learning and memory function as well as insulin resistance, hyperglycemia-induced neuronal damage, and neuroinflammation.
Conclusion: This study provided useful strategies to explore the potential material basis for compound prescriptions such as Ranasampel. These hub genes and common pathways also provided new ideas for further study of therapeutic targets of DCI and the pharmacological mechanism of Ranasampel.
1 Introduction
Diabetes has become an increasingly serious global problem, the global prevalence of which has continued to grow in recent years, reaching 463 million people aged 20−79 in 2019, and expecting to reach 629 million by 2045 (Saeedi et al., 2019). As we all know, the most terrible thing about diabetes is its complications. The complications caused by diabetes are not only difficult to cure but also seriously affect the quality of life of patients and add a heavy burden to families and the social economy (Demir et al., 2021). Neurological impairment is a common chronic complication of diabetes, and the combination of type 2 diabetes and cognitive dysfunction is also very common in clinical practice (Kullmann et al., 2020). Recent epidemiological studies have shown that diabetes significantly increases the risk of Alzheimer’s disease (AD), suggesting a possible correlation between diabetes and the pathogenesis of AD (Baglietto-Vargas et al., 2016). At the same time, many molecular mechanisms and pathological manifestations of diabetes may overlap with factors leading to AD, and common diabetic symptoms such as hyperglycemia, altered insulin signaling, advanced glycosylation, and chronic low-grade inflammation are common underlying mechanisms of vascular and neurological degeneration, as also found in modern studies (Pugazhenthi et al., 2017; Srikanth et al., 2020). In addition to increasing the risk of cerebrovascular disease and stroke by severely affecting cardiovascular health, hyperglycemia also increases the risk of neurodegeneration through a mechanism related to amyloid (Moran et al., 2015; Takenoshita et al., 2018). Furthermore, central neural circuits respond to insulin-related signals that not only enhance systemic insulin sensitivity, regulate peripheral metabolism, and inhibit endogenous gluconeogenesis, but also modulate the cognitive function and appetite to suppress food intake, yet these response mechanisms are impaired in individuals who develop insulin resistance, suggesting that disturbances in insulin signaling represent a potential link between metabolism and cognitive function (Cai et al., 2018; Manaserh et al., 2020).
Traditional Tibetan medicine has a long history of over 2000 years, which is the second-largest traditional medicine system in China and one of the oldest known medical systems in the world (Dakpa, 2014). It combines theories from early Chinese medicine, Indian medicine, and Arabic medicine, then gradually developed into a unique theory and medical system (Li Q. et al., 2018). Qishiwei Zhenzhu Pills (Ranasampel), first recorded in the Four Medical Tantras (Luo et al., 2015), has the therapeutic effect of calming, tranquilizing, restoring consciousness and inducing resuscitation, dredging channels and activating collaterals, harmonizing the qi and blood, which is the most prestigious large group of Tibetan medicinal formula used in the treatment of cardiovascular and cerebrovascular diseases in clinical practice (Xu et al., 2020, 2022). At present, there are no clear and well-defined protocols for the management of cognitive dysfunction in diabetes (Chow et al., 2022). Given those diabetic patients with comorbid cognitive impairment tend to be frailer, it is even more important to avoid under or over-treatment, and there is no evidence that intensive glycemic control or the use of specific anti-diabetic drugs can prevent cognitive decline (Draznin et al., 2022). Glucose-lowering drugs are often unable to prevent and treat the risk of cognitive dysfunction, while traditional Chinese medicine, with its multi-targeted, multi-pathway, and precise efficacy, has a relative advantage (Palleria et al., 2016; Chi et al., 2020). Ranasampel has been proven in clinical and experimental studies to improve cerebrovascular, neuroprotective and learning and memory abilities, which could be used in the treatment of cognitive dysfunction and other related disorders (Wu et al., 2016; Zhao et al., 2022).
Nowadays, much remains unknown about the deeper mechanisms underlying the association between diabetes and AD, and there are no specific drugs to prevent and treat diabetes-related cognitive dysfunction. So, the use of the Tibetan medicinal formula Ranasampel may provide a new vision for the prevention and treatment strategy of diabetic cognitive impairment. Therefore, in this study, we firstly identified the blood-entering active components and performed target prediction of Ranasampel by serum pharmacochemistry. Then, two gene expression datasets (GSE125387 and GSE164461) from the Gene Expression Omnibus (GEO) database were obtained to screen the possible targets and corresponding mechanisms for the regulation of diabetic cognitive impairment by the serum active ingredients of Ranasampel through bioinformatics study. Finally, the relevant target genes were validated by a microarray dataset of db/db mice treated with Ranasampel, to explore the possible pharmacodynamic basis of Ranasampel and provide new ideas and insights for the pharmacology research related to the prevention and treatment of cognitive impairment in diabetes. The flowchart of this study was shown in Figure 1.
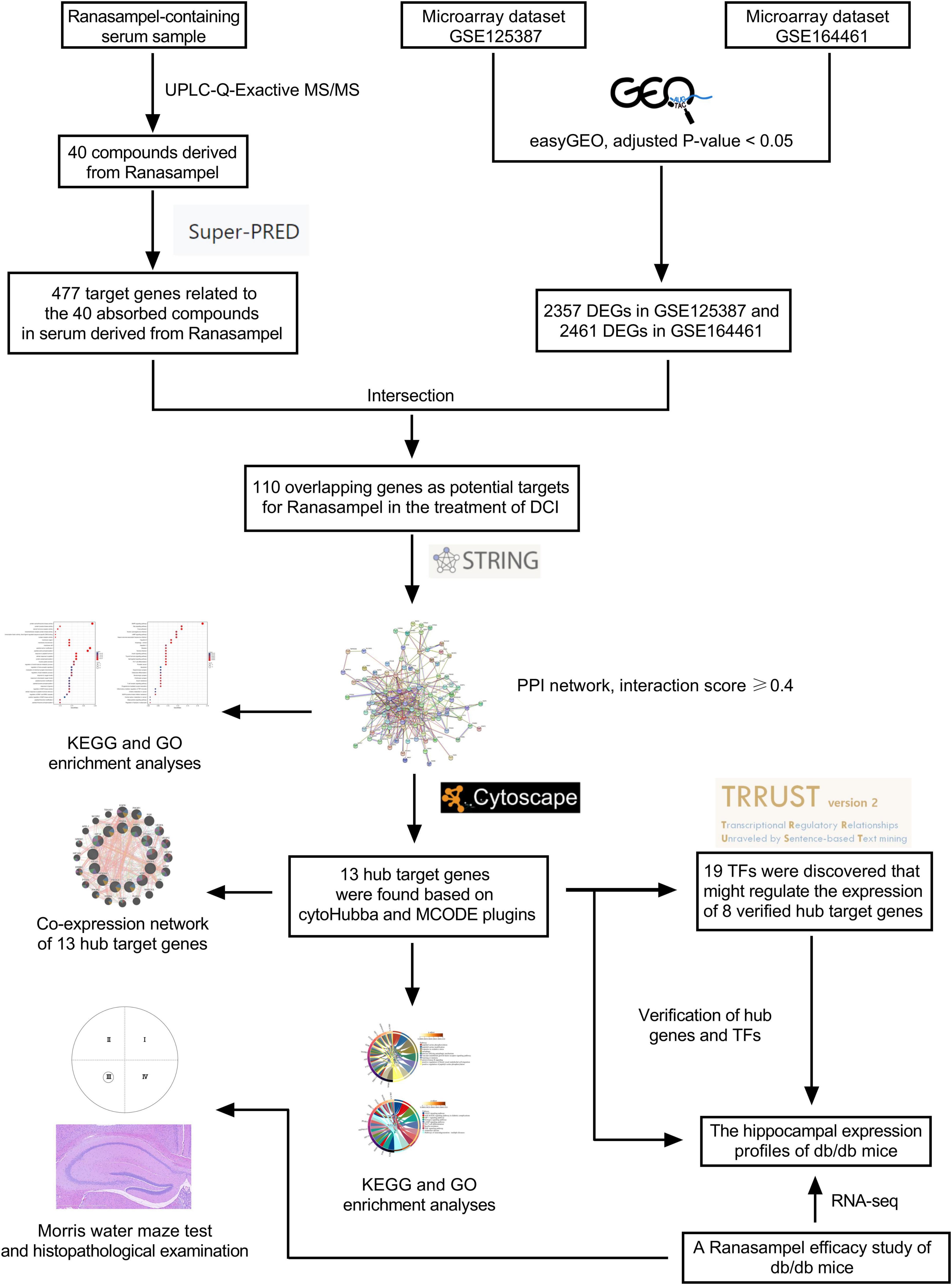
Figure 1. Flow chart of serum pharmacochemistry study and microarray data analysis of Ranasampel for diabetes cognitive impairment (DCI) treatment.
2 Materials and methods
2.1 Preparation of drug-containing serum
According to the Chinese Pharmacopeia (2015 version), the Tibetan medicinal formula Qishiwei Zhenzhu Pills (Ranasampel) consists of more than 70 traditional pharmaceutical ingredients, including Margarita (pearl), Lignum Santali Albi (sandalwood), Lignum Dalbergiae Odoriferae (rosewood), Benzoinum (benzoin), Radix Glycyrrhizae (liquorice root), Lagotis Brachystachya (Shortspike Lagotis), Concretio Silicea Bambusae (tabasheer), Croci Stigma (saffron), Fructus Chebulae (medicine terminalia fruit), Moschus (Musk), Calculus Bovis (bezoar), and Cornu Saigae Tataricae (antelope horn) (Schwabl and Vennos, 2015; Fu et al., 2020). The Ranasampel used in the study were purchased from the Ganlu Tibetan Medicine Co., Ltd., Tibet Autonomous Region, China (Lot No. 210500114).
The male C57BL/6 mice aged 10-week-old were purchased from the Beijing Vital River Laboratory Animal Technology Co., Ltd. [No. SYXK (Jing) 2016-0006, Beijing, China]. The animals were housed in a standard animal feeding room (room temperature: 22 ± 2°C; relative humidity: 50 ± 15%; light condition: 12 h:12 h light: dark cycle) and fed a standard rodent diet. The clinical dosage of Ranasampel for adults (standard weight 70 kg) is 1 g/day (Nie et al., 2021), therefore the dose for animal experiments was calculated according to the body surface area-based equivalent dose calculation method (Chatelut and Puisset, 2014) for humans and mice, and the gavage dose for mice was 0.125 g/kg/day.
This study was performed in agreement with the recommendations of the existing current animal welfare guidelines. The animal experimental protocols were approved by the Institutional Animal Care and Use Committee of the Beijing University of Chinese Medicine (No. BUCM-4-2022012105-1060). A total of 80 mice were assigned to 8 groups (n = 10), control group with no gavage, Ranasampel groups were administrated with Ranasampel in doses of 0.125 g/kg (group 1x); 0.25 g/kg (group 2x); 0.5 g/kg (group 4x); 0.75 g/kg (group 6x); 1.0 g/kg (group 8x); 1.25 g/kg (group 10x); and 1.5 g/kg (group 12x). The drug-containing serum of Ranasampel was prepared as previously reported (Ge et al., 2010; Du et al., 2020). Briefly, drug intervention lasted for 1 week, and fasting for 12 h after gavage on day 6. The abdominal aortic blood was taken under aseptic condition within 2 h after gavage on day 7, then centrifuged at 4°C 4,000 r/min for 10 mins to obtain Ranasampel drug-containing serum. Finally, serum samples were inactivated by water bath at 56°C for 30 mins and filtered through a 0.22 μm filter membrane, then stored at −80°C for the serum pharmacochemistry analysis.
2.2 Serum pharmacochemistry approach
The serum pharmacochemistry method was performed to identify the serum components of Ranasampel using Vanquish UHPLC System with Q-Exactive HF Mass Spectrometer (Thermo Fisher Scientific) (Wang et al., 2019), then the network pharmacological method was used to predict the targets of identified compounds in the drug-containing serum.
Chromatographic separation was conducted on a Zorbax Eclipse C18 column (1.8 μm × 2.1 × 100 mm, Agilent technologies, CA, United States). The column temperature was set at 30°C and the flow rate was 0.3 mL/min. The mobile phase consisted of deionized water with 0.1% formic acid (A) and pure acetonitrile (B). The gradient elution program was as follows: 0–2 min, 5% B; 2–6 min, 30% B; 6–7 min, 30% B; 7–12 min, 78% B; 12–14 min, 78% B; 14–17 min, 95% B; 17–20 min, 95% B; 20–21 min, 5% B; 21–25 min, 5% B.
The mass spectrometer analysis was performed using an ESI ion source in both positive and negative ion modes. The heating temperatures and capillary temperatures were 330°C and 335°C, respectively. The flow rates of the sheath gas, the auxiliary gas, and the sweep gas were 45 arb, 15 arb, and 1 arb, respectively. The electrospray voltage was 3.5 kV and the S-Lens RF Level was 55%. The LC-MS data were collected in the Full Scan/ddMS2 mode (m/z 100–1500; Top N = 10) and analyzed by Compound Discoverer 3.2. According to the processing parameters (ppm; mass tolerance; RT tolerance; and Area) and mass spectrometry information (mass spectrometry fragment patterns; chemical composition; chromatographic elution behavior; and corresponding reference alignments), the compounds were identified by using the Thermo mzCloud online database and the Thermo mzValut local database (Rohde et al., 2021; Wang et al., 2021). SMILES files of the compounds of Ranasampel drug-containing serum were obtained from PubChem1 (Kim et al., 2021) and ChemSpider2 (Little et al., 2012) databases, then the target genes were predicted through the Super-PRED3 (Zhou et al., 2019) database. The Cytoscape (version 3.7.24, Boston, MA, United States) (Wu et al., 2020) was used to establish the “Active ingredients in drug-containing serum of Ranasampel − Predicted target genes” network.
2.3 Collation of datasets
The GEO database5, a public database created and maintained by the National Center for Biotechnology Information (NCBI), archives and freely distributes a large number of high-throughput gene expression and other functional genomics data submitted by research institutions worldwide (Clough and Barrett, 2016). “Alzheimer’s disease,” “diabetes mellitus,” and “cognitive dysfunction” were the search terms, while the test specimen included was mice. Two microarray datasets (GSE125387 and GSE164461) including RNA expression profiling were screened out. The GSE125387 dataset was performed on mice hippocampal tissue samples that differentiate between the db/db mice and db/m mice, while the GSE164461 dataset contained APPswe/PSEN1dE9 mice and wild-type mice. The platform used to analyze these data was the GPL23479 BGISEQ-500 (Mus musculus) (Zhang et al., 2022).
2.4 Identification of Ranasampel target genes
The easy Visualization and Inference Toolbox for Transcriptome Analysis (eVITTA6) (Cheng et al., 2021) is a web-based toolbox for transcriptome analysis, which provides an R package (edgeR and limma)-based online tool for analysis and exploration of studies published in NCBI GEO (easyGEO). Microarray data from the GSE125387 and GSE164461 datasets were submitted to the easyGEO tool7 to determine differentially expressed genes (DEGs) in the hippocampal tissue samples between the diseased group and the control group. The genes with adjusted P-value < 0.05 were considered as DEGs, and the probes without corresponding gene symbols were excluded. Then, the predicted target genes of Ranasampel and the DEGs of two datasets were overlapped by the online Venn diagram tool to obtain the potential therapeutic target genes of Ranasampel for diabetes cognitive impairment. The DEGs and Ranasampel target genes were visualized using volcano plots and hierarchical clustering heat maps (R package: ggplot2 and pheatmap).
2.5 Protein–protein interaction network analysis
The Search Tool for the Retrieval of Interacting Genes (version 11.0) (STRING8) (Szklarczyk et al., 2019) was used to construct a protein–protein interaction (PPI) network of potential target genes of Ranasampel with complex regulatory relationships. The interaction score ≥ 0.4 were considered statistically significant. The Gene ontology (GO) function and Kyoto Encyclopedia of Genes and Genomes (KEGG) pathway analysis of the Ranasampel target genes was performed with the R package (clusterProfiler), and the enrichment analysis results with adjusted P-value < 0.05 were considered significant. Then, Cytoscape was used to visualize the network, and the molecular complex detection technology (MCODE) plugin of the software was used to analyze functional sub-modules. The selection criteria were set as follows: degree cutoff = 2, node score cutoff = 0.2, K-core = 2 and max depth = 100.
2.6 Screening and analysis of hub genes
The target genes in the PPI network were identified with the cytoHubba plugin of Cytoscape. Here, the common algorithms (MCC, MNC, EPC, Degree, Closeness, Stress, and Radiality) were used to explore the key genes (Su et al., 2021). Subsequently, the GO and KEGG enrichment analysis was used to identify the biological function of modules containing these key genes, while the key genes in the modules were defined as hub genes. Then, a co-expression network of these hub genes was constructed based on the GeneMANIA9 (Warde-Farley et al., 2010), which is a large set of functional association databases to find protein and genetic interactions, pathways, co-expression, co-localization, and protein domain similarity of gene sets.
2.7 RNA microarray verification of hub genes expression
A Ranasampel efficacy study of db/db mice was used to validate hub genes. Eight-week-old male db/db and db/m mice were purchased from Changzhou Cave Laboratory Animal Co., Ltd. (No. SCXK (Su) 2016-0010, Changzhou, China). Mice were divided into 3 groups (n = 11 for each group): (1) control group (db/m), (2) diabetes cognitive impairment (DCI) group (db/db), and (3) Ranasampel group (db/db + Ranasampel). All mice were housed in the Beijing University of Chinese Medicine animal feeding room under standard conditions with a temperature of 22 ± 2°C, relative humidity at 50 ± 15%, and 12 h:12 h light: dark cycle. Mice were fed regular chow and pure water. Control group and DCI group mice with no gavage, while Ranasampel group mice were daily administrated with Ranasampel in doses of 1.25 g/kg (10x the dose of Ranasampel based on serum pharmacochemistry study) for 4 weeks starting at 10 weeks of age. Then, 8 mice from each group were sacrificed to collect hippocampal tissues for RNA microarray analysis.
The RNA concentration and purity of hippocampal tissue samples were measured using NanoDrop 2000 (Thermo Fisher Scientific, DE, United States). RNA integrity was assessed using the RNA Nano 6000 Assay Kit of the Agilent Bioanalyzer 2100 system (Agilent Technologies, CA, United States). A total amount of 1 μg RNA per sample was used as input material for the RNA sample preparations. Sequencing libraries were generated using NEBNext UltraTM RNA Library Prep Kit for Illumina (NEB, United States) and index codes were added to attribute sequences to each sample (Tvedte et al., 2021). The clustering of the index-coded samples was performed on a cBot Cluster Generation System with TruSeq PE Cluster Kit v4-cBot-HS (Illumina) (Lynch et al., 2015). Then, the library preparations were sequenced on an Illumina platform and paired-end reads were generated. The raw reads were further processed with BMKCloud10 online platform, which is a bioinformatic pipeline tool, and the raw data (raw reads) of fastq format were processed through in-house perl scripts. At last, the quantification of gene expression was estimated by fragments per kilobase of transcript per million fragments mapped (FPKM), and the FPKM values were used to evaluate the expression levels of hub genes.
2.8 Behavior and histopathological assessment
The spatial learning and memory behaviors of the DCI mice in the Ranasampel efficacy study were measured using Morris water-maze test. The protocol was conducted and modified as previously described (Jiang et al., 2021). In brief, the water maze apparatus (XRXM101, Shanghai Xinruan Information Technology Co., Ltd., China) consists of a circular pool (diameter, 150 cm; height, 35 cm) and a high-definition video recorder connected with an automated tracking system. The pool area was equally divided into four quadrants (I, II, III, and IV). The hidden platform trials were conducted for 5 consecutive days (day 1–day 5) with a visible platform placed at quadrant III. The poll was filled with opaque water (temperature, 24 ± 1°C) which helped to hide the submerged part of the platform. On day 6, the platform was removed and a probe trial was conducted. The latency to the platform and the time spent in the target quadrant of mice in 60 s were recorded, and the data were automatically analyzed by SuperMaze software (Shanghai Xinruan Information Technology Co., Ltd, China).
The whole brain tissues of 3 mice in each group were fixed in a 4% PFA solution to prepare brain sections which included hippocampal cornu ammonis 1 (CA1), cornu ammonis 3 (CA3), and dentate gyrus (DG) regions based on the stereotaxic atlases of the mouse brain (Konsman, 2003). The sections were subjected to hematoxylin-eosin (H&E) staining to observe the hippocampal neurocytes and inflammatory cell infiltration. A four-point severity scale (0, normal; 1, mild; 2, moderate; 3, severe) was used to score the H&E stained sections by two experienced pathologists in a blinded manner (Yan et al., 2021).
2.9 Prediction and validation of transcription factors
The Transcriptional Regulatory Relationships Unraveled by Sentence-based Text mining (TRRUST11) (Han et al., 2018) database was used to obtain transcription factors (TFs) that regulate the validated hub genes, then the expressions of these TFs were verified in the microarray data. The transcriptional regulatory network of hub genes and TFs was visualized by Cytoscape software.
2.10 Statistical analysis
Except for the RNA sequencing data, the visualized data were expressed as means ± SEM, and the comparison between groups was performed by one-way ANOVA with T-test when equal variances were assumed. When the data had a normal distribution but the variances were not homogeneous, then Dunnett’s T3 test was used. P-value < 0.05 was considered statistically significant and the statistics were visualized by R package (ggplot2).
3 Results
3.1 Serum pharmacochemistry analysis
After optimizing the chromatogram and mass spectrometric conditions, the UPLC-Q-Exactive MS/MS method was used to identify the serum compounds of Ranasampel. As shown in Figure 2, drug-containing serum samples from mice administered with Ranasampel were analyzed using both positive and negative ion modes. A total of 40 compounds derived from Ranasampel were identified according to the mzCloud and mzValut databases. These compounds were summarized in Table 1, which included organic acids, steroids, volatile oils, terpenoids, etc. In addition, the serum compounds of mice in the 10x dose group (1.25 g/kg) had a more comprehensive composition than the rest of the dose groups. However, the prototype components and metabolites in serum were not identified because of the complexity of Ranasampel compound metabolites.
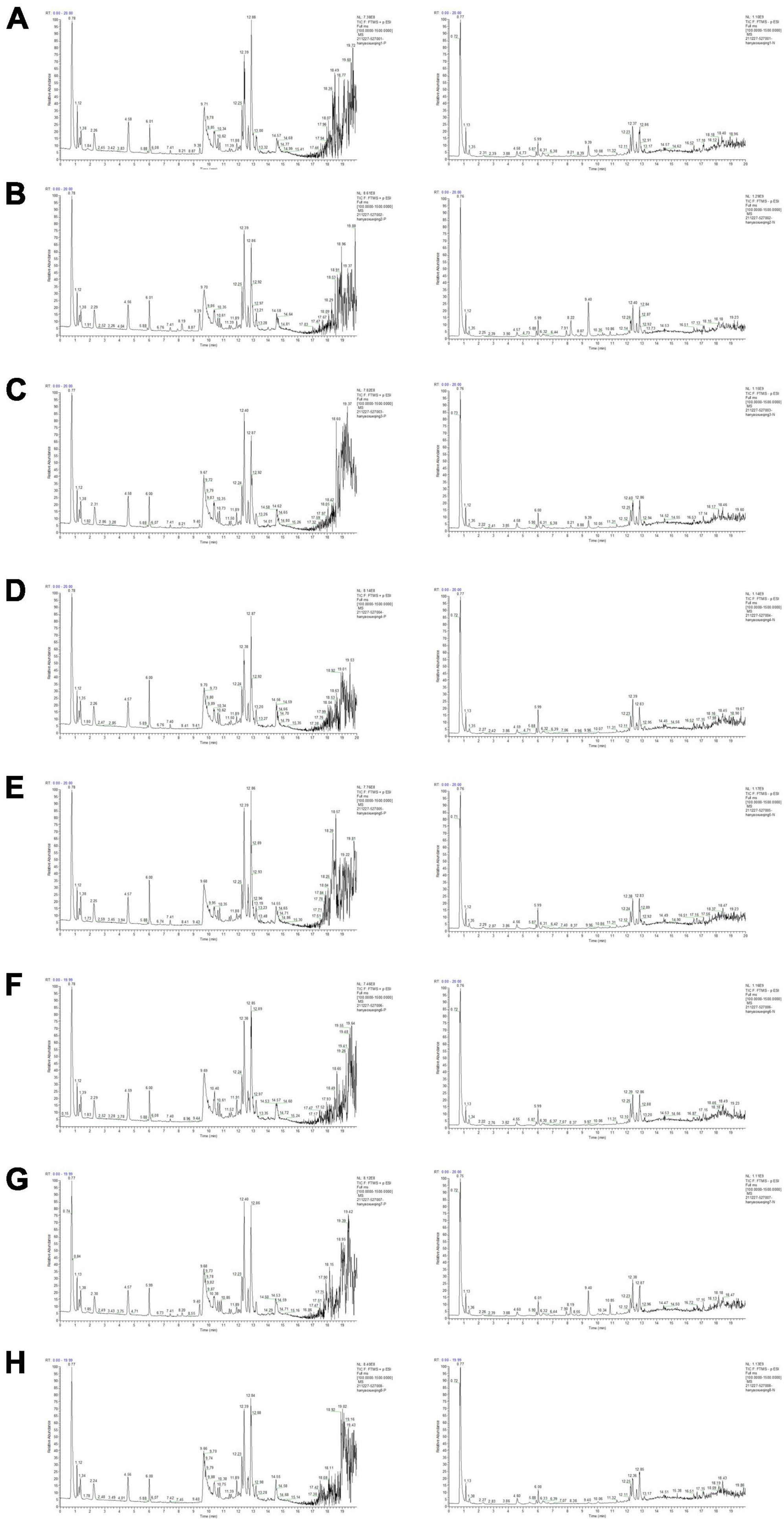
Figure 2. Total ion chromatographs of drug-containing serum samples from mice with oral administration of Ranasampel. (A) Serum samples of control group; (B) Serum samples of 1x dose group (0.125 g/kg); (C) Serum samples of 2x dose group (0.25 g/kg); (D) Serum samples of 4x dose group (0.5 g/kg); (E) Serum samples of 6x dose group (0.75 g/kg); (F) Serum samples of 8x dose group (1.0 g/kg); (G) Serum samples of 10x dose group (1.25 g/kg); (H) Serum samples of 12x dose group (1.5 g/kg).
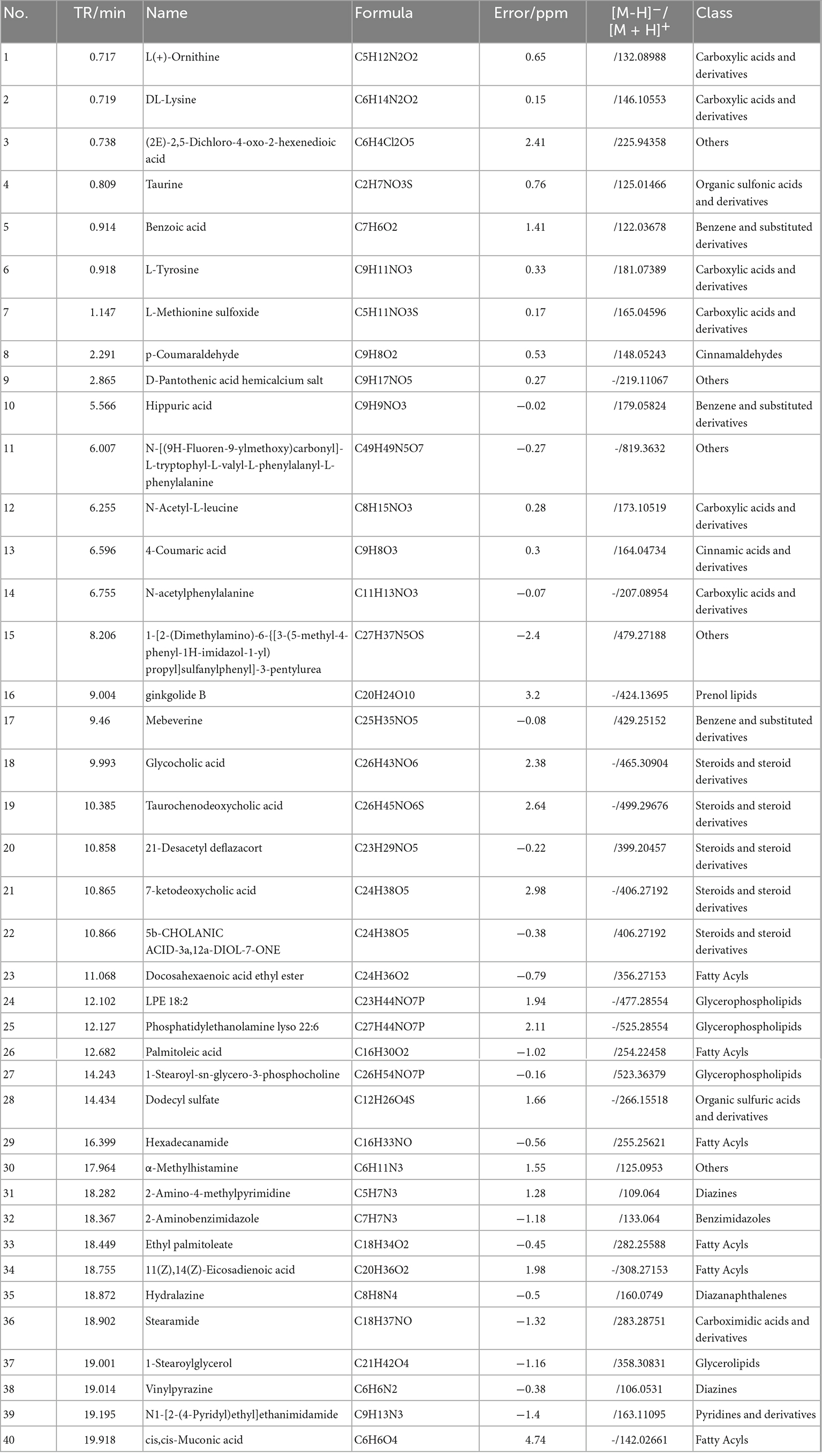
Table 1. UPLC-Q-Exactive MS/MS method was used to identify the active components in Ranasampel-containing serum.
Forty absorbed components were considered to be the potential active compounds in serum containing Ranasampel. These absorbed components were then used to predict the potential targets of Ranasampel. By searching the Super-PRED database, a total of 477 target genes related to the 40 absorbed compounds in serum derived from Ranasampel were predicted. As shown in Figure 3, the “active compounds in the drug-containing serum of Ranasampel − predicted target genes” network were constructed using Cytoscape software.
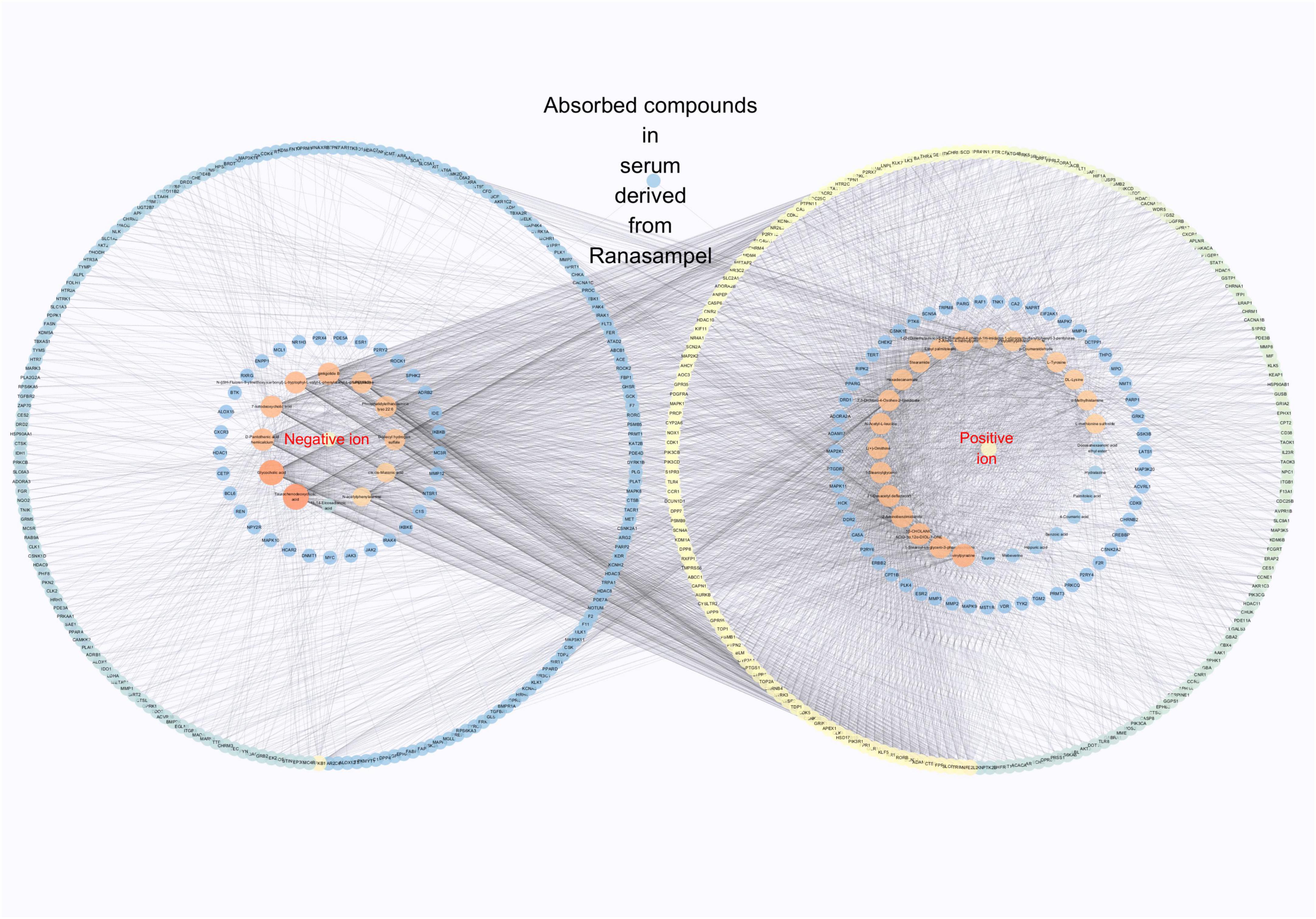
Figure 3. Active ingredients in drug-containing serum of Ranasampel – predicted target genes” network. These 40 absorbed components in serum derived from Ranasampel could regulate 477 potential target genes.
3.2 Analysis of the Ranasampel target genes
After standardizing the microarray results, DEGs (2357 in GSE125387 and 2461 in GSE164461, Supplementary Tables 1, 2) were identified (Figures 4A, B). Then, the potential target genes of Ranasampel and these DEGs were taken the intersection. As shown in the Venn diagram (Figure 4C), 110 overlapping genes were identified, which were considered as potential targets for Ranasampel in the treatment of DCI. The heat maps of Ranasampel target genes in the GSE125387 and GSE164461 were also displayed (Figures 4D, E).
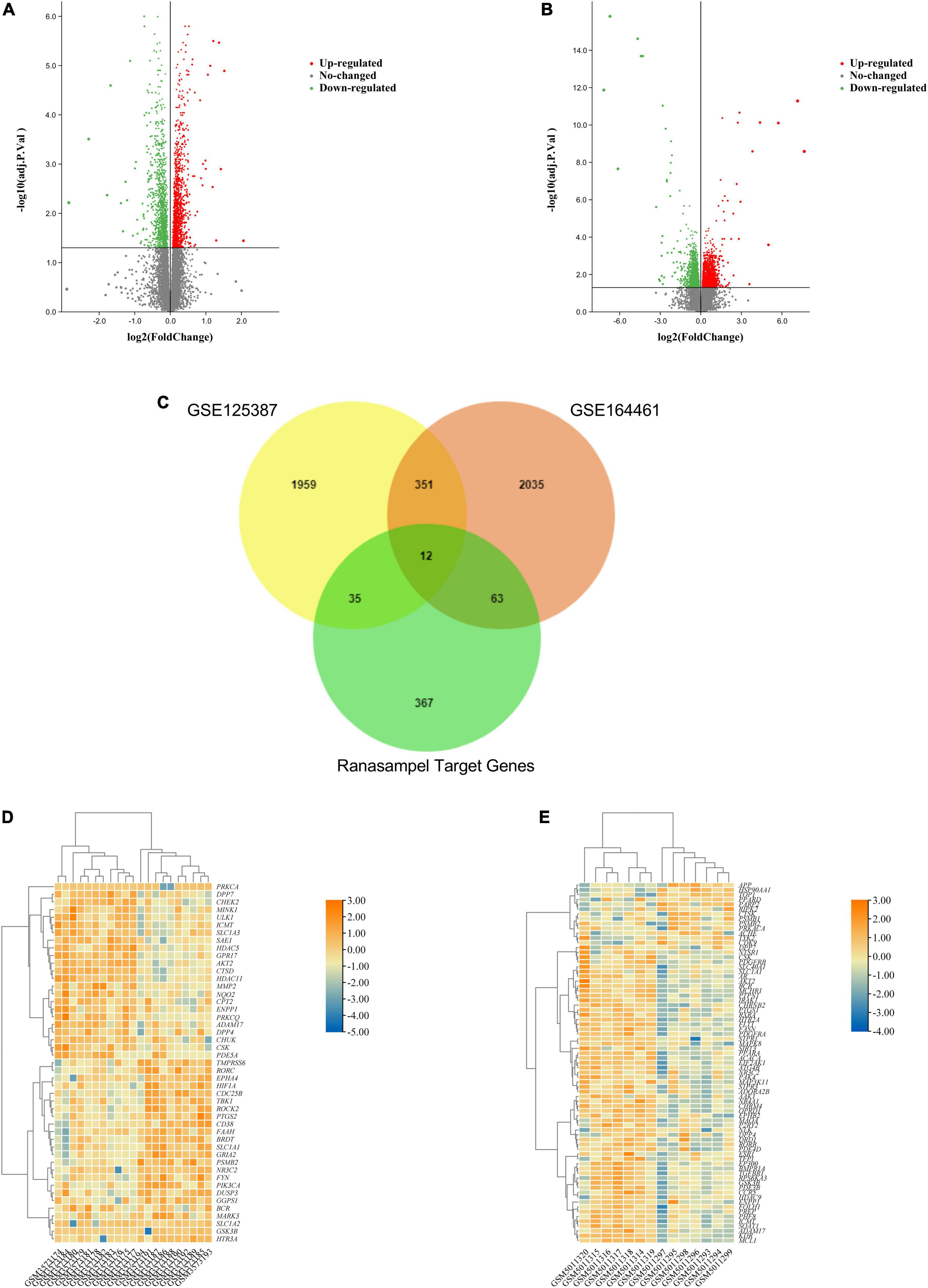
Figure 4. Differentially expressed genes of RNA microarray results and potential targets of Ranasampel for DCI. (A) The volcano map of GSE125387; (B) The volcano map of GSE164461. Red dots represent upregulated genes, green dots represent downregulated genes, adjust P < 0.05. (C) Venn diagram showed that an overlap of 47 potential target genes in GSE125387, 75 potential target genes in GSE164461, and 110 potential target genes for Ranasampel in the treatment of DCI; (D) The heat map of Ranasampel target genes in GSE125387; (E) The heat map of Ranasampel target genes in GSE164461.
These Ranasampel target genes with interaction scores greater than 0.4 were used to establish the PPI network in the STRING website, which contained 101 nodes and 402 edges (Figure 5A). Meanwhile, the PPI network file was maintained from STRING for module analysis by using Cytoscape. Five crucial sub-modules were screened through the MCODE plugin of Cytoscape, including 31 nodes and 67 edges (Figures 5B-F).
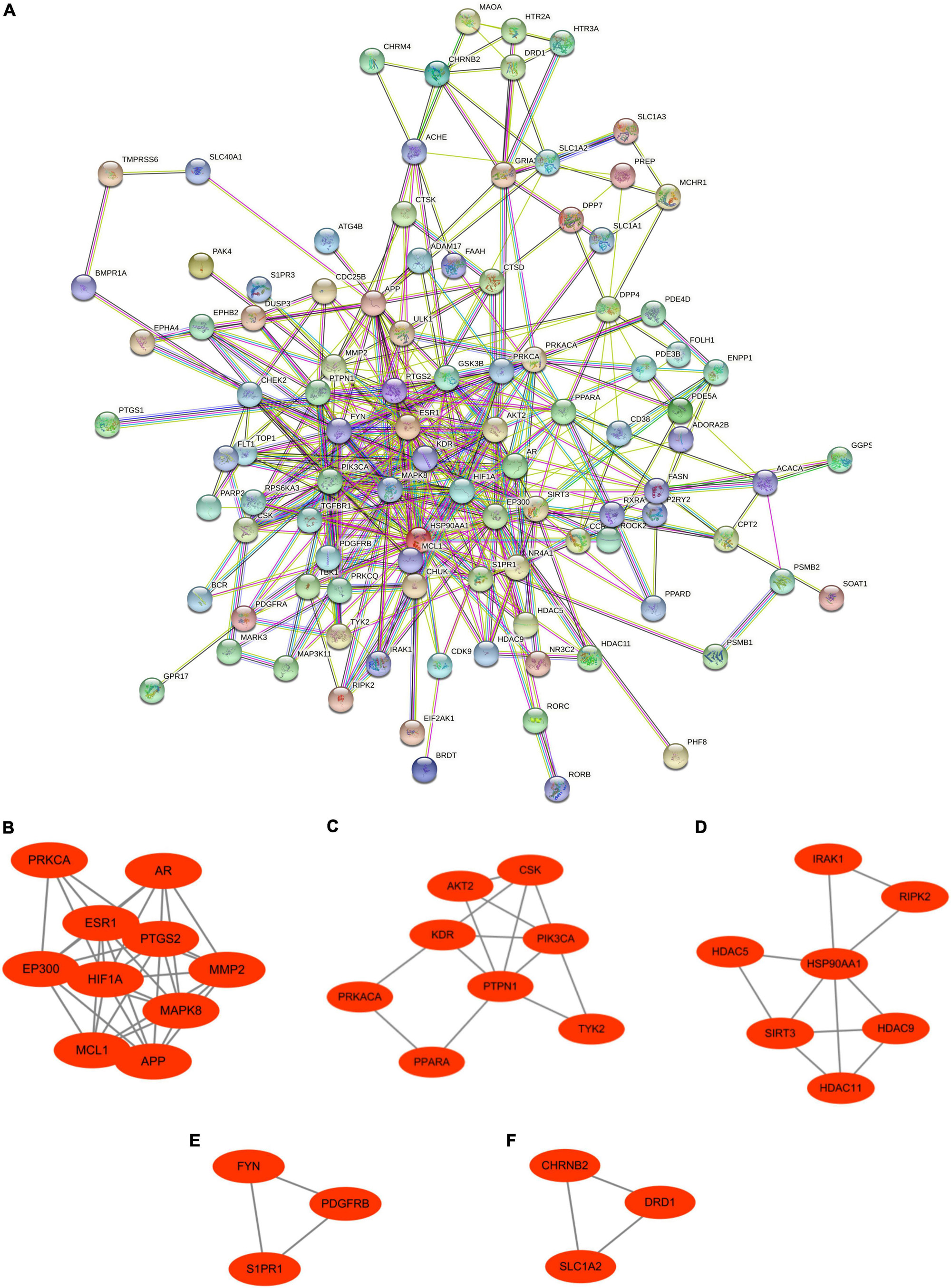
Figure 5. Protein–protein interaction network and significant gene modules of Ranasampel target genes. (A) The PPI network contained 101 nodes and 402 edges; (B–F) Five significant gene clustering modules contained 31 nodes and 67 edges.
Furthermore, to analyze the biological functions and signaling pathways involved in the 101 interact genes, GO and KEGG enrichment analyses were performed. GO analysis results exhibited that these genes were mainly enriched in protein serine/threonine kinase activity (adjust P = 1.15195E-14), response to oxygen levels (adjust P = 1.04485E-06), peptidyl-serine phosphorylation (adjust P = 7.08448E-16), protein autophosphorylation (adjust P = 9.4354E-12), and regulation of ERK1 and ERK2 cascade (adjust P = 1.82485E-06) (Figure 6A). These functional terms are relevant to systemic inflammation and neuroinflammation, which is critical in metabolic and central diseases. KEGG analysis results showed that genes to be mainly involved in the MAPK signaling pathway (adjust P = 5.00047E-05), Cholinergic synapse (adjust P = 0.000533567), Insulin resistance (adjust P = 0.000446102), cAMP signaling pathway (adjust P = 0.000475452), and Th17 cell differentiation (adjust P = 0.000171201) (Figure 6B), indicated that these pathways might be closely related to the Ranasampel efficacy.
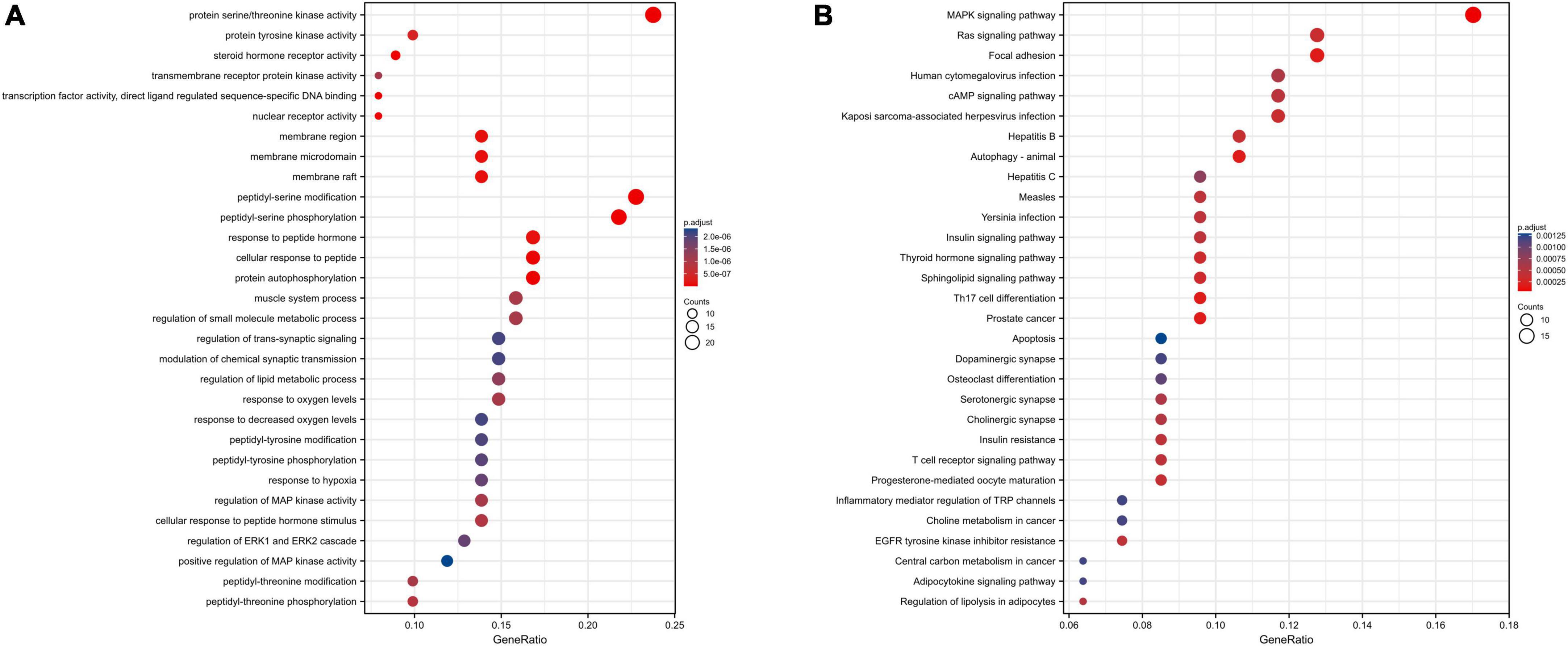
Figure 6. Functional enrichment analysis of interact genes in the PPI network. (A) Top 30 enriched GO terms; (B) Top 30 enriched KEGG pathways. The abscissa represented the frequency of the genes involved in total genes of this GO term, and the ordinate indicated the functional description of the enriched GO term. The size of the circle represented the number of genes enriched to this function, and the chromatograms from red to blue represented the adjusted P-value.
3.3 Identification and analysis of hub genes
To explore the key target genes of Ranasampel, the top 20 hub genes of the PPI network were calculated through the 7 algorithms of the cytoHubba plugin (Supplementary Table 3). The UpSet plot was used to demonstrate the common genes obtained by the 7 algorithms (Figure 7A). A total of 14 hub genes were discovered, including APP, EP300, ESR1, FYN, GSK3B, HIF1A, HSP90AA1, KDR, MAPK8, MMP2, PIK3CA, PPARA, PRKCA, and PTGS2. Then, after overlapping these hub genes with sub-modules of the PPI network, 13 hub target genes were found in 4 modules (Figures 7B-E).
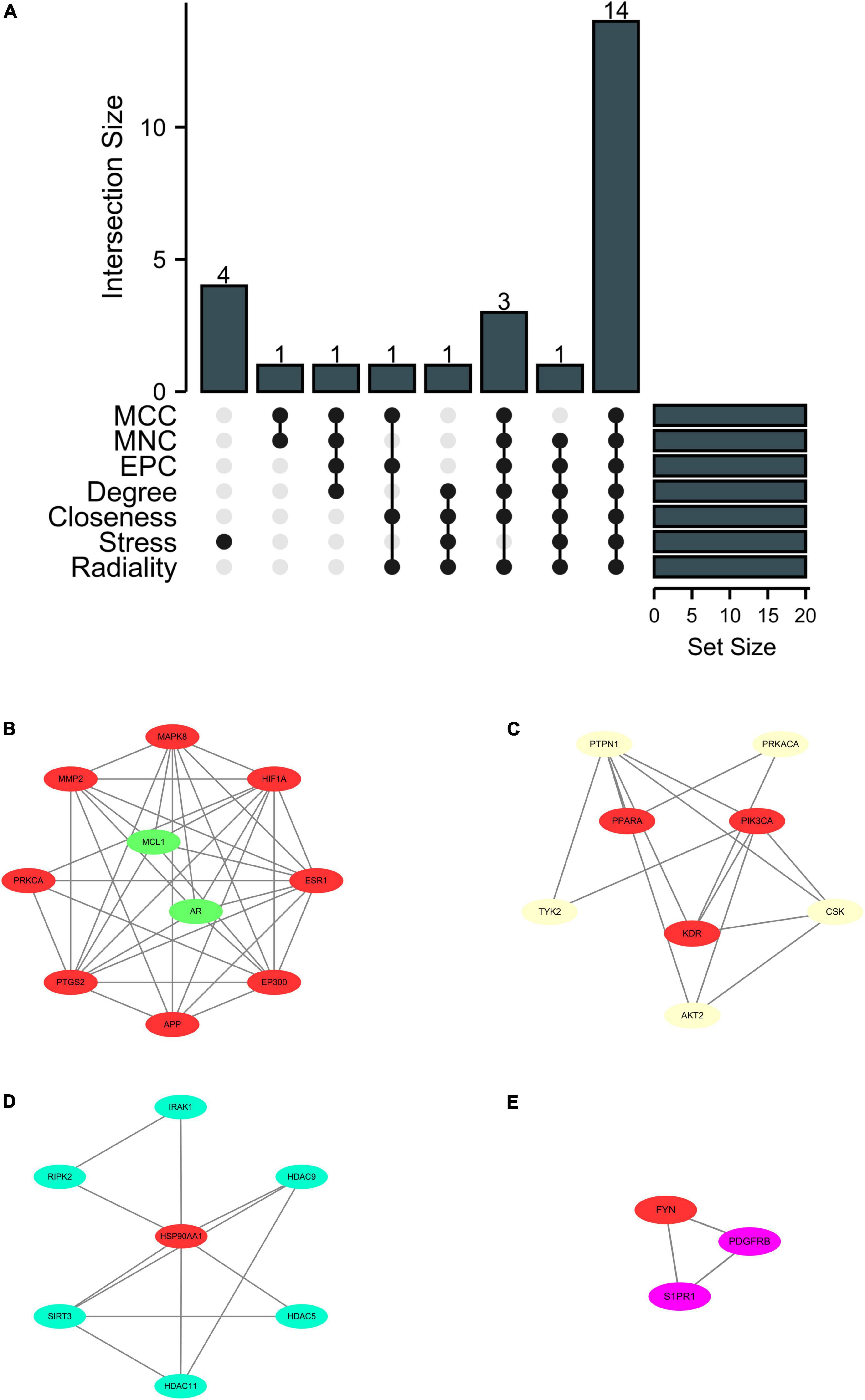
Figure 7. UpSet plot and modules overlapped with hub genes. (A) The UpSet plot represented 14 hub genes obtained from the cytoHubba plugin; (B–E) Four significant gene clustering modules from the PPI network overlapped 13 hub target genes. Red nodes represented the hub target genes in modules.
Based on the GeneMANIA database, the co-expression network and related functions of these 13 hub target genes were analyzed. Among 13 hub genes with interacting proteins, it was found that 77.64% had physical interactions, 8.01% exerted co-localization, 5.37% of the predicted rate, 3.63% displayed co-localization, 2.87% exhibited genetic interactions, 1.88% existed pathway and 0.6% shared protein domains (Figure 8A). To study further the 13 hub target genes, GO and KEGG enrichment analyses were carried out. GO analysis showed that 13 hub target genes were mainly involved in vascular endothelial growth factor receptor signaling pathway (adjust P = 6.60E-06), peptidyl-serine phosphorylation (adjust P = 2.05E-05), response to oxidative stress (adjust P = 5.00047E-05), autophagy (adjust P = 1.12E-04) and learning or memory (adjust P = 1.12E-04) (Figure 8B). The GO terms of results emphasized that the efficacy of Ranasampel might be relevant to the mechanisms of vascular endothelial growth, oxidative stress, inflammation, autophagy, and learning or memory. Meanwhile, KEGG analysis showed that these genes were mainly involved in VEGF signaling pathway (adjust P = 7.00E-05), AGE-RAGE signaling pathway in diabetic complications (adjust P = 2.32E-04), HIF-1 signaling pathway (adjust P = 2.97E-04), Insulin resistance (adjust P = 3.11E-03) and Alzheimer disease (adjust P = 7.36E-03) (Figure 8C).
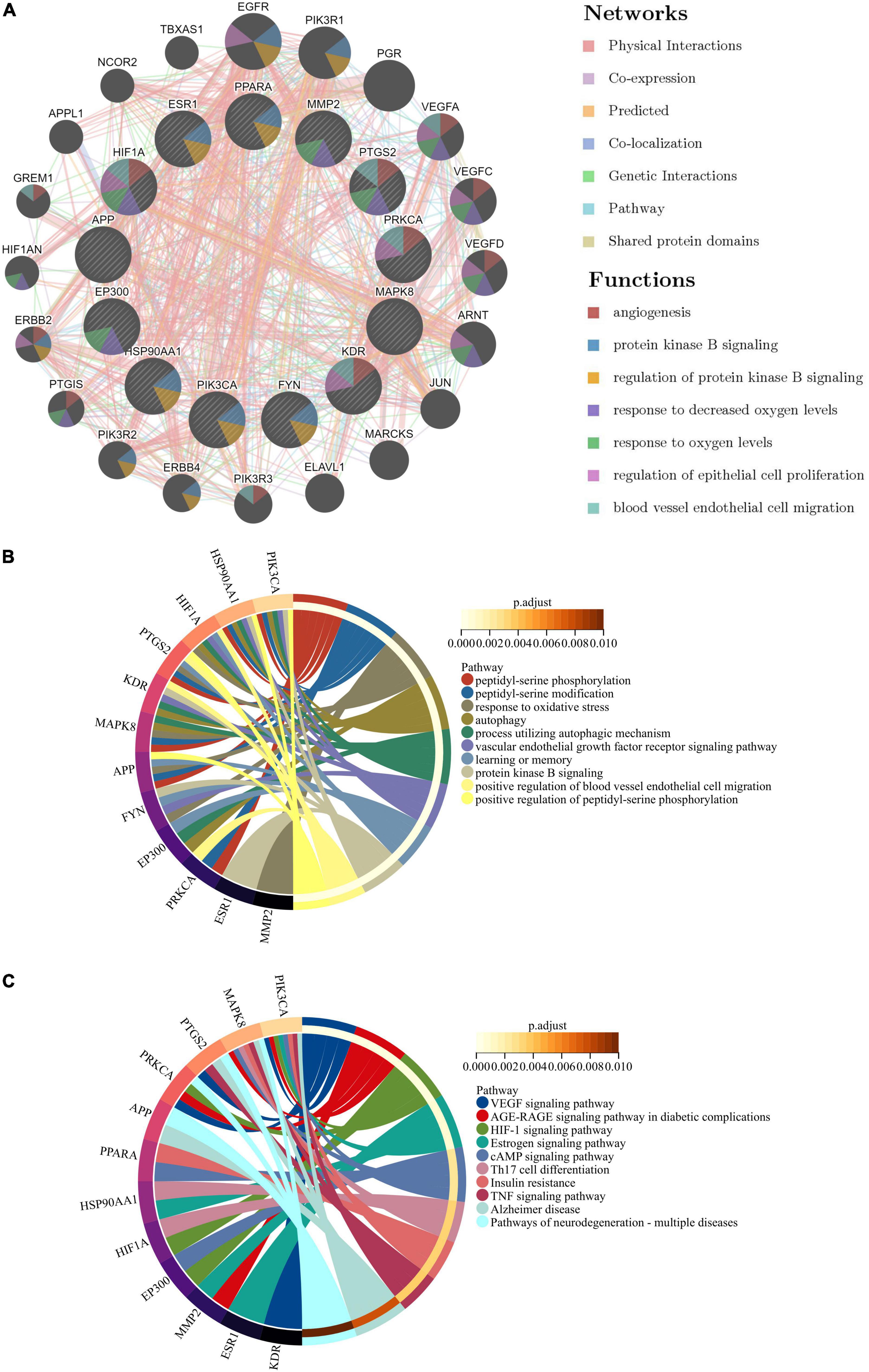
Figure 8. Co-expression network and enrichment analysis of module overlapped hub target genes. (A) Thirteen hub target genes and their co-expression genes were analyzed via GeneMANIA; (B, C) GO and KEGG analysis of 13 hub target genes. The outermost circle on the right represented GO and KEGG terms, and the inner circle on the left represented the significant adjust P-value of enrichment results.
3.4 Ranasampel alleviated cognitive impairment in db/db mice
The Morris water-maze test was performed to assess the effects of Ranasampel on cognitive impairment in db/db diabetic mice. As shown in Figure 9, the 5-day hidden platform trials reflected that Ranasampel improved learning and memory deficits of db/db mice (P < 0.05). Furthermore, the probe trial on day 6 observed the time spent in the target quadrant indicating Ranasampel increased the spatial memory of db/db mice (P < 0.01).
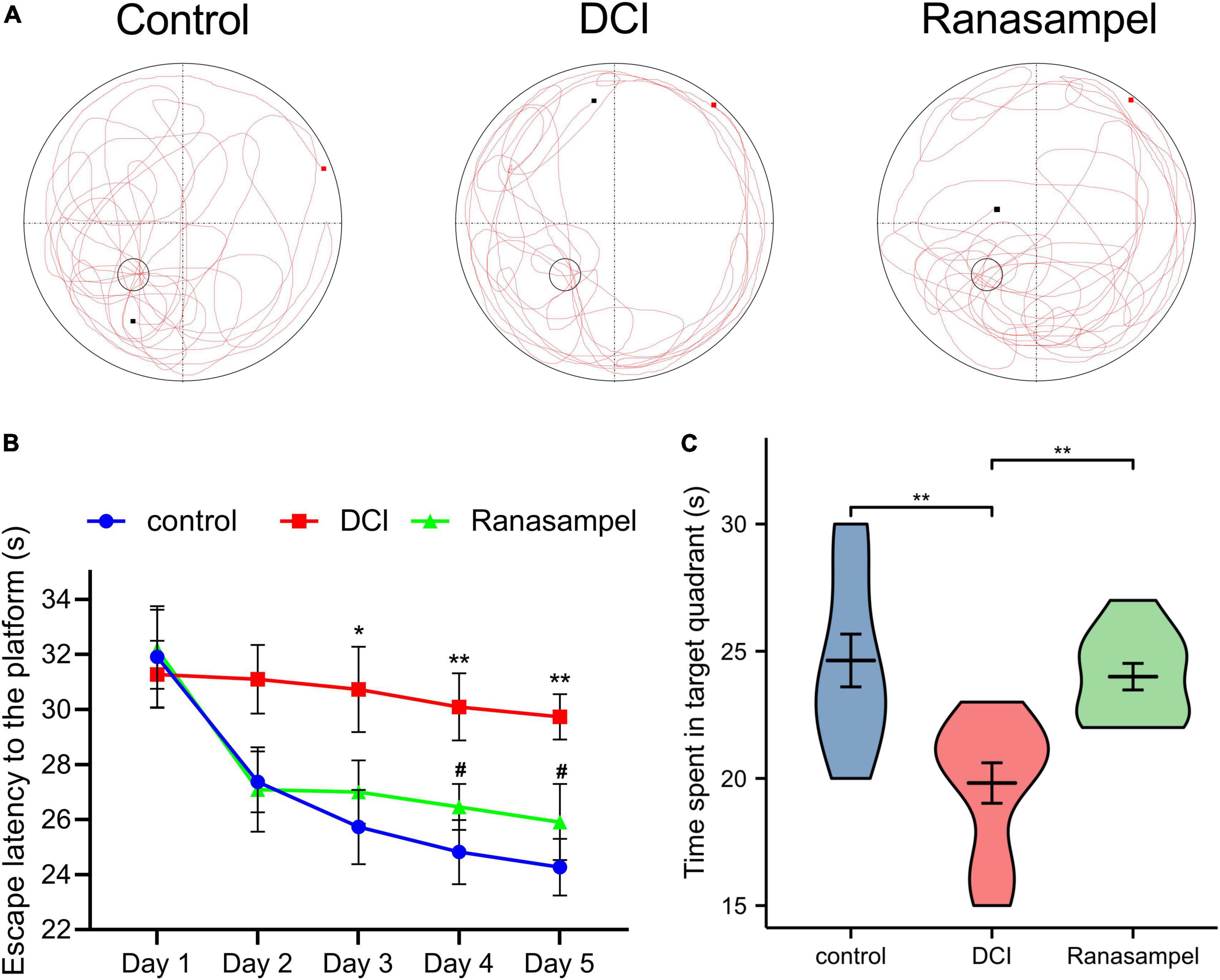
Figure 9. The Morris water-maze results of the Ranasampel efficacy study in db/db mice. (A) The representative moving trails in the Morris water-maze test of mice in each group; (B) The escape latency to the platform during the 5-day hidden platform trials (n = 11; *, DCI group compared with the control group; #, Ranasampel group compared with DCI group); (C) The results of the time spent in the target quadrant in the probe trial (n = 11). Data were expressed as means ± SEM, *P < 0.05; **P < 0.01; #P < 0.05.
Next, H&E staining was performed on the histopathological changes in mice hippocampal CA1, CA3, and DG regions to evaluate the neuronal damage and neuroinflammation. As exhibited in Figure 10A, the obvious neuronal loss, tissue cavitation, and abnormal shapes with hyperchromatic nuclei were found in the DCI group, while Ranasampel notably mitigated these histopathological changes, as determined according to the morphology and histological scores of the mouse hippocampus (P < 0.05 or P < 0.01) (Figures 10B-D).
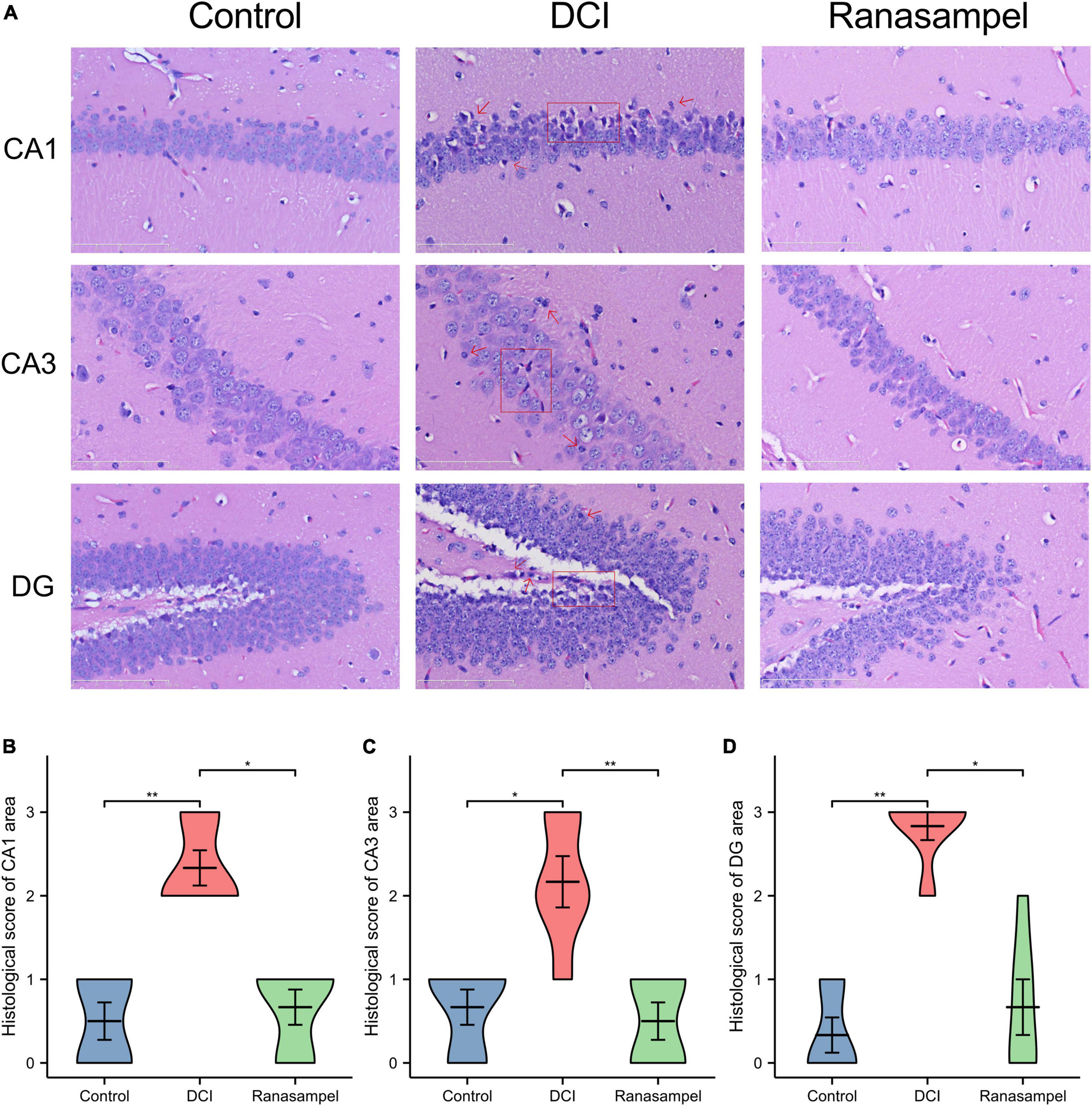
Figure 10. The histological changes in CA1, CA3, and DG regions of the mouse hippocampus. (A) Representative micrographs of H&E staining (scale bar = 200 μm, 400 × magnification) in the CA1 and CA3 regions (red arrows: hyperchromatic nuclei; red boxes: tissue cavitation and neuronal loss); (B) The histological scores in the CA1 region (n = 3); (C) The histological scores in the CA3 region (n = 3); (D) The histological scores in the DG region (n = 3). Data were expressed as means ± SEM, *P < 0.05; **P < 0.01.
3.5 Verification of hub genes expression
The hippocampal expression profiles of db/db mice after Ranasampel treatment (Supplementary Table 4) were used to verify the reliability of 13 hub target gene expression levels. The results showed that compared with the DCI group, the expressions of APP, EP300, FYN, HIF1A, KDR, MMP2, PIK3CA, and PPARA were significantly up-regulated (P < 0.05 or P < 0.01), while the expression of PTGS2 was significantly down-regulated (P < 0.05) (Figure 11). However, the expression levels of ESR1, HSP90AA1, MAPK8, and PPKCA had no significant changes in this microarray dataset.
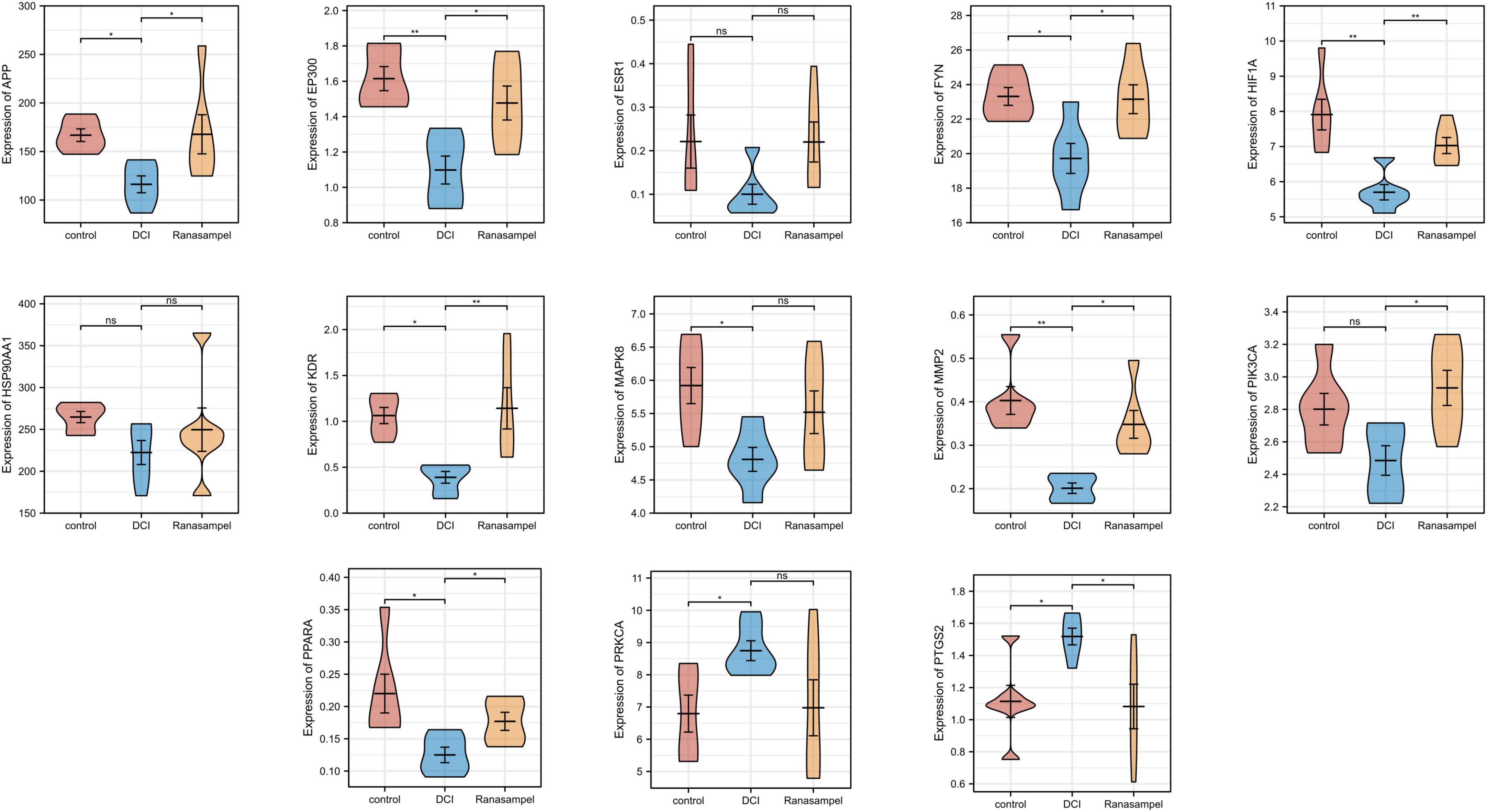
Figure 11. The expression levels of hub target genes in the hippocampal expression profiles of db/db mice after Ranasampel treatment. Data were expressed as means ± SEM, *P < 0.05; **P < 0.01. ns, no significance.
3.6 Prediction and validation of TFs
According to the TRRUST database, 19 TFs were discovered that might regulate the expression of 8 verified hub target genes (No TFs of FYN were found in the database; HIF1A was both the hub target gene and transcription factor) (Figure 12A and Table 2). Further validation, the expressions of CREB1, CREBBP, ETS2, HIF1A, RELA, SP1, and STAT3 were highly expressed in the dataset (P < 0.05 or P < 0.01) (Figure 12B). They synergistically participated in the regulation of 7 hub target genes (APP, EP300, HIF1A, MMP2, PIK3CA, PPARA, and PTGS2).
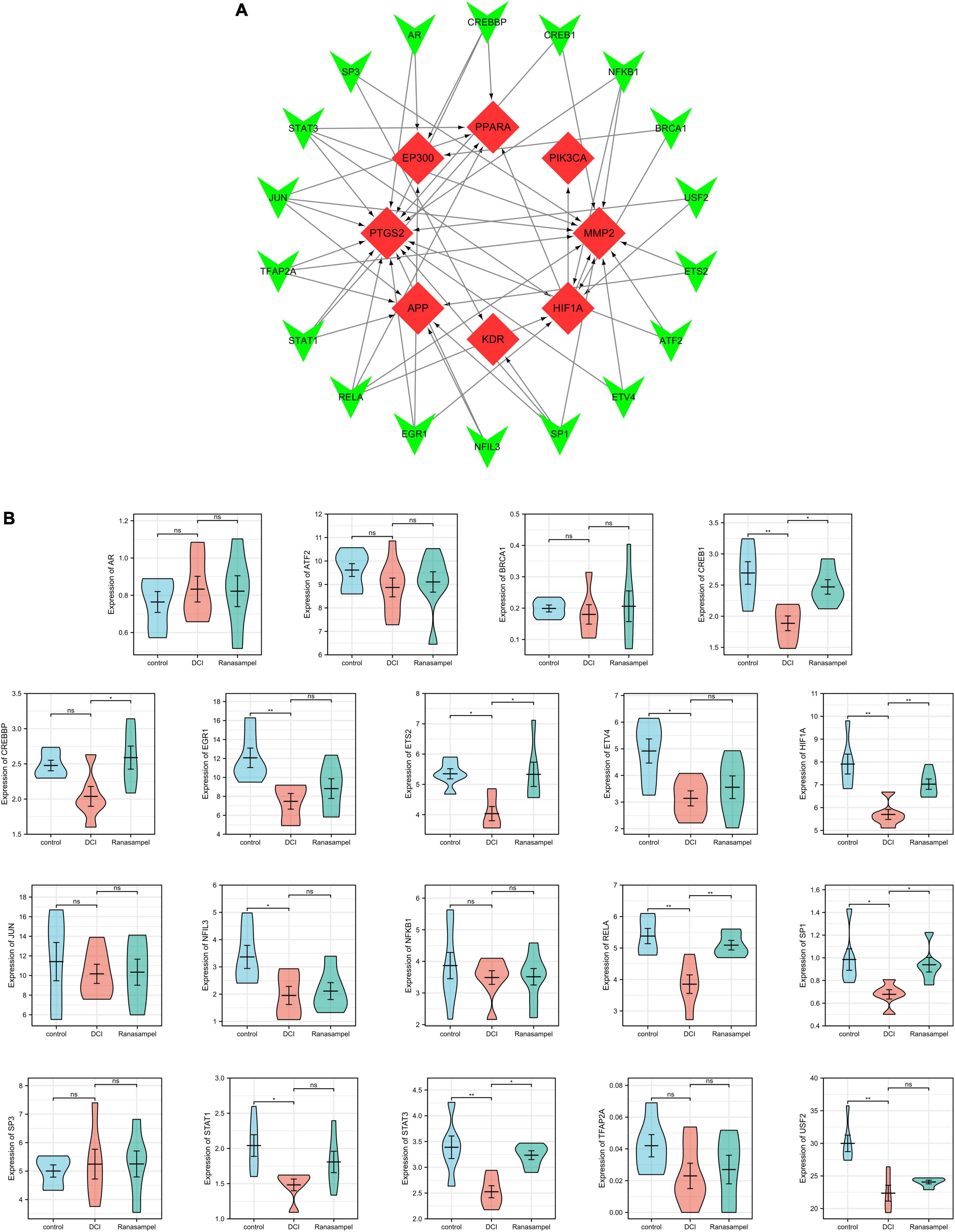
Figure 12. Transcription factors (TFs) regulatory network and validation of TFs. (A) The “TFs – verified hub target genes” network, TFs were marked in green, and the verified hub target genes were marked in red; (B) The expressions of TFs in the hippocampal expression profiles of db/db mice after Ranasampel treatment. Data were expressed as means ± SEM, *P < 0.05; **P < 0.01. ns, no significance.
4 Discussion
Clinical and epidemiological data suggest that diabetes is associated with less severe cognitive dysfunction and that people with diabetes are at high risk for cognitive dysfunction (Koekkoek et al., 2015; Biessels and Despa, 2018). According to statistics, 20% to 30% of people with diabetes will develop mild cognitive impairment and 17.3% will develop dementia (Alzheimer’s disease and vascular dementia), of which 20% of Type 2 diabetic patients over 60 years of age may develop dementia, with a significantly higher risk than non-diabetics (Albai et al., 2019; Kim et al., 2019). Therefore, given the increasing incidence of diabetes, the aging population and the impact of cognitive impairment on affected individuals and society as a whole, preventive treatment of diabetes is necessary (Zhang et al., 2018). However, while the evolving scientific literature does provide important clues, the understanding of potential therapeutic targets and underlying mechanisms of diabetes cognitive impairment (DCI) remains incomplete.
In recent years, the role of traditional Chinese medicine applied in the prevention and treatment of DCI has attracted much attention, whether it is classical prescriptions, traditional Chinese patent medicines, and simple preparations, or individual herbs, the active ingredients of which have unique advantages and efficacy, which deserve in-depth study (Seto et al., 2015). The efficacy of Ranasampel in improving vascular circulation, learning and memory functions, and neuroprotection is well documented for various cardiovascular and neurological diseases (Zhao et al., 2022). It is found that Ranasampel could reduce cerebral ischemia-reperfusion injury by inhibiting neuroinflammation and regulating gut microbiota in the rat cerebral ischemia model (Fu et al., 2021; Song et al., 2021). Also, Ranasampel may protect the neurons and glial cells by adjusting BBB function as well as lipid, fatty acid, and energy metabolism (Xu et al., 2020). Therefore, whether Ranasampel could be a complementary alternative medicine for the prevention and treatment of DCI also needed to be studied and demonstrated. In this study, we investigated the possible targets of Ranasampel to alleviate DCI and the related mechanisms by using serum medicinal chemistry and bioinformatics in this study, then verified these findings in a hippocampal expression profile of db/db mice treated with Ranasampel.
Traditional Chinese drugs are mostly administered orally and enter the bloodstream through the metabolism of various enzymes and microorganisms in the process of gastrointestinal and hepatic-intestinal circulation, which has multi-component, multi-pathway, and multi-target therapeutic characteristics, leading to complex and numerous components. This also indicates that the natural drugs do not only exert their therapeutic effect through direct action but may produce a combined effect through the integration of the natural drug ingredients, the metabolites of the natural ingredient and the endogenous physiologically active substances produced by the body as a result (Li et al., 2016). Serum pharmacochemistry is based on the serum after oral administration, considers blood components and their metabolites as the active ingredients for the action of a specific drug (Chen et al., 2022). Only by analyzing blood components can we avoid the blindness of in vitro chemical composition studies and the one-sidedness of taking individual components for pharmacokinetic studies, and be able to clarify the basis and mechanism of action of drugs in a more scientific manner.
In the present study, a total of 40 absorbed components were found in the Ranasampel-containing serum, which had 477 predicted target genes. The extensive overlap of these target genes across the components, suggesting that the therapeutic effects of Ranasampel are inextricably linked to the synergistic effects among the components. After that, we searched the GEO datasets and obtained hippocampal DEGs for diabetes and AD. Comparison of these DEGs with predicted targets identified 110 overlapping genes, predicting that Ranasampel might modulate these target genes and thereby alleviate DCI. The results of PPI network analysis acquired 101 targets of Ranasampel acting on DCI, of which 14 were hub genes. Then, 13 hub genes were cluster in modules of the PPI network, including APP, EP300, ESR1, FYN, GSK3B, HIF1A, HSP90AA1, KDR, MAPK8, MMP2, PIK3CA, PPARA, PRKCA, and PTGS2. The GO enrichment analysis revealed that these genes were significantly enriched in the vascular endothelial growth factor receptor (VEGF) signaling pathway, response to oxidative stress, positive regulation of blood vessel endothelial cell migration, autophagy, learning or memory, cognition, and positive regulation of peptidyl-serine phosphorylation. The KEGG results indicated that the therapeutic effect of Ranasampel in DCI mainly involved inflammatory and immune, diabetic complications and neurodegeneration pathways.
Insulin acts on tissues throughout the body to maintain plasma glucose within the physiological range by promoting glucose uptake and inhibiting glucose production and release by the liver (Wortham and Sander, 2016). Meanwhile, insulin also functions as an anabolic hormone that promotes fatty acid and amino acid uptake, energy storage, and cell growth (Saltiel, 2021). In addition, insulin also affects brain bioenergetics, enhances synaptic viability and dendritic spine formation, and increases neurotransmitter turnover. It also regulates vascular function through effects on vascular reactivity, lipid metabolism, and inflammation, and abnormal insulin regulation may lead to neurodegeneration (Kellar and Craft, 2020). Diabetes is characterized by elevated blood glucose levels caused by insufficient insulin production or insufficient insulin activity (Blüher, 2013). Among which, type 2 diabetes mellitus (T2DM) is caused by the inability of β cells to produce enough insulin to overcome systemic insulin resistance and is usually associated with obesity, inactivity, and aging (Van Dyken and Lacoste, 2018). Therefore, insulin resistance is a major pathogenic link in the development of T2DM and is emerging as a potentially important feature of Alzheimer’s disease and related dementias (Arnold et al., 2018). Most insulin in the brain is derived from circulating pancreatic insulin, which enters the brain mainly through selective, saturable transport across the capillary endothelium of the blood-brain barrier (BBB) (Unger and Betz, 1998; Banks et al., 2012). Insulin resistance prevents the normal intake and utilization of blood glucose, leading to loss of effective glucose control and causing hyperglycemia and T2DM. Hyperglycemia decreases BBB permeability to insulin, resulting in lower brain insulin levels and lower insulin-promoted neural and glial cell activity (Heni et al., 2014). On the other hand, T2DM causes damage to the BBB, which increases permeability to a variety of substances, and increased leukocyte extravasation, pro-inflammatory cytokine release, and microglia activation occur, resulting in central neuroinflammation and neuronal damage, further disrupting hippocampal function leading to cognitive impairment (Stranahan et al., 2016; Varatharaj and Galea, 2017). In the neuroinflammation process, increased glucose concentration in cells leads to increased oxidative respiration and reactive oxygen species (ROS) production (Wang et al., 2012). Then, ROS reacts with NO to form peroxynitrite. Increased glucose also leads to the formation of advanced glycosylation end products (AGEs), which act on the receptor for advanced glycosylation end products (RAGE) and increase the activation of NF-κB (Ding et al., 2020). Activated NF-κB in turn increases the expression of pro-inflammatory genes, including RAGE and cytokines. VEGF released from astrocytes activates protein kinase C (PKC) and Rho-associated kinase (ROCK), which further promotes inflammation (Van Dyken and Lacoste, 2018).
The Morris water-maze test is primarily used to test the learning and memory abilities of experimental animals (Hernández-Mercado and Zepeda, 2021). In previous studies, it was found that db/db mice had long-term memory impairment (Ye et al., 2018; Ma et al., 2020). In this study, the escape latency of mice in the Ranasampel group was found to be significantly shorter with increasing training days during the 5-day hidden platform trials. In the subsequent probe trial, mice in the Ranasampel group spent significantly more time in the target quadrant than mice in the DCI group. These suggested that Ranasampel improves spatial memory in db/db mice. We also found that Ranasampel attenuated neuronal cell injury and inflammation in the hippocampal CA1, CA3, and DG regions of db/db mice in the histological examination. In the following RNA microarray study, the expressions of 9 hub genes (APP, EP300, FYN, HIF1A, KDR, MMP2, PIK3CA, PPARA, and PTGS2) in the hippocampus were verified. Then, 19 TFs were discovered according to the validated hub genes. It also found that 7 validated TFs (CREB1, CREBBP, ETS2, HIF1A, RELA, SP1, and STAT3) participated in the regulation of APP, EP300, HIF1A, MMP2, PIK3CA, PPARA, and PTGS2. Amyloid precursor protein (APP) is a membrane-intrinsic protein expressed in a variety of tissues and concentrated at the synapses of neurons. The Amyloid beta (Aβ) derived from APP can lead to the formation of Aβ fibers in the brain and neural cell death, which is an important factor in AD (Luu et al., 2021). Impairment of learning and memory abilities is a major manifestation of AD. CREB1, a member of the cAMP response element binding protein family, regulates the transcription of long-term potentiation (LTP)-related genes, which are important for hippocampus-dependent memory (Li Y. et al., 2018). Past work has shown that insulin resistance causes elevated blood glucose and systemic metabolic disturbances, while hyperglycemia stimulates VEGF angiogenesis, leading to immature and unstable blood vessels (Kida et al., 2021). Moreover, VEGF also regulates angiogenesis in response to hypoxia through hypoxia-inducible factor 1 (HIF1) and PTGS2 (Wu et al., 2006; Masoud and Li, 2015). These lead to increased BBB permeability, neuroinflammation, and neuronal damage. HIF1, one of the upstream regulators of VEGF, is also upregulated in response to hyperglycemia and the resulting AGEs and ROS (Catrina, 2014). Downstream, VEGF signaling activates PKC, an enzyme involved in endothelial permeability, then hyperglycemia promotes dysfunction in its pathway, leading to increased NADPH oxidase and MMP2, further promoting cytokine expression and oxidative stress (Shao and Bayraktutan, 2013; Dorfman and Thaler, 2015). Some AGEs can cross-link proteins and produce substrates that inhibit normal protein function. Their production leads to the formation of ROS that causes damage to cells through oxidative stress (Ceriello, 2012; Perrone et al., 2020). ROS can also inhibit the downstream pathway of PIK3CA/AKT1/CREB, leading to mitochondrial function, which in turn weakens the intra-central ROS clearance and impairs neuronal development and nutrition (Ji et al., 2021). In addition, RAGE activates immune response by regulating many cytokines, while many RAGE effects are mediated through NF-κB (Tóbon-Velasco et al., 2014). Activation of NF-κB leads to upregulation of pro-inflammatory cytokines TNF-α, TGF-β1, and IL-1α expression, which enhances leukocyte infiltration and promoted further expression of RAGE (Van Dyken and Lacoste, 2018). Therefore, the inflammatory state induced by hyperglycemia will persist for a long time after the blood glucose level is controlled, and the alleviation of DCI requires effective control of neuroinflammation in addition to hypoglycemia. As shown in our results, the therapeutic effect of Ranasampel on DCI is not only related to its efficacy in improving learning and memory functions in the hippocampus but also associated with the modulation of HIF1A, EP300, MMP2, PIK3CA, PPARA, PTGS2, RELA, SP1, and STAT3 related neuroinflammation and neuronal damage in DCI (Figure 13). Although further studies are needed to determine the underlying mechanisms, our study highlights the potential of the traditional Tibetan formula Ranasampel as an effective treatment for DCI.
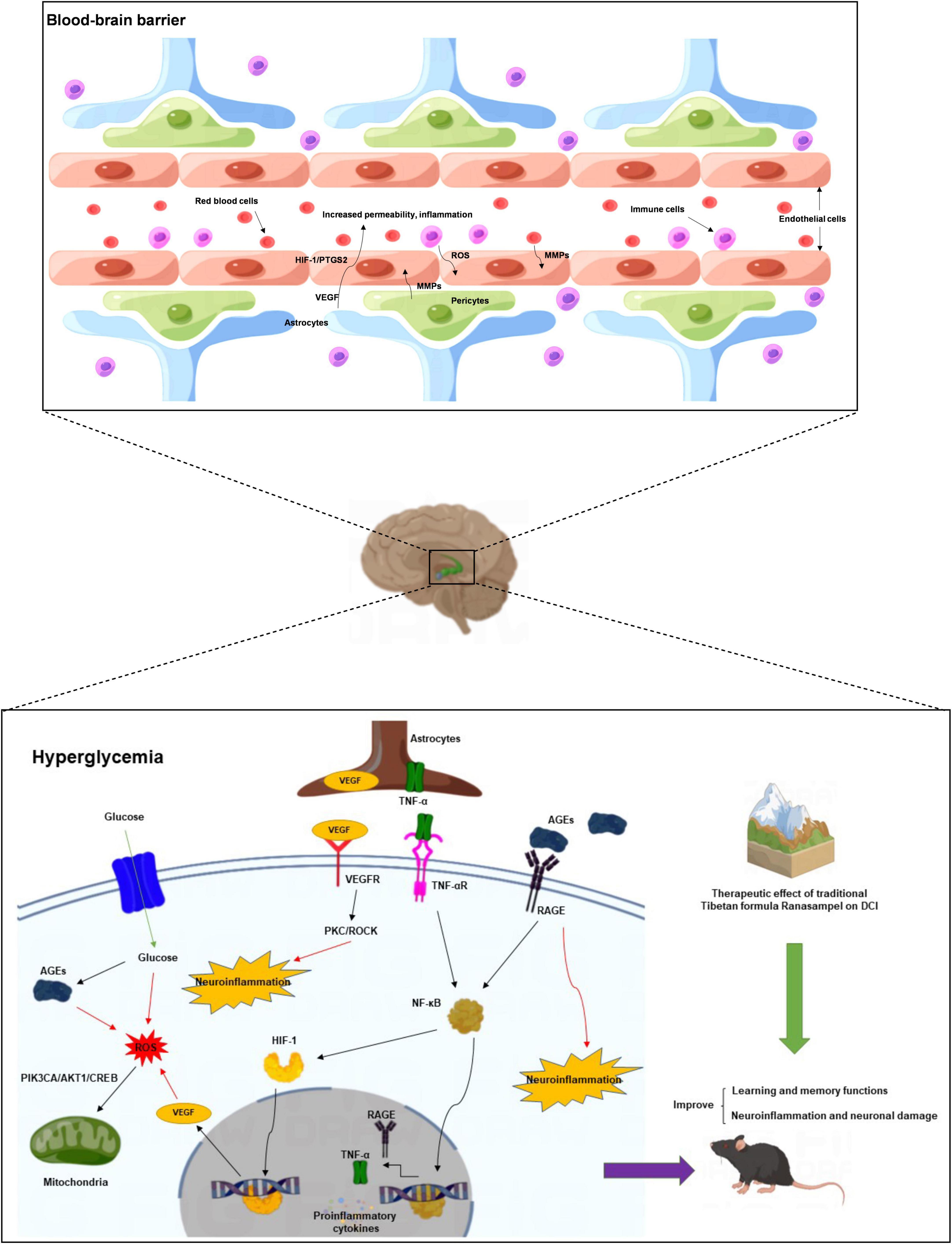
Figure 13. The mechanism of Ranasampel treatment of DCI may be related to the improvement of learning and memory function as well as insulin resistance and hyperglycemia-induced neuronal damage and neuroinflammation.
5 Conclusion
Generally, the current research combined serum pharmacochemistry and bioinformatics approaches to provide numerous testable hypotheses about the molecular mechanisms of the Ranasampel formula for the treatment of DCI. As predicted and verified, Ranasampel treatment improved diabetes mellitus-associated cognitive impairment behaviors and pathological changes in db/db mice and regulated many biological processes (response to oxidative stress, autophagy, learning or memory, cognition, neuron death, etc.) and pathways (VEGF signaling pathway, AGE-RAGE signaling pathway in diabetic complications, HIF-1 signaling pathway, Insulin resistance, TNF signaling pathway, etc.). These results provided a preliminary explanation of the ameliorative effect of Ranasampel against DCI and might precipitate the development of Ranasampel or its active compounds as an alternative therapy for DCI. However, the efficacy of Ranasampel on changes in metabolites and genes requires further evaluation in clinical and basic research, which will be the focus of our future research.
Data availability statement
The datasets presented in this study can be found in online repositories. The names of the repository/repositories and accession number(s) can be found in the article/Supplementary material.
Ethics statement
The animal study was reviewed and approved by this study was performed in agreement with the recommendations by the existing current animal welfare guidelines. The animal experimental protocols were approved by the Institutional Animal Care and Use Committee of Beijing University of Chinese Medicine (No. BUCM-4-2022012105-1060).
Author contributions
ZY, YZ, and TL conceived and designed the study. ZY drafted and wrote the manuscript. YZ, CZ, and ZH carried out the animal experiment. LW and LQ analyzed the data. All authors read and approved the final manuscript.
Funding
This research was supported by grants from the Science and Technology Project of Tibet Autonomous Region-Major Project (No. XZ201901-GA-02), and the Cooperative Innovation Center Project of Traditional Tibetan Medicine (Nos. 2018XTCX003 and 2019XTCX005).
Conflict of interest
The authors declare that the research was conducted in the absence of any commercial or financial relationships that could be construed as a potential conflict of interest.
Publisher’s note
All claims expressed in this article are solely those of the authors and do not necessarily represent those of their affiliated organizations, or those of the publisher, the editors and the reviewers. Any product that may be evaluated in this article, or claim that may be made by its manufacturer, is not guaranteed or endorsed by the publisher.
Supplementary material
The Supplementary Material for this article can be found online at: https://www.frontiersin.org/articles/10.3389/fnagi.2022.1033128/full#supplementary-material
Abbreviations
Aβ, amyloid beta; AD, Alzheimer’s disease; AGEs, advanced glycosylation end products; APP, amyloid precursor protein; BBB, blood-brain barrier; CA1, cornu ammonis 1; CA3, cornu ammonis 3; DCI, diabetes cognitive impairment; DEGs, differentially expressed genes; DG, dentate gyrus; GEO, Gene Expression Omnibus; GO, gene ontology; H&E, hematoxylin-eosin; HIF1, hypoxia-inducible factor 1; KEGG, Kyoto Encyclopedia of Genes and Genomes; LTP, long-term potentiation; MCODE, molecular complex detection technology; NCBI, National Center for Biotechnology Information; PKC, protein kinase C; PPI, protein-protein interaction; RAGE, receptor for advanced glycosylation end products; ROCK, Rho-associated kinase; ROS, reactive oxygen species; T2DM, type 2 diabetes mellitus; TFs, transcription factors; VEGF, vascular endothelial growth factor receptor.
Footnotes
- ^ http://pubchem.ncbi.nlm.nih.gov/
- ^ http://www.chemspider.com/
- ^ http://prediction.charite.de/
- ^ http://www.cytoscape.org/
- ^ http://www.ncbi.nlm.nih.gov/geo/
- ^ https://tau.cmmt.ubc.ca/eVITTA/
- ^ https://tau.cmmt.ubc.ca/eVITTA/easyGEO/
- ^ https://string-db.org/
- ^ http://genemania.org/
- ^ http://en.biocloud.net/
- ^ http://www.grnpedia.org/trrust/
References
Albai, O., Frandes, M., Timar, R., Roman, D., and Timar, B. (2019). Risk factors for developing dementia in type 2 diabetes mellitus patients with mild cognitive impairment. Neuropsychiatr. Dis. Treat. 15, 167–175. doi: 10.2147/NDT.S189905
Arnold, S., Arvanitakis, Z., Macauley-Rambach, S., Koenig, A., Wang, H., Ahima, R., et al. (2018). Brain insulin resistance in type 2 diabetes and Alzheimer disease: Concepts and conundrums. Nat. Rev. Neurol. 14, 168–181. doi: 10.1038/nrneurol.2017.185
Baglietto-Vargas, D., Shi, J., Yaeger, D., Ager, R., and LaFerla, F. (2016). Diabetes and Alzheimer’s disease crosstalk. Neurosci. Biobehav. Rev. 64, 272–287. doi: 10.1016/j.neubiorev.2016.03.005
Banks, W., Owen, J., and Erickson, M. (2012). Insulin in the brain: There and back again. Pharmacol. Ther. 136, 82–93. doi: 10.1016/j.pharmthera.2012.07.006
Biessels, G., and Despa, F. (2018). Cognitive decline and dementia in diabetes mellitus: Mechanisms and clinical implications. Nat. Rev. Endocrinol. 14, 591–604.
Blüher, M. (2013). Adipose tissue dysfunction contributes to obesity related metabolic diseases. Best Pract. Res. Clin. Endocrinol. Metab. 27, 163–177. doi: 10.1016/j.beem.2013.02.005
Cai, W., Xue, C., Sakaguchi, M., Konishi, M., Shirazian, A., Ferris, H., et al. (2018). Insulin regulates astrocyte gliotransmission and modulates behavior. J. Clin. Invest. 128, 2914–2926. doi: 10.1172/JCI99366
Catrina, S. (2014). Impaired hypoxia-inducible factor (HIF) regulation by hyperglycemia. J. Mol. Med. 92, 1025–1034. doi: 10.1007/s00109-014-1166-x
Ceriello, A. (2012). The emerging challenge in diabetes: The “metabolic memory”. Vasc. Pharmacol. 57, 133–138. doi: 10.1016/j.vph.2012.05.005
Chatelut, E., and Puisset, F. (2014). The scientific basis of body surface area-based dosing. Clin. Pharmacol. Ther. 95, 359–361. doi: 10.1038/clpt.2014.7
Chen, Y., Dai, Y., Xia, J., Liu, J., Zhou, G., Chen, C., et al. (2022). Serum pharmacochemistry combining network pharmacology to discover the active constituents and effect of Xijiao Dihuang Tang prescription for treatment of blood-heat and blood-stasis syndrome-related disease. Oxid. Med. Cell. Longev. 2022:6934812. doi: 10.1155/2022/6934812
Cheng, X., Yan, J., Liu, Y., Wang, J., and Taubert, S. (2021). EVITTA: A web-based visualization and inference toolbox for transcriptome analysis. Nucleic Acids Res. 49, W207–W215. doi: 10.1093/nar/gkab366
Chi, X., Zhang, H., Zhang, S., and Ma, K. (2020). Chinese herbal medicine for gout: A review of the clinical evidence and pharmacological mechanisms. Chin. Med. 15:17. doi: 10.1186/s13020-020-0297-y
Chow, Y., Verdonschot, M., McEvoy, C., and Peeters, G. (2022). Associations between depression and cognition, mild cognitive impairment and dementia in persons with diabetes mellitus: A systematic review and meta-analysis. Diabetes Res. Clin. Pract. 185:109227. doi: 10.1016/j.diabres.2022.109227
Clough, E., and Barrett, T. (2016). The gene expression omnibus database. Methods Mol. Biol. 1418, 93–110. doi: 10.1007/978-1-4939-3578-9_5
Dakpa, T. (2014). Unique aspect of Tibetan medicine. Acupunct. Electrother. Res. 39, 27–43. doi: 10.3727/036012914X13966138791145
Demir, S., Nawroth, P., Herzig, S., and Ekim Üstünel, B. (2021). Emerging targets in type 2 diabetes and diabetic complications. Adv. Sci. 8:e2100275. doi: 10.1002/advs.202100275
Ding, B., Lin, C., Liu, Q., He, Y., Ruganzu, J., Jin, H., et al. (2020). Tanshinone IIA attenuates neuroinflammation via inhibiting RAGE/NF-κB signaling pathway in vivo and in vitro. J. Neuroinflammation 17:302. doi: 10.1186/s12974-020-01981-4
Dorfman, M., and Thaler, J. (2015). Hypothalamic inflammation and gliosis in obesity. Curr. Opin. Endocrinol. Diabetes Obes. 22, 325–330. doi: 10.1097/MED.0000000000000182
Draznin, B., Aroda, V., Bakris, G., Benson, G., Brown, F., Freeman, R., et al. (2022). 13. Older adults: Standards of medical care in diabetes-2022. Diabetes Care 45, S195–S207. doi: 10.2337/dc22-S013
Du, X., Chen, Q., Song, J., Xie, Y., Zhi, Y., Sun, R., et al. (2020). Rhubarb-Aconite Decoction (RAD) drug-containing serum alleviated endotoxin-induced oxidative stress injury and inflammatory response in caco-2 cells in vitro. Evid. Based Complement. Alternat. Med. 2020:5834502. doi: 10.1155/2020/5834502
Fu, K., Xu, M., Zhou, Y., Li, X., Wang, Z., Liu, X., et al. (2020). The status quo and way forwards on the development of Tibetan medicine and the pharmacological research of Tibetan materia medica. Pharmacol. Res. 155:104688. doi: 10.1016/j.phrs.2020.104688
Fu, K., Zhang, D., Song, Y., Xu, M., Wu, R., Xiong, X., et al. (2021). Tibetan medicine Qishiwei Zhenzhu pills can reduce cerebral ischemia-reperfusion injury by regulating gut microbiota and inhibiting inflammation. Evid. Based Complement. Alternat. Med. 2021:2251679. doi: 10.1155/2021/2251679
Ge, J., Wang, D., He, R., Zhu, H., Wang, Y., and He, S. (2010). Medicinal herb research: Serum pharmacological method and plasma pharmacological method. Biol. Pharm. Bull. 33, 1459–1465. doi: 10.1248/bpb.33.1459
Han, H., Cho, J., Lee, S., Yun, A., Kim, H., Bae, D., et al. (2018). TRRUST v2: An expanded reference database of human and mouse transcriptional regulatory interactions. Nucleic Acids Res. 46, D380–D386. doi: 10.1093/nar/gkx1013
Heni, M., Schöpfer, P., Peter, A., Sartorius, T., Fritsche, A., Synofzik, M., et al. (2014). Evidence for altered transport of insulin across the blood-brain barrier in insulin-resistant humans. Acta Diabetol. 51, 679–681. doi: 10.1007/s00592-013-0546-y
Hernández-Mercado, K., and Zepeda, A. (2021). Morris water maze and contextual fear conditioning tasks to evaluate cognitive functions associated with adult hippocampal neurogenesis. Front. Neurosci. 15:782947. doi: 10.3389/fnins.2021.782947
Ji, Y., Luo, J., Zeng, J., Fang, Y., Liu, R., Luan, F., et al. (2021). Xiaoyao pills ameliorate depression-like behaviors and oxidative stress induced by olfactory bulbectomy in rats via the activation of the PIK3CA-AKT1-NFE2L2/BDNF signaling pathway. Front. Pharmacol. 12:643456. doi: 10.3389/fphar.2021.643456
Jiang, J., Liu, H., Wang, Z., Tian, H., Wang, S., Yang, J., et al. (2021). Electroacupuncture could balance the gut microbiota and improve the learning and memory abilities of Alzheimer’s disease animal model. PLoS One 16:e0259530. doi: 10.1371/journal.pone.0259530
Kellar, D., and Craft, S. (2020). Brain insulin resistance in Alzheimer’s disease and related disorders: Mechanisms and therapeutic approaches. Lancet Neurol. 19, 758–766. doi: 10.1016/S1474-4422(20)30231-3
Kida, T., Oku, H., Osuka, S., Horie, T., and Ikeda, T. (2021). Hyperglycemia-induced VEGF and ROS production in retinal cells is inhibited by the mTOR inhibitor, rapamycin. Sci. Rep. 11:1885. doi: 10.1038/s41598-021-81482-3
Kim, J., Ku, Y., Kim, H., Trinh, N., Kim, W., Jeong, B., et al. (2019). Oral diabetes medication and risk of dementia in elderly patients with type 2 diabetes. Diabetes Res. Clin. Pract. 154, 116–123. doi: 10.1016/j.diabres.2019.07.004
Kim, S., Chen, J., Cheng, T., Gindulyte, A., He, J., He, S., et al. (2021). PubChem in 2021: New data content and improved web interfaces. Nucleic Acids Res. 49, D1388–D1395. doi: 10.1093/nar/gkaa971
Koekkoek, P., Kappelle, L., van den Berg, E., Rutten, G., and Biessels, G. (2015). Cognitive function in patients with diabetes mellitus: Guidance for daily care. Lancet Neurol. 14, 329–340. doi: 10.1016/S1474-4422(14)70249-2
Konsman, J. (2003). The mouse brain in stereotaxic coordinates : Second edition (Deluxe) By Paxinos G. and Franklin, K.B.J., Academic Press, New York, 2001, ISBN 0-12-547637-X. Psychoneuroendocrinology 28, 827–828. doi: 10.1016/S0306-4530(03)00088-X
Kullmann, S., Kleinridders, A., Small, D., Fritsche, A., Häring, H., Preissl, H., et al. (2020). Central nervous pathways of insulin action in the control of metabolism and food intake. Lancet Diabetes Endocrinol. 8, 524–534. doi: 10.1016/S2213-8587(20)30113-3
Li, F., Zhang, Y., Wei, X., Song, C., Qiao, M., and Zhang, H. (2016). Metabolic profiling of Shu-Yu capsule in rat serum based on metabolic fingerprinting analysis using HPLC-ESI-MSn. Mol. Med. Rep. 13, 4191–4204. doi: 10.3892/mmr.2016.5082
Li, Q., Li, H., Xu, T., Du, H., Huan Gang, C., Fan, G., et al. (2018). Natural medicines used in the traditional tibetan medical system for the treatment of liver diseases. Front. Pharmacol. 9:29. doi: 10.3389/fphar.2018.00029
Li, Y., Zhang, W., Shi, R., Sun, M., Zhang, L., Li, N., et al. (2018). Prenatal caffeine damaged learning and memory in rat offspring mediated by ARs/PKA/CREB/BDNF pathway. Phys. Res. 67, 975–983. doi: 10.33549/physiolres.933906
Little, J., Williams, A., Pshenichnov, A., and Tkachenko, V. (2012). Identification of “known unknowns” utilizing accurate mass data and ChemSpider. J. Am. Soc. Mass Spectrom. 23, 179–185. doi: 10.1007/s13361-011-0265-y
Luo, W., Li, Q., Chen, J., Dor, J., Tao, S., Shi, J. B., et al. (2015). Textual research for Tibetan medicine Qumazi. Zhongguo Zhong Yao Za Zhi 40, 2047–2049.
Luu, L., Ciccotosto, G., and Cappai, R. (2021). The Alzheimer’s disease amyloid precursor protein and its neuritogenic actions. Curr. Alzheimer Res. 18, 772–786. doi: 10.2174/1567205018666211208141017
Lynch, K., Lucid, A., Arendt, E., Sleator, R., Lucey, B., and Coffey, A. (2015). Genomics of Weissella cibaria with an examination of its metabolic traits. Microbiology 161, 914–930. doi: 10.1099/mic.0.000053
Ma, H., Jiang, T., Tang, W., Ma, Z., Pu, K., Xu, F., et al. (2020). Transplantation of platelet-derived mitochondria alleviates cognitive impairment and mitochondrial dysfunction in db/db mice. Clin. Sci. 134, 2161–2175. doi: 10.1042/CS20200530
Manaserh, I., Maly, E., Jahromi, M., Chikkamenahalli, L., Park, J., and Hill, J. (2020). Insulin sensing by astrocytes is critical for normal thermogenesis and body temperature regulation. J. Endocrinol. 247, 39–52. doi: 10.1530/JOE-20-0052
Masoud, G., and Li, W. (2015). HIF-1α pathway: Role, regulation and intervention for cancer therapy. Acta Pharm. Sin. B 5, 378–389. doi: 10.1016/j.apsb.2015.05.007
Moran, C., Beare, R., Phan, T., Bruce, D., Callisaya, M., and Srikanth, V. (2015). Type 2 diabetes mellitus and biomarkers of neurodegeneration. Neurology 85, 1123–1130. doi: 10.1212/WNL.0000000000001982
Nie, Y., Xu, S., Lu, Y., Zhao, X., Li, C., Wei, L., et al. (2021). Zuotai (β-HgS)-containing 70 Wei Zhen-Zhu-Wan differs from mercury chloride and methylmercury on hepatic cytochrome P450 in mice. F1000Res. 10:203. doi: 10.12688/f1000research.40667.2
Palleria, C., Leporini, C., Maida, F., Succurro, E., De Sarro, G., Arturi, F., et al. (2016). Potential effects of current drug therapies on cognitive impairment in patients with type 2 diabetes. Front. Neuroendocrinol. 42:76–92. doi: 10.1016/j.yfrne.2016.07.002
Perrone, A., Giovino, A., Benny, J., and Martinelli, F. (2020). Advanced glycation end products (AGEs): Biochemistry, signaling, analytical methods, and epigenetic effects. Oxid. Med. Cell. Longev. 2020:3818196. doi: 10.1155/2020/3818196
Pugazhenthi, S., Qin, L., and Reddy, P. (2017). Common neurodegenerative pathways in obesity, diabetes, and Alzheimer’s disease. Biochim. Biophys. Acta Mol. Basis Dis. 1863, 1037–1045. doi: 10.1016/j.bbadis.2016.04.017
Rohde, E., Goudarzi, M., Madajka, M., Said, S., Ordenana, C., Rezaei, M., et al. (2021). Metabolic profiling of skeletal muscle during ex-vivo normothermic limb perfusion. Mil. Med. 186, 358–363. doi: 10.1093/milmed/usaa268
Saeedi, P., Petersohn, I., Salpea, P., Malanda, B., Karuranga, S., Unwin, N., et al. (2019). Global and regional diabetes prevalence estimates for 2019 and projections for 2030 and 2045: Results from the International Diabetes Federation Diabetes Atlas, 9(th) edition. Diabetes Res. Clin. Pract. 157:107843. doi: 10.1016/j.diabres.2019.107843
Saltiel, A. (2021). Insulin signaling in health and disease. J. Clin. Invest. 131:e142241. doi: 10.1172/JCI142241
Schwabl, H., and Vennos, C. (2015). From medical tradition to traditional medicine: A Tibetan formula in the European framework. J. Ethnopharmacol. 167, 108–114. doi: 10.1016/j.jep.2014.10.033
Seto, S., Yang, G., Kiat, H., Bensoussan, A., Kwan, Y., and Chang, D. (2015). Diabetes mellitus, cognitive impairment, and traditional Chinese medicine. Int. J. Endocrinol. 2015:810439. doi: 10.1155/2015/810439
Shao, B., and Bayraktutan, U. (2013). Hyperglycaemia promotes cerebral barrier dysfunction through activation of protein kinase C-β. Diabetes Obes. Metab. 15, 993–999. doi: 10.1111/dom.12120
Song, Y., Fu, K., Zhang, D., Xu, M., Wu, R., Xiong, X., et al. (2021). The absorption, distribution, and excretion of 18 elements of Tibetan medicine Qishiwei zhenzhu pills in rats with cerebral ischemia. Evid. Based Complement. Alternat. Med. 2021:4508533. doi: 10.1155/2021/4508533
Srikanth, V., Sinclair, A., Hill-Briggs, F., Moran, C., and Biessels, G. (2020). Type 2 diabetes and cognitive dysfunction-towards effective management of both comorbidities. Lancet Diabetes Endocrinol. 8, 535–545. doi: 10.1016/S2213-8587(20)30118-2
Stranahan, A., Hao, S., Dey, A., Yu, X., and Baban, B. (2016). Blood-brain barrier breakdown promotes macrophage infiltration and cognitive impairment in leptin receptor-deficient mice. J. Cereb. Blood Flow Metab. 36, 2108–2121. doi: 10.1177/0271678X16642233
Su, W., Zhao, Y., Wei, Y., Zhang, X., Ji, J., and Yang, S. (2021). Exploring the pathogenesis of psoriasis complicated with atherosclerosis via microarray data analysis. Front. Immunol. 12:667690. doi: 10.3389/fimmu.2021.667690
Szklarczyk, D., Gable, A., Lyon, D., Junge, A., Wyder, S., Huerta-Cepas, J., et al. (2019). STRING v11: Protein-protein association networks with increased coverage, supporting functional discovery in genome-wide experimental datasets. Nucleic Acids Res. 47, D607–D613. doi: 10.1093/nar/gky1131
Takenoshita, N., Fukasawa, R., Ogawa, Y., Shimizu, S., Umahara, T., Ishii, K., et al. (2018). Amyloid and tau positron emission tomography in suggested diabetesrelated dementia. Curr. Alzheimer Res. 15, 1062–1069. doi: 10.2174/1567205015666180709113338
Tóbon-Velasco, J., Cuevas, E., and Torres-Ramos, M. (2014). Receptor for AGEs (RAGE) as mediator of NF-kB pathway activation in neuroinflammation and oxidative stress. CNS Neurol. Disord. Drug Targets 13, 1615–1626. doi: 10.2174/1871527313666140806144831
Tvedte, E., Michalski, J., Cheng, S., Patkus, R., Tallon, L., Sadzewicz, L., et al. (2021). Evaluation of a high-throughput, cost-effective Illumina library preparation kit. Sci. Rep. 11:15925. doi: 10.1038/s41598-021-94911-0
Unger, J., and Betz, M. (1998). Insulin receptors and signal transduction proteins in the hypothalamo-hypophyseal system: A review on morphological findings and functional implications. Histol. Histopathol. 13, 1215–1224.
Van Dyken, P., and Lacoste, B. (2018). Impact of metabolic syndrome on neuroinflammation and the blood-brain barrier. Front. Neurosci. 12:930. doi: 10.3389/fnins.2018.00930
Varatharaj, A., and Galea, I. (2017). The blood-brain barrier in systemic inflammation. Brain Behav. Immun. 60, 1–12. doi: 10.1016/j.bbi.2016.03.010
Wang, J., Li, G., Wang, Z., Zhang, X., Yao, L., Wang, F., et al. (2012). High glucose-induced expression of inflammatory cytokines and reactive oxygen species in cultured astrocytes. Neuroscience 202, 58–68. doi: 10.1016/j.neuroscience.2011.11.062
Wang, L., Pu, X., Nie, X., Wang, D., Jiang, H., Chen, Y., et al. (2021). Integrated serum pharmacochemistry and network pharmacological analysis used to explore possible anti-rheumatoid arthritis mechanisms of the Shentong-Zhuyu decoction. J. Ethnopharmacol. 273:113988. doi: 10.1016/j.jep.2021.113988
Wang, X., Chang, X., Luo, X., Su, M., Xu, R., Chen, J., et al. (2019). An integrated approach to characterize intestinal metabolites of four phenylethanoid glycosides and intestinal microbe-mediated antioxidant activity evaluation in vitro using UHPLC-Q-exactive high-resolution mass spectrometry and a 1,1-diphenyl-2-picrylhydrazyl-based assay. Front. Pharmacol. 10:826. doi: 10.3389/fphar.2019.00826
Warde-Farley, D., Donaldson, S., Comes, O., Zuberi, K., Badrawi, R., Chao, P., et al. (2010). The GeneMANIA prediction server: Biological network integration for gene prioritization and predicting gene function. Nucleic Acids Res. 38, W214–W220. doi: 10.1093/nar/gkq537
Wortham, M., and Sander, M. (2016). Mechanisms of β-cell functional adaptation to changes in workload. Diabetes Obes. Metab. 18, (Suppl 1) 78–86. doi: 10.1111/dom.12729
Wu, G., Luo, J., Rana, J., Laham, R., Sellke, F., and Li, J. (2006). Involvement of COX-2 in VEGF-induced angiogenesis via P38 and JNK pathways in vascular endothelial cells. Cardiovasc. Res. 69, 512–519. doi: 10.1016/j.cardiores.2005.09.019
Wu, P., Luo, Y., Zhen, L., Hu, X., Shang, Y., Liao, Y., et al. (2016). Rannasangpei is a therapeutic agent in the treatment of vascular dementia. Evid. Based Complement. Alternat. Med. 2016:2530105. doi: 10.1155/2016/2530105
Wu, X., Sui, Z., Zhang, H., Wang, Y., and Yu, Z. (2020). Integrated analysis of lncRNA-mediated ceRNA network in lung adenocarcinoma. Front. Oncol. 10:554759. doi: 10.3389/fonc.2020.554759
Xu, M., Wu, R., Li, X., Zeng, Y., Liang, J., Fu, K., et al. (2022). Traditional medicine in China for ischemic stroke: Bioactive components, pharmacology, and mechanisms. J. Integr. Neurosci. 21:26. doi: 10.31083/j.jin2101026
Xu, M., Wu, R., Liang, Y., Fu, K., Zhou, Y., Li, X., et al. (2020). Protective effect and mechanism of Qishiwei Zhenzhu pills on cerebral ischemia-reperfusion injury via blood-brain barrier and metabonomics. Biomed. Pharmacother. 131:110723. doi: 10.1016/j.biopha.2020.110723
Yan, Z., Jiao, H., Chen, J., Zhang, K., Wang, X., Jiang, Y., et al. (2021). Antidepressant mechanism of traditional Chinese medicine formula Xiaoyaosan in CUMS-induced depressed mouse model via RIPK1-RIPK3-MLKL mediated necroptosis based on network pharmacology analysis. Front. Pharmacol. 12:773562. doi: 10.3389/fphar.2021.773562
Ye, T., Meng, X., Wang, R., Zhang, C., He, S., Sun, G., et al. (2018). Gastrodin alleviates cognitive dysfunction and depressive-like behaviors by inhibiting ER stress and NLRP3 inflammasome activation in db/db Mice. Int. J. Mol. Sci. 19:3977. doi: 10.3390/ijms19123977
Zhang, S., Yuan, L., Zhang, L., Li, C., and Li, J. (2018). Prophylactic use of troxerutin can delay the development of diabetic cognitive dysfunction and improve the expression of Nrf2 in the hippocampus on STZ diabetic rats. Behav. Neurol. 2018:8678539. doi: 10.1155/2018/8678539
Zhang, Y., Jiang, W., Xia, Q., Lin, J., Xu, J., Zhang, S., et al. (2022). Construction of a potential microRNA and messenger RNA regulatory network of acute lung injury in mice. Sci. Rep. 12:777. doi: 10.1038/s41598-022-04800-3
Zhao, M., Li, Y., and Wang, Z. (2022). Mercury and mercury-containing preparations: History of use, clinical applications, pharmacology, toxicology, and pharmacokinetics in traditional Chinese medicine. Front. Pharmacol. 13:807807. doi: 10.3389/fphar.2022.807807
Keywords: Qishiwei Zhenzhu Pills (Ranasampel), traditional Tibetan medicine, serum pharmacochemistry, microarray data analysis, diabetes cognitive impairment, hippocampus
Citation: Yan Z, Zong Y, Zhang C, Han Z, Wu L, Qin L and Liu T (2022) Exploring the role of Tibetan medicinal formula Qishiwei Zhenzhu Pills (Ranasampel) against diabetes mellitus-linked cognitive impairment of db/db mice through serum pharmacochemistry and microarray data analysis. Front. Aging Neurosci. 14:1033128. doi: 10.3389/fnagi.2022.1033128
Received: 31 August 2022; Accepted: 07 December 2022;
Published: 22 December 2022.
Edited by:
Ming Chen, ShanghaiTech University, ChinaReviewed by:
Fuqian He, Sichuan University, ChinaKundlik Gadhave, Johns Hopkins University, United States
Copyright © 2022 Yan, Zong, Zhang, Han, Wu, Qin and Liu. This is an open-access article distributed under the terms of the Creative Commons Attribution License (CC BY). The use, distribution or reproduction in other forums is permitted, provided the original author(s) and the copyright owner(s) are credited and that the original publication in this journal is cited, in accordance with accepted academic practice. No use, distribution or reproduction is permitted which does not comply with these terms.
*Correspondence: Tonghua Liu, ✉ dGhsaXVAdmlwLjE2My5jb20=
†These authors have contributed equally to this work