- 1Stark Neurosciences Research Institute, Indiana University School of Medicine, Indianapolis, IN, United States
- 2Medical Scientist Training Program, Indiana University School of Medicine, Indianapolis, IN, United States
- 3Medical Neuroscience Graduate Program, Indiana University School of Medicine, Indianapolis, IN, United States
- 4Department of Medical and Molecular Genetics, Indiana University School of Medicine, Indianapolis, IN, United States
- 5Department of Biochemistry and Molecular Biology, Indiana University School of Medicine, Indianapolis, IN, United States
Alzheimer’s disease (AD) genetics studies have identified a coding variant within ABI3 gene that increases the risk of developing AD. Recently, we demonstrated that deletion of the Abi3 gene locus dramatically exacerbates AD neuropathology in a transgenic mouse model of amyloidosis. In the course of this AD project, we unexpectedly found that deletion of the Abi3 gene locus resulted in a dramatic obese phenotype in non-transgenic mice. Here, we report our investigation into this serendipitous metabolic finding. Specifically, we demonstrate that mice with deletion of the Abi3 gene locus (Abi3–/–) have dramatically increased body weight and body fat. Further, we determined that Abi3–/– mice have impaired energy expenditure. Additionally, we found that deletion of the Abi3 gene locus altered gene expression within the hypothalamus, particularly within immune-related pathways. Subsequent immunohistological analysis of the central nervous system (CNS) revealed that microglia number and area were decreased specifically within the mediobasal hypothalamus of Abi3–/– mice. Altogether, this investigation establishes the functional importance of the Abi3 gene locus in the regulation of systemic metabolism and maintenance of healthy body weight. While our previous findings indicated the importance of Abi3 in neurodegeneration, this study indicates that Abi3 related functions are also essential for metabolic regulation.
1 Introduction
Perturbations in immune function have been demonstrated to exacerbate neurodegeneration and metabolic diseases (Lumeng and Saltiel, 2011; Ransohoff, 2016; Reilly and Saltiel, 2017). Additionally, it has been reported that metabolic diseases, such as obesity, increase the risk of developing neurodegenerative disease such as Alzheimer’s disease (AD) (Mazon et al., 2017; Zhuang et al., 2021). Intriguingly, it has been suggested that disrupted immune function is a potential link between these disease states (Pugazhenthi et al., 2017; Newcombe et al., 2018). However, the field currently has limited knowledge regarding the specific molecular drivers that alter the immune system to jointly modulate metabolic and neurodegenerative disease states. In this report, we describe how an unexpected discovery revealed that an AD-related gene, Abelson interactor family member 3 (Abi3), is involved in both immune and metabolic regulation. In combination with our previous report demonstrating the importance of Abi3 in AD-like pathology (Karahan et al., 2021), we have provided evidence to suggest that Abi3 may serve important roles at the intersection of metabolic, neurodegenerative, and immune regulation.
Our initial interest in the ABI3 gene was inspired by a human genetics study that identified a rare coding variant within ABI3 gene locus that is associated with increased risk of AD (Sims et al., 2017). Subsequently, we investigated the impact of Abi3 deletion on AD neuropathology in the 5xFAD amyloid-β (Aβ) amyloidosis mouse model. We recently reported that deletion of the Abi3 gene locus significantly exacerbated Aβ pathology and neuroinflammation in these mice (Karahan et al., 2021). In the course of that study, we unexpectedly discovered that Abi3 knock-out (Abi3–/–) mice had an obese phenotype.
Abi3 is predominately expressed in myeloid derived monocytic and microglial immune cells (Zhang et al., 2014; Han et al., 2018). This is relevant in the context of metabolism because recent studies have demonstrated that microglia, in particular microglia within the hypothalamus, affects the progression and onset of obesity (Coats et al., 2017; Valdearcos et al., 2017, 2019; Hill et al., 2018). However, the underlying molecular mechanisms by which these cells contribute to metabolic dysfunction and obesity require further exploration. While much remains to be discovered regarding the cellular functions of Abi3, it is suggested to exert its function through its participation in the WASP family verprolin-homologous protein-2 (WAVE2) complex, a regulator of cytoskeletal remodeling (Takenawa and Suetsugu, 2007; Moraes et al., 2017). However, the role of Abi3 in the context of metabolic regulation and metabolic disease is completely unknown.
In this report, we aim to address this knowledge gap by investigating the impact of Abi3 deletion on measures of systemic metabolism and obesity. Specifically, we demonstrated that Abi3 knock-out (Abi3–/–) mice exhibited an obese phenotype characterized by increased body weight and body fat, as well as impaired glucose tolerance and insulin sensitivity. Further, we determined that Abi3–/– mice had no changes in food intake but exhibited reduced energy expenditure. Additionally, through RNA-seq analysis, we found that deletion of the Abi3 gene locus altered gene expression, when controlling for differences in body weight, in the hypothalamus but not adipose tissue. Additionally, microglia number and area were decreased specifically within the mediobasal hypothalamus of Abi3–/– mice. Altogether, this study is the first to determine the functional importance of the Abi3 gene locus in the regulation of systemic metabolism and maintenance of healthy weight.
2 Material and materials
2.1 Animals
The mice utilized in this study were initially generated from a cross between 5XFAD and Abi3–/– mice; our study utilized the non-transgenic littermates from this cross. The 5xFAD mice were acquired from Jackson Laboratory [MMRRC 34840, B6SJL-Tg (APPSwFlLon, PSEN1* M146L* L286V) 6799Vas/Mmjax)]. The Abi3–/– mice were acquired from the University of California-Davis MMRRC Mouse Biology Program [C57BL/6N-Abi3tm1.1(KOMP)Vlcg]. The velocigene vector targeted the Abi3 gene locus on chromosome 11 from 95,842,143 to 95,832,627. Mice were genotyped for presenilin 1 (PSEN1) using the primers PSEN1-forward TCATGACTATCCTCCTGGTGG, PS1-reverse CGT TATAGGTTTTAAACACTTCCCC, internal positive control (IPC)-forward CTAGGCCACAGA ATTGAAAGATCT, and IPC-reverse GTAGGTGGAAATTCTAGCATCATCC. Non-transgenic mice were used in this study and genotyped for Abi3 using the following primers: wild-type forward (Wt-F) GGGTCATCTGACAGGTATCTGTTCC, wild-type reverse (Wt-R) CGTGAGTGGGAAGTGTTCTGGTTC, Reg-LacF forward (Reg-Lac-F) ACTTGCTTTAAAAAACCTCCCACA, and Reg-LacF reverse (Reg-Lac-R) GGTTGAGATTGAG AGTCCTTCTGGG. WT-F and WT-R primers produce a band from the Abi3 WT allele, whereas Reg-Lac-F and Reg-Lac-R produce a band from the KO allele. The non-transgenic (no 5xFAD) mice produced from the initial cross were used in this study. Our experiment utilized male mice at 15 months of age. The mice were provided standard housing conditions with ad libitum access to food and water.
Ethics Statement- The present study was performed in accordance with our reviewed and approved animal protocol from the Institutional Animal Care and Use Committee of Indiana University School of Medicine.
2.2 Tissue preparation
Mice were given Avertin intraperitoneally to induce anesthesia. Mice were then perfused transcardially with PBS. The brains and brown adipose tissue (BAT) were then rapidly removed. For RNA samples, the brains were dissected and immediately frozen in dry ice, along with the brown adipose tissue. For immunofluorescence, brain samples were fixed with 4% paraformaldehyde. The immunofluorescence samples were then passed through a sucrose gradient with 15% sucrose in PBS, followed by 30% sucrose in PBS overnight, followed by embedding and freezing in Fisher Healthcare OCT compound (cat # 23-730-571). All samples were then stored in −80°C prior to their final processing.
2.3 Body weight and composition
The mice were weighed on an Ohaus-SCOUT™-SPX622 Portable Precision Balance. Body composition was measured via EchoMRI™ 500. For EchoMRI, mice were guided into a containment tube which restricts movement, and imaging was acquired over a 1–4 min period using a 0.05 T electromagnetic field. Lean mass and fat mass were then calculated from T1 and T2 relaxing curves via standard algorithms.
2.4 Glucose tolerance test
Mice were fasted for 16 h prior to the start of the experiment. The mice then received 1 g/kg glucose by intraperitoneal injection. The tails of the mice were snipped, and blood was then collected at 0, 10, 20, 30, 60, 90, and 120 min. Blood glucose was measured via glucometer at each time point.
2.5 Insulin tolerance test
Mice were fasted for 2 h prior to the start of the experiment. The mice then received 0.75 units/kg insulin via intraperitoneal injection. The protocol for measuring blood glucose was identical to GTT, except readings were recorded only at 0, 15, 30, 45, and 60 min.
2.6 Metabolic cage analysis
PhenoMaster/LabMaster System (TSE Systems) calorimetry unit was used to measure food and water intake, gas consumption and production, respiratory exchange rate and locomotor activity of the mice. From these recordings, additional measures of metabolism such as energy expenditure and respiratory exchange ratio were generated. Mice were transferred and singly housed in the Phenomaster system, where they were given 3 days to acclimate. Following acclimation, recordings were collected over 84 h.
2.7 Total cholesterol assay
Total cholesterol levels were measured in plasma samples using a total cholesterol assay kit (Cell Biolabs, STA-384). Briefly, plasma was added to a reaction mixture that produces a colorimetric probe proportional to the amount of total cholesterol in the sample. The absorbance of the samples and standardized controls were then measured at 562 nm to determine the total amount of cholesterol in each sample, using a BioTek Synergy HTX Multi-Mode Plate Reader. The samples were run at a 1:50 dilution.
2.8 Leptin ELISA
Leptin concentration was measured in plasma samples using the U-Plex Mouse Leptin ELISA (Meso Scale Discovery, K1525ZK). Electrochemiluminescence signal was read on a MESO QuickPlex SQ 120. The samples were run at a 1:2 dilution.
2.9 RNA extraction
RNA was extracted from the hypothalami and brown adipose tissue via a standard phenol/chloroform extraction using TRIzol reagent (MRC). The concentration and purity of the RNA samples were then assessed via Nanodrop 2000 spectrophotometer. The isolated RNA was then stored at −80°C until further processing.
2.10 QuantSeq 3’ mRNA-seq library preparation, sequencing, and processing
Isolated RNA was shipped to Lexogen for library preparation and sequencing. Libraries were prepared manually according to the manufacturer’s instructions using QuantSeq 3’ mRNA-Seq FWD Library Prep Kit as outlined previously (Karahan et al., 2021). Sequencing was performed on a NextSeq 500 instrument with SR75 High Output Kit (Illumina). Seventy-six-base-pair single-end reads were generated. FastQ files were processed based on the workflow outlined in the Lexogen’s user guide for QuantSeq 3’ mRNA-Seq Integrated Data Analysis (Version 015UG108V0310).
2.11 Differential gene expression analysis
Gene read count files were imported into RStudio (R v.4.2.0). Differential expression analysis was performed using DESeq2 v.1.36.0 (Love et al., 2014). When body weight was used as a covariate, the weights were first scaled with the scale function from the base R package. Then, the DESeq data set was created using the design argument ∼ Weight + Condition where Weight is the scaled body weight and Condition is the genotype as indicated before. Only genes with total read counts greater than or equal to 5 were included in the model fitting. Log-fold change shrinkage was employed using the lfcShrink function as previously described (Zhu et al., 2018). Differential expressed genes were considered to be genes with an FDR-adjusted (Benjamini-Hochberg) p-value ≤ 0.05.
2.12 Immunofluorescence
Frozen brains embedded in OCT were coronally sectioned in 30 μm increments, rinsed with PBS, and mounted onto glass slides. Slides were blocked with 2.5% normal goat serum in PBS for 1 h, followed by overnight incubation with Iba1 antibody (1:1,000, Abcam, ab178846) at 4°C. Slides were then incubated with goat anti-rabbit secondary (1:500, Alexa Fluor 568, A11036) for 1.5 h. Slides were then mounted with coverslips using Vectashield Mounting Medium with DAPI (H-1200).
2D images were then acquired with a Leica DMi8 fluorescent microscope at 100× magnification. Images were analyzed by in-house pipelines developed using CellProfiler (Broad Institute) and ImageJ (Schneider et al., 2012; Stirling et al., 2021). For each mouse, three sections from differing anatomical coordinates (separated by 720 μm) were analyzed and the average value from these sections was used. Iba1+ signal and DAPI signal were measured and normalized by the total area of the section. Using CellProfiler, both Iba1+ cell number, cell size, and Iba+ signal area were quantified, as described previously (Valdearcos et al., 2017; Karahan et al., 2021).
2.13 Statistical analysis
Statistics were performed with GraphPad Prism 9 software. Comparisons between Abi3+/+ and Abi3–/–were performed using unpaired t-tests. For GTT and ITT, two-way ANOVA with Tukey’s post-hoc test was utilized for the time-course graphs. The energy expenditure vs. weight graphs were analyzed using simple linear regression. Data were represented as means ± SEM.
3 Results
3.1 Increase in body weight and fat mass and impairment of glucose and insulin tolerance in Abi3–/– mice
In the course of our AD project, we unexpectedly found that deletion of the Abi3 gene locus resulted in a dramatic obese phenotype in non-transgenic mice. Because of this unexpected nature, our investigation into the role of ABI3 in metabolism began with already aged mice (15 months). First, we measured the body weight of the Abi3+/+ and Abi3–/– mice (Figure 1A). Deletion of the Abi3 gene locus significantly increased body weight, with Abi3–/– mice weighing an average of 10.70 g, or 32.4%, more than Abi3+/+ mice (Figure 1A). Next, to determine whether this increase in weight was due to increased fat and/or lean mass, we performed EchoMRI body composition analysis on these mice (Figures 1B, C). Similar to body weight, fat mass was significantly increased in Abi3–/– mice. Specifically, Abi3–/– mice had more fat mass than Abi3+/+ mice by an average of 7.11 g, or 208.4% (Figure 1B). Lean mass, however, was not significantly altered in Abi3–/– mice (Figure 1C).
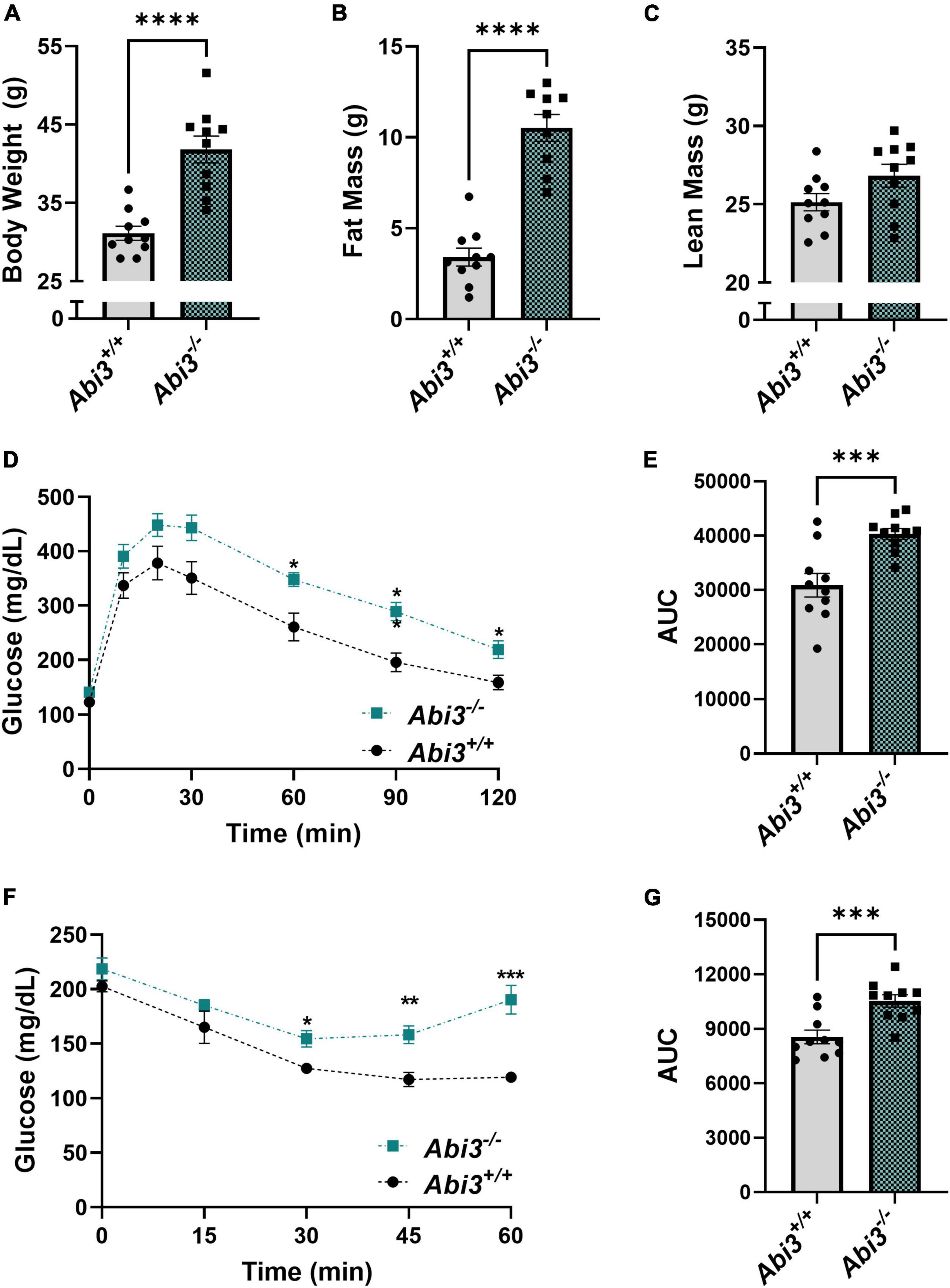
Figure 1. Abi3–/– mice exhibit increased body weight and fat mass and impaired glucose tolerance and insulin sensitivity. (A) Body weight was measured in 15-month-old male Abi3+/+ (n = 10) and Abi3–/– mice (n = 10). (B,C) Body composition was measured in these mice using EchoMRI. (D–G) Glucose (GTT) and Insulin tolerance tests (ITT) were performed, and the glucose levels of 15-month-old male Abi3+/+ (n = 10) and Abi3–/– mice (n = 10) were recorded over time. (D) In the GTT, mice were injected with 1 g/kg glucose following a 16 h fast. Blood glucose (mg/dL) was measured via glucometer at 30, 60, 90, and 120 min post injection. (E) The area under the curve for the GTT was calculated. (F) In the ITT, mice were injected with 0.75 units/kg insulin following a 2 h fast. Blood glucose (mg/dL) was measured via glucometer at 15, 30, 45, and 60 min post injection. (G) The area under the curve for ITT was calculated. Data represent mean ± SEM. For GTT and ITT time-course, 2-way ANOVA with Tukey’s post-hoc test was used. For all other comparisons, unpaired t-test was used; *p < 0.05, **p < 0.01, ***p < 0.001, and ****p < 0.0001.
To further understand the impact of the loss of ABI3 function on systemic glucose regulation, we performed glucose tolerance (GTT) and insulin tolerance (ITT) tests (Figures 1D–G). In the GTT, Abi3–/– mice exhibited significantly elevated blood glucose levels as compared to Abi3+/+ mice at 60, 90, and 120 min following glucose administration (Figure 1D). Similarly, area under the curve analysis of GTT data revealed that Abi3–/– mice had significantly greater area than Abi3+/+ mice (Figure 1E). These data indicate that Abi3–/– mice had impaired glucose tolerance relative to control. In the ITT, Abi3–/– mice had significantly elevated blood glucose levels at 30, 45, and 60 min, indicative of insulin resistance, as compared to Abi3+/+ mice (Figure 1F). Area under the curve analysis of ITT data revealed that Abi3–/– mice had significantly greater area than Abi3+/+ mice, indicating that Abi3–/– mice had impaired insulin response relative to control (Figure 1G).
3.2 Energy expenditure during light cycle is impaired, while food intake is unchanged, in Abi3–/– mice
To determine if the obese phenotype was secondary to differences in food intake, energy expenditure, or a combination of these factors, we performed comprehensive metabolic phenotyping and indirect calorimetry, via metabolic cage, on the Abi3+/+ and Abi3–/– mice (Figure 2). First, we measured the food consumed during the 84-h testing period within the Phenomaster system. The deletion of Abi3 gene locus did not alter food intake between groups (Figures 2A, B). Next, we evaluated energy expenditure from the calorimetry experiment during the light, dark, and combined full day cycles. There was no gross change in average energy expenditure between the genotypes (Figures 2C, D). However, further regression analysis comparing the relationship of energy expenditure vs. weight revealed that Abi3–/– mice had significantly lower slope than Abi3+/+ mice during the light cycle, indicative of impaired energy expenditure (Figure 2E). No significant differences in slope were detected between Abi3–/– and Abi3+/+ mice for the dark cycle or full day regression analyses (Figures 2F, G). Additionally, no differences in respiratory exchange ratio, a measure of carbohydrate vs. lipid fuel utilization, were observed between the Abi3+/+ and Abi3–/– mice (Supplementary Figure 1B). Finally, to determine if differences in energy expenditure were secondary to changes in overall movement, we evaluated the total and average movement of the Abi3+/+ and Abi3–/– mice during the calorimetry experiment (Supplementary Figure 2). Abi3 genotype status had no impact on total or average movement counts (Supplementary Figure 2C).
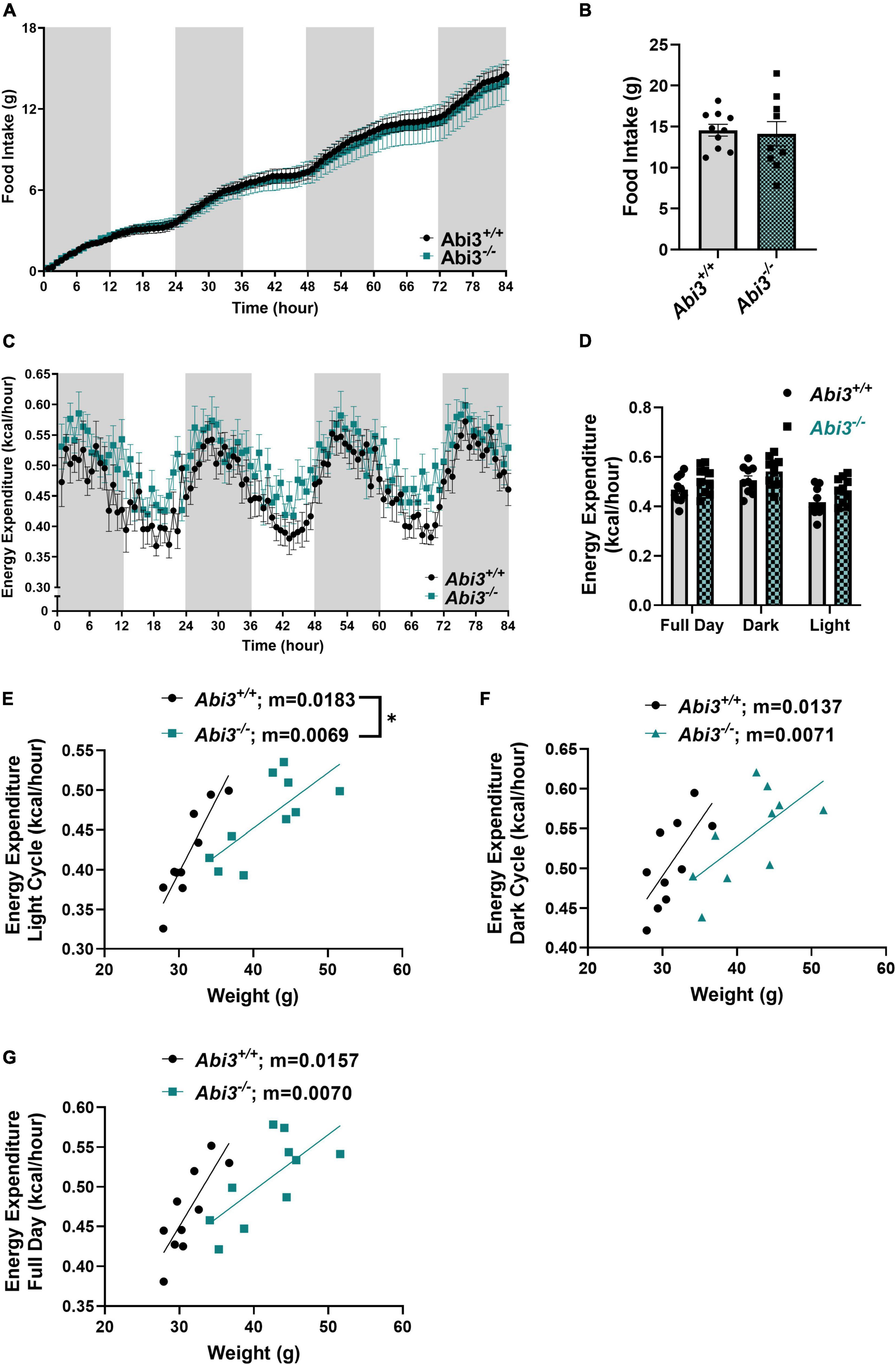
Figure 2. Abi3– /– mice exhibit impaired energy expenditure and unaltered food intake. 15-month-old male Abi3+/+ (n = 10) and Abi3– /– mice (n = 10) were singly housed in metabolic cages and (A,B) food intake and (C,D) energy expenditure were recorded over 84 h. For time course graphs (A,C), the shaded portions represent night hours (dark cycle) and the unshaded portions represent daytime (light cycle). (A) Food intake over time and (B) overall total food intake were recorded. (C) Energy expenditure over time and (D) average energy expenditure were measured. (D) Average energy expenditure was calculated for the daytime/light hours (light cycle), night/dark hours (dark cycle), and the entire 84 h (full day). (E–G) Subsequent linear regression analysis of average energy expenditure vs. weight was performed to assess the relationship between lean mass and energy expenditure. Regressions were performed for (E) light cycle, (F) dark cycle, and (G) full day. Data represent mean ± SEM. Unpaired t-test, simple linear regression; *p < 0.05.
3.3 Total cholesterol and leptin concentration are increased in the plasma of Abi3–/– mice
In order to further evaluate the metabolic consequences observed in Abi3–/– mice, we performed cholesterol and leptin measurements on the plasma from Abi3+/+ and Abi3–/– mice. Using a colorimetric total cholesterol assay, we found that Abi3–/– mice had significantly elevated cholesterol levels (Supplementary Figure 3). Then, we performed a leptin ELISA on the plasma from these mice and similarly observed that Abi3–/– mice had significantly elevated leptin concentration (Supplementary Figure 3). Elevated leptin and cholesterol levels are characteristic of obesity, and therefore these results further demonstrate the extent of the obese phenotype observed in Abi3–/– mice (Klop et al., 2013; Obradovic et al., 2021).
3.4 Abi3 locus deletion alters the transcriptome, independent of differences in weight, in the hypothalamus but not in brown adipose tissue
To gain insight into the potential pathways that are regulated by Abi3 gene locus deletion, we evaluated the gene expression profile of tissues central to body weight and energy expenditure regulation by performing bulk RNA-seq on the hypothalami and brown adipose tissue of Abi3+/+ and Abi3–/– mice. To isolate changes that were due to the absence of Abi3 itself, rather than secondary to Abi3 dependent differences in body weight, we performed weight-covariate controlled analysis of the RNA-seq data. For our analysis, differentially expressed genes (DEGs) were defined by a cut off of adjusted p-values less than 0.05. Within the brown adipose tissue, no DEGs were identified between Abi3–/– vs. Abi3+/+ mice (Supplementary Figure 4). Within the hypothalamus, however, 31 weight covariate controlled DEGs were identified, including Shank1 and Cartpt (Figure 3A).
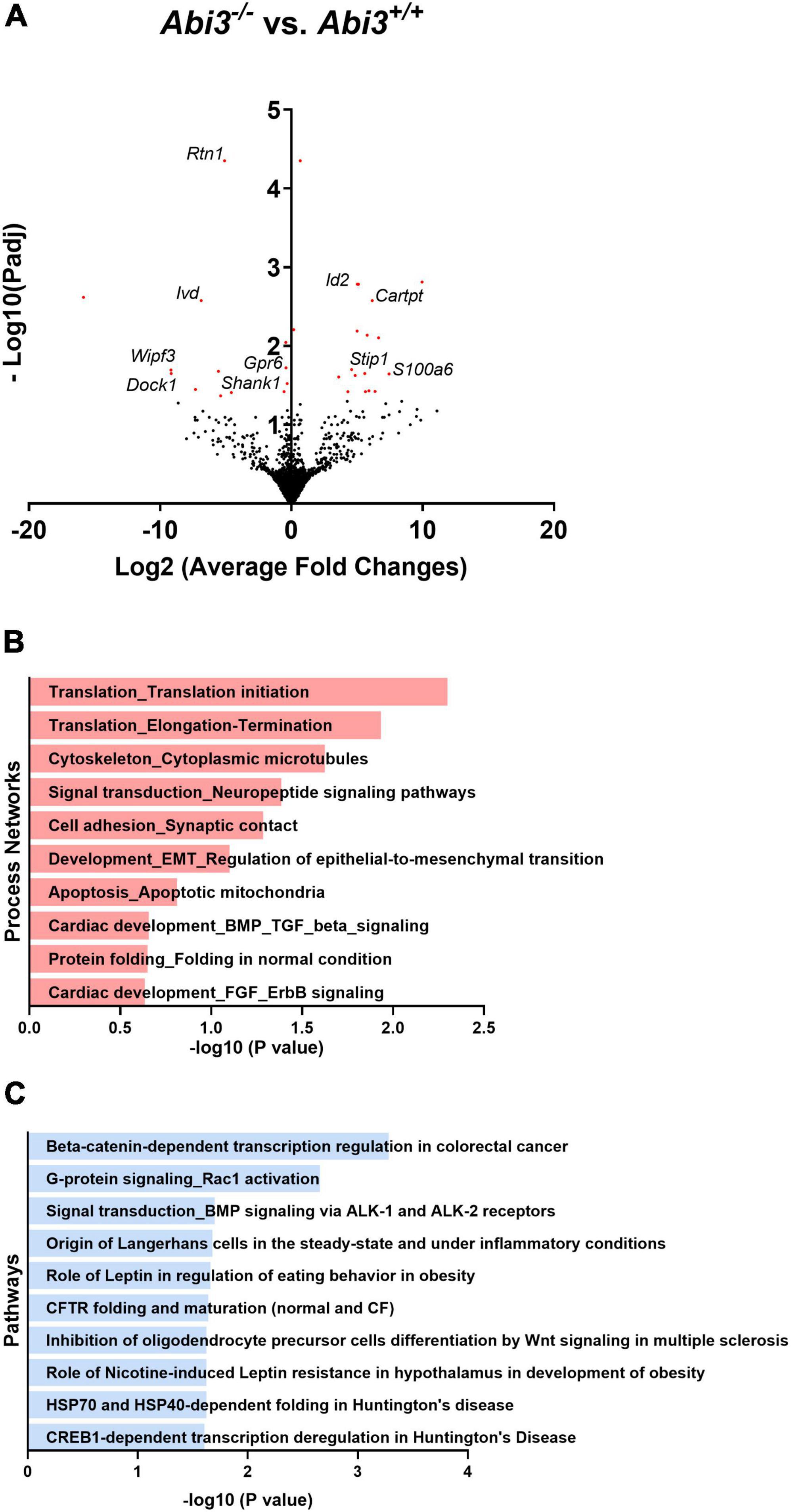
Figure 3. Deletion of Abi3 gene locus, independent of differences in body weight, alters the transcriptome within the hypothalamus. Bulk RNA sequencing was performed with the hypothalami of 15-month-old male Abi3+/+ (n = 4) and Abi3– /– mice (n = 4). Differentially expressed genes (DEGs), defined by an adjusted p-value < 0.05, were then identified. (A) Volcano plot demonstrating the DEGs from the hypothalami of Abi3– /– vs. Abi3+/+mice. The red points represent differentially expressed genes. (B,C) The DEGs were used to perform (B) process network and (C) pathway analysis using the Metacore software. The significantly enriched (B) process networks and (C) pathways are listed. For additional information on (B,C) the Metacore figures, see Supplementary Table 1.
To determine the potential biologic relevance of these DEGs identified within the hypothalamus, we performed secondary process network and pathway analyses using the Metacore software. The DEGs identified in Abi3–/– mice were significantly enriched in biologic processes including cytoskeletal regulation, synaptic cellular adhesion, and neuropeptide signaling, among others (Figure 3B). Further, these DEGs were significantly enriched in pathways including G-protein/Rac1 signaling and leptin regulation, among others (Figure 3C).
3.5 Microglia number and area were decreased in the mediobasal hypothalamus, but not other brain areas in Abi3–/– mice
Because Abi3 is predominantly expressed by microglia within the CNS, we performed Iba1 immunofluorescence on brain sections from Abi3–/– and Abi3+/+ mice. Specifically, we assessed the changes in microglia number and area throughout multiple CNS regions (Figure 4). The total number or area of Iba1+ cells were not altered in the cortex or hippocampus of Abi3–/– mice (Figures 4A–D). Iba1+ cell size was increased only in the hippocampus, but not other brain regions of Abi3–/– mice (Supplementary Figure 5). Further, there was no significant difference in Iba1+ cell number or area when evaluating the entire hypothalamus (Figures 4E, F). However, within the mediobasal subregion of the hypothalamus, a region critical to the regulation of energy balance, Abi3–/– mice showed significantly lower number and area of Iba1+ cells (Figures 4G, H). The mediobasal hypothalamus is an area that is central to the regulation of systemic metabolism, and it is postulated that microglia play a key role in that regulation. Therefore, the change in microglia number could indicate that the loss of Abi3 might induce obesity through altered microglia function within the mediobasal hypothalamus.
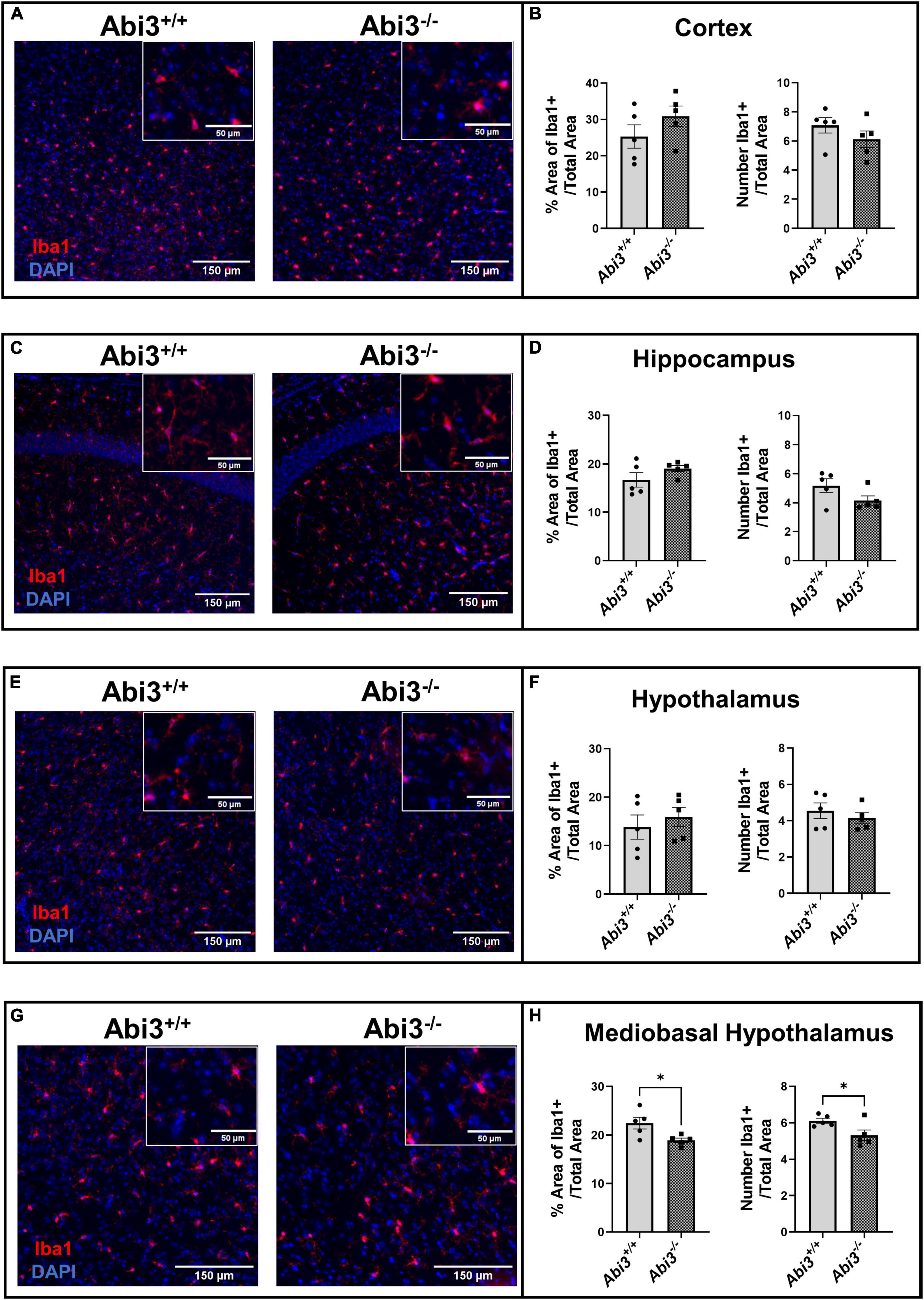
Figure 4. Deletion of Abi3 gene locus reduces microglia number and area within the mediobasal hypothalamus, but not other brain regions. Coronal brain sections from 15-month-old male Abi3+/+ (n = 5) and Abi3– /– mice (n = 5) were stained with Iba1+ (microglia marker) and DAPI (nuclear counterstain). (A,C,E,G) Representative images, for each brain region, show Iba1 + signal in red and DAPI in blue. (B,D,F,H) The number of microglia and area occupied by microglia were quantified across these brain regions. The area and number of Iba1 + signal was measured in the (A,B) cortex, (C,D) hippocampus, (E,F) whole hypothalamus, and (G,H) mediobasal hypothalamus. Data represent mean ± SEM. Unpaired t-test; *p < 0.05.
4 Discussion
In this report, we demonstrated that Abi3–/– mice had significantly elevated body and fat mass, and reduced energy expenditure. Importantly, these mice had reduced microglia number and area within the mediobasal hypothalamus, the brain region critical to the regulation of energy balance. Altogether, our data suggest that the functions of ABI3 are not only important in the response to neurodegenerative pathology (Karahan et al., 2021) but also in the regulation of systemic metabolism.
Due to the unexpected nature of our initial discovery regarding the impact of Abi3 deletion on body weight, our original observation of the obese phenotype did not occur until the mice were 15 months old. In these mice, we found that body weight and fat mass were significantly increased in Abi3–/– mice, while lean mass was not altered. These findings indicated that the increased weight was driven predominantly by increased fat mass, which is indicative of an obese phenotype. Furthermore, we found that Abi3–/– mice had impairments in both glucose tolerance and insulin sensitivity. This likely suggests that the obesity in Abi3–/– mice induced impairments to systemic glucose regulation, as is typically observed in obese mice (King, 2012). Additionally, as these findings were observed in aged mice, it is possible that the obese phenotype is an age dependent phenomenon. Future study can help elucidate whether deletion of Abi3 at earlier time points induces similar metabolic effects. Additionally, only male mice were available for this investigation because female mice were already used for another project. Therefore, future studies will be necessary to determine the potential impact of sex dependent differences on the obese phenotype.
We further investigated whether the difference in weight was driven by increased food intake, decreased energy expenditure, or a combination of both. The food intake and the overall movement of the mice did not change between the genotypes. However, Abi3–/– mice had significantly reduced slope on the regression of energy expenditure vs. body weight during the light cycle but not the dark cycle. This indicates that Abi3–/– mice have reduced energy expenditure during their more inactive hours relative to Abi3+/+ mice. Overall, these findings suggest that the loss of ABI3 function may drive obesity through impairments to energy expenditure. It is important, however, to note that these findings were observed in mice that were already obese. Therefore, it is possible that there might be additional mechanisms driving the obese phenotype in Abi3–/– mice. Critically, however, the regression analysis addressed the potential confounding effects of differing body weight (Tschöp et al., 2011), thus isolating the direct effect of the deletion of Abi3 on energy expenditure.
Energy expenditure in mice is predominantly regulated by the hypothalamus, centrally, and largely by thermogenic brown adipose tissue (BAT), peripherally (Varela and Horvath, 2012; Harms and Seale, 2013; Schneeberger et al., 2014). Since we observed that Abi3–/–mice exhibited impaired energy expenditure, we performed bulk RNA-seq on these tissues from Abi3+/+ and Abi3–/– mice. In an attempt to isolate the direct effect of Abi3 locus deletion on gene expression within these tissues, we performed a weight-covariate controlled analysis. This approach did not identify any DEGs within the BAT but did identify DEGs within the hypothalamus. This suggests that the deletion of Abi3 gene locus itself, as opposed to Abi3 dependent differences in body weight, induced changes to gene expression within the hypothalamus but not within BAT. This could suggest that hypothalamus, not BAT, triggered the initial dysfunction that subsequently drives the impairment to energy expenditure. Furthermore, our pathway analysis indicated that the hypothalamic DEGs were enriched in biologic processes including cellular adhesion at the synapse, energy balance related neuropeptide signaling (Cartpt) and cytoskeletal remodeling (Shank1). The enrichment of the cytoskeletal related processes matches with the previously reported functions of ABI3. Specifically, ABI3 has been reported to be participant in cellular processes that require remodeling of the actin cytoskeleton, including cellular migration and membrane protrusion (Sekino et al., 2015; Karahan et al., 2021). As Abi3 is predominantly expressed by microglia within the CNS, our transcriptomic analyses suggests that the deletion of Abi3 is impacting the function of microglia within the hypothalamus. Further, microglia are highly dynamic cells that are constantly surveying their microenvironment and communicating with other CNS cells, both of which are functions that require cytoskeletal remodeling (Uhlemann et al., 2016; Prinz et al., 2019). Therefore, these sequencing data might point toward impaired cytoskeletal remodeling, and subsequently an impaired ability of microglia to perform their homeostatic functions, as a contributing factor to the induction of the obese phenotype. However, future investigation will be required to comprehensively test this hypothesis.
Importantly, altered microglia function within the hypothalamus has been connected to the maintenance of body weight and obesity (Valdearcos et al., 2014, 2017; Andre et al., 2017; Dorfman et al., 2017; Gao et al., 2017; Rosin et al., 2018; De Luca et al., 2019; Kim et al., 2019; Campolim et al., 2020). Multiple studies have demonstrated that microglia number increases within the mediobasal hypothalamus during diet induced obesity (Thaler et al., 2012; de Kloet et al., 2014; Valdearcos et al., 2014; Schur et al., 2015; Waise et al., 2015; Baufeld et al., 2016; Guillemot-Legris et al., 2016). Further, it has been demonstrated that reducing this dietary induced microgliosis can mitigate weight gain (Andre et al., 2017; Valdearcos et al., 2017; Kim et al., 2019). It has also been reported that genetic or pharmacologic treatments that increase microgliosis within the mediobasal hypothalamus are capable of inducing obesity even in the absence of dietary challenge (Valdearcos et al., 2017; Campolim et al., 2020). Conversely, a recent study demonstrated that by deleting a gene specifically within microglia, the number of microglia within the mediobasal hypothalamus decreased but diet induced obesity was exacerbated (Gao et al., 2017). Given the importance of microglia within the mediobasal hypothalamus to obesity, in conjunction with our sequencing analysis, we evaluated whether Abi3–/– mice had altered microglia number within the mediobasal hypothalamus or any other CNS region. Intriguingly, we found that microglia number and area were decreased specifically within the mediobasal hypothalamus, but not in other areas, of Abi3–/– mice compared to Abi3+/+. We are not the first to report a decrease in the number of mediobasal hypothalamic microglia in the setting of impaired body weight regulation; however, to the best of our knowledge, we are the first to report such findings in the absence of dietary intervention.
Our data contribute to the growing concept that an appropriate level of microglia activity within the hypothalamus is necessary to maintain proper control of energy balance (Valdearcos et al., 2019). It appears that both hyperreactivity and the reduced reactivity of hypothalamic microglia may lead to dysfunctional regulation of systemic metabolism. Intriguingly, a similar paradigm regarding microglia activity has become well-established in the neurodegenerative disease field (Hickman et al., 2018). Currently, however, little is known about the exact cellular mechanisms by which hypothalamic microglia modulate energy balance. It has been postulated that microglia regulate control of energy balance via the modulation of neuronal signaling within the hypothalamus through microglia-neuron interactions (Folick et al., 2022). Recent studies have shown that both increased and decreased microglial inflammatory activity within the mediobasal hypothalamus can disrupt leptin/melanocortin related neuronal signaling, a pathway that is central to the regulation of energy balance (Gao et al., 2017; Valdearcos et al., 2017; Kim et al., 2019). Further, it has been suggested that microglia may directly alter the organization of melanocortin system neuronal synapses (Horvath et al., 2010; Kim et al., 2019).
In the context of this study, it is possible that the deletion of the Abi3 gene locus impaired the ability of microglia to perform the complex functions that are necessary to regulate energy balance (Valdearcos et al., 2019; De Luca et al., 2020). This possibility seems feasible when considering the importance of dynamic cytoskeletal remodeling for microglia to perform their basic functions (migration, surveillance, phagocytosis, etc.), along with the reported importance of ABI3 in processes that require cytoskeletal remodeling such as migration and phagocytosis (Sekino et al., 2015; Karahan et al., 2021). However, future study will be necessary to uncover the specific functional impairment in microglia that led to impaired regulation of energy balance in Abi3–/– mice. Additionally, while our data are suggestive of a CNS driven disruption of energy balance, it remains possible that peripherally driven dysfunction could partly underly the obese phenotype. Future studies could utilize microglial specific inducible knock-out of Abi3 to address this limitation more definitely.
Overall, this report provides a novel, initial exploration into the role of Abi3 in the regulation of systemic metabolism, opening the door to future investigation aimed at uncovering how the manipulation of ABI3-related function can modulate metabolic disease states. Furthermore, our study has now demonstrated how deletion of Abi3 gene locus can have dramatic impacts on the seemingly distinct disease states of obesity and neurodegeneration, likely due to disruptions in microglia functions (Karahan et al., 2021). Together, our study helps to address the critical knowledge gap regarding the specific molecular drivers that may jointly underly the microglia dysfunction observed in metabolic and neurodegenerative disease.
Data availability statement
The datasets presented in this study can be found in online repositories. The names of the repository/repositories and accession number(s) can be found below: https://www.ncbi.nlm.nih.gov/geo/, GSE212299.
Ethics statement
The animal study was reviewed and approved by the IUPUI Animal Care and Use Committee Indiana University School of Medicine at Indianapolis.
Author contributions
DS, HK, and JK created the overall study design and interpreted the findings. DS, HK, BM, and YM performed the experiments. DS performed the metabolic cage data analysis. HW and HK performed the bioinformatic analyses. DS and MA-A analyzed the immunofluorescence data. DS, HK, HW, and JK wrote the manuscript. All authors contributed to the article and approved the submitted version.
Funding
We thank the following sources of funding for supporting this work: National Institutes of Health (NIH) grants-Pilot and Feasibility Award within the CDMD NIH/NIDDK P30 DK097512 (JK), R01AG071281 (JK), RF1AG074543 (JK), and R21AG072738 (JK) and NIH Predoctoral Fellowship-provided through T32DK064466-20 (DS), T32AG071444 (HW), and F30AG079580 (HW).
Acknowledgments
We thank the Center for Diabetes and Metabolic Disease for their assistance with the glucose tolerance and insulin tolerance tests. We also thank the Indiana Biosciences Research Institute for their assistance with the metabolic phenotyping/calorimetry experiments.
Conflict of interest
The authors declare that the research was conducted in the absence of any commercial or financial relationships that could be construed as a potential conflict of interest.
Publisher’s note
All claims expressed in this article are solely those of the authors and do not necessarily represent those of their affiliated organizations, or those of the publisher, the editors and the reviewers. Any product that may be evaluated in this article, or claim that may be made by its manufacturer, is not guaranteed or endorsed by the publisher.
Supplementary material
The Supplementary Material for this article can be found online at: https://www.frontiersin.org/articles/10.3389/fnagi.2022.1035572/full#supplementary-material
References
Andre, C., Guzman-Quevedo, O., Rey, C., Remus-Borel, J., Clark, S., Castellanos-Jankiewicz, A., et al. (2017). Inhibiting microglia expansion prevents diet-induced hypothalamic and peripheral inflammation. Diabetes 66, 908–919. doi: 10.2337/db16-0586
Baufeld, C., Osterloh, A., Prokop, S., Miller, K. R., and Heppner, F. L. (2016). High-fat diet-induced brain region-specific phenotypic spectrum of CNS resident microglia. Acta Neuropathol. 132, 361–375. doi: 10.1007/s00401-016-1595-4
Campolim, C. M., Weissmann, L., Ferreira, C. K. O., Zordão, O. P., Dornellas, A. P. S., de Castro, G., et al. (2020). Short-term exposure to air pollution (PM2.5) induces hypothalamic inflammation, and long-term leads to leptin resistance and obesity via Tlr4/Ikbke in mice. Sci. Rep. 10:10160. doi: 10.1038/s41598-020-67040-3
Coats, B. R., Schoenfelt, K. Q., Barbosa-Lorenzi, V. C., Peris, E., Cui, C., Hoffman, A., et al. (2017). Metabolically activated adipose tissue macrophages perform detrimental and beneficial functions during diet-induced obesity. Cell Rep. 20, 3149–3161. doi: 10.1016/j.celrep.2017.08.096
de Kloet, A. D., Pioquinto, D. J., Nguyen, D., Wang, L., Smith, J. A., Hiller, H., et al. (2014). Obesity induces neuroinflammation mediated by altered expression of the renin-angiotensin system in mouse forebrain nuclei. Physiol. Behav. 136, 31–38. doi: 10.1016/j.physbeh.2014.01.016
De Luca, S. N., Miller, A. A., Sominsky, L., and Spencer, S. J. (2020). Microglial regulation of satiety and cognition. J. Neuroendocrinol. 32:e12838. doi: 10.1111/jne.12838
De Luca, S. N., Sominsky, L., Soch, A., Wang, H., Ziko, I., Rank, M. M., et al. (2019). Conditional microglial depletion in rats leads to reversible anorexia and weight loss by disrupting gustatory circuitry. Brain Behav. Immun. 77, 77–91. doi: 10.1016/j.bbi.2018.12.008
Dorfman, M. D., Krull, J. E., Douglass, J. D., Fasnacht, R., Lara-Lince, F., Meek, T. H., et al. (2017). Sex differences in microglial CX3CR1 signalling determine obesity susceptibility in mice. Nat. Commun. 8:14556. doi: 10.1038/ncomms14556
Folick, A., Cheang, R. T., Valdearcos, M., and Koliwad, S. K. (2022). Metabolic factors in the regulation of hypothalamic innate immune responses in obesity. Exp. Mol. Med. 54, 393–402. doi: 10.1038/s12276-021-00666-z
Gao, Y., Vidal-Itriago, A., Kalsbeek, M. J., Layritz, C., García-Cáceres, C., Tom, R. Z., et al. (2017). Lipoprotein lipase maintains microglial innate immunity in obesity. Cell Rep. 20, 3034–3042. doi: 10.1016/j.celrep.2017.09.008
Guillemot-Legris, O., Masquelier, J., Everard, A., Cani, P. D., Alhouayek, M., and Muccioli, G. G. (2016). High-fat diet feeding differentially affects the development of inflammation in the central nervous system. J. Neuroinflammation 13, 206–206. doi: 10.1186/s12974-016-0666-8
Han, X., Wang, R., Zhou, Y., Fei, L., Sun, H., Lai, S., et al. (2018). Mapping the mouse cell atlas by microwell-Seq. Cell 172, 1091–1107.e17. doi: 10.1016/j.cell.2018.02.001
Harms, M., and Seale, P. (2013). Brown and beige fat: Development, function and therapeutic potential. Nat. Med. 19, 1252–1263. doi: 10.1038/nm.3361
Hickman, S., Izzy, S., Sen, P., Morsett, L., and El Khoury, J. (2018). Microglia in neurodegeneration. Nat. Neurosci. 21, 1359–1369. doi: 10.1038/s41593-018-0242-x
Hill, D. A., Lim, H. W., Kim, Y. H., Ho, W. Y., Foong, Y. H., Nelson, V. L., et al. (2018). Distinct macrophage populations direct inflammatory versus physiological changes in adipose tissue. Proc. Natl. Acad. Sci. U.S.A. 115, E5096–E5105. doi: 10.1073/pnas.1802611115
Horvath, T. L., Sarman, B., García-Cáceres, C., Enriori, P. J., Sotonyi, P., Shanabrough, M., et al. (2010). Synaptic input organization of the melanocortin system predicts diet-induced hypothalamic reactive gliosis and obesity. Proc. Natl. Acad. Sci. U.S.A. 107, 14875–14880. doi: 10.1073/pnas.1004282107
Karahan, H., Smith, D. C., Kim, B., Dabin, L. C., Al-Amin, M. M., Wijeratne, H. R. S., et al. (2021). Deletion of Abi3 gene locus exacerbates neuropathological features of Alzheimer’s disease in a mouse model of Aβ amyloidosis. Sci. Adv. 7:eabe3954. doi: 10.1126/sciadv.abe3954
Kim, J. D., Yoon, N. A., Jin, S., and Diano, S. (2019). Microglial UCP2 mediates inflammation and obesity induced by high-fat feeding. Cell Metab. 30, 952–962.e5. doi: 10.1016/j.cmet.2019.08.010
King, A. J. F. (2012). The use of animal models in diabetes research. Br. J. Pharmacol. 166, 877–894. doi: 10.1111/j.1476-5381.2012.01911.x
Klop, B., Elte, J. W., and Cabezas, M. C. (2013). Dyslipidemia in obesity: Mechanisms and potential targets. Nutrients 5, 1218–1240. doi: 10.3390/nu5041218
Love, M. I., Huber, W., and Anders, S. (2014). Moderated estimation of fold change and dispersion for RNA-seq data with DESeq2. Genome Biol. 15:550. doi: 10.1186/s13059-014-0550-8
Lumeng, C. N., and Saltiel, A. R. (2011). Inflammatory links between obesity and metabolic disease. J. Clin. Invest. 121, 2111–2117. doi: 10.1172/JCI57132
Mazon, J. N., de Mello, A. H., Ferreira, G. K., and Rezin, G. T. (2017). The impact of obesity on neurodegenerative diseases. Life Sci. 182, 22–28. doi: 10.1016/j.lfs.2017.06.002
Moraes, L., Zanchin, N. I. T., and Cerutti, J. M. (2017). ABI3, a component of the WAVE2 complex, is potentially regulated by PI3K/AKT pathway. Oncotarget 8, 67769–67781. doi: 10.18632/oncotarget.18840
Newcombe, E. A., Camats-Perna, J., Silva, M. L., Valmas, N., Huat, T. J., and Medeiros, R. (2018). Inflammation: The link between comorbidities, genetics, and Alzheimer’s disease. J. Neuroinflammation 15:276. doi: 10.1186/s12974-018-1313-3
Obradovic, M., Sudar-Milovanovic, E., Soskic, S., Essack, M., Arya, S., Stewart, A. J., et al. (2021). Leptin and Obesity: Role and clinical implication. Front. Endocrinol. 12:585887. doi: 10.3389/fendo.2021.585887
Prinz, M., Jung, S., and Priller, J. (2019). Microglia biology: One century of evolving concepts. Cell 179, 292–311. doi: 10.1016/j.cell.2019.08.053
Pugazhenthi, S., Qin, L., and Reddy, P. H. (2017). Common neurodegenerative pathways in obesity, diabetes, and Alzheimer’s disease. Biochim. Biophys. Acta Mol. Basis Dis. 1863, 1037–1045. doi: 10.1016/j.bbadis.2016.04.017
Ransohoff, R. M. (2016). How neuroinflammation contributes to neurodegeneration. Science 353, 777–783. doi: 10.1126/science.aag2590
Reilly, S. M., and Saltiel, A. R. (2017). Adapting to obesity with adipose tissue inflammation. Nat. Rev. Endocrinol. 13, 633–643. doi: 10.1038/nrendo.2017.90
Rosin, J. M., Vora, S. R., and Kurrasch, D. M. (2018). Depletion of embryonic microglia using the CSF1R inhibitor PLX5622 has adverse sex-specific effects on mice, including accelerated weight gain, hyperactivity and anxiolytic-like behaviour. Brain Behav. Immun. 73, 682–697. doi: 10.1016/j.bbi.2018.07.023
Schneeberger, M., Gomis, R., and Claret, M. (2014). Hypothalamic and brainstem neuronal circuits controlling homeostatic energy balance. J. Endocrinol. 220, T25–T46. doi: 10.1530/JOE-13-0398
Schneider, C. A., Rasband, W. S., and Eliceiri, K. W. (2012). NIH Image to ImageJ: 25 years of image analysis. Nat. Methods 9, 671–675. doi: 10.1038/nmeth.2089
Schur, E. A., Melhorn, S. J., Oh, S.-K., Lacy, J. M., Berkseth, K. E., Guyenet, S. J., et al. (2015). Radiologic evidence that hypothalamic gliosis is associated with obesity and insulin resistance in humans. Obesity (Silver Spring) 23, 2142–2148. doi: 10.1002/oby.21248
Sekino, S., Kashiwagi, Y., Kanazawa, H., Takada, K., Baba, T., Sato, S., et al. (2015). The NESH/Abi-3-based WAVE2 complex is functionally distinct from the Abi-1-based WAVE2 complex. Cell Commun. Signal. 13:41. doi: 10.1186/s12964-015-0119-5
Sims, R., van der Lee, S. J., Naj, A. C., Bellenguez, C., Badarinarayan, N., Jakobsdottir, J., et al. (2017). Rare coding variants in PLCG2, ABI3, and TREM2 implicate microglial-mediated innate immunity in Alzheimer’s disease. Nat. Genet. 49, 1373–1384. doi: 10.1038/ng.3916
Stirling, D. R., Swain-Bowden, M. J., Lucas, A. M., Carpenter, A. E., Cimini, B. A., and Goodman, A. (2021). CellProfiler 4: Improvements in speed, utility and usability. BMC Bioinformatics 22:433. doi: 10.1186/s12859-021-04344-9
Takenawa, T., and Suetsugu, S. (2007). The WASP-WAVE protein network: Connecting the membrane to the cytoskeleton. Nat. Rev. Mol. Cell Biol. 8, 37–48. doi: 10.1038/nrm2069
Thaler, J. P., Yi, C.-X., Schur, E. A., Guyenet, S. J., Hwang, B. H., Dietrich, M. O., et al. (2012). Obesity is associated with hypothalamic injury in rodents and humans. J. Clin. Invest. 122, 153–162. doi: 10.1172/JCI59660
Tschöp, M. H., Speakman, J. R., Arch, J. R. S., Auwerx, J., Brüning, J. C., Chan, L., et al. (2011). A guide to analysis of mouse energy metabolism. Nat. Methods 9, 57–63. doi: 10.1038/nmeth.1806
Uhlemann, R., Gertz, K., Boehmerle, W., Schwarz, T., Nolte, C., Freyer, D., et al. (2016). Actin dynamics shape microglia effector functions. Brain Struct. Funct. 221, 2717–2734. doi: 10.1007/s00429-015-1067-y
Valdearcos, M., Douglass, J. D., Robblee, M. M., Dorfman, M. D., Stifler, D. R., Bennett, M. L., et al. (2017). Microglial inflammatory signaling orchestrates the hypothalamic immune response to dietary excess and mediates obesity susceptibility. Cell Metab. 26, 185–197.e3. doi: 10.1016/j.cmet.2017.05.015
Valdearcos, M., Myers, M. G., and Koliwad, S. K. (2019). Hypothalamic microglia as potential regulators of metabolic physiology. Nat. Metab. 1, 314–320. doi: 10.1038/s42255-019-0040-0
Valdearcos, M., Robblee, M. M., Benjamin, D. I., Nomura, D. K., Xu, A. W., and Koliwad, S. K. (2014). Microglia dictate the impact of saturated fat consumption on hypothalamic inflammation and neuronal function. Cell Rep. 9, 2124–2138. doi: 10.1016/j.celrep.2014.11.018
Varela, L., and Horvath, T. L. (2012). Leptin and insulin pathways in POMC and AgRP neurons that modulate energy balance and glucose homeostasis. EMBO Rep. 13, 1079–1086. doi: 10.1038/embor.2012.174
Waise, T. M. Z., Toshinai, K., Naznin, F., NamKoong, C., Md Moin, A. S., Sakoda, H., et al. (2015). One-day high-fat diet induces inflammation in the nodose ganglion and hypothalamus of mice. Biochem. Biophys. Res. Commun. 464, 1157–1162. doi: 10.1016/j.bbrc.2015.07.097
Zhang, Y., Chen, K., Sloan, S. A., Bennett, M. L., Scholze, A. R., O’Keeffe, S., et al. (2014). An RNA-sequencing transcriptome and splicing database of glia, neurons, and vascular cells of the cerebral cortex. J. Neurosci. 34, 11929–11947. doi: 10.1523/jneurosci.1860-14.2014
Zhu, A., Ibrahim, J. G., and Love, M. I. (2018). Heavy-tailed prior distributions for sequence count data: Removing the noise and preserving large differences. Bioinformatics 35, 2084–2092. doi: 10.1093/bioinformatics/bty895
Keywords: obesity, microglia, Abi3, hypothalamus, Alzheimer’s disease
Citation: Smith DC, Karahan H, Wijeratne HRS, Al-Amin M, McCord B, Moon Y and Kim J (2022) Deletion of the Alzheimer’s disease risk gene Abi3 locus results in obesity and systemic metabolic disruption in mice. Front. Aging Neurosci. 14:1035572. doi: 10.3389/fnagi.2022.1035572
Received: 02 September 2022; Accepted: 06 December 2022;
Published: 22 December 2022.
Edited by:
Shannon L. Macauley, Wake Forest University, United StatesReviewed by:
Angela Gomez-Arboledas, University of California, Irvine, United StatesGiedre Milinkeviciute, University of California, Irvine, United States
Peichang Wang, Capital Medical University, China
Copyright © 2022 Smith, Karahan, Wijeratne, Al-Amin, McCord, Moon and Kim. This is an open-access article distributed under the terms of the Creative Commons Attribution License (CC BY). The use, distribution or reproduction in other forums is permitted, provided the original author(s) and the copyright owner(s) are credited and that the original publication in this journal is cited, in accordance with accepted academic practice. No use, distribution or reproduction is permitted which does not comply with these terms.
*Correspondence: Jungsu Kim, ✉ amsxMjNAaXUuZWR1