- 1Department of Rehabilitation Medicine, The First Affiliated Hospital of Nanjing Medical University, Nanjing, China
- 2Department of Neurology, Jiuquan City People’s Hospital, Jiuquan, China
- 3Department of Rehabilitation Medicine, The First People’s Hospital of Lianyungang, Lianyungang, China
- 4Department of Rehabilitation Medicine, Cangzhou Central Hospital, Cangzhou, China
- 5Department of Neurology, Xingtai People’s Hospital, Xingtai, China
- 6Department of Rehabilitation Medicine, The First People’s Hospital of Yibin, Yibin, China
- 7Department of Rehabilitation Medicine, The Affiliated Jiangning Hospital of Nanjing Medical University, Nanjing, China
Introduction: Prediction of post-stroke functional outcome is important for personalized rehabilitation treatment, we aimed to develop an effective nomogram for predicting long-term unfavorable functional outcomes in ischemic stroke patients after acute phase.
Methods: We retrospectively analyzed clinical data, rehabilitation data, and longitudinal follow-up data from ischemic stroke patients who underwent early rehabilitation at multiple centers in China. An unfavorable functional outcome was defined as a modified Rankin Scale (mRS) score of 3–6 at 90 days after onset. Patients were randomly allocated to either a training or test cohort in a ratio of 4:1. Univariate and multivariate logistic regression analyses were used to identify the predictors for the development of a predictive nomogram. The area under the receiver operating characteristic curve (AUC) was used to evaluate predictive ability in both the training and test cohorts.
Results: A total of 856 patients (training cohort: n = 684; test cohort: n = 172) were included in this study. Among them, 518 patients experienced unfavorable outcomes 90 days after ischemic stroke. Trial of ORG 10172 in Acute Stroke Treatment classification (p = 0.024), antihypertensive agents use [odds ratio (OR) = 1.86; p = 0.041], 15-day Barthel Index score (OR = 0.930; p < 0.001) and 15-day mRS score (OR = 13.494; p < 0.001) were selected as predictors for the unfavorable outcome nomogram. The nomogram model showed good predictive performance in both the training (AUC = 0.950) and test cohorts (AUC = 0.942).
Conclusion: The constructed nomogram model could be a practical tool for predicting unfavorable functional outcomes in ischemic stroke patients underwent early rehabilitation after acute phase.
1. Introduction
Annual data has shown that of the 16 million people who suffer from stroke worldwide, 87% had an ischemic stroke. More than 6 million deaths or disabilities are attributable to stroke, resulting in heavy social, family, and economic burdens. Reduction of disabilities and improvement of the independence of stroke survivors have been the primary goals of rehabilitation (Langhorne et al., 2011). Early identification of individuals at risk of an unfavorable outcome is important for decision-making in clinical practice and could help improve the disease outcome (Ali-Ahmed et al., 2019; Diener and Hankey, 2020). Predictive factors are not only used to predict prognosis but also stratify patients for individualized treatment (Gravanis and Tsirka, 2008; Diener and Hankey, 2020; Lopatkiewicz et al., 2020).
Previous research on risk prediction of outcome in ischemic stroke patients relied mostly on demographic variables, physical examination variables, disease-related variables, and laboratory and imaging variables (Fahey et al., 2018). The most commonly used risk factors in the prediction of functional outcomes included age, baseline National Institutes of Health Stroke Scale (NIHSS) score, stroke subtypes, and lesion size (Lucke-Wold et al., 2012; Yu et al., 2015), whereas mortality, recurrence rate, and complication rate were the target outcomes (Lin et al., 2019; You et al., 2019; Montellano et al., 2021; Noubiap et al., 2021). The majority of the available data used for prediction focused mostly on the acute phase of the disease, with few extended longitudinal follow-ups (Faura et al., 2021). Early rehabilitation efforts have been shown to have a positive effect on functional outcomes at 3 months, in acute stroke patients (Winstein et al., 2016; Ahmed et al., 2020). However, studies on diagnostics, rehabilitation, and prognostics of stroke recovery have not kept pace (Moradi et al., 2021; Preston et al., 2021). During this prolonged rehabilitation process, although there were intermittent functional assessments, prognosis judgment was mainly based on the experience of the physician.
Different nomograms have been developed to predict mortality, stroke-associated infections, malignant cerebral edema, post-stroke depression, hemorrhagic transformation, or unfavorable outcomes in stroke populations with different characteristics (Du et al., 2020; Lan et al., 2020, 2022; Szlachetka et al., 2022; Zhang C. et al., 2022; Zhang K. et al., 2022). Zhang C. et al. (2022) innovatively developed a dynamic nomogram to predict the 3-month unfavorable outcome of patients with acute ischemic stroke based on glycosylated hemoglobin, the Alberta Stroke Program Early Computed Tomography Score (ASPECTS), and NIHSS score at day 14, with C-index of 0.891 (95% CI, 0.854–0.928). However, this model still has some limitations, such as being limited by the sample size (n = 93), this model lacks external validation, and requires invasive blood draw and early Computed Tomography (CT) scan (Zhang C. et al., 2022).
We developed an effective and practical nomogram for predicting unfavorable functional outcomes following the acute phase using multidimensional data such as rehabilitation intervention-related features and longitudinally collected data from several Chinese institutions.
2. Materials and methods
2.1. Study design
This multicenter retrospective observational study was based on baseline data and longitudinally collected rehabilitation features extracted from various institutions in China. This retrospective study was approved by the Institutional Ethics Review Board, which waived the requirement for informed consent.
2.2. Patient selection
Ischemic stroke patients who underwent rehabilitation between August 2018 and November 2020 at these institutions were screened, and randomly allocated to either the training cohort or the test cohort in a ratio of 4:1. Patients diagnosed as acute ischemic stroke (within 24 h of onset) based on clinical and computed tomography findings, aged 18 years or older, and underwent early rehabilitation were included in this study. Patients with hemorrhagic stroke, transient ischemic attack, baseline NIHSS score <2, without modified Rankin Scale (mRS) score 90 days after stroke, and those with severe cognitive and mental dysfunction were excluded from this study.
2.3. Data collection
We collected baseline demographic data (such as age, gender, occupation, or education), laboratory and clinical examination data (such as hemoglobin A1c, triglycerides, or total cholesterol), pharmaceutical and invasive therapy-related data (such as the use of intravenous thrombolysis, endovascular therapy or antiplatelet therapy within 48 h) within 48 h after onset, and rehabilitation featured data (such as time from onset to first rehabilitation intervention, time from onset to first effective mobilization or total length of effective mobilization within first 14 days) on the 15th day after onset. In addition, longitudinal follow-up data such as the NIHSS score, Barthel Index (BI), and mRS score were collected 24 h and 15 days following ischemic stroke. The target outcome was functional status, which was assessed 90 days following onset using the mRS questionnaire. The favorable functional outcome was defined as an mRS score of 0–2, while the unfavorable outcome was an mRS score of 3–6 (Zheng et al., 2021).
2.4. Statistical analysis
All statistical analysis was performed using SPSS 19.0 software (SPSS Inc., Chicago, IL, USA). The missing data at baseline were managed with mean imputation, whereas missing data at follow-up were imputed using the last observation carried forward method (Kim et al., 2019). Baseline demographics were presented as a mean (standard deviation) or a number (percentage). Student’s T-test or Mann–Whitney U test was used to compare baseline data between the training cohort and the test cohort for continuous variables, while Chi-square or Fisher’s exact test was used for categorical variables. Variables with statistical significance (p < 0.05) in the univariate logistic regression were included in the multivariate logistic regression to identify predictors of unfavorable functional outcomes. The nomogram was developed using predictors in the training cohort identified by multivariate logistic regression. The nomogram was constructed using the regression modeling strategies package in R version 3.0.2 (R Project for Statistical Computing).1 After establishing the predictive nomogram, the C-statistic and the receiver operating characteristic (ROC) curve were used to validate the accuracy and discriminative ability of the nomogram both internally (training cohort) and externally (test cohort). The C-statistic was calculated as the area under the ROC curve (AUC) and was used to evaluate the predictive performance of the model. The optimal cut-off value for clinical use was determined by maximizing the Youden index (sensitivity + specificity-1). Statistical significance was defined by a two-tailed p-value of less than 0.05. Figure 1 shows a flowchart displaying the process of building the nomogram.
3. Results
3.1. Patient characteristics
A total of 856 ischemic stroke patients (684 in the training cohort and 172 in the test cohort) were included in our analysis. Our data revealed that the mean age of the participants was 63.73 years, and 585 of them were males (Table 1). A total of 518 participants experienced unfavorable outcomes 90 days following an ischemic stroke (Supplementary Table 1). There were no statistically significant differences between the training cohort and the test cohort in terms of demographic variables, Trial of ORG 10172 in Acute Stroke Treatment (TOAST) classification, or 90-day mRS proportion. The rate of missing data was less than 3.7% for all variables except education, which had a missing data rate of 9.1%. The overall missing data rate was approximately 0.7%.
3.2. Predictors of unfavorable functional outcomes
Overall, 45 variables were subjected to univariate analyses (Table 2). Among them, 17 variables with p < 0.05, including 5 demographic and anthropometric variables (age, occupation, atrial fibrillation history, coronary heart disease history, and myocardial infarction history), 2 laboratory and clinical examination variables (TOAST classification and hemoglobin A1c), 1 pharmaceutical and invasive therapy-related variable (antihypertensive agents), 3 rehabilitation variables (time from onset to first effective mobilization, effective mobilization in first rehabilitation intervention, a total length of effective mobilization within first 14 days in minutes), and 6 longitudinal follow-up data (NIHSS, BI, and mRS scores assessed at both baseline and 15-day) were selected as possible predictors.

Table 2. Correlation coefficients and odds ratios in the univariate logistic regression analysis of the training cohort.
Multivariate logistic regression analysis revealed that TOAST classification (p = 0.024), antihypertensive agents use [odds ratio (OR) = 1.86; 95% CI: 1.026, 3.373; p = 0.041], 15-day BI score (OR = 0.930; 95% CI: 0.902, 0.960; p < 0.001), and 15-day mRS score (OR = 13.494; 95% CI: 6.871, 26.501; p < 0.001) were significant predictors. Using large artery atherosclerosis (LAA) subtype as reference, stroke of other determined cause (OR = 0.105; 95% CI: 0.015, 0.720; p = 0.022) and stroke of undermined cause (OR = 0.038; 95% CI: 0.003, 0.483; p = 0.012) subtypes exhibited a lower risk (Table 3).
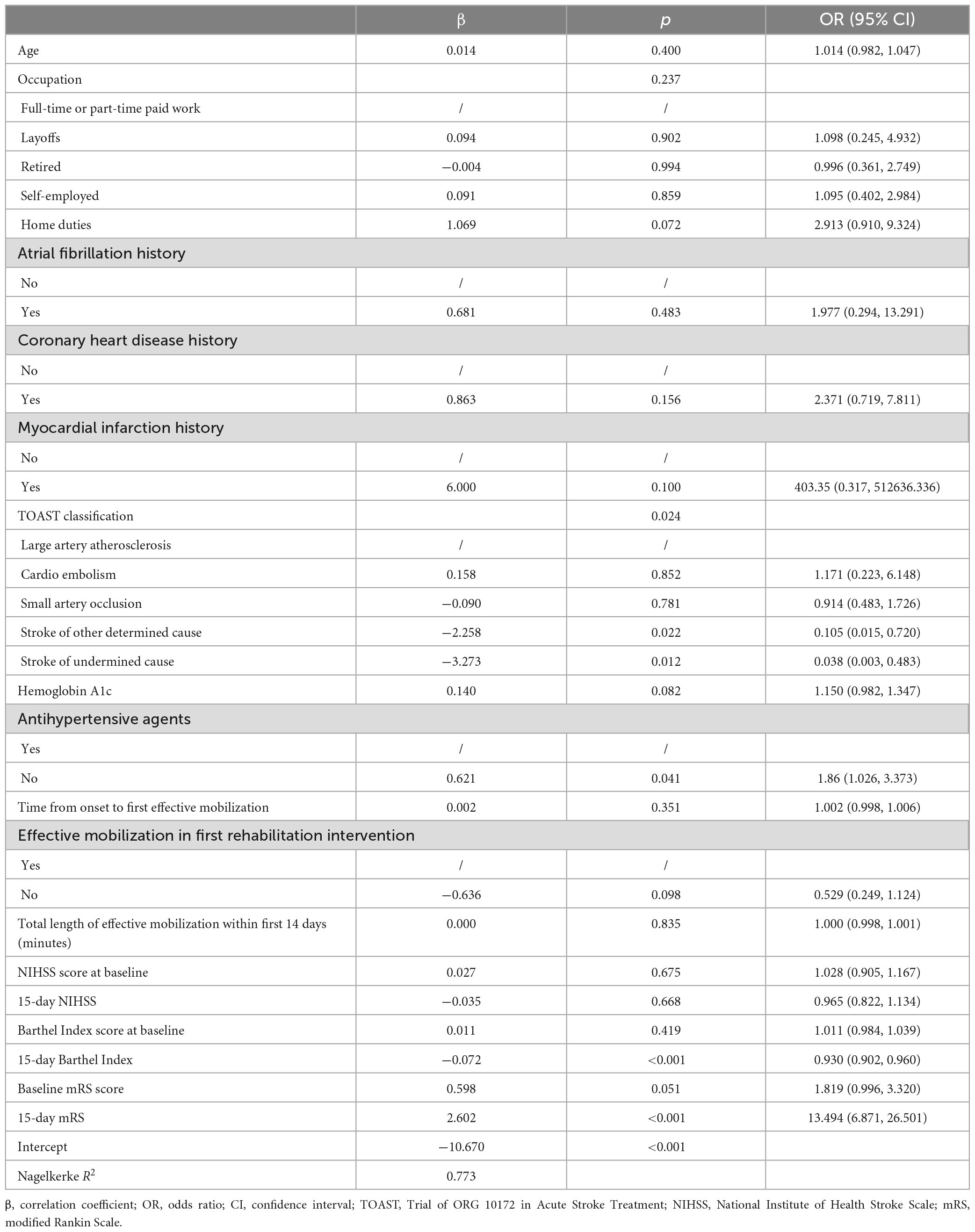
Table 3. Multivariate logistic regression for unfavorable functional outcomes in the training cohort.
According to the ROC curve analyses, the TOAST classification and antihypertensive agents use did not perform significantly well when used for individual prediction. The AUC value of TOAST classification in the test cohort was 0.589 (95% CI: 0.503, 0.674), while the AUC value of antihypertensive agents use was 0.536 (95% CI: 0.447, 0.625). In contrast, the 15-day BI score had an AUC value of 0.868 (95% CI: 0.815, 0.921), and the 15-day mRS score had an even better AUC value of 0.903 (95% CI: 0.858, 0.948) in the test cohort, indicating their good predictive performance (Supplementary Figure 1).
3.3. Development and validation of the nomogram
Based on the predictors, a nomogram was developed to predict unfavorable functional outcomes (Figure 2). In the nomogram, each patient received a total score based on baseline characteristics (TOAST classification and antihypertensive agents) and 15-day follow-up characteristics (15-day BI score and 15-day mRS score) to predict the occurrence of unfavorable functional outcomes with risk percentages. According to the maximized Youden index, the optimal cut-off value for an unfavorable functional outcome risk was 0.45.
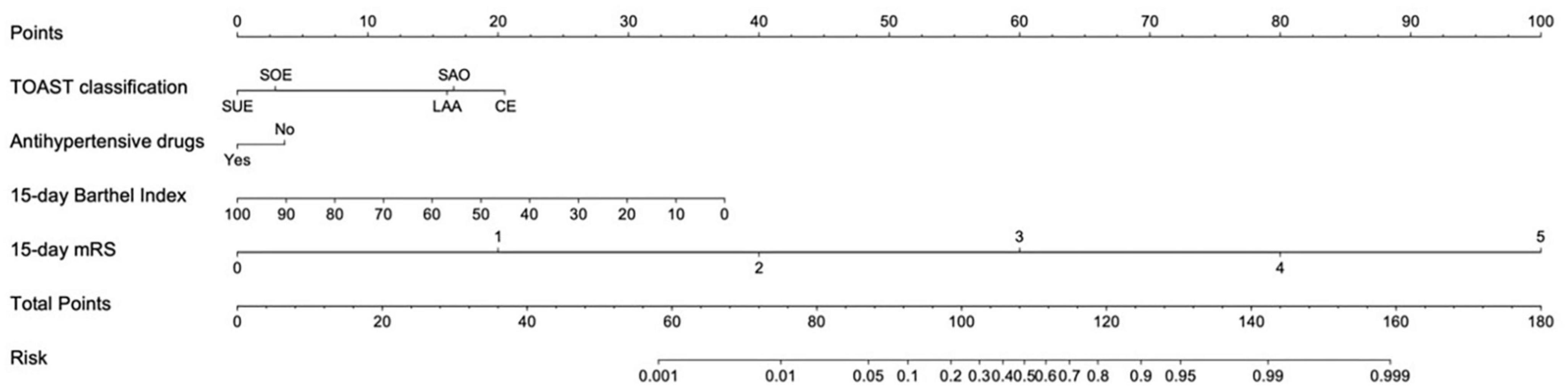
Figure 2. Nomogram for predicting unfavorable functional outcome 90 days after ischemic stroke. LAA, large artery atherosclerosis; CE, cardio embolism; SAO, small artery occlusion; SOE, stroke of other determined cause; SUE, stroke of undermined cause. Antihypertensive agents use refers to whether antihypertensive agents were used within 48 h after the onset of ischemic stroke. The cut-off value for an unfavorable functional outcome risk was 0.45.
The AUC of the model in the training cohort was 0.950 (95% CI, 0.935–0.965; Figure 3A), while that of the test cohort was 0.942 (95% CI, 0.910–0.974; Figure 3B).
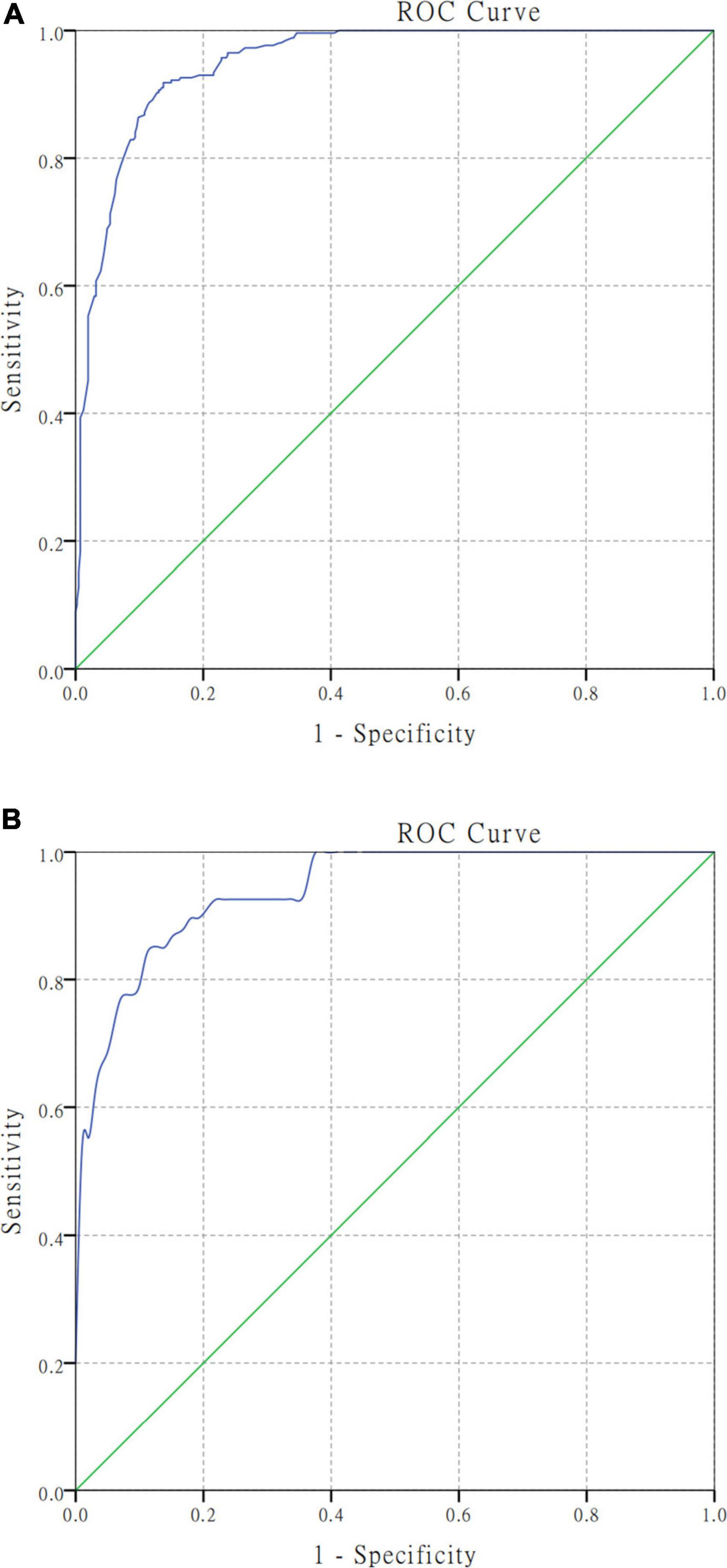
Figure 3. Receiver operating characteristic curve analyses for the nomogram model in the training and test cohort. Harrell’s C−statistic was calculated as the AUC (area under the curve) value. (A) The AUC was 0.950 (95% CI, 0.935–0.965) in the training cohort for predicting unfavorable functional outcomes. (B) The AUC was 0.942 (95% CI, 0.910–0.974) in the test cohort for predicting unfavorable functional outcomes.
4. Discussion
Early rehabilitation is considered an important treatment after an ischemic stroke and has been widely accepted in stroke units. Previous studies have demonstrated that early rehabilitation has positive effects on poststroke disability and health-related quality of life after ischemic stroke (Askew et al., 2020; Huang et al., 2020), however, there are no practical tool predicting the long-term functional outcomes during the rehabilitation phase. Previous predictive tools have focused on predicting outcomes before intervention, which has aided in the development of early treatment decision-making, such as thrombolysis, mechanical thrombectomy or rehabilitation treatment plans (Ang et al., 2003; Cho et al., 2008; Lan et al., 2020; Zhang C. et al., 2022). However, it is important to note that the recovery process after ischemic stroke is a long-term and continuous process. As such, the rehabilitation treatment plan may require continuous adjustments, and evaluation and new predictions serve as the basis for adjusting the plan. Our nomogram predicts long-term functional outcomes for patients 15 days after onset, providing more accurate predictions for physicians and patients during this period and helping to adjust subsequent treatment plans. Unlike previous tools, our tool is designed for patients who have undergone treatment for a period of time, rather than those with recent onset of ischemic stroke. A significant advantage of our tool is that the variables required for prediction are easily obtainable. Only BI and mRS scale assessments are required 15 days after onset, combined with retrospective collection of antihypertensive drug use and TOAST classification results. Our tool does not require invasive examinations or strong dependence on magnetic resonance imaging results, making it easier to promote and use compared to previous tools.
Previous research suggests that stroke severity (NIHSS score), age, gender, and other factors all influence stroke prognosis (Clua-Espuny et al., 2019). However, few studies have focused on features of rehabilitation therapy and prediction aiding in the design and adjustment of rehabilitation programs. As patients’ health conditions improve, a growing number of them receive early rehabilitation and continuous follow-up, which has made it possible to use rehabilitation characteristics to predict the risk of unfavorable functional outcomes in this population.
Similar to previous studies (Zhang et al., 2019), this study discovered that LAA (47.8%) and small artery occlusion (SAO) (38.8%) were the predominant subtypes in ischemic stroke. Studies have shown that the severity and prognosis of stroke patients are correlated with the TOAST classification (Leira et al., 2008). Cardio embolism (CE) subtype had the highest risk, whereas SAO and LAA subtypes had a higher risk than a stroke caused by other specified causes and stroke of undermined cause subtypes. This may be because the severity of the CE subtype is relatively severe, and its prognosis is worse, which is likely due to underlying cardiac pathologies such as arrhythmias and heart failure. A study by Bjerkreim et al. (2019) revealed that the 5-year risk of mortality and readmission for all causes was significantly higher in patients with CE compared with other subtypes. Chen et al. (2022) performed an outcome prediction of 10,967 patients with transient ischemic attack and minor stroke, and found that the TOAST classification was the influencing factor, the risk of LAA subtype was the highest, and the risk of SAO subtype was the lowest. A few studies have been conducted on people undergoing rehabilitation, disparate outcomes are likely attributable to distinct populations. However, in general, early assessment of TOAST classification in patients with ischemic stroke is of great significance for secondary prevention and prediction of poor prognosis.
In this retrospective study, the use of antihypertensive agents within 48 h after ischemic stroke was associated with a higher risk of unfavorable outcomes (OR = 1.86). However, neither systolic nor diastolic blood pressure (BP) levels were significant predictors. The management of hypertension in the acute stage of an ischemic stroke remains controversial, as observational studies are unable to determine whether elevated BP level is a poor prognostic factor following acute ischemic stroke (Ntaios et al., 2010, 2011). Following an acute ischemic stroke, prospective trials are necessary to validate the timing of BP lowering and choice of agent. As reported, acute and aggressive BP lowering within 24 h of stroke onset could jeopardize the outcome (Georgianou et al., 2018) and the benefit of starting antihypertensive therapy within 48 or 72 h after onset remains unclear (He et al., 2014). For patients not receiving intravenous recombinant tissue-type plasminogen activator (rt-PA), endovascular treatment and with no compelling medical condition to dictate acute BP lowering therapy, BPs up to 220/120 mmHg may be observed without BP lowering therapy in the first several days according to the latest American Heart Association/American Stroke Association [AHA/ASA] (2022) guidelines. At the same time, the AHA/ASA guidelines recommended that BP should be controlled to a level <185/110 mmHg before intervention and maintained at levels <180/105 mmHg in patients who require administration of rt-PA, intra-arterial fibrinolysis or mechanical thrombectomy (Powers et al., 2018). The Enhanced Control of Hypertension and Thrombolysis Stroke Study, on the other hand, found that achieving early and persistently low levels of SBP <140 mmHg within 24 h, even as low as 110–120 mmHg, was associated with better outcomes in thrombolytic-eligible acute ischemic stroke patients (Wang et al., 2022). Our findings suggest a potential benefit of antihypertensive agent use, but because this was an observational study, we cannot conclude that there is a direct causal relationship between antihypertensive agent use and outcomes. The deep relationship requires additional investigation. This study included patients who received thrombolysis, thrombectomy, and conservative treatment, limiting further interpretation of the results.
The NIHSS score is the world’s most widely used acute ischemic stroke scale (Saber and Saver, 2020). A previous study reported that discharged patients with high NIHSS scores were generally more severely ill and had larger brain infarct volumes, which were associated with 90-day outcomes in ischemic stroke patients (Wouters et al., 2018). In this study, the NIHSS score was also a potential influencing factor, although it was not included in the final model. The mRS score is widely used to assess disability; the higher the score, the higher the risk of unfavorable outcomes. The BI is widely used to assess daily activities of living, and the higher the score, the lower the risk of poor outcomes. The NIHSS, mRS, and BI are all associated with more severe disease, a larger infarct volume in brain tissue, and a 90-day prognosis in ischemic stroke patients (Govan et al., 2009). Unlike the results of Zhang C. et al. (2022), the NIHSS score was not included in our final model, which could be attributed to the increased contribution of mRS and BI scores. Furthermore, the NIHSS is an 11-item scale that assesses consciousness, vision, language, sensory, and motor function. The lack of strong correlation between NIHSS and outcomes may be due to the existence of multiple subscales. This could be the reason why NIHSS does not perform as well as BI and mRS in predicting 90-day mRS score (Schmid et al., 2011). At the same time, the mRS and BI scores at 15 days were more valuable in predicting outcome than the mRS and BI scores at baseline. Similarly, Chen et al. (2022) found that discharge mRS and discharge NIHSS score were associated with a poor 90-day prognosis, with discharge mRS having a greater predictive contribution than admission mRS. These findings indicated the importance of follow-up data in outcome prediction. Therefore, we emphasize the importance of reassessment after the acute phase, such as 15 days after onset.
There are some advantages and limits to this study. The first strength is that this study provides a basis for predicting the prognosis of patients in the rehabilitation stage. Second, although our study only used four predictors for modeling, the model and nomogram built by logistic regression achieved great prediction performance and were more convenient for external validation and nomogram use due to the small number and easy to obtain of features. The following are the limitations. First, this is a retrospective study and it is subject to the selection and follow-up bias. Second, we included the variables selected by univariate logistic regression in the multivariate logistic regression, which may lead to underestimation of interactions between variables. Third, the data included in this study is from patients who received early rehabilitation, limiting the generalizability of our findings to countries with lower rates of patients receiving early rehabilitation. As stated previously, however, an increasing number of stroke patients can receive early rehabilitation following the acute phase, and this population is growing. Fourth, our nomogram uses 15-day follow-up data, therefore patients lacking follow-up data will be limited to use this nomogram. Finally, the model can also incorporate machine learning methods to increase the accuracy of future predictions.
Future studies will use rehabilitation-related features to make more precise predictions of long-term functions such as swallowing function, walking ability, and aphasia. The unresolved question is how to identify individuals who can transition from higher mRS levels to lower mRS levels.
5. Conclusion
In conclusion, the nomogram can accurately predict the risk of unfavorable functional outcomes in the early rehabilitation population 90 days after ischemic stroke, with longitudinally collected data and rehabilitation-specific characteristics such as the 15-day mRS score and the 15-day BI score, serving as the predominant predictors. Patients at high risk (>0.45) should receive additional care to prevent unfavorable outcomes.
Data availability statement
The raw data supporting the conclusions of this article will be made available by the authors, without undue reservation.
Ethics statement
The studies involving human participants were reviewed and approved by the Ethics Committee of the First Affiliated Hospital of Nanjing Medical University. Written informed consent for participation was not required for this study in accordance with the national legislation and the institutional requirements.
Author contributions
CY, YZhe, XZ, CG, and XLu contributed to the conception and design of the study. SW, YZhu, YJ, XLi, GF, HP, MT, JL, and KQ collected the data. CY and LX performed the statistical analysis. CY drafted the manuscript. YZhe and XLu revised the manuscript. All authors contributed to the article and approved the submitted version.
Funding
This study was supported by grants from the Stroke Prevention and Treatment Project of the National Health Commission – Research and Popularization of Appropriate Intervention Technology for the Stroke High Risk Group in China (Task No. GN-2018R0010).
Acknowledgments
We would like to thank each of the participants in this study for their effort.
Conflict of interest
The authors declare that the research was conducted in the absence of any commercial or financial relationships that could be construed as a potential conflict of interest.
Publisher’s note
All claims expressed in this article are solely those of the authors and do not necessarily represent those of their affiliated organizations, or those of the publisher, the editors and the reviewers. Any product that may be evaluated in this article, or claim that may be made by its manufacturer, is not guaranteed or endorsed by the publisher.
Supplementary material
The Supplementary Material for this article can be found online at: https://www.frontiersin.org/articles/10.3389/fnagi.2023.1161016/full#supplementary-material
Footnotes
References
Ahmed, N., Mauad, V. A. Q., Gomez-Rojas, O., Sushea, A., Castro-Tejada, G., Michel, J., et al. (2020). The impact of rehabilitation-oriented virtual reality device in patients with ischemic stroke in the early subacute recovery phase: study protocol for a phase iii, single-blinded, randomized, controlled clinical trial. J. Cent. Nerv. Syst. Dis. 12:1179573519899471. doi: 10.1177/1179573519899471
Ali-Ahmed, F., Federspiel, J. J., Liang, L., Xu, H., Sevilis, T., Hernandez, A. F., et al. (2019). Intravenous tissue plasminogen activator in stroke mimics. Circ. Cardiovasc. Qual. Outcomes 12:e005609. doi: 10.1161/CIRCOUTCOMES.119.005609
American Heart Association/American Stroke Association [AHA/ASA] (2022). Types of Stroke. Washington, DC: American Heart & Stroke Association.
Ang, Y. H., Chan, D. K., Heng, D. M., and Shen, Q. (2003). Patient outcomes and length of stay in a stroke unit offering both acute and rehabilitation services. Med. J. Aust. 178, 333–336. doi: 10.5694/j.1326-5377.2003.tb05225.x
Askew, R. L., Capo-Lugo, C. E., Naidech, A., and Prabhakaran, S. (2020). Differential effects of time to initiation of therapy on disability and quality of life in patients with mild and moderate to severe ischemic stroke. Arch. Phys. Med. Rehabil. 151:e1511. doi: 10.1016/j.apmr.2020.05.005
Bjerkreim, A. T., Khanevski, A. N., Thomassen, L., Selvik, H. A., Waje-Andreassen, U., Naess, H., et al. (2019). Five-year readmission and mortality differ by ischemic stroke subtype. J. Neurol. Sci. 403, 31–37. doi: 10.1016/j.jns.2019.06.007
Chen, S. D., You, J., Yang, X. M., Gu, H. Q., Huang, X. Y., Liu, H., et al. (2022). Machine learning is an effective method to predict the 90-day prognosis of patients with transient ischemic attack and minor stroke. BMC Med. Res. Methodol. 22:195. doi: 10.1186/s12874-022-01672-z
Cho, Y. M., Choi, I. S., Bian, R. X., Kim, J. H., Han, J. Y., and Lee, S. G. (2008). Serum albumin at admission for prediction of functional outcome in ischaemic stroke patients. Neurol. Sci. 29, 445–449. doi: 10.1007/s10072-008-1024-0
Clua-Espuny, J. L., Abilleira, S., Queralt-Tomas, L., Gonzalez-Henares, A., Gil-Guillen, V., Muria-Subirats, E., et al. (2019). Long-term survival after stroke according to reperfusion therapy. cardiovascular therapy and gender. Cardiol. Res. 10, 89–97. doi: 10.14740/cr839
Diener, H. C., and Hankey, G. J. (2020). Primary and secondary prevention of ischemic stroke and cerebral hemorrhage: JACC Focus Seminar. J. Am. Coll. Cardiol. 75, 1804–1818. doi: 10.1016/j.jacc.2019.12.072
Du, M., Huang, X., Li, S., Xu, L., Yan, B., Zhang, Y., et al. (2020). a nomogram model to predict malignant cerebral edema in ischemic stroke patients treated with endovascular thrombectomy: an observational study. Neuropsychiatr. Dis. Treat. 16, 2913–2920. doi: 10.2147/NDT.S279303
Fahey, M., Crayton, E., Wolfe, C., and Douiri, A. (2018). Clinical prediction models for mortality and functional outcome following ischemic stroke: A systematic review and meta-analysis. PLoS One 13:e0185402. doi: 10.1371/journal.pone.0185402
Faura, J., Bustamante, A., Reverte, S., Garcia-Berrocoso, T., Millan, M., Castellanos, M., et al. (2021). Blood biomarker panels for the early prediction of stroke-associated complications. J. Am. Heart Assoc. 10:e018946. doi: 10.1161/JAHA.120.018946
Georgianou, E., Georgianos, P. I., Petidis, K., Athyros, V. G., Sarafidis, P. A., and Karagiannis, A. (2018). Antihypertensive therapy in acute ischemic stroke: where do we stand? J. Hum. Hypertens. 32, 799–807. doi: 10.1038/s41371-018-0105-7
Govan, L., Langhorne, P., and Weir, C. J. (2009). Categorizing stroke prognosis using different stroke scales. Stroke 40, 3396–3399. doi: 10.1161/STROKEAHA.109.557645
Gravanis, I., and Tsirka, S. E. (2008). Tissue-type plasminogen activator as a therapeutic target in stroke. Expert. Opin. Ther. Targets 12, 159–170. doi: 10.1517/14728222.12.2.159
He, J., Zhang, Y., Xu, T., Zhao, Q., Wang, D., Chen, C. S., et al. (2014). Effects of immediate blood pressure reduction on death and major disability in patients with acute ischemic stroke: the CATIS randomized clinical trial. JAMA 311, 479–489. doi: 10.1001/jama.2013.282543
Huang, J. B., Chen, N. C., Chen, C. L., Fu, M. H., Pan, H. Y., Hsu, C. Y., et al. (2020). Serum levels of soluble triggering receptor expressed on myeloid cells-1 associated with the severity and outcome of acute ischemic stroke. J. Clin. Med. 10:10061. doi: 10.3390/jcm10010061
Kim, M., Merrill, J. T., Wang, C., Viswanathan, S., Kalunian, K., Hanrahan, L., et al. (2019). SLE clinical trials: impact of missing data on estimating treatment effects. Lupus Sci. Med. 6:e000348. doi: 10.1136/lupus-2019-000348
Lan, Y., Pan, C., Qiu, X., Miao, J., Sun, W., Li, G., et al. (2022). Nomogram for persistent post-stroke depression and decision curve analysis. Clin. Interv. Aging 17, 393–403. doi: 10.2147/CIA.S357639
Lan, Y., Sun, W., Chen, Y., Miao, J., Li, G., Qiu, X., et al. (2020). Nomogram including neutrophil-to-lymphocyte ratio for the prediction of stroke-associated infections. Front. Neurol. 11:574280. doi: 10.3389/fneur.2020.574280
Langhorne, P., Bernhardt, J., and Kwakkel, G. (2011). Stroke rehabilitation. Lancet 377, 1693–1702. doi: 10.1016/S0140-6736(11)60325-5
Leira, E. C., Adams, H. P. Jr., Rosenthal, G. E., and Torner, J. C. (2008). Baseline NIH stroke scale responses estimate the probability of each particular stroke subtype. Cerebrovasc. Dis. 26, 573–577. doi: 10.1159/000165109
Lin, C. H., Yen, C. C., Hsu, Y. T., Chen, H. H., Cheng, P. W., Tseng, C. J., et al. (2019). Baroreceptor sensitivity predicts functional outcome and complications after acute ischemic stroke. J. Clin. Med. 8, 300. doi: 10.3390/jcm8030300
Lopatkiewicz, A. M., Gradek-Kwinta, E., Czyzycki, M., Pera, J., Slowik, A., and Dziedzic, T. (2020). Glucocorticoid resistance is associated with poor functional outcome after stroke. Cell Mol. Neurobiol. 40, 1321–1326. doi: 10.1007/s10571-020-00818-1
Lucke-Wold, B. P., Turner, R., Lucke-Wold, A., Rosen, C., and Huber, J. D. (2012). Age and the metabolic syndrome as risk factors for ischemic stroke: Improving preclinical models of ischemic stroke. Yale J. Biol. Med. 85, 523–539.
Montellano, F. A., Ungethum, K., Ramiro, L., Nacu, A., Hellwig, S., Fluri, F., et al. (2021). Role of blood-based biomarkers in ischemic stroke prognosis: A systematic review. Stroke 52, 543–551. doi: 10.1161/STROKEAHA.120.029232
Moradi, V., Mafi, H., Shariat, A., Cleland, J. A., Nakhostin Ansari, N., and Savari, S. (2021). Neurorehabilitation, the practical method of returning to work after stroke. Iran J. Public Health 50, 209–210. doi: 10.18502/ijph.v50i1.5092
Noubiap, J. J., Fitzgerald, J. L., Gallagher, C., Thomas, G., Middeldorp, M. E., and Sanders, P. (2021). Rates, predictors, and impact of smoking cessation after stroke or transient ischemic attack: a systematic review and meta-analysis. J. Stroke Cerebrovasc. Dis. 30, 106012. doi: 10.1016/j.jstrokecerebrovasdis.2021.106012
Ntaios, G., Bath, P., and Michel, P. (2010). Blood pressure treatment in acute ischemic stroke: a review of studies and recommendations. Curr. Opin. Neurol. 23, 46–52. doi: 10.1097/WCO.0b013e328334e9d9
Ntaios, G., Lambrou, D., and Michel, P. (2011). Blood pressure change and outcome in acute ischemic stroke: the impact of baseline values, previous hypertensive disease and previous antihypertensive treatment. J. Hypertens. 29, 1583–1589. doi: 10.1097/HJH.0b013e328348c957
Powers, W. J., Rabinstein, A. A., Ackerson, T., Adeoye, O. M., Bambakidis, N. C., Becker, K., et al. (2018). 2018 Guidelines for the early management of patients with acute ischemic stroke: a guideline for healthcare professionals from the American Heart Association/American Stroke Association. Stroke 49, e46–e110. doi: 10.1161/STR.0000000000000158
Preston, E., Ada, L., Stanton, R., Mahendran, N., and Dean, C. M. (2021). Prediction of independent walking in people who are nonambulatory early after stroke: A systematic review. Stroke 52, 3217–3224. doi: 10.1161/STROKEAHA.120.032345
Saber, H., and Saver, J. L. (2020). Distributional validity and prognostic power of the National Institutes of Health Stroke Scale in US Administrative Claims Data. JAMA Neurol. 77, 606–612. doi: 10.1001/jamaneurol.2019.5061
Schmid, A. A., Kroenke, K., Hendrie, H. C., Bakas, T., Sutherland, J. M., and Williams, L. S. (2011). Poststroke depression and treatment effects on functional outcomes. Neurology 76, 1000–1005. doi: 10.1212/WNL.0b013e318210435e
Szlachetka, W. A., Pana, T. A., Mamas, M. A., Bettencourt-Silva, J. H., Metcalf, A. K., Potter, J. F., et al. (2022). Predicting 10-year stroke mortality: Development and validation of a nomogram. Acta Neurol. Belg. 122, 685–693. doi: 10.1007/s13760-021-01752-9
Wang, X., Minhas, J. S., Moullaali, T. J., Di Tanna, G. L., Lindley, R. I., Chen, X., et al. (2022). Associations of early systolic blood pressure control and outcome after thrombolysis-eligible acute ischemic stroke: Results from the ENCHANTED Study. Stroke 53, 779–787. doi: 10.1161/STROKEAHA.121.034580
Winstein, C. J., Stein, J., Arena, R., Bates, B., Cherney, L. R., Cramer, S. C., et al. (2016). Guidelines for adult stroke rehabilitation and recovery: A guideline for healthcare professionals from the American Heart Association/American Stroke Association. Stroke 47, e98–e169. doi: 10.1161/STR.0000000000000098
Wouters, A., Nysten, C., Thijs, V., and Lemmens, R. (2018). Prediction of outcome in patients with acute ischemic stroke based on initial severity and improvement in the first 24 h. Front. Neurol. 9:308. doi: 10.3389/fneur.2018.00308
You, S., Ou, Z., Zhang, W., Zheng, D., Zhong, C., Dong, X., et al. (2019). Combined utility of white blood cell count and blood glucose for predicting in-hospital outcomes in acute ischemic stroke. J. Neuroinflammation 16:37. doi: 10.1186/s12974-019-1422-7
Yu, C., An, Z., Zhao, W., Wang, W., Gao, C., Liu, S., et al. (2015). Sex differences in stroke subtypes, severity, risk factors, and outcomes among elderly patients with acute ischemic stroke. Front. Aging Neurosci. 7:174. doi: 10.3389/fnagi.2015.00174
Zhang, C., Zhang, W., Huang, Y., Qiu, J., and Huang, Z. X. (2022). A dynamic nomogram to predict the 3-month unfavorable outcome of patients with acute ischemic stroke. Risk Manag. Healthc. Policy 15, 923–934. doi: 10.2147/RMHP.S361073
Zhang, K., Luan, J., Li, C., and Chen, M. (2022). Nomogram to predict hemorrhagic transformation for acute ischemic stroke in Western China: A retrospective analysis. BMC Neurol. 22:156. doi: 10.1186/s12883-022-02678-2
Zhang, H., Li, Z., Dai, Y., Guo, E., Zhang, C., and Wang, Y. (2019). Ischaemic stroke etiological classification system: the agreement analysis of CISS, SPARKLE and TOAST. Stroke Vasc. Neurol. 4, 123–128. doi: 10.1136/svn-2018-000226
Keywords: nomogram, ischemic stroke, modified Rankin Scale, rehabilitation, predictive factor
Citation: Yan C, Zheng Y, Zhang X, Gong C, Wen S, Zhu Y, Jiang Y, Li X, Fu G, Pan H, Teng M, Xia L, Li J, Qian K and Lu X (2023) Development and validation of a nomogram model for predicting unfavorable functional outcomes in ischemic stroke patients after acute phase. Front. Aging Neurosci. 15:1161016. doi: 10.3389/fnagi.2023.1161016
Received: 07 February 2023; Accepted: 03 July 2023;
Published: 14 July 2023.
Edited by:
Jie Jia, Fudan University, ChinaReviewed by:
Junfen Fan, Capital Medical University, ChinaWen Wu, Southern Medical University, China
Yue Lan, Guangzhou First People’s Hospital, China
Copyright © 2023 Yan, Zheng, Zhang, Gong, Wen, Zhu, Jiang, Li, Fu, Pan, Teng, Xia, Li, Qian and Lu. This is an open-access article distributed under the terms of the Creative Commons Attribution License (CC BY). The use, distribution or reproduction in other forums is permitted, provided the original author(s) and the copyright owner(s) are credited and that the original publication in this journal is cited, in accordance with accepted academic practice. No use, distribution or reproduction is permitted which does not comply with these terms.
*Correspondence: Xiao Lu, bHV4aWFvMTk3MkAxNjMuY29t
†These authors have contributed equally to this work and share first authorship