- 1School of Mechanical Engineering, Pusan National University, Busan, South Korea
- 2Department of Robotics Engineering, Daegu Gyeongbuk Institute of Science and Technology, Daegu, South Korea
- 3Department of Cogno-Mechatronics Engineering, Pusan National University, Busan, South Korea
For those individuals with upper-extremity amputation, a daily normal living activity is no longer possible or it requires additional effort and time. With the aim of restoring their sensory and motor functions, theoretical and technological investigations have been carried out in the field of neuroprosthetic systems. For transmission of sensory feedback, several interfacing modalities including indirect (non-invasive), direct-to-peripheral-nerve (invasive), and cortical stimulation have been applied. Peripheral nerve interfaces demonstrate an edge over the cortical interfaces due to the sensitivity in attaining cortical brain signals. The peripheral nerve interfaces are highly dependent on interface designs and are required to be biocompatible with the nerves to achieve prolonged stability and longevity. Another criterion is the selection of nerves that allows minimal invasiveness and damages as well as high selectivity for a large number of nerve fascicles. In this paper, we review the nerve-machine interface modalities noted above with more focus on peripheral nerve interfaces, which are responsible for provision of sensory feedback. The invasive interfaces for recording and stimulation of electro-neurographic signals include intra-fascicular, regenerative-type interfaces that provide multiple contact channels to a group of axons inside the nerve and the extra-neural-cuff-type interfaces that enable interaction with many axons around the periphery of the nerve. Section Current Prosthetic Technology summarizes the advancements made to date in the field of neuroprosthetics toward the achievement of a bidirectional nerve-machine interface with more focus on sensory feedback. In the Discussion section, the authors propose a hybrid interface technique for achieving better selectivity and long-term stability using the available nerve interfacing techniques.
Introduction
According to Ziegler-Graham et al. (2008), approximately 2 million people in the United States have suffered from the loss of limb(s). Substantial progress has been made in the form of highly sensorized cybernetic prostheses for restoration of the sensorimotor functionalities of limbs for those who have undergone an amputation. Achieving an effective interface between the nervous system and prostheses takes a long time due to the limitations of necessary components. The recent research in prosthetic technologies reveals a trend toward natural bidirectional communication between the amputee and the bionic arm/prosthesis and subsequently possible solutions in developing such a prosthesis that enables sensory restoration. The ultimate goal is to develop a bidirectional interface between the nervous system and a given prosthesis (e.g., a bionic hand, arm, or leg). This can be achieved using the form of a closed-loop control that sends motor commands on efferent pathways and returns the sensory feedback on afferent pathways by means of stimulation. According to several studies (Micera et al., 2008; Rossini et al., 2010; Raspopovic et al., 2014), an ideal closed-loop bidirectional prosthesis-user interface has the following mandatory components (see Figure 1 below): (i) peripheral-nerve data-recording electrodes; (ii) decoding of user intention; (iii) production of motor commands for the prosthesis system; (iv) passage of this information to the controller for controlling the speed/force for handling an object; (v) sensors embedded in the bionic hand/arm to capture the environmental information; and (vi) a sensory subsystem to encode the feedback to an amputee through a nerve stimulator (stimulation electrodes), which evokes the sensation produced through a contact with the manipulated object. Particularly for those who have lost upper limbs, natural sensory feedback through the prostheses is especially desired (Biddiss et al., 2007; Pylatiuk et al., 2007; Wijk and Carlsson, 2015). Witteveen et al. (2012, 2015) found that sensory-feedback systems improved the control of prosthetic hands, eradicated the unpleasant phantom limb pain sensation (Flor et al., 2001), and enhanced the sense of self-esteem. Object discrimination tasks were successfully accomplished by an amputee using artificial tactile and proprioceptive feedbacks without visual or auditory cues (Horch et al., 2011).
Lost functions were restored to the patients with central nervous system damages by electrically activating the intact tissues distal to neural lesions. This kind of activation can be realized via interfaces positioned either on the body surface or on the muscles near the motor points, or can be directly implanted in the motor nerves (Kuiken et al., 2004; Clemente et al., 2016; Davis et al., 2016; Patel et al., 2016). Nerve-fiber recording and stimulation from the nervous system have been carried out with the help of electrodes (Leventhal and Durand, 2003). Over the past 70 years, peripheral nerve interfaces enabling the restoration of sensorimotor functions have been improving and evolving (Branner and Normann, 2000). Now, with these systems, researchers are able to record the activity of the nerves elicited by volitional movements and electrically stimulate the peripheral nerves to move muscles. Direct nerve stimulation has several advantages over alternative methods, which include the low power consumption, multiple-muscle control via a single implantation, and the inherent capability of positioning the interface away from the contracting muscles (Koole et al., 1997). Peripheral-nerve electrodes, to be effective in neuroprosthetic applications, must have the capacity of targeting specific axons for activation without stimulating others. Moreover, they must be safe and biocompatible, and have stimulation characteristics that will remain stable over many years. This review mainly focuses on the amputees having limb loss(es) (not those having spinal cord injuries), because the scope of this review is restricted to peripheral nerve interfaces: In those amputees who lost only sensory end organs, the nerves connecting the end organs to the brain are functioning normally.
The rapid development of bidirectional peripheral-nerve interfaces has made available several methods for recording of motor commands from nerves and/or stimulation on nerve fibers. The main characteristics in bidirectional interfaces are the selectivity of axons and the degree of invasiveness. Achieving an optimal effectiveness entails a tradeoff between the reduced invasiveness for stability and the sufficient invasiveness for greater selectivity, in which the latter might damage the nerve and also affect chronic stability. For applications, an interface involving multiple electrodes implanted in an amputee's peripheral-nerve should be able to evoke a sensory response, record motor commands, and control multiple limb functions. Micera et al. (2008) suggested that the first step toward a suitable reproduction of sensorimotor functionality is to determine the topographical location in the nerve for desired functions. According to Gustafson et al. (2009) and Badia et al. (2010), the identification of fascicular contact points for electrode positioning is absolutely essential to selective interfacing with individual fascicles in a particular nerve. A various types of devices to improve the nerve-machine interface for the restoration of lost neural functions can be pursued. However, the long-term stability and selectivity of neural recording and stimulation remains a challenge. A key for an advanced neuro-prosthetics is the achievement of selective sensory perception, durability, and natural discernment from the nerve-machine interface. The selectivity, longevity, and long-term stability are absolutely an integral part for the establishment of long-lasting and functional sensory feedback for an amputee (Warren et al., 2016; Wurth et al., 2017).
The aim of the present review is to discuss the invasive and non-invasive methods in detail for evoking sensory feedback and recording intended motor commands from peripheral nerves. The authors first briefly outline the structure of the peripheral nerves that are responsible for transmitting motor commands and sensory feedback signals from/to the brain. Second, three different methodologies for evoking sensory feedback will be discussed: (i) Indirect (non-invasive) elicitation feedback by applying pressure or current, (ii) direct (invasive) elicitation through the peripheral nerve using several types of interfaces, and (iii) a cortical stimulation method to activate the somatosensory cortex in the brain. Figure 2 breaks down the methods discussed in this paper for providing sensory feedback to a bionic prosthesis. We will also discuss two hybrid techniques for bidirectional control of a bionic arm from the perspective of selectivity and longevity. Finally, in section Discussion, we propose a scheme for enhanced selectivity, longevity, and long-term stability for sensory feedback using the current techniques available in the field.
Structure of Peripheral Nerves
The roots of the peripheral nervous system lie within the spinal cord, and the axons spread inside the peripheral nerves to reach the target organs. In this way, the peripheral nervous system fulfills its chief responsibility; the transmission of information between the brain and the extremities. In the peripheral nerves, numerous motor (called efferent) and sensory (called afferent) fibers are present (Johnson et al., 2000; Fisher et al., 2014; Mildren and Bent, 2016). The afferent fibers ranging 2–20 μm in diameter, either myelinated or unmyelinated, terminate at specialized sensory receptors in the skin, tissues and muscles. These fibers are responsible for sending mechanical, thermal, and noxious stimuli to the brain. Efferent motor fibers, on the other hand, send movement/motor commands from the brain to muscles. Both afferent and efferent nerve fibers are clustered individually in the form of fascicles that are surrounded by connective tissues in the peripheral nerves. In addition to the bundles of nerve fibers, three supportive sheaths bind the fibers in an organized structure (namely, epineurium surrounding the nerve, perineurium surrounding the fascicle, and endoneurium surrounding the fiber). Several researchers over the years have developed interfaces for accessing multiple and distinct levels of the nerves by penetrating the protective sheaths longitudinally and/or transversally. In this review, we will focus more on afferent fiber interface techniques for provision of sensory feedback.
Afferent Receptors
Touch, pressure, proprioception, temperature, and pain fall under the rubric of somatic sensation. The somatic receptors are divided according to the following broad categories: (i) proprioceptors that provide information on joint position and motion; (ii) nociceptors for temperature, pressure, different chemical stimuli, and a combination of these; (iii) thermoreceptors for mild temperature; (iv) cutaneous mechanoreceptors for touch and pressure, and (v) chemoreceptors for detection of certain chemical stimuli. Several types of sensory receptors are available in the body, and their quantity varies with respect to their body location. According to Johansson and Vallbo (1979), the most innervated parts in the body are hands, which permit fine manipulation and precise perception of the environment. As an illustration of a human finger's utility, the fingertip has approximately 241 units/cm2 of cutaneous mechanoreceptors for very fine sensory resolution, as compared with the palm that has 58 units/cm2 only. The important sensory feedbacks required for prostheses are proprioception and tactile sensation. For instance, cutaneous mechanoreceptors are essential for executing daily routines and simple tasks such as the grasping a glass. Johnson et al. (2000) and Johansson and Flanagan (2009) have demonstrated that detection of minute slippage of an object from a hand is by Meissner corpuscles and rapidly adapting afferents; to avoid slippage, a reflexive force is triggered. This type of testing, therefore, might be useful for assessment of the complete functionality of a rapidly adapting system. In the past, several approaches have been used to test and provide sensory feedback to bionic arms/prostheses. In the next section, non-invasive methods utilized for indirect sensory feedback are discussed.
Non-Invasive Methods of Sensory Feedback
Several substitutions for sensory perception have been developed, which do not require implantable interfaces (Lundborg and Rosen, 2001; Visell, 2009; Khasnobish et al., 2016): Sensory substitution is a technique to provide an alternative path for necessary sensory information to the body using other sensory passages that are different from those naturally used. For instance, Kaczmarek et al. (1991) revealed that hearing and vibration can serve as a substitute for touch and pressure, respectively. Due to the essential need to restore physiological sensory information for those who have gone through a traumatic event and/or amputation, many of these sensory substitutions have been employed for battery-powered prostheses to achieve sensation. However, these modalities are not yet robust or effective enough to be applicable to routine tasks of daily living. Their use in commercially available prosthetics has not yet been widely adopted; however, they have been successful in controlled environments with certain limitations. The commonly used techniques are electro-tactile and vibro-tactile sensory substitutions as well as modality-matched feedback, which use an electric current and mechanical vibration in the residual skin area of the limb. This can help in encoding information on object manipulation, grasping force, elbow angle, and approaching direction (Hsiao et al., 2011; Chen et al., 2016; Clemente et al., 2016; Isakovic et al., 2016; Xu et al., 2016).
Electro-Tactile Stimulation
The electro-tactile sensory modality is a method of passing electrical current through the skin of an amputee to elicit perception (Yem and Kajimoto, 2017). The sensation is not necessarily confined to the zone under the stimulating device, and the elicited sensations can spread if these are placed near the nerve bundles. Electro-tactile (electrocutaneous) stimulation is either voltage- or current-regulated. The use of voltage-regulated stimulation can minimize the chance of skin burns. Visell (2009) showed that the change in impedance and load at the site of interface might not affect the value of current in electro-tactile-current-regulated stimulation. Multiple features of this modality can be controlled to elicit sensory percepts. These features include (i) the place, material, and geometrical properties of the interface, (ii) the parameters of the current (duration, frequency, and amplitude), and (iii) end-organ thickness and location of the skin stimulated (Boldt et al., 2014; Hartmann et al., 2015; Paredes et al., 2015; Strbac et al., 2016). Subjects often describe electro-tactile sensations, qualitatively, as burning, pain, touch, pinch, tingle, pressure, vibrations, itch, sharp, etc. depending on the stimulation voltage or current. Although electro-tactile feedback cannot replicate direct forces and special types of touch sensations yet, it can produce a wide range of tactile sensations. Also, electro-tactile stimulation has been used for providing an alternative way to visual perception to the blind (Kaczmarek et al., 1991). The experimental study with electro-tactile feedback by Perovic et al. (2013) showed its suitability for haptic perception as well. Their results revealed that electro-tactile feedback has a potential for delivering haptic sensations from devices such as prosthetic hands. The users of this modality have been able to regulate the grasping forces as well as to the angular displacement of the prosthetic hand to predefined levels (Clemente et al., 2016; Schweisfurth et al., 2016). Further, Patel et al. (2016) improved the control performance by the grasping function by providing tactile stimulations to individual fingers (multiple degrees of freedom) in comparison to open-loop controls (Jorgovanovic et al., 2014; Dosen et al., 2015; Isakovic et al., 2016; Xu et al., 2016). Brain-computer interface (BCI) is a method of communication between brain and hardware by means of signals generated from the brain without the involvement of muscles and peripheral nerves (Naseer and Hong, 2013, 2015; Naseer et al., 2016; Rutkowski, 2016). An opposite modality of it is known as BrainPort, which has been designed to support a direct link from a computerized environment to the human brain non-invasively (Danilov and Tyler, 2005). In the application of electro-tactile stimulation (Tyler et al., 2003), the BCI and a head-mounted accelerometer could be served as a vestibular substitution that uses the unique patterns of electrotactile stimulation on the tongue for restoration of head-body postural coordination. Most types of electro-tactile stimulation are percutaneous (direct stimulation of the nerves) and transcutaneous (through the skin) stimulations. A major disadvantage of the percutaneous type of electro-tactile stimulation is the additional distress or pain to the patient. High voltage stimulation is required in stimulation through the skin without any insertion leads because of high impedance of the dry skin. This can be considered as a disadvantage of the transcutaneous electro-tactile stimulation. However the advantage of these types of feedback is the requirement of relatively simple circuits and electrodes placed on the skin, which can considerably reduce both the cost and amount of hardware needed to deliver tactile sensations to a user.
Vibro-Tactile Stimulation
Percept sensation in the residual limb also can be generated using vibro-tactile stimulation elicited by mechanical vibrations of the skin (Tanaka et al., 2015). Different stimulation parameters (i.e., frequency and amplitude of the vibration) result in different types of sensory information such as proprioception (Kaczmarek et al., 1991; Mildren and Bent, 2016). However, other parameters such as duty cycle, shape, and pulse duration also can be used to convey different types of feedback (Cipriani et al., 2012; Dosen et al., 2016). The discriminating values of amplitude thresholds differ by prostheses and interface locations. Several sensory substitutions have been achieved through vibro-tactile stimulation, which includes the mapping of sensation from the prosthetic hand to the phantom hand for transradial amputees (Antfolk et al., 2013b; Papetti et al., 2016), the increase and decrease of texture roughness feeling on fingers (Asano et al., 2015), the sensation through stretching of the skin (Wheeler et al., 2010; Motamedi et al., 2017), the improvement in grasping force (Chatterjee et al., 2008; Cipriani et al., 2008, 2014; Saunders and Vijayakumar, 2011; Montagnani et al., 2015; Witteveen et al., 2015), the evoked sense of proprioception by the vibrations of a tendon (Thyrion and Roll, 2010), an object manipulation (Rombokas et al., 2013), the sense of embodiment (D'Alonzo et al., 2015; Ko et al., 2015), and the sensation on the surface of the skin (Kangas et al., 2017). The early devices were heavy and energy consuming. However, with the help of sophisticated electronics, tactile vibrators are now low powered and easily used for prosthetic applications. Generally, the use of vibrotactile feedback improves the user performance through a better control of grip forces and by lowering the number of errors in task execution. Unfortunately, due to the lack of intuitiveness and usability, the users don't feel comfortable with these types of indirect feedback systems.
Modality-Matched Feedback
The most recent non-invasive technique used for conveying sensory information is the modality-matched feedback (see the mechano-tactile stimulation below): The input sensory stimulus must be the same modality as that of the sensory output. For example, for the sensation produced by touching, the prosthesis is required to be perceived as touch. This methodology is closer to naturally generated percepts. In theory, the coupling of non-invasive electro-mechanical devices with thermoelectric devices (e.g., Peltier cells) has made it possible to regain modality-matched touch sensations including contact, vibration, texture, temperature as well as normal and shear force/pressure. These coupled devices can be applied on the skin (Davalli et al., 2000), to the residual limb (Antfolk et al., 2012; Bjorkman et al., 2016), and to the chest and other body parts (Panarese et al., 2009) for achieving modality-matched touch sensations. This technique as applied for restoration of proprioception is inherently a challenge for engineers and researchers, as the angle of the wrist or hand joints is required to be manipulated to another intact joint in order to match the modality.
Mechano-tactile stimulation is utilized as one of the modality-matched techniques, which uses the application of force on a residual limb to evoke sensory feedback. An increase of the force in the prosthesis brings a proportional increase of the force applied to the skin in the residual limb. This technique may be superior to vibro-tactile substitution in that it reduces the grasping force error of prostheses, thereby providing more accurate spatial sensory information (Patterson and Katz, 1992; Antfolk et al., 2013a). However, Antfolk et al. (2013b) explained that the inherent design constraints on the size, weight, and response time of an actuator reduce the impact of this approach. D'Alonzo et al. (2014a,b) used hybrid vibro-electro-tactile stimulation and found it to be an efficient approach for obtaining multi-channel sensory feedback.
Regarding all the modalities noted above, achieving a selective and stable interface remains a challenge. Hence, researchers have looked for other near-to-natural-sensation modalities, among which peripheral implants are popular these days. In the next section, we will review the progress made in the neuroprosthetics using implantable interfaces at neuraxis and we will explore peripheral nerve-machine interfaces used for recording and stimulation.
Invasive Methods of Sensory Feedback
In the indirect methods noted above, complete selectivity and longevity have not yet been achieved. These modalities are still unable to provide selective sensory information in a stable manner. To overcome this issue, the peripheral interface technology has advanced more in recent years, and researchers are now focusing more on invasive technologies (Navarro et al., 1998, 2005). Tyler and Durand (1997) focused on the fact that for those amputees who only lost sensory end organs (limbs), the peripheral nerves connecting the brain to the lost organs retain the normal functionality. Thus, through a synthetic activation of these pathways, perception of sensation can be achieved (Micera and Navarro, 2009). There are several locations at which the interaction with the residual somatosensory system can be established (Weber et al., 2012). Direct stimulation on the nerve has earned extensive popularity in providing sensory feedback to prostheses. Preclinical works on the approaches related to the brain (Bensmaia and Miller, 2014), dorsal root ganglion (Weber et al., 2006, 2011; Bruns et al., 2013), and intra-spinal microstimulation (Gaunt et al., 2006; Capogrosso et al., 2016) are progressing.
Neural Interface and Advantages
In the twenty first century, two important developments have transformed the field of neuroprosthetics. The first is the improvement in bionic arms (or prosthetic limbs) that can replicate the functions of a natural human arm. The second is the enhancement of algorithms that decode the intended movements of an amputee from neuronal activities in the motor cortex area of the brain. By combining these innovations, it is now possible for a human patient to perform tasks with a bionic arm by thoughts alone (Collinger et al., 2013; Wodlinger et al., 2015). Most existing interfaces are either from the nerve or the cortex (Tabot et al., 2015). The restoration of somatosensation, either from cortical stimulation or peripheral-nerve stimulation, is required for effective bidirectional communication and a sense of feeling or embodiment (Dornfeld et al., 2016) for the patient. The essential need for touch in everyday life has led several research groups to develop different techniques for its artificial restoration. The necessary methodology entails stimulation of the peripheral nerve or a somatosensory area of the brain (S1) with trains of electrical pulses to evoke percepts that transmit information from the grasping object (Downey et al., 2016). Amputees or patients that use a bionic limb controlled through such a bidirectional peripheral-nerve-interface perceive the prosthesis as an integral part of themselves rather than as a piece of hardware attached to their arm (Marasco et al., 2011; Limerick et al., 2014; D'Alonzo et al., 2015). The dexterity of a bionic arm can be improved through the restoration of somatosensation by stimulating the peripheral nerve, because in some cases such a manipulation of an object, visual feedback is considered to be a poor substitute (Bensmaia, 2015). To obtain such a feedback, several peripheral-nerve-interface approaches have been devised over the past two decades.
As noted above, electrical interfaces can be established anywhere in the cortex of the brain to the end organ. Sometimes interfaces are penetrative and sometimes stimulation can be given externally. In a broad spectrum, evaluation of these interfaces can be based on selectivity and longevity: Longevity is the measure of stable interaction of the electrodes with the same population of sensory afferents over time, and selectivity is the measure of its interaction with specific parts of sensory afferents. For the evaluation purpose, computational models of tactile afferents also have been deployed, which can simulate a population of afferents, in real time, in milliseconds and with precision (Kim et al., 2016). Furthermore, stability can be defined as the duration that the information (related with the activity measured by the electrode) should remain constant over the life of the interface. Greater stability of a stimulating electrode results in a more natural feeling of an artificial touch and is also important in achieving longevity for sensory feedback. Meanwhile, a higher selectivity can be achieved through intra-neural implants and, along with that, stability over a longer period also can be achieved. To increase selectivity, penetrating array type interfaces are used. However, extra-neural implants might stimulate a population of axons.
An interface with a cortical region of the brain faces similar longevity/stability and selectivity challenges, but in some different manner from the nerve case. The fundamental problem in cortical interfaces is longevity (Warren et al., 2016): The neurons (or neuronal tissues) at the implanted surface and the electrodes (implanted interface) degrade over time (McCreery et al., 2010; Prasad et al., 2012; Kane et al., 2013; Chen et al., 2014). These changes can affect the stimulation and recording abilities of the electrodes for sensory feedback or decoding the attempted movements (Perge et al., 2013).
Peripheral nerve stimulation through different interfaces is the hot issue for restoring sensations. In the next section, we will explain the recent advances for peripheral-nerve interfaces. The functional properties, selectivity, and biocompatibility will be discussed along with the advantages and disadvantages of various peripheral-nerve interfaces. The techniques in wide use for restoration of sensory feedback through electrical stimulation will be highlighted, though some other potential stimulation techniques such as the optogenetic modality (targeted neural signaling) (Towne et al., 2013; Warden et al., 2014; Pisanello et al., 2016) and the infrared-light-based technique (Wells et al., 2007; Cayce et al., 2015) are excluded.
Extra-Neural Interfaces
In general, two broad categories of peripheral-nerve-interfaces are extra-neural and intra-neural. The extra-neural (or extra-fascicular) interface with a circular shape that surrounds the peripheral-nerve is a noninvasive method to the nerve itself. The electrodes do not penetrate the protective sheath (perineurium), and thus are less invasive and minimize the disturbances to the neural tissue. The cuff interface is most common among the extra-fascicular types. This electrode configuration can provide several distinct stimulation/recording according to the contacts around the periphery of the nerve. The helical (Agnew et al., 1989) and spiral (Naples et al., 1988) type interfaces have been proven to be stable for decades in clinical applications (Fisher et al., 2009; Polasek et al., 2009). Their circular shape offers a disadvantage of minimal interaction with neural tissues. Tyler and Durand (2002) introduced the Flat Interface Nerve Electrode (FINE), which is another form of extra-neural interface that was developed to increase the surface area without penetrating inside the nerve and to maintain the naturalistic shape of the nerve. Its recording ability (Yoo and Durand, 2005) and selectivity of the periphery of the stimulated nerve have been demonstrated (Schiefer et al., 2013; Ortiz-Catalan et al., 2014). Since the electrodes and the nerve fibers are separated by the perineurium, higher stimulation currents are required to achieve sensations than the case of intra-fascicular interfaces (Grinberg et al., 2008). Leventhal and Durand (2003) have employed a higher value of current resulting in activation at subfascicle level and achieved a low selectivity, and also their results were not repeatable: Hence, a similar tactile perception, which is not a naturalistic pattern of neuronal activation, was evoked. During grasping with an intact hand, every afferent fiber perceived differently and responded as per the object' features that invaded the fiber's receptive field, while electrical stimulation through the electrode sometimes produced a highly unnatural feeling or paresthesia due to synchronous activation of a large population of axons. The temporal modulation of stimulation pulse trains somewhat mitigated the effect of tingling and paresthesia. A stable and selective configuration of FINE and spiral interfaces has been achieved in clinical trials for more than 3 years for individuals with limb loss (Tan et al., 2014, 2015). Selectivity also has been achieved in this way, but only on the periphery of the nerve, not up to the axonal level. The main drawback of extra-neural interfaces is their low selectivity. In fact, being wrapped around the nerve, the electrode records the whole electrical activity of the nerve. As noted above, in order to reach afferent axons from the periphery of a nerve, they must provide high stimulation currents as compared to the intra-neural ones. For these reasons, an intra-fascicular type that penetrates the nerve itself has been introduced.
Intra-Fascicular Interfaces
As the name suggests, an intra-neural interface penetrates the protective sheaths. The least invasive designs such as the groove interface (Koole et al., 1997) and the slowly penetrating inter-fascicular nerve interface (Tyler and Durand, 1997) penetrate only the epineurium. These interfaces physically insert electrical contacts within the nerve. Afferent/efferent fibers are in reach of these types of electrodes, and recording/stimulation is not as difficult to achieve as in case of other modalities. These interfaces, unlike extra-neural ones, tend to have more contacts within the peripheral nerve. Among the most invasive penetrating intra-neural interfaces placed inside the nerve fascicles, there are the Longitudinal Intra-Fascicular Electrode (LIFE) (Yoshida and Horch, 1993; Dhillon et al., 2004; Thota et al., 2015), a conducting wire or polymer filament implanted longitudinally and laid parallel to the nerve fibers, and the Transverse Intra-fascicular Multi-channel Electrode (TIME) (Boretius et al., 2010) having aligned contacts perpendicular to the nerve, respectively. LIFE has some drawbacks that include (i) a fixed distance between the electrode and the nerve which limits its selectivity, (ii) the interface stiffness causes micro-movements that, in the long term, may damage the nerve. The thin film Longitudinal Intra-Fascicular Electrode (tfLIFE) is an upgraded form of LIFE. It has been fabricated on a micro-patterned polyimide substrate, allowing several contacts in one interface. Its flexible structure allows a better adaptation to the nerve shape preventing damage due to an excessive stiffness. Selective interfacing is possible with LIFE and tfLIFE, which acquire signals from a small number of axons (Kundu et al., 2014). They are less invasive than recent multichannel array type interfaces. Selective stimulation and recording have been achieved through TIME at both intra-fascicular and inter-fascicular levels (Badia et al., 2011a, 2016). Also, multi-electrode-array-based interfaces (Byun et al., 2017) have been developed recently to demonstrate its viability for achieving sensorimotor information from the peripheral nerves. These interfaces have great benefits for individuals who have suffered a limb loss. Moreover, another penetrating type of interface is the Utah Slanted Electrode Array (USEA). This type has varying electrode densities according to several designs proposed in the literature with multiple penetration depths (Branner et al., 2001; Ledbetter et al., 2013; Wark et al., 2013). They are inserted perpendicularly into the peripheral nerve leading to a higher risk of nerve damage. They require a lower current for activation of nerve fibers due to their close proximity to axons and fibers; significantly therefore, a small group of fibers can selectively be stimulated (Branner et al., 2004). In this chronic implantation, the stability of an electrode of 80% silicon has been demonstrated for 7-months (Lacour et al., 2016). Davis et al. (2016) have achieved an increased selectivity and a stable sensory feedback by chronically implanted USEA in the median and ulnar nerves of human, which leads to an intuitive and dexterous control of prosthetic fingers with sensory feedback in the future for bidirectional prosthetic control. Coordinated grasp and sensory responses by stimulating the peripheral nerves of a monkey was demonstrated with the implantation of USEA. Moreover, with short-term implantation of intra-fascicular electrodes, increasing stimulation thresholds have been observed (Boretius et al., 2010; Rossini et al., 2010). The reported number of sensory perceptions and the locations evoked by intra-neural interfaces are very similar to those of the long-term extra-neural approaches, which led Grill et al. (2009) to conclude that the two approaches are equivalent. One of their disadvantages is the tendency to damage the nerve by penetration, which reduces the long-term stability. Nerve stimulation and excitation generated in the motor fibers can cause contraction of residual muscles, which may result in an obstructed control of bionic arms/prostheses.
Regenerative Peripheral-Nerve Interface (RPNI)
RPNI consists of an electrode and a residual peripheral nerve, which is neurotized by transacting the nerve and inserting the electrode in between them; it is an internal interface for signal transmission with the external electronics of a prosthetic limb. Effectively, it is a sieve made of silicon, ceramic or polymer with a large number of fine holes that is placed inside the transected nerve. The increase in growth rate of regeneration of individual or a group of fibers through those holes can activate selective stimulation and make it possible to record action potentials (Micera and Navarro, 2009; Sando et al., 2016) from individual axons or a small group. In principle, a high number of selective contacts can be achieved by reducing the size of the sieve along with an increased number of fine holes (Lago et al., 2007a). The neurotized interface serves as a biological amplifier and provides a long-lasting site for implantation. Studies revealed that the RPNI undergoes robust regeneration, neurotization, and revascularization (Urbanchek et al., 2016), and that signals are reliably transduced across the interface with longevity and reproducibility. Polyimide-based sieve electrodes have been shown to be biocompatible (Stieglitz et al., 1997) and stable over several months for in vivo implantation and testing (Navarro et al., 1998). Further experimentation with the interface revealed the stability and formation of new neuromuscular junctions within the muscles for improved function in amputees. Kung et al. (2014) proposed a new interface strategy to harness motor commands from transected peripheral nerves for control of a prosthesis. Also they demonstrated the 7-month viability of the RPNI. On the other hand, stimulation of a small number of regenerated fibers was shown feasible using the regenerative electrodes. By matching the regenerated nerve fascicles with the original receptive fields, an adequate feedback might be delivered. By targeting the fiber growth for a specific feedback of perception from a distinct neural population, there might be a possibility of generating a different fiber population that might not be required for the receptive field (Lotfi et al., 2011). Sensory feedback has been achieved from tactile and force sensors embedded in the prosthesis by stimulation of appropriate afferent fibers in the transected nerves. Another methodology to attain feedback has been proposed by Nghiem et al. (2015), which can be used for transferring sensory signals from a prosthetic sensor to the residual nerve. In this method, an insulated electrode placed on the surface of a muscle was used to stimulate the muscle, which then depolarized the afferent nerve within the muscle to provide sensory feedback. This new design can eliminate the problem of direct nerve stimulation that is inherent in intra-neural interfaces and also limits potential peripheral nerve damage. This sensory RPNI has the ability to transduce distinguishable and graded sensory signals across the peripheral nerve when being stimulated electrically. Although RPNI is still in an early stage of development, this technique has the capability to access sensory pathways and provide stable sensory feedback. A disadvantage of this modality is that the functional use of electrodes might require several months due to nerve regeneration. Furthermore, the nerve might degenerate with time, which will lead to a substantial loss of stimulation ability of the implanted electrode (Lago et al., 2005). A number of useful results have been obtained using the RPNI technique in experimental models (Ceballos et al., 2002; Ursu et al., 2016). However, some challenges remain, which hinder its clinical usability. The most important breakthrough of regenerative electrodes will be their implantation in the peripheral nerves of an amputee for bidirectional interfaces.
Targeted Reinnervation (TR)
TR is a nerve-machine interface that has been developed to make prosthetic control and feedback more intuitive. This method is considered to be fully neither invasive nor non-invasive; it lies in between due to the surgical requirement for its implementation. It has demonstrated a success in improving the motor control signals for both transhumeral and shoulder disarticulation amputation (Dumanian et al., 2009). It has also shown promising to sensory outcomes by using rerouted residual median, radial, and ulnar nerves. Instead of a direct electrical interface with the residual nerves in the arm, those in the arm can be moved to reinnervate the intact muscles in the chest, see Figure 3. This can help the transmission of sensory feedback and the attainment of electromyographic (EMG) signals by surface electrodes from the reinnervated site to control the prosthetic limb (Kung et al., 2013; Cheesborough et al., 2015). The EMG signals corresponding to the intended movement of a patient are generated from muscle contractions of redirected nerves. When the target skin of these patients was touched, they felt as if their missing limb was being touched (Kuiken et al., 2007a; Marasco and Kuiken, 2010). Studies of two amputees also have demonstrated a touch perception that aroused in the target skin: The amputees had a strong impression that the sensations arising from stimulation of the reinnervated skin site were projected to their missing limb. Furthermore, the sensory afferents remained stable for years after surgery. This methodology is being expanded in the field, evoking cutaneous or tactile sensations (Hebert et al., 2016) from the skin of reinnervated muscles. Using TR, sensation in the hand as evoked by a reinnervated chest skin along with other senses like touch, temperature, pain, and a limited graded force discrimination have been demonstrated (Kuiken et al., 2007b; Marasco et al., 2009, 2011; Schultz et al., 2009; Sensinger et al., 2009). There is a possibility of generating tactile feedback, but this technique is not naturalistic in restoring proprioception.
The advantages of TR technique, as stated by Hebert et al. (2014a,b), are as follows: (i) A long-term stable interface is possible, (ii) after rerouting of the nerves, there is no additional surgical procedure, (iii) the body is free of implanted interfaces, (iv) electrical stimulation evokes sensation to the reinnervated skin patch, and (v) there is no paresthesia or tingling. A series of results for patients who have undergone TR are; (i) non-invasive stimulation at the innervated site resulting in a generation of perceived sensory information (a cutaneous sensation) in the median nerve of the hand, (ii) effective detection of touch, pain, temperature, and proprioception to some extent, and (iii) stable reinnervated area for the detection of graded forces. TR does not only help in providing sensory feedback but also increases the size of the sites that can be used to control the prosthetic hand (Kuiken et al., 2004). However, Kristeva-Feige et al. (1996) have explained that there is a difficulty in restoring both sensory and motor control simultaneously because sensory-afferent signals are suppressed by motor-efferent commands. The proximity of the sites of sensory feedback and motor control can be considered as the major disadvantage of the TR technique. Also, the tactor array used for elicitation of sensory feedback at the TR location has only a limited ability in producing a wide range of sensation. The required calibration routine for taking the tactor array on and off has made this option rather difficult for daily use.
Biocompatibility and Neural Recording/Stimulation
Long-term stability and selectivity can be obtained if implanted interfaces are bio-integrated (or biocompatible) with the peripheral nerve. Foreign body reaction developed around the implants can affect the signal to noise ratio of the recorded motor commands and change the stimulation threshold values for sensory feedback. Therefore, existing interfaces and their fabrication have been considered as a practical choice for a prosthesis that intends to the recovery of sensorimotor abilities with characterization of long-term usability and biocompatibility. Such interfaces have offered a high-resolution means to access the information from the peripheral nerve by processing the multi-unit recordings and stimulations. Electrical stimulation of a single or a micro-electrode array has required current pulsations for eliciting action potentials. However, this approach is required to fulfill some conditions; (i) specifically designed interfaces for peripheral nerves, (ii) invasive enough to reach a target axon, (iii) ability to communicating bi-directionally, (iv) selective and stable electrical interfacing, (v) minimal tissue damages and influence of foreign body responses, and (vi) most importantly a biocompatible and biostable interface. Several types of interfaces proposed in the literature showed different material and chemical properties. Out of which, polymer based neural interface is popular. A conducting polymer is often used as a coating material on electrodes to increase charge-injection capacity for neural stimulation and to get high signal-to-noise ratio (SNR) for neural recording. Polypyrrole (PPy) and poly (3,4-ethylenedioxythiophene, PEDOT) are the most widely used polymer coatings for neural electrodes due to their biocompatibility. Different types of interfaces have been tested by several research groups on animals for checking their biocompatibility, recording and stimulating abilities for instance; Polyimide-based intra-neural implants (Wurth et al., 2017), USEA (Christensen et al., 2014), tfLIFE and tripolar cuff (Qiao et al., 2016), stretchable polymeric multi-electrode array (Guo et al., 2014), TIME-2 and TIME-3 with different chemical properties (Badia et al., 2011b), LIFE (Zheng et al., 2008), polyimide, platinum intra-fascicular electrodes (Lago et al., 2007b), and polymer-based longitudinal intra-fascicular electrodes polyLIFEs (Malmstrom et al., 1998; Lawrence et al., 2002).
As noted in the previous sections, several research groups have tested the capacities of intra- and extra-fascicular interfaces by implantation in the ulnar and median nerves to selectively evoke sensation in amputees through multiple electrodes. LIFE, TIME, FINE and the most recently used USEA have demonstrated interfacing capability with peripheral-nerves for sensory feedback. The details of the interface configurations and stimulation parameters in each human study are tabulated in Table 1. The artificial sensory signals are transmitted to the peripheral nerves by implanting electrodes providing a stimulated current that is proportional to the original input. By means of real-time sensory feedback, the amputee is able to move a prosthetic arm, apply a grip force via the recording of fine motor commands, and also feel a sensation without any audio and visual aids. Some studies have shown such ability of an amputee to differentiate objects according to their perceived characteristics (e.g., size, shape, and stiffness) and execute motor outputs such as grip strength (Horch et al., 2011; Raspopovic et al., 2014). The ability of object recognition and simultaneous encoding of sensory information for manipulation of grip forces are the excitatory developments for amputees. However, its widespread applicability is reduced by the limited number of studies and participants to date. Given the limited long-term data, it is significant to consider the physical conditions of the electrodes implanted in the peripheral nerves along with their capacity to provide a long-term stable interface (Yoshida et al., 2010). Structural changes of nerves can occur due to implantation. Fibrosis can hinder the response of an electrode and constantly reduce its performance. Implantation of tf-LIFE 4 in humans has shown a complete termination of sensory detection after 10 days of stimulation, which was due to the foreign-body response of tissue fibrosis (Rossini et al., 2010).
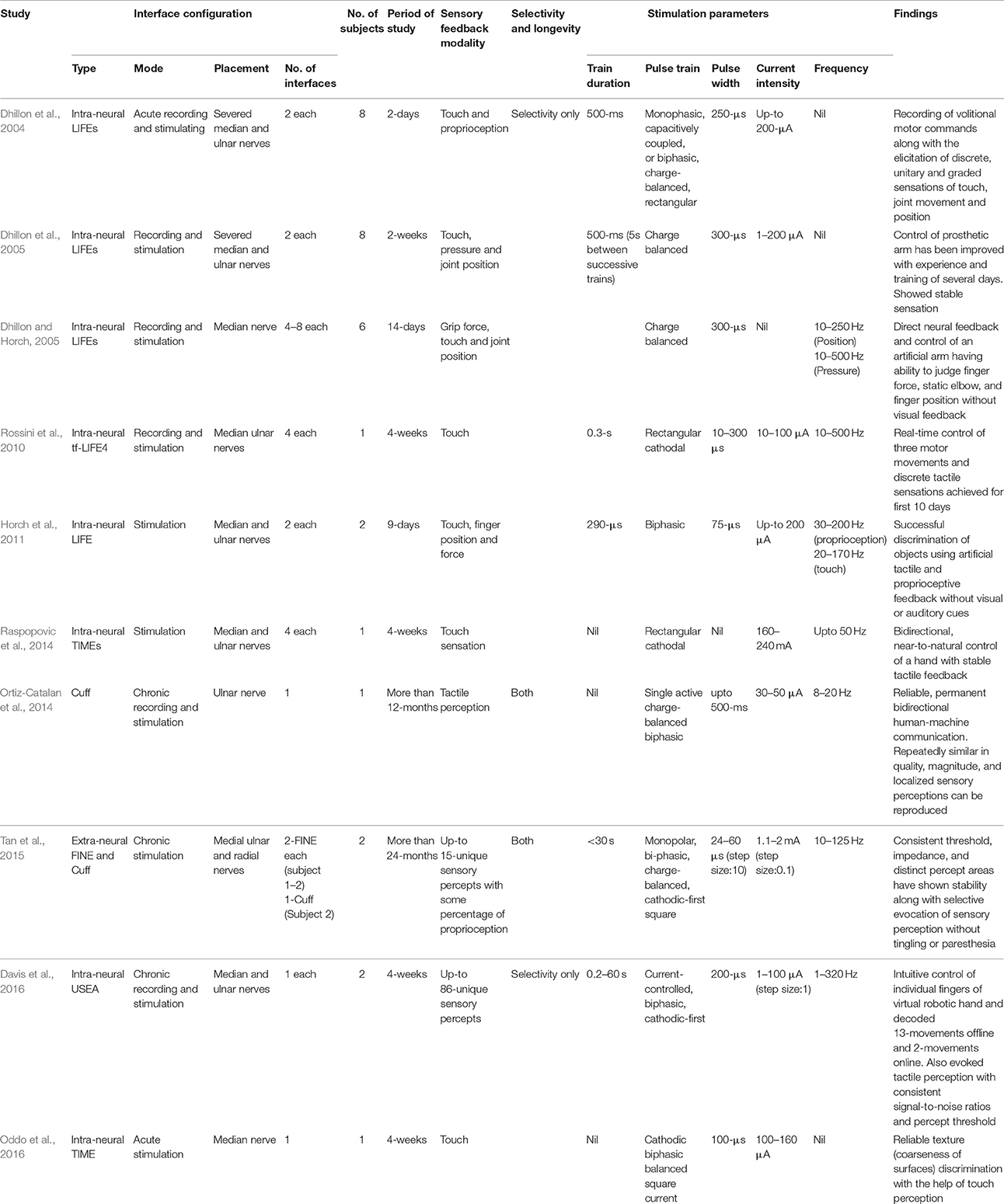
Table 1. Summary of human neural implant studies and modalities for restoration of sensory feedback.
Additionally, some cortical implants like the Utah Electrode Array and the customized multi-channel stimulator for a cortical visual neuroprosthesis can be used for blinds (Ferrandez et al., 2007). Such stimulators have been proven to inject current (charge) in a safe and precise way in an acute animal experimentation. Another implant is the planar multi-electrode array for recording from a rat auditory cortex and visualizing a spatiotemporal structure of the cortical activities (Tsytsarev et al., 2006). The key to a further development of neuroprosthetics is to record simultaneous single-unit, multi-unit, and local field potential activity from multiple brain sites. Most recent study by Pothof et al. (2016) described the fabrication process of a chronic neural probe that has similar material and geometrical properties with that of clinical probes that enable recording of a single neuron activity at a high spatial resolution. These probes have successfully recorded single unit activities up to 26 days from the brain of a monkey that suggests its potential usefulness on human applications. Guitchounts et al. (2013) demonstrated the viability of long-term recording using a carbon-fiber electrode array, which have provided stable multi-unit recordings over a time-scale of months.
It is evident from above discussions that more research into long-term interfacing implants for bidirectional control of prostheses is required. In the following section, some parameters that can help in selective neural stimulation for elicitation of distinct and graded sensations (sensory feedback) are discussed.
Characteristics of Stimulation
Spikes are responsible for conveying information in the axons of a nerve, and the spike rate or frequency is involved in transmitting sensorimotor commands in a single axon (Tyler, 2015). Electrical stimulation in the form of spikes has been given to the residual stump nerve of a patient through typically implanted electrodes, and psychophysical judgments and verbal reports were gathered (Polasek et al., 2009; Schiefer et al., 2016). Stimulation through different electrodes is one way of manipulating evoked sensory percepts. Different populations of afferents can be activated through these individual electrodes, which can have distinct receptive field locations (Saal and Bensmaia, 2014) and different sub-modality compositions of sensory afferents (mix of slowly adapting (SA), rapidly adapting (RA), and pacinian corpuscles (PC) afferents). Thus, by evoking sensations through different electrodes at different locations in a phantom or residual limb, the field of projections of active fibers, which is the area where sensation is felt, can be determined. These sensations can also be determined through a sub-modality composition of activated fibers. For instance, if afferent populations of SA, RA, or PC fibers are stimulated, the evoked sensation will be of pressure, flutter, or vibration, respectively (Torebjork et al., 1987).
Moreover, the pulse shape is of extreme importance. The electrical pulse is transmitted to the nerve fiber using implanted electrodes. The field of pulse magnitude decays as the distance from the implanted electrode increases. Rattay and Aberham (1993) concluded that nerve activation is proportional to the rate of change of the voltage along the axons. Therefore, large, myelinated and closer axons can be activated by square pulses before small, unmyelinated and distant axons. A ramp pulse can be used to activate distal axons before closer ones (Grill and Mortimer, 1997; Grill et al., 2009), while a quasi-trapezoidal pulse have been employed for recruitment of smaller axons before larger ones (Fang and Mortimer, 1991). In implanted devices, exponential-shaped waveforms, utilizing minimal energy, can be used to minimize the power requirement for activation of larger and closer axons (Wongsarnpigoon and Grill, 2010; Krouchev et al., 2014).
As explained above, providing stimulation using different electrodes can elicit sensation of several different qualities. In addition to the closeness of intra-neural interfaces physically, another powerful tool is the design and manipulation of the spatiotemporal electro-magnetic field using extra-neural interfaces. These interfaces, with the help of multiple electrodes (Schiefer et al., 2008), generate an electrical field, the variation of which can change the activation areas of sensory percepts on an artificial hand/arm (using controlled inputs). The standard nerve stimulation paradigm is a train of identical, charge-balanced, square electrical pulses characterized by pulse amplitude, pulse width, and pulse repetition period (or inter-pulse interval). Traditionally, these three parameters are time-invariant and fixed in value. The intensity of a pulse can be manipulated in three different ways by varying pulse width (Tan et al., 2014), pulse frequency (Dhillon et al., 2005), and pulse amplitude (Raspopovic et al., 2014). It ranges from the lowest charge that can evoke a sensation all the way up to the value that elicits unnatural sensation (or paresthesia). By alteration of these stimulation parameters, sensations of varying quality can be evoked. The details of stimulation parameters used in different human studies for elicitation of sensory feedback are tabulated in Table 1.
In somatosensory applications, intensity is proportional to the variation of the stimulation frequency, and the resulting sensory perceptions can be changed with bursting pulse trains (Tan et al., 2015). In the patterned frequency paradigm, synchronous activity in a population of axons can be generated by maintaining the strength of the stimulation pulse constant. However, this cannot help in distinguishing complex sensory information. For generation of a patterned fiber activity, several modalities such as patterned field distribution of stimulation and patterned stimulation intensity have been employed. In each paradigm, non-synchronous activity in a population of axons is generated by creating a shift in the field between stimulation pulses. This information, if controlled properly, can be utilized for the restoration of several different somatosensory percepts. The nerve stimulation has been shown to induce different types of sensations; for instance, proprioceptive and touch sensations. Complex qualities of touch such as vibration on the skin, tingling, tapping, stinging, brushing, and itch have been observed during experimentation (Tan et al., 2014). Stimulation can infrequently evoke proprioceptive sensations, for example, sensations of movement of a finger or joint or a specific hand configuration. Systematic study of percept qualities and gradedness of these sensations have not been completed yet. Data on contact forces between the perceptually available object and the skin are required for grasping and manipulating an object (Johansson and Flanagan, 2009), because too much force is likely to damage the prosthesis or the object, while too little force can cause slippage (Witney et al., 2004). In previous studies, contact force has been manipulated through the intensity of stimulation; hence the greater intensity and the greater contact force.
Restoration of Somatosensory Feedback through Cortical Stimulation
Although this review has focused more on peripheral-nerve recording, stimulation, and the methods adopted for encoding, we will briefly address the stimulation issue in the cortical region for restoration of somatosensory feedback. The field of neuroprosthetics has reached an age of maturity. When similar questions need to be addressed, different strategies are pursued (Courtine and Bloch, 2015). Elicitation of percept by electrical stimulation was first demonstrated by Wilder Penfield in 1937. They showed that somatosensory cortex (S1) neurons are organized into separate columns representing different regions of the body (Penfield and Boldrey, 1937). They later induced tactile sensation by applying electrical stimulation to the somatosensory area of a neurosurgical patient (Rasmussen and Penfield, 1947). The locations of percept areas on a body are systematically the same as those required on the cortical surface of the brain for stimulation (Rasmussen et al., 2009). The neurons that can react to similar types of stimuli have their functional columns in area S1. Studies also have found evidence for S1 regions that encode sensory information for individual digits of a hand along with different types of receptors within those digits (Merzenich et al., 1983; Sur et al., 1984).
Intra-cortical microstimulation (ICMS) has been applied to primates having the pulse frequency corresponding to the evoked perception of cutaneous flutter (Romo et al., 1998), which depicts the temporal configuration of pulse-train shape for elicitation of different sensations in a systematic mode. Recently, several studies have demonstrated the characteristics of ICMS by varying the parameters of stimulation for sensory feedback and recording of voluntary movements in primates (Fitzsimmons et al., 2007; O'Doherty et al., 2011; Zaaimi et al., 2013; Higo et al., 2016) and rodents (Fridman et al., 2010). Another important breakthrough is the first bidirectional brain-machine interface in which a signal from the motor cortex of a non-human primate was able to control the cursor, while stimulation is applied simultaneously to the brain area S1 to give sensory feedback on the movements, though the primate had to go through a learning process for mapping the afferent interface (Rajan et al., 2015; Tabot et al., 2015). These research advances have highlighted several methods of evoking broader ranges of near-to-natural sensations of touch and proprioception (Blank et al., 2010), with the hope that they will prove applicable to individuals who have lost limbs and/or senses. Somatosensation including proprioception is an essential element of natural motor abilities. Without having the sense of proprioception, it is difficult to plan a dynamic limb movement (Sainburg et al., 1995); indeed, an experiment on a monkey with a created lesion in S1 showed uncoordinated finger movements (Liu and Rouiller, 1999).
Many research groups are trying to use biomimetic approaches to achieve the sense of touch and proprioception integral to everyday behavior (Saal and Bensmaia, 2015). However, at present, large-scale neuronal activation relating specifically to the elicitation of detailed desired responses is not possible, due to the sensitivity of the brain. In other studies, ICMS pulse trains have been transferred through implanted electrodes (UEAs) in a monkey for restoration of sensory feedback (Bensmaia and Miller, 2014; Chen et al., 2014; Kim et al., 2015). In one study on rodents, the implementation of a biomimetic approach was tested by applying ICMS to the barrel cortex using micro-wires for the generation of artificial tactile percepts to navigate around a virtual target on the screen (Venkatraman and Carmena, 2011; O'Connor et al., 2013). With the help of this artificial sensation, the rats were able to find the virtual objects in order to obtain a reward with improved accuracy after training. Another experiment was conducted, in which the task was to detect the pulses of ICMS over a variable time interval. The rats were able to differentiate between pulses of ICMS and distractor mechanical stimuli to replicate the target stimulus.
ICMS can also be employed to deliver localized tactile percepts with natural features as well as in human-brain-computer interface applications for better control using area S1 (Collinger et al., 2013; Wodlinger et al., 2015; Downey et al., 2016). An experiment on the biomimetic method in non-human primates was conducted by delivering ICMS to area S1 in order to convey sensory information on the location of a contact in the hand; it was found that the receptive field of ICMS was highly localized to elicit percepts on the hand or even fingertips (Tabot et al., 2013). Various combinations of receptive fields can be used to signal which area of a prosthetic limb is in-contact with an object. The response of a sensor placed on the prosthesis can be used to activate neurons in area S1 with corresponding receptive fields. These approaches and advances can be served as a basis for improved somatosensory feedback in amputees (or tetraplegic patients). In patients, either a limb is missing or its connection with the brain has been lost, though the brain area responsible for tactile stimulation or sensory feedback for the corresponding limb is still intact (Flesher et al., 2016). These patients often feel a sensation at the residual limb (Ramachandran and Hirstein, 1998). One approach is to ask the patient how and where he/she feels sensation while delivering ICMS; then, the force with which an object is grasped with a prosthesis can be controlled by sending feedback through pulse trains of ICMS that elicits percepts of the correct magnitude (Berg et al., 2013). Task-specific somatosensory feedback has been generated in humans using cortical stimulation via electrocorticographic electrodes (Cronin et al., 2016). In the future, patients will be able to learn the meaning behind each combination of stimulation patterns with several types of sensory modalities and, by using that information, operate the prosthesis in more natural and effective ways. Above all, this method of evoking sensation is sensitive for an amputee, as all surgery and stimulation requirements are directly related to the brain, which is the most sensitive human organ, especially for those who have suffered from sense and/or limb loss. Therefore, peripheral interfaces have an edge over cortical interfaces.
Current Prosthetic Technology
A substantial progress has been made over the past few decades in the neuroprosthetics technology. There are two types of prosthetics: Myoelectric- and body-powered prosthetics. Body-powered prosthetics have the advantage over myoelectric-powered ones, because an amputee can touch or feel the interaction with objects through the body harness operating the prosthesis (Carey et al., 2015). Battery-powered myoelectic prostheses, on the other hand, are based on muscle signals generated from the residual limb of an amputee through surface electrodes. These signals are transmitted to the actuators of the prosthetic limb to perform programmed movements. To evaluate prosthesis user functionality, several protocols have been developed (Chadwell et al., 2016). Pattern recognition control of myoelectric prostheses is also getting popularity due to its better classification of EMG signals (user intentions) using various methods, i.e., linear discriminant analysis classifier, tacit learning system, deep learning with convolutional neural network (Adewuyi et al., 2016; Atzori et al., 2016; Fani et al., 2016; Oyama et al., 2016), etc. Among the most recent achievements is the battery-powered myoelectric prosthesis with 16 degrees of freedom comparable to natural hand motions (Cipriani et al., 2011). Nonetheless, they provide less sensory feedback than body-powered prostheses. Myoelectric devices have relied only on auditory and visual sensory feedback, while body-powered devices rely on contrast and pressure, and the grip-strength information is transmitted through the hand using a cable and interfacing system. In some cases, the sensory feedback might not be the same as provided by the user's visual experience, but does provide a sense of control and connection to the device. Fifty percent of users do still prefer body-powered prostheses, possibly for improved feedback or referred sensation (Biddiss et al., 2007). The developments in volitional motor control have outpaced the advancements in sensory feedback for better control of prosthetic arms (Parri et al., 2017). Optimization of sensory feedback can provide better results, which include the restoration of a sense of touch that allows amputees to experience the world in a natural way and that also helps in allowing the bionic arm/prosthesis to be incorporated into their body image (Tyler, 2015). Along with visual feedback for monitoring of motor commands, the prosthesis must have tactile and proprioception feedback. Feeling the prosthesis as a part of his/her body has strong psychological benefits for patients. In any case, choosing prosthetics is always a tradeoff between sensation and function.
In comparison to EMG based control, the BCI is also getting popularity for control of prostheses in a non-invasive method. Electroencephalography (EEG) and functional near-infrared spectroscopy (fNIRS) are two major modalities used for BCI (Hong and Khan, 2017). EEG-based BCI mostly uses steady state visual evoked potentials and P300 for control applications (Turnip et al., 2011; Turnip and Hong, 2012). In comparison to EEG, fNIRS has lower temporal resolution. Still fNIRS-based BCI are effectively used for control applications (Santosa et al., 2013, 2014; Hong and Santosa, 2016). To overcome the issue of lower temporal resolution in fNIRS (Bhutta et al., 2015), hybridization (Khan and Hong, 2017), initial dip based feature extraction (Hong and Naseer, 2016; Zafar and Hong, 2017), and bundled-optode schemes are introduced for rehabilitation (Nguyen and Hong, 2016; Nguyen et al., 2016). However, the BCI modalities only decode the brain signals using active, passive, and reactive tasks (Khan and Hong, 2015; Hong et al., 2017; Liu and Hong, 2017). The current BCI systems do not have the ability to decode movement intention, instead they use imagination based tasks for control (Hong and Nguyen, 2014; Khan et al., 2014; Hong et al., 2015). Thus, for an amputee, the invasive nerve implants may be a more effective solution to BCI in comparison with EMG-based control.
The technology has been transferred to applications involving direct interfacing with peripheral nerves for intuitive control and sensory feedback. Different peripheral nerves can be activated through selective electrical stimulation. Hence, tactile or position sensations can be selectively and focally elicited without associated pain, while motor signals to extrafusal fibers are recorded more easily than those to intrafusal. The advancement in new peripheral interfaces has capitalized on this property to evoke touch perception without eliciting pain. Repeated clinical demonstration with replicated results has been achieved for peripheral interfaces (Schiefer et al., 2016). Bensmaia (2015) and Tyler (2015) concluded that many challenges are ahead before selective activation of all receptors. For instance, thermoreceptors for temperature feedback without simultaneous activation of others are required to be achieved yet. Several options are still required to be explored for nerve-machine interfaces (Rutten, 2002).
Discussion
Upper-extremity amputations are usually associated with significant disabilities. Daily living activities are either no longer possible or require additional effort and time. This high level of disability serves to emphasize the obligation of the engineering research community to investigate, develop, and achieve innovative technologies for upper-limb prosthetics that can improve the quality of life. In fact, several advances already have been made in the upper-limb prosthetic technologies: battery- and body-powered, mechanically complex, and multifunctional prosthetic arms are now commercially available: However, there remains a lack of truly effective control and sensory-feedback interfaces.
As alluded to in the previous sections, natural sensory feedback in prostheses is highly desirable for amputees. A stable sensory-feedback system can enhance the control of prostheses. In fact, for a clinical use, a stable interface that is capable of selectively evoking several sensory percepts at several locations is indispensable. However, challenges to produce selective stimulation for chronically stable and long-lasting clinical applications for using peripheral nerve interfaces are still remaining. Although selectivity can be achieved through interfaces that penetrate the nerve for stimulation of one-to-one axons, this is with the cost of increased invasiveness. Additionally, whereas the modern prosthetic technology has achieved greater selectivity through regenerative, intra-fascicular and microelectrode-array-type interfaces, the problems are to test its biocompatibility and long-term stable recruitment in humans. These technologies have advanced the field of neuromodulation, but most human studies shown in Table 1 are of one or less than 1 month duration, which are helpful only in providing initial findings/data. Desirable features of peripheral nerve interfaces are low stimulation currents, selectivity, longevity, and long-term stability for stimulation/recording. These properties depend on the proximity of the exposed electrode surface to the target nerve fibers. Stability and selectivity have been achieved for longer periods of time, for instance, more than 3 years using the cuff-type interface (Tan et al., 2015). Therefore, more experimentation and changes in the design of implanted interfaces can provide selectively long-term graded sensation, tactile perception, and a sense of embodiment to improve the quality of prostheses. The non-invasive techniques in prostheses have been widely used in myoelectric (battery-) and body-powered prostheses. Their major advantage is the indirectness in communicating with the amputee (or patient), which means that they are non-invasive. However, these types of prostheses also have a severe disadvantage in that they lack wide sensory feedback. Some other modalities, such as the electro-tactile, vibro-tactile, and modality-matched feedback types discussed earlier do not provide appropriate feedback to an amputee and are less intuitive. In these cases, usability of these prostheses is decreased and the cognitive workload of the patient or amputee often increases.
With these considerations in mind, several groups have started developing invasive-type interfaces for prostheses that acquire signals and also provide sensory feedback directly from/to the peripheral nerves, which provides the amputee with a sense of perception and embodiment. An ideal bidirectional nerve-machine interface for prosthetic applications was shown in Figure 1. For its achievement, several methodologies have long been studied, based on which the most important goal is to design an interface that is stable and biocompatible and that can selectively acquire signals from the peripheral-nerve. The most recent study of Wurth et al. (2017) has demonstrated the selectivity, stability, longevity, and biocompatibility of intra-neural type interfaces. Additionally, the same nerve-machine interface is responsible for providing sensory feedback through stimulation that depends upon the area of the prosthetic hand that is in contact with an object. As noted above, several types of interfaces necessary for the completion of this nerve-machine interface have been designed, tested, and reported in the literature.
We propose a hybrid scheme in Figure 4, whereby two interfaces are added at a time. It is evident, from the fact indicated in Table 1, that LIFE has achieved better selectivity at the fascicular level in the peripheral-nerve (Dhillon et al., 2004, 2005) and that long-term stability and selectivity has demonstrated using FINE (Ortiz-Catalan et al., 2014; Tan et al., 2014). Like LIFE, TIME has also demonstrated greater selectivity in the peripheral nerve (Boretius et al., 2010; Badia et al., 2011b; Kundu et al., 2014; Oddo et al., 2016). Although USEA has showed better selectivity, it incurs a nerve-damage risk greater than that with LIFE or TIME, due to its approximately 96 microelectrodes that penetrate the nerve. It is pertinent to mention here that TIME and LIFE have multiple electrodes in one direction only, while USEA has multiple electrodes in the 2D plane. As noted in the previous sections, a lower current is required with TIME, LIFE, and USEA, while more current is required with FINE to elicit sensory feedback. By using a combination of these interfaces, which is to say hybrid FINE-LIFE or hybrid FINE-TIME, both selectivity and longevity, with increased numbers of stable sensory percepts and sensations, can be achieved. Because of the penetrating nature of LIFE and TIME, they can provide contacts at the axonal level, and FINE may not damage the nerve, as demonstrated clinically in previous studies. FINE's more-than-3-year stable implantation and sensory percepts in humans has showed its viability, although it obtained only 15–20 sensory percepts without tingling or paresthesia. For an increased number of sensory percepts at different locations in a hand, multiple electrodes were required as demonstrated by Davis et al. (2016). This type of interface configuration, with multiple-electrodes inside the nerve, incurs a greater risk of nerve damage as well as biocompatibility issues. In this strategy for achieving selectivity at the fascicular or axonal level, the implantation of TIME and LIFE, along with the combination of FINE, will prove beneficial in providing more sensory percepts selectively with an increased number of recording contacts for bidirectional control of prosthesis. Although clinical demonstration of this concept has not yet, to the authors' best knowledge, been achieved, it might prove useful in delivering better results for wide, distinct and stable sensory feedback in the neuroprosthetic technology.
Conclusion
In this paper, we have reviewed the state of the art of peripheral-nerve-machine interfaces for restoration of sensory feedback. We have also discussed all of the invasive and non-invasive modalities of bionic/prosthetic arms for intuitive control and sensory feedback. The ultimate goal of all these interfaces is to provide a prosthesis with the ability to serve as an actual near-to-natural-limb replacement. Such prosthesis must be able to perform routine tasks and provide discrete sensation to the amputee. The lack of sensory feedback in myoelectric prostheses or those incorporating indirect methods of stimulation for sensation is a key limitation relative to the achievement of full control. Many researchers have tried several methods for providing sensory feedback. Among them, the peripheral-nerve-machine interface has become widely popular by providing selective and long-term stable feedback with more or less invasive type interfaces. Ultimately, the proposed hybrid LIFE-FINE or hybrid TIME-FINE interfaces based on the reviewed studies will provide an improved selectivity and longevity for bidirectional control of bionic arms.
Author Contributions
UG conducted the literature survey and wrote the first draft of the manuscript. SK participated in revising the manuscript. KH has conceived the idea, corrected the manuscript, and finalized the work. All the authors have approved the final manuscript.
Funding
This work was supported by the National Research Foundation (NRF) of Korea under the Ministry of Science and ICT, Korea (grant no. NRF-2017R1A2A1A17069430 and NRF-2017R1A4A1015627).
Conflict of Interest Statement
The authors declare that the research was conducted in the absence of any commercial or financial relationships that could be construed as a potential conflict of interest.
References
Adewuyi, A. A., Hargrove, L. J., and Kuiken, T. A. (2016). Evaluating EMG feature and classifier selection for application to partial-hand prosthesis control. Front. Neurorobotics 10:15. doi: 10.3389/fnbot.2016.00015
Agnew, W. F., McCreery, D. B., Yuen, T. G., and Bullara, L. A. (1989). Histologic and physiologic evaluation of electrically stimulated peripheral nerve: considerations for the selection of parameters. Ann. Biomed. Eng. 17, 39–60. doi: 10.1007/BF02364272
Antfolk, C., Bjorkman, A., Frank, S. O., Sebelius, F., Lundborg, G., and Rosen, B. (2012). Sensory feedback from a prosthetic hand based on air-mediated pressure from the hand to the forearm skin. J. Rehabil. Med. 44, 702–707. doi: 10.2340/16501977-1001
Antfolk, C., D'Alonzo, M., Controzzi, M., Lundborg, G., Rosen, B., Sebelius, F., et al. (2013a). Artificial redirection of sensation from prosthetic fingers to the phantom hand map on transradial amputees: vibrotactile versus mechanotactile sensory feedback. IEEE Trans. Neural Syst. Rehabil. Eng. 21, 112–120. doi: 10.1109/TNSRE.2012.2217989
Antfolk, C., D'Alonzo, M., Rosen, B., Lundborg, G., Sebelius, F., and Cipriani, C. (2013b). Sensory feedback in upper limb prosthetics. Expert Rev. Med. Devices 10, 45–54. doi: 10.1586/ERD.12.68
Asano, S., Okamoto, S., and Yamada, Y. (2015). Vibrotactile stimulation to increase and decrease texture roughness. IEEE Trans. Hum. Mach. Syst. 45, 393–398. doi: 10.1109/THMS.2014.2376519
Atzori, M., Cognolato, M., and Muller, H. (2016). Deep learning with convolutional neural networks applied to electromyography data: a resource for the classification of movements for prosthetic hands. Front. Neurorobotics 10:9. doi: 10.3389/fnbot.2016.00009
Badia, J., Pascual-Font, A., Vivo, M., Udina, E., and Navarro, X. (2010). Topographical distribution of motor fascicles in the sciatic-tibial nerve of the rat. Muscle Nerve 42, 192–201. doi: 10.1002/mus.21652
Badia, J., Boretius, T., Andreu, D., Azevedo-Coste, C., Stieglitz, T., and Navarro, X. (2011a). Comparative analysis of transverse intrafascicular multichannel, longitudinal intrafascicular and multipolar cuff electrodes for the selective stimulation of nerve fascicles. J. Neural Eng. 8:036023. doi: 10.1088/1741-2560/8/3/036023
Badia, J., Boretius, T., Pascual-Font, A., Udina, E., Stieglitz, T., and Navarro, X. (2011b). Biocompatibility of chronically implanted transverse intrafascicular multichannel electrode (TIME) in the rat sciatic nerve. IEEE Trans. Biomed. Eng. 58, 2324–2332. doi: 10.1109/TBME.2011.2153850
Badia, J., Raspopovic, S., Carpaneto, J., Micera, S., and Navarro, X. (2016). Spatial and functional selectivity of peripheral nerve signal recording with the transversal intrafascicular multichannel electrode (TIME). IEEE Trans. Neural Syst. Rehabil. Eng. 24, 20–27. doi: 10.1109/TNSRE.2015.2440768
Bhutta, M. R., Hong, M. J., Kim, Y.-H., and Hong, K.-S. (2015). Single-trial lie detection using a combined fNIRS-polygraph system. Front. Psychol. 6:709. doi: 10.3389/fpsyg.2015.00709
Boldt, R., Gogulski, J., Guzman-Lopez, J., Carlson, S., and Pertovaara, A. (2014). Two-point tactile discrimination ability is influenced by temporal features of stimulation. Exp. Brain Res. 232, 2179–2185. doi: 10.1007/s00221-014-3908-y
Berg, J. A., Dammann, J. F. III., Tenore, F. V., Tabot, G. A., Boback, J. L., Manfredi, L. R., et al. (2013). Behavioral demonstration of a somatosensory neuroprosthesis. IEEE Trans. Neural Syst. Rehabil. Eng. 21, 500–507. doi: 10.1109/TNSRE.2013.2244616
Bensmaia, S. J., and Miller, L. E. (2014). Restoring sensorimotor function through intracortical interfaces: progress and looming challenges. Nat. Rev. Neurosci. 15, 313–325. doi: 10.1038/nrn3724
Bensmaia, S. J. (2015). Biological and bionic hands: natural neural coding and artificial perception. Philos. Trans. R. Soc. B Biol. Sci. 370:20140209. doi: 10.1098/rstb.2014.0209
Biddiss, E., Beaton, D., and Chau, T. (2007). Consumer design priorities for upper limb prosthetics. Disabil. Rehabil. Assist. Technol. 2, 346–357. doi: 10.1080/17483100701714733
Bjorkman, A., Wijk, U., Antfolk, C., Bjorkman-Burtscher, I., and Rosen, B. (2016). Sensory qualities of the phantom hand map in the residual forearm of amputees. J. Rehabil. Med. 48, 365–370. doi: 10.2340/16501977-2074
Blank, A., Okamura, A. M., and Kuchenbecker, K. J. (2010). Identifying the role of proprioception in upper-limb prosthesis control: Studies on targeted motion. ACM Trans. Appl. Percept. 7:15. doi: 10.1145/1773965.1773966
Boretius, T., Badia, J., Pascual-Font, A., Schuettler, M., Navarro, X., Yoshida, K., et al. (2010). A transverse intrafascicular multichannel electrode (TIME) to interface with the peripheral nerve. Biosens.Bioelectron. 26, 62–69. doi: 10.1016/j.bios.2010.05.010
Branner, A., and Normann, R. A. (2000). A multielectrode array for intrafascicular recording and stimulation in sciatic nerve of cats. Brain Res. Bull. 51, 293–306. doi: 10.1016/S0361-9230(99)00231-2
Branner, A., Stein, R. B., and Normann, R. A. (2001). Selective stimulation of cat sciatic nerve using an array of varying-length microelectrodes. J. Neurophysiol. 85, 1585–1594.
Branner, A., Stein, R. B., Fernandez, E., Aoyagi, Y., and Normann, R. A. (2004). Long-term stimulation and recording with a penetrating microelectrode array in cat sciatic nerve. IEEE Trans. Biomed. Eng. 51, 146–157. doi: 10.1109/TBME.2003.820321
Bruns, T. M., Wagenaar, J. B., Bauman, M. J., Gaunt, R. A., and Weber, D. J. (2013). Real-time control of hind limb functional electrical stimulation using feedback from dorsal root ganglia recordings. J. Neural Eng. 10:026020. doi: 10.1088/1741-2560/10/2/026020
Byun, D., Cho, S. J., Lee, B. H., Min, J., Lee, J. H., and Kim, S. (2017). Recording nerve signals in canine sciatic nerves with a flexible penetrating microelectrode array. J. Neural Eng. 14:046023. doi: 10.1088/1741-2552/aa7493
Capogrosso, M., Milekovic, T., Borton, D., Wagner, F., Moraud, E. M., Mignardot, J. B., et al. (2016). A brain–spine interface alleviating gait deficits after spinal cord injury in primates. Nature 539, 284–288. doi: 10.1038/nature20118
Carey, S. L., Lura, D. J., and Highsmith, M. J. (2015). Differences in myoelectric and body-powered upper-limb prostheses: systematic literature review. J. Rehabil. Res. Dev. 52, 247–262. doi: 10.1682/JRRD.2014.08.0192
Cayce, J. M., Wells, J. D., Malphrus, J. D., Kao, C., Thomsen, S., Tulipan, N. B., et al. (2015). Infrared neural stimulation of human spinal nerve roots in vivo. Neurophotonics 2:015007. doi: 10.1117/1.NPh.2.1.015007
Ceballos, D., Valero-Cabre, A., Valderrama, E., Schuttler, M., Stieglitz, T., and Navarro, X. (2002). Morphological and functional evaluation of peripheral nerve fibers regenerated through polyimide sieve electrodes over long term implantation. J. Biomed. Mater. Res. 60, 517–528. doi: 10.1002/jbm.10099
Cheesborough, J. E., Smith, L. H., Kuiken, T. A., and Dumanian, G. A. (2015). Targeted muscle reinnervation and advanced prosthetic arms. Semin. Plast. Surg. 29, 062–072. doi: 10.1055/s-0035-1544166
Chadwell, A., Kenney, L., Thies, S., Galpin, A., and Head, J. (2016). The reality of myoelectric prostheses: understanding what makes these devices difficult for some users to control. Front. Neurorobotics 10:7. doi: 10.3389/fnbot.2016.00007
Chatterjee, A., Chaubey, P., Martin, J., and Thakor, N. (2008). Testing a prosthetic haptic feedback simulator with an interactive force matching task. J. Prosthet Orthot. 20, 27–34. doi: 10.1097/01.JPO.0000311041.61628.be
Chen, K. H., Dammann, J. F., Boback, J. L., Tenore, F. V., Otto, K. J., Gaunt, R. A., et al. (2014). The effect of chronic intracortical microstimulation on the electrode–tissue interface. J. Neural Eng. 11:026004. doi: 10.1088/1741-2560/11/2/026004
Chen, B., Feng, Y., and Wang, Q. (2016). Combining vibrotactile feedback with volitional myoelectric control for robotic transtibial prostheses. Front. Neurorobotics 10:8. doi: 10.3389/fnbot.2016.00008
Christensen, M. B., Pearce, S. M., Ledbetter, N. M., Warren, D. J., Clark, G. A., and Tresco, P. A. (2014). The foreign body response to the Utah Slant Electrode Array in the cat sciatic nerve. Acta Biomater. 10, 4650–4660. doi: 10.1016/j.actbio.2014.07.010
Cipriani, C., Zaccone, F., Micera, S., and Carrozza, M. C. (2008). On the shared control of an EMG-controlled prosthetic hand: analysis of user–prosthesis interaction. IEEE Trans. Robot. 24, 170–184. doi: 10.1109/TRO.2007.910708
Cipriani, C., Controzzi, M., and Carrozza, M. C. (2011). The SmartHand transradial prosthesis. J. NeuroEng. Rehabil. 8:29. doi: 10.1186/1743-0003-8-29
Cipriani, C., D'Alonzo, M., and Carrozza, M. C. (2012). A miniature vibrotactile sensory substitution device for multifingered hand prosthetics. IEEE Trans. Biomed. Eng. 59, 400–408.
Cipriani, C., Segil, J. L., Clemente, F., and Edin, B. (2014). Humans can integrate feedback of discrete events in their sensorimotor control of a robotic hand. Exp. Brain Res. 232, 3421–3429. doi: 10.1007/s00221-014-4024-8
Courtine, G., and Bloch, J. (2015). Defining ecological strategies in neuroprosthetics. Neuron 86, 29–33. doi: 10.1016/j.neuron.2015.02.039
Clemente, F., D'Alonzo, M., Controzzi, M., Edin, B. B., and Cipriani, C. (2016). Non-invasive, temporally discrete feedback of object contact and release improves grasp control of closed-loop myoelectric transradial prostheses. IEEE Trans. Neural Syst. Rehabil. Eng. 24, 1314–1322. doi: 10.1109/TNSRE.2015.2500586
Collinger, J. L., Wodlinger, B., Downey, J. E., Wang, W., Tyler-Kabara, E. C., Weber, D. J., et al. (2013). High-performance neuroprosthetic control by an individual with tetraplegia. Lancet 381, 557–564. doi: 10.1016/S0140-6736(12)61816-9
Cronin, J. A., Wu, J., Collins, K. L., Sarma, D., Rao, R. P. N., Ojemann, J. G., et al. (2016). Task-Specific somatosensory feedback via cortical stimulation in humans. IEEE Trans. Haptics 9, 515–522, doi: 10.1109/TOH.2016.2591952
D'Alonzo, M., Dosen, S., Cipriani, C., and Farina, D. (2014a). HyVE: hybrid vibro-electrotactile stimulation for sensory feedback and substitution in rehabilitation. IEEE Trans. Neural Syst. Rehabil. Eng. 22, 290–301. doi: 10.1109/TNSRE.2013.2266482
D'Alonzo, M., Dosen, S., Cipriani, C., and Farina, D. (2014b). HyVE-hybrid vibro-electrotactile stimulation-is an efficient approach to multi-channel sensory feedback. IEEE Trans. Haptics 7, 181–190. doi: 10.1109/TOH.2013.52.
D'Alonzo, M., Clemente, F., and Cipriani, C. (2015). Vibrotactile stimulation promotes embodiment of an alien hand in amputees with phantom sensations. IEEE Trans. Neural Syst. Rehabil. Eng. 23, 450–457. doi: 10.1109/TNSRE.2014.2337952
Danilov, Y., and Tyler, M. (2005). Brainport: an alternative input to the brain. J. Integr. Neurosci. 4, 537–550, doi: 10.1142/S0219635205000914
Davalli, A., Sacchetti, R., Fanin, S., Avanzolini, G., and Urbano, E. (2000). Biofeedback for upper limb myoelectric prostheses. Technol. Disabil. 13, 161–172.
Davis, T. S., Wark, H. A. C., Hutchinson, D. T., Warren, D. J., O'Neill, K., Scheinblum, T., et al. (2016). Restoring motor control and sensory feedback in people with upper extremity amputations using arrays of 96 microelectrodes implanted in the median and ulnar nerves. J. Neural Eng. 13:036001. doi: 10.1088/1741-2560/13/3/036001
Dornfeld, C., Swanston, M., Cassella, J., Beasley, C., Green, J., Moshayev, Y., et al. (2016). Is the Prosthetic Homologue Necessary for Embodiment? Front. Neurorobotics 10:21. doi: 10.3389/fnbot.2016.00021
Dosen, S., Markovic, M., Hartmann, C., and Farina, D. (2015). Sensory feedback in prosthetics: a standardized test bench for closed-loop control. IEEE Trans. Neural Syst. Rehabil. Eng. 23, 267–276. doi: 10.1109/TNSRE.2014.2371238
Dosen, S., Ninu, A., Yakimovich, T., Dietl, H., and Farina, D. (2016). A novel method to generate amplitude-frequency modulated vibrotactile stimulation. IEEE Trans. Haptics 9, 3–12. doi: 10.1109/TOH.2015.2497229
Downey, J. E., Weiss, J. M., Muelling, K., Venkatraman, A., Valois, J. S., Hebert, M., et al. (2016). Blending of brain-machine interface and vision-guided autonomous robotics improves neuroprosthetic arm performance during grasping. J. NeuroEng. Rehabil. 13:28. doi: 10.1186/s12984-016-0134-9
Dhillon, G. S., Lawrence, S. M., Hutchinson, D. T., and Horch, K. W. (2004). Residual function in peripheral nerve stumps of amputees: implications for neural control of artificial limbs. J. Hand Surg. 29, 605–615. doi: 10.1016/j.jhsa.2004.02.006
Dhillon, G. S., and Horch, K. W. (2005). Direct neural sensory feedback and control of a prosthetic arm. IEEE Trans. Neural Syst. Rehabil. Eng. 13, 468–472. doi: 10.1109/TNSRE.2005.856072
Dhillon, G. S., Krüger, T. B., Sandhu, J. S., and Horch, K. W. (2005). Effects of short-term training on sensory and motor function in severed nerves of long-term human amputees. J. Neurophysiol. 93, 2625–2633. doi: 10.1152/jn.00937.2004
Dumanian, G. A., Ko, J. H., O'Shaughnessy, K. D., Kim, P. S., Wilson, C. J., and Kuiken, T. A. (2009). Targeted reinnervation for transhumeral amputees: current surgical technique and update on results. Plast. Reconstr. Surg. 124, 863–869. doi: 10.1097/PRS.0b013e3181b038c9
Fang, Z. P., and Mortimer, J. T. (1991). Selective activation of small motor axons by quasitrapezoidal current pulses. IEEE Trans. Biomed. Eng. 38, 168–174. doi: 10.1109/10.76383
Fani, S., Bianchi, M., Jain, S., Neto, J. S. P., Boege, S., Grioli, G., et al. (2016). Assessment of myoelectric controller performance and kinematic behavior of a novel soft synergy-inspired robotic hand for prosthetic applications. Front. Neurorobotics 10:11. doi: 10.3389/fnbot.2016.00011
Ferrandez, J. M., Liaao, E., Bonomini, P., Martinez, J. J., Toledo, J., and Fernandez, E. (2007). A customizable multi-channel stimulator for cortical neuroprosthesis. Conf. Proc. IEEE Eng. Med. Biol. Soc. 2007, 4707–4710. doi: 10.1109/IEMBS.2007.4353390
Fisher, L. E., Tyler, D. J., Anderson, J. S., and Triolo, R. J. (2009). Chronic stability and selectivity of four-contact spiral nerve-cuff electrodes in stimulating the human femoral nerve. J. Neural Eng. 6:046010. doi: 10.1088/1741-2560/6/4/046010
Fisher, L. E., Ayers, C. A., Ciollaro, M., Ventura, V., Weber, D. J., and Gaunt, R. A. (2014). Chronic recruitment of primary afferent neurons by microstimulation in the feline dorsal root ganglia. J. Neural Eng. 11:036007. doi: 10.1088/1741-2560/11/3/036007
Fitzsimmons, N. A., Drake, W., Hanson, T. L., Lebedev, M. A., and Nicolelis, M. A. L. (2007). Primate reaching cued by multichannel spatiotemporal cortical microstimulation. J. Neurosci. 27, 5593–5602. doi: 10.1523/JNEUROSCI.5297-06.2007
Flesher, S. N., Collinger, J. L., Foldes, S. T., Weiss, J. M., Downey, J. E., Tyler.-Kabara, E. C., et al. (2016). Intracortical microstimulation of human somatosensory. Sci. Transl. Med. 8:361ra141. doi: 10.1126/scitranslmed.aaf8083
Flor, H., Denke, C., Schaefer, M., and Grusser, S. (2001). Effect of sensory discrimination training on cortical reorganisation and phantom limb pain. Lancet 357, 1763–1764. doi: 10.1016/S0140-6736(00)04890-X
Fridman, G. Y., Blair, H. T., Blaisdell, A. P., and Judy, J. W. (2010). Perceived intensity of somatosensory cortical electrical stimulation. Exp. Brain Res. 203, 499–515. doi: 10.1007/s00221-010-2254-y
Gaunt, R. A., Prochazka, A., Mushahwar, V. K., Guevremont, L., and Ellaway, P. H. (2006). Intraspinal microstimulation excites multisegmental sensory afferents at lower stimulus levels than local α-motoneuron responses. J. Neurophysiol. 96, 2995–3005. doi: 10.1152/jn.00061.2006
Grill, W. M., and Mortimer, J. T. (1997). Inversion of the current-distance relationship by transient depolarization. IEEE Trans. Biomed. Eng. 44, 1–9. doi: 10.1109/10.553708
Grill, W. M., Norman, S. E., and Bellamkonda, R. V. (2009). Implanted neural interfaces: biochallenges and engineered solutions. Annu. Rev. Biomed. Eng. 11, 1–24. doi: 10.1146/annurev-bioeng-061008-124927
Grinberg, Y., Schiefer, M. A., Tyler, D. J., and Gustafson, K. J. (2008). Fascicular perineurium thickness, size, and position affect model predictions of neural excitation. IEEE Trans. Neural Syst. Rehabil. Eng. 16, 572–581. doi: 10.1109/TNSRE.2008.2010348
Gustafson, K. J., Pinault, G. C., Neville, J. J., Syed, I., Davis, J. A. J. R., Jean-Claude, J., et al. (2009). Fascicular anatomy of human femoral nerve: implications for neural prostheses using nerve cuff electrodes. J. Rehabil Res Dev. 46, 973–984. doi: 10.1682/JRRD.2008.08.0097
Guitchounts, G., Markowitz, J. E., Liberti, W. A., and Gardner, T. J. (2013). A carbon-fiber electrode array for long-term neural recording. J. Neural Eng. 10:046016. doi: 10.1088/1741-2560/10/4/046016
Guo, L., Ma, M. M., Zhang, N., Langer, R., and Anderson, D. G. (2014). Stretchable polymeric multielectrode array for conformal neural interfacing. Adv. Mater. 26, 1427–1433. doi: 10.1002/adma.201304140
Hartmann, C., Dosen, S., Amsuess, S., and Farina, D. (2015). Closed-loop control of myoelectric prostheses with electrotactile feedback: influence of stimulation artifact and blanking. IEEE Trans. Neural Syst. Rehabil. Eng. 23, 807–816. doi: 10.1109/TNSRE.2014.2357175
Hebert, J. S., Elzinga, K., Chan, K. M., Olson, J., and Morhart, M. (2014a). Updates in targeted sensory reinnervation for upper limb amputation. Curr. Surg. Rep. 2:45. doi: 10.1007/s40137-013-0045-7
Hebert, J. S., Olson, J. L., Morhart, M. J., Dawson, M. R., Marasco, P. D., Kuiken, T. A., et al. (2014b). Novel targeted sensory reinnervation technique to restore functional hand sensation after transhumeral amputation. IEEE Trans. Neural Syst. Rehabil. Eng. 22, 765–773. doi: 10.1109/TNSRE.2013.2294907
Hebert, J. S., Chan, K. M., and Dawson, M. R. (2016). Cutaneous sensory outcomes from three transhumeral targeted reinnervation cases. Prosthet. Orthot. Int. 40, 303–310. doi: 10.1177/0309364616633919
Higo, N., Kunori, N., and Murata, Y. (2016). Neural activity during voluntary movements in each body representation of the intracortical microstimulation-derived map in the macaque motor cortex. PLoS ONE 11:e0160720. doi: 10.1371/journal.pone.0160720
Hong, K.-S., and Nguyen, H.-D. (2014). State-space models of impulse hemodynamic responses over motor, somatosensory, and visual cortices. Biomed. Opt. Exp. 5, 1778–1798. doi: 10.1364/BOE.5.001778
Hong, K.-S., Naseer, N., and Kim, Y. H. (2015). Classification of prefrontal and motor cortex signals for three-class fNIRS-BCI. Neurosci. Lett. 587, 87–92. doi: 10.1016/j.neulet.2014.12.029
Hong, K.-S., and Naseer, N. (2016). Reduction of delay in detecting initial dips from functional near-infrared spectroscopy signals using vector-based phase analysis. Int. J. Neural Syst. 26:1650012. doi: 10.1142/S012906571650012X
Hong, K.-S., and Santosa, H. (2016). Decoding four different sound-categories in the auditory cortex using functional near-infrared spectroscopy. Hear. Res. 333, 157–166. doi: 10.1016/j.heares.2016.01.009
Hong, K.-S., and Khan, M. J. (2017). Hybrid brain-computer interface techniques for improved classification accuracy and increased number of commands: A review. Front. Neurorobotics 11:35. doi: 10.3389/fnbot.2017.00035
Hong, K.-S., Bhutta, M. R., Liu, X., and Shin, Y. I. (2017). Classification of somatosensory cortex activities using fNIRS. Behav Brain Res. 333, 225–234. doi: 10.1016/j.bbr.2017.06.034
Horch, K., Meek, S., Taylor, T. G., and Hutchinson, D. T. (2011). Object discrimination with an artificial hand using electrical stimulation of peripheral tactile and proprioceptive pathways with intrafascicular electrodes. IEEE Trans. Neural Syst. Rehabil. Eng. 19, 483–489. doi: 10.1109/TNSRE.2011.2162635
Hsiao, S. S., Fettiplace, M., and Darbandi, B. (2011). Sensory feedback for upper limb prostheses. Prog. Brain Res. 192, 69–81. doi: 10.1016/B978-0-444-53355-5.00005-1
Isakovic, M., Belic, M., Strbac, M., Popovic, I., Dosen, S., Farina, D., et al. (2016). Electrotactile feedback improves performance and facilitates learning in the routine grasping task. Eur. J. Transl. Myol. 26, 197–202. doi: 10.4081/ejtm.2016.6069
Johansson, R. S., and Flanagan, J. R. (2009). Coding and use of tactile signals from the fingertips in object manipulation tasks. Nat. Rev. Neurosci. 10, 345–359. doi: 10.1038/nrn2621
Johansson, R. S., and Vallbo, A. B. (1979). Tactile sensibility in the human hand: relative and absolute densities of four types of mechanoreceptive units in glabrous skin. J. Physiol. 286, 283–300. doi: 10.1113/jphysiol.1979.sp012619
Johnson, K. O., Yoshioka, T., and Vega-Bermudez, F. (2000). Tactile functions of mechanoreceptive afferents innervating the hand. J. Clin. Neurophysiol. 17, 539–558. doi: 10.1097/00004691-200011000-00002
Jorgovanovic, N., Dosen, S., Djozic, D. J., Krajoski, G., and Farina, D. (2014). Virtual grasping: closed-loop force control using electrotactile feedback. Comput. Math Methods Med. 2014:120357. doi: 10.1155/2014/120357
Kaczmarek, K. A., Webster, J. G., Bach-y-Rita, P., and Tompkins, W. J. (1991). Electrotactile and vibrotactile displays for sensory substitution systems. IEEE Trans. Biomed. Eng. 38, 1–16. doi: 10.1109/10.68204
Kangas, J., Rantala, J., Akkil, D., Isokoski, P., Majaranta, P., and Raisamo, R. (2017). Vibrotactile stimulation of the head enables faster gaze gestures. Int. J. Hum. Comp. Studies 98, 62–71. doi: 10.1016/j.ijhcs.2016.10.004
Kane, S. R., Cogan, S. F., Ehrlich, J., Plante, T. D., McCreery, D. B., and Troyk, P. R. (2013). Electrical performance of penetrating microelectrodes chronically implanted in cat cortex. IEEE Trans. Biomed. Eng. 60, 2153–2160. doi: 10.1109/TBME.2013.2248152
Khan, M. J., Hong, M. J., and Hong, K.-S. (2014). Decoding of four movement directions using hybrid NIRS-EEG brain-computer interface. Front. Hum. Neurosci. 8:244. doi: 10.3389/fnhum.2014.00244
Khan, M. J., and Hong, K.-S. (2015). Passive BCI based on drowsiness detection: an fNIRS study. Biomed. Opt. Express 6, 4063–4078. doi: 10.1364/BOE.6.004063
Khan, M. J., and Hong, K.-S. (2017). Hybird EEG-fNIRS-based eight command decoding for BCI: Application to quadcopter control. Front. Neurorobotics 11:6. doi: 10.3389/fnbot.2017.00006
Khasnobish, A., Pal, M., Sardar, D., Tibarewala, D. N., and Konar, A. (2016). Vibrotactile feedback for conveying object shape information as perceived by artificial sensing of robotic arm. Cogn. Neurodyn. 10, 327–338. doi: 10.1007/s11571-016-9386-0
Kim, S., Callier, T., Tabot, G. A., Gaunt, R. A., Tenore, F. V., and Bensmaia, S. J. (2015). Behavioral assessment of sensitivity to intracortical microstimulation of primate somatosensory cortex. Proc. Natl. Acad. Sci. U.S.A. 112, 15202–15207. doi: 10.1073/pnas.1509265112
Kim, S., Callier, T., and Bensmaia, S. J. (2016). A computational model that predicts behavioral sensitivity to intracortical microstimulation. J. Neural Eng. 14:016012. doi: 10.1088/1741-2552/14/1/016012
Ko, C. Y., Chang, Y., Kim, S. B., Kim, S., Kim, G., Ryu, J., et al. (2015). Evaluation of physical and emotional responses to vibrotactile stimulation of the forearm in young adults, the elderly, and transradial amputees. Physiol. Behav. 143, 166–166. doi: 10.1016/j.physbeh.2015.01.034
Koole, P., Holsheimer, J., Struijk, J. J., and Verloop, A. J. (1997). Recruitment characteristics of nerve fascicles stimulated by a multigroove electrode. IEEE Trans. Rehabil. Eng. 5, 40–50. doi: 10.1109/86.559348
Kuiken, T. A., Dumanian, G. A., Lipschutz, R. D., Miller, L. A., and Stubblefield, K. A. (2004). The use of targeted muscle reinnervation for improved myoelectric prosthesis control in a bilateral shoulder disarticulation amputee. Prosthet. Orthot. Int. 28, 245–253. doi: 10.3109/03093640409167756
Kuiken, T. A., Marasco, P. D., Lock, B. A., Harden, R. N., and Dewald, J. P. (2007a). Redirection of cutaneous sensation from the hand to the chest skin of human amputees with targeted reinnervation. Proc. Natl. Acad. Sci. U.S.A. 104, 20061–20066. doi: 10.1073/pnas.0706525104
Kuiken, T. A., Miller, L. A., Lipschutz, R. D., Lock, B. A., Stubblefield, K., Marasco, P. D., et al. (2007b). Targeted reinnervation for enhanced prosthetic arm function in a woman with a proximal amputation: a case study. Lancet 369, 371–380. doi: 10.1016/S0140-6736(07)60193-7
Kung, T. A., Bueno, R. A., Alkhalefah, G. K., Langhals, N. B., Urbanchek, M. G., and Cederna, P. S. (2013). Innovations in prosthetic interfaces for the upper extremity. Plast. Reconstr. Surg. 132, 1515–1523, doi: 10.1097/PRS.0b013e3182a97e5f
Kung, T. A., Langhals, N. B., Martin, D. C., Johnson, P. J., Cederna, P. S., and Urbanchek, M. G. (2014). Regenerative peripheral nerve interface viability and signal transduction with an implanted electrode. Plast. Reconstr. Surg. 133, 1380–1394. doi: 10.1097/PRS.0000000000000168
Kundu, A., Harreby, K. R., Yoshida, K., Boretius, T., Stieglitz, T., and Jensen, W. (2014). Stimulation selectivity of the “thin-film longitudinal intrafascicular electrode”(tfLIFE) and the “transverse intrafascicular multi-channel electrode”(TIME) in the large nerve animal model. IEEE Trans. Neural Syst. Rehabil. Eng. 22, 400–410. doi: 10.1109/TNSRE.2013.2267936
Kristeva-Feige, R., Rossi, S., Pizzella, V., Lopez, L., Erne, S. N., Edrich, J., et al. (1996). A neuromagnetic study of movement-related somatosensory gating in the human brain. Exp. Brain Res. 107, 504–514. doi: 10.1007/BF00230430
Krouchev, N. I., Danner, S. M., Vinet, A., Rattay, F., and Sawan, M. (2014). Energy-optimal electrical-stimulation pulses shaped by the least-action principle. PLoS ONE 9:e90480. doi: 10.1371/journal.pone.0090480
Lacour, S. P., Courtine, G., and Guck, J. (2016). Materials and technologies for soft implantable neuroprostheses. Nat. Rev. Mater. 1:16063. doi: 10.1038/natrevmats.2016.63
Lago, N., Ceballos, D., Rodriguez, F. J., Stieglitz, T., and Navarro, X. (2005). Long term assessment of axonal regeneration through polyimide regenerative electrodes to interface the peripheral nerve. Biomaterials 26, 2021–2031. doi: 10.1016/j.biomaterials.2004.06.025
Lago, N., Udina, E., Ramachandran, A., and Navarro, X. (2007a). Neurobiological assessment of regenerative electrodes for bidirectional interfacing injured peripheral nerves. IEEE Trans. Biomed. Eng. 54, 1129–1137. doi: 10.1109/TBME.2007.891168
Lago, N., Yoshida, K., Koch, K. P., Klaus, P., and Navarro, X. (2007b). Assessment of biocompatibility of chronically implanted polyimide and platinum intrafascicular electrodes. IEEE Trans. Biomed. Eng. 54, 281–290, doi: 10.1109/TBME.2006.886617
Lawrence, S. M., Larsen, J. O., Horch, K. W., Riso, R., and Sinkjaer, T. (2002). Long-term biocompatibility of implanted polymer-based intrafascicular electrodes. J. Biomed. Mater. Res. 63, 501–506. doi: 10.1002/jbm.10303
Ledbetter, N. M., Ethier, C., Oby, E. R., Hiatt, S. D., Wilder, A. M., Ko, J. H., et al. (2013). Intrafascicular stimulation of monkey arm nerves evokes coordinated grasp and sensory responses. J. Neurophysiol. 109, 580–590. doi: 10.1152/jn.00688.2011
Leventhal, D. K., and Durand, D. M. (2003). Subfascicle stimulation selectivity with the flat interface nerve electrode. Ann. Biomed. Eng. 31, 643–652. doi: 10.1114/1.1569266
Limerick, H., Coyle, D., and Moore, J. W. (2014). The experience of agency in human- computer interactions: a review. Front. Hum. Neurosci. 8:643. doi: 10.3389/fnhum.2014.00643
Liu, Y., and Rouiller, E. M. (1999). Mechanisms of recovery of dexterity following unilateral lesion of the sensorimotor cortex in adult monkeys. Exp. Brain Res. 128, 149–159. doi: 10.1007/s002210050830
Liu, X., and Hong, K.-S. (2017). Detection of primary RGB colors projected on a screen using fNIRS. J. Innov. Opt. Health Sci. 10:6. doi: 10.1142/S1793545817500067
Lotfi, P., Garde, K., Chouhan, A. K., Bengali, E., and Romero-Ortega, M. I. (2011). Modality-specific axonal regeneration: toward selective regenerative neural interfaces. Front. Neuroeng. 4:11. doi: 10.3389/fneng.2011.00011
Malmstrom, J. A., McNaughton, T. G., and Horch, K. W. (1998). Recording properties and biocompatibility of chronically implanted polymer-based intrafascicular electrodes. Ann. Biomed. Eng. 26, 1055–1064. doi: 10.1114/1.35
Marasco, P. D., Schultz, A. E., and Kuiken, T. A. (2009). Sensory capacity of reinnervated skin after redirection of amputated upper limb nerves to the chest. Brain 132, 1441–1448. doi: 10.1093/brain/awp082
Marasco, P. D., and Kuiken, T. A. (2010). Amputation with median nerve redirection (targeted reinnervation) reactivates forepaw barrel subfield in rats. J. Neurosci. 30, 16008–16014. doi: 10.1523/JNEUROSCI.4044-10.2010
Marasco, P. D., Kim, K., Colgate, J. E., Peshkin, M. A., and Kuiken, T. A. (2011). Robotic touch shifts perception of embodiment to a prosthesis in targeted reinnervation amputees. Brain 134, 747–758. doi: 10.1093/brain/awq361
McCreery, D., Pikov, V., and Troyk, P. R. (2010). Neuronal loss due to prolonged controlled-current stimulation with chronically implanted microelectrodes in the cat cerebral cortex. J. Neural Eng. 7:036005. doi: 10.1088/1741-2560/7/3/036005
Merzenich, M. M., Kaas, J. H., Wall, J., Nelson, R. J., Sur, M., and Felleman, D. (1983). Topographic reorganization of somatosensory cortical areas 3b and 1 in adult monkeys following restricted deafferentation. Neuroscience 8, 33–55. doi: 10.1016/0306-4522(83)90024-6
Mildren, R. L., and Bent, L. R. (2016). Vibrotactile stimulation of fast-adapting cutaneous afferents from the foot modulates proprioception at the ankle joint. J. Appl. Physiol. 120, 855–864. doi: 10.1152/japplphysiol.00810.2015
Micera, S., Navarro, X., Carpaneto, J., Citi, L., Tonet, O., Rossini, P. M., et al. (2008). On the use of longitudinal intrafascicular peripheral interfaces for the control of cybernetic hand prostheses in amputees. IEEE Trans. Neural Syst. Rehabil. Eng. 16, 453–472. doi: 10.1109/TNSRE.2008.2006207
Micera, S., and Navarro, X. (2009). Bidirectional interfaces with the peripheral nervous system. Int. Rev. Neurobiol. 86, 23–38. doi: 10.1016/S0074-7742(09)86002-9
Montagnani, F., Controzzi, M., and Cipriani, C. (2015). Non-back-drivable rotary mechanism with intrinsic compliance for robotic thumb abduction/adduction. Adv. Robot. 29, 561–571. doi: 10.1080/01691864.2014.992957
Motamedi, M., Florant, D., and Duchaine, V. (2017). Comparing the exteroceptive feedback of normal stress, skin stretch and vibrotactile stimulation for restitution of static events. Front. Robotics AI 4:6. doi: 10.3389/frobt.2017.00006
Nghiem, B. T., Sando, I. C., Gillespie, R. B., McLaughlin, B. L., Gerling, G. J., Langhals, N. B., et al. (2015). Providing a sense of touch to prosthetic hands. Plast. Reconstr. Surg. 135, 1652–1663. doi: 10.1097/PRS.0000000000001289
Naseer, N., and Hong, K.-S. (2013). Classification of functional near-infrared spectroscopy signals corresponding to the right- and left-wrist motor imagery for development of a brain-computer interface. Neurosci. Lett. 553, 84–89. doi: 10.1016/j.neulet.2013.08.021
Naseer, N., and Hong, K.-S. (2015). fNIRS-based brain-computer interfaces: a review. Front. Hum. Neurosci. 9:3. doi: 10.3389/fnhum.2015.00003
Naseer, N., Noori, F. M., Qureshi, N. K., and Hong, K.-S. (2016). Determining optimal feature-combination for LDA classification of functional near-infrared spectroscopy signals in brain-computer interface application. Front. Hum. Neurosci. 10:237. doi: 10.3389/fnhum.2016.00237
Naples, G. G., Mortimer, J. T., Scheiner, A., and Sweeney, J. D. (1988). A spiral nerve cuff electrode for peripheral nerve stimulation. IEEE Trans. Biomed. Eng. 35, 1140–1141. doi: 10.1109/10.8670
Navarro, X., Krueger, T. B., Lago, N., Micera, S., Stieglitz, T., and Dario, P. (2005). A critical review of interfaces with the peripheral nervous system for the control of neuroprostheses and hybrid bionic systems. J. Peripher. Nerv. Syst. 10, 229–258. doi: 10.1111/j.1085-9489.2005.10303.x
Navarro, X., Calvet, S., Rodriguez, C., Blau, C., Buti, M., Valderrama, E., et al. (1998). Stimulation and recording from regenerated peripheral nerves through polyimide sieve electrodes. J. Periph. Nerv. Syst. 3, 91–101.
Nguyen, H.-D., and Hong, K.-S. (2016). Bundled-optode implementation for 3D imaging in functional near-infrared spectroscopy. Biomed. Opt. Express 7, 3491–3507. doi: 10.1364/BOE.7.003491
Nguyen, H.-D., Hong, K.-S., and Shin, Y.-I. (2016). Bundled-optode method in functional near-infrared spectroscopy. PLoS ONE 11:e0165146. doi: 10.1371/journal.pone.0165146
O'Connor, D. H., Hires, S. A., Guo, Z. V., Li, N., Yu, J., Sun, Q. Q., et al. (2013). Neural coding during active somatosensation revealed using illusory touch. Nat. Neurosci. 16, 958–965. doi: 10.1038/nn.3419
Oddo, C. M., Raspopovic, S., Artoni, F., Mazzoni, A., Spigler, G., Petrini, F., et al. (2016). Intraneural stimulation elicits discrimination of textural features by artificial fingertip in intact and amputee humans. Elife 5:e09148. doi: 10.7554/eLife.09148
O'Doherty, J. E., Lebedev, M. A., Ifft, P. J., Zhuang, K. Z., Shokur, S., Bleuler, H., et al. (2011). Active tactile exploration using a brain-machine-brain interface. Nature 479, 228–231. doi: 10.1038/nature10489
Ortiz-Catalan, M., Hakansson, B., and Branemark, R. (2014). An osseointegrated human-machine gateway for long-term sensory feedback and motor control of artificial limbs. Sci. Transl. Med. 6:257re6. doi: 10.1126/scitranslmed.3008933
Oyama, S., Shimoda, S., Alnajjar, F. S. K., Iwatsuki, K., Hoshiyama, M., Tanaka, H., et al. (2016). Biomechanical reconstruction using the tacit learning system: intuitive control of prosthetic hand rotation. Front. Neurorobotics 10:19. doi: 10.3389/fnbot.2016.00019
Panarese, A., Edin, B. B., Vecchi, F., Carrozza, M. C., and Johansson, R. S. (2009). Humans can integrate force feedback to toes in their sensorimotor control of a robotic hand. IEEE Trans. Neural Syst. Rehabil. Eng. 17, 560–567. doi: 10.1109/TNSRE.2009.2021689
Paredes, L. P., Dosen, S., Rattay, F., Graimann, B., and Farina, D. (2015). The impact of the stimulation frequency on closed-loop control with electrotactile feedback. J. NeuroEng. Rehabil. 12:35. doi: 10.1186/s12984-015-0022-8
Parri, A., Martini, E., Geeroms, J., Flynn, L., Pasquini, G., Crea, S., et al. (2017). Whole body awareness for controlling a robotic transfemoral prosthesis. Front. Neurorobotics 11:25. doi: 10.3389/fnbot.2017.00025
Papetti, S., Jarvelainen, H., Giordano, B. L., Schiesser, S., and Frohlich, M. (2016). Vibrotactile sensitivity in active touch: effect of pressing force. IEEE Trans. Haptics. 10, 113–122. doi: 10.1109/TOH.2016.2582485
Patterson, P. E., and Katz, J. A. (1992). Design and evaluation of a sensory feedback system that provides grasping pressure in a myoelectric hand. J. Rehabil. Res. Dev. 29, 1–8. doi: 10.1682/JRRD.1992.01.0001
Patel, G. K., Dosen, S., Castellini, C., and Farina, D. (2016). Multichannel electrotactile feedback for simultaneous and proportional myoelectric control. J. Neural Eng. 13:056015. doi: 10.1088/1741-2560/13/5/056015
Penfield, W., and Boldrey, E. (1937). Somatic motor and sensory representation in the cerebral cortex of man as studied by electrical stimulation. Brain 1x:389. doi: 10.1093/brain/60.4.389
Perovic, M., Stevanovic, M., Jevtic, T., Strbac, M., Bijelic, G., Vucetic, C., et al. (2013). Electrical stimulation of the forearm: a method for transmitting sensory signals from the artificial hand to the brain. J. Automatic. Cont. 21, 13–18. doi: 10.2298/JAC1301013P
Perge, J. A., Homer, M. L., Malik, W. Q., Cash, S., Eskandar, E., Friehs, G., et al. (2013). Intra-day signal instabilities affect decoding performance in an intracortical neural interface system. J. Neural Eng. 10:036004. doi: 10.1088/1741-2560/10/3/036004
Pisanello, F., Sileo, L., and De Vittorio, M. (2016). Micro-and nanotechnologies for optical neural interfaces. Front. Neurosci. 10:70. doi: 10.3389/fnins.2016.00070
Polasek, K. H., Hoyen, H. A., Keith, M. W., Kirsch, R. F., and Tyler, D. J. (2009). Stimulation stability and selectivity of chronically implanted multicontact nerve cuff electrodes in the human upper extremity. IEEE Trans. Neural Syst. Rehabil. Eng. 17, 428–437. doi: 10.1109/TNSRE.2009.2032603
Pothof, F., Bonini, L., Lanzilotto, M., Livi, A., Fogassi, L., Orban, G. A., et al. (2016). Chronic neural probe for simultaneous recording of single-unit, multi-unit, and local field potential activity from multiple brain sites. J. Neural Eng. 13:046006. doi: 10.1088/1741-2560/13/4/046006
Prasad, A., Xue, Q. S., Sankar, V., Nishida, T., Shaw, G., Streit, W. J., et al. (2012). Comprehensive characterization and failure modes of tungsten microwire arrays in chronic neural implants. J. Neural Eng. 9:056015. doi: 10.1088/1741-2560/9/5/056015
Pylatiuk, C., Schulz, S., and Doderlein, L. (2007). Results of an Internet survey of myoelectric prosthetic hand users. Prosthet. Orthot. Int. 31, 362–370. doi: 10.1080/03093640601061265
Qiao, S. Y., Stieglitz, T., and Yoshida, K. (2016). Estimation of the electrode-fiber bioelectrical coupling from extracellularly recorded single fiber action potentials. IEEE Trans. Neural Syst. Rehabil. Eng. 24, 951–960. doi: 10.1109/TNSRE.2015.2489924
Rajan, A. T., Boback, J. L., Dammann, J. F., Tenore, F. V., Wester, B. A., Otto, K. J., et al. (2015). The effects of chronic intracortical microstimulation on neural tissue and fine motor behavior. J. Neural Eng. 12:066018. doi: 10.1088/1741-2560/12/6/066018
Ramachandran, V. S., and Hirstein, W. (1998). The perception of phantom limbs. The DO Hebb lecture. Brain 121, 1603–1630. doi: 10.1093/brain/121.9.1603
Rasmussen, P., Brassard, P., Adser, H., Pedersen, M. V., Leick, L., Hart, E., et al. (2009). Evidence for a release of brain-derived neurotrophic factor from the brain during exercise. Exp. Physiol. 94, 1062–1069. doi: 10.1113/expphysiol.2009.048512
Rasmussen, T., and Penfield, W. (1947). The human sensorimotor cortex as studied by electrical stimulation. Fed. Proc. 6:184.
Raspopovic, S., Capogrosso, M., Petrini, F. M., Bonizzato, M., Rigosa, J., Di Pino, G., et al. (2014). Restoring natural sensory feedback in real-time bidirectional hand prostheses. Sci. Transl. Med. 6:222ra19. doi: 10.1126/scitranslmed.3006820
Rattay, F., and Aberham, M. (1993). Modeling axon membranes for functional electrical stimulation. IEEE Trans. Biomed. Eng. 40, 1201–1209. doi: 10.1109/10.250575
Rombokas, E., Stepp, C. E., Chang, C., Malhotra, M., and Matsuoka, Y. (2013). Vibrotactile sensory substitution for electromyographic control of object manipulation. IEEE Trans. Biomed.Eng. 60, 2226–2232. doi: 10.1109/TBME.2013.2252174
Romo, R., Hernandez, A., Zainos, A., and Salinas, E. (1998). Somatosensory discrimination based on cortical microstimulation. Nature 392, 387–390. doi: 10.1038/32891
Rossini, P. M., Micera, S., Benvenuto, A., Carpaneto, J., Cavallo, G., Citi, L., et al. (2010). Double nerve intraneural interface implant on a human amputee for robotic hand control. Clin. Neurophysiol. 121, 777–783. doi: 10.1016/j.clinph.2010.01.001
Rutkowski, T. M. (2016). Robotic and virtual reality BCIs using spatial tactile and auditory oddball paradigms. Front. Neurorobotics 10:20. doi: 10.3389/fnbot.2016.00020
Rutten, W. L. (2002). Selective electrical interfaces with the nervous system. Annu. Rev. Biomed. Eng. 4, 407–452. doi: 10.1146/annurev.bioeng.4.020702.153427
Saal, H. P., and Bensmaia, S. J. (2014). Touch is a team effort: interplay of submodalities in cutaneous sensibility. Trends Neurosci. 37, 689–697. doi: 10.1016/j.tins.2014.08.012
Saal, H. P., and Bensmaia, S. J. (2015). Biomimetic approaches to bionic touch through a peripheral nerve interface. Neuropsychologia 79, 344–353. doi: 10.1016/j.neuropsychologia.2015.06.010
Sainburg, R. L., Ghilardi, M. F., Poizner, H. O. W. A. R. D., and Ghez, C. L. A. U. D. E. (1995). Control of limb dynamics in normal subjects and patients without proprioception. J. Neurophysiol. 73, 820–835.
Sando, I. C., Leach, M. K., Woo, S. L., Moon, J. D., Cederna, P. S., Langhals, N. B., et al. (2016). Regenerative peripheral nerve interface for prostheses control: electrode comparison. J. Reconstr. Microsurg. 32, 194–199. doi: 10.1055/s-0035-1565248
Santosa, H., Hong, M. J., Kim, S. P., and Hong, K.-S. (2013). Noise reduction in functional near-infrared spectroscopy signals by independent component analysis. Rev. Sci. Instrum. 84:073106. doi: 10.1063/1.4812785
Santosa, H., Hong, M. J., and Hong, K.-S. (2014). Lateralization of music processing auditory cortex: an fNIRS study. Front. Behav. Neurosci. 8:418. doi: 10.3389/fnbeh.2014.00418
Saunders, I., and Vijayakumar, S. (2011). The role of feed-forward and feedback processes for closed-loop prosthesis control. J. NeuroEng. Rehabil. 8:60. doi: 10.1186/1743-0003-8-60
Schiefer, M. A., Triolo, R. J., and Tyler, D. J. (2008). A model of selective activation of the femoral nerve with a flat interface nerve electrode for a lower extremity neuroprosthesis. IEEE Trans. Neural Syst. Rehabil. Eng. 16, 195–204. doi: 10.1109/TNSRE.2008.918425
Schiefer, M. A., Freeberg, M., Pinault, G. J. C., Anderson, J., Hoyen, H., Tyler, D. J., et al. (2013). Selective activation of the human tibial and common peroneal nerves with a flat interface nerve electrode. J. Neural Eng. 10:056006. doi: 10.1088/1741-2560/10/5/056006
Schiefer, M. A., Tan, D., Sidek, S. M., and Tyler, D. J. (2016). Sensory feedback by peripheral nerve stimulation improves task performance in individuals with upper limb loss using a myoelectric prosthesis. J. Neural Eng. 13:016001. doi: 10.1088/1741-2560/13/1/016001
Schultz, A. E., Marasco, P. D., and Kuiken, T. A. (2009). Vibrotactile detection thresholds for chest skin of amputees following targeted reinnervation surgery. Brain Res. 1251, 121–129, doi: 10.1016/j.brainres.2008.11.039
Schweisfurth, M. A., Markovic, M., Dosen, S., Teich, F., Graimann, B., and Farina, D. (2016). Electrotactile EMG feedback improves the control of prosthesis grasping force. J. Neural Eng. 13:056010. doi: 10.1088/1741-2560/13/5/056010
Sensinger, J. W., Schultz, A. E., and Kuiken, T. A. (2009). Examination of force discrimination in human upper limb amputees with reinnervated limb sensation following peripheral nerve transfer. IEEE Trans. Neural Syst. Rehabil. Eng. 17, 438–444. doi: 10.1109/TNSRE.2009.2032640
Stieglitz, T., Beutel, H., and Meyer, J. U. (1997). A flexible, light-weight multichannel sieve electrode with integrated cables for interfacing regenerating peripheral nerves. Sens. Actuator A Phys. 60, 240–243. doi: 10.1016/S0924-4247(97)01494-5
Strbac, M., Belic, M., Isakovic, M., Kojic, V., Bijelic, G., Popovic, I., et al. (2016). Integrated and flexible multichannel interface for electrotactile stimulation. J. Neural Eng. 13:046014. doi: 10.1088/1741-2560/13/4/046014
Sur, M., Wall, J. T., and Kaas, J. H. (1984). Modular distribution of neurons with slowly adapting and rapidly adapting responses in area 3b of somatosensory cortex in monkeys. J. Neurophysiol. 51, 724–744.
Tabot, G. A., Dammann, J. F., Berg, J. A., Tenore, F. V., Boback, J. L., Vogelstein, R. J., et al. (2013). Restoring the sense of touch with a prosthetic hand through a brain interface. Proc. Natl. Acad. Sci. U.S.A. 110, 18279–18284. doi: 10.1073/pnas.1221113110
Tabot, G. A., Kim, S. S., Winberry, J. E., and Bensmaia, S. J. (2015). Restoring tactile and proprioceptive sensation through a brain interface. Neurobiol Dis. 83, 191–198. doi: 10.1016/j.nbd.2014.08.029
Tanaka, Y., Ueda, Y., and Sano, A. (2015). Effect of skin-transmitted vibration enhancement on vibrotactile perception. Exp. Brain Res. 233, 1721–1731. doi: 10.1007/s00221-015-4246-4
Tan, D. W., Schiefer, M. A., Keith, M. W., Anderson, J. R., Tyler, J., and Tyler, D. J. (2014). A neural interface provides long-term stable natural touch perception. Sci. Transl. Med. 6:257ra138. doi: 10.1126/scitranslmed.3008669
Tan, D. W., Schiefer, M. A., Keith, M. W., Anderson, J. R., and Tyler, D. J. (2015). Stability and selectivity of a chronic, multi-contact cuff electrode for sensory stimulation in human amputees. J. Neural Eng. 12:026002. doi: 10.1088/1741-2560/12/2/026002
Thota, A. K., Kuntaegowdanahalli, S., Starosciak, A. K., Abbas, J. J., Orbay, J., Horch, K. W., et al. (2015). A system and method to interface with multiple groups of axons in several fascicles of peripheral nerves. J. Neurosci. Methods 244, 78–84. doi: 10.1016/j.jneumeth.2014.07.020
Thyrion, C., and Roll, J. P. (2010). Predicting any arm movement feedback to induce three-dimensional illusory movements in humans. J. Neurophysiol. 104, 949–959. doi: 10.1152/jn.00025.2010
Torebjork, H. E., Vallbo, A. B., and Ochoa, J. L. (1987). Intraneural microstimulation in man-its relation to specificity of tactile sensations. Brain, 110, 1509–1529.
Towne, C., Montgomery, K. L., Iyer, S. M., Deisseroth, K., and Delp, S. L. (2013). Optogenetic control of targeted peripheral axons in freely moving animals. PLoS ONE 8:e72691. doi: 10.1371/journal.pone.0072691
Tsytsarev, V., Taketani, M., Schottler, F., Tanaka, S., and Hara, M. (2006). A new planar multielectrode array: recording from a rat auditory cortex. J. Neural Eng. 3, 293–298. doi: 10.1088/1741-2560/3/4/006
Turnip, A., Hong, K.-S., and Jeong, M. Y. (2011). Real-time feature extraction of P300 component using adaptive nonlinear principal component analysis. Biomed. Eng. Online 10:83. doi: 10.1186/1475-925X-10-83
Turnip, A., and Hong, K.-S. (2012). Classifying mental activities from EEG-P300 signals using adaptive neural networks. Int. J. Innov. Comp. Inf. Control 8, 6429–6443.
Tyler, D. J., and Durand, D. M. (1997). A slowly penetrating interfascicular nerve electrode for selective activation of peripheral nerves. IEEE Trans. Rehabil. Eng. 5, 51–61. doi: 10.1109/86.559349
Tyler, D. J., and Durand, D. M. (2002). Functionally selective peripheral nerve stimulation with a flat interface nerve electrode. IEEE Trans. Neural Syst. Rehabil. Eng. 10, 294–303. doi: 10.1109/TNSRE.2002.806840
Tyler, M., Danilov, Y., and Bach-y-Rita, P. (2003). Closing an open-loop control system: vestibular substitution through the tongue. J. Integr. Neurosci. 2, 159–164. doi: 10.1142/S0219635203000263
Tyler, D. J. (2015). Neural interfaces for somatosensory feedback: Bringing life to a prosthesis. Curr. Opin. Neurol. 28, 574–581. doi: 10.1097/WCO.0000000000000266
Urbanchek, M. G., Kung, T. A., Frost, C. M., Martin, D. C., Larkin, L. M., Wollstein, A., et al. (2016). Development of a regenerative peripheral nerve interface for control of a neuroprosthetic limb. Biomed Res. Int. 2016:5726730. doi: 10.1155/2016/5726730
Ursu, D. C., Urbanchek, M. G., Nedic, A., Cederna, P. S., and Gillespie, R. B. (2016). In vivo characterization of regenerative peripheral nerve interface function. J. Neural Eng. 13:026012. doi: 10.1088/1741-2560/13/2/026012
Venkatraman, S., and Carmena, J. M. (2011). Active sensing of target location encoded by cortical microstimulation. IEEE Trans. Neural Syst. Rehabil. Eng. 19, 317–324. doi: 10.1109/TNSRE.2011.2117441
Visell, Y. (2009). Tactile sensory substitution: models for enaction in HCI. Interact. Comput. 21, 38–53. doi: 10.1016/j.intcom.2008.08.004
Warden, M. R., Cardin, J. A., and Deisseroth, K. (2014). Optical neural interfaces. Annu. Rev. Biomed. Eng. 16, 103–129. doi: 10.1146/annurev-bioeng-071813-104733
Wark, H. A. C., Sharma, R., Mathews, K. S., Fernandez, E., Yoo, J., Christensen, B., et al. (2013). A new high-density (25 electrodes/mm2) penetrating microelectrode array for recording and stimulating sub-millimeter neuroanatomical structures. J. Neural Eng. 10:045003. doi: 10.1088/1741-2560/10/4/045003
Warren, D. J., Kellis, S., Nieveen, J. G., Wendelken, S. M., Dantas, H., Davis, T. S., et al. (2016). Recording and decoding for neural prostheses. Proc. IEEE 104, 374–391. doi: 10.1109/JPROC.2015.2507180
Weber, D. J., Stein, R. B., Everaert, D. G., and Prochazka, A. (2006). Decoding sensory feedback from firing rates of afferent ensembles recorded in cat dorsal root ganglia in normal locomotion. IEEE Trans. Neural Syst. Rehabil. Eng. 14, 240–243. doi: 10.1109/TNSRE.2006.875575
Weber, D. J., London, B. M., Hokanson, J. A., Ayers, C. A., Gaunt, R. A., Torres, R. R., et al. (2011). Limb-state information encoded by peripheral and central somatosensory neurons: implications for an afferent interface. IEEE Trans. Neural Syst. Rehabil. Eng. 19, 501–513. doi: 10.1109/TNSRE.2011.2163145
Weber, D. J., Friesen, R., and Miller, L. E. (2012). Interfacing the somatosensory system to restore touch and proprioception: essential considerations. J. Mot. Behav. 44, 403–418. doi: 10.1080/00222895.2012.735283
Wells, J., Konrad, P., Kao, C., Jansen, E. D., and Mahadevan-Jansen, A. (2007). Pulsed laser versus electrical energy for peripheral nerve stimulation. J. Neurosci. Methods, 163, 326–337. doi: 10.1016/j.jneumeth.2007.03.016
Wheeler, J., Bark, K., Savall, J., and Cutkosky, M. (2010). Investigation of rotational skin stretch for proprioceptive feedback with application to myoelectric systems. IEEE Trans. Neural Syst. Rehabil. Eng. 18, 58–66. doi: 10.1109/TNSRE.2009.2039602
Witney, A. G., Wing, A., Thonnard, J. L., and Smith, A. M. (2004). The cutaneous contribution to adaptive precision grip. Trends Neurosci. 27, 637–643. doi: 10.1016/j.tins.2004.08.006
Witteveen, H. J., Droog, E. A., Rietman, J. S., and Veltink, P. H. (2012). Vibro-and electrotactile user feedback on hand opening for myoelectric forearm prostheses. IEEE Trans. Biomed. Eng. 59, 2219–2226. doi: 10.1109/TBME.2012.2200678
Witteveen, H. J., Rietman, H. S., and Veltink, P. H. (2015). Vibrotactile grasping force and hand aperture feedback for myoelectric forearm prosthesis users. Prosthet. Orthot. Int. 39, 204–212. doi: 10.1177/0309364614522260
Wijk, U., and Carlsson, I. (2015). Forearm amputees' views of prosthesis use and sensory feedback. J. Hand Ther. 28, 269–278. doi: 10.1016/j.jht.2015.01.013
Wodlinger, B., Downey, J. E., Tyler-Kabara, E. C., Schwartz, A. B., Boninger, M. L., and Collinger, J. L. (2015). Ten-dimensional anthropomorphic arm control in a human brain– machine interface: difficulties, solutions, and limitations. J. Neural Eng. 12:016011. doi: 10.1088/1741-2560/12/1/016011
Wongsarnpigoon, A., and Grill, W. M. (2010). Energy-efficient waveform shapes for neural stimulation revealed with a genetic algorithm. J. Neural Eng. 7:046009. doi: 10.1088/1741-2560/7/4/046009
Wurth, S., Capogrosso, M., Raspopovic, S., Gandar, J., Federici, G., Kinany, N., et al. (2017). Long-term usability and bio-integration of polyimide-based intra-neural stimulating electrodes. Biomaterials 122, 114–129. doi: 10.1016/j.biomaterials.2017.01.014
Xu, H., Zhang, D., Huegel, J. C., Xu, W., and Zhu, X. (2016). Effects of different tactile feedback on myoelectric closed-loop control for grasping based on electrotactile stimulation. IEEE Trans. Neural Syst. Rehabil. Eng. 24, 827–836. doi: 10.1109/TNSRE.2015.2478153
Yem, V., and Kajimoto, H. (2017). Comparative evaluation of tactile sensation by electrical and mechanical stimulation. IEEE Trans. Haptics. 10, 130–134. doi: 10.1109/TOH.2016.2605084
Yoo, P. B., and Durand, D. M. (2005). Selective recording of the canine hypoglossal nerve using a multicontact flat interface nerve electrode. IEEE Trans. Biomed. Eng. 52, 1461–1469. doi: 10.1109/TBME.2005.851482
Yoshida, K., and Horch, K. (1993). Selective stimulation of peripheral nerve fibers using dual intrafascicular electrodes. IEEE Trans. Biomed. Eng. 40, 492–494. doi: 10.1109/10.243412
Yoshida, K., Farina, D., Akay, M., and Jensen, W. (2010). Multichannel intraneural and intramuscular techniques for multiunit recording and use in active prostheses. Proc. IEEE 98, 432–449. doi: 10.1109/JPROC.2009.2038613
Zaaimi, B., Ruiz-Torres, R., Solla, S. A., and Miller, L. E. (2013). Multi-electrode stimulation in somatosensory cortex increases probability of detection. J. Neural Eng. 10:056013. doi: 10.1088/1741-2560/10/5/056013
Zafar, A., and Hong, K.-S. (2017). Detection and classification of three class initial dips from prefrontal cortex. Biomed. Opt. Express 8, 367–383. doi: 10.1364/BOE.8.000367
Zheng, X., Zhang, M., Chen, T., and Chen, Z. (2008). Recording and stimulating properties of chronically implanted longitudinal intrafascicular electrodes in peripheral fascicles in an animal model. Microsurgery 28, 203–209. doi: 10.1002/micr.20465
Keywords: peripheral-nerve, longevity, neuroprosthetics, selectivity, amputation, nerve-machine interfaces, stability, electro-neurographic signals
Citation: Ghafoor U, Kim S and Hong K-S (2017) Selectivity and Longevity of Peripheral-Nerve and Machine Interfaces: A Review. Front. Neurorobot. 11:59. doi: 10.3389/fnbot.2017.00059
Received: 22 April 2017; Accepted: 17 October 2017;
Published: 31 October 2017.
Edited by:
Florian Röhrbein, Technische Universität München, GermanyReviewed by:
Vassiliy Tsytsarev, University of Maryland, Baltimore, United StatesJoel C. Bornstein, University of Melbourne, Australia
Copyright © 2017 Ghafoor, Kim and Hong. This is an open-access article distributed under the terms of the Creative Commons Attribution License (CC BY). The use, distribution or reproduction in other forums is permitted, provided the original author(s) or licensor are credited and that the original publication in this journal is cited, in accordance with accepted academic practice. No use, distribution or reproduction is permitted which does not comply with these terms.
*Correspondence: Keum-Shik Hong, a3Nob25nQHB1c2FuLmFjLmty