- 1Rehab Technologies, Istituto Italiano di Tecnologia, Genoa, Italy
- 2Department of Informatics, Bioengineering, Robotics and Systems Engineering, University of Genoa, Genoa, Italy
One of the current challenges for translational rehabilitation research is to develop the strategies to deliver accurate evaluation, prediction, patient selection, and decision-making in the clinical practice. In this regard, the robot-assisted interventions have gained popularity as they can provide the objective and quantifiable assessment of the motor performance by taking the kinematics parameters into the account. Neurophysiological parameters have also been proposed for this purpose due to the novel advances in the non-invasive signal processing techniques. In addition, other parameters linked to the motor learning and brain plasticity occurring during the rehabilitation have been explored, looking for a more holistic rehabilitation approach. However, the majority of the research done in this area is still exploratory. These parameters have shown the capability to become the “biomarkers” that are defined as the quantifiable indicators of the physiological/pathological processes and the responses to the therapeutical interventions. In this view, they could be finally used for enhancing the robot-assisted treatments. While the research on the biomarkers has been growing in the last years, there is a current need for a better comprehension and quantification of the neuromechanical processes involved in the rehabilitation. In particular, there is a lack of operationalization of the potential neuromechanical biomarkers into the clinical algorithms. In this scenario, a new framework called the “Rehabilomics” has been proposed to account for the rehabilitation research that exploits the biomarkers in its design. This study provides an overview of the state-of-the-art of the biomarkers related to the robotic neurorehabilitation, focusing on the translational studies, and underlying the need to create the comprehensive approaches that have the potential to take the research on the biomarkers into the clinical practice. We then summarize some promising biomarkers that are being under investigation in the current literature and provide some examples of their current and/or potential applications in the neurorehabilitation. Finally, we outline the main challenges and future directions in the field, briefly discussing their potential evolution and prospective.
Introduction
Motor impairment due to neural diseases, such as stroke, is the third most common cause of the global burden of disease according to the WHO following neonatal conditions and heart diseases (WHO, 2019). In 2016, there were 80.1 million prevalent cases and 13.7 million new stroke cases in the world (Johnson et al., 2019). In particular, motor impairment of the upper limb occurs in 73–88% of the first time stroke survivors and in 55–75% of the patients with chronic stroke (Lawrence et al., 2001). The economic impact of this issue represents €60 billion annually only in the European Union, comprising healthcare costs of €27 billion, social care costs of €5 billion, and €16 billion due to the opportunity cost of the informal care by the support system of the patient (family and friends), along with a loss of the productivity costing €12 billion caused by the morbidity or death (Luengo-Fernandez et al., 2020).
Growing efforts have been done to improve the rehabilitation interventions (Frontera et al., 2017; Hayward et al., 2019), which rely on the effective diagnostic of the motor deficit, the accurate evaluation of the recovery or adaptation, and the optimized treatment for the recovery during the chronic stage. For this reason, a wide variety of strategies has been developed for the purpose of the motor restoration (Lin et al., 2019).
For example, stroke rehabilitation usually involves a rehabilitation training program based on a multidisciplinary approach (including physical, occupational, psychological, and speech therapy), which requires the intervention of many specialists (Figure 1, top).
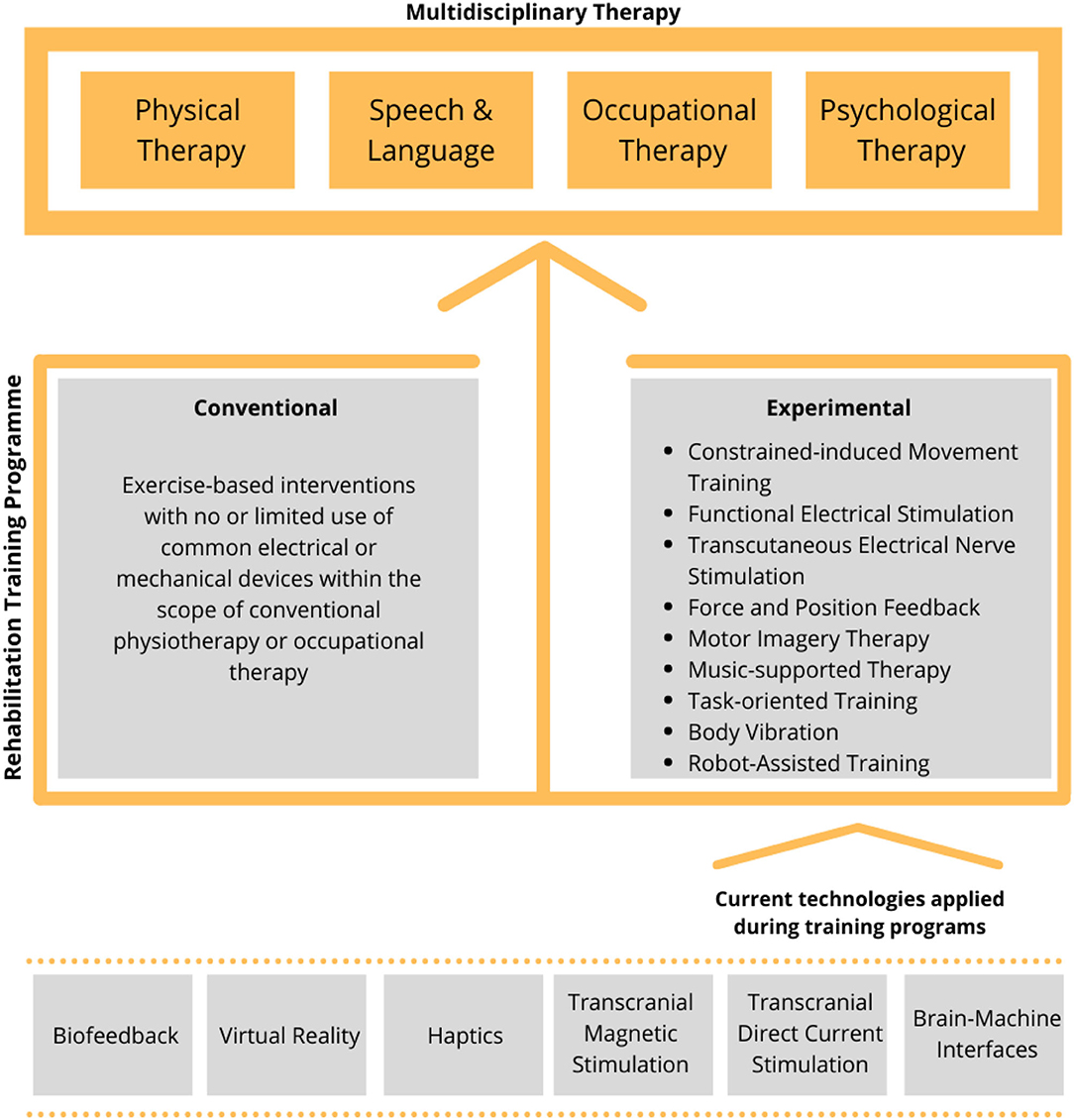
Figure 1. Outline of the current training approaches and technologies used in the rehabilitation. A rehabilitation training program (middle) is used to support the multidisciplinary therapy (top). Rehabilitation training can be either conventional or experimental and the latter being found on one or more available technologies (bottom).
During the rehabilitation intervention, the training program is continuously tuned and monitored to maximize the functional independence of the patient. These programs aim at promoting the motor learning by stimulating the mechanisms of the brain plasticity, especially during the first 3 months following the brain injury when the probability of the function recovery is greater (Prabhakaran et al., 2008). However, there is solid evidence that the mechanisms of the brain plasticity associated to recovery may continue many years after stroke and the chronic patient can also benefit from the rehabilitation interventions (Irimia et al., 2018).
The rehabilitation training itself can be either conventional or experimental (Figure 1, middle) (Lin et al., 2019) and the latter supported by one or more available technologies such as robotics, muscle and brain stimulation, and virtual reality (Figure 1, bottom). In particular, in the recent years, robot-mediated therapy has been increasingly used in the rehabilitation to enable the highly adaptive, repetitive, intensive, and quantifiable physical training (Semprini et al., 2018; Iandolo et al., 2019). Robot-based rehabilitation is mainly supported by the end-effector robots, exoskeletons, and brain–computer interfaces (BCIs) (Figure 2, top panel), used in combination with real-time feedback to the patient, which is based on a feedback technology such as electrical stimulation, haptics, electromyography (EMG)-based assistance, and/or virtual reality (Figure 2, middle panel). The combination of these technologies can be used to create a personalized rehabilitation training program (Figure 2, bottom panel). For a comprehensive review on the current robotic technologies applied on the neurorehabilitation see (Nizamis et al., 2021).
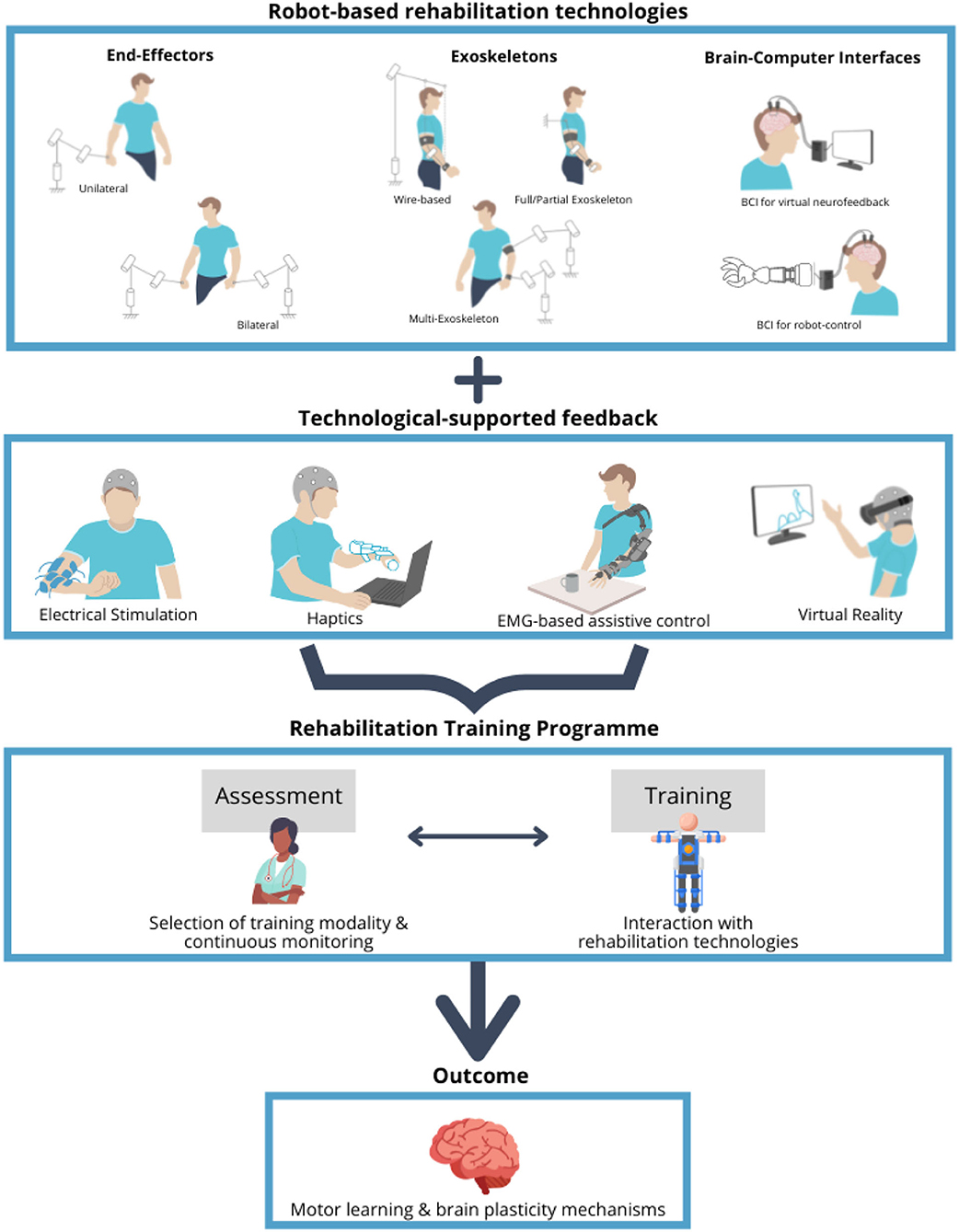
Figure 2. Overview of the robot-based rehabilitation technologies, feedback modalities, and rehabilitation training program. Robot-based rehabilitation technologies (top panel), which include the end-effector robots, exoskeletons, and brain–computer interfaces (BCIs), are used in combination with the feedback modalities (middle panel), ranging from electrical stimulation to haptics, electromyography (EMG)-based assistance, and virtual reality, in order to support the rehabilitation training program (bottom panel). Training program includes the assessment sessions to tune and monitor the specific treatment, aimed at promoting the motor learning by stimulating the mechanisms of the brain plasticity. Schematics in the top panel represent the degrees of freedom of movement for the different types of the end-effector robots and exoskeletons.
What is a Biomarker and its Relevance for Robot-Assisted Rehabilitation?
Many studies have shown that multidisciplinary robot-assisted training results in an additional reduction of motor impairments in comparison to the traditional rehabilitation approach in the different stages of recovery (Franceschini et al., 2020; Khalid et al., 2021). These effects on motor learning are mainly due to the precise feedback and assistance provided to the patients during practice. It has been demonstrated that not only this can improve the motivation of the patient, engagement, and adherence to the treatment, but also enhance the learning and recovery (Schmidt and Young, 1991; Zhang et al., 2017).
Although there are many studies addressing the clinical benefits of these interventions, the comparison of the clinical effectiveness of the robot-assisted training has had diverse results, with some clinical trials showing that the robot-assisted training did not improve motor function when compared with usual care (Rodgers et al., 2019), thus leading to the controversy in the field.
This has been primarily attributed to the individual clinical factors (age, stroke severity, infarct location, and comorbidities) and the unique profile of the patient (Prabhakaran et al., 2008), which lead to the need of tailoring the treatment and developing the useful parameters to interpret the heterogeneous clinical outcomes (Irimia et al., 2018). In this regard, the robot-assisted interventions provide the therapists with the objective, accurate, and repeatable measurements of the functions of the patient, which allow to objectively follow progress, to evaluate the effectiveness of the different treatments, or to adapt to the specific needs of the patients.
These measurements are formally named biomarkers. The term refers to a broad subcategory of the medical signs, which are “indicators of the normal biological processes, pathogenic processes, or responses to an exposure or intervention, including therapeutic interventions accurately and reproducibly measured from outside the patient” (Biomarkers Definitions Working Group, 2001). Thus, a biomarker can be molecular, histologic, radiographic, or physiologic and they can be formally classified according to its alleged application (Figure 3). The use of the biomarkers that have been well-characterized and validated across a variety of treatments and populations has become common in the research and in the clinical practice (Mayeux, 2004).
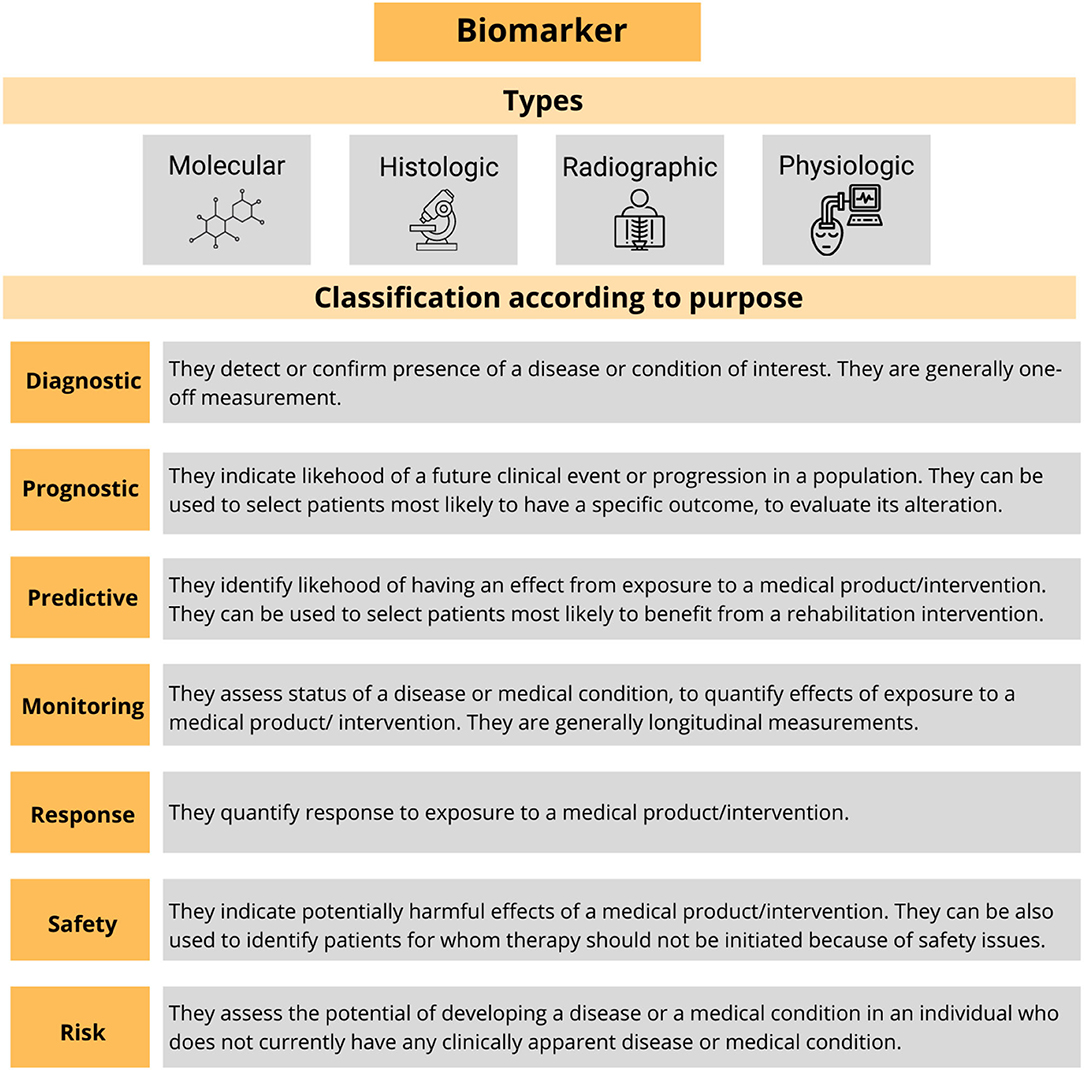
Figure 3. Summary of the types of the biomarkers and their formal classification. Adapted from Biomarkers Definitions Working Group (2001).
Nevertheless, in many cases, the level of evidence for the validation of the biomarkers does not allow their translation to clinical practice. This is the case of motor rehabilitation, where there is a current need for the objective evaluation and the correct prediction of the outcomes by using the robust biomarkers specific to an intervention. Thus, robot-assisted rehabilitation may help to improve the motor rehabilitation after stroke, traumatic brain injury, and the other neurologic disorders.
For example, the randomized controlled trials comparing the robot-assisted arm training with the other rehabilitation or placebo interventions showed improvement of the activities of daily living, arm function, and arm muscle strength in the post-stroke individuals (Mehrholz et al., 2018). However, the huge variations in terms of intensity, duration, amount of training, type of treatment, characteristics of the participant, and measurements used so far suggest caution in the interpretation of these results (Mehrholz et al., 2018). In this regard, the biomarkers might help to harmonize these results by providing more accurate information and helping to identify the proper respondent at the different technologies, enhancing the stratification of the patients. Nevertheless, the majority of this research is still exploratory: while the literature indicates a growing number of the potential biomarkers and indicators for the several pathologies characterized by the motor impairments, a gold standard rehabilitation-focused biomarker is still lacking at the clinical and preclinical levels (Wagner, 2014).
The growing number of clinical studies evaluating the effects of robotic training on rehabilitation generally relies on the traditional human-administered clinical scales, which often lack of resolution to detect subtle changes in the performance of the patient and can be subjective to the expertise of the physician. Recent studies are indicating that these clinical behavioral biomarkers are less predictive of the motor recovery compared to the neurophysiological biomarkers (Cramer et al., 2007; Quinlan et al., 2018; Lim et al., 2020).
Rehabilitation biomarkers are gradually evolving from simple clinical behavioral metrics based on quantitative scales to brain imaging and neurophysiological measurements (Babrak et al., 2019). There are many studies addressing the relationship between the validated clinical scales and instrumented biomarkers (Zollo et al., 2011; Kim et al., 2016; Connell et al., 2018; Do Tran et al., 2018; Saes et al., 2019; Rech et al., 2020; Riahi et al., 2020; Agrafiotis et al., 2021), but a standardized approach is still missing.
In this regard, efforts like the International Classification of Functioning, Disability, and Health (ICF), proposed by the WHO in 2001 (Stucki et al., 2002; World Health Organization, 2002), have been developed as a standardized framework of assessment, with the purpose of providing an integrated biopsychosocial model to describe the functioning in the rehabilitation (Figure 4). This model describes the health condition as influenced by the several factors related not only to the conditions of body structures and functions as a consequence of the impairment, but also to the repercussions on the activities and social participation of the subjects, which are, in turn, related to both the environmental barriers and personal factors. The ICF model allows for an assessment of the degree of disability regardless of the health condition, etiology of the disease, cultural background, age group, and gender (World Health Organization, 2002).
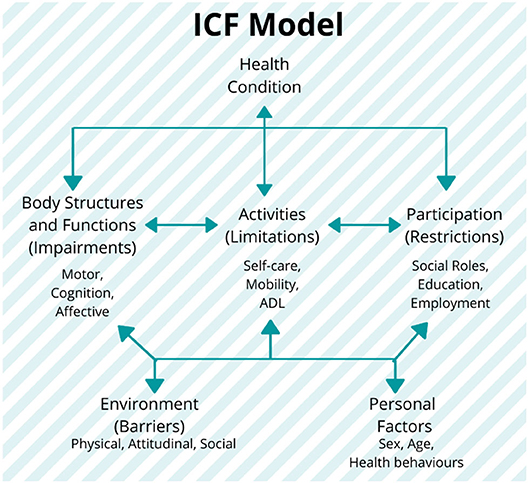
Figure 4. The International Classification of Functioning, Disability, and Health (ICF) model and its components: the model establishes the three levels of human functioning: (1) at the level of body or body part (body structures and functions domain), (2) the whole person (activities domain), and (3) the whole person considered in a social context (participation domain). In this classification, disability implies a certain degree of dysfunction at one or more of these same levels: impairments, activity limitations, and participation restrictions, respectively. It also includes the additional information on the personal and environmental factors (World Health Organization, 2002). Figure is open access courtesy of the National Academies of Sciences (2021) (Trang et al., 2020).
Thus, this framework introduces the need of a standardized and multidisciplinary approach for the development of measurement that can describe and evaluate the motor rehabilitations focusing on the unique (and multidomain) profile of the patient. Currently, this model has been used as a reference for the clinical practice, but its use in the research is still limited, mostly due to a lack of correlation in the literature between the clinical outcome measures and quantitative parameters such as kinematic and neurophysiological measurements. The categorization of these parameters in accordance with the ICF domains and their connection with clinical scales could provide the additional insights for the selection of the appropriate biomarkers and clinical scales in the assessment of the motor performance (see section Toward Personalized Neurorehabilitation: Adopting the Rehabilomics Approaches in the Robot-Assisted Rehabilitation for the further details).
Focus on Stroke: Current Biomarkers Related to Motor Recovery
Among the neurological diseases characterized by motor impairments, stroke is one of the most commonly studied. In this context, viable biomarkers of motor recovery have evolved along with brain imaging and neurophysiological technology in the past decades. While brain imaging techniques such as diffusion tensor imaging (DTI), transcranial magnetic stimulation (TMS), functional MRI (fMRI), and conventional structural MRI (sMRI) have been systematically used for establishing the neurologic biomarkers (Buma et al., 2010; Kim and Winstein, 2017), the neurophysiological techniques [such as electroencephalography (EEG) and surface EMG (sEMG)] and kinematic measurements have been explored mainly in the research contexts (Stinear, 2017). Thus, regardless of the evident evolution, there is a shortfall in the high-level evidence for defining the most critical biomarkers of the motor rehabilitation based on the electrophysiology and kinematics measurements (Kim and Winstein, 2017).
In view of the wide variety of the biomarkers under development and their heterogeneity of the applications in the rehabilitation (depending on the neuroimaging method, condition of the patient, training modality, etc.), the following subsections provide an non-exhaustive overview of the biomarkers for the robot-assisted upper limb rehabilitation post-stroke focused on: (1) sEMG, which has been considered a “muscle activation measurement tool” in the past four decades, leading to a wide exploration in neurorehabilitation (Campanini et al., 2020) (Table 1); (2) EEG, which is widely used in the different clinical areas as non-invasive real-time tool to extract the features from the electrical activity of brain and presents high correlation with the various different pathologies (Table 2); and (3) robotic-based kinematic measurements, which have been extensively explored as a potential tool for assessing the motor functions (Table 3).
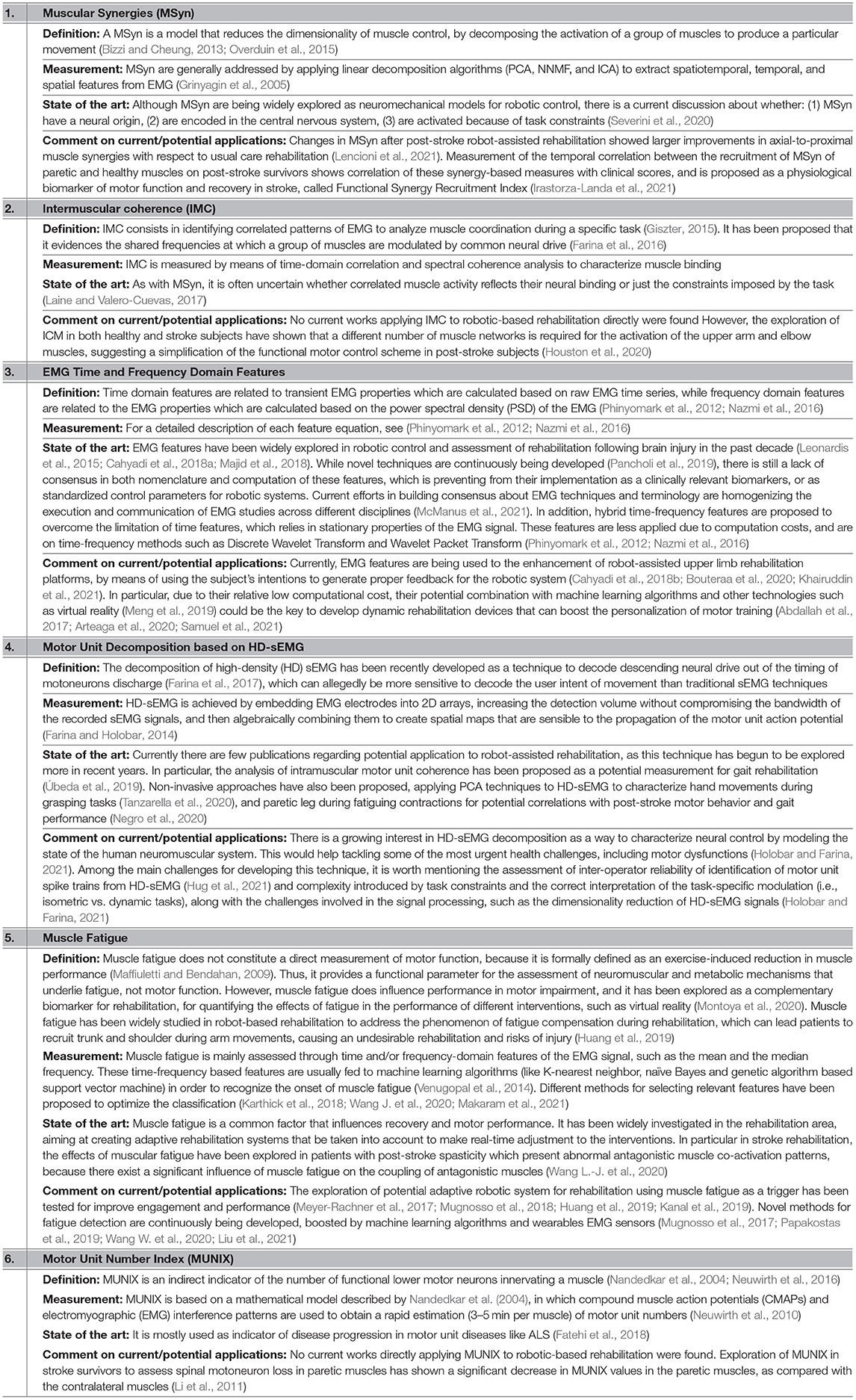
Table 1. List of the electromyography (EMG)-based biomarkers related to the motor rehabilitation focused on stroke.
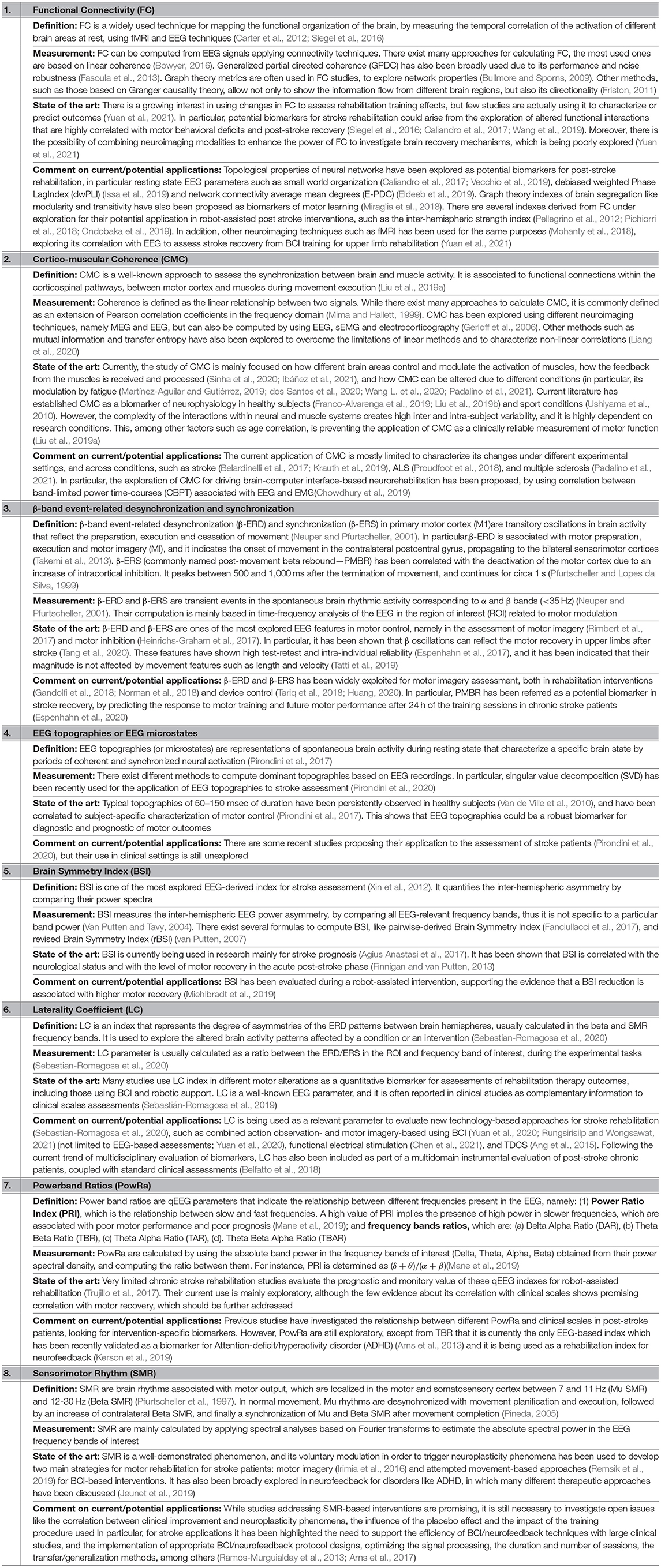
Table 2. List of the electroencephalography (EEG)-based biomarkers related to the motor rehabilitation focused on stroke.
While there exists a wide variety of the kinematic parameters used to describe the temporal and spatial features of the endpoint or joint movement (such as the position, velocity, movement time, or the execution of a task or action), systematic reviews on the kinematic assessments show that these parameters are poorly standardized and the unbiased clinimetrics is rarely addressed (Schwarz et al., 2019).
Due to the great number of biomarkers in this category and their large variability across the literature in terms of the nomenclature and level of evidence, examples in Table 3 are presented according to the guidelines introduced in Schwarz et al. (2019), in which the clinically relevant kinematic measurements for the upper limb after stroke were selected from a large database according to their available clinimetric evidence and clustered according to their presumed physiological interpretation for both the three-dimensional (3D) and two-dimensional (2D) tasks. With respect to the previous efforts in standardization and the expertise of the authors, this classification considers the following categories:
1. Efficacy: Indication if the task or the objective was successfully achieved or not.
2. Efficiency: Quantification of the performance of a task.
3. Precision: Description of the variability of performance of the goal-directed movements.
4. Accuracy: Quantification of error of the performed movements compared with an optimal movement.
5. Smoothness: Deviation of the velocity profile from an optimal profile.
6. Spatial posture: Position-related aspects of the joints.
7. Temporal posture: Time-related aspects of the joints.
8. Workspace: Description of the reachable area or volume with a specific joint.
9. Speed: Velocity of the performance of the movements.
Toward Personalized Neurorehabilitation: Adopting the Rehabilomics Approaches in the Robot-Assisted Rehabilitation
The idea of the state-of-the-art biomarker platforms and the technologies focused on rehabilitation have led to the concept of the “Rehabilomics” (Wagner, 2010), i.e., a transdisciplinary evaluation of the biomarkers to understand the rehabilitation-relevant phenotypes related to biology, function, prognosis, treatment, and recovery for the patients with disabilities (Wagner, 2010).
In this context, the development of the biomarkers based on the models of the motor control mechanisms needs to take into account how the real-world behavior emerges from the interaction between the neural, biomechanical, and environmental dynamics, in order to understand the healthy functions, disability, and rehabilitation progress. This perspective is the main purpose of the studies of the neuromechanics (Nishikawa et al., 2007; Valero-Cuevas, 2016), which aims at modeling the healthy movement and studying how these patterns change in the motor deficits, mainly for the robotic design and control (Pham et al., 2014; Szczecinski et al., 2017; Kühn et al., 2018). The research on the biomarker has been mainly focused in a physiological perspective and there is a need for the methodological approaches based on the neuromechanical assessments. In this scenario, the Rehabilomics can provide the new tools to better understand the motor rehabilitation from a multidisciplinary perspective (Figure 5).
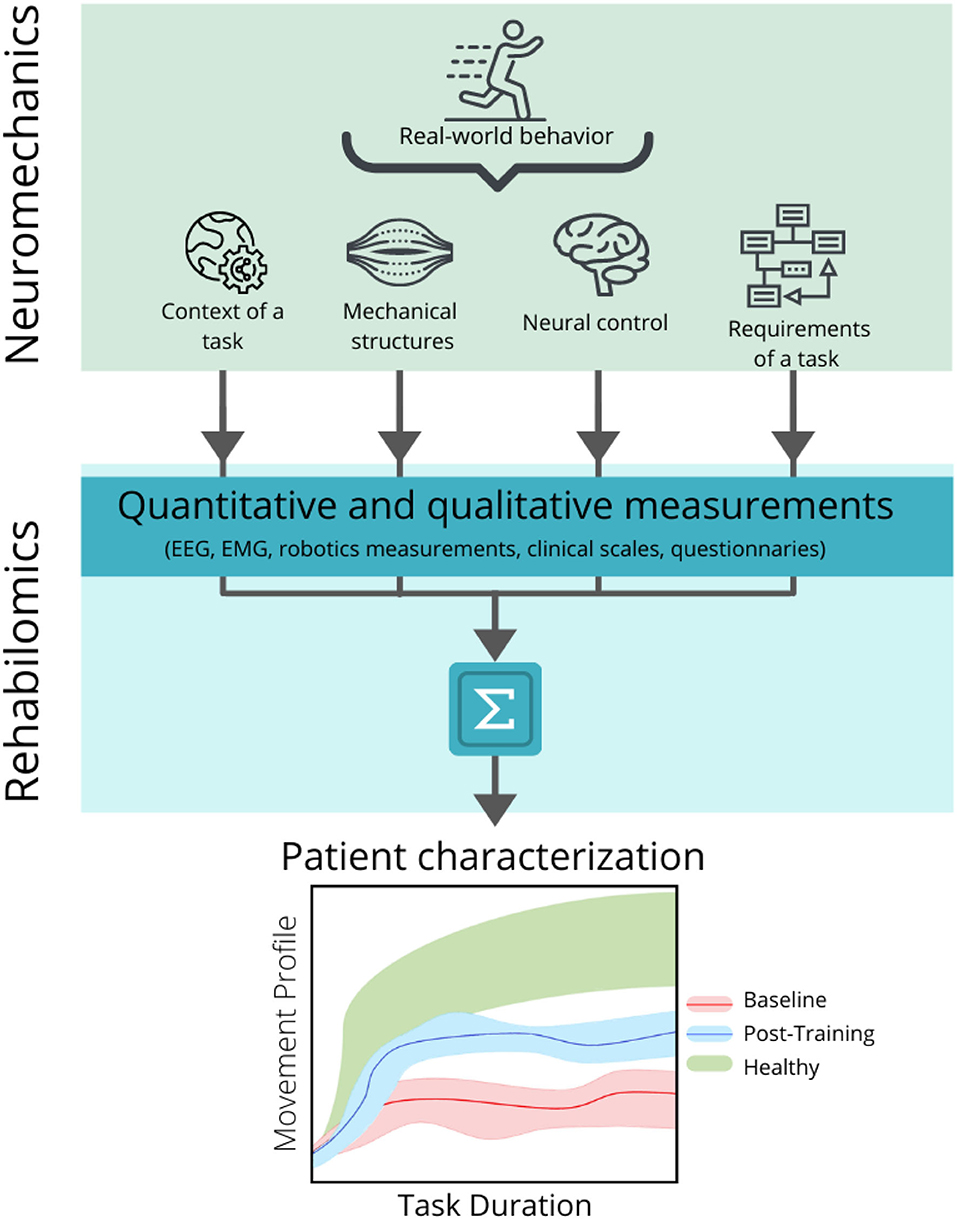
Figure 5. Relationship between the neuromechanical models and the Rehabilomics approach in the development of the motor-related biomarkers. Neuromechanics addresses the real-world behavior by considering the interaction between the context of the motor task, the mechanical structures of the body that are activated to produce the movement, the neural control necessary to produce and modulate the movement, and the specific requirements of the task (top panel). These parameters can be converted into quantitative and qualitative measurements by applying the recording techniques (such as electroencephalography, electromyography (EMG), kinematic measurements, validated clinical scales, and questionnaires) and can be combined to create a personalized profile of the patient (middle panel), in order to assess and predict the motor outcomes related to a specific intervention (bottom panel), before (bottom panel, baseline band in red) and after (bottom panel, post-training band in blue) the rehabilitation, and compare it with a normative band (bottom panel, healthy band in green).
Since the Rehabilomics has been primarily focused on the proteomics, genomics and metabolomics (Wagner and Zitelli, 2013; Skriver et al., 2014; Wagner, 2017; Wagner and Kumar, 2019), kinematics measures, and neuroimaging and electrophysiological recordings, they have also been widely explored as the potential biomarkers in the field of the robot-assisted neurorehabilitation (Philips et al., 2017; Belfatto et al., 2018; Pirondini et al., 2018; Krauth et al., 2019; Mane et al., 2019; Irastorza-Landa et al., 2021). In particular, the kinematics and electrophysiological indicators can be exploited as biomarkers, mainly because they are non-invasive and portable techniques, suitable for measuring the activity in both the acute and chronic phases.
In addition, the Rehabilomics approach has been directly related to the ICF framework (as shown in Stinear, 2017 and Section What Is a Biomarker and Its Relevance for Robot-Assisted Rehabilitation? Figure 4) by linking the profile of the patients (personal factors, their conditions and complications, and physiological environment) to the different dimensions of the ICF model (Figure 6). In this approach, the biomarkers could improve the stratification of the patients based on their individual biopsychosocial profiles, which could increase the statistical power of the trials to detect the intervention effects and enhance the outcomes assessment (Wagner, 2017). Thus, the consideration of such biomarkers into the ICF domains by using the Rehabilomics approach is most likely the next step in developing an integrated assessment of the robot-assisted rehabilitation treatments, optimizing clinical assessment procedures, and enhancing the effectiveness of such interventions (Do Tran et al., 2018).
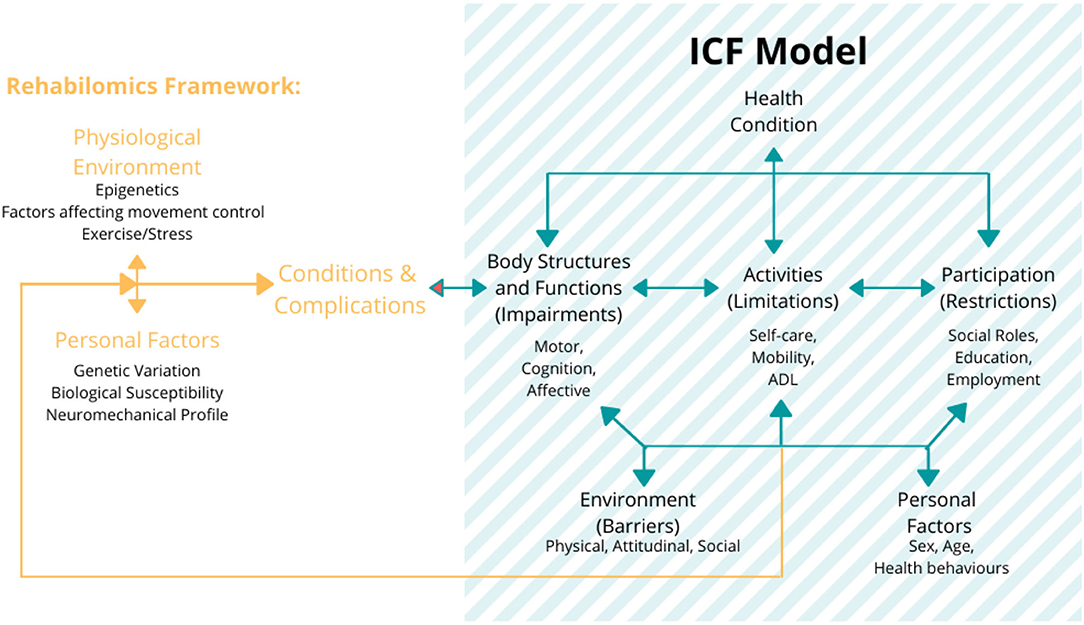
Figure 6. The Rehabilomics research framework uses the WHO ICF model as a foundational representation of function for the biomarker-based assessments of the brain injury response to demonstrate how these biological constructs inform the multidimensional aspects of the motor function. The figure also describes that these functional domains affect the life satisfaction and also have feedback effects on the biological impact on the health and function. Figure is open access courtesy of the National Academies of Sciences (2021) (Trang et al., 2020). Adapted from Wang et al. (2014) with permission.
Current Gaps in the Area
Currently, both the robotic-based interventions and the potential neurorehabilitation-based biomarkers are the presenting limitations, which are preventing their translation into the clinical practice. These can be clustered into knowledge, research, clinical, and translational gaps, which are summarized in Figure 7 and further described in Table 4.
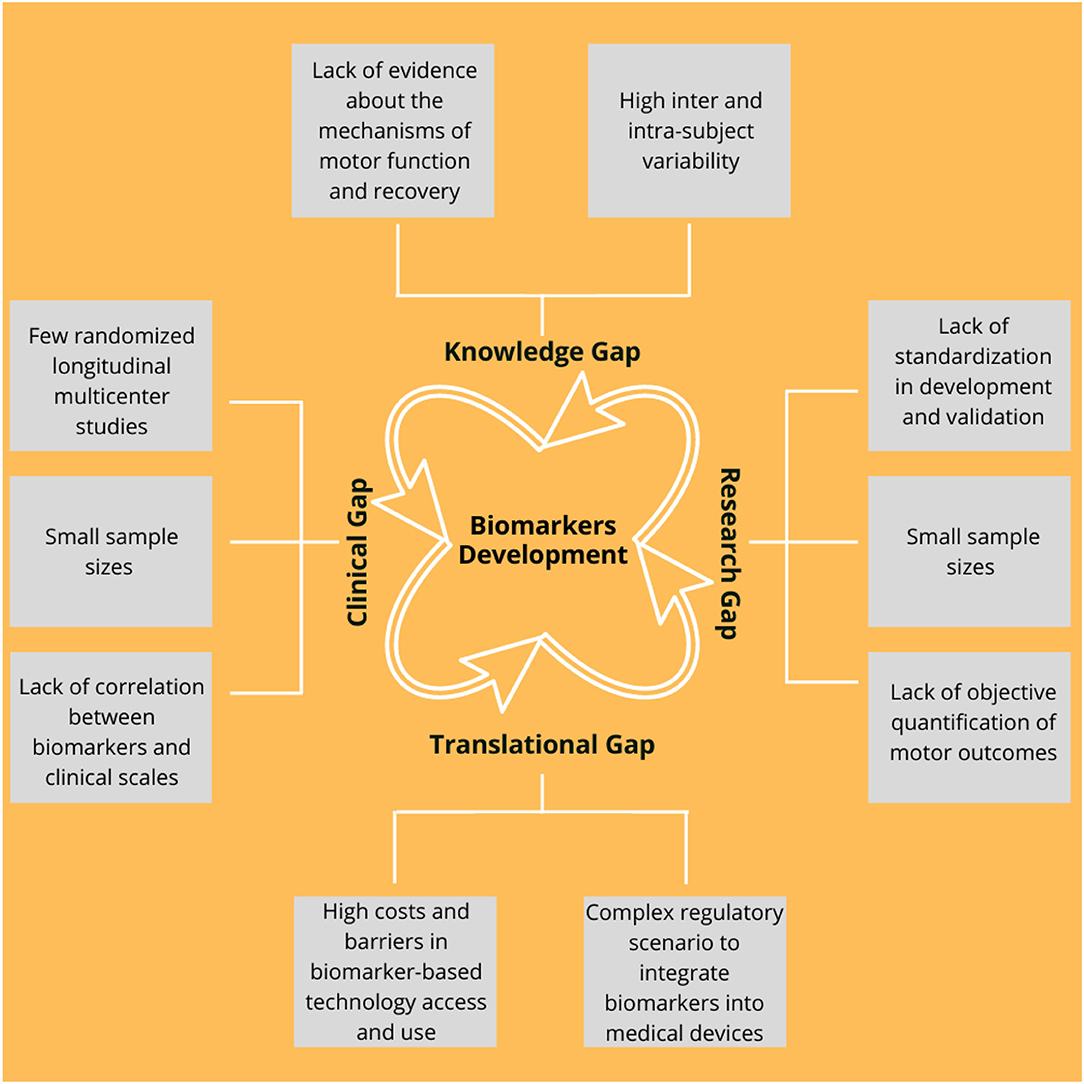
Figure 7. Main current gaps in the development of the biomarkers that can be grouped into the four main categories as follows: (1) Knowledge, (2) Research, (3) Translational, and (4) Clinical. A detailed description is illustrated in Table 4.
Latest Trends and Perspectives in the Field
In the previous section, some insights and future research directions have been identified. Highlights in these emerging topics are summarized in the following subsections.
Digital Biomarkers Based on At-Home Digital Surveillance
Growing efforts in the field of mobile health are being done for improving rehabilitation therapies. On one side, the possibility of self-assessment, large-scale population screening, and continuous monitoring through mobile applications are giving rise to the development of self-paced at-home therapies by using the commonly available devices and gadgets such as smartphones and smartwatches (Zhang et al., 2020). On the other hand, the current trends on telerehabilitation (providing the rehabilitation therapies through the information and communication technologies; Cramer, 2016) have opened the possibility of providing the rehabilitation training remotely in the home of the patient or the other environments outside of the typical rehabilitation setting. The development of such remote tools for the rehabilitation management is creating a new field in the digital biomarkers (which are defined as biomarkers collected and measured by means of the digital devices; Babrak et al., 2019) related to the motor rehabilitation.
In particular, in stroke rehabilitation, wearable motor sensors are being combined with digital biomarkers to monitor the longitudinal performance of the patients (Hou et al., 2018). The state-of-the-art biomarkers such as functional range of motion (fROM) for the quantification of upper limb reaching in the 3D visualizations, convergence points (CPs) for walking analysis based on the gait parameters, and physical activity (PA) for evaluation of the energy consumption (Derungs et al., 2020) are opening the door for the exploitation of the digital biomarkers in the rehabilitation.
Initiatives such as the Parkinson's Disease Digital Biomarker DREAM Challenge (Sieberts et al., 2021) are boosting the design of the digital biomarkers-based applications for the rehabilitation. For instance, recent algorithms for the self-reported symptoms of the Parkinson's disease (Ryu et al., 2019; Zhang et al., 2020) and the biomarker-based assessments of the tremor and bradykinesia through a wrist-worn wearable (Mahadevan et al., 2020) have been published. Additionally, the exploitation of the personal devices such as the smartphones and tablets has led to the birth of the novel methods to evaluate the performance of the users. For example, tappigraphy is a non-invasive and unobtrusive method based on the screen tapping actions, which contains the important indicators of homeostasis both in the healthy and pathological conditions: for some neurological diseases, it has been already shown the efficacy of the tapping activity for the prognostic and diagnostic functions (Gindrat et al., 2015; Balerna and Ghosh, 2018; Akeret et al., 2020; Duckrow et al., 2021; Ghosh, 2021). These new type of biomarkers need not only to be clinically relevant to correctly assess the status of the patient (Manta et al., 2020), but also have to be robust enough to be recorded and interpreted under the different conditions and by the different users. Another major challenge is the requirement of the high-quality engagement of the patient necessary to obtain and deploy these biomarkers (Goldsack et al., 2021).
Creating the Computational Neurorehabilitation Models for the Patient-Tailored Therapies
Computational models in neurorehabilitation (CMN) are encompassed by the personalized medicine and computational intelligence. CNM describes the complex human motor system in terms of the interactions between the sensorimotor activity and the behavioral outcomes of the patient by applying a computational model of the mechanisms of plasticity that are involved in recovery (Reinkensmeyer et al., 2016). It has set a framework to design the clinical experiments by simulating the rehabilitative parameters instead of using the current trial-and-error approach. This could not only allow to optimize the therapy design, but also personalize it in terms of content, timing, dosage, scheduling, etc., according to the profile of the individuals (Reinkensmeyer et al., 2016).
The concept of the patient-tailored therapies by using the computational neurorehabilitation is currently exploring the development of the new biomarkers from three main perspectives: (1) a neuroscience perspective (i.e., developing the mathematical models of the mechanisms of the activity-dependent plasticity; Reinkensmeyer et al., 2016), (2) a clinical perspective in which the clinically relevant biomarkers are being identified and used to create the algorithms for decision-making (i.e., prescribing the individualized intensities of the rehabilitation; Jeffers et al., 2018), and (3) a personalized biomechanical and sensor perspective in which the biomarkers are being used to complement the human movement analysis and wearable system design (Derungs and Amft, 2020). In particular, biomechanical simulations and motion data models are being used to create the personalized “digital twins.” This concept refers to the digital representation of the patient based on their profile health (Schwartz et al., 2020), which allows to simulate the different types of the biomarkers through this model, making the predictions and simulations of the evolution of the patient (Voigt et al., 2021) and testing and evaluating the wearable robotic systems before deploying the physical prototypes (Derungs and Amft, 2020).
Developing an Integrated Treatment of Stroke-Induced Motor, Cognitive, and Affect-Related Deficits
Following the notion that the robot-assisted neurorehabilitation demands a highly patient-tailored process, which entails the identification of the unique needs, priorities, and recovery profile of the patient, the integration of the biomarkers belonging to the different domains (sensorimotor, cognitive-behavioral, autonomic, psychological, and psychosocial) is being undertaken (Bui and Johnson, 2018; Zariffa, 2018; Picelli et al., 2020). The idea of developing the profile of the patient that combines the relevance of the multifactorial biomarkers is a new approach that is starting to being explored, with the design of the dedicated study protocols for defining a related profile of the biomarkers of long-term recovery after stroke (Picelli et al., 2020) and the exploration of the novel biomarkers related to the other aspects of the motor function rather than sensorimotor such as alterations in the body representations (Maggio et al., 2021), eye–hand coupling assessment (Rizzo et al., 2017), quantification of visuospatial neglect (VSN) (Svaerke et al., 2019), and somatic (or cognitive-related) biomarkers (Martinez-Pernia, 2020). Additionally, the combination of the neuroimaging technologies is supporting this multifactorial exploration by combining EMG, EEG, and inertial data to obtain the rehabilitation-relevant biomarkers (Gao et al., 2018; Zhang et al., 2019; Picelli et al., 2020).
This approach could lead to the potential development of reliable one-off measures to evaluate the functionality of a single patient by developing a biomarker profile in which a reference value is present. The reference value could be a curve adjusted to the stratification of the patient with respect to the healthy population and, therefore, the value obtained from the patient could be compared against this reference, allowing to quantify the motor function in a single shot. It would be necessary to obtain and validate these reference values (or profiles) by collecting the standardized information from a large number of the patients and healthy subjects.
These multidisciplinary assessments must take into account the feasibility of their implementation in the clinical practice in which the time spent for the assessment and the level of the invasiveness and comfort for the patient are major constraints. Hence, the optimization of the calculation of biomarkers, by means of the dimensionality reduction and standardization, along with the inclusion of user-centered design principles to the process of developing new interventions and biomarkers (Markopoulos et al., 2011; Almenara et al., 2017; Wentink et al., 2019), will lead not only to the creation of the truly personalized and integrated rehabilitation technologies, but also to a significant reduction in the time spent in assessing the status of the patient.
Conclusion
In this study, the most current relevant biomarker candidates for the rehabilitation were shortlisted and for many of them promising correlations with clinical outcomes have been found. Their use in the robot-assisted rehabilitation is at a point of the fast advancement due to the diffusion of the robotic technologies and new frameworks for multidisciplinary work such as the concept of the Rehabilomics. In particular, the development of the biomarkers based on EEG, EMG, and kinematics is a promising area in which exploratory work reported in the literature has been increasing in the recent years. Nevertheless, there are still important gaps in the area to overcome and the future studies should take into consideration more robust cross-validation protocols, addressing issues such as standardized procedures, proper sample sizes, and stratification of the patient. Further research is needed in order to identify the most informative biomarker (or set of biomarkers) to design the more optimized and patient-tailored rehabilitation therapies. This will also provide the better understanding of the prognosis and recovery and help to developing the more quantitative grounded treatment strategies to improve the recovery. This approach potentially allows a deeper understanding of the robot-assisted rehabilitation process and its interaction with the human motor control and behavioral mechanisms, boosting the development of the better human-inspired assistive technologies.
Author Contributions
FG, MC, and MS conceived the study and reviewed the figures. FG and MS designed the figures. FG wrote the first draft of the manuscript and prepared the figures. All authors contributed to the writing of the manuscript and approved its final version.
Funding
This study was supported by the Istituto Nazionale Assicurazione Infortuni sul Lavoro (INAIL) (Project grant number PR19-RR-P2).
Conflict of Interest
The authors declare that the research was conducted in the absence of any commercial or financial relationships that could be construed as a potential conflict of interest.
Publisher's Note
All claims expressed in this article are solely those of the authors and do not necessarily represent those of their affiliated organizations, or those of the publisher, the editors and the reviewers. Any product that may be evaluated in this article, or claim that may be made by its manufacturer, is not guaranteed or endorsed by the publisher.
Acknowledgments
The authors gracefully acknowledge Silvia Chiappalone for providing the graphics of Figure 2.
References
Abdallah, I. B., Bouteraa, Y., and Rekik, C. (2017). Design and development of 3D printed myoelectric robotic exoskeleton for hand rehabilitation. Int. J. Smart Sens. Intelligent Syst. 10, 341–366. doi: 10.21307/ijssis-2017-215
Agius Anastasi, A., Falzon, O., Camilleri, K., Vella, M., and Muscat, R. (2017). Brain symmetry index in healthy and stroke patients for assessment and prognosis. Stroke Res. Treat. 2017:8276136. doi: 10.1155/2017/8276136
Agrafiotis, D. K., Yang, E., Littman, G. S., Byttebier, G., Dipietro, L., DiBernardo, A., et al. (2021). Accurate prediction of clinical stroke scales and improved biomarkers of motor impairment from robotic measurements. PLoS ONE 16:e0245874. doi: 10.1371/journal.pone.0245874
Akeret, K., Vasella, F., Zindel-Geisseler, O., Dannecker, N., Brugger, P., Regli, L., et al. (2020). Passive smartphone-based assessment of cognitive changes in neurosurgery. MedRxiv [Preprint]. doi: 10.1101/2020.11.10.20228734
Almenara, M., Cempini, M., Gómez, C., Cortese, M., Martín, C., Medina, J., et al. (2017). Usability test of a hand exoskeleton for activities of daily living: an example of user-centered design. Disabil. Rehabil. Assist. Technol. 12, 84–96. doi: 10.3109/17483107.2015.1079653
Amano, S., Umeji, A., Uchita, A., Hashimoto, Y., Takebayashi, T., Takahashi, K., et al. (2018). Clinimetric properties of the Fugl-Meyer assessment with adapted guidelines for the assessment of arm function in hemiparetic patients after stroke. Top. Stroke Rehabil. 25, 500–508. doi: 10.1080/10749357.2018.1484987
Ang, K. K., Guan, C., Phua, K. S., Wang, C., Zhao, L., Teo, W. P., et al. (2015). Facilitating effects of transcranial direct current stimulation on motor imagery brain-computer interface with robotic feedback for stroke rehabilitation. Arch. Phys. Med. Rehabil. 96 S79–S87. doi: 10.1016/j.apmr.2014.08.008
Arns, M., Batail, J. M., Bioulac, S., Congedo, M., Daudet, C., Drapier, D., et al. (2017). Neurofeedback: one of today's techniques in psychiatry? L'Encéphale 43, 135–145. doi: 10.1016/j.encep.2016.11.003
Arns, M., Conners, C. K., and Kraemer, H. C. (2013). A decade of EEG theta/beta ratio research in ADHD: a meta-analysis. J. Atten. Disord. 17, 374–383. doi: 10.1177/1087054712460087
Arteaga, M. V., Castiblanco, J. C., Mondragon, I. F., Colorado, J. D., and Alvarado-Rojas, C. (2020). EMG-driven hand model based on the classification of individual finger movements. Biomed. Signal Process. Control 58:101834. doi: 10.1016/j.bspc.2019.101834
Babiloni, C., Barry, R. J., Başar, E., Blinowska, K. J., Cichocki, A. W., et al. (2020). International Federation of Clinical Neurophysiology (IFCN) – EEG research workgroup: recommendations on frequency and topographic analysis of resting state EEG rhythms. Part 1: applications in clinical research studies. Clin. Neurophysiol. 131, 285–307. doi: 10.1016/j.clinph.2019.06.234
Babrak, L. M., Menetski, J., Rebhan, M., Nisato, G., Zinggeler, M., Brasier, N., et al. (2019). Traditional and digital biomarkers: two worlds apart? Digital Biomark. 3, 92–102. doi: 10.1159/000502000
Baldan, F., Turolla, A., Rimini, D., Pregnolato, G., Maistrello, L., Agostini, M., et al. (2021). Robot-assisted rehabilitation of hand function after stroke: development of prediction models for reference to therapy. J. Electromyogr. Kinesiol. 57:102534. doi: 10.1016/j.jelekin.2021.102534
Balerna, M., and Ghosh, A. (2018). The details of past actions on a smartphone touchscreen are reflected by intrinsic sensorimotor dynamics. NPJ Dig. Med. 1, 1–5. doi: 10.1038/s41746-017-0011-3
Beaton, D. E., Boers, M., and Wells, G. A. (2002). Many faces of the minimal clinically important difference (MCID): a literature review and directions for future research. Curr. Opin. Rheumatol. 14, 109–114. doi: 10.1097/00002281-200203000-00006
Belardinelli, P., Laer, L., Ortiz, E., Braun, C., and Gharabaghi, A. (2017). Plasticity of premotor cortico-muscular coherence in severely impaired stroke patients with hand paralysis. Neuroimage Clin. 14, 726–733. doi: 10.1016/j.nicl.2017.03.005
Belfatto, A., Scano, A., Chiavenna, A., Mastropietro, A., Mrakic-Sposta, S., Pittaccio, S., et al. (2018). A multiparameter approach to evaluate post-stroke patients: an application on robotic rehabilitation. Appl. Sci. 8:2248. doi: 10.3390/app8112248
Bernhardt, J., Hayward, K. S., Kwakkel, G., Ward, N. S., Wolf, S. L., Borschmann, K., et al. (2017). Agreed definitions and a shared vision for new standards in stroke recovery research: the Stroke Recovery and Rehabilitation Roundtable taskforce. Int. J. Stroke 12, 444–450. doi: 10.1177/1747493017711816
Biomarkers Definitions Working Group (2001). Biomarkers and surrogate endpoints: preferred definitions and conceptual framework. Clin. Pharmacol. Ther. 69, 89–95. doi: 10.1067/mcp.2001.113989
Birkeland, M. L., and McClure, J. S. (2015). Optimizing the clinical utility of biomarkers in oncology: the NCCN Biomarkers Compendium. Arch. Pathol. Lab. Med. 139, 608–611. doi: 10.5858/arpa.2014-0146-RA
Bizzi, E., and Cheung, V. C. (2013). The neural origin of muscle synergies. Front. Comput. Neurosci. 7:51. doi: 10.3389/fncom.2013.00051
Bouteraa, Y., Abdallah, I. B., and Elmogy, A. (2020). Design and control of an exoskeleton robot with EMG-driven electrical stimulation for upper limb rehabilitation. Industrial Robot Int. J. Robot. Res. Appl. 47, 489–501. doi: 10.1108/IR-02-2020-0041
Bowyer, S. M. (2016). Coherence a measure of the brain networks: past and present. Neuropsychiatr. Electrophysiol. 2:1. doi: 10.1186/s40810-015-0015-7
Bui, K. D., and Johnson, M. J. (2018). Designing robot-assisted neurorehabilitation strategies for people with both HIV and stroke. J. Neuroeng. Rehabil. 15:75. doi: 10.1186/s12984-018-0418-3
Bullmore, E., and Sporns, O. (2009). Complex brain networks: graph theoretical analysis of structural and functional systems. Nat. Rev. Neurosci. 10, 186–198. doi: 10.1038/nrn2575
Buma, F. E., Lindeman, E., Ramsey, N. F., and Kwakkel, G. (2010). Functional neuroimaging studies of early upper limb recovery after stroke: a systematic review of the literature. Neurorehabil. Neural Repair 24, 589–608. doi: 10.1177/1545968310364058
Cahyadi, B. N., Khairunizam, W., Muhammad, M. N., Zunaidi, I., Majid, S. H., Rudzuan, M. N., et al. (2018a). “Analysis of EMG based arm movement sequence using mean and median frequency, in 2018 5th International Conference on Electrical Engineering, Computer Science and Informatics (EECSI) (IEEE), 440–444.
Cahyadi, B. N., Zunaidi, I., Bakar, S. A., Khairunizam, W., Majid, S. H., Razlan, Z. M., et al. (2018b). “Upper limb muscle strength analysis for movement sequence based on maximum voluntary contraction using EMG Signal,” in 2018 International Conference on Computational Approach in Smart Systems Design and Applications (ICASSDA) (IEEE), pp. 1–5.
Caliandro, P., Vecchio, F., Miraglia, F., Reale, G., Della Marca, G., La Torre, G., et al. (2017). Small-world characteristics of cortical connectivity changes in acute stroke. Neurorehabil. Neural Repair 31, 81–94. doi: 10.1177/1545968316662525
Campanini, I., Disselhorst-Klug, C., Rymer, W. Z., and Merletti, R. (2020). Surface EMG in clinical assessment and neurorehabilitation: barriers limiting its use. Front. Neurol. 11:934. doi: 10.3389/fneur.2020.00934
Carter, A. R., Shulman, G. L., and Corbetta, M. (2012). Why use a connectivity-based approach to study stroke and recovery of function? Neuroimage 62, 2271–2280. doi: 10.1016/j.neuroimage.2012.02.070
Chen, L., Gu, B., Wang, Z., Zhang, L., Xu, M., Liu, S., et al. (2021). EEG-controlled functional electrical stimulation rehabilitation for chronic stroke: system design and clinical application. Front. Med. doi: 10.1007/s11684-020-0794-5. [Epub ahead of print].
Chowdhury, A., Raza, H., Meena, Y. K., Dutta, A., and Prasad, G. (2019). An EEG-EMG correlation-based brain-computer interface for hand orthosis supported neuro-rehabilitation. J. Neurosci. Methods 312, 1–11. doi: 10.1016/j.jneumeth.2018.11.010
Connell, L. A., Smith, M. C., Byblow, W. D., and Stinear, C. M. (2018). Implementing biomarkers to predict motor recovery after stroke. NeuroRehabilitation 43, 41–50. doi: 10.3233/NRE-172395
Cramer, S. C. (2016). “59 - Interventions to improve recovery after stroke,” in Stroke, 6th ed, eds J. C. Grotta, G. W. Albers, J. P. Broderick, S. E. Kasner, E. H. Lo, A. D. Mendelow (London: Elsevier), 972–980.e5.
Cramer, S. C., Parrish, T. B., Levy, R. M., Stebbins, G. T., Ruland, S. D., Lowry, D. W., et al. (2007). Predicting functional gains in a stroke trial. Stroke 38, 2108–2114. doi: 10.1161/STROKEAHA.107.485631
Derungs, A., and Amft, O. (2020). Estimating wearable motion sensor performance from personal biomechanical models and sensor data synthesis. Sci. Rep. 10:11450. doi: 10.1038/s41598-020-68225-6
Derungs, A., Schuster-Amft, C., and Amft, O. (2020). Wearable motion sensors and digital biomarkers in stroke rehabilitation. Curr. Direct. Biomed. Eng. 6, 229–232. doi: 10.1515/cdbme-2020-3058
Do Tran, V., Dario, P., and Mazzoleni, S. (2018). Kinematic measures for upper limb robot-assisted therapy following stroke and correlations with clinical outcome measures: a review. Med. Eng. Phys. 53, 13–31. doi: 10.1016/j.medengphy.2017.12.005
dos Santos, P. C. R., Lamoth, C. J. C., Barbieri, F. A., Zijdewind, I., Gobbi, L. T. B., and Hortobágyi, T. (2020). Age-specific modulation of intermuscular beta coherence during gait before and after experimentally induced fatigue. Sci. Rep. 10:15854. doi: 10.1038/s41598-020-72839-1
Duan, W., Chen, X., Wang, Y. J., Zhao, W., Yuan, H., and Lei, X. (2021). Reproducibility of power spectrum, functional connectivity and network construction in resting-state EEG. J. Neurosci. Methods 348:108985. doi: 10.1016/j.jneumeth.2020.108985
Duckrow, R. B., Ceolini, E., Zaveri, H. P., Brooks, C., and Ghosh, A. (2021). Artificial neural network trained on smartphone behavior can trace epileptiform activity in epilepsy. iScience 24:102538. doi: 10.1016/j.isci.2021.102538
Eldeeb, S., Akcakaya, M., Sybeldon, M., Foldes, S., Santarnecchi, E., Pascual-Leone, A., et al. (2019). EEG-based functional connectivity to analyze motor recovery after stroke: a pilot study. Biomed. Signal Process. Control 49, 419–426. doi: 10.1016/j.bspc.2018.12.022
Espenhahn, S., de Berker, A. O., van Wijk, B. C. M., Rossiter, H. E., and Ward, N. S. (2017). Movement-related beta oscillations show high intra-individual reliability. Neuroimage 147, 175–185. doi: 10.1016/j.neuroimage.2016.12.025
Espenhahn, S., Rossiter, H. E., van Wijk, B. C. M., Redman, N., Rondina, J. M., Diedrichsen, J., et al. (2020). Sensorimotor cortex beta oscillations reflect motor skill learning ability after stroke. Brain Commun. 2:fcaa161. doi: 10.1093/braincomms/fcaa161
Esteve-Pastor, M. A., Roldan, V., Rivera-Caravaca, J. M., Ramirez-Macias, I., Lip, G. Y., and Marin, F. (2019). The use of biomarkers in clinical management guidelines: a critical appraisal. Thromb. Haemost. 119, 1901–1919. doi: 10.1055/s-0039-1696955
Fanciullacci, C., Bertolucci, F., Lamola, G., Panarese, A., Artoni, F., Micera, S., et al. (2017). Delta power is higher and more symmetrical in ischemic stroke patients with cortical involvement. Front. Hum. Neurosci. 11:385. doi: 10.3389/fnhum.2017.00385
Farina, D., and Holobar, A. (2014). Human/Machine interfacing by decoding the surface electromyogram. IEEE Signal Proc. Mag. 32, 115–120. doi: 10.1109/MSP.2014.2359242
Farina, D., Negro, F., Muceli, S., and Enoka, R. M. (2016). Principles of motor unit physiology evolve with advances in technology. Physiology 31, 83–94. doi: 10.1152/physiol.00040.2015
Farina, D., Vujaklija, I., Sartori, M., Kapelner, T., Negro, F., Jiang, N., et al. (2017). Man/machine interface based on the discharge timings of spinal motor neurons after targeted muscle reinnervation. Nat. Biomed. Eng. 1, 1–12. doi: 10.1038/s41551-016-0025
Fasoula, A., Attal, Y., and Schwartz, D. (2013). Comparative performance evaluation of data-driven causality measures applied to brain networks. J. Neurosci. Methods 215, 170–189. doi: 10.1016/j.jneumeth.2013.02.021
Fatehi, F., Grapperon, A. M., Fathi, D., Delmont, E., and Attarian, S. (2018). The utility of motor unit number index: a systematic review. Neurophysiol. Clin. 48, 251–259. doi: 10.1016/j.neucli.2018.09.001
Finnigan, S., and van Putten, M. J. (2013). EEG in ischaemic stroke: quantitative EEG can uniquely inform (sub-) acute prognoses and clinical management. Clin. Neurophysiol. 124, 10–19. doi: 10.1016/j.clinph.2012.07.003
Franceschini, M., Mazzoleni, S., Goffredo, M., Pournajaf, S., Galafate, D., Criscuolo, S., et al. (2020). Upper limb robot-assisted rehabilitation versus physical therapy on subacute stroke patients: a follow-up study. J. Bodyw. Mov. Ther. 24, 194–198. doi: 10.1016/j.jbmt.2019.03.016
Franco-Alvarenga, P. E., Brietzke, C., Canestri, R., Goethel, M. F., Viana, B. F., and Pires, F. O. (2019). Caffeine increased muscle endurance performance despite reduced cortical activation and unchanged neuromuscular efficiency and corticomuscular coherence. Nutrients 11:2471. doi: 10.3390/nu11102471
Friston, K. J. (2011). Functional and effective connectivity: a review. Brain Connect. 1, 13–36. doi: 10.1089/brain.2011.0008
Frontera, W. R., Bean, J. F., Damiano, D., Ehrlich-Jones, L., Fried-Oken, M., Jette, A., et al. (2017). Rehabilitation research at the National Institutes of Health. Neurorehabil. Neural Repair 31, 304–314. doi: 10.1177/1545968317698875
Gandolfi, M., Formaggio, E., Geroin, C., Storti, S. F., Boscolo Galazzo, I., Bortolami, M., et al. (2018). Quantification of upper limb motor recovery and EEG power changes after robot-assisted bilateral arm training in chronic stroke patients: a prospective pilot study. Neural Plast. 2018:8105480. doi: 10.1155/2018/8105480
Gao, Y., Ren, L., Li, R., and Zhang, Y. (2018). Electroencephalogram–electromyography coupling analysis in stroke based on symbolic transfer entropy. Front. Neurol. 8:716. doi: 10.3389/fneur.2017.00716
Gerloff, C., Braun, C., Staudt, M., Hegner, Y. L., Dichgans, J., and Krägeloh-Mann, I. (2006). Coherent corticomuscular oscillations originate from primary motor cortex: evidence from patients with early brain lesions. Hum. Brain Mapp. 27, 789–798. doi: 10.1002/hbm.20220
Ghosh, A. (2021). Smartphone deprivation alters cortical sensorimotor processing of the hand. bioRxiv [Preprint]. doi: 10.1101/2021.03.04.433898
Gindrat, A.-D., Chytiris, M., Balerna, M., Rouiller, E. M., and Ghosh, A. (2015). Use-dependent cortical processing from fingertips in touchscreen phone users. Curr. Biol. 25, 109–116. doi: 10.1016/j.cub.2014.11.026
Giszter, S. F. (2015). Motor primitives–new data and future questions. Curr. Opin. Neurobiol. 33, 156–165. doi: 10.1016/j.conb.2015.04.004
Gladstone, D. J., Danells, C. J., and Black, S. E. (2002). The Fugl-Meyer assessment of motor recovery after stroke: a critical review of its measurement properties. Neurorehabil. Neural Repair 16, 232–240. doi: 10.1177/154596802401105171
Goldsack, J. C., Dowling, A. V., Samuelson, D., Patrick-Lake, B., and Clay Evaluation, I. (2021). Acceptance, and qualification of digital measures: from proof of concept to endpoint. Dig. Biomark. 5, 53–64. doi: 10.1159/000514730
Grinyagin, I. V., Biryukova, E. V., and Maier, M. A. (2005). Kinematic and dynamic synergies of human precision-grip movements. J. Neurophysiol. 94, 2284–2294. doi: 10.1152/jn.01310.2004
Habermehl, C., Benner, A., and Kopp-Schneider, A. (2018). Addressing small sample size bias in multiple-biomarker trials: inclusion of biomarker-negative patients and Firth correction. Biom. J. 60, 275–287. doi: 10.1002/bimj.201600226
Hayward, K. S., Kramer, S. F., Thijs, V., Ratcliffe, J., Ward, N. S., Churilov, L., et al. (2019). A systematic review protocol of timing, efficacy and cost effectiveness of upper limb therapy for motor recovery post-stroke. Syst. Rev. 8:187. doi: 10.1186/s13643-019-1093-6
Heinrichs-Graham, E., Kurz, M. J., Gehringer, J. E., and Wilson, T. W. (2017). The functional role of post-movement beta oscillations in motor termination. Brain Struct. Funct. 222, 3075–3086. doi: 10.1007/s00429-017-1387-1
Holobar, A., and Farina, D. (2021). Noninvasive neural interfacing with wearable muscle sensors: combining convolutive blind source separation methods and deep learning techniques for neural decoding. IEEE Signal Process. Mag. 38, 103–118. doi: 10.1109/MSP.2021.3057051
Horvath, A. R., Kis, E., and Dobos, E. (2010). Guidelines for the use of biomarkers: principles, processes and practical considerations. Scand. J. Clin. Lab. Invest. 70, 109–116. doi: 10.3109/00365513.2010.493424
Hou, Y.-R., Chiu, Y.-L., Chiang, S.-L., Chen, H.-Y., and Sung, W.-H. (2018). Feasibility of a smartphone-based balance assessment system for subjects with chronic stroke. Comput. Methods Programs Biomed. 161, 191–195. doi: 10.1016/j.cmpb.2018.04.027
Houston, M., Li, R., Roh, J., and Zhang, Y. (2020). “Altered muscle networks in post-stroke survivors,” in 2020 42nd Annual International Conference of the IEEE Engineering in Medicine and Biology Society (EMBC) (IEEE), 3771–3774.
Hu, M., Schindler, M. K., Dewey, B. E., Reich, D. S., Shinohara, R. T., and Eloyan, A. (2020). Experimental design and sample size considerations in longitudinal magnetic resonance imaging-based biomarker detection for multiple sclerosis. Stat. Methods Med. Res. 29, 2617–2628. doi: 10.1177/0962280220904392
Huang, S., Cai, S., Li, G., Chen, Y., Ma, K., and Xie, L. (2019). sEMG-based detection of compensation caused by fatigue during rehabilitation therapy: a pilot study. IEEE Access 7, 127055–127065. doi: 10.1109/ACCESS.2019.2933287
Huang, Y. (2020). Investigation of Robot Assisted Sensorimotor Upper Limb Rehabilitation After Stroke. Hong Kong Polytechnic University. Available online at: https://theses.lib.polyu.edu.hk/handle/200/10417
Hug, F., Avrillon, S., Del Vecchio, A., Casolo, A., Ibanez, J., Nuccio, S., et al. (2021). Analysis of motor unit spike trains estimated from high-density surface electromyography is highly reliable across operators. J. Electromyogr. Kinesiol. 58:102548. doi: 10.1016/j.jelekin.2021.102548
Iandolo, R., Marini, F., Semprini, M., Laffranchi, M., Mugnosso, M., Cherif, A., et al. (2019). Perspectives and challenges in robotic neurorehabilitation. Appl. Sci. 9:3183. doi: 10.3390/app9153183
Ibáñez, J., Del Vecchio, A., Rothwell, J. C., Baker, S. N., and Farina, D. (2021). Only the fastest corticospinal fibers contribute to β corticomuscular coherence. J. Neurosci. 41, 4867–4879. doi: 10.1523/JNEUROSCI.2908-20.2021
Irastorza-Landa, N., Garcia-Cossio, E., Sarasola-Sanz, A., Broetz, D., and Ramos-Murguialday, A. (2021). Functional synergy recruitment index as a reliable biomarker of motor function and recovery in chronic stroke patients. J. Neural Eng. 18:046061. doi: 10.1088/1741-2552/abe244
Irimia, D., Sabathiel, N., Ortner, R., Poboroniuc, M., Coon, W., Allison, B. Z., et al. (2016). “recoveriX: a new BCI-based technology for persons with stroke,” in 2016 38th Annual International Conference of the IEEE Engineering in Medicine and Biology Society (EMBC) (IEEE), 1504–1507.
Irimia, D. C., Ortner, R., Poboroniuc, M. S., Ignat, B. E., and Guger, C. (2018). High classification accuracy of a motor imagery based brain-computer interface for stroke rehabilitation training. Front. Robot AI 5:130. doi: 10.3389/frobt.2018.00130
Issa, M. F., Gyulai, A., Kozmann, G., Nagy, Z., and Juhasz, Z. (2019). “Functional connectivity biomarkers based on resting-state EEG for stroke recovery,” in 2019 12th International Conference on Measurement (IEEE), 133–136.
Jaeschke, R., Singer, J., and Guyatt, G. H. (1989). Measurement of health status: ascertaining the minimal clinically important difference. Control. Clin. Trials 10, 407–415. doi: 10.1016/0197-2456(89)90005-6
Jeffers, M. S., Karthikeyan, S., Gomez-Smith, M., Gasinzigwa, S., Achenbach, J., Feiten, A., et al. (2018). Does stroke rehabilitation really matter? Part B: an algorithm for prescribing an effective intensity of rehabilitation. Neurorehabil. Neural Repair 32, 73–83. doi: 10.1177/1545968317753074
Jeunet, C., Glize, B., McGonigal, A., Batail, J.-M., and Micoulaud-Franchi, J.-A. (2019). Using EEG-based brain computer interface and neurofeedback targeting sensorimotor rhythms to improve motor skills: theoretical background, applications and prospects. Neurophysiol. Clin. 49, 125–136. doi: 10.1016/j.neucli.2018.10.068
Johnson, C. O., Nguyen, M., Roth, G. A., Nichols, E., Alam, T., Abate, D., et al. (2019). Global, regional, and national burden of stroke, 1990–2016: a systematic analysis for the Global Burden of Disease Study 2016. Lancet Neurol. 18, 439–458. doi: 10.1016/S1474-4422(19)30034-1
Julianjatsono, R., Ferdiana, R., and Hartanto, R. (2017). “High-resolution automated Fugl-Meyer Assessment using sensor data and regression model,” in 2017 3rd International Conference on Science and Technology - Computer (ICST) (IEEE), 28–32.
Kanal, V., Abujelala, M., Brady, J., Wylie, G., and Makedon, F. (2019). “Adaptive robotic rehabilitation using muscle fatigue as a trigger,” in Proceedings of the 16th EAI International Conference on Mobile and Ubiquitous Systems: Computing, Networking and Services, Association for Computing Machinery (Houston, TX), 135–142.
Kanzler, C. M., Rinderknecht, M. D., Schwarz, A., Lamers, I., Gagnon, C., Held, J. P. O., et al. (2020). A data-driven framework for selecting and validating digital health metrics: use-case in neurological sensorimotor impairments. NPJ Dig. Med. 3:80. doi: 10.1038/s41746-020-0286-7
Karthick, P., Ghosh, D. M., and Ramakrishnan, S. (2018). Surface electromyography based muscle fatigue detection using high-resolution time-frequency methods and machine learning algorithms. Comput. Methods Programs Biomed. 154, 45–56. doi: 10.1016/j.cmpb.2017.10.024
Kelly, C. J., Karthikesalingam, A., Suleyman, M., Corrado, G., and King, D. (2019). Key challenges for delivering clinical impact with artificial intelligence. BMC Med. 17, 1–9. doi: 10.1186/s12916-019-1426-2
Kerson, C., deBeus, R., Lightstone, H., Arnold, L. E., Barterian, J., Pan, X., et al. (2019). EEG theta/beta ratio calculations differ between various eeg neurofeedback and assessment software packages: clinical interpretation. Clin. EEG Neurosci. 51, 114–120. doi: 10.1177/1550059419888320
Khairuddin, I. M., Sidek, S. N., Majeed, A. P. A., Razman, M. A. M., Puzi, A. A., and Yusof, H. M. (2021). The classification of movement intention through machine learning models: the identification of significant time-domain EMG features. PeerJ Comput. Sci. 7:e379. doi: 10.7717/peerj-cs.379
Khalid, S., Alnajjar, F., Gochoo, M., Renawi, A., and Shimoda, S. (2021). “Robotic assistive and rehabilitation devices leading to motor recovery in upper limb: a systematic review,” in Disability and Rehabilitation: Assistive Technology (Taylor & Francis), 1–15.
Kidd, D., Stewart, G., Baldry, J., Johnson, J., Rossiter, D., Petruckevitch, A., et al. (1995). The Functional Independence Measure: a comparative validity and reliability study. Disabil. Rehabil. 17, 10–14. doi: 10.3109/09638289509166622
Kim, B., and Winstein, C. (2017). Can neurological biomarkers of brain impairment be used to predict poststroke motor recovery? A systematic review. Neurorehabil. Neural Repair 31, 3–24. doi: 10.1177/1545968316662708
Kim, W. S., Cho, S., Baek, D., Bang, H., and Paik, N. J. (2016). Upper extremity functional evaluation by Fugl-Meyer assessment scoring using depth-sensing camera in hemiplegic stroke patients. PLoS ONE 11:e0158640. doi: 10.1371/journal.pone.0158640
Krauth, R., Schwertner, J., Vogt, S., Lindquist, S., Sailer, M., Sickert, A., et al. (2019). Cortico-muscular coherence is reduced acutely post-stroke and increases bilaterally during motor recovery: a pilot study. Front. Neurol. 10:126. doi: 10.3389/fneur.2019.00126
Krebs, H. I., Krams, M., Agrafiotis, D. K., DiBernardo, A., Chavez, J. C., Littman, G. S., et al. (2014). Robotic measurement of arm movements after stroke establishes biomarkers of motor recovery. Stroke 45, 200–204. doi: 10.1161/STROKEAHA.113.002296
Kühn, J., Hu, T., Schappler, M., and Haddadin, S. (2018). “Dynamics simulation for an upper-limb human-exoskeleton assistance system in a latent-space controlled tool manipulation task,” in 2018 IEEE International Conference on Simulation, Modeling, and Programming for Autonomous Robots (SIMPAR) (IEEE), 158–165.
Laine, C. M., and Valero-Cuevas, F. J. (2017). Intermuscular coherence reflects functional coordination. J. Neurophysiol. 118, 1775–1783. doi: 10.1152/jn.00204.2017
Lang, C. E., Edwards, D. F., Birkenmeier, R. L., and Dromerick, A. W. (2008). Estimating minimal clinically important differences of upper-extremity measures early after stroke. Arch. Phys. Med. Rehabil. 89, 1693–1700. doi: 10.1016/j.apmr.2008.02.022
Lawrence, E. S., Coshall, C., Dundas, R., Stewart, J., Rudd, A. G., Howard, R., et al. (2001). Estimates of the prevalence of acute stroke impairments and disability in a multiethnic population. Stroke 32, 1279–1284. doi: 10.1161/01.STR.32.6.1279
Lee, S., Lee, Y. S., and Kim, J. (2018). Automated evaluation of upper-limb motor function impairment using Fugl-Meyer assessment. IEEE Transac. Neural Syst. Rehabil. Eng. 26, 125–134. doi: 10.1109/TNSRE.2017.2755667
Lencioni, T., Fornia, L., Bowman, T., Marzegan, A., Caronni, A., Turolla, A., et al. (2021). A randomized controlled trial on the effects induced by robot-assisted and usual-care rehabilitation on upper limb muscle synergies in post-stroke subjects. Sci. Rep. 11:5323. doi: 10.1038/s41598-021-84536-8
Leonardis, D., Barsotti, M., Loconsole, C., Solazzi, M., Troncossi, M., Mazzotti, C., et al. (2015). An EMG-controlled robotic hand exoskeleton for bilateral rehabilitation. IEEE Trans. Haptics 8, 140–151. doi: 10.1109/TOH.2015.2417570
Levin, M. F., Desrosiers, J., Beauchemin, D., Bergeron, N., and Rochette, A. (2004). Development and validation of a scale for rating motor compensations used for reaching in patients with hemiparesis: the reaching performance scale. Phys. Ther. 84, 8–22. doi: 10.1093/ptj/84.1.8
Li, S., Zhuang, C., Niu, C. M., Bao, Y., Xie, Q., Lan, N., et al. (2017). Evaluation of functional correlation of task-specific muscle synergies with motor performance in patients poststroke. Front. Neurol. 8:337. doi: 10.3389/fneur.2017.00337
Li, X., Wang, Y. C., Suresh, N. L., Rymer, W. Z., and Zhou, P. (2011). Motor unit number reductions in paretic muscles of stroke survivors. IEEE Trans. Inf. Technol. Biomed. 15, 505–512. doi: 10.1109/TITB.2011.2140379
Liang, T., Zhang, Q., Liu, X., Lou, C., Liu, X., and Wang, H. (2020). Time-frequency maximal information coefficient method and its application to functional corticomuscular coupling. IEEE Transac. Neural Syst. Rehabil. Eng. 28, 2515–2524. doi: 10.1109/TNSRE.2020.3028199
Lim, J. Y., Oh, M. K., Park, J., and Paik, N. J. (2020). Does measurement of corticospinal tract involvement add value to clinical behavioral biomarkers in predicting motor recovery after stroke? Neural Plast. 2020:8883839. doi: 10.1155/2020/8883839
Lin, I. H., Tsai, H. T., Wang, C. Y., Hsu, C. Y., Liou, T. H., and Lin, Y. N. (2019). Effectiveness and superiority of rehabilitative treatments in enhancing motor recovery within 6 months poststroke: a systemic review. Arch. Phys. Med. Rehabil. 100, 366–378. doi: 10.1016/j.apmr.2018.09.123
Liu, J., Sheng, Y., and Liu, H. (2019a). Corticomuscular coherence and its applications: a review. Front. Hum. Neurosci. 13:100. doi: 10.3389/fnhum.2019.00100
Liu, J., Sheng, Y., Zeng, J., and Liu, H. (2019b). Corticomuscular coherence for upper arm flexor and extensor muscles during isometric exercise and cyclically isokinetic movement. Front. Neurosci. 13:522. doi: 10.3389/fnins.2019.00522
Liu, Q., Liu, Y., Zhang, C., Ruan, Z., Meng, W., Cai, Y., et al. (2021). sEMG-based dynamic muscle fatigue classification using svm with improved whale optimization algorithm. IEEE Internet Things J. 7, 4387–4394. doi: 10.1109/JIOT.2021.3056126
Luengo-Fernandez, R., Violato, M., Candio, P., and Leal, J. (2020). Economic burden of stroke across Europe: a population-based cost analysis. Eur. Stroke J. 5, 17–25. doi: 10.1177/2396987319883160
Madden, R. H., and Bundy, A. (2019). The ICF has made a difference to functioning and disability measurement and statistics. Disabil. Rehabil. 41, 1450–1462. doi: 10.1080/09638288.2018.1431812
Maffiuletti, N. A., and Bendahan, D. (2009). Measurement Methods of Muscle Fatigue. London: Routledge, 36–66.
Maggio, M. G., Naro, A., Manuli, A., Maresca, G., Balletta, T., Latella, D., et al. (2021). Effects of robotic neurorehabilitation on body representation in individuals with stroke: a preliminary study focusing on an EEG-based approach. Brain Topogr. 34, 348–362. doi: 10.1007/s10548-021-00825-5
Mahadevan, N., Demanuele, C., Zhang, H., Volfson, D., Ho, B., Erb, M. K., et al. (2020). Development of digital biomarkers for resting tremor and bradykinesia using a wrist-worn wearable device. NPJ Dig. Med. 3:5. doi: 10.1038/s41746-019-0217-7
Maier, M., Ballester, B. R., and Verschure, P. (2019). Principles of neurorehabilitation after stroke based on motor learning and brain plasticity mechanisms. Front. Syst. Neurosci. 13:74. doi: 10.3389/fnsys.2019.00074
Majid, M. S. H., Khairunizam, W., Shahriman, A. B., Zunaidi, I., Sahyudi, B. N., and Zuradzman, M. R. (2018). “EMG feature extractions for upper-limb functional movement during rehabilitation,” in 2018 International Conference on Intelligent Informatics and Biomedical Sciences (ICIIBMS), 314–320.
Makaram, N., Karthick, P., Gopinath, V., and Swaminathan, R. (2021). Surface electromyography-based muscle fatigue analysis using binary and weighted visibility graph features. Fluctuation Noise Lett. 20:2150016. doi: 10.1142/S0219477521500164
Mane, R., Chew, E., Phua, K. S., Ang, K. K., Robinson, N., Vinod, A. P., et al. (2019). Prognostic and monitory EEG-biomarkers for BCI upper-limb stroke rehabilitation. IEEE Trans. Neural Syst. Rehabil. Eng. 27, 1654–1664. doi: 10.1109/TNSRE.2019.2924742
Manta, C., Mahadevan, N., Bakker, J., Ozen Irmak, S., Izmailova, E., Park, S., et al. (2021). EVIDENCE publication checklist for studies evaluating connected sensor technologies: explanation and elaboration. Dig. Biomark. 5, 127–147. doi: 10.1159/000515835
Manta, C., Patrick-Lake, B., and Goldsack, J. C. (2020). Digital measures that matter to patients: a framework to guide the selection and development of digital measures of health. Dig. Biomark. 4, 69–77. doi: 10.1159/000509725
Markopoulos, P., Timmermans, A. A. A., Beursgens, L., Donselaar, R.v, and Seelen, H. A. M. (2011). “Us'em: the user-centered design of a device for motivating stroke patients to use their impaired arm-hand in daily life activities,” in 2011 Annual International Conference of the IEEE Engineering in Medicine and Biology Society (IEEE), 5182–5187.
Martínez-Aguilar, G. M., and Gutiérrez, D. (2019). Using cortico-muscular and cortico-cardiac coherence to study the role of the brain in the development of muscular fatigue. Biomed. Signal Process. Control 48, 153–160. doi: 10.1016/j.bspc.2018.10.011
Martinez-Pernia, D. (2020). Experiential neurorehabilitation: a neurological therapy based on the enactive paradigm. Front. Psychol. 11:924. doi: 10.3389/fpsyg.2020.00924
Maselli, A., Dhawan, A., Russo, M., Cesqui, B., Lacquaniti, F., and d'Avella, A. (2019). A whole body characterization of individual strategies, gender differences, and common styles in overarm throwing. J. Neurophysiol. 122, 2486–2503. doi: 10.1152/jn.00011.2019
Mayeux, R. (2004). Biomarkers: potential uses and limitations. NeuroRx 1, 182–188. doi: 10.1602/neurorx.1.2.182
McManus, L., Lowery, M., Merletti, R., Søgaard, K., Besomi, M., Clancy, E. A., et al. (2021). Consensus for experimental design in electromyography (CEDE) project: terminology matrix. J. Electromyogr. Kinesiol. 59:102565. doi: 10.1016/j.jelekin.2021.102565
Mehrholz, J., Pohl, M., Platz, T., Kugler, J., and Elsner, B. (2018). Electromechanical and robot-assisted arm training for improving activities of daily living, arm function, and arm muscle strength after stroke. Cochrane Database Syst. Rev. 9:CD006876. doi: 10.1002/14651858.CD006876.pub5
Meng, Q., Zhang, J., and Yang, X. (2019). Virtual rehabilitation training system based on surface EMG feature extraction and analysis. J. Med. Syst. 43:48. doi: 10.1007/s10916-019-1166-z
Meyer-Rachner, P., Passon, A., Klauer, C., and Schauer, T. (2017). Compensating the effects of FES-induced muscle fatigue by rehabilitation robotics during arm weight support. Curr. Direct. Biomed. Eng. 3, 31–34. doi: 10.1515/cdbme-2017-0007
Miehlbradt, J., Pierella, C., Kinany, N., Coscia, M., Pirondini, E., Vissani, M., et al. (2019). Evolution of Cortical Asymmetry with Post-stroke Rehabilitation: A Pilot Study. Cham: Springer International Publishing, 1111–1115.
Mima, T., and Hallett, M. (1999). Corticomuscular coherence: a review. J. Clin. Neurophysiol. 16:501. doi: 10.1097/00004691-199911000-00002
Miraglia, F., Vecchio, F., and Rossini, P. M. (2018). Brain electroencephalographic segregation as a biomarker of learning. Neural Netw. 106, 168–174. doi: 10.1016/j.neunet.2018.07.005
Mohanty, R., Sinha, A. M., Remsik, A. B., Dodd, K. C., Young, B. M., Jacobson, T., et al. (2018). Machine learning classification to identify the stage of brain-computer interface therapy for stroke rehabilitation using functional connectivity. Front. Neurosci. 12:353. doi: 10.3389/fnins.2018.00353
Montoya, M. F., Muñoz, J. E., and Henao, O. A. (2020). Enhancing virtual rehabilitation in upper limbs with biocybernetic adaptation: the effects of virtual reality on perceived muscle fatigue, game performance and user experience. IEEE Transac. Neural Syst. Rehabil. Eng. 28, 740–747. doi: 10.1109/TNSRE.2020.2968869
Mugnosso, M., Marini, F., Gillardo, M., Morasso, P., and Zenzeri, J. (2017). “A novel method for muscle fatigue assessment during robot-based tracking tasks,” in 2017 International Conference on Rehabilitation Robotics (ICORR) (IEEE), 84–89.
Mugnosso, M., Marini, F., Holmes, M., Morasso, P., and Zenzeri, J. (2018). Muscle fatigue assessment during robot-mediated movements. J. Neuroeng. Rehabil. 15:119. doi: 10.1186/s12984-018-0463-y
Nandedkar, S. D., Nandedkar, D. S., Barkhaus, P. E., and Stalberg, E. V. (2004). Motor unit number index (MUNIX). IEEE Trans. Biomed. Eng. 51, 2209–2211. doi: 10.1109/TBME.2004.834281
National Academies of Sciences Engineering, and Medicine. (2021). Examining the Use of Biomarkers in Establishing the Presence and Severity of Impairments: Proceedings of a Workshop. National Academies Press.
Nazmi, N., Abdul Rahman, M. A., Yamamoto, S.-I., Ahmad, S. A., Zamzuri, H., and Mazlan, S. A. (2016). A review of classification techniques of emg signals during isotonic and isometric contractions. Sensors 16:1304. doi: 10.3390/s16081304
Negro, F., Bathon, K. E., Nguyen, J. N., Bannon, C. G., Orizio, C., Hunter, S. K., et al. (2020). Impaired firing behavior of individually tracked paretic motor units during fatiguing contractions of the dorsiflexors and functional implications post stroke. Front. Neurol. 11, 540893–540893. doi: 10.3389/fneur.2020.540893
Neuper, C., and Pfurtscheller, G. (2001). Event-related dynamics of cortical rhythms: frequency-specific features and functional correlates. Int. J. Psychophysiol. 43, 41–58. doi: 10.1016/S0167-8760(01)00178-7
Neuwirth, C., Burkhardt, C., Alix, J., Castro, J., de Carvalho, M., Gawel, M., et al. (2016). Quality Control of Motor Unit Number Index (MUNIX) measurements in 6 muscles in a single-subject “Round-Robin” setup. PLoS ONE 11:e0153948. doi: 10.1371/journal.pone.0153948
Neuwirth, C., Nandedkar, S., Stalberg, E., and Weber, M. (2010). Motor unit number index (MUNIX): a novel neurophysiological technique to follow disease progression in amyotrophic lateral sclerosis. Muscle Nerve 42, 379–384. doi: 10.1002/mus.21707
Nishikawa, K., Biewener, A. A., Aerts, P., Ahn, A. N., Chiel, H. J., Daley, M. A., et al. (2007). Neuromechanics: an integrative approach for understanding motor control. Integr. Comp. Biol. 47, 16–54. doi: 10.1093/icb/icm024
Nizamis, K., Athanasiou, A., Almpani, S., Dimitrousis, C., and Astaras, A. (2021). Converging robotic technologies in targeted neural rehabilitation: a review of emerging solutions and challenges. Sensors 21:2084. doi: 10.3390/s21062084
Norman, S., McFarland, D., Miner, A., Cramer, S., Wolbrecht, E., Wolpaw, J., et al. (2018). Controlling pre-movement sensorimotor rhythm can improve finger extension after stroke. J. Neural Eng. 15:056026. doi: 10.1088/1741-2552/aad724
Ondobaka, S., Ward, N., and Kuppuswamy, A. (2019). Inter-hemispheric inhibition in stroke survivors is related to fatigue and cortical excitability. bioRxiv [Preprint] 831511. doi: 10.1101/831511
Overduin, S. A., d'Avella, A., Roh, J., Carmena, J. M., and Bizzi, E. (2015). Representation of muscle synergies in the primate brain. J. Neurosci. 35, 12615–12624. doi: 10.1523/JNEUROSCI.4302-14.2015
Padalino, M., Scardino, C., Zito, G., Cancelli, A., Cottone, C., Bertoli, M., et al. (2021). Effects on motor control of personalized neuromodulation against multiple sclerosis fatigue. Brain Topogr. 34, 363–372. doi: 10.1007/s10548-021-00820-w
Pancholi, S., Jain, P., Varghese, A., and Joshi, A. M. (2019). “A novel time-domain based feature for emg-pr prosthetic and rehabilitation application,” in 2019 41st Annual International Conference of the IEEE Engineering in Medicine and Biology Society (EMBC) (IEEE), 5084–5087.
Papakostas, M., Kanal, V., Abujelala, M., Tsiakas, K., and Makedon, F. (2019). “Physical fatigue detection through EMG wearables and subjective user reports: a machine learning approach towards adaptive rehabilitation,” in Proceedings of the 12th ACM International Conference on PErvasive Technologies Related to Assistive Environments, Association for Computing Machinery (Rhodes), 475–481.
Pellegrino, G., Tomasevic, L., Tombini, M., Assenza, G., Bravi, M., Sterzi, S., et al. (2012). Inter-hemispheric coupling changes associate with motor improvements after robotic stroke rehabilitation. Restor. Neurol. Neurosci. 30, 497–510. doi: 10.3233/RNN-2012-120227
Pfurtscheller, G., and Lopes da Silva, F. H. (1999). Event-related EEG/MEG synchronization and desynchronization: basic principles. Clin. Neurophysiol. 110, 1842–57. doi: 10.1016/S1388-2457(99)00141-8
Pfurtscheller, G., Neuper, C., Andrew, C., and Edlinger, G. N. (1997). Foot and hand area mu rhythms. Int. J. Psychophysiol. 26, 121–135. doi: 10.1016/S0167-8760(97)00760-5
Pham, H., Ariga, Y., Tominaga, K., Oku, T., Nakayama, K., Uemura, M., et al. (2014). Extraction and implementation of muscle synergies in neuro-mechanical control of upper limb movement. Adv. Robot. 28, 745–757. doi: 10.1080/01691864.2013.876940
Philips, G. R., Daly, J. J., and Principe, J. C. (2017). Topographical measures of functional connectivity as biomarkers for post-stroke motor recovery. J. Neuroeng. Rehabil. 14:67. doi: 10.1186/s12984-017-0277-3
Phinyomark, A., Phukpattaranont, P., and Limsakul, C. (2012). Feature reduction and selection for EMG signal classification. Expert Syst. Appl. 39, 7420–7431. doi: 10.1016/j.eswa.2012.01.102
Picelli, A., Filippetti, M., Del Piccolo, L., Schena, F., Chelazzi, L., Della Libera, C., et al. (2020). Rehabilitation and biomarkers of stroke recovery: study protocol for a randomized controlled trial. Front. Neurol. 11:618200. doi: 10.3389/fneur.2020.618200
Pichiorri, F., Petti, M., Caschera, S., Astolfi, L., Cincotti, F., and Mattia, D. (2018). An EEG index of sensorimotor interhemispheric coupling after unilateral stroke: clinical and neurophysiological study. Eur. J. Neurosci. 47, 158–163. doi: 10.1111/ejn.13797
Pineda, J. A. (2005). The functional significance of mu rhythms: translating “seeing” and “hearing” into “doing”. Brain Res. Rev. 50, 57–68. doi: 10.1016/j.brainresrev.2005.04.005
Pirondini, E., Coscia, M., Minguillon, J., Millán, J. D. R., Van De Ville, D., and Micera, S. (2017). EEG topographies provide subject-specific correlates of motor control. Sci. Rep. 7:13229. doi: 10.1038/s41598-017-13482-1
Pirondini, E., Goldshuv-Ezra, N., Zinger, N., Britz, J., Soroker, N., Deouell, L. Y., et al. (2020). Resting-state EEG topographies: reliable and sensitive signatures of unilateral spatial neglect. Neuroimage Clin. 26, 102237–102237. doi: 10.1016/j.nicl.2020.102237
Pirondini, E., Pierella, C., Kinany, N., Coscia, M., Miehlbradt, J., Magnin, C., et al. (2018). “On the potential of EEG biomarkers to inform robot-assisted rehabilitation in stroke patients,” in International Conference on NeuroRehabilitation (Springer), 956–960.
Prabhakaran, S., Zarahn, E., Riley, C., Speizer, A., Chong, J. Y., Lazar, R. M., et al. (2008). Inter-individual variability in the capacity for motor recovery after ischemic stroke. Neurorehabil. Neural Repair 22, 64–71. doi: 10.1177/1545968307305302
Proudfoot, M., van Ede, F., Quinn, A., Colclough, G. L., Wuu, J., Talbot, K., et al. (2018). Impaired corticomuscular and interhemispheric cortical beta oscillation coupling in amyotrophic lateral sclerosis. Clin. Neurophysiol. 129, 1479–1489. doi: 10.1016/j.clinph.2018.03.019
Quinlan, E. B., Dodakian, L., See, J., McKenzie, A., Stewart, J. C., and Cramer, S. C. (2018). Biomarkers of rehabilitation therapy vary according to stroke severity. Neural Plast. 2018:9867196. doi: 10.1155/2018/9867196
Quinn, T. J., Dawson, J., Walters, M., and Lees, K. R. (2009). Reliability of the modified Rankin Scale: a systematic review. Stroke 40, 3393–3395. doi: 10.1161/STROKEAHA.109.557256
Ramos-Murguialday, A., Broetz, D., Rea, M., Läer, L., Yilmaz, Ö., Brasil, F. L., et al. (2013). Brain–machine interface in chronic stroke rehabilitation: a controlled study. Ann. Neurol. 74, 100–108. doi: 10.1002/ana.23879
Rech, K. D., Salazar, A. P., Marchese, R. R., Schifino, G., Cimolin, V., and Pagnussat, A. S. (2020). Fugl-Meyer assessment scores are related with kinematic measures in people with chronic hemiparesis after stroke. J. Stroke Cerebrovasc. Dis. 29:104463. doi: 10.1016/j.jstrokecerebrovasdis.2019.104463
Reinkensmeyer, D. J., Burdet, E., Casadio, M., Krakauer, J. W., Kwakkel, G., Lang, C. E., et al. (2016). Computational neurorehabilitation: modeling plasticity and learning to predict recovery. J. Neuroeng. Rehabil. 13:42. doi: 10.1186/s12984-016-0148-3
Remsik, A. B., Williams, L., Gjini, K., Dodd, K., Thoma, J., Jacobson, T., et al. (2019). Ipsilesional Mu rhythm desynchronization and changes in motor behavior following post stroke BCI intervention for motor rehabilitation. Front. Neurosci. 13:53. doi: 10.3389/fnins.2019.00053
Riahi, N., Vakorin, V. A., and Menon, C. (2020). Estimating Fugl-Meyer upper extremity motor score from functional-connectivity measures. IEEE Trans. Neural Syst. Rehabil. Eng. 28, 860–868. doi: 10.1109/TNSRE.2020.2978381
Rimbert, S., Lindig-León, C., Fedotenkova, M., and Bougrain, L. (2017). “Modulation of beta power in EEG during discrete and continuous motor imageries,” in 2017 8th International IEEE/EMBS Conference on Neural Engineering (NER) (IEEE), 333–336.
Rizzo, J.-R., Fung, J. K., Hosseini, M., Shafieesabet, A., Ahdoot, E., Pasculli, R. M., et al. (2017). Eye control deficits coupled to hand control deficits: eye–hand incoordination in chronic cerebral injury. Front. Neurol. 8:330. doi: 10.3389/fneur.2017.00330
Robinson, M. A., Vanrenterghem, J., and Pataky, T. C. (2021). Sample size estimation for biomechanical waveforms: Current practice, recommendations and a comparison to discrete power analysis. J. Biomech. 122:110451. doi: 10.1016/j.jbiomech.2021.110451
Rodgers, H., Bosomworth, H., Krebs, H. I., van Wijck, F., Howel, D., Wilson, N., et al. (2019). Robot assisted training for the upper limb after stroke (RATULS): a multicentre randomised controlled trial. Lancet 394, 51–62. doi: 10.1016/S0140-6736(19)31055-4
Rungsirisilp, N., and Wongsawat, Y. (2021). Combined action observation-and motor imagery-based brain computer interface (BCI) for stroke rehabilitation: a case report. doi: 10.21203/rs.3.rs-610878/v1
Ryu, J., Vero, J., Dobkin, R. D., and Torres, E. B. (2019). Dynamic digital biomarkers of motor and cognitive function in Parkinson's disease. JoVE 2019:e59827. doi: 10.3791/59827
Saes, M., Meskers, C. G. M., Daffertshofer, A., de Munck, J. C., Kwakkel, G., van Wegen, E. E. H., et al. (2019). How does upper extremity Fugl-Meyer motor score relate to resting-state EEG in chronic stroke? A power spectral density analysis. Clin. Neurophysiol. 130, 856–862. doi: 10.1016/j.clinph.2019.01.007
Samuel, O. W., Asogbon, M. G., Geng, Y., Jiang, N., Mzurikwao, D., Zheng, Y., et al. (2021). Decoding movement intent patterns based on spatiotemporal and adaptive filtering method towards active motor training in stroke rehabilitation systems. Neural Comput. Appl. 33, 4793–4806. doi: 10.1007/s00521-020-05536-9
Scano, A., Chiavenna, A., Malosio, M., Molinari Tosatti, L., and Molteni, F. (2018). Kinect V2 implementation and testing of the reaching performance scale for motor evaluation of patients with neurological impairment. Med. Eng. Phys. 56, 54–58. doi: 10.1016/j.medengphy.2018.04.005
Scano, A., Dardari, L., Molteni, F., Giberti, H., Tosatti, L. M., and d'Avella, A. (2019). A Comprehensive spatial mapping of muscle synergies in highly variable upper-limb movements of healthy subjects. Front. Physiol. 10:1231. doi: 10.3389/fphys.2019.01231
Schmidt, R. A., and Young, D. E. (1991). Methodology for motor learning: a paradigm for kinematic feedback. J. Mot. Behav. 23, 13–24. doi: 10.1080/00222895.1991.9941590
Schwartz, S. M., Wildenhaus, K., Bucher, A., and Byrd, B. (2020). Digital twins and the emerging science of self: implications for digital health experience design and “small” data. Front. Comput. Sci. 2:31. doi: 10.3389/fcomp.2020.00031
Schwarz, A., Kanzler, C. M., Lambercy, O., Luft, A. R., and Veerbeek, J. M. (2019). Systematic review on kinematic assessments of upper limb movements after stroke. Stroke 50, 718–727. doi: 10.1161/STROKEAHA.118.023531
Sebastián-Romagosa, M., Ortner, R., Udina-Bonet, E., Dinarès-Ferran, J., Mayr, K., Cao, F., et al. (2019). “Laterality coefficient: an EEG parameter related with the functional improvement in stroke patients,” in 2019 IEEE EMBS International Conference on Biomedical and Health Informatics (BHI), 1–4.
Sebastian-Romagosa, M., Udina, E., Ortner, R., Dinares-Ferran, J., Cho, W., Murovec, N., et al. (2020). EEG biomarkers related with the functional state of stroke patients. Front. Neurosci. 14:582. doi: 10.3389/fnins.2020.00582
Semprini, M., Laffranchi, M., Sanguineti, V., Avanzino, L., De Icco, R., De Michieli, L., et al. (2018). Technological approaches for neurorehabilitation: from robotic devices to brain stimulation and beyond. Front. Neurol. 9:212. doi: 10.3389/fneur.2018.00212
Severini, G., Koenig, A., Adans-Dester, C., Cajigas, I., Cheung, V. C. K., and Bonato, P. (2020). Robot-driven locomotor perturbations reveal synergy-mediated, context-dependent feedforward and feedback mechanisms of adaptation. Sci. Rep. 10:5104. doi: 10.1038/s41598-020-61231-8
Sieberts, S. K., Schaff, J., Duda, M., Pataki, B. Á., Sun, M., Snyder, P., et al. (2021). Crowdsourcing digital health measures to predict Parkinson's disease severity: the Parkinson's Disease Digital Biomarker DREAM Challenge. NPJ Dig. Med. 4:53. doi: 10.1038/s41746-021-00414-7
Siegel, J. S., Ramsey, L. E., Snyder, A. Z., Metcalf, N. V., Chacko, R. V., Weinberger, K., et al. (2016). Disruptions of network connectivity predict impairment in multiple behavioral domains after stroke. Proc. Nat. Acad. Sci. 113 E4367–E4376. doi: 10.1073/pnas.1521083113
Sinha, N., Dewald, J. P. A., Heckman, C. J., and Yang, Y. (2020). Cross-frequency coupling in descending motor pathways: theory and simulation. Front. Syst. Neurosci. 13:86. doi: 10.3389/fnsys.2019.00086
Skriver, K., Roig, M., Lundbye-Jensen, J., Pingel, J., Helge, J. W., Kiens, B., et al. (2014). Acute exercise improves motor memory: exploring potential biomarkers. Neurobiol. Learn. Mem. 116, 46–58. doi: 10.1016/j.nlm.2014.08.004
Stinear, C. M. (2017). Prediction of motor recovery after stroke: advances in biomarkers. Lancet Neurol. 16, 826–836. doi: 10.1016/S1474-4422(17)30283-1
Stinear, C. M., Byblow, W. D., Barber, P. A., Ackerley, S. J., Smith, M.-C., and Cramer, S. C. (2018). Biomarker-based patient selection improves stroke rehabilitation trial efficiency. bioRxiv [Preprint] 459776. doi: 10.1101/459776
Stinear, C. M., Lang, C. E., Zeiler, S., and Byblow, W. D. (2020). Advances and challenges in stroke rehabilitation. Lancet Neurol. 19, 348–360. doi: 10.1016/S1474-4422(19)30415-6
Stucki, G., Cieza, A., Ewert, T., Kostanjsek, N., Chatterji, S., and Ustun, T. B. (2002). Application of the International Classification of Functioning, Disability and Health (ICF) in clinical practice. Disabil. Rehabil. 24, 281–282. doi: 10.1080/09638280110105222
Svaerke, K. W., Omkvist, K. V., Havsteen, I. B., and Christensen, H. K. (2019). Computer-Based Cognitive rehabilitation in patients with visuospatial neglect or homonymous hemianopia after stroke. J. Stroke Cerebrovasc. Dis. 28:104356. doi: 10.1016/j.jstrokecerebrovasdis.2019.104356
Szczecinski, N. S., Hunt, A. J., and Quinn, R. D. (2017). Design process and tools for dynamic neuromechanical models and robot controllers. Biol. Cybern. 111, 105–127. doi: 10.1007/s00422-017-0711-4
Takemi, M., Masakado, Y., Liu, M., and Ushiba, J. (2013). Event-related desynchronization reflects downregulation of intracortical inhibition in human primary motor cortex. J. Neurophysiol. 110, 1158–1166. doi: 10.1152/jn.01092.2012
Tang, C.-W., Hsiao, F.-J., Lee, P.-L., Tsai, Y.-A., Hsu, Y.-F., Chen, W.-T., et al. (2020). β-oscillations reflect recovery of the paretic upper limb in subacute stroke. Neurorehabil. Neural Repair 34, 450–462. doi: 10.1177/1545968320913502
Tanzarella, S., Muceli, S., Del Vecchio, A., Casolo, A., and Farina, D. (2020). Non-invasive analysis of motor neurons controlling the intrinsic and extrinsic muscles of the hand. J. Neural Eng. 17:046033. doi: 10.1088/1741-2552/aba6db
Tariq, M., Trivailo, P. M., and Simic, M. (2018). EEG-based BCI control schemes for lower-limb assistive-robots. Front. Hum. Neurosci. 12:312. doi: 10.3389/fnhum.2018.00312
Tatti, E., Ricci, S., Mehraram, R., Lin, N., George, S., Nelson, A. B., et al. (2019). Beta modulation depth is not linked to movement features. Front. Behav. Neurosci. 13:49. doi: 10.3389/fnbeh.2019.00049
Trang, C., Lustig, T. A., and Snair, M. (2020). Examining the Use of Biomarkers in Establishing the Presence and Severity of Impairments: Proceedings of a Workshop.
Trujillo, P., Mastropietro, A., Scano, A., Chiavenna, A., Mrakic-Sposta, S., Caimmi, M., et al. (2017). Quantitative EEG for predicting upper limb motor recovery in chronic stroke robot-assisted rehabilitation. IEEE Trans. Neural Syst. Rehabil. Eng. 25, 1058–1067. doi: 10.1109/TNSRE.2017.2678161
Úbeda, A., Del Vecchio, A., Vujaklija, I., and Farina, D. (2019). Analysis of Intramuscular Motor Unit Coherence in the Tibialis Anterior Muscle as a Tool for the Assessment of Robot-Assisted Rehabilitation. Cham: Springer International Publishing, 231–235.
Ushiyama, J., Takahashi, Y., and Ushiba, J. (2010). Muscle dependency of corticomuscular coherence in upper and lower limb muscles and training-related alterations in ballet dancers and weightlifters. J. Appl. Physiol. 109, 1086–1095. doi: 10.1152/japplphysiol.00869.2009
Van de Ville, D., Britz, J., and Michel, C. M. (2010). EEG microstate sequences in healthy humans at rest reveal scale-free dynamics. Proc. Natl. Acad. Sci. U.S.A. 107, 18179–18184. doi: 10.1073/pnas.1007841107
van Putten, M. J. (2007). The revised brain symmetry index. Clin. Neurophysiol. 118, 2362–2367. doi: 10.1016/j.clinph.2007.07.019
Van Putten, M. J., and Tavy, D. L. (2004). Continuous quantitative EEG monitoring in hemispheric stroke patients using the brain symmetry index. Stroke 35, 2489–2492. doi: 10.1161/01.STR.0000144649.49861.1d
Vecchio, F., Tomino, C., Miraglia, F., Iodice, F., Erra, C., Di Iorio, R., et al. (2019). Cortical connectivity from EEG data in acute stroke: a study via graph theory as a potential biomarker for functional recovery. Int. J. Psychophysiol. 146, 133–138. doi: 10.1016/j.ijpsycho.2019.09.012
Venugopal, G., Navaneethakrishna, M., and Ramakrishnan, S. (2014). Extraction and analysis of multiple time window features associated with muscle fatigue conditions using sEMG signals. Expert Syst. Appl. 41, 2652–2659. doi: 10.1016/j.eswa.2013.11.009
Voigt, I., Inojosa, H., Dillenseger, A., Haase, R., Akgün, K., and Ziemssen, T. (2021). Digital twins for multiple sclerosis. Front. Immunol. 12:669811. doi: 10.3389/fimmu.2021.669811
Wagner, A. K. (2010). TBI translational rehabilitation research in the 21st Century: exploring a Rehabilomics research model. Eur. J. Phys. Rehabil. Med. 46, 549–556.
Wagner, A. K. (2014). A Rehabilomics framework for personalized and translational rehabilitation research and care for individuals with disabilities: perspectives and considerations for spinal cord injury. J. Spinal Cord Med. 37, 493–502. doi: 10.1179/2045772314Y.0000000248
Wagner, A. K. (2017). TBI rehabilomics research: an exemplar of a biomarker-based approach to precision care for populations with disability. Curr. Neurol. Neurosci. Rep. 17:84. doi: 10.1007/s11910-017-0791-5
Wagner, A. K., and Kumar, R. G. (2019). TBI rehabilomics research: conceptualizing a humoral triad for designing effective rehabilitation interventions. Neuropharmacology 145, 133–144. doi: 10.1016/j.neuropharm.2018.09.011
Wagner, A. K., and Sowa, G. (2014). Rehabilomics research: a model for translational rehabilitation and comparative effectiveness rehabilitation research. Am. J. Phys. Med. Rehabil. 93, 913–916. doi: 10.1097/PHM.0000000000000114
Wagner, A. K., and Zitelli, K. T. (2013). A Rehabilomics focused perspective on molecular mechanisms underlying neurological injury, complications, and recovery after severe TBI. Pathophysiology 20, 39–48. doi: 10.1016/j.pathophys.2012.02.007
Wang, J., Sun, Y., and Sun, S. (2020). Recognition of muscle fatigue status based on improved wavelet threshold and CNN-SVM. IEEE Access 8, 207914–207922. doi: 10.1109/ACCESS.2020.3038422
Wang, K. K. W., Zhang, Z., and Kobeissy, F. H. (2014). Biomarkers of Brain Injury and Neurological Disorders. CRC Press, 236–264. Available online at: https://books.google.it/books?id=YKrNBQAAQBAJ
Wang, L., Xie, Z., Lu, A., Lu, T., Zhang, S., Zheng, F., et al. (2020). Antagonistic muscle prefatigue weakens the functional corticomuscular coupling during isometric elbow extension contraction. Neuroreport 31, 372–380. doi: 10.1097/WNR.0000000000001387
Wang, L.-J., Yu, X.-M., Shao, Q.-N., Wang, C., Yang, H., Huang, S.-J., et al. (2020). Muscle fatigue enhance beta band EMG-EMG coupling of antagonistic muscles in patients with post-stroke spasticity. Front. Bioeng. Biotechnol. 8:1007. doi: 10.3389/fbioe.2020.01007
Wang, W., Li, H., Kong, D., Xiao, M., and Zhang, P. (2020). A novel fatigue detection method for rehabilitation training of upper limb exoskeleton robot using multi-information fusion. Int. J. Adv. Robot. Syst. 17:1729881420974295. doi: 10.1177/1729881420974295
Wang, X., Seguin, C., Zalesky, A., Wong, W.-W., Chu, W. C.-W., and Tong, R. K.-Y. (2019). Synchronization lag in post stroke: relation to motor function and structural connectivity. Netw. Neurosci. 3, 1121–1140. doi: 10.1162/netn_a_00105
Wentink, M., van Bodegom-Vos, L., Brouns, B., Arwert, H., Houdijk, S., Kewalbansing, P., et al. (2019). How to improve eRehabilitation programs in stroke care? A focus group study to identify requirements of end-users. BMC Med. Inform. Decis. Mak. 19:145. doi: 10.1186/s12911-019-0871-3
Wilkinson, B., and van Boxtel, R. (2020). The medical device regulation of the European Union intensifies focus on clinical benefits of devices. Ther. Innov. Regul. Sci. 54, 613–617. doi: 10.1007/s43441-019-00094-2
World Health Organization (2002). Towards a Common Language for Functioning, Disability, and Health: ICF. The International Classification of Functioning, Disability and Health.
Xin, X., Gao, Y., Zhang, H., Cao, K., and Shi, Y. (2012). Correlation of continuous electroencephalogram with clinical assessment scores in acute stroke patients. Neurosci. Bull. 28, 611–617. doi: 10.1007/s12264-012-1265-z
Yuan, K., Chen, C., Wang, X., Chu, W. C.-W., and Tong, R. K.-Y. (2021). BCI training effects on chronic stroke correlate with functional reorganization in motor-related regions: a concurrent EEG and fMRI study. Brain Sci. 11:56. doi: 10.3390/brainsci11010056
Yuan, K., Wang, X., Chen, C., Lau, C. C. Y., Chu, W. C. W., and Tong, R. K. Y. (2020). Interhemispheric functional reorganization and its structural base after BCI-guided upper-limb training in chronic stroke. IEEE Transac. Neural Syst. Rehabil. Eng. 28, 2525–2536. doi: 10.1109/TNSRE.2020.3027955
Zariffa, J. (2018). “Improving neurorehabilitation of the upper limb through big data,” in Signal Processing and Machine Learning for Biomedical Big Data (CRC Press), 533–550.
Zhang, C., Li-Tsang, C. W., and Au, R. K. (2017). Robotic approaches for the rehabilitation of upper limb recovery after stroke: a systematic review and meta-analysis. Int. J. Rehabil. Res. 40, 19–28. doi: 10.1097/MRR.0000000000000204
Zhang, H., Deng, K., Li, H., Albin, R. L., and Guan, Y. (2020). Deep learning identifies digital biomarkers for self-reported parkinson's disease. Patterns 1:100042. doi: 10.1016/j.patter.2020.100042
Zhang, X., Tang, X., Zhu, X., Gao, X., Chen, X., and Chen, X. (2019). A regression-based framework for quantitative assessment of muscle spasticity using combined EMG and inertial data from wearable sensors. Front. Neurosci. 13:398. doi: 10.3389/fnins.2019.00398
Keywords: robotic rehabilitation, upper limb rehabilitation, motor control, EMG, EEG, kinematic measurement, stroke, exoskeleton
Citation: Garro F, Chiappalone M, Buccelli S, De Michieli L and Semprini M (2021) Neuromechanical Biomarkers for Robotic Neurorehabilitation. Front. Neurorobot. 15:742163. doi: 10.3389/fnbot.2021.742163
Received: 15 July 2021; Accepted: 22 September 2021;
Published: 27 October 2021.
Edited by:
Diego Torricelli, Consejo Superior de Investigaciones Científicas (CSIC), SpainReviewed by:
Ye Ma, Ningbo University, ChinaLongbin Zhang, Royal Institute of Technology, Sweden
Marta Gandolla, Politecnico di Milano, Italy
Copyright © 2021 Garro, Chiappalone, Buccelli, De Michieli and Semprini. This is an open-access article distributed under the terms of the Creative Commons Attribution License (CC BY). The use, distribution or reproduction in other forums is permitted, provided the original author(s) and the copyright owner(s) are credited and that the original publication in this journal is cited, in accordance with accepted academic practice. No use, distribution or reproduction is permitted which does not comply with these terms.
*Correspondence: Marianna Semprini, bWFyaWFubmEuc2VtcHJpbmlAaWl0Lml0