A Dual Nanosensor Approach to Determine the Cytosolic Concentration of ATP in Astrocytes
- 1Carl-Ludwig-Institute for Physiology, Faculty of Medicine, University Leipzig, Leipzig, Germany
- 2Wilhelm-Ostwald-Schule, Gymnasium der Stadt Leipzig, Leipzig, Germany
- 3Department of Neurogenetics, Max-Planck-Institute for Experimental Medicine, Göttingen, Germany
Adenosine triphosphate (ATP) is the central energy carrier of all cells and knowledge on the dynamics of the concentration of ATP ([ATP]) provides important insights into the energetic state of a cell. Several genetically encoded fluorescent nanosensors for ATP were developed, which allow following the cytosolic [ATP] at high spatial and temporal resolution using fluorescence microscopy. However, to calibrate the fluorescent signal to [ATP] has remained challenging. To estimate basal cytosolic [ATP] ([ATP]0) in astrocytes, we here took advantage of two ATP nanosensors of the ATeam-family (ATeam1.03; ATeam1.03YEMK) with different affinities for ATP. Altering [ATP] by external stimuli resulted in characteristic pairs of signal changes of both nanosensors, which depend on [ATP]0. Using this dual nanosensor strategy and epifluorescence microscopy, [ATP]0 was estimated to be around 1.5 mM in primary cultures of cortical astrocytes from mice. Furthermore, in astrocytes in acutely isolated cortical slices from mice expressing both nanosensors after stereotactic injection of AAV-vectors, 2-photon microscopy revealed [ATP]0 of 0.7 mM to 1.3 mM. Finally, the change in [ATP] induced in the cytosol of cultured cortical astrocytes by application of azide, glutamate, and an increased extracellular concentration of K+ were calculated as −0.50 mM, −0.16 mM, and 0.07 mM, respectively. In summary, the dual nanosensor approach adds another option for determining the concentration of [ATP] to the increasing toolbox of fluorescent nanosensors for metabolites. This approach can also be applied to other metabolites when two sensors with different binding properties are available.
Introduction
The metabolism of the brain is realized by a joint effort of all cell types including neurons, glial cells as well as cells constituting the blood vessels. Almost all aspects of metabolism in the brain involve several types of cells, including energy metabolism (Pellerin and Magistretti, 1994; Guzmán and Blázquez, 2001; Barros and Deitmer, 2010; Nave, 2010a; Hirrlinger and Nave, 2014; Barros et al., 2018b; Díaz-García and Yellen, 2019; Vicente-Gutierrez et al., 2019; Zuend et al., 2020), and neurotransmitter metabolism (van den Berg et al., 1978; Waagepetersen et al., 2003; Bak et al., 2006; Le Douce et al., 2020). Furthermore, brain cells are structurally intermingled, heavily interdigitating their numerous cellular processes (Somjen, 1988; Grosche et al., 1999; Bushong et al., 2002; Nave, 2010b). Therefore, to study the dynamics of brain metabolism in detail, methods allowing to assess metabolites at high spatial and temporal resolution are required. These requirements are not fulfilled by most of the classical biochemical methods, as well as by techniques like PET or fMRI.
However, fluorescence nanosensors for metabolites are well suited to follow the concentration of a growing set of metabolites at high spatial and temporal resolution in vitro, in situ, and in vivo (Imamura et al., 2009; Hung et al., 2011; San Martin et al., 2014; Lange et al., 2015; Mächler et al., 2016; Mongeon et al., 2016; Trevisiol et al., 2017; Winkler et al., 2017; Köhler et al., 2018; Barros et al., 2018a; Díaz-García et al., 2019; Zuend et al., 2020). Conceptually, these sensors are proteins composed of a domain which specifically binds the metabolite of interest as well as one or more fluorescent proteins, which change their fluorescent properties upon metabolite binding. Different types of such sensors have been described. In single fluorophore sensors the fluorophore changes its fluorescence intensity upon metabolite binding. In ratiometric sensors the signal change is either derived from Förster resonance energy transfer (FRET) involving two fluorescent proteins. Alternatively, a second “reference fluorophore” is integrated in the sensor protein, which is unaffected by metabolite binding. Examples of such sensors include sensors for adenosine triphosphate (ATP; e.g., sensors of the ATeam family, Imamura et al., 2009; Queen, Yaginuma et al., 2014; Takaine et al., 2019), the ATP/ADP ratio (Perceval, Berg et al., 2009; PercevalHR, Nguyen et al., 2019), the NADH/NAD+-redox ratio (Peredox, Hung et al., 2011; Sonar, Zhao et al., 2015), NADPH (iNap family, Zhao et al., 2016), glucose (FlipGlu, Fehr et al., 2003; SweetieTS, Díaz-García et al., 2019), lactate (Laconic, San Martín et al., 2013), and pyruvate (Pyronic, San Martín et al., 2014; PyronicSF, Arce-Molina et al., 2020).
Fluorescent nanosensors for metabolites can be used to monitor relative changes of the respective metabolite within a cell or cellular compartment during an experiment by monitoring the time course of the fluorescent signal. However, the fluorescence signal is often not linearly related to the concentration of the metabolite (or only in a small concentration range). Furthermore, all sensors show a basal, metabolite independent fluorescence. Therefore, to calculate the concentration of the metabolite from this fluorescence signal has remained challenging and several different approaches have been employed (San Martin et al., 2014; Yellen and Mongeon, 2015; Barros et al., 2018a). All of these different approaches have their own advantages, but also limitations (see discussion section and Table 1). We here add a novel approach to the toolbox of fluorescent nanosensors for metabolites, which allows estimating the intracellular concentration of a metabolite under basal conditions by combining two nanosensors for the same metabolite with different binding equilibrium constants. This approach is exemplified using two ATP sensitive nanosensors (ATeam1.03, abbreviated AT, and ATeam1.03YEMK, abbreviated ATY) which differ in their dissociation constants (kD; Imamura et al., 2009). The feasibility of this approach is shown in cultured cortical astrocytes as well as in cortical astrocytes in situ in acutely isolated brain slices.
Materials and Methods
Ethics Statement
In accordance with the guidelines for the welfare of experimental animals issued by the European Communities Council Directive (2010/63/EU) and with the German Protection of Animals Act (Tierschutzgesetz), mice were bred in the animal facility of the Medical Faculty of the University of Leipzig. Mice were housed in individually ventilated cages in a specific pathogen free environment in a 12 h/12 h light dark cycle with access to food and water ad libitum. Experiments were approved by the animal welfare office of the Faculty of Medicine, University of Leipzig and the governmental authorities of Saxony (Landesdirektion Sachsen, registration number T20/16; TVV62/15).
Cell Culture and Transfection
Plasmids pDR-GW AT1.03, pDR-GW AT1.03YEMK, and pDR-GW AT1.03R122K/R126K (Bermejo et al., 2010) were obtained from Wolf Frommer (via Addgene; plasmids 28003, 28004, and 28005). The open reading frames of ATeam1.03, ATeam1.03YEMK, and AT1.03R122K/R126K (Imamura et al., 2009) were subcloned into pDEST expression vectors using Gateway cloning. Primary cortical astrocytes were prepared from the brains of newborn mice of the C57Bl/6J background as described (Requardt et al., 2010, 2012; Winkler et al., 2017). Cells were seeded on glass coverslips (30 mm diameter) with custom made silicon rings, which allow splitting the surface of the coverslip into two independent chambers (Supplementary Figure 1), and were cultured in DMEM/10% FCS/25 mM glucose for 1 week. The medium was exchanged to DMEM/10% FCS/5 mM glucose and cells were further cultured for at least 7 days with exchange of the medium twice per week. Cells on one coverslip were transfected with expression plasmids encoding ATeam1.03 in one chamber and ATeam1.03YEMK in the other chamber using lipofectamine (Thermo Fisher Scientific, Schwerte, Germany) and the standard protocol suggested by the supplier. Cells were used for experiments 1 day after transfection.
Epifluorescence Imaging
Epifluorescence live cell imaging was performed as essentially described before (Winkler et al., 2017). In brief, coverslips were mounted into a custom-made flow chamber which was continuously perfused at 37°C with incubation medium containing (in mM) 145 NaCl, 5.4 KCl, 1 MgCl2, 1.8 CaCl2, 20 HEPES, 0.8 Na2HPO4, 5 glucose; pH 7.4; bubbled with 20% O2, 80% N2 to ensure a constant O2 partial pressure in all experiments. Cells were observed using an Axio-ObserverZ1 microscope (Zeiss, Jena, Germany) using a Plan-Apochromat 20x/0.8 objective and an Axiocam 506 camera (688 × 552 pixels, 4 × 4 binning, pixel size 0.91 μm × 0.91 μm) with acquisition times of 150 ms (CFP) and 100 ms (FRET). The two channels were recorded with the following filter sets: CFP: excitation 436/25 nm, beam splitter 455 nm, and emission 480/40 nm; and FRET: excitation 436/25 nm, beam splitter 455 nm, and emission 535/30 nm. Using the motorized x-y stage of the microscope, images of cells in both chambers were recorded almost simultaneously with only the time delay necessary to move the stage to the other positions (<1 s). Images were obtained every 20 s. At the end of each experiment, iodoacetate (1 mM; Applichem, Darmstadt, Germany) and sodium azide (10 mM; Serva, Heidelberg, Germany) were applied. This treatment blocks all ATP-producing pathways allowing to normalize the sensor signal to nominally [ATP] = 0.
AAV Injection and 2-Photon Imaging in Acute Brain Slices
To image the dynamics of ATP in cortical astrocytes in acute brain slices, ATeam1.03 or ATeam1.03YEMK-open reading frames (Imamura et al., 2009) were cloned into a vector for packaging into adeno-associated viruses (AAV) and astrocyte-specific expression was driven by GFAP-promoter elements (Lee et al., 2008; Mächler et al., 2016; Stobart et al., 2018). The plasmids were packaged into AAV serotype 5 by the Viral Vector Facility at the Neuroscience Center Zurich, University of Zurich, Switzerland. 0.5 μl of AAV containing solution (virus titer: 2.2 × 1012 vg/ml (AT); 2.75 × 1012 vg/ml (ATY) were stereotactically injected into cortex of 2 to 3 month old mice (coordinates: 0.5 mm caudal to bregma, lateral 2.0 mm, and ventral 1.1 mm), i.e., in the primary somatosensory cortex. 4 weeks later, mice were sacrificed and 250 μm thick acute coronal brain slices were prepared (Pätz et al., 2018). Slices were transferred to the stage of a 2-photon laser scanning microscope (Olympus FV1000) equipped with a XLPlan N 25x/1.05 W objective (Olympus) and a Mai Tai DeepSee laser (Spectra-Physics, Darmstadt, Germany) and continuously superfused at room temperature with aCSF solution (in mM): 130 NaCl, 2.5 KCl, 1 MgCl2, 2 CaCl2 1.25 NaH2PO4, 26 NaHCO3, 10 glucose, pH 7.4. The medium was continuously bubbled with carbogen. Imaging was performed within 500 μm of the site of AAV injection. The following imaging parameters were used: excitation: 810 nm; emission 510 DC XR (F33-511 OD, 266685; AHF, Germany); pixel size: 0.552 × 0.552 μm; 512 × 512 pixels; pixel dwell time: 2 μs; time resolution: 60 s; stack of 23 single z-planes. All experiments were started by perfusing the slice with aCSF for 20 min followed by aCSF containing 1 μM TTX (Tocris, Bristol, United Kingdom) to prevent neuronal activity and to define the sensor signals under baseline conditions. Afterward, glutamate (100 μM in aCSF) was applied for 20 min. At the end of each experiment, 1 mM iodoacetate and 10 mM azide was added to deplete the cells of ATP. All solutions were adjusted to same pH and osmolality.
Data Analysis and Analytical Solution
For a detailed description of the variables and indices used for this calculation see Supplementary Table 1. Regions of interest (ROI), each containing a single cell, were defined manually using Fiji (Schindelin et al., 2012). Background subtracted mean fluorescence intensities averaged over all pixels within a ROI were determined for the FRET (IFRET) and the CFP (ICFP) channel. The ratio of the intensities (I) was calculated as I = IFRET/ICFP. The relative sensor signal ratio (R) was calculated as
where Imin is I at [ATP] of 0 mM and I0 being the pre-treatment baseline I at the unknown baseline [ATP]0. This results in a minimum R (Rmin) of 0 for [ATP] of 0 mM and a pre-treatment R value (R0) of 1 at the unknown baseline [ATP]0. Notably, R as defined by Eq. 1, does not directly relate to the sensor occupancy B, defined as
with nH being the Hill-coefficient and kD the dissociation constant. B scales between 0 (at [ATP] = 0) and 1 (at full saturation of the sensor; Figures 1A,B). However, while at [ATP] = 0 both, B and R are equal to 0, R = 1 at [ATP]0 for each experiment but B = 1 at full saturation of the sensor (Figures 1A,B). As [ATP]0 within a cell is not known, the value of B0 at baseline conditions is also not known; therefore, a direct calculation of changes in [ATP] (d[ATP]) from changes in R (dR) is not possible.
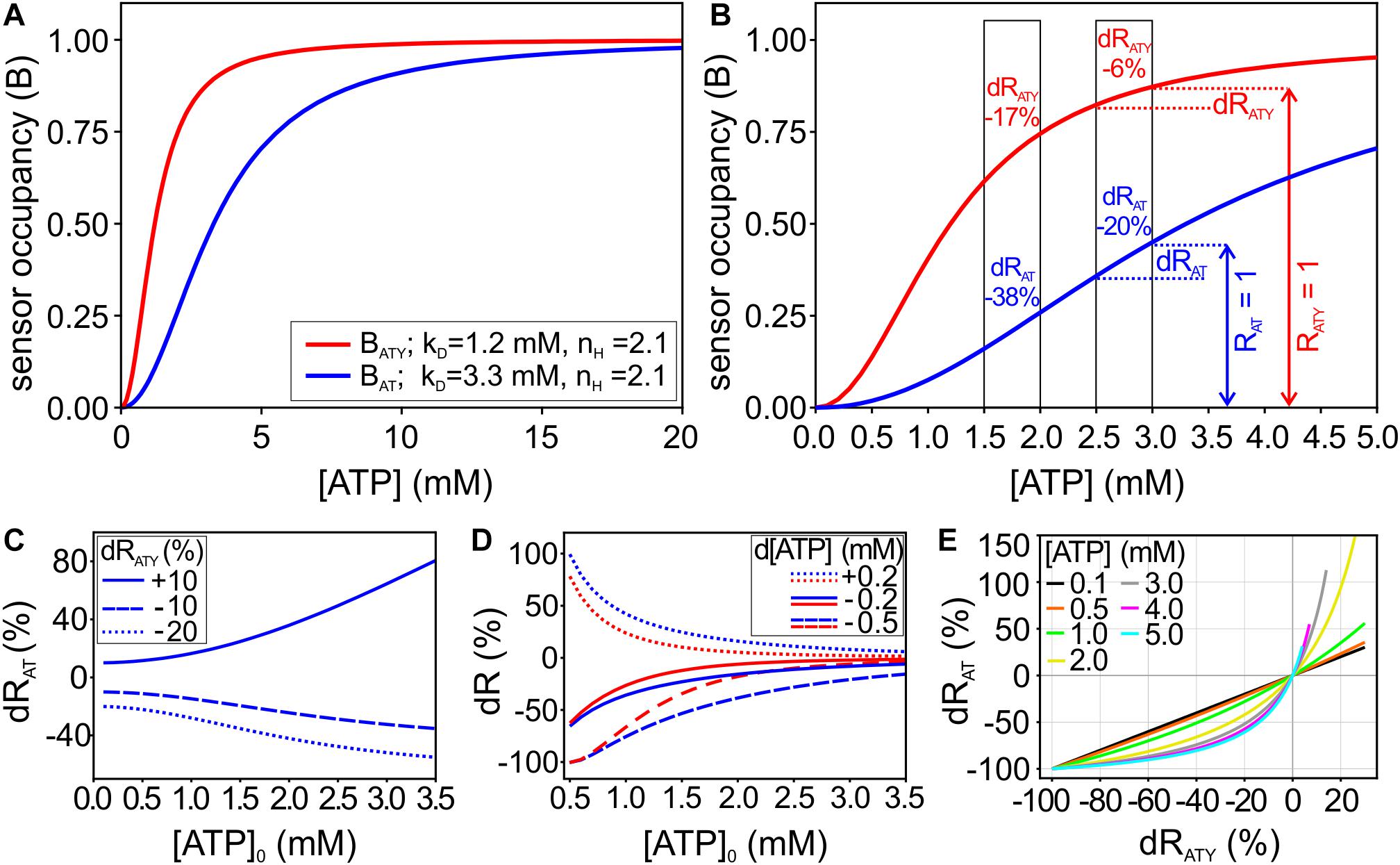
Figure 1. Mathematical framework of the dual nanosensor approach. (A) Binding curves for the ATP sensors ATeam1.03 (AT; blue) and ATeam1.03YEMK (ATY; red), showing the sensor occupancy (B) at different cytosolic [ATP]. (B) Enlargement of A for [ATP] from 0 to 5 mM. The boxes highlight two examples with d[ATP] = −0.5 mM starting at [ATP]0 = 2 mM and [ATP]0 = 3 mM, respectively. Note that RAT = 1 and RATY = 1 (i.e., the sensor signal at the starting [ATP]0, indicated by the vertical arrows for the example of [ATP]0 = 3 mM) correspond to different values of sensor occupancy (B) for both sensors. The resulting relative changes of the sensor signals dRAT and dRATY are given within the boxes. (C) Changes of RAT (dRAT) depending on [ATP]0 at given values of dRATY (dRATY = −10%, −20%, and +10%). (D) A change in [ATP] results in a characteristic pair of signal changes dRAT and dRATY, which depend also on [ATP]0. Shown are examples of d[ATP] = −0.2 mM, d[ATP] = −0.5 mM, and d[ATP] = +0.2 mM. (E) For each [ATP]0, unique pairs of dRAT and dRATY describe a given d[ATP]. Therefore, [ATP]0 can be deduced from pairs of dRAT and dRATY obtained experimentally.
If R values at full saturation (Rmax) can be measured, it is possible to relate R to the metabolite concentration, as has been done for the ATeam-type ATP sensors using purified sensor proteins (Imamura et al., 2009):
Different from this Rmax approach, the dual nanosensor approach introduced here avoids the necessity to determine Rmax. Yet, it still relies on knowledge about kD and nH (Table 1). To calculate [ATP]0 and [ATP] during treatment ([ATP]treat), dR values for measurements with AT and ATY, respectively, were obtained experimentally for different treatments; kD and nH values for the indicators were taken from the literature. The following sets of parameters were used:
kD,AT = 3.3 mM, kD,ATY = 1.2 mM, nH,AT = nH,ATY = 2.1 (Imamura et al., 2009).
kD,AT = 9.4 mM, kD,ATY = 2.7 mM, nH,AT = nH,ATY = 1.0 (Lerchundi et al., 2020).
To calculate [ATP]0 and [ATP]treat from dRAT and dRATY measured in the experiments, a system of two non-linear equations was used which allowed deriving analytical solutions:
Introducing R according to Eq. 3 into Eqs 4a,b the following equations result (for simplicity “nH” is replaced by “n” in Eqs 5a,b and 6a,b):
Solving Eqs 5a,b as a system of non-linear equations using “Solve” of Mathematica 12.1. yielded the following analytical expressions for [ATP]0 and [ATP]treat:
Of note, this analytical solution requires that nH,AT = nH,ATY, a prerequisite met by the ATeam sensors. If nH,1 ≠ nH,2, solving the system of non-linear equations results in expressions involving complex numbers. In this case, numerical solving of Eqs 5a,b using “NSolve” of Mathematica is preferable.
Having established the mean [ATP]0 of all cells observed in a given experiment according to Eq. 6a, R values (Eq. 1) can be correlated to sensor occupancy B. First, B0 is calculated from [ATP]0 using Eq. 2. Then, all R values are multiplied by B0 resulting in R values reflecting sensor occupancy with a range of R = 0 at [ATP] = 0 to R = Rmax = 1 at saturation of the sensor. Consecutively, [ATP] for each single cell at any time point during an experiment (including [ATP]0) is revealed by
Using Eq. 7, [ATP] can be calculated for both sensors separately. Finally, rates of [ATP] changes were calculated by linear regression of [ATP] over the time period of interest.
Data Processing and Presentation
Microscopic images were processed using Zeiss ZEN software, Fiji and Corel Draw X4 Graphic. Data were analyzed and calculated using Fiji and Microsoft Excel. Analytical solutions of the system of non-linear equations as well as values for [ATP]0 and [ATP]treat according to Eqs. 6a,b were obtained using Mathematica. Diagrams were generated using Sigma Plot. In the boxplots, the box spans from the 25th to 75th percentile, the whiskers span from the 10th to the 90th percentile and dots highlighting the 5th to the 95th percentile. In addition, in Figures 5A,B all outliers are indicated by light blue and light red circles. Within the box dashed lines represent the mean value, solid lines show the median. In Figure 2A, lines represent the mean and the shaded area indicates the standard deviation (SD). In Figures 2B–D circles and associated error bars represent the mean ± SD. All data presented in Figures 1–5 are obtained on 3 independent astrocytic cell cultures. Data in the text are given as mean ± SD. Final illustrations were arranged using Corel Draw X4 Graphic.
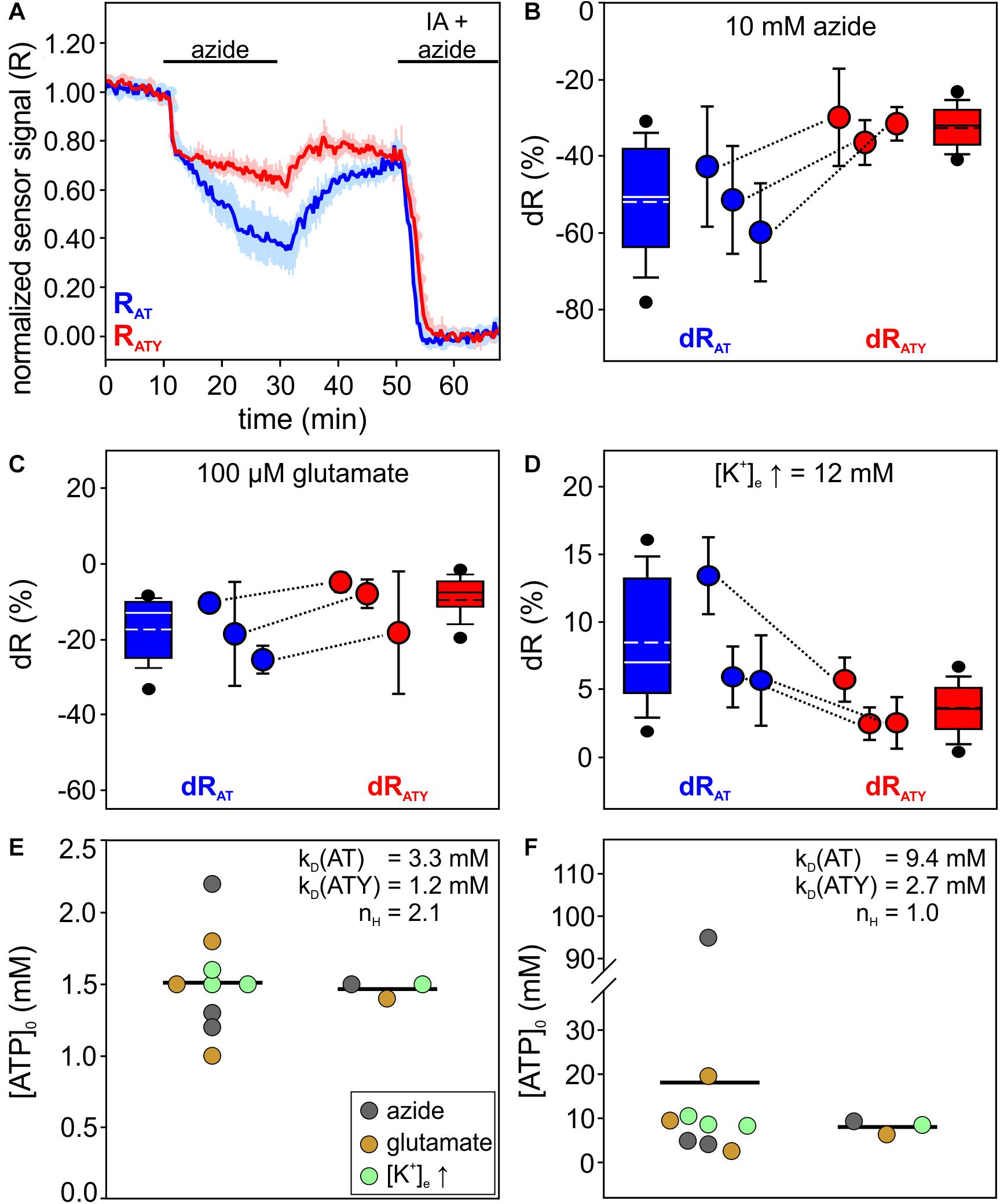
Figure 2. Determination of [ATP]0 in primary cultured cortical astrocytes using the dual nanosensor approach. (A) Time course of the signal of AT (blue) and ATY (red) during an experiment exposing the cells to azide (10 mM). At the end of the experiment, cells were treated with iodoacetate (IA) and azide to deplete cellular ATP. Shown is the normalized sensor signal R for all cells of one representative experiment (n = 25 cells for both AT and ATY; mean ± SD). (B–D) Signal changes dR obtained for AT (dRAT; blue) and ATY (dRATY; red) during incubation of astrocytes with azide (B; 10 mM, 20 min), glutamate (C; 100 μM, 15 min) or an increase in [K+]e (D; from 5.4 mM to 12 mM, 10 min). Shown is the distribution of dR of all cells (box plots), as well as the mean ± SD of each experiment (circles and error bars). Corresponding means of both sensors from the paired experiments are connected with lines. Each condition was replicated in N = 3 independent experiments. In each experiment, the following number of cells were analyzed: B, AT: 50, 50, 40. B, ATY: 48, 50, 47. C, AT: 50, 17, 40; C, ATY: 49, 44, 34. D, AT: 43, 46, 44; and D, ATY: 44, 46, 36. (E) [ATP]0 values calculated from the dRAT – dRATY pairs shown in panels (B–D), using the parameters indicated within the graph. On the left, values calculated from the dRAT – dRATY pairs from the paired experiments (circles) as well as the mean (line) are shown. Right: values obtained from the dRAT – dRATY pairs obtained from the pooled cells of all experiments. (F) Same calculation as in (E), but with different values of kD and nH as indicated within the graph.
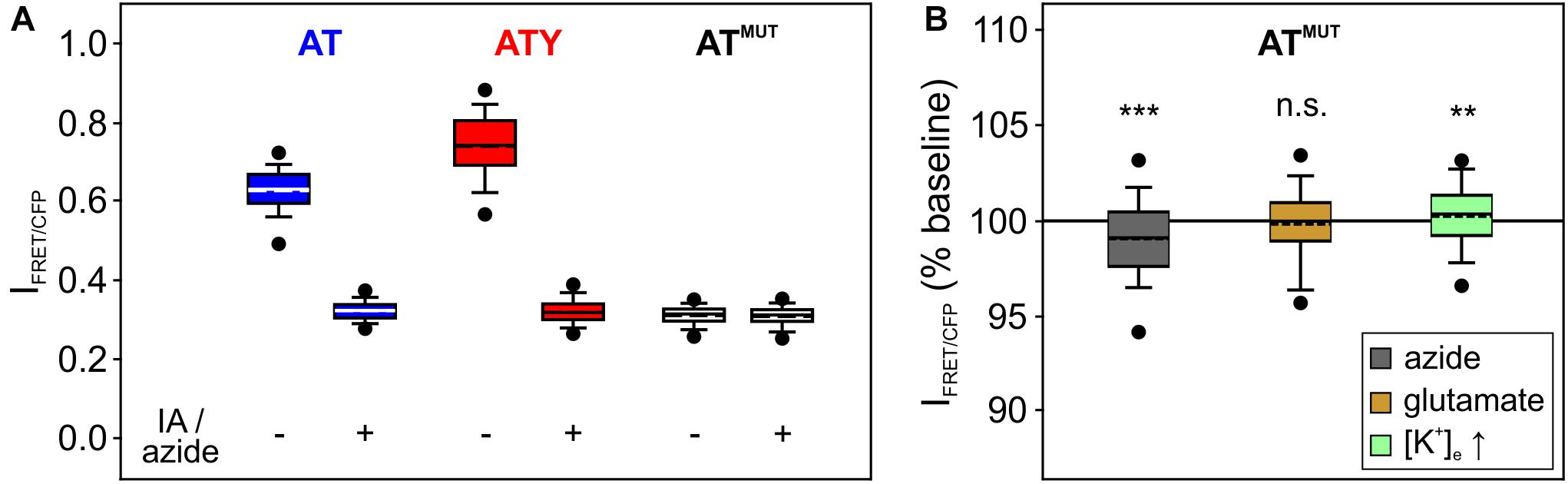
Figure 3. Control experiments using the ATP-binding deficient AT1.03R122K/R126K. (A) The signal of the ATP sensor AT and ATY during inhibition of ATP synthesis approaches the signal of AT1.03R122K/R126K (ATMUT). Shown is the non-normalized intensity ratio I = IFRET/ICFP to allow for comparison between AT, ATY, and ATMUT in the absence or presence of IA + azide. The following number of cells was included in the analysis (from left to right): 381; 381; 400; 400; 810; 810 (each from N = 9 experiments). (B) The signal of ATMUT is only marginally affected by application of azide, glutamate or increases in [K+]e. ***p < 0.001; **p < 0.01; and n.s. p > 0.05 compared to baseline, paired t-test. n = 285, 260, and 265 cells from 3 experiments each for azide, glutamate and high [K+], respectively.
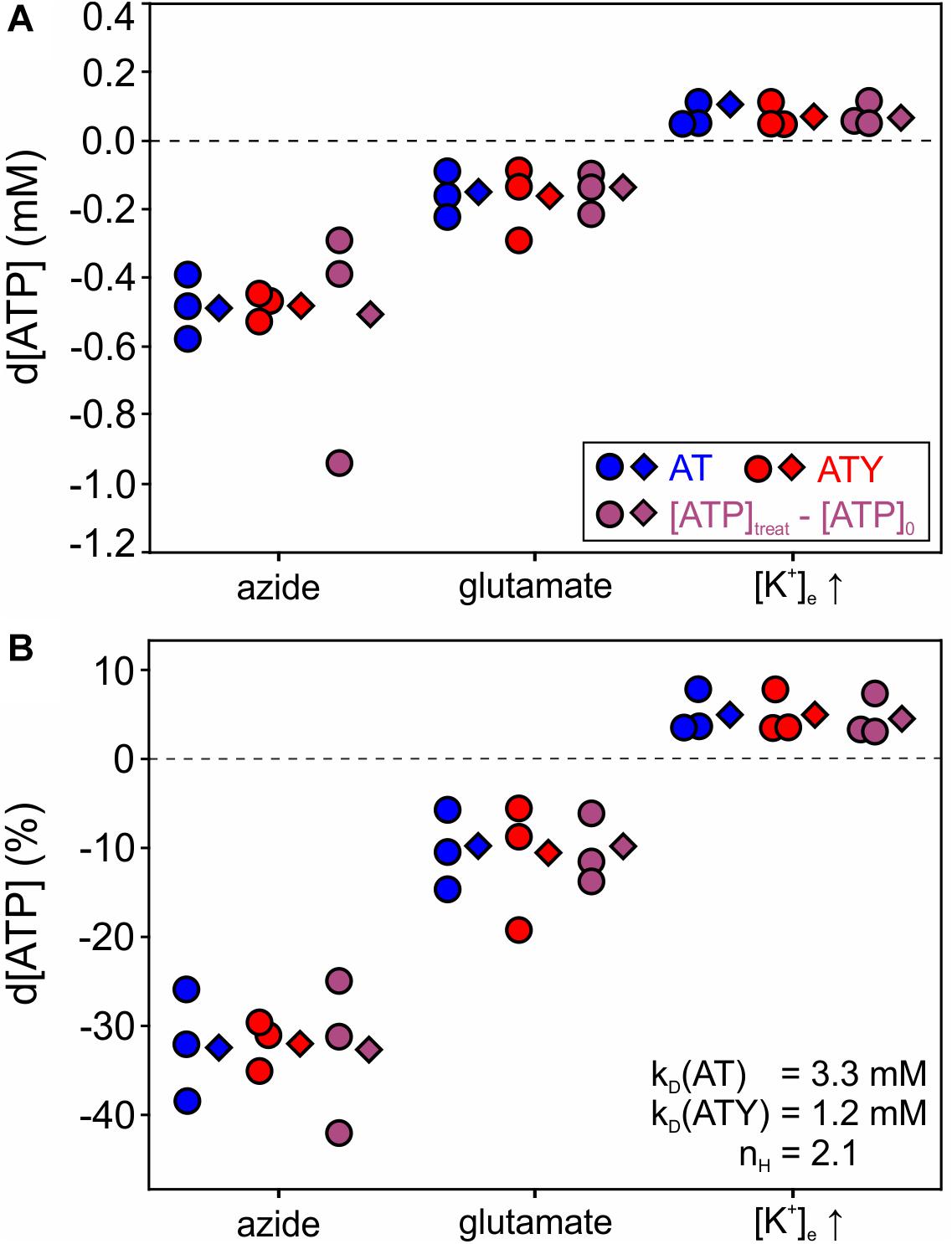
Figure 4. Changes in cytosolic [ATP] in astrocytes during application of azide, glutamate and increasing [K+]e. Astrocytes were incubated with azide (10 mM, 20 min), glutamate (100 μM, 15 min), or increased [K+]e (12 mM, 10 min) and d[ATP] was calculated from nanosensor signals of AT (blue), ATY (red), or as d[ATP] = [ATP]treat – [ATP]0 derived from Eqs 6a,b (violet). Circles represent data calculated from individual paired experiments (imaging of AT and ATY on the same coverslip in parallel), while diamonds represent calculation using pooled data from all experiments. kD and nH values used for this analysis are from Imamura et al. (2009) and are indicated in the figure. (A) d[ATP] (mM). (B) Same data as in A expressed as relative change (%) of [ATP]. All experiments were performed with N = 3 independent experiments, for number of cells in each experiment see legend of Figure 2.
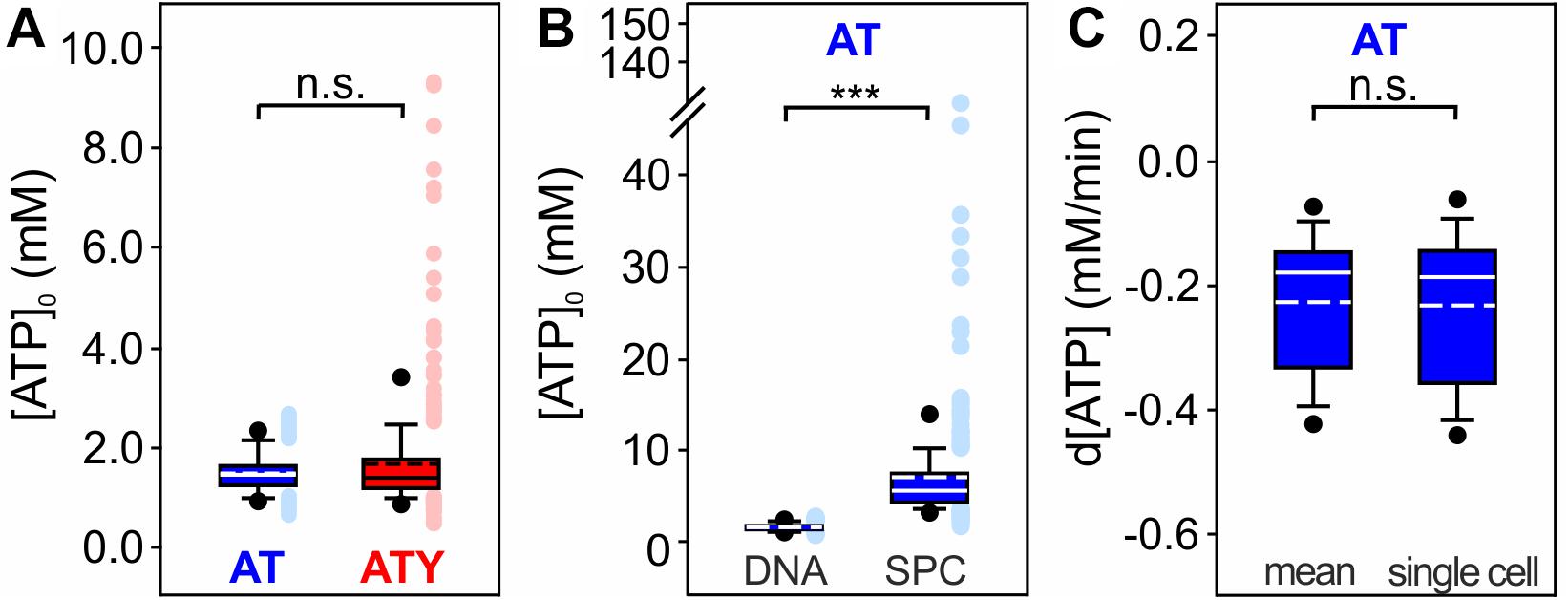
Figure 5. Single cell [ATP]0 and analysis of the dynamics of changes in [ATP]0. (A) [ATP]0 of each single cell calculated from the sensor signal of AT or ATY. n.s. p > 0.05; Mann–Whitney Rank Sum Test; n = 382 and 394 cells from N = 3 experiments for AT and ATY, respectively. (B) Comparison of single cell [ATP]0 obtained by the dual nanosensor approach (DNA) and a single point calibration protocol (SPC) assuming Rmax = 2.3 × Rmin (Imamura et al., 2009). The analysis was restricted to the AT sensor as no estimate for Rmax for ATY is available. Data for the dual nanosensor approach is the same as in panel (A). ***p < 0.001; Mann–Whitney Rank Sum Test; n = 382 and 346 cells from N = 3 experiments for dual nanosensor approach and single point calibration, respectively. (C) Rate of change of [ATP] during exposure of the cells to azide (10 mM). Shown is the slope of a linear regression for the first 1.3 min of azide application. Rates were calculated either using the mean [ATP]0 (mean, left box) or [ATP]0 calculated for each cell individually (single cell, right box); however, no significant difference was observed (p > 0.05; Mann–Whitney Rank Sum Test; n = 140 cells from N = 3 experiments).
Results
The ATP sensors ATeam1.03 (abbreviated AT in the following) and ATeam1.03YEMK (abbreviated as ATY) are two cytosolic, ATP specific nanosensors, which differ in their affinity for ATP (Imamura et al., 2009). Dissociation constants (kD) of 3.3 mM and 1.2 mM were described for AT and ATY, respectively; for both sensors a Hill coefficient nH of 2.1 was found (Imamura et al., 2009). Therefore, the occupancy of the sensor (i.e., the ratio of ATP bound sensor/total sensor; referred to as B here; see Eq. 2) is different for both sensors at a given cytosolic concentration of ATP ([ATP]; Figure 1A). The [ATP] of a cell under baseline resting conditions ([ATP]0) is a very important parameter for cellular energy metabolism, which is difficult to determine and not known in many cases. We reasoned that a treatment of cells, which results in a given change in [ATP] (d[ATP]), will cause different relative changes of the sensor signal for AT (dRAT) and ATY (dRATY; Figure 1B). For example, at [ATP]0 = 2 mM a change in [ATP] of −0.5 mM will result in dRAT = −38% and dRATY = −17%; while at [ATP]0 = 3 mM the same change in ATP will result in dRAT = −20% and dRATY = −6% (Figure 1B). Therefore, at a given dRATY, the corresponding dRAT correlates to [ATP]0, or else, depending on [ATP]0 a change in [ATP] will result in a characteristic pair of values for dRAT and dRATY (Figures 1C,D). Accordingly, [ATP]0 can be derived from pairs of dRAT and dRATY obtained by treating cells with conditions which change [ATP] (Figure 1E). Importantly, for this approach prior knowledge of the actual d[ATP] caused by these conditions is not necessary.
To test this approach experimentally, primary cultured cortical astrocytes were studied, which have been reported before to respond to different stimuli with changes in [ATP] (Winkler et al., 2017). After transfection with either AT or ATY, cells were imaged by epifluorescence microscopy in a custom-made system allowing to image cells transfected with the different plasmids in parallel in the same experiment. Cells readily responded to treatments with a change in [ATP] reflected by both AT and ATY (Figure 2A). At the end of each experiment, cells were incubated with iodoacetate (IA) and azide to block cellular ATP production thereby depleting cellular ATP, allowing to normalize the sensor signal to the sensor signal at [ATP] = 0 mM (Figure 2A).
Cells were treated with three different conditions: (a) 10 mM azide (Figure 2B); (b) 100 μM glutamate (Figure 2C); and (c) by increasing the extracellular concentration of potassium ([K+]e) from 5.4 mM to 12 mM (Figure 2D). From the resulting pairs of dRAT and dRATY, [ATP]0 was calculated both for the single experiments with simultaneous imaging of both AT and ATY as well as for the data pooled from all experiments using the same condition (Figure 2E, Eq. 6a). Both types of analysis revealed a resting, basal [ATP]0 of about 1.5 mM (Figure 2E; 1.51 ± 0.35 mM, n = 9 experiments/1.47 ± 0.06 mM; N = 3 conditions).
The calculation of [ATP]0 using this dual nanosensor approach depends on knowledge of the kD values of the two versions of the sensor. In the original description of ATP sensors of the ATeam family kD values of 3.3 mM and 1.2 mM (and nH,AT = nH,ATY = 2.1) have been reported for AT and ATY, respectively (Imamura et al., 2009). However, based on calibration of the sensors in astrocytes and neurons in organotypic brain slice cultures, recently kD values of 9.4 mM and 2.7 mM were determined (Gerkau et al., 2019; Lerchundi et al., 2020). The latter studies used a Michaelis–Menten equation for fitting the data, which inherently implies nH = 1. [ATP]0 calculated using these parameters (Figure 2F) revealed 18.10 ± 29.23 mM (n = 9 experiments) and 8.04 ± 1.54 mM (N = 3 conditions). These values are substantially higher compared to previously published data on astrocytes and neurons (1 mM to 4 mM; Fukuda et al., 1983; Ainscow et al., 2002; Mollajew et al., 2013; Rangaraju et al., 2014; Toloe et al., 2014; Pathak et al., 2015), suggesting that this set of parameters might not be fully applicable on the experimental system used here. Despite these uncertainties regarding the kD values, these analyses show that the dual nanosensor approach is well suited to estimate the intracellular cytosolic [ATP] within cells. We note, that for further analysis of the data obtained on cultured astrocytes (Figures 4, 5) the original kD and nH values will be used (i.e., kD,AT = 3.3 mM, kD,ATY = 1.2 mM, nH,AT = nH,ATY = 2.1; Imamura et al., 2009).
Two crucial assumptions are inherent in this approach: (a) treatment of the cells with iodoacetate + azide depletes the cell of cytosolic ATP; (b) no other factors, like, e.g., pH, affect the sensor signal during treatment of the cells. To test the validity of these assumptions, experiments were repeated with cells expressing AT1.03R122K/R126K (abbreviated ATMUT), a mutated version of the ATeam sensors consisting of the same fluorophores and the same ATP binding protein, but which does not bind ATP due to two point mutations within the ATP binding domain (Imamura et al., 2009). First, the signal of ATMUT is very similar to the signal of AT and ATY during application of iodoacetate + azide (Figure 3A), suggesting that cytosolic [ATP] reaches nominally zero and confirming previous observations (Trevisiol et al., 2017; Winkler et al., 2017). Secondly, when cells expressing ATMUT were treated with azide, glutamate or with increased [K+]e, only a minor change in the ATMUT signal was observed (Figure 3B; azide: −0.9 ± 2.9%; n = 285 cells; glutamate: −0.2 ± 2.2%; n = 260 cells; [K+]e: 0.2 ± 2.2%; n = 265 cells). As ATMUT has the same properties as AT and ATY with the exception of ATP binding, these data indicate that other factors than [ATP] do not contribute substantially to the change of the sensor signal of AT and ATY under the conditions of these experiments.
Having established [ATP]0 using the dual nanosensor approach, the changes in [ATP] induced by the different treatments were calculated (Figure 4, Eq. 6b). Exposure of astrocytes to azide, which blocks the respiratory chain, reduced [ATP] by −0.50 ± 0.15 mM reflecting −32.4 ± 5.2% of [ATP]0 (Figure 4; n = 3 experiments with 285 cells analyzed), indicating that astrocytes maintain the cytosolic [ATP] at about 70% of the basal value in the absence of oxidative phosphorylation. Of note, vice versa this finding also implies that glycolysis alone is not sufficient to maintain [ATP] at control levels. Furthermore, application of glutamate (100 μM, 15 min) resulted in a reduction of [ATP] of about −0.16 ± 0.06 mM (−10.8 ± 5.0%; n = 3 experiments with 234 cells analyzed; Figure 4). Finally, increasing [K+]e induced a rise in [ATP] of 0.07 ± 0.04 mM, i.e., 4.7 ± 2.4% (n = 3 experiments with 259 cells analyzed; Figure 4). The results were very similar irrespective of whether each experiment was analyzed separately, or whether all data from all cells were pooled prior to analysis (compare circles and diamonds in Figure 4), suggesting that paired experiments are not a prerequisite of the dual nanosensor approach. Furthermore, similar results were obtained when calculating d[ATP] from the AT or ATY signal (blue and red symbols in Figure 4), or by calculating d[ATP] = [ATP]treat – [ATP]0 using the equations derived from solving the system of non-linear equations (Eqs 6a,b; violet symbols in Figure 4).
Astrocytes are a heterogenous cell population which also includes heterogeneity of metabolism (Bittner et al., 2010; Matyash and Kettenmann, 2010; Zhang and Barres, 2010; Bayraktar et al., 2015; Farmer and Murai, 2017; Köhler et al., 2018, 2019; Miller, 2018; Morel et al., 2018; Batiuk et al., 2020). Therefore, information on the heterogeneity of [ATP]0 within the astrocytic cell population is of major interest. While calculating [ATP]0 using the dual nanosensor method initially results in the mean [ATP]0 of all cells included in the analysis, [ATP]0 for each single cell can be obtained in a second calculation step using the mean [ATP]0 as additional parameter. This analysis revealed similar results when calculated either from the AT or ATY signals (Figure 5A; AT: 1.51 ± 0.40 mM; n = 382 cells from N = 9 experiments; ATY: 1.70 ± 1.06 mM; n = 394 cells from N = 9 experiments). However, the ATY-based calculation resulted in more cells with very high [ATP]0, most likely because the ATY binding curve (Figure 1A) is flat in this concentration range and even very small differences in RATY result in a rather large difference in [ATP]0.
Another method to obtain single cell data from fluorescent nanosensor imaging is the single point calibration protocol (e.g., Sotelo-Hitschfeld et al., 2012; Fernández-Moncada and Barros, 2014; Arce-Molina et al., 2020). Only one nanosensor is needed for this approach, and kD and nH are derived from other experimental systems like in the dual nanosensor approach (Table 1). In addition, an estimate for Rmax needs to be included in the calculation. For AT, a dynamic range of Rmax = 2.3 × Rmin has been reported (Imamura et al., 2009). As no explicit information on Rmax of ATY is available, only data obtained by imaging of AT were reanalyzed using the single point calibration protocol (Figure 5B). This analysis revealed a higher mean [ATP]0 and a larger variability of [ATP]0 compared to the dual nanosensor approach (Figure 5B; 7.00 ± 8.08 mM; n = 346 cells from N = 9 experiments).
Calibration of fluorescent nanosensors to the concentration of the metabolite also allows assessing kinetic changes of the concentration of the metabolite. As an example, the rate of the [ATP] decrease during inhibition of oxidative phosphorylation by azide was calculated, using either [ATP]0 obtained as the mean [ATP]0 of all cells within an experiment or [ATP]0 calculated for each cell individually (Figure 5C). No significant differences were observed between the two modes of calculation (p > 0.05; Mann–Whitney Rank Sum Test). [ATP] decreased with a rate of −0.23 ± 0.11 mM/min (Figure 5C; data calculated with mean [ATP]0; n = 140 cells from N = 3 experiments).
Finally, to validate the dual nanosensor approach in a more intact system than primary cultured cortical astrocytes, AT and ATY were expressed in cortical astrocytes in vivo by stereotactic injection of AAV vectors, in which expression of the sensor is driven by the GFAP promoter. Acute brain slices were prepared from these mice and imaged using 2-photon microscopy (Figures 6A,B). Application of glutamate induced changes in [ATP] and corresponding values of dRAT and dRATY were recorded (Figure 6C). As the two sensors were not expressed within the same mice, no pairing of experiments was possible. Based on the two different sets of kD-values (Imamura et al., 2009; Lerchundi et al., 2020), a basal [ATP]0 of 0.7 mM or 1.3 mM was calculated, respectively (Figure 6D), showing that the dual nanosensor approach can also be applied to more intact preparations like acute brain slices as well as to other imaging techniques like 2-photon laser scanning microscopy.
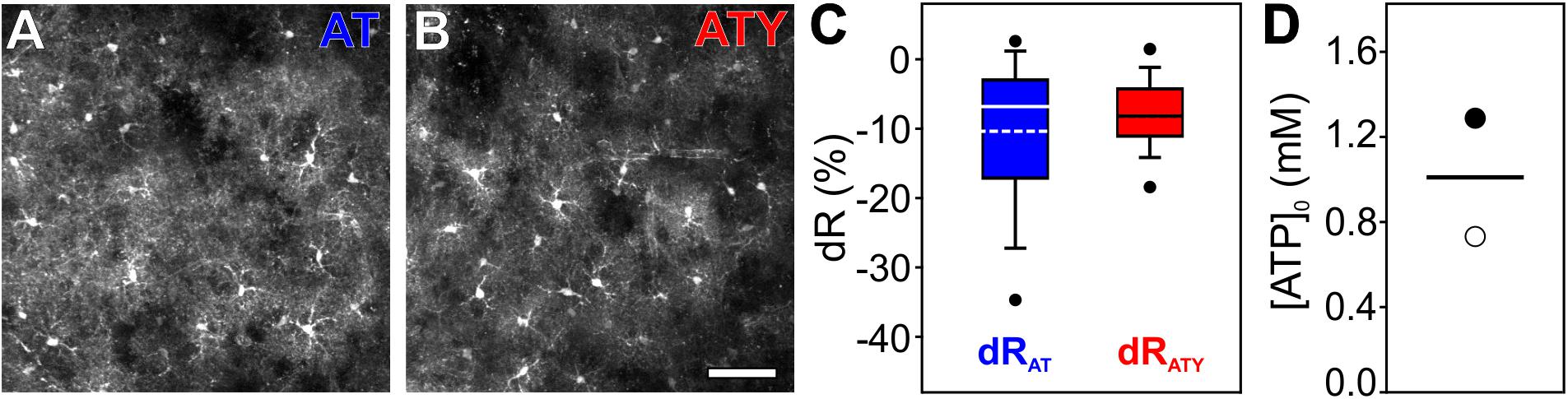
Figure 6. Determination of [ATP]0 in cortical astrocytes in acutely isolated brain slices using 2-photon imaging. AT or ATY were expressed in astrocytes in the primary somatosensory cortex in vivo by stereotactic injections of AAV-based vectors and acutely isolated brain slices were prepared. (A, B) 2-photon images showing expression of AT (A) or ATY (B) in cortical astrocytes. Scale bar: 50 μm. (C) dR values obtained for AT (blue) and ATY (red) during incubation of the slices with glutamate (100 μM) for 20 min. (D) [ATP]0 values obtained from the dRAT-dRATY pair shown in C calculated using the following sets of parameters: kD,AT = 3.3 mM, kD,ATY = 1.2 mM, nH = 2.1 (open circle; Imamura et al., 2009); and kD,AT = 9.4 mM, kD,ATY = 2.7 mM, nH = 1 (filled circle; Lerchundi et al., 2020). n = 80, 92 cells from N = 4, 6 mice were included in the analysis shown in (C and D) for AT and ATY, respectively.
Discussion
Fluorescent nanosensors for metabolites have strongly contributed to a much deeper knowledge on the metabolism and its dynamics of the mammalian brain (and of other organs and organisms; Deuschle et al., 2006; Tsuyama et al., 2013; Yaginuma et al., 2014; Masia et al., 2018; Volkenhoff et al., 2018; Nguyen et al., 2019; Takaine et al., 2019; Arce-Molina et al., 2020; Kioka et al., 2020). As these sensors are proteins which can be genetically encoded, they allow cell type specific expression using specific promoters as well as subcellular targeting using appropriate targeting sequences. Combined with different state-of-the art microscopy technologies, the dynamics of metabolites can be followed in cultured cells, in tissue preparations like brain slices or the isolated optic nerve, but also in vivo in living and even awake animals (Bittner et al., 2011; Ruminot et al., 2011; Mächler et al., 2016; Díaz-García et al., 2017, 2019; Trevisiol et al., 2017; Köhler et al., 2018; Baeza-Lehnert et al., 2019; Gerkau et al., 2019; Lerchundi et al., 2019; Arce-Molina et al., 2020; Zuend et al., 2020). However, while these nanosensors readily allow for monitoring relative changes of the metabolite concentration, deduction of absolute concentrations and absolute concentration changes (i.e., in mol/l) during treatments has remained challenging as calibration of the signal of the nanosensors to the actual concentration of the metabolite is hampered by both, theoretical and practical problems (Barros et al., 2018a). We here introduce the dual nanosensor approach, a novel strategy to determine [ATP]0 using two nanosensors for ATP with different binding equilibrium constants kD.
Properties, Assumptions and Limitations of the Dual Nanosensor Approach
The dual nanosensor approach requires the availability of two nanosensors sensitive to the concentration of the metabolite of interest (here ATP), which differ in their binding equilibrium constants kD (Table 1). Parallel experiments with cells expressing one or the other sensor reveal the signal change dR of both sensors (Figure 2). If both sensors would differ in their spectral properties, which is unfortunately not the case for AT and ATY (but has been reported for ATP sensors using a single fluorophore; Arai et al., 2018), both sensors could even be recorded within a single cell. Values for kD and nH need to be obtained from other experimental systems, e.g., from measurements of the purified sensor protein (Imamura et al., 2009), assuming that these values can be applied to the indicators in the cytosolic environment of the cells of interest (Table 1). While such transfer of parameters is often used also for other calibration strategies (e.g., Sotelo-Hitschfeld et al., 2012; Fernández-Moncada and Barros, 2014; Arce-Molina et al., 2020), several studies indicate that parameters of various genetically encoded nanosensors can be affected by the cellular environment (Hires et al., 2008; Pérez Koldenkova and Nagai, 2013; Yaginuma et al., 2014; Lerchundi et al., 2019, 2020). Moreover, care has to be taken that these values are determined by appropriate methods including similar or –ideally– identical excitation and emission wavelengths (Pomorski et al., 2013). In addition, Rmin needs to be measured for each cell during the experiments (Table 1), thereby requiring a method to deplete the cells from ATP. Application of azide, which blocks oxidative phosphorylation at complex IV of the respiratory chain, and iodoacetate, which blocks glycolysis at glycerine-aldehyde-3-phosphate dehydrogenase (GAPDH), resulted in a decrease of the AT- and ATY-sensor signal reaching the signal of the ATP-binding deficient AT1.03R122K/R126K (ATMUT; Figure 3A), suggesting that the fluorescence signal observed under these conditions is at least close to Rmin and reflects a concentration of cytosolic ATP of nominally zero. This observation is in line with previous observations using the same treatment to deplete ATP (Trevisiol et al., 2017; Winkler et al., 2017), but also with a different approach blocking glycolysis using 2-deoxyglucose and oxidative phosphorylation by oligomycin (Shulman et al., 2015). Finally, it has to be assumed that a given treatment of a cell results in the same change of [ATP] when either AT or ATY is expressed. This might be of special importance if the concentration of the analyte of interest is within the same range (or lower) as the concentration of the sensor, e.g., in the case of Ca2+-sensors. However, [ATP]0 is at least one to two orders of magnitude higher than the typical concentration of genetically expressed sensor proteins (Barros et al., 2018a), suggesting that the difference in kD of the two sensors does not have a major influence on the dynamics of [ATP].
The dual nanosensors approach adds another option to the list of strategies for quantifying metabolite concentrations from fluorescent signals. The approach eliminates the necessity to determine Rmax (or dRmax) of the nanosensor (Table 1) and is applicable to complex tissues like, e.g., the highly myelinated axons in the optic nerve (Trevisiol et al., 2017) or the brain in vivo, because it does not require experimental control of the metabolite concentration within the cell compartment of interest. Furthermore, any stimulation, which results in a reproducible change of the concentration of the metabolite, can be used to calculate [ATP]0 without prior knowledge of the actual concentration change d[ATP]. Finally, once the mean [ATP]0 of the observed cells was determined, [ATP]0 of each single cell can be calculated (Table 1 and Figure 5A). Therefore, this approach will be an interesting option for determining basal metabolite concentrations based on fluorescence imaging.
Comparison to Other Methods for Calibration of Nanosensors
In the following, two other methods of calibration of metabolic nanosensors will be discussed and compared to the dual nanosensor approach: (a) single point calibration (e.g., Sotelo-Hitschfeld et al., 2012; Fernández-Moncada and Barros, 2014; Arce-Molina et al., 2020); and (b) full calibration within the cell(-compartment) of interest (used, e.g., in Bittner et al., 2010; Hung et al., 2011; San Martín et al., 2013; Mongeon et al., 2016; Köhler et al., 2018).
For calculating the metabolite concentration using the single point calibration method, kD and nH obtained in other experimental systems are needed and Rmin has to be determined in each experiment (Table 1) similar to the dual nanosensor approach. While only one nanosensor is needed, additionally an estimate of Rmax is required. However, Rmax of, for example, the lactate sensor Laconic and the pyruvate sensor Pyronic differs by a factor of two between purified protein and when expressed in cells (San Martín et al., 2013, 2014). Therefore, a determination of Rmax within the cell of interest is preferable, but requires experimental access to increase the concentration of the metabolite to saturating levels. Such a saturation of the sensor is feasible for metabolites for which endogenous transporters with favorable kinetic properties are expressed allowing equilibration of the extra- and intracellular concentration of the metabolite under appropriate experimental conditions, as exemplified, e.g., for glucose, lactate and pyruvate (Bittner et al., 2010; San Martín et al., 2013, 2014; Mächler et al., 2016; Arce-Molina et al., 2020). However, cells do not express transporters for ATP. Therefore, one option to increase [ATP] would be to inhibit all ATP consuming processes and to rely on cellular ATP production. However, inhibition of all ATP consuming enzymes is not feasible, and the total amount of adenine nucleotides, which can be phosphorylated to ATP, might be insufficient to achieve sensor saturation. Alternatively, cell membranes are permeabilized without leakage of the sensor protein to allow access of ATP from the extracellular environment (Gerkau et al., 2019; Lerchundi et al., 2020). However, the permeabilizing agent itself or changes of the intracellular ion composition might also affect the sensor signal. Taken together, reliable determination of Rmax for the ATP sensor in the cell of interest is a difficult task; therefore, one advantage of the dual nanosensor approach is to avoid the need of estimating Rmax. Reevaluation of our imaging data for the AT sensor by the single point calibration method revealed mean [ATP]0 = 7 mM (Figure 5B), i.e., higher as calculated by the dual nanosensor approach as well as higher than previously reported for glial cells in culture (1.4 mM; Ainscow et al., 2002), suggesting that the Rmax value obtained for the purified protein (2.3 × Rmin; Imamura et al., 2009) does not reflect Rmax in cells. Taken together, compared to the dual nanosensor approach, the single point calibration method (Bittner et al., 2010; Mollajew et al., 2013; San Martín et al., 2013; Takaine, 2019; Arce-Molina et al., 2020) provides direct single cell information on the metabolite concentration with only a single nanosensor, but requires an additional estimate of Rmax (Table 1).
Ideally, nanosensors are calibrated within the cell of interest at the subcellular location of interest by applying the metabolite of interest at (numerous) different defined concentrations without interfering with the cellular environment (full calibration; Table 1). Unfortunately, at present this is feasible only in a very limited set of experimental systems. First, controlling the metabolite concentration within the cell requires good accessibility to the cell of interest which is limited in more complex systems like in axons of a highly myelinated nerve or in the brain in vivo. In addition, access of the metabolite to the inside of the cell requires either endogenous transporters or permeabilization. Such an approach has been used, e.g., for calibration of glucose sensors (Bittner et al., 2010), the lactate sensor Laconic (San Martín et al., 2013), or Peredox reporting the NADH/NAD+ ratio (Hung et al., 2011; Hung and Yellen, 2014; Köhler et al., 2018). These studies took advantage of the inherent permeability of the cells to either glucose, or lactate and pyruvate due to the expression of appropriate transporters. In contrast, cell membranes are not permeable for ATP and a plethora of reactions consume ATP within the cell. Nevertheless, also ATP sensors have recently been calibrated in organotypic brain slice cultures using permeabilization of cell membranes (Gerkau et al., 2019; Lerchundi et al., 2020). On the other hand, full calibration of nanosensors in readily accessible systems like purified proteins relies on the assumption that these calibration curves are valid also in cellular and/or more complex systems, but such approaches have successfully been used for obtaining quantitative information in various settings (e.g., San Martín et al., 2013; Fernández-Moncada and Barros, 2014; Mongeon et al., 2016). Furthermore, full calibration of metabolic nanosensors in combination with fluorescence life time imaging (FLIM) allows quantifying metabolite concentrations as, e.g., shown for Peredox, a sensor for the NADH/NAD+-redox ratio, or the glucose sensor SweetieTS (Mongeon et al., 2016; Díaz-García et al., 2017, 2019).
In summary, the dual nanosensor approach is a novel method for quantifying [ATP] from fluorescence data with its own advantages and limitations when compared to other methods (Table 1). Nevertheless, for certain applications like, e.g., complex and difficult to access tissues, it will provide an alternative/additional way for obtaining quantitative data.
ATP in Astrocytes
The dual nanosensor approach was employed to determine the basal concentration of ATP in the cytosol of astrocytes both in primary cultures and acutely isolated brain slices. [ATP]0 was determined at around 1.5 mM for cultured cells (Figure 2) as well as between 0.7 mM and 1.3 mM for cortical astrocytes in brain slices (Figure 6). These values are well within the range of [ATP] reported for glial cells in culture (1.4 mM; Ainscow et al., 2002) as well as other cells like, e.g., neurons (1 mM to 4 mM; Fukuda et al., 1983; Ainscow et al., 2002; Mollajew et al., 2013; Rangaraju et al., 2014; Toloe et al., 2014; Pathak et al., 2015). However, the values are lower than data obtained from biochemical assays (3–7.5 mM; Schousboe et al., 1975; Silver and Erecinska, 1997; 10 mM calculated from ATP content: 40 nmol/mg protein, Winkler et al., 2017; and cytosolic volume of cultured astrocytes: 4.1 μl/mg protein, Dringen and Hamprecht, 1998). This difference is most likely due to the fact that nanosensors measure the concentration of free cytosolic ATP, while biochemical assays measure the total amount of ATP within a cell including organelles as well as ATP bound to proteins.
Based on the dual nanosensor approach, changes of [ATP] induced by the three different incubation conditions were calculated. Importantly, the resulting d[ATP] did not differ between the different methods of calculation (Figure 4). Furthermore, the values obtained using the calculation from each paired experiment, but also from the pooled data of all experiments yielded very similar results suggesting that this approach is robust against these experimental variables. In cultured cortical astrocytes, glutamate application induced a d[ATP] of −0.16 mM, amounting to about 11% of [ATP]0 (Figure 4), consistent with previous reports showing a (non-quantified) decrease in [ATP] (Magistretti and Chatton, 2005; Langer et al., 2017; Winkler et al., 2017). Uptake of glutamate released from synapses during neurotransmission is a major task for gray matter astrocytes (van den Berg et al., 1978; Bak et al., 2006) and the associated decrease in [ATP] has been implicated in the stimulation of astrocytic metabolism to support neighboring neurons (astrocyte-neuron-lactate shuttle hypothesis; Pellerin and Magistretti, 1994; Voutsinos-Porche et al., 2003).
Another sign of neuronal activity relevant for regulation of astrocytic metabolism is an increase of the concentration of extracellular K+ ([K+]e; Rash, 2010; Bittner et al., 2011; Ruminot et al., 2019). K+ is released from all neurons during repolarization and is, therefore, not restricted to glutamatergic neurons (Rash, 2010; MacVicar and Choi, 2017). An increase in [K+]e induces glycogenolysis and activates astrocytic metabolism (Hof et al., 1988; Ruminot et al., 2011; Choi et al., 2012; MacVicar and Choi, 2017; Fernandez-Moncada et al., 2018; Köhler et al., 2018). Previously, an increase in the ATY signal induced by increasing [K+]e from 3 mM to 8 mM or 12 mM was reported for astrocytes in culture and acutely isolated brain slices (Fernandez-Moncada et al., 2018; Lerchundi et al., 2019). Using the dual nanosensor approach and increasing [K+]e from 5.4 mM to 12 mM, d[ATP] was quantified here as 0.07 mM or about 5% of [ATP]0 in cultured cortical astrocytes (Figure 4). The K+ induced increase of [ATP] is likely limited by the availability of free ADP within the cell and, therefore, the rather small increase in [ATP] might underestimate the level of activation of metabolism.
Finally, when blocking mitochondrial ATP production using azide, a decrease of [ATP] of about −0.5 mM (i.e., −32% of [ATP]0; Figure 4) with an initial rate of d[ATP] of −0.23 mM/min (Figure 5C) was observed, consistent with our previous biochemical measurements on the same culture preparations (Winkler et al., 2017). Astrocytes express all enzymes of glycolysis at rather high levels, but are also equipped with the enzymes necessary for fully oxidizing pyruvate and generating ATP in mitochondria (Lovatt et al., 2007). Nevertheless, astrocytes tolerate inhibition of mitochondrial ATP production well by upregulating glycolysis resulting in an increased NADH/NAD+-redox ratio and increased lactate production (Dringen et al., 1993; Bittner et al., 2010; Wilhelm and Hirrlinger, 2011; San Martín et al., 2013; Supplie et al., 2017; Westhaus et al., 2017), consistent with the surprising finding that mice lacking functional mitochondria in astrocytes survive for more than a year without any phenotype (Supplie et al., 2017). However, because numerous mechanisms can contribute to an increase of the glycolytic rate including, e.g., upregulation of glucose transport, upregulation of glycolytic enzymes or activation by allosteric regulators like, e.g., fructose-2,6-bisphosphate, the precise mechanism remains to be elucidated.
In summary, the dual nanosensor approach allows estimating the basal concentration of a metabolite of interest (here ATP) based on the signal changes of two nanosensors with different equilibrium constants. It adds an option to the toolbox for quantifying changes in cellular metabolite concentrations from fluorescence changes, which is particularly useful if determination of Rmax is difficult or impeded since the dual sensor approach is independent of this parameter. Finally, this method is not only applicable to metabolic nanosensors, but also for other sensors reporting, e.g., pH or the concentration of ions or second messengers (San Martin et al., 2014; Zhang et al., 2018; Bischof et al., 2019; Depaoli et al., 2019).
Data Availability Statement
All datasets presented in this study are included in the article/Supplementary Material.
Ethics Statement
The animal study was reviewed and approved by Animal Welfare Office of the Faculty of Medicine, University of Leipzig and Landesdirektion Sachsen.
Author Contributions
JH conceptualized and designed the study. SK, PF, and UW acquired data. SK, PF, HS, JH, and UW analyzed and interpreted the data. HS performed analysis using Mathematica. JH and UW supervised the project. JH acquired funding. SK, JH, and UW wrote the manuscript. All authors contributed to manuscript revision, read, and approved the submitted version.
Funding
This work was supported by grants from the Deutsche Forschungsgemeinschaft (DFG; HI1414/6-1, HI1414/7-1). The funding sources were not involved in study design, data collection and interpretation, or the decision to submit the work for publication.
Conflict of Interest
The authors declare that the research was conducted in the absence of any commercial or financial relationships that could be construed as a potential conflict of interest.
Acknowledgments
JH would like to thank Klaus-Armin Nave, Göttingen, for longstanding collaboration and ongoing support. We thank Eva Kendzia, Christopher Wolff and Marit Sicker for help with cell culture, Grit Marx for help with mouse management, the Medizinisch-Experimentelles Zentrum of the Medical Faculty, University of Leipzig for excellent mouse husbandry as well as the mechanics workshop of the Max-Planck-Institute for Experimental Medicine for developing the silicon devices and flow-chambers used for cell culture. We acknowledge support from the German Research Foundation (DFG) and Universität Leipzig within the program of Open Access Publishing.
Supplementary Material
The Supplementary Material for this article can be found online at: https://www.frontiersin.org/articles/10.3389/fncel.2020.565921/full#supplementary-material
References
Ainscow, E. K., Mirshamsi, S., Tang, T., Ashford, M. L. J., and Rutter, G. A. (2002). Dynamic imaging of free cytosolic ATP concentration during fuel sensing by rat hypothalamic neurones: evidence for ATP-independent control of ATP-sensitive K+ channels. J. Physiol. 544, 429–445. doi: 10.1113/jphysiol.2002.022434
Arai, S., Kriszt, R., Harada, K., Looi, L.-S., Matsuda, S., Wongso, D., et al. (2018). RGB-Color intensiometric indicators to visualize spatiotemporal dynamics of ATP in single cells. Angew. Chem. 57, 10873–10878. doi: 10.1002/anie.201804304
Arce-Molina, R., Cortés-Molina, F., Sandoval, P. Y., Galaz, A., Alegría, K., Schirmeier, S., et al. (2020). A highly responsive pyruvate sensor reveals pathway-regulatory role of the mitochondrial pyruvate carrier MPC. eLife 9:e53917. doi: 10.7554/eLife.53917
Baeza-Lehnert, F., Saab, A. S., Gutierrez, R., Larenas, V., Diaz, E., Horn, M., et al. (2019). Non-canonical control of neuronal energy status by the Na+ Pump. Cell Metab. 29, 668.e4–680.e4. doi: 10.1016/j.cmet.2018.11.005
Bak, L. K., Schousboe, A., and Waagepetersen, H. S. (2006). The glutamate/GABA-glutamine cycle: aspects of transport, neurotransmitter homeostasis and ammonia transfer. J. Neurochem. 98, 641–653. doi: 10.1111/j.1471-4159.2006.03913.x
Barros, L. F., Bolaños, J. P., Bonvento, G., Bouzier-Sore, A.-K., Brown, A., Hirrlinger, J., et al. (2018a). Current technical approaches to brain energy metabolism. Glia 66, 1138–1159. doi: 10.1002/glia.23248
Barros, L. F., Brown, A., and Swanson, R. A. (2018b). Glia in brain energy metabolism: a perspective. Glia 66, 1134–1137. doi: 10.1002/glia.23316
Barros, L. F., and Deitmer, J. W. (2010). Glucose and lactate supply to the synapse. Brain Res. Rev. 63, 149–159. doi: 10.1016/j.brainresrev.2009.10.002
Batiuk, M. Y., Martirosyan, A., Wahis, J., Vin, F., de Marneffe, C., and Kusserow, C. (2020). Identification of region-specific astrocyte subtypes at single cell resolution. Nat. Commun. 11:1220. doi: 10.1038/s41467-019-14198-8
Bayraktar, O. A., Fuentealba, L. C., Alvarez-Buylla, A., and Rowitch, D. H. (2015). Astrocyte development and heterogeneity. Cold Spring Harb. Perspect. Biol. 7:a020362. doi: 10.1101/cshperspect.a020362
Berg, J., Hung, Y. P., and Yellen, G. (2009). A genetically encoded fluorescent reporter of ATP:ADP ratio. Nat. Methods 6, 161–166. doi: 10.1038/nmeth.1288
Bermejo, C., Haerizadeh, F., Takanaga, H., Chermak, D., and Frommer, W. B. (2010). Dynamic analysis of cytosolic glucose and ATP levels in yeast using optical sensors. Biochem. J. 432, 399–406. doi: 10.1042/BJ20100946
Bischof, H., Burgstaller, S., Waldeck-Weiermair, M., Rauter, T., Schinagl, M., Ramadani-Muja, J., et al. (2019). Live-cell imaging of physiologically relevant metal ions using genetically encoded FRET-based probes. Cells 8:492. doi: 10.3390/cells8050492
Bittner, C. X., Loaiza, A., Ruminot, I., Larenas, V., Sotelo-Hitschfeld, T., Gutiérrez, R., et al. (2010). High resolution measurement of the glycolytic rate. Front. Neuroenerget. 2:26. doi: 10.3389/fnene.2010.00026
Bittner, C. X., Valdebenito, R., Ruminot, I., Loaiza, A., Larenas, V., Sotelo-Hitschfeld, T., et al. (2011). Fast and reversible stimulation of astrocytic glycolysis by K+ and a delayed and persistent effect of glutamate. J. Neurosci. 31, 4709–4713. doi: 10.1523/JNEUROSCI.5311-10.2011
Bushong, E. A., Martone, M. E., Jones, Y. Z., and Ellisman, M. H. (2002). Protoplasmic astrocytes in CA1 stratum radiatum occupy separate anatomical domains. J. Neurosci. 22, 183–192. doi: 10.1523/jneurosci.22-01-00183.2002
Choi, H. B., Gordon, G. R. J., Zhou, N., Tai, C., Rungta, R. L., Martinez, J., et al. (2012). Metabolic communication between astrocytes and neurons via bicarbonate-responsive soluble adenylyl cyclase. Neuron 75, 1094–1104. doi: 10.1016/j.neuron.2012.08.032
Depaoli, M. R., Bischof, H., Eroglu, E., Burgstaller, S., Ramadani-Muja, J., Rauter, T., et al. (2019). Live cell imaging of signaling and metabolic activities. Pharmacol. Therapeut. 202, 98–119. doi: 10.1016/j.pharmthera.2019.06.003
Deuschle, K., Chaudhuri, B., Okumoto, S., Lager, I., Lalonde, S., and Frommer, W. B. (2006). Rapid metabolism of glucose detected with FRET glucose nanosensors in epidermal cells and intact roots of Arabidopsis RNA-silencing mutants. Plant Cell 18, 2314–2325. doi: 10.1105/tpc.106.044073
Díaz-García, C. M., Lahmann, C., Martínez-François, J. R., Li, B., Koveal, D., Nathwani, N., et al. (2019). Quantitative in vivo imaging of neuronal glucose concentrations with a genetically encoded fluorescence lifetime sensor. J. Neurosci. Res. 97, 946–960. doi: 10.1002/jnr.24433
Díaz-García, C. M., Mongeon, R., Lahmann, C., Koveal, D., Zucker, H., and Yellen, G. (2017). Neuronal stimulation triggers neuronal glycolysis and not lactate uptake. Cell Metab. 26, 361–374. doi: 10.1016/j.cmet.2017.06.021
Díaz-García, C. M., and Yellen, G. (2019). Neurons rely on glucose rather than astrocytic lactate during stimulation. J. Neurosci. Res. 97, 883–889. doi: 10.1002/jnr.24374
Dringen, R., Gebhardt, R., and Hamprecht, B. (1993). Glycogen in astrocytes: possible function as lactate supply for neighboring cells. Brain Res. 623, 208–214. doi: 10.1016/0006-8993(93)91429-V
Dringen, R., and Hamprecht, B. (1998). Glutathione restoration as indicator for cellular metabolism of astroglial cells. Dev. Neurosci. 20, 401–407. doi: 10.1159/000017337
Farmer, W. T., and Murai, K. (2017). Resolving astrocyte heterogeneity in the CNS. Front. Cell Neurosci. 11:300. doi: 10.3389/fncel.2017.00300
Fehr, M., Lalonde, S., Lager, I., Wolff, M. W., and Frommer, W. B. (2003). In vivo imaging of the dynamics of glucose uptake in the cytosol of COS-7 cells by fluorescent nanosensors. J. Biol. Chem. 278, 19127–19133. doi: 10.1074/jbc.M301333200
Fernández-Moncada, I., and Barros, L. F. (2014). Non-preferential fuelling of the Na+/K+-ATPase pump. Biochem. J. 460, 353–361. doi: 10.1042/BJ20140003
Fernandez-Moncada, I., Ruminot, I., Robles-Maldonado, D., Alegria, K., Deitmer, J. W., and Barros, L. F. (2018). Neuronal control of astrocytic respiration through a variant of the Crabtree effect. Proc. Natl. Acad. Sci. U. S. A. 115, 1623–1628. doi: 10.1073/pnas.1716469115
Fukuda, J., Fujita, Y., and Ohsawa, K. (1983). ATP content in isolated mammalian nerve cells assayed by a modified luciferin-luciferase method. J. Neurosci. Methods 8, 295–302. doi: 10.1016/0165-0270(83)90042-0
Gerkau, N. J., Lerchundi, R., Nelson, J. S. E., Lantermann, M., Meyer, J., Hirrlinger, J., et al. (2019). Relation between activity-induced intracellular sodium transients and ATP dynamics in mouse hippocampal neurons. J. Physiol. 597, 5687–5705. doi: 10.1113/JP278658
Grosche, J., Matyash, V., Moller, T., Verkhratsky, A., Reichenbach, A., and Kettenmann, H. (1999). Microdomains for neuron-glia interaction: parallel fiber signaling to Bergmann glial cells. Nat. Neurosci. 2, 139–143. doi: 10.1038/5692
Guzmán, M., and Blázquez, C. (2001). Is there an astrocyte–neuron ketone body shuttle? Trends Endocrinol. Metab. 12, 169–173. doi: 10.1016/S1043-2760(00)00370-2
Hires, S. A., Tian, L., and Looger, L. L. (2008). Reporting neural activity with genetically encoded calcium indicators. Brain Cell Biol. 36, 69–86. doi: 10.1007/s11068-008-9029-4
Hirrlinger, J., and Nave, K. A. (2014). Adapting brain metabolism to myelination and long-range signal transduction. Glia 62, 1749–1761. doi: 10.1002/glia.22737
Hof, P. R., Pascale, E., and Magistretti, P. J. (1988). K+ at concentrations reached in the extracellular space during neuronal activity promotes a Ca2 +-dependent glycogen hydrolysis in mouse cerebral cortex. J. Neurosci. 8, 1922–1928. doi: 10.1523/JNEUROSCI.08-06-01922.1988
Hung, Y. P., Albeck, J. G., Tantama, M., and Yellen, G. (2011). Imaging cytosolic NADH-NAD+ redox state with a genetically encoded fluorescent biosensor. Cell Metab. 14, 545–554. doi: 10.1016/j.cmet.2011.08.012
Hung, Y. P., and Yellen, G. (2014). Live-cell imaging of cytosolic NADH-NAD+ redox state using a genetically encoded fluorescent biosensor. Methods Mol. Biol. 1071, 83–95. doi: 10.1007/978-1-62703-622-1_7
Imamura, H., Nhat, K. P., Togawa, H., Saito, K., Iino, R., Kato-Yamada, Y., et al. (2009). Visualization of ATP levels inside single living cells with fluorescence resonance energy transfer-based genetically encoded indicators. Proc. Natl. Acad. Sci. U.S.A. 106, 15651–15656.
Kioka, H., Kato, H., Fujita, T., Asano, Y., Shintani, Y., Yamazaki, S., et al. (2020). In vivo real-time ATP imaging in zebrafish hearts reveals G0s2 induces ischemic tolerance. FASEB J. 34, 2041–2054. doi: 10.1096/fj.201901686R
Köhler, S., Winkler, U., Sicker, M., and Hirrlinger, J. (2018). NBCe1 mediates the regulation of the NADH/NAD+ redox state in cortical astrocytes by neuronal signals. Glia 66, 2233–2245. doi: 10.1002/glia.23504
Köhler, S., Winkler, U., and Hirrlinger, J. (2019). Heterogeneity of astrocytes in grey and white matter. Neurochem. Res. doi: 10.1007/s11064-019-02926-x [Epub ahead of print].
Lange, S. C., Winkler, U., Andresen, L., Byhro, M., Waagepetersen, H. S., Hirrlinger, J., et al. (2015). Dynamic changes in cytosolic ATP levels in cultured glutamatergic neurons during NMDA-induced synaptic activity supported by glucose or lactate. Neurochem. Res. 40, 2517–2526. doi: 10.1007/s11064-015-1651-9
Langer, J., Gerkau, N. J., Derouiche, A., Kleinhans, C., Moshrefi-Ravasdjani, B., Fredrich, M., et al. (2017). Rapid sodium signaling couples glutamate uptake to breakdown of ATP in perivascular astrocyte endfeet. Glia 65, 293–308. doi: 10.1002/glia.23092
Le Douce, J., Maugard, M., Veran, J., Matos, M., Jégo, P., Vigneron, P.-A., et al. (2020). Impairment of glycolysis-derived l-serine production in astrocytes contributes to cognitive deficits in Alzheimer’s Disease. Cell Metab. 31, 503.e8–517.e8. doi: 10.1016/j.cmet.2020.02.004
Lee, Y., Messing, A., Su, M., and Brenner, M. (2008). GFAP promoter elements required for region-specific and astrocyte-specific expression. Glia 56, 481–493. doi: 10.1002/glia.20622
Lerchundi, R., Huang, N., and Rose, C. R. (2020). Quantitative imaging of changes in astrocytic and neuronal ATP using two different variants of ATeam. Front. Cell. Neurosci. 14:80. doi: 10.3389/fncel.2020.00080
Lerchundi, R., Kafitz, K. W., Winkler, U., Färfers, M., Hirrlinger, J., and Rose, C. R. (2019). FRET-based imaging of intracellular ATP in organotypic brain slices. J. Neurosci. Res. 97, 933–945. doi: 10.1002/jnr.24361
Lovatt, D., Sonnewald, U., Waagepetersen, H. S., Schousboe, A., He, W., Lin, J. H., et al. (2007). The transcriptome and metabolic gene signature of protoplasmic astrocytes in the adult murine cortex. J. Neurosci. 27, 12255–12266. doi: 10.1523/jneurosci.3404-07.2007
Mächler, P., Wyss, M. T., Elsayed, M., Stobart, J., Gutierrez, R., von Faber-Castell, A., et al. (2016). In vivo evidence for a lactate gradient from astrocytes to neurons. Cell Metab. 23, 94–102. doi: 10.1016/j.cmet.2015.10.010
MacVicar, B. A., and Choi, H. B. (2017). Astrocytes provide metabolic support for neuronal synaptic function in response to extracellular K+. Neurochem. Res. 42, 2588–2594. doi: 10.1007/s11064-017-2315-8
Magistretti, P. J., and Chatton, J. Y. (2005). Relationship between L-glutamate-regulated intracellular Na+ dynamics and ATP hydrolysis in astrocytes. J. Neural. Transm. 112, 77–85. doi: 10.1007/s00702-004-0171-6
Masia, R., McCarty, W. J., Lahmann, C., Luther, J., Chung, R. T., Yarmush, M. L., et al. (2018). Live cell imaging of cytosolic NADH/NAD+ ratio in hepatocytes and liver slices. Am. J. Physiol. Gastrointest. Liver Physiol. 314, G97–G108. doi: 10.1152/ajpgi.00093.2017
Matyash, V., and Kettenmann, H. (2010). Heterogeneity in astrocyte morphology and physiology. Brain Res. Rev. 63, 2–10. doi: 10.1016/j.brainresrev.2009.12.001
Miller, S. J. (2018). Astrocyte heterogeneity in the adult central nervous system. Front. Cell Neurosci. 12:401. doi: 10.3389/fncel.2018.00401
Mollajew, R., Toloe, J., and Mironov, S. L. (2013). Single KATP channel opening in response to stimulation of AMPA/kainate receptors is mediated by Na+ accumulation and submembrane ATP and ADP changes. J. Physiol. 591, 2593–2609. doi: 10.1113/jphysiol.2012.248369
Mongeon, R., Venkatachalam, V., and Yellen, G. (2016). Cytosolic NADH-NAD+ Redox visualized in brain slices by two-photon fluorescence lifetime biosensor imaging. Antioxid. Redox Signal. 25, 553–563. doi: 10.1089/ars.2015.6593
Morel, L., Men, Y., Chiang, M. S. R., Tian, Y., Jin, S., Yelick, J., et al. (2018). Intracortical astrocyte subpopulations defined by astrocyte reporter Mice in the adult brain. Glia 67, 171–181. doi: 10.1002/glia.23545
Nave, K. A. (2010a). Myelination and support of axonal integrity by glia. Nature 468, 244–252. doi: 10.1038/nature09614
Nave, K.-A. (2010b). Myelination and the trophic support of long axons. Nat. Rev. Neurosci. 11, 275–283. doi: 10.1038/nrn2797
Nguyen, P. T. M., Ishiwata-Kimata, Y., and Kimata, Y. (2019). Monitoring ADP/ATP ratio in yeast cells using the fluorescent-protein reporter PercevalHR. Biosci. Biotechnol. Biochem. 83, 824–828. doi: 10.1080/09168451.2019.1574204
Pathak, D., Shields, L. Y., Mendelsohn, B. A., Haddad, D., Lin, W., Gerencser, A. A., et al. (2015). The role of mitochondrially derived ATP in synaptic vesicle recycling. J. Biol. Chem. 290, 22325–22336. doi: 10.1074/jbc.M115.656405
Pätz, C., Brachtendorf, S., and Eilers, J. (2018). The transgenic mouse line Igsf9-eGFP allows targeted stimulation of inferior olive efferents. J. Neurosci. Methods 296, 84–92. doi: 10.1016/j.jneumeth.2017.12.024
Pellerin, L., and Magistretti, P. J. (1994). Glutamate uptake into astrocytes stimulates aerobic glycolysis: a mechanism coupling neuronal activity to glucose utilization. Proc. Natd. Acad. Sci. U.S.A. 91, 10625–10629. doi: 10.1073/pnas.91.22.10625
Pérez Koldenkova, V., and Nagai, T. (2013). Genetically encoded Ca2 + indicators: properties and evaluation. Biochim. Biophys. Acta 1833, 1787–1797. doi: 10.1016/j.bbamcr.2013.01.011
Pomorski, A., Kochańczyk, T., Miłoch, A., and Krêżel, A. (2013). Method for accurate determination of dissociation constants of optical ratiometric systems: chemical probes, genetically encoded sensors, and interacting molecules. Anal. Chem. 85, 11479–11486. doi: 10.1021/ac402637h
Rangaraju, V., Calloway, N., and Ryan, T. A. (2014). Activity-driven local ATP synthesis is required for synaptic function. Cell 156, 825–835. doi: 10.1016/j.cell.2013.12.042
Rash, J. E. (2010). Molecular disruptions of the panglial syncytium block potassium siphoning and axonal saltatory conduction: pertinence to neuromyelitis optica and other demyelinating diseases of the central nervous system. Neuroscience 168, 982–1008. doi: 10.1016/j.neuroscience.2009.10.028
Requardt, R. P., Hirrlinger, P. G., Wilhelm, F., Winkler, U., Besser, S., and Hirrlinger, J. (2012). Ca2 + signals of astrocytes are modulated by the NAD+/NADH redox state. J. Neurochem. 120, 1014–1025. doi: 10.1111/j.1471-4159.2012.07645.x
Requardt, R. P., Wilhelm, F., Rillich, J., Winkler, U., and Hirrlinger, J. (2010). The biphasic NAD(P)H fluorescence response of astrocytes to dopamine reflects the metabolic actions of oxidative phosphorylation and glycolysis. J. Neurochem. 115, 483–492. doi: 10.1111/j.1471-4159.2010.06940.x
Ruminot, I., Gutiérrez, R., Peña-Münzenmayer, G., Añazco, C., Sotelo-Hitschfeld, T., Lerchundi, R., et al. (2011). NBCe1 mediates the acute stimulation of astrocytic glycolysis by extracellular K+. J. Neurosci. 31, 14264–14271. doi: 10.1523/JNEUROSCI.2310-11.2011
Ruminot, I., Schmälzle, J., Leyton, B., Barros, L. F., and Deitmer, J. W. (2019). Tight coupling of astrocyte energy metabolism to synaptic activity revealed by genetically encoded FRET nanosensors in hippocampal tissue. J. Cereb. Blood Flow Metabol. 39, 513–523. doi: 10.1177/0271678X17737012
San Martín, A., Ceballo, S., Baeza-Lehnert, F., Lerchundi, R., Valdebenito, R., Contreras-Baeza, Y., et al. (2014). Imaging mitochondrial flux in single cells with a FRET sensor for pyruvate. PLoS One 9:e85780. doi: 10.1371/journal.pone.0085780
San Martín, A., Ceballo, S., Ruminot, I., Lerchundi, R., Frommer, W. B., and Barros, L. F. (2013). A genetically encoded FRET lactate sensor and its use to detect the Warburg effect in single cancer cells. PLoS One 8:e0057712. doi: 10.1371/journal.pone.0057712
San Martin, A., Sotelo-Hitschfeld, T., Lerchundi, R., Fernandez-Moncada, I., Ceballo, S., Valdebenito, R., et al. (2014). Single-cell imaging tools for brain energy metabolism: a review. Neurophotonics 1:11004. doi: 10.1117/1.NPh.1.1.011004
Schindelin, J., Arganda-Carreras, I., Frise, E., Kaynig, V., Longair, M., Pietzsch, T., et al. (2012). Fiji: an open-source platform for biological-image analysis. Nat. Methods 9, 676–682. doi: 10.1038/nmeth.2019
Schousboe, A., Fosmark, H., and Hertz, L. (1975). High content of glutamate and of ATP in astrocytes cultured from rat brain hemispheres: effect of serum withdrawal and of cyclic AMP. J. Neurochem. 25, 909–911. doi: 10.1111/j.1471-4159.1975.tb04429.x
Shulman, Y., Stavsky, A., Fedorova, T., Mikulincer, D., Atias, M., Radinsky, I., et al. (2015). ATP binding to synaspsin IIa regulates usage and clustering of vesicles in terminals of hippocampal neurons. J. Neurosci. 35, 985–998. doi: 10.1523/JNEUROSCI.0944-14.2015
Silver, I. A., and Erecinska, M. (1997). Energetic demands of the Na+/K+ ATPase in mammalian astrocytes. Glia 21, 35–45. doi: 10.1002/(SICI)1098-1136(199709)21:1<35:AID-GLIA4<3.0.CO;2-0
Somjen, G. G. (1988). Nervenkitt: notes on the history of the concept of neuroglia. Glia 1, 2–9. doi: 10.1002/glia.440010103
Sotelo-Hitschfeld, T., Fernandez-Moncada, I., and Barros, L. F. (2012). Acute feedback control of astrocytic glycolysis by lactate. Glia 60, 674–680. doi: 10.1002/glia.22304
Stobart, J. L., Ferrari, K. D., Barrett, M. J. P., Stobart, M. J., Looser, Z. J., Saab, A. S., et al. (2018). Long-term in vivo calcium imaging of astrocytes reveals distinct cellular compartment responses to sensory stimulation. Cereb. Cortex 28, 184–198. doi: 10.1093/cercor/bhw366
Supplie, L. M., Düking, T., Campbell, G., Diaz, F., Moraes, C. T., Götz, M., et al. (2017). Respiration-deficient astrocytes survive as glycolytic cells in vivo. J. Neurosci. 37, 4231–4242. doi: 10.1523/JNEUROSCI.0756-16.2017
Takaine, M. (2019). QUEEN-based spatiotemporal ATP imaging in budding and fission yeast. Bio Protoc. 9:e3320. doi: 10.21769/BioProtoc.3320
Takaine, M., Ueno, M., Kitamura, K., Imamura, H., and Yoshida, S. (2019). Reliable imaging of ATP in living budding and fission yeast. J. Cell Sci. 132:jcs230649. doi: 10.1242/jcs.230649
Toloe, J., Mollajew, R., Kugler, S., and Mironov, S. L. (2014). Metabolic differences in hippocampal ‘Rett’ neurons revealed by ATP imaging. Mol. Cell Neurosci. 59, 47–56. doi: 10.1016/j.mcn.2013.12.008
Trevisiol, A., Saab, A. S., Winkler, U., Marx, G., Imamura, H., Mobius, W., et al. (2017). Monitoring ATP dynamics in electrically active white matter tracts. eLife 6:e24241. doi: 10.7554/eLife.24241
Tsuyama, T., Kishikawa, J.-I., Han, Y.-W., Harada, Y., Tsubouchi, A., Noji, H., et al. (2013). In vivo fluorescent adenosine 5’-triphosphate (ATP) imaging of Drosophila melanogaster and Caenorhabditis elegans by using a genetically encoded fluorescent ATP biosensor optimized for low temperatures. Anal. Chem. 85, 7889–7896. doi: 10.1021/ac4015325
van den Berg, C. J., Matheson, D. F., and Nijemanting, W. V. (1978). “Compartimentation of amino acids in brain: the GABA glutamine-glutamate cycle,” in Amino Acids and Chemical Transmitters, ed. F. Fonnum (New York, NY: Plenum Press), 709–723. doi: 10.1007/978-1-4613-4030-0_50
Vicente-Gutierrez, C., Bonora, N., Bobo-Jimenez, V., Jimenez-Blasco, D., Lopez-Fabuel, I., Fernandez, E., et al. (2019). Astrocytic mitochondrial ROS modulate brain metabolism and mouse behaviour. Nat. Metab. 1, 201–211. doi: 10.1038/s42255-018-0031-6
Volkenhoff, A., Hirrlinger, J., Kappel, J. M., Klämbt, C., and Schirmeier, S. (2018). Live imaging using a FRET glucose sensor reveals glucose delivery to all cell types in the Drosophila brain. J. Insect. Physiol. 106, 55–64. doi: 10.1016/j.jinsphys.2017.07.010
Voutsinos-Porche, B., Bonvento, G., Tanaka, K., Steiner, P., Welker, E., Chatton, J. Y., et al. (2003). Glial glutamate transporters mediate a functional metabolic crosstalk between neurons and astrocytes in the mouse developing cortex. Neuron 37, 275–286. doi: 10.1016/s0896-6273(02)01170-4
Waagepetersen, H. S., Sonnewald, U., and Schousboe, A. (2003). Compartmentation of glutamine, glutamate, and GABA metabolism in neurons and astrocytes: functional implications. Neuroscientist 9, 398–403. doi: 10.1177/1073858403254006
Westhaus, A., Blumrich, E. M., and Dringen, R. (2017). The antidiabetic drug metformin stimulates glycolytic lactate production in cultured primary rat astrocytes. Neurochem. Res. 42, 294–305. doi: 10.1007/s11064-015-1733-8
Wilhelm, F., and Hirrlinger, J. (2011). The NAD+/NADH redox state in astrocytes: independent control of the NAD+ and NADH content. J. Neurosci. Res. 89, 1956–1964. doi: 10.1002/jnr.22638
Winkler, U., Seim, P., Enzbrenner, Y., Kohler, S., Sicker, M., and Hirrlinger, J. (2017). Activity-dependent modulation of intracellular ATP in cultured cortical astrocytes. J. Neurosci. Res. 95, 2172–2181. doi: 10.1002/jnr.24020
Yaginuma, H., Kawai, S., Tabata, K. V., Tomiyama, K., Kakizuka, A., Komatsuzaki, T., et al. (2014). Diversity in ATP concentrations in a single bacterial cell population revealed by quantitative single-cell imaging. Sci. Rep. 4:6522. doi: 10.1038/srep06522
Yellen, G., and Mongeon, R. (2015). Quantitative two-photon imaging of fluorescent biosensors. Curr. Opin. Chem. Biol. 27, 24–30. doi: 10.1016/j.cbpa.2015.05.024
Zhang, Y., and Barres, B. A. (2010). Astrocyte heterogeneity: an underappreciated topic in neurobiology. Curr. Opin. Neurobiol. 20, 588–594. doi: 10.1016/j.conb.2010.06.005
Zhang, Z., Chen, W., Zhao, Y., and Yang, Y. (2018). Spatiotemporal imaging of cellular energy metabolism with genetically-encoded fluorescent sensors in brain. Neurosci. Bull. 34, 875–886. doi: 10.1007/s12264-018-0229-3
Zhao, F.-L., Zhang, C., Zhang, C., Tang, Y., and Ye, B.-C. (2016). A genetically encoded biosensor for in vitro and in vivo detection of NADP+. Biosens Bioelectron. 77, 901–906. doi: 10.1016/j.bios.2015.10.063
Zhao, Y., Hu, Q., Cheng, F., Su, N., Wang, A., Zou, Y., et al. (2015). SoNar, a highly responsive NAD+/NADH sensor, allows high-throughput metabolic screening of anti-tumor agents. Cell Metab. 21, 777–789. doi: 10.1016/j.cmet.2015.04.009
Keywords: ATeam, ATP, astrocyte, calibration, genetically encoded sensors for metabolites
Citation: Köhler S, Schmidt H, Fülle P, Hirrlinger J and Winkler U (2020) A Dual Nanosensor Approach to Determine the Cytosolic Concentration of ATP in Astrocytes. Front. Cell. Neurosci. 14:565921. doi: 10.3389/fncel.2020.565921
Received: 26 May 2020; Accepted: 26 August 2020;
Published: 18 September 2020.
Edited by:
Christian Lohr, University of Hamburg, GermanyReviewed by:
Ignacio Fernández-Moncada, INSERM U1215 Neurocentre Magendie, FranceTakeharu Nagai, Osaka University, Japan
Copyright © 2020 Köhler, Schmidt, Fülle, Hirrlinger and Winkler. This is an open-access article distributed under the terms of the Creative Commons Attribution License (CC BY). The use, distribution or reproduction in other forums is permitted, provided the original author(s) and the copyright owner(s) are credited and that the original publication in this journal is cited, in accordance with accepted academic practice. No use, distribution or reproduction is permitted which does not comply with these terms.
*Correspondence: Johannes Hirrlinger, johannes.hirrlinger@medizin.uni-leipzig.de; hirrlinger@em.mpg.de
†ORCID: Johannes Hirrlinger, orcid.org/0000-0002-6327-0089