- Affiliated Jinling Hospital, Medical School of Nanjing University, Nanjing, China
Objective: To investigate the impact of Alzheimer’s disease (AD)-related risk gene (ATP-binding cassette A7-ABCA7 and Clusterin-CLU) on the functional connectivity pattern of default mode network (DMN) in healthy middle-age adults.
Methods: A total of 147 healthy middle-aged volunteers were enrolled in this study. All subjects completed MRI scans, neuropsychological assessments, and AD-related genotyped analysis. All subjects were divided into high, middle and low risk groups according to the score of risk genotypes, which included CLU (rs11136000, rs2279590, rs9331888, and rs9331949) and ABCA7 (rs3764650 and rs4147929). The genetic effects of CLU, ABCA7, and CLU × ABCA7 on DMN functional connectivity pattern were further explored. Moreover, the genetic effect of Apolipoprotein ε4 (APOEε4) was also considered. Finally, correlation analysis was performed between the signals of brain regions with genetic effect and neuropsychological test scores.
Results: Compared with the low-risk group, the high-risk group of CLU showed decreased functional connectivity in posterior cingulate cortex (PCC) and the left middle frontal cortex (P < 0.05, GRF correction). As for the interaction between the CLU and ABCA7, all the subjects were divided into high, middle, and low risk group; the middle-risk group was divided into CLU and ABCA7-dominated middle-risk group. The function connectivity pattern of DMN among the three or four groups were distributed in the bilateral medial prefrontal cortex (MPFC) and bilateral superior frontal gyrus (SFG) (P < 0.05, GRF correction). When APOEε4 carriers were excluded, the CLU-predominant middle-risk group displayed the decreased functional connectivity in MPFC when compared with the low-risk group, while ABCA7-prodominant middle-risk group displayed decreased functional connectivity in cuneus when compared with the high-risk group (all P < 0.05, GRF correction). The z values of left middle frontal cortex were positively correlated with the scores of Serial Dotting Test (SDT) in high-risk group of CLU, while z values of MPFC and cuneus were positively correlated to the scores of Montreal Cognitive Assessment (MoCA) in low-risk group of three or four groups.
Conclusion: The functional connectivity of MPFC-PCC might be modulated by the interaction of CLU and ABCA7. Moreover, APOEε4 might be interacted with ABCA7 and CLU modulation in the middle-aged carriers.
Introduction
Alzheimer’s disease (AD) is a neurodegenerative disease with complex genetic characteristics (Lane et al., 2018). According to the findings of some genetic research, apolipoprotein ε4 (APOEε4) has been considered as the major high risk genes for AD (Rajabli et al., 2018). CLU and ABCA7 are listed as the top high risk genes secondary to APOEε4 (Corneveaux et al., 2010; Nelson et al., 2017) and both have the similar roles in lipid metabolism, immune response and clearance of Aβ aggregation (Harold et al., 2009; Tan et al., 2016). It was found that the CLU and ApoE risk variants had combined effects on both volumetric expansion and lateral ventricle enlargement in the elderly (Yu et al., 2010; Roussotte et al., 2014). ABCA7 had been verified to be an independent predictor of AD (Liao et al., 2014). It is thus indeed important to study the combined effects of CLU and ABCA7 on brain to explore the underlying common pathophysiologic mechanism of AD.
Neuroimaging has been widely applied to investigate the effects of AD-related genes on brain function and structure (Patel et al., 2013; Valkanova and Ebmeier, 2014). The default mode network (DMN) is one of the most vulnerable targets during the development of AD (Greicius et al., 2004). There was significant decline in functional connectivity among core regions of DMN in AD-related genes carriers with normal cognition. Prominent features of functional connectivity within DMN included (1) an overall strong level of interaction between the precuneus/posterior cingulate region and the rest of the DMN; (2) a high degree of interaction between the left and right medial temporal lobes (MTLs) combined with weak interactions between the MTLs and the rest of DMN; (3) strong interactions between the precuneus/posterior cingulate cortex (PCC) and the left inferior parietal lobe as well as between the dorsal and ventral sections of the medial prefrontal cortex (MPFC) (Fransson and Marrelec, 2008; Patel et al., 2013). Ye et al. found that CLU may affect the functional connectivity in the prefrontal cortex of AD patients, and CLU-T allele might be associated with compensatory neurological processes in the alteration of DMN in elderly subjects (Ye et al., 2017). Sinha et al. (2018) found that ABCA7 genetic risk differentially affects intra- MTL functional connectivity between MTL subfields, versus internetwork connectivity of the MTL with the MPFC, in non-demented older African Americans. Liao et al. (2014) found that ABCA7 rs3764650 was significantly associated with AD and the influence of ABCA7 was only evident in individuals without APOEε4 alleles in elderly subjects (Liao et al., 2014). Mcfall et al. (2016) found that the cumulative genetic risk of APOE plus CLU had relation to executive function performance in older adults. Considering the findings of the above studies, we speculated that CLU and ABCA7 may have a early regulation of DMN, and ABCA7 and CLU and their genetic polymorphisms could affect DMN functional connectivity.
Thus, the purpose of this study is to investigate the impact of ABCA7 and CLU on the functional connectivity pattern of DMN in healthy middle-age adults and further explore the possible pathogenic mechanism of AD.
Materials and Methods
Subjects
This study enrolled 147 middle age adults with normal cognition from the local community between May 2015 and January 2017. All subjects were 45–64 years old (Fennemanotestine et al., 2011; Natalya et al., 2013), right-handedness. All the participants underwent MRI scans, neuropsychological tests and genotype analysis. The exclusion criteria were as follow: (1) obvious systematic diseases; (2) history of psychiatric or neurological diseases; (3) previous head trauma history; (4) drug or alcohol abuse; (5) MR imaging contraindications; (6) head motions with translation more than 1.0 mm or rotation more than 1.0°. This study was approved by the local Ethics Committee and all subjects provided written informed consent.
Neuropsychological Assessments
All volunteers completed the following neuropsychological assessments before MR examination. Mini-Mental State Examination (MMSE) and Montreal Cognitive Assessment (MoCA) tests were used to measured global cognitive states, and MMSE > 26 score and MoCA > 26 score are identified as having intact cognition (Galea and Woodward, 2005; Nasreddine et al., 2005). Number Connection Test type A (NCT-A) and Digit Symbol Test (DST) were used to assess attention/executive function. Self-rating Anxiety Scale (SAS) and Self-rating Depression Scale (SDS) were used to assess the anxiety and depression state. Other neuropsychological assessments such as Line Tracing Test (LTT) and Serial Dotting Test (SDT) were used to assess executive function, attention or information processing speed (Salmon and Lange, 2001; Sankari et al., 2011).
Genotyping and Grouping
We collected subjects between May 2015 and January 2017, all volunteer, none of the volunteers met the exclusion criteria. All the participants underwent MRI scans, neuropsychological tests. Each qualified volunteer draws 2 ml fasting blood before or after examination. All the samples were collected and stored in the −80°C after ED-TA anticoagulation, and were sent to Shanghai Tianhao Biotechnology company for genomic DNA extraction and SNP testing. All subjects were genotyped for CLU and ABCA7 mutations. SNPs of CLU and ABCA7, including CLUrs11136000, CLUrs2279590, CLUrs9331888, CLUrs9331949, ABCA7rs3764650, and ABCA7rs4147929 were reported to have high correlation with the onset of the late onset AD (Shi et al., 2012; Karch and Goate, 2015). Specifically, CLUrs11136000-T, rs2279590-T, rs9331888-G and rs9331949-C and ABCA7rs3764650-G, rs4147929-A are the risk alleles (Supplementary Table S1). Subsequently, each subject was recorded as 2, 1, or 0 score according to the amount of risk allele (Chouraki et al., 2016). There were 13 subjects with APOE ε4. APOE ε4 (n = 13) has been considered as the main risk gene of AD (Abushakra et al., 2017), and previous studies have shown the altered functional or structural connectivity pattern of DMN in APOEε4 healthy carriers (Filippini et al., 2009; Machulda et al., 2011). Firstly, we excluded the subjects with APOEε4. Based on the distribution of scores of risk alleles of CLU and ABCA7 without APOE ε4 (n = 13), the subjects were divided into the CLU low-risk group (0, 1, and 2 scores, n = 93) and CLU high risk group (3, 4, 5, and 6 scores, n = 41). Similarly, ABCA7 (0 and 1 scores, n = 70) as low-risk group, ABCA7 (2, 3, 4, 5, and 6 scores, n = 64) as high-risk group (Sleegers et al., 2015). Then, in all subjects included APOEε4. In order to explore the interactive effect of CLU and ABCA7, all subjects were further divided into high-risk (GG + GG, GG + GT, GC + GG, and GC + GT, n = 56), middle-risk (GG + TT, GC + TT, CC + GG, and CC + GT, n = 63), and low-risk group (CC + TT, n = 28) according to CLU rs933188 and ABCA7rs3764650. Furthermore, we divided middle-risk group into CLU-dominated (GG + TT and GC + TT, n = 22) and ABCA7-dominated (CC + GG and CC + GT, n = 41). Considering that APOEε4 might have an impact on the subjects. We further analyzed the interactive effect in APOEε4 non-carriers. Similarly, the genotypes of the APOE, ε2, ε3, and ε4 were determined by testing the SNPs rs429358 and rs7412 (Rajan et al., 2019) (Supplementary Table S2).
MR Imaging Data Acquisition
All subjects were scanned on a 3.0-T MR scanner (TIM Trio, Siemens, Germany) which equipped with the standard 12-channel head coil. Foam paddings were used to minimize head motion. During the MRI scans, all participants were instructed to rest with their eyes closed and avoid thinking anything particular. T2 fluid attenuated inversion-recovery (T2-FLAIR) images were obtained to exclude the silent injury in brain, with its parameters as following: 25 axial slices, slice thickness = 4 mm, slice gap = 1.2 mm, image matrix = 232 × 256, field of view [FOV] = 220 × 220 mm2, repetition time [TR]/echo time [TE] = 9000/93 ms, flip angle = 130°, and inversion time = 2,500 ms. To obtain rs-fMRI data, we used a single-shot, gradient-recalled echo planar imaging sequence aligned along the anterior-posterior commissure to cover the whole brain which lasting 500 s. The parameters were as following: 250 volumes, image matrix = 64 × 64, FOV = 240 × 240 mm2, voxel size = 3.75 × 3.75 × 4 mm3, 30 axial slices, TR/TE = 2,000/40 ms, flip angle = 90°. Besides, a magnetization-prepared rapid gradient-echo sequence was conducted for acquiring the high-resolution, T1-weighted 3D anatomical images (T1W-3D-MPRAGE) with the parameters as follows: TR/TE = 2300/2.98 ms, flip angle = 9°, 176 slices, FOV = 256 × 256 mm2, acquisition matrix = 256 × 256, slice thickness = 1 mm (Wang et al., 2017; Kura et al., 2018).
Data Preprocessing
All the image preprocessing was conducted using the Statistical Parametric Mapping software1 (SPM) based on MATLAB platform. Considering the subjects’ adaptation to the scanning state, first 10 volumes of each fMRI scan were discarded for the signal equilibrium. Then, the remaining 240 time points were processed (Andrewshanna et al., 2010). We excluded the subjects with translation or rotation parameters more than 1.0 mm or 1.0 degree (39 subjects were excluded due to movement). The T1W-3D-MPRAGE structure images were co-registered to the functional MRI data. Segmentation of T1WI was conducted into gray matter, white matter, and cerebrospinal fluid, and T1WI was spatially normalized to the Montreal Neurological Institute space with a voxel size of 3 × 3 × 3 mm3 by using unified segmentation algorithm, and smoothed by convolution with an isotropic Gaussian kernel of 6 mm. Finally, the processed data were detrended and nuisance covariates were regressed as well.
Functional Connectivity Analysis
Default mode network was constructed by the method of PCC-based functional connectivity (Lee and Xue, 2018). PCC was determined by the sphere [radius with 8 mm, centered by MNI coordinates (−5, −49, and 40)] (Fernándezespejo et al., 2010). The average time series based on the seed was extracted, and the positive value of function connectivity of each voxel in the whole brain was calculated and transformed to z values by Fisher transform.
Statistical Analysis
The demographic or neuropsychiatric data were analyzed using the SPSS version 20.0 (SPSS Inc., Chicago, IL, United States). The Kolmogorov-Smirnov test was conducted to assess the normality of quantitative data. Distributed data were expressed as mean ± standard deviation and then assessed by two sample t-test and ANOVA test. Independent sample non-parametric testing was used to analyze the data with non-normal distribution, which were reported as median and inter-quartile range [M (QU − QL)].
ANCOVA and two-sample t-tests were performed to detect the functional connectivity differences of DMN among the groups with different risk alleles. Age, gender, and education were set as covariates. All results were then corrected by the Gaussian random field (GRF) (P < 0.001 at the voxel level and P < 0.05 at the cluster level). z values of brain regions with statistical differences were extracted and correlated with the scores of the neuropsychological tests across all subjects as well as in each group, respectively. Correlation analyses were performed by using Pearson (normal distribution data) or Spearman correlation analysis (non-normal distribution data). P < 0.05 was regarded as significantly different.
Results
Demographical and Neuropsychological Results
This study included 147 healthy middle-aged (with 13 APOEε4 carriers). Mostly of our subjects were female (45/89 of Male/Female without APOE and 49/98 with APOE, average age of female with APOE is 55, and average age of female without APOE is 55). By calculation of the risk genotypes of CLU (rs11136000, rs2279590, rs9331888, and rs9331949) and ABCA7 (rs3764650 and rs4147929), there were no significant differences for age, sex, educational level or neuropsychological tests between high-risk and low-risk groups, respectively, in CLU and ABCA7 (all P > 0.05) (Supplementary Tables S3, S4). Besides, there were no significant differences for age, sex, educational level or neuropsychological tests among the three groups (low-risk group, middle-risk group, and high-risk group) and the four groups (low-risk group, CLU-predominant middle-risk group, ABCA7-prodominant middle-risk group, and high-risk group) (all P > 0.05) (Supplementary Tables S5–S8).
Function Connectivity of DMN Altered by the Risk Degree of CLU and ABCA7
High-risk CLU group showed decreased function connectivity in PCC and the left middle frontal cortex, when compared with the low-risk carriers (P < 0.05 after GRF-corrected) (Figure 1A). Functional connectivity pattern of DMN modulated by the risk of ABCA7 was distributed in the right precuneus, while no significant difference was found after GRF correction (Figure 1B).
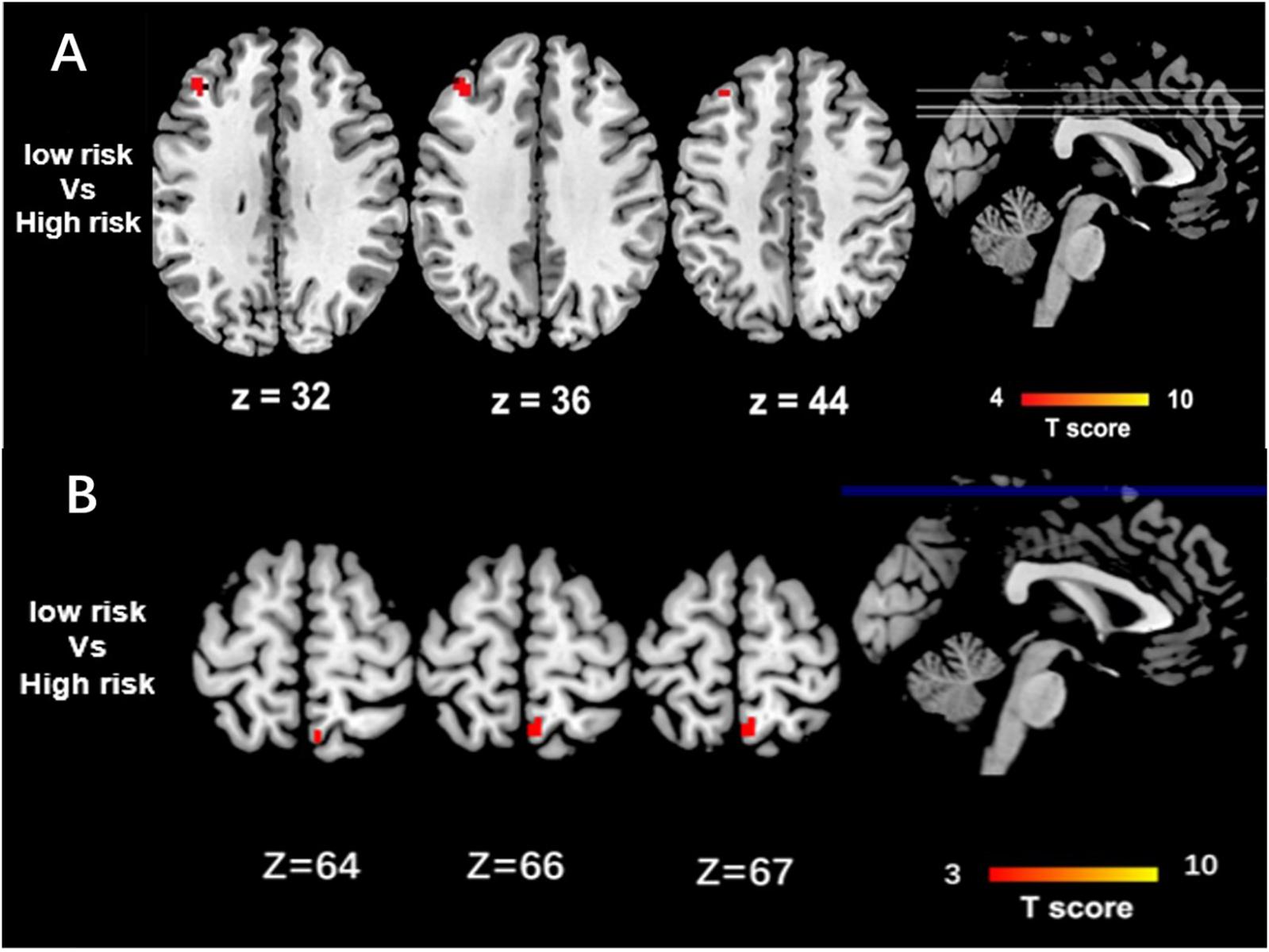
Figure 1. Group Differences of Functional Connectivity in CLU and ABCA7. (A) The two-sample t-test between risk degree of the total risk gene scores in CLU and DMN shows that the major differences between high-risk and low-risk group are located in the left middle frontal cortex (P < 0.05 after GRF correction). Based on the distribution of scores of risk alleles of CLU without APOEε4. High-risk = scored 3, 4, 5, and 6; low-risk = scored 0, 1, and 2. (B) The two-sample t-test between risk degree of the total risk gene scores in ABCA7 and DMN shows that the major differences between high-risk and low-risk group are located in the right precuneus, while there is no significant difference between the two groups (P < 0.05 after GRF correction). Based on the distribution of scores of risk alleles of ABCA7 without APOEε4. High-risk = GG + GG/GG + GT/GC + GG/GC + GT; low-risk = CC + TT.
Function Connectivity of DMN Influenced by the Interaction of CLU and ABCA7
As for the interaction between the CLU rs9331888 and ABCA7 rs376465, function connectivity pattern of DMN among the three groups or the four groups were distributed in the bilateral MPFC and bilateral superior frontal gyrus (SFG). The CLU-predominant middle-risk group displayed the decreased functional connectivity in MPFC when compared with the low risk group (P < 0.05 after GRF-corrected), while ABCA7-prodominant middle-risk group displayed decreased activity in cuneus when compared with the high-risk group (P < 0.05 after GRF-corrected). When APOEε4 carriers were excluded, the ANOVA test result among four groups demonstrated the altered activity in MPFC and cuneus. Specifically, the CLU-predominant middle-risk group displayed the decreased functional connectivity in MPFC when compared with the low-risk group, while ABCA7-prodominant middle-risk group displayed decreased activity in cuneus when compared with the high-risk group (P < 0.05 after GRF-corrected) (Table 1 and Figures 2, 3).
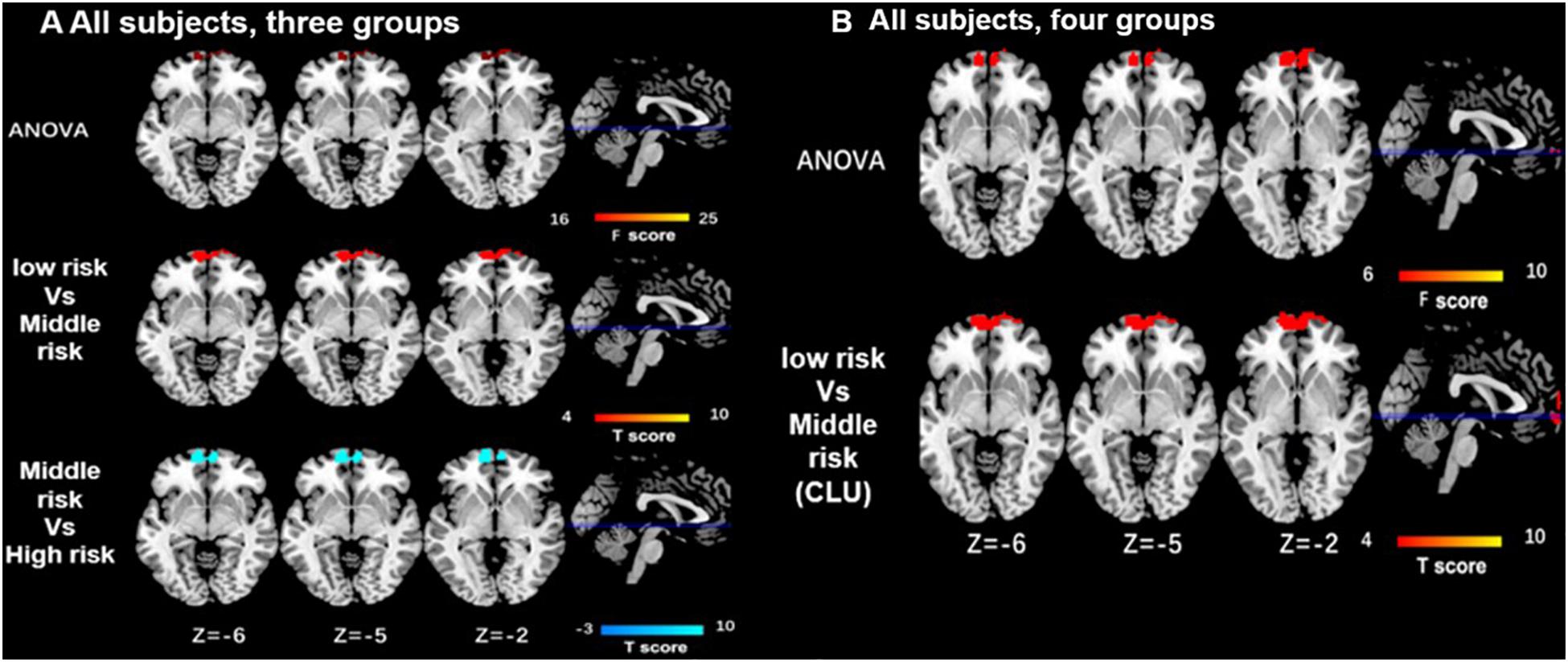
Figure 2. Differences of functional connectivity influenced by the interaction of CLU and ABCA7 in all subjects. (A) In all subjects, the major differences of function connectivity of DMN among the three groups are distributed in the bilateral medial prefrontal cortex (MPFC) and bilateral superior frontal gyri (SFG). When compared with the low- and high-risk group, middle-risk group displays the lower functional connectivity in MPFC/SFG (i < 0.05 after GRF correction). (B) In all subjects, the differences resulted from ANOVA test among four groups are located in the bilateral MPFC, while the low-risk group displays the increased functional connectivity in MPFC only when compared with CLU-predominant middle-risk group (P < 0.05 after GRF correction). All subjects included APOEε4. interactive effect of CLU and ABCA7, high-risk = GG + GG/GG + GT/GC + GG/GC + GT; middle-risk = GG + TT/GC + TT/CC + GG/CC + GT; low-risk = CC + TT; middle-risk1 (CLU-dominated) = GG + TT/GC + TT; middle-risk2 (ABCA7-dominated) = CC + GG/CC + GT.
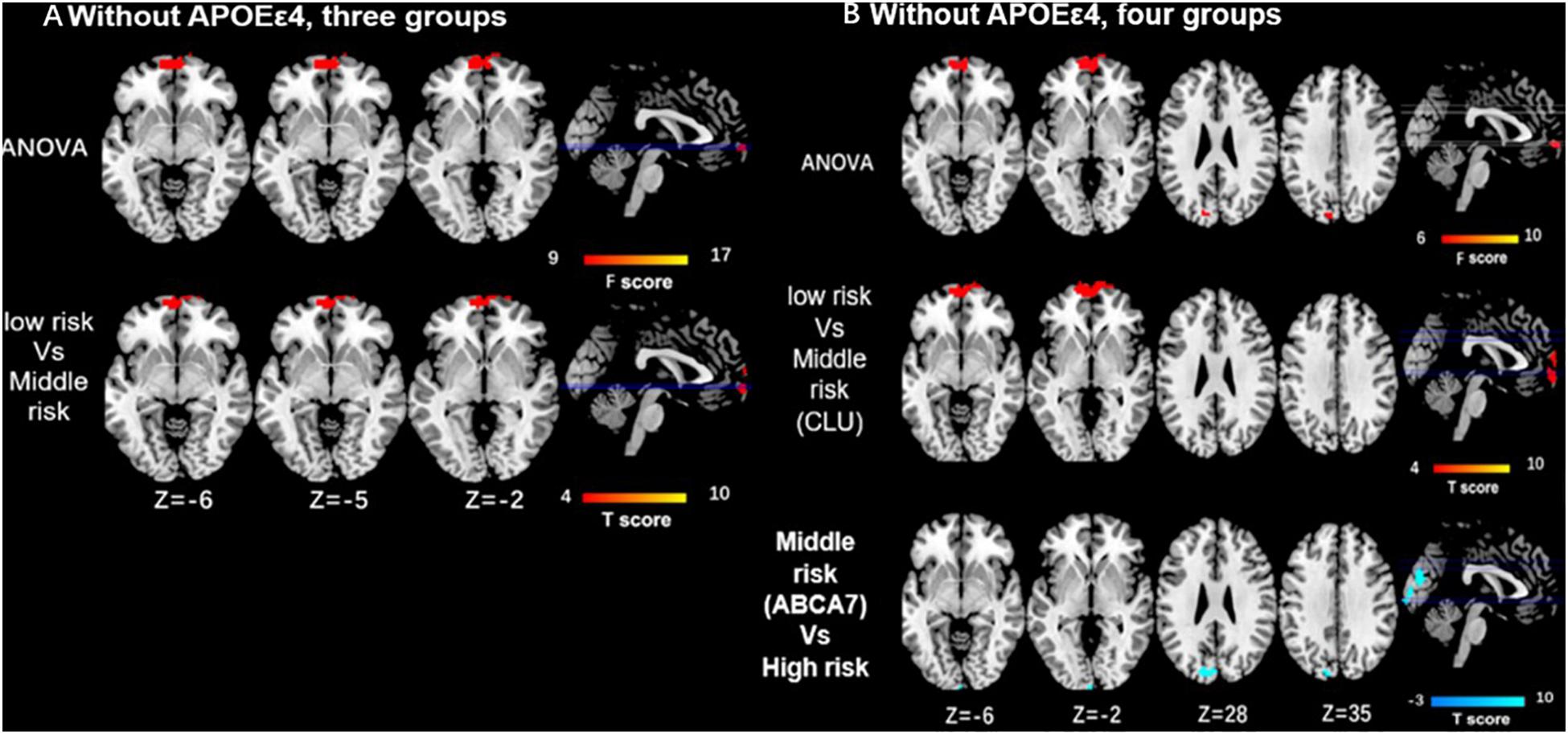
Figure 3. Differences of functional connectivity influenced by the interaction of CLU and ABCA7 in subjects without APOEε4. (A) Without including APOEε4, the major differences among the three groups are located in the MPFC/SFG, and the low-risk group displays the enhanced activity in MPFC/SFG when compared with middle-risk group (P < 0.05 after GRF correction). (B) ANOVA resulted from four groups demonstrates the altered activity in MPFC and cuneus. Specifically, the low-risk group shows enhanced functional connectivity in MPFC when compared with CLU-predominant middle-risk group, while the high-risk group displays increased activity in cuneus when compared with ABCA7-prodominant middle-risk group (P < 0.05 after GRF correction). All subjects without APOEε4. Interactive effect of CLU and ABCA7, high-risk = GG + GG/GG + GT/GC + GG/GC + GT; middle-risk = GG + TT/GC + TT/CC + GG/CC+GT; low-risk = CC + TT; middle-risk1 (CLU-dominated) = GG + TT/GC + TT; middle-risk2 (ABCA7-dominated) = CC + GG/CC + GT.
Correlation Analysis Results
The z values of left middle frontal cortex extracted from the result of ANOVA were positively related to the scores of SDT in high-risk group of CLU (r = 0.335, P = 0.037). Furthermore, based on genotyping of CLU rs9331888 and ABCA7 rs3764650, the z values of MPFC and cuneus distracted from the result of ANOVA were positively related to the scores of MoCA in low-risk group of among the three groups or the four groups (P < 0.05) (Supplementary Tables S9, S10).
Discussion
This study demonstrated that MPFC-PCC was the common target brain region modulated by the interaction of CLU and ABCA7. Moreover, APOEε4 might be interacted with ABCA7 and CLU modulation in the middle-aged carriers.
Our research indicated that CLU-G and ABCA7-G could impact DMN connectivity, and MPFC-PCC was the common target modulated by the interaction of CLU and ABCA7. For ABCA7, ANOVA test results resulted from four groups demonstrated the altered activity in cuneus. However, there was no significant difference between the two groups after GRF correction, indicating that the effect on the functional connectivity of DMN may be weak. Zhang et al. (2015) studied the effects of PICALM and CLU on resting-state functional connectivity of hippocampus in healthy young people and found the interaction between two genotypes. Liao et al. (2014) found that there was a certain correlation between ABCA7 and APOEε4 in Han population. While a study of Han people with AD in northern China confirmed that ABCA7 rs3764650 was associated with AD (Liu et al., 2014; Choi et al., 2017). In our study, the CLU-predominant middle-risk group displayed the decreased functional connectivity in MPFC when compared with the low risk group, indicating that CLU-G and ABCA7-G could impact DMN connectivity. MPFC is thought to be related to cognitive and can dynamically regulate processing of emotion. It is closely related to the function of the limbic system and can integrate information from the internal and external environment and emotional information (Fransson and Marrelec, 2008; Kwapis et al., 2015). Many studies suggested that there was a difference of DMN in patients with AD in MPFC which was similar to our results (Beason-held, 2011; Wang et al., 2017; Kura et al., 2018). Tan et al. (2016) and Ye et al. (2017) found that CLU could continue affect the functional connectivity of DMN in the MPFC, indicating that MPFC-PCC was the common target modulated by the interaction of CLU and ABCA7.
APOEε4 is a recognized risk gene for AD (Andrewshanna et al., 2010). APOEε4 might have an influence on the effect of CLU and ABCA7. In our study, when APOEε4 carriers were excluded, the CLU-predominant middle-risk group displayed the decreased functional connectivity in MPFC when compared with the low-risk group, while ABCA7-prodominant middle-risk group displayed decreased activity in cuneus when compared with the high-risk group. DeMattos et al. (2004) pointed out that APOEε4 and CLU could cooperate to inhibit the deposition of Aβ, APOEε4 and CLU might severely alter the clearance of Aβ on the blood-brain barrier (Haight et al., 2018). In another study of brain functional connectivity in healthy young adults, the DMN functional connectivity of APOEε4 carriers was enhanced (Nagai et al., 2004). Consistent with our results that when APOEε4 carriers were excluded, the ANOVA resulting from four groups demonstrated the altered activity in MPFC and cuneus, which indicated that APOEε4 might be interacted with ABCA7 and CLU modulation in the middle-aged carriers. Otherwise, the changes of functional connectivity density were significantly correlated with neuropsychological scores. Studies have shown the short-term functional connectivity density decreases in the PCC and precuneus, increases in the medial frontal cortex and middle frontal gyrus (Zhao et al., 2017). This is consistent with the results of our study that the functional connectivity changes of MPFC are significantly correlated with the scores of MoCA.
This study had some limitations. Firstly, we only chose middle age adults for investigating the influence of ABCA7 and CLU on the DMN function connectivity. Natalya et al. (2013) found that the influence of CLU genotype was found to be higher in the subjects older than 50 years old. Fennemanotestine et al. (2011) found that presence of APOEε4 was associated with thinner frontal cortex in middle age adults. However, all the subjects were middle-aged, and the differences in image representation might be small, which could not fully explain the differences in gene effects. Secondly, ε2 genotype was reported as a protective gene for delayed AD, the influence of ε2 was not further observed in this study. Thirdly, other factors, including social, economic, family composition and some other conditions should be taken into account in the future to exclude the impact of environmental factors.
In summary, this study indicates MPFC-PCC connectivity was the common target which was modulated by the interaction of CLU and ABCA7. Moreover, APOEε4 might be interacted with ABCA7 and CLU modulation in the middle-aged carriers.
Data Availability Statement
The original contributions presented in the study are included in the article/Supplementary Material, further inquiries can be directed to the corresponding author.
Ethics Statement
The studies involving human participants were reviewed and approved by Affiliated Jinling Hospital, Medical School of Nanjing University. The patients/participants provided their written informed consent to participate in this study.
Author Contributions
XZ analyzed data and wrote the first draft of the manuscript. YW and LiZ contributed equally to this work in study design, literature search, and manuscript editing. HZ and LL were responsible for recruiting the subjects and collecting data. GL reviewed and revised the manuscript. LoZ designed the study, conducted the initial analyses, and reviewed and revised the manuscript. All authors have contributed to and have approved the final manuscript.
Funding
Supported by the grants from the Natural Scientific Foundation of China (81322020, 81230032, and 8180071661 to LiZ).
Conflict of Interest
The authors declare that the research was conducted in the absence of any commercial or financial relationships that could be construed as a potential conflict of interest.
Supplementary Material
The Supplementary Material for this article can be found online at: https://www.frontiersin.org/articles/10.3389/fnmol.2020.00145/full#supplementary-material
TABLE S1 | CLU rs11136000, rs2279590, rs9331888, rs9331949 score, and ABCA7 rs3764650, rs4147929 score.
TABLE S2 | Genotypes of CLUrs9331888 and ABCA7rs3764650.
TABLE S3 | Demographic, clinical, and neuropsychological data of CLU risk gene scores without APOE.
TABLE S4 | Demographic, clinical, and neuropsychological data of ABCA7 risk gene scores without APOE.
TABLE S5 | Demographic, clinical, and neuropsychological data of three groups (low-risk group, middle-risk group, high-risk group) with APOE.
TABLE S6 | Demographic, clinical, and neuropsychological data of four groups (low-risk group, CLU-predominant middle-risk group, ABCA7-prodominant middle-risk group, high-risk group) with APOE.
TABLE S7 | Demographic, clinical, and neuropsychological data of three groups (low-risk group, middle-risk group, high-risk group) without APOE.
TABLE S8 | Demographic, clinical, and neuropsychological data of four groups (low-risk group, CLU-predominant middle-risk group, ABCA7-prodominant middle-risk group, high-risk group) without APOE.
TABLE S9 | Correlation analysis results of the z values of MPFC and cuneus distracted from the result of ANOVA with APOE.
TABLE S10 | Correlation analysis results of the z values of MPFC and cuneus distracted from the result of ANOVA without APOE.
Abbreviations
ABCA7, ATP-binding cassette A7; AD, Alzheimer’s disease; APOE, apolipoprotein E; CLU, clusterin; CUN, cuneus; DMN, default mode network; MFG, middle frontal gyrus; MPFC, medial prefrontal cortex; PCC/PCu, posterior cingulate cortex/precuneus; rs-fMRI, resting-state functional MR imaging; SFG, superior frontal gyrus.
Footnotes
References
Abushakra, S., Porsteinsson, A., Scheltens, P., Sadowsky, C., Vellas, B., Cummings, J., et al. (2017). Clinical effects of tramiprosate in APOE4/4 homozygous patients with mild Alzheimer’s disease suggest disease modification potential. J. Prev. Alzheimers Dis. 4, 149–156.
Andrewshanna, J. R., Reidler, J. S., Sepulcre, J., Poulin, R., and Buckner, R. L. (2010). Functional-. (anatomic). fractionation of the brain’s default network. Neuron 65, 550–562. doi: 10.1016/j.neuron.2010.02.005
Beason-held, L. L. (2011). Dementia and the default mode. Curr. Alzheimer Res. 8, 361–365. doi: 10.2174/156720511795745294
Choi, J. H., Lee, B. H., Sun, H. H., Kim, G. H., Kim, Y. M., Kim, D. S., et al. (2017). Clinical characteristics and mutation spectrum of GLA in Korean patients with Fabry disease by a nationwide survey: underdiagnosis of late-onset phenotype. Medicine 96:e7387. doi: 10.1097/md.0000000000007387
Chouraki, V., Reitz, C., Maury, F., Bis, J. C., Bellenguez, C., Yu, L., et al. (2016). Evaluation of a genetic risk score to improve risk prediction for Alzheimer’s disease. J. Alzheimers Dis. 53, 921–932.
Corneveaux, J. J., Myers, A. J., Allen, A. N., Pruzin, J. J., Ramirez, M., Engel, A., et al. (2010). Association of CR1, CLU and PICALM with Alzheimer’s disease in a cohort of clinically characterized and neuropathologically verified individuals. Hum. Mol. Genet. 19, 3295–3301. doi: 10.1093/hmg/ddq221
DeMattos, R. B., Cirrito, J. R., Parsadanian, M., May, P. C., O’Dell, M. A., Taylor, J.W., et al. (2004). ApoE and clusterin cooperatively suppress Aβ levels and deposition: evidence that ApoE regulates extracellular Aβ metabolism in vivo. Neuron 41, 193–202. doi: 10.1016/s0896-6273(03)00850-x
Fennemanotestine, C., Panizzon, M. S., Thompson, W. R., Chen, C. H., Eyler, L. T., Fischl, B., et al. (2011). Presence of ApoE ε4 allele associated with thinner frontal cortex in middle age. J. Alzheimers Dis. 26(Suppl. 3), 49–60. doi: 10.3233/jad-2011-0002
Fernándezespejo, D., Junque, C., Cruse, D., Bernabeu, M., Roig-Rovira, T., Fábregas, N., et al. (2010). Combination of diffusion tensor and functional magnetic resonance imaging during recovery from the vegetative state. BMC Neurol. 10:77. doi: 10.1186/1471-2377-10-77
Filippini, N., Macintosh, B. J., Hough, M. G., Goodwin, G. M., Frisoni, G. B., Smith, S. M., et al. (2009). Distinct patterns of brain activity in young carriers of the APOE-ε4 allele. Proc. Natl. Acad. Sci. U.S.A. 106, 7209–7214. doi: 10.1073/pnas.0811879106
Fransson, P., and Marrelec, G. (2008). The precuneus/posterior cingulate cortex plays a pivotal role in the default mode network: evidence from a partial correlation network analysis. Neuroimage 42, 1178–1184. doi: 10.1016/j.neuroimage.2008.05.059
Galea, M., and Woodward, M. (2005). Mini-mental state examination (MMSE). Aust. J. Physiother. 51:198. doi: 10.1016/s0004-9514(05)70034-9
Greicius, M. D., Srivastava, G., Reiss, A. L., and Menon, V. (2004). Default-mode network activity distinguishes Alzheimer’s disease from healthy aging: evidence from functional MRI. Proc. Natl. Acad. Sci. U.S.A. 101, 4637–4642. doi: 10.1073/pnas.0308627101
Haight, T., Bryan, R. N., Meirelles, O., Tracy, R., Fornage, M., Richard, M., et al. (2018). Associations of plasma clusterin and Alzheimer’s disease-related MRI markers in adults at mid-life: the CARDIA Brain MRI sub-study. PLoS One 13:e0190478. doi: 10.1371/journal.pone.0190478
Harold, D., Abraham, R., Hollingworth, P., Sims, R., Gerrish, A., Hamshere, M., et al. (2009). Genome-wide association study identifies variants at CLU and PICALM associated with Alzheimer’s disease. Nat. Genet. 41, 1088–1093.
Karch, C. M., and Goate, A. M. (2015). Alzheimer’s disease risk genes and mechanisms of disease pathogenesis. Biol. Psychiatry 77, 43–51. doi: 10.1016/j.biopsych.2014.05.006
Kura, S., Xie, H., Fu, B., Ayata, C., Boas, D. A., and Sakadžiæ, S. (2018). Intrinsic optical signal imaging of the blood volume changes is sufficient for mapping the resting state functional connectivity in the rodent cortex. J. Neural Eng. 15:035003. doi: 10.1088/1741-2552/aaafe4
Kwapis, J. L., Jarome, T. J., Lee, J. L., and Helmstetter, F. J. (2015). The retrosplenial cortex is involved in the formation of memory for context and trace fear conditioning. Neurobiol. Learn. Mem. 123, 110–116. doi: 10.1016/j.nlm.2015.06.007
Lee, T. W., and Xue, S. W. (2018). Functional connectivity maps based on hippocampal and thalamic dynamics may account for the default-mode network. Eur. J. Neurosci. 47, 388–389.
Liao, Y. C., Lee, W. J., Hwang, J. P., Wang, Y. F., Tsai, C. F., Wang, P. N., et al. (2014). ABCA7 gene, and the risk of Alzheimer’s disease in Han Chinese in Taiwan. Neurobiol. Aging 35, 2423.e7–2423.e13. doi: 10.1016/j.neurobiolaging.2014.05.009
Liu, L. H., Xu, J., Deng, Y. L., Tang, H. D., Wang, Y., Ren, R. J., et al. (2014). A complex association of ABCA7 genotypes with sporadic Alzheimer disease in Chinese Han population. Alzheimer Dis. Assoc. Disord. 28, 141–144. doi: 10.1097/wad.0000000000000000
Machulda, M. M., Jones, D. T., Vemuri, P., McDade, E., Avula, R., Przybelski, S., et al. (2011). Effect of APOE ε4 status on intrinsic network connectivity in cognitively normal elderly subjects. Arch. Neurol. 68, 1131–1136.
Mcfall, G. P., Sapkota, S., Mcdermott, K. L., and Dixon, R. A. (2016). Risk-reducing Apolipoprotein E and Clusterin genotypes protect against the consequences of poor vascular health on executive function performance and change in non-demented older adults. Neurobiol. Aging 42, 91–100. doi: 10.1016/j.neurobiolaging.2016.02.032
Nagai, Y., Critchley, H. D., Featherstone, E., Trimble, M. R., and Dolan, R. J. (2004). Activity in ventromedial prefrontal cortex covaries with sympathetic skin conductance level: a physiological account of a “default mode” of brain function. Neuroimage 22, 243–251. doi: 10.1016/j.neuroimage.2004.01.019
Nasreddine, Z. S., Phillips, N. A., Bédirian, V., Charbonneau, S., Whitehead, V., Collin, I., et al. (2005). The montreal cognitive assessment, MoCA: a brief screening tool for mild cognitive impairment. J. Am. Geriatr. Soc. 53, 695–699. doi: 10.1111/j.1532-5415.2005.53221.x
Natalya, P., Tatiana, A., Maria, P., Shagam, L., Malina, D., Goltsov, A., et al. (2013). Age-dependent effect of Alzheimer’s risk variant of CLU on EEG alpha rhythm in non-demented adults. Front. Aging Neurosci. 5:86. doi: 10.3389/fnagi.2013.00086
Nelson, A. R., Sagare, A. P., and Zlokovic, B. V. (2017). Role of clusterin in the brain vascular clearance of amyloid-Î2. Proc. Natl. Acad. Sci. U.S.A. 114, 8681–8682. doi: 10.1073/pnas.1711357114
Patel, K. T., Stevens, M. C., Pearlson, G. D., Winkler, A. M., Hawkins, K. A., Skudlarski, P., et al. (2013). Default mode network activity, and white matter integrity in healthy middle-aged ApoE4 carriers. Brain Imaging Behav. 7, 60–67. doi: 10.1007/s11682-012-9187-y
Rajabli, F., Feliciano, B. E., Celis, K., Hamilton-Nelson, K. L., Whitehead, P. L., Adams, L. D., et al. (2018). Ancestral origin of ApoE ε4 Alzheimer disease risk in Puerto Rican and African American populations. PLoS Genet. 14:e1007791. doi: 10.1371/journal.pgen.1007791
Rajan, K. B., Barnes, L. L., Wilson, R. S., Weuve, J., McAninch, E. A., and Evans, D. A. (2019). Apolipoprotein E genotypes, age, race, and cognitive decline in a population sample. J. Am. Geriatr. Soc. 67, 734–740. doi: 10.1111/jgs.15727
Roussotte, F. F., Gutman, B. A., Madsen, S. K., Colby, J. B., Thompson, P. M., and Alzheimer’s Disease, et al. (2014). Combined effects of Alzheimer risk variants in the CLU and ApoE genes on ventricular expansion patterns in the elderly. J. Neurosci. 34, 6537–6545. doi: 10.1523/jneurosci.5236-13.2014
Salmon, D. P., and Lange, K. L. (2001). Cognitive screening and neuropsychological assessment in early Alzheimer’s disease. Clin. Geriatr. Med. 17, 229–254. doi: 10.1016/s0749-0690(05)70067-7
Sankari, S. E., Gondry-Jouet, C., Fichten, A., Godefroy, O., Serot, J. M., Deramond, H., et al. (2011). Cerebrospinal fluid and blood flow in mild cognitive impairment and Alzheimer’s disease: a differential diagnosis from idiopathic normal pressure hydrocephalus. Fluids Barriers CNS 8:12. doi: 10.1186/2045-8118-8-12
Shi, H., Belbin, O., Medway, C., Brown, K., Kalsheker, N., Carrasquillo, M., et al. (2012). Genetic variants influencing human aging from late-onset Alzheimer’s disease (LOAD) genome-wide association studies. Neurobiol. Aging 33:1849.
Sinha, N., Reagh, Z. M., Tustison, N. J., Berg, C. N., Shaw, A., Myers, C. E., et al. (2018). ABCA7 risk variant in healthy older african americans is associated with a functionally isolated entorhinal cortex mediating deficient generalization of prior discrimination training. Hippocampus 29, 527–538. doi: 10.1002/hipo.23042
Sleegers, K., Bettens, K., De Roeck, A., Van Cauwenberghe, C., Cuyvers, E., Verheijen, J., et al. (2015). A 22-single nucleotide polymorphism Alzheimer risk score correlates with family history, onset age, and cerebrospinal fluid Aβ42. Alzheimers Dement. 11, 1452–1460. doi: 10.1016/j.jalz.2015.02.013
Tan, L., Wang, H. F., Tan, M. S., Tan, C. C., Zhu, X. C., Miao, D., et al. (2016). Effect of CLU genetic variants on cerebrospinal fluid and neuroimaging markers in healthy, mild cognitive impairment and Alzheimer’s disease cohorts. Sci. Rep 6:26027.
Wang, L., Shen, H., Lei, Y., Zeng, L. L., Cao, F., Su, L., et al. (2017). Altered default mode, fronto-parietal and salience networks in adolescents with Internet addiction. Addict. Behav. 70, 1–6. doi: 10.1016/j.addbeh.2017.01.021
Ye, Q., Su, F., Shu, H., Gong, L., Xie, C. M., Zhou, H., et al. (2017). Shared effects of the clusterin gene on the default mode network among individuals at risk for Alzheimer’s disease. CNS Neurosci. Ther. 23, 395–404. doi: 10.1111/cns.12682
Yu, J. T., Li, L., Zhu, Q. X., Zhang, Q., Zhang, W., Wu, Z. C., et al. (2010). Implication of CLU gene polymorphisms in Chinese patients with Alzheimer’s disease. Clin. Chim. Acta 411, 1516–1519. doi: 10.1016/j.cca.2010.06.013
Zhang, P., Qin, W., Wang, D., Liu, B., Zhang, Y., Jiang, T., et al. (2015). Impacts of PICALM and CLU variants associated with Alzheimer’s disease on the functional connectivity of the hippocampus in healthy young adults. Brain Struct. Funct. 220, 1463–1475. doi: 10.1007/s00429-014-0738-4
Keywords: ATP-binding cassette A7, clusterin, default mode network, Alzheimer’s disease, middle-age adults
Citation: Zhang XY, Wang YF, Zheng LJ, Zhang H, Lin L, Lu GM and Zhang LJ (2020) Impacts of AD-Related ABCA7 and CLU Variants on Default Mode Network Connectivity in Healthy Middle-Age Adults. Front. Mol. Neurosci. 13:145. doi: 10.3389/fnmol.2020.00145
Received: 02 April 2020; Accepted: 13 July 2020;
Published: 31 July 2020.
Edited by:
Albert I. Chen, Nanyang Technological University, SingaporeReviewed by:
Massimo Marano, Campus Bio-Medico University, ItalyGina L. Forster, University of Otago, New Zealand
Copyright © 2020 Zhang, Wang, Zheng, Zhang, Lin, Lu and Zhang. This is an open-access article distributed under the terms of the Creative Commons Attribution License (CC BY). The use, distribution or reproduction in other forums is permitted, provided the original author(s) and the copyright owner(s) are credited and that the original publication in this journal is cited, in accordance with accepted academic practice. No use, distribution or reproduction is permitted which does not comply with these terms.
*Correspondence: Long Jiang Zhang, a2V2aW56aGxqQDE2My5jb20=
†These authors have contributed equally to this work