- 1Department of Diagnostic and Interventional Radiology, University of Leipzig, Leipzig, Germany
- 2Institute of Medical Epidemiology, Biostatistics and Informatics, Martin-Luther-University Halle-Wittenberg, Halle, Germany
Background: The purpose of the present meta-analysis was to provide evident data about use of apparent diffusion coefficient (ADC) values for distinguishing malignant and benign lesions in the head and neck region.
Material and Methods: MEDLINE and Scopus databases were screened for associations between ADC and malignancy/benignancy of head and neck lesions up to December 2018. Overall, 22 studies met the inclusion criteria. The following data were extracted: authors, year of publication, study design, number of patients/lesions, lesion type, mean value, and standard deviation of ADC. The primary endpoint of the systematic review was the analysis of the association between lesion nature and ADC values. The methodological quality of the involved studies was checked according to the Quality Assessment of Diagnostic Accuracy Studies (QUADAS) instrument. The meta-analysis was undertaken by using RevMan 5.3 software. DerSimonian and Laird random-effects models with inverse-variance weights were used without further correction to account for the heterogeneity between the studies. Mean ADC values including 95% confidence intervals were calculated separately for benign and malignant lesions.
Results: The acquired 22 studies comprised 1,227 lesions. Different malignant lesions were diagnosed in 818 cases (66.7%) and benign lesions in 409 cases (33.3%). The mean ADC value of the malignant lesions was 1.04 × 10−3 mm2/s, and the mean value of the benign lesions was 1.46 × 10−3 mm2/s. Lymphomas and sarcomas showed the lowest calculated mean ADC values, 0.7 and 0.79 × 10−3 mm2/s, respectively. Adenoid cystic carcinomas had the highest ADC values (1.5 × 10−3 mm2/s). None of the analyzed malignant tumors had mean ADC values above 1.75 × 10−3 mm2/s.
Conclusion: ADC values play a limited role in distinguishing between malignant and benign lesions in the head and neck region. It may be only suggested that lesions with mean ADC values above 1.75 × 10−3 mm2/s are probably benign. Further large studies are needed for the analysis of the role of diffusion-weighted imaging (DWI)/ADC in the discrimination of benign and malignant lesions in the head and neck region.
Introduction
Diffusion-weighted imaging (DWI) is a magnetic resonance imaging (MRI) technique based on measure of water diffusion in tissues (1). Restriction of water diffusion can be quantified by apparent diffusion coefficient (ADC) (1). Numerous studies have reported that DWI/ADC can provide information regarding histological architecture of tissues. According to the literature, ADC is associated with several histopathological features, such as cell count and expression of proliferation markers (2, 3). So it has been shown that ADC correlated well with expression of Ki67 in head and neck squamous cell carcinoma (4, 5). Furthermore, ADC can predict other important histopathological features, such as expression of vascular endothelial growth factor, tumor suppressor protein p53, hypoxia-inducible factor (HIF)-1α, CD3-positive cell count, and human papilloma virus (p16) (5–7).
In clinical setting, however, a key question is whether DWI/ADC can be used for distinguishing between malignant and benign lesions. Overall, it is well-known that malignant tumors have lower ADC values than have benign lesions. However, the physician needs plausible threshold values in his or her daily practice. Previously, some reports analyzed the diagnostic potential of DWI in the head and neck region (HNR). However, most reported studies investigated relatively small samples of up to 100 patients/lesions, and, therefore, the provided data cannot be applied as evident. Furthermore, the reported studies provided a broad spectrum of ADC threshold values. For example, Wang et al., based on an analysis of 97 different head and neck lesions, proposed a diagnostic scale of ADC values to predict malignancy in HNR (8). It has been shown that ADC values ≤0.65 × 10−3 mm2/s had a positive predictive value of malignancy of 100% and that ADC values ≤1.01 × 10−3 mm2/s had a positive predictive value of malignancy of 90% (8). In the study of Das et al. investigating 79 sinonasal masses, a cutoff ADC value of 1.791 × 10−3 mm2/s was identified to differentiate malignant and benign lesions with a sensitivity of 80% and specificity of 83.3% (9). Finally, Li et al. studied 78 patients with lingual lesions and calculated a threshold ADC value of <1.31 × 10−3 mm2/s (sensitivity, 92.6%; specificity, 97.3%) (10).
The aim of the present meta-analysis was to provide data regarding use of ADC for distinguishing malignant and benign lesions in the HNR based on a large sample.
Materials and Methods
Data Acquisition and Proving
MEDLINE and Scopus databases were screened for associations between ADC and malignancy/benignancy of head and neck lesions up to December 2018 (Figure 1). The search terms/combinations were as follows:
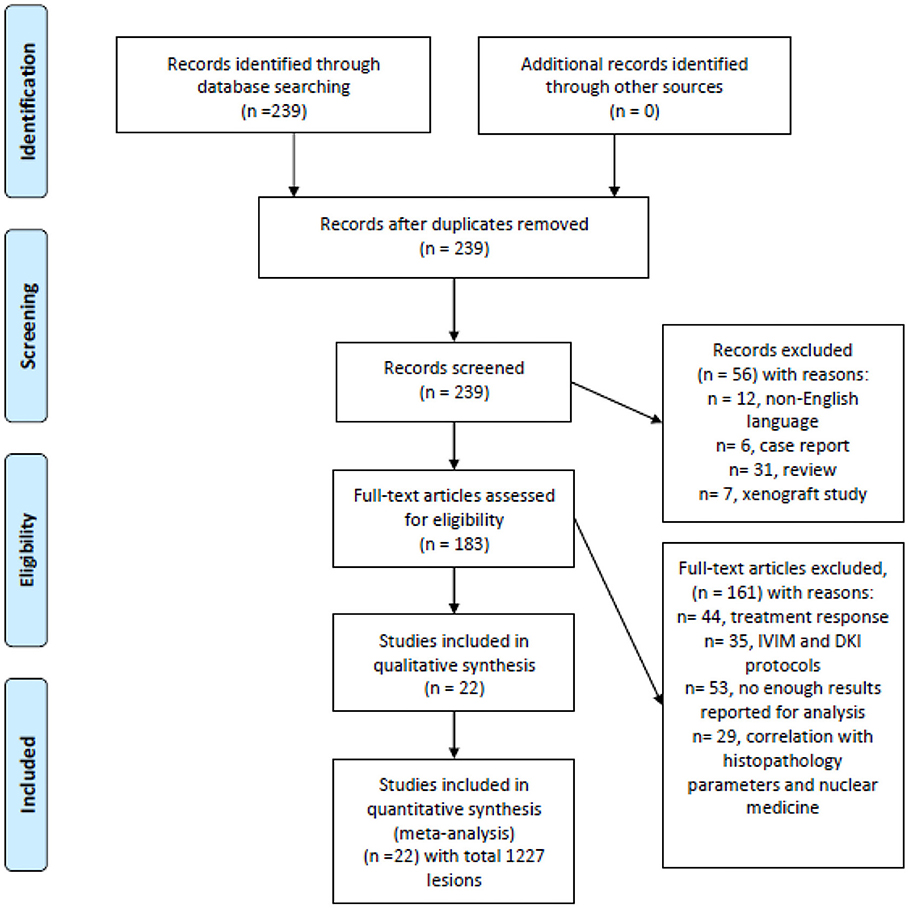
Figure 1. Preferred reporting items for systematic reviews and meta-analyses (PRISMA) flowchart of the data acquisition.
“DWI or diffusion weighted imaging or diffusion-weighted imaging or ADC or apparent diffusion coefficient or DWI or diffusion weighted imaging AND head and neck OR neck carcinoma OR neck cancer OR neck neoplasm OR neck tumor.” Secondary references were also manually checked. The Preferred Reporting Items for Systematic Reviews and Meta-Analyses (PRISMA) statement was used for the research (11).
The primary endpoint of the systematic review was the analysis of association between the nature of head and neck lesions and their ADC values. The primary search identified 239 records. The abstracts of the items were checked. Inclusion criteria for this work were as follows:
- data regarding ADC derived from DWI,
- available mean and standard deviation values of ADC,
- original studies that investigated humans, and
- written in English.
Overall, 22 studies meet the inclusion criteria (9, 10, 12–31). Other 217 records were excluded from the analysis. Exclusion criteria were as follows:
- studies unrelated to the research subjects,
- studies with incomplete data,
- not written in English,
- duplicate publications,
- experimental animal and in vitro studies, and
- review, meta-analysis, and case report articles.
On the next step, the following data were extracted from the literature: authors, year of publication, study design, number of patients/tumors, tumor/lesion type, and mean value and standard deviation of ADC.
Meta-Analysis
The methodological quality of the identified 22 studies was checked according to the Quality Assessment of Diagnostic Accuracy Studies (QUADAS) instrument (32) independently by two observers (A.S. and H.J.M.) (Figure 2).
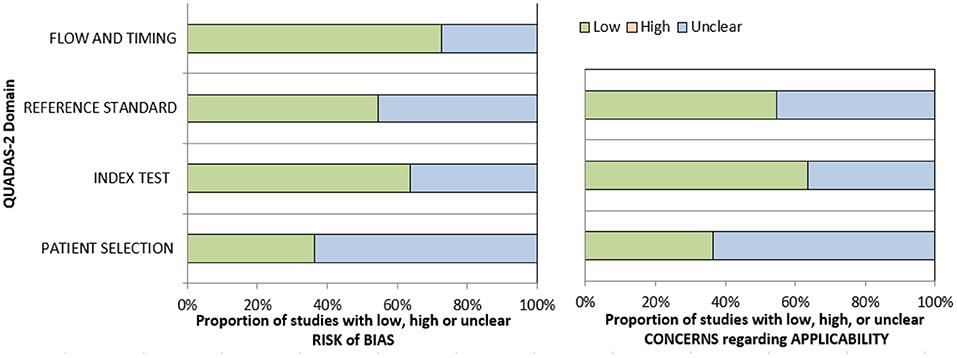
Figure 2. Quality assessment of diagnostic accuracy studies (QUADAS)-2 quality assessment of the included studies.
The meta-analysis was undertaken by using RevMan (RevMan 2014, the Cochrane Collaboration Review Manager Version 5.3). Heterogeneity was calculated by means of the inconsistency index I2 (33, 34). DerSimonian and Laird random-effects models with inverse-variance weights were used without corrections (35). Mean ADC values including 95% confidence intervals were calculated separately for benign and malignant lesions.
Results
Of the included 22 studies, 14 (64%) were retrospective, and 8 (36%) prospective. Overall, these studies comprised 1,227 lesions. Different malignancies of the HNR were diagnosed in 818 cases (66.7%) and benign lesions in 409 cases (33.3%) (Table 1). The mean ADC values of the malignant lesions ranged from 0.75 to 1.35 × 10−3 mm2/s, and the calculated mean value was 1.04 × 10−3 mm2/s (Figure 3).
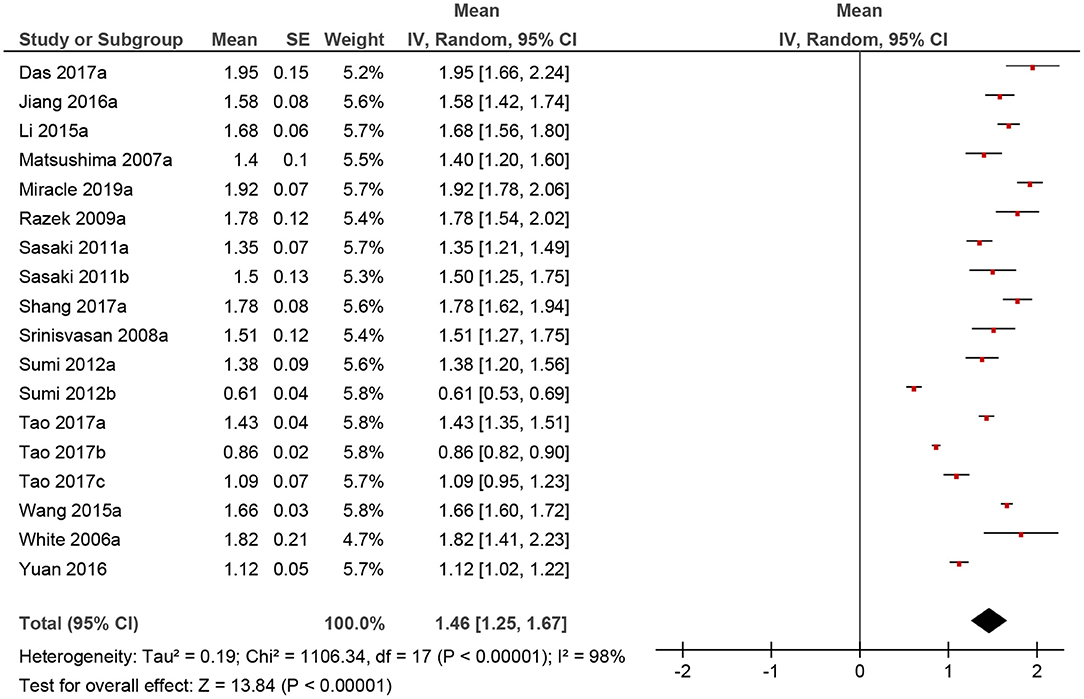
Figure 3. Forrest plots of apparent diffusion coefficient (ADC) values of benign lesions of the head and neck region.
The calculated mean value of the benign lesions was 1.46 × 10−3 mm2/s, and the range of the collected ADC values was 0.61–1.95 × 10−3 mm2/s (Figure 4). The graphical distribution of ADC values in malignant and benign lesions is shown in Figure 5. The ADC values overlapped significantly.
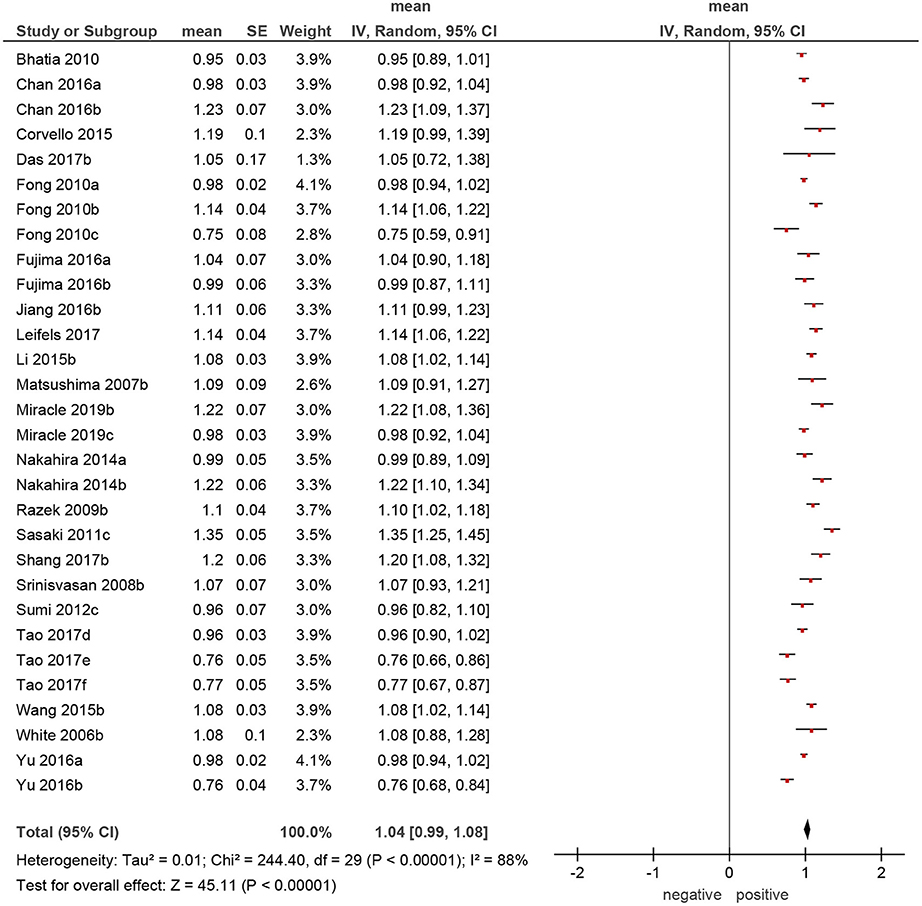
Figure 4. Forrest plots of apparent diffusion coefficient (ADC) values of malignant tumors of the head and neck region.
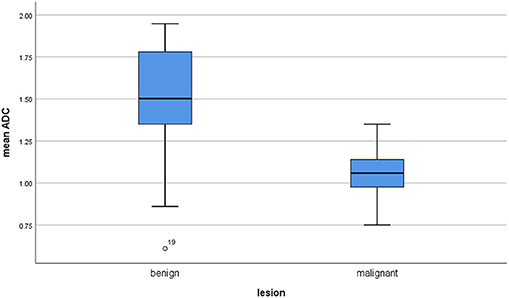
Figure 5. Comparison of apparent diffusion coefficient (ADC) values between malignant and benign lesions.
Furthermore, the reported mean ADC values of different malignant lesions were analyzed (Figure 6). Lymphomas and sarcomas showed the lowest calculated mean ADC values of 0.7 and 0.79 × 10−3 mm2/s, respectively. Adenoid cystic carcinomas had the highest ADC values (1.5 × 10−3 mm2/s). The calculated mean ADC values of squamous cell carcinomas and neuroblastomas were 1.09 and 1.02 × 10−3 mm2/s, respectively. None of the analyzed malignant tumors had mean ADC values above 1.75 × 10−3 mm2/s.
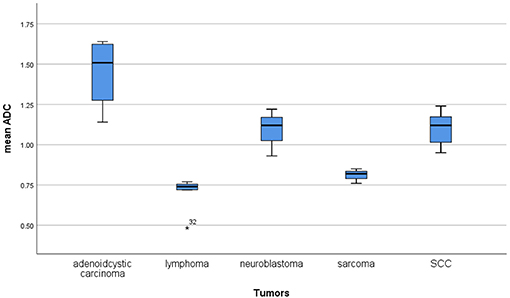
Figure 6. Comparison of apparent diffusion coefficient (ADC) values between different malignant lesions.
Discussion
Our analysis showed that both malignant and benign lesions in HNR presented with a broad spectrum of ADC values. Although malignant tumors had lower ADC values than had benign lesions, the reported ADC values overlapped significantly. This fact made it impossible to define a reliable threshold for distinguishing malignant and benign lesion in HNR. Furthermore, our finding can explain contradictory results of the previous studies. It is well-known that some benign head/neck lesions, such as cholesteatomas and adenoid hypertrophy, show very low ADC values (36, 37), and some tumors like adenoid cystic carcinomas have high ADC values (8, 9). Presumably, studies with different malignant and/or benign lesions of HNR may have different threshold ADC values. This fact is very important. Therefore, analyses of ADC values between malignant and benign HNR lesions should include all possible entities.
We included all published ADC values of different HNR lesions into the present analysis without selection bias. To the best of our knowledge, our analysis comprises the largest cohort to date. We could not find thresholds in the lower areas of ADC values because malignant and benign lesions overlapped significantly. However, the reported ADC values of all malignant lesions were under 1.75 × 10−3 mm2/s. Therefore, it may be postulated that lesions with mean ADC values above 1.75 × 10−3 mm2/s are probably benign. Our results also demonstrated that no real thresholds can be found in the area with <1.75 × 10−3 mm2/s for the discrimination of malignant and benign lesions. Furthermore, the present analysis showed that lymphomas and sarcomas had the lowest mean ADC values and that adenoid cystic carcinomas had the highest ADC values. This finding is in agreement with that of previous reports (8, 38).
Overall, the present analysis showed that DWI/ADC alone cannot be used as an imaging biomarker of malignancy in the HNR. However, it is known that areas of high cellularity and high proliferation potentially have lower ADC values than have areas of low cellularity, independent of lesion nature (2, 3). Furthermore, numerous previous reports mentioned that necrotic tumor areas show lower ADC values than do solid parts. Therefore, areas of low ADC values may be used as an additional target for biopsies.
Our analysis contains some limitations. Firstly, it is based only on results written in English. Secondly, it analyzed DWI technique using 2 b values. However, more advanced imaging techniques, like intravoxel incoherent motion imaging and diffusion kurtosis imaging, which might show a better accuracy in discriminating benign from malignant tumors, were not included in the analysis. Thirdly, we did not analyze a possible influence of some technical details, such as sequence type, choice of b values, and Tesla strength. The following aspect should also be addressed: Previously, some authors indicated that ADC values depended significantly on ADC measurements (39, 40). It has been shown that different drawing methods, for example, whole tumor measurements, choice of multiple regions of interest, and/or single region measure, can influence ADC values (39, 40). Therefore, different ADC measurements should be also considered as an important factor. However, a recent large meta-analysis showed that relationships of ADC values between malignant and benign breast lesions were independent of MR technique and measurements (41).
Overall, our analysis is based on heterogenous and predominantly retrospective samples. However, it reflects a real clinical situation in the daily routine.
In conclusion, our analysis showed that ADC values play a limited role in distinguishing between malignant and benign lesions in the HNR.
Lesions with mean ADC values above 1.75 × 10−3 mm2/s are probably benign. Further large studies are needed for the analysis of the role of DWI/ADC in the discrimination of benign and malignant lesions in the HNR.
Author Contributions
All authors listed have made a substantial, direct and intellectual contribution to the work, and approved it for publication.
Conflict of Interest
The authors declare that the research was conducted in the absence of any commercial or financial relationships that could be construed as a potential conflict of interest.
Abbreviations
HNR, head and neck region; MRI, magnetic resonance imaging; ADC, apparent diffusion coefficient.
References
1. Fornasa F. Diffusion-weighted magnetic resonance imaging: what makes water run fast or slow? J Clin Imaging Sci. (2011) 1:27. doi: 10.4103/2156-7514.81294
2. Surov A, Meyer HJ, Wienke A. Correlation between apparent diffusion coefficient (ADC) and cellularity is different in several tumors: a meta-analysis. Oncotarget. (2017) 8:59492–9. doi: 10.18632/oncotarget.17752
3. Surov A, Meyer HJ, Wienke A. Correlation between apparent diffusion coefficient (ADC) and KI 67 in different tumors: a meta-analysis. Part 1: ADCmean. Oncotarget. (2017) 8:75434–44. doi: 10.18632/oncotarget.20406
4. Surov A, Stumpp P, Meyer HJ, Gawlitza M, Höhn AK, Boehm A, et al. Simultaneous (18)F-FDG-PET/MRI: associations between diffusion, glucose metabolism and histopathological parameters in patients with head and neck squamous cell carcinoma. Oral Oncol. (2016) 58:14–20. doi: 10.1016/j.oraloncology.2016.04.009
5. Swartz JE, Driessen JP, van Kempen PMW, de Bree R, Janssen LM, Pameijer FA, et al. Influence of tumor and microenvironment characteristics on diffusion-weighted imaging in oropharyngeal carcinoma: a pilot study. Oral Oncol. (2018) 77:9–15. doi: 10.1016/j.oraloncology.2017.12.001
6. Meyer HJ, Leifels L, Hamerla G, Höhn AK, Surov A. ADC-histogram Analysis in head and neck squamous cell carcinoma. Associations with different histopathological features including expression of EGFR, VEGF, HIF-1α, Her 2, and p53. A preliminary study. Magn Reson Imaging. (2018) 54:214–7 doi: 10.1016/j.mri.2018.07.013
7. Surov A, Meyer HJ, Wienke A. Can imaging parameters provide information regarding histopathology in head and neck squamous cell carcinoma? A meta-analysis. Transl Oncol. (2018) 11:498–503. doi: 10.1016/j.tranon.2018.02.004
8. Wang J, Takashima S, Takayama F, Kawakami S, Saito A, Matsushita T, et al. Head and neck lesions: characterization with diffusion-weighted echo-planar MR imaging. Radiology. (2001) 220:621–30. doi: 10.1148/radiol.2202010063
9. Das A, Bhalla AS, Sharma R, Kumar A, Thakar A, Vishnubhatla SM, et al. Can diffusion weighted imaging aid in differentiating benign from malignant sinonasal masses? A useful adjunct. Pol J Radiol. (2017) 82:345–55. doi: 10.12659/PJR.900633
10. Li S, Cheng J, Zhang Y, Zhang Z. Differentiation of benign and malignant lesions of the tongue by using diffusion-weighted MRI at 3.0 T. Dentomaxillofac Radiol. (2015) 44:20140325. doi: 10.1259/dmfr.20140325
11. Moher D, Liberati A, Tetzlaff J, Altman DG. Preferred reporting items for systematic reviews and meta-analyses: the PRISMA statement. PLoS Med. (2009) 6:e1000097. doi: 10.1371/journal.pmed.1000097
12. Bhatia KS, King AD, Yeung DK, Mo F, Vlantis AC, Yu K-H, et al. Can diffusion-weighted imaging distinguish between normal and squamous cell carcinoma of the palatine tonsil? Br J Radiol. (2010) 83:753–8. doi: 10.1259/bjr/58331222
13. Chan MW, Higgins K, Enepekides D, Poon I, Symons SP, Moineddin R, et al. Radiologic differences between human papillomavirus-related and human papillomavirus-unrelated oropharyngeal carcinoma on diffusion-weighted imaging. ORL J Otorhinolaryngol Relat Spec. (2016) 78:344–52. doi: 10.1159/000458446
14. Covello M, Cavaliere C, Aiello M, Cianelli MS, Mesolella M, Iorio B, et al. Simultaneous PET/MR head-neck cancer imaging: preliminary clinical experience and multiparametric evaluation. Eur J Radiol. (2015) 84:1269–76. doi: 10.1016/j.ejrad.2015.04.010
15. Fong D, Bhatia KS, Yeung D, King AD. Diagnostic accuracy of diffusion-weighted MR imaging for nasopharyngeal carcinoma, head and neck lymphoma and squamous cell carcinoma at the primary site. Oral Oncol. (2010) 46:603–6. doi: 10.1016/j.oraloncology.2010.05.004
16. Fujima N, Sakashita T, Homma A, Shimizu Y, Yoshida A, Harada T, et al. Advanced diffusion models in head and neck squamous cell carcinoma patients: goodness of fit, relationships among diffusion parameters and comparison with dynamic contrast-enhanced perfusion. Magn Reson Imaging. (2017) 36:16–23. doi: 10.1016/j.mri.2016.10.024
17. Jiang JX, Tang ZH, Zhong YF, Qiang JW. Diffusion kurtosis imaging for differentiating between the benign and malignant sinonasal lesions. J Magn Reson Imaging. (2017) 45:1446–54. doi: 10.1002/jmri.25500
18. Leifels L, Purz S, Stumpp P, Schob S, Meyer HJ, Kahn T, et al. Associations between 18F-FDG-PET, DWI, and DCE parameters in patients with head and neck squamous cell carcinoma depend on tumor grading. Contrast Media Mol Imaging. (2017) 2017:5369625. doi: 10.1155/2017/5369625
19. Matsushima N, Maeda M, Takamura M, Takeda K. Apparent diffusion coefficients of benign and malignant salivary gland tumors. Comparison to histopathological findings. J Neuroradiol. (2007) 34:183–9. doi: 10.1016/j.neurad.2007.04.002
20. Miracle AC, El-Sayed IH, Glastonbury CM. Diffusion weighted imaging of esthesioneuroblastoma: differentiation from other sinonasal masses. Head Neck. (2019) 41:1161–4. doi: 10.1002/hed.25365
21. Nakahira M, Saito N, Yamaguchi H, Kuba K, Sugasawa M. Use of quantitative diffusion-weighted magnetic resonance imaging to predict human papilloma virus status in patients with oropharyngeal squamous cell carcinoma. Eur Arch Otorhinolaryngol. (2014) 271:1219–25. doi: 10.1007/s00405-013-2641-7
22. Razek AA, Sieza S, Maha B. Assessment of nasal and paranasal sinus masses by diffusion-weighted MR imaging. J Neuroradiol. (2009) 36:206–11. doi: 10.1016/j.neurad.2009.06.001
23. Sasaki M, Eida S, Sumi M, Nakamura T. Apparent diffusion coefficient mapping for sinonasal diseases: differentiation of benign and malignant lesions. AJNR Am J Neuroradiol. (2011) 32:1100–6. doi: 10.3174/ajnr.A2434
24. Shang DS, Ruan LX, Zhou SH, Bao YY, Cheng KJ, Wang QY. Differentiating laryngeal carcinomas from precursor lesions by diffusion-weighted magnetic resonance imaging at 3.0 T: a preliminary study. PLoS ONE. (2013) 8:e68622. doi: 10.1371/journal.pone.0068622
25. Srinivasan A, Dvorak R, Perni K, Rohrer S, Mukherji SK. Differentiation of benign and malignant pathology in the head and neck using 3T apparent diffusion coefficient values: early experience. AJNR Am J Neuroradiol. (2008) 29:40–4. doi: 10.3174/ajnr.A0743
26. Sumi M, Van Cauteren M, Sumi T, Obara M, Ichikawa Y, Nakamura T. Salivary gland tumors: use of intravoxel incoherent motion MR imaging for assessment of diffusion and perfusion for the differentiation of benign from malignant tumors. Radiology. (2012) 263:770–7. doi: 10.1148/radiol.12111248
27. Tao X, Yang G, Wang P, Wu Y, Zhu W, Shi H, et al. The value of combining conventional, diffusion-weighted and dynamic contrast-enhanced MR imaging for the diagnosis of parotid gland tumours. Dentomaxillofac Radiol. (2017) 46:20160434. doi: 10.1259/dmfr.20160434
28. Wang XY, Yan F, Hao H, Wu JX, Chen QH, Xian JF. Improved performance in differentiating benign from malignant sinonasal tumors using diffusion-weighted combined with dynamic contrast-enhanced magnetic resonance imaging. Chin Med J. (2015) 128:586–92. doi: 10.4103/0366-6999.151649
29. White ML, Zhang Y, Robinson RA. Evaluating tumors and tumorlike lesions of the nasal cavity, the paranasal sinuses, and the adjacent skull base with diffusion-weighted MRI. J Comput Assist Tomogr. (2006) 30:490–5. doi: 10.1097/00004728-200605000-00023
30. Yu XP, Hou J, Li FP, Wang H, Hu PS, Bi F, et al. Intravoxel incoherent motion diffusion weighted magnetic resonance imaging for differentiation between nasopharyngeal carcinoma and lymphoma at the primary site. J Comput Assist Tomogr. (2016) 40:413–8. doi: 10.1097/RCT.0000000000000391
31. Yuan Y, Shi H, Tao X. Head and neck paragangliomas: diffusion weighted and dynamic contrast enhanced magnetic resonance imaging characteristics. BMC Med Imaging. (2016) 16:12. doi: 10.1186/s12880-016-0114-3
32. Whiting PF, Rutjes AW, Westwood ME, Mallett S, Deeks JJ, Reitsma JB, et al. QUADAS-2: a revised tool for the quality assessment of diagnostic accuracy studies. Ann Intern Med. (2011) 155:529–36. doi: 10.7326/0003-4819-155-8-201110180-00009
33. Leeflang MM, Deeks JJ, Gatsonis C, Bossuyt PM. Systematic reviews of diagnostic test accuracy. Ann Intern Med. (2008) 149:889–97. doi: 10.7326/0003-4819-149-12-200812160-00008
34. Zamora J, Abraira V, Muriel A, Khan K, Coomarasamy A. Meta-DiSc: a software for meta-analysis of test accuracy data. BMC Med Res Methodol. (2006) 6:31. doi: 10.1186/1471-2288-6-31
35. DerSimonian R, Laird N. Meta-analysis in clinical trials. Control Clin Trials. (1986) 7:177–88. doi: 10.1016/0197-2456(86)90046-2
36. Lingam RK, Khatri P, Hughes J, Singh A. Apparent diffusion coefficients for detection of postoperative middle ear cholesteatoma on non-echo-planar diffusion-weighted images. Radiology. (2013) 269:504–10. doi: 10.1148/radiol.13130065
37. Surov A, Ryl I, Bartel-Friedrich S, Wienke A, Kösling S. Diffusion weighted imaging of nasopharyngeal adenoid hypertrophy. Acta Radiol. (2015) 56:587–91. doi: 10.1177/0284185114534107
38. Sumi M, Ichikawa Y, Nakamura T. Diagnostic ability of apparent diffusion coefficients for lymphomas and carcinomas in the pharynx. Eur Radiol. (2007) 17:2631–37. doi: 10.1007/s00330-007-0588-z
39. Moreau B, Iannessi A, Hoog C, Beaumont H. How reliable are ADC measurements? A phantom and clinical study of cervical lymph nodes. Eur Radiol. (2018) 28:3362–71. doi: 10.1007/s00330-017-5265-2
40. Payabvash S. Quantitative diffusion magnetic resonance imaging in head and neck tumors. Q Imaging Med Surg. (2018) 8:1052–65. doi: 10.21037/qims.2018.10.14
Keywords: head and neck, tumors, apparent diffusion coefficient, ADC, magnetic resonance imaging, MRI
Citation: Surov A, Meyer HJ and Wienke A (2020) Apparent Diffusion Coefficient for Distinguishing Between Malignant and Benign Lesions in the Head and Neck Region: A Systematic Review and Meta-Analysis. Front. Oncol. 9:1362. doi: 10.3389/fonc.2019.01362
Received: 30 July 2019; Accepted: 18 November 2019;
Published: 08 January 2020.
Edited by:
Johannes Kaanders, Radboud University Nijmegen Medical Centre, NetherlandsReviewed by:
Kerem Ozturk, University of Minnesota Twin Cities, United StatesPhilip Touska, Guy's and St Thomas' NHS Foundation Trust, United Kingdom
Copyright © 2020 Surov, Meyer and Wienke. This is an open-access article distributed under the terms of the Creative Commons Attribution License (CC BY). The use, distribution or reproduction in other forums is permitted, provided the original author(s) and the copyright owner(s) are credited and that the original publication in this journal is cited, in accordance with accepted academic practice. No use, distribution or reproduction is permitted which does not comply with these terms.
*Correspondence: Alexey Surov, alexey.surov@medizin.uni-leipzig.de
†These authors have contributed equally to this work