- 1School of Medicine, Southeast University, Nanjing, China
- 2School of Medicine, Nanjing University, Nanjing, China
- 3Department of Neurosurgery, Jinling Hospital, Nanjing, China
- 4School of Nanjing Medicine, Southern Medical University, Guangzhou, China
Background: Pituitary adenomas (PAs) are the most common tumor of the sellar region. PA resection is the preferred treatment for patients with clear indications for surgery. Intraoperative cerebrospinal fluid (iCSF) leakage is a major complication of PA resection surgery. Risk factors for iCSF leakage have been studied previously, but a predictive nomogram has not yet been developed. We constructed a nomogram for preoperative prediction of iCSF leakage in endoscopic pituitary surgery.
Methods: A total of 232 patients who underwent endoscopic PA resection at the Department of Neurosurgery in Jinling Hospital between January of 2018 and October of 2020 were enrolled in this retrospective study. Patients treated by a board-certified neurosurgeon were randomly classified into a training cohort or a validation cohort 1. Patients treated by other qualified neurosurgeons were included in validation cohort 2. A range of demographic, clinical, radiological, and laboratory data were acquired from the medical records. The Least Absolute Shrinkage and Selection Operator (LASSO) algorithm and uni- and multivariate logistic regression were utilized to analyze these features and develop a nomogram model. We used a receiver operating characteristic (ROC) curve and calibration curve to evaluate the predictive performance of the nomogram model.
Results: Variables were comparable between the training cohort and validation cohort 1. Tumor height and albumin were included in the final prediction model. The area under the curve (AUC) of the nomogram model was 0.733, 0.643, and 0.644 in training, validation 1, and validation 2 cohorts, respectively. The calibration curve showed satisfactory homogeneity between the predicted probability and actual observations. Nomogram performance was stable in the subgroup analysis.
Conclusions: Tumor height and albumin were the independent risk factors for iCSF leakage. The prediction model developed in this study is the first nomogram developed as a practical and effective tool to facilitate the preoperative prediction of iCSF leakage in endoscopic pituitary surgery, thus optimizing treatment decisions.
Introduction
Pituitary adenomas (PAs) comprise approximately 15% of primary intracranial neoplasms, and comprehensive management of PAs includes transsphenoidal surgical resection, radiotherapy, and medications (1). Endoscopic transsphenoidal surgery is a highly effective first-line treatment for PAs. However, there are many potential complications in this surgical approach (2). Intraoperative cerebrospinal fluid (iCSF) leakage is one of the major complications and remains a major driver of postoperative CSF leakage and meningitis (3, 4). Moreover, iCSF leakage leads to more thorough and meticulous reconstruction strategies and impacts postoperative management (5).
The preoperative prediction for iCSF leakage is valuable and could allow for improved patient counseling and impact surgical plans. Victor et al. proposed a machine learning (ML) model based on clinical and radiological data which performed well, achieving an AUC of 0.84 and an accuracy of 88% (4). However, applying this ML model in actual practice requires supporting software and platforms, which have not been assessed so far (6).
Nomogram is an easy-to-use predictive tool with user-friendly graphical interfaces, providing visualization of complex statistical predictive models (7). Nomograms have been widely utilized to predict both binary and prognostic outcomes (7). However, there is no nomogram for preoperatively predicting iCSF leakage during endoscopic pituitary surgery in patients with PAs. Therefore, we aimed to construct and validate the first predictive nomogram for preoperatively forecasting iCSF leakage during endoscopic pituitary surgery in patients with PAs.
Methods
Patients Selection and Data Collection
We reviewed the clinical records of PA patients who underwent endoscopic PA resection at the Neurosurgery Department in Jinling Hospital between January of 2018 and October of 2020. The inclusion criteria were as follows: (1) pathologically confirmed PA, (2) patients who underwent PA resection via transsphenoidal endoscopic approach, (3) patients with a clear surgery record regarding iCSF leakage, and (4) patients who had at least one collected variable. The exclusion criteria were: (1) patients without histopathological examination, and (2) patients who had no collected variables. This retrospective study was approved by our institutional research ethics board (2021NZKY-037-01). Informed consent was waived because of the data anonymization before analysis and the retrospective nature of the investigation.
Overall, 96 items were collected in this study. Clinical characteristics collected from the medical records included age, gender, primary-recurrence subtype, treatment history for PAs (medication, surgery, and radiotherapy), and preoperative signs and symptoms (moon face, acromegalia, headache, visual impairment, and visual field defect). Included patients were also diagnosed with clinical subtypes including nonfunctioning, growth hormone (GH) secreting (8, 9), prolactin (PRL) secreting (9), and adrenocorticotropic hormone (ACTH) secreting (10) PAs. We also collected information on radiological features including tumor size (tumor volume and lengths of tumor maximum dimension, height, width, and thickness), the minimum intercarotid distance at the horizontal C4 segment of the internal carotid artery (ICDC4h) (11), Knosp grade, Hardy grade, tumor shape 1 (in sella, ellipsoid, or hourglass signs), tumor shape 2 (lobulated shape), tumor signal intensity (T2-weighted magnetic resonance imaging (MRI) signal intensity compared with white matter), sellar barrier (12) (strong or weak), multiple lesions, optic nerve compression, and pituitary apoplexy. Grades 0–2 and grades 3–4 were classified into noninvasive and invasive classes, respectively, for Knosp grade (13) and Hardy grade for sellar invasion (14). We extracted 74 variables from preoperative laboratory tests, including pituitary hormones, routine blood work, coagulation, renal and hepatic functions, and electrolytes, which were based on preoperative peripheral blood samples (Supplementary Table S2). The outcome, iCSF leakage, was extracted from the surgical records.
Nomogram Development and Validation
First, the patients treated by a board-certified neurosurgeon (CY-M) were randomly divided into a training cohort and a validation cohort 1. Patients treated by other qualified neurosurgeons were included in a validation cohort 2. Then, included variables were submitted to a least absolute shrinkage and selection operator (LASSO) algorithm to filter features missing < 60% of data using the “glmnet” R package (version 4.1). Mean imputation was utilized for missing data only during the LASSO analysis. Missing data were not imputed in the following analyses to simulate model performance in real-world conditions. Finally, independent risk factors associated with iCSF leakage were identified with uni- and multivariate logistic regressions and visualized as a nomogram using the “rms” R package (version 6.1.0). To evaluate the model’s predictive performance, the receiver operating characteristic (ROC) curve and the calibration curve were computed separately with “pROC” (version 1.17.0.1) and “rms” R packages. We also conducted subgroup analysis on validation cohorts to assess the robustness of the model performance according to age, gender, primary-recurrence subtype, clinical subtype, Knosp and Hardy grades, and characteristics included in the final model. The mean values of continuous variables in the validation cohort 1 were used as the cutoff values in the subgroup analysis.
Statistical Analysis
The processes of model construction and validation in the current study were carried out according to “Transparent Reporting of a Multivariable Prediction Model for Individual Prognosis or Diagnosis” (TRIPOD) guidance (Supplementary Table S1) (15). Due to a lack of generally accepted sample size estimation techniques for risk prediction models, we applied the events per variable (EVP) = 10 criteria (16). Based on the criteria, the event number in the training dataset needs to exceed 10 × the number of variables included in the multivariate regression analysis. Although there were 21 variables processed into the regression analysis, we only analyzed all possible combinations up to five variables in the multivariate logistic regression analysis. As there are 51 events in the training cohort, the sample size was sufficient for this research.
Continuous data were expressed as the mean ± standard deviation (SD). We used Student’s t-test to compare two continuous variables and the chi-squared test or Fisher’s exact test for comparisons between categorized variables. Spearman correlation analysis was used to evaluate the relationship between two continuous variables, and the results were visualized with “ggplot” (version 3.3.3) R packages. The R software (version 3.6.0) was applied for these statistical analyses, and p < 0.05 was considered statistically significant.
Results
Baseline Patient Characteristics
A total of 158 eligible patients, treated by a board-certified neurosurgeon (CY-M), were randomly divided into the training cohort (n = 119) and the validation cohort 1 (n = 39). Another 74 patients, treated by other qualified neurosurgeons, were enrolled into validation cohort 2 according to the inclusion and exclusion criteria. Detailed baseline characteristics for the training cohort and the validation cohort 1 were summarized in Supplementary Table S2 and showed homogeneity in these cohorts. The baseline characteristics of samples with and without iCSF leakage (Table 1 and Supplementary Table S3) revealed significant differences in lengths of tumor height and thickness, tumor volume, triiodothyronine (T3), tumor shape 1, tumor shape 2, and sellar barrier.
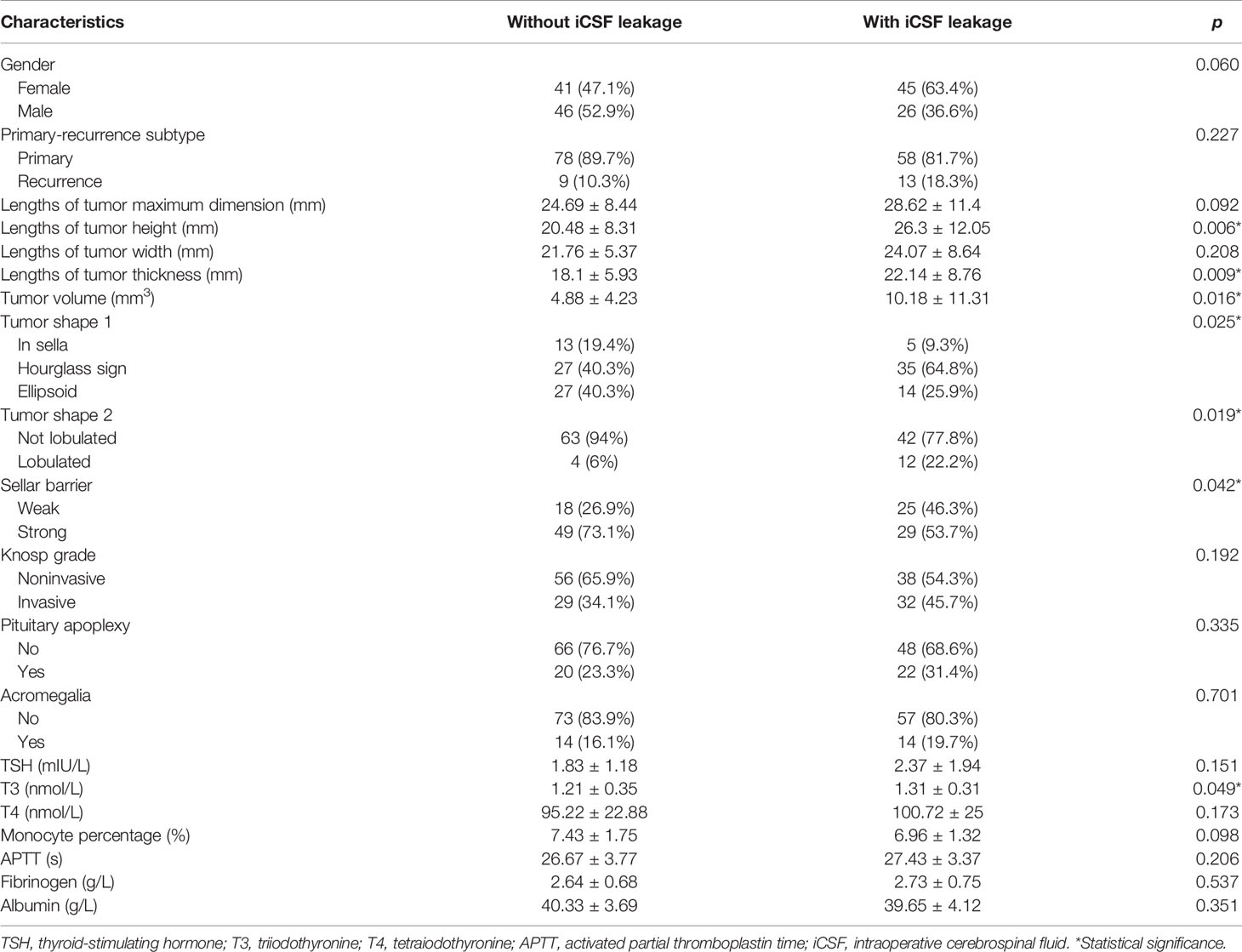
Table 1 Important characteristics of patients in the without iCSF leakage group and in the with iCSF leakage group.
Filtering Process for Collected Variables
In a univariate logistic regression analysis, the Hardy grade for suprasellar extension, tumor shape 1, Knosp grade, tumor shape 2, sellar barrier, lengths of tumor maximum dimension, height, width and thickness, and tumor volume were predictive factors with p < 0.05 (Supplementary Table S4). Among these variables, only the sellar barrier was a protective factor for iCSF leakage, whereas the other factors were all risk factors. We then conducted the LASSO analysis, and the following 15 features were screened out of the original 87 (Supplementary Table S5 and Figure 1): Hardy grade for suprasellar extension, gender, acromegalia, pituitary apoplexy, tumor shape 2, lengths of tumor height and thickness, tumor volume, thyroid-stimulating hormone (TSH), T3, tetraiodothyronine (T4), albumin, monocyte percentage, activated partial thromboplastin time (APTT), and fibrinogen.
Independent Predictors for iCSF leakage
After the filtering process, a total of 21 variables were screened out by univariate analysis and LASSO analysis. These variables were further analyzed in a multivariable logistic regression analysis. We analyzed all possible combinations of up to five variables in the multivariate logistic regression analysis. Finally, lengths of tumor height (odds ratio (OR), 95% Confidence Interval (CI): 1.1141, 1.0485–1.1839, p = 0.0005) and albumin (OR, 95% CI: 0.8698, 0.7576–0.9986, p = 0.0477) were incorporated into the multivariate model, as shown in Table 2.
Development and Validation of the Nomogram
A nomogram was constructed based on the multivariate model (Figure 2). For each patient, users need to draw virtual vertical lines from each variable to the “Points” axis, identify the points for each variable, and sum these scores to calculate the total point. Then, users should compare the total point with the probability scale to evaluate the probability of iCSF leakage. The areas under the curve (AUCs) of the training cohort, validation cohort 1, and validation cohort 2 were 0.733, 0.643, and 0.644, respectively (Figures 3A, C, D). A calibration curve was generated showing adequate prediction accuracy using this model (Figure 3B). Subgroup analysis revealed the nomogram had stable predictive performance in validation cohort 1 (Supplementary Figure S1 and Table 3) and validation cohort 2 (Supplementary Figure S2 and Table 3).
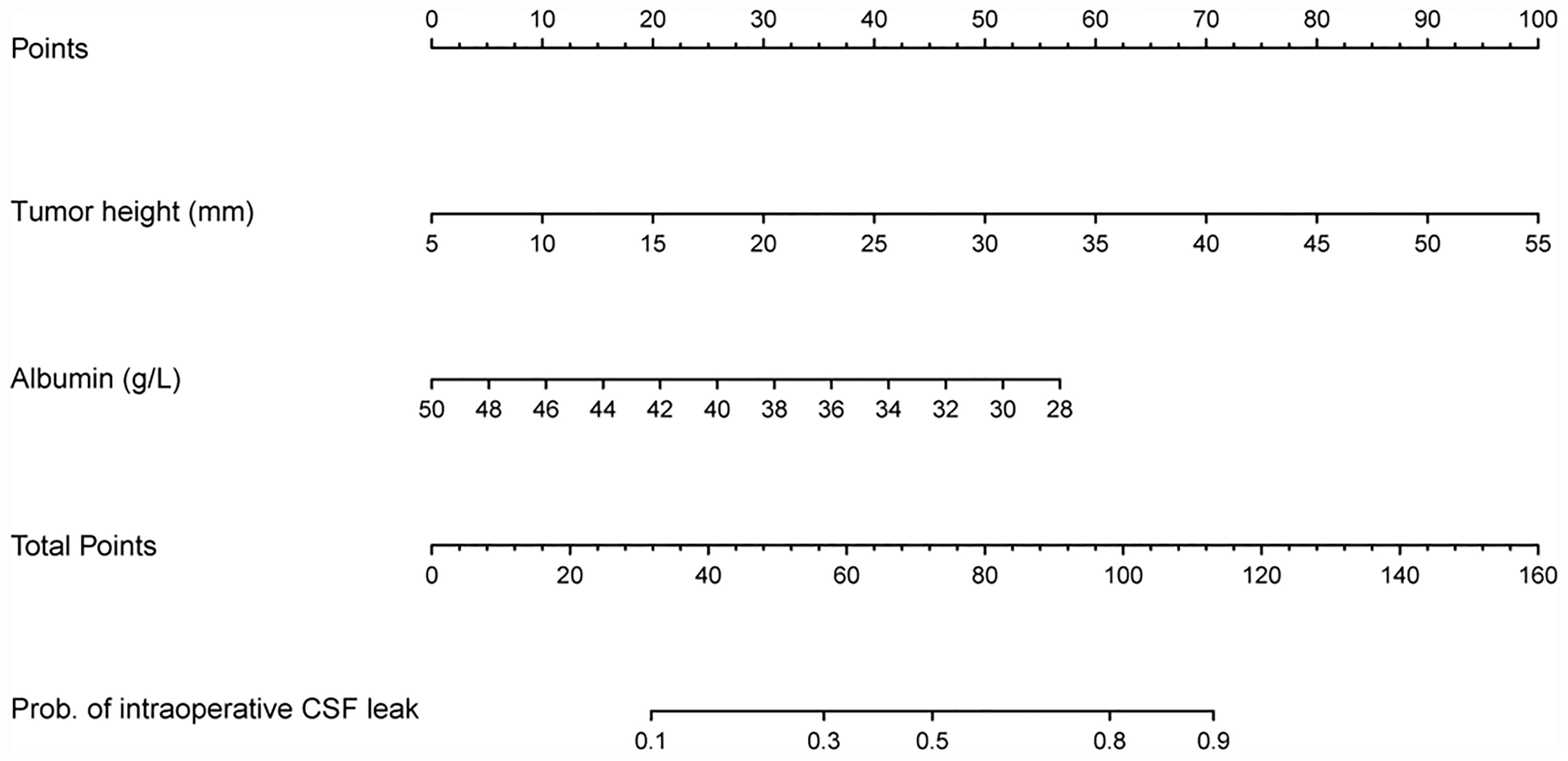
Figure 2 Nomogram for preoperatively predicting the proportion of iCSF leakage during endoscopic pituitary surgery in patients with pituitary tumor.
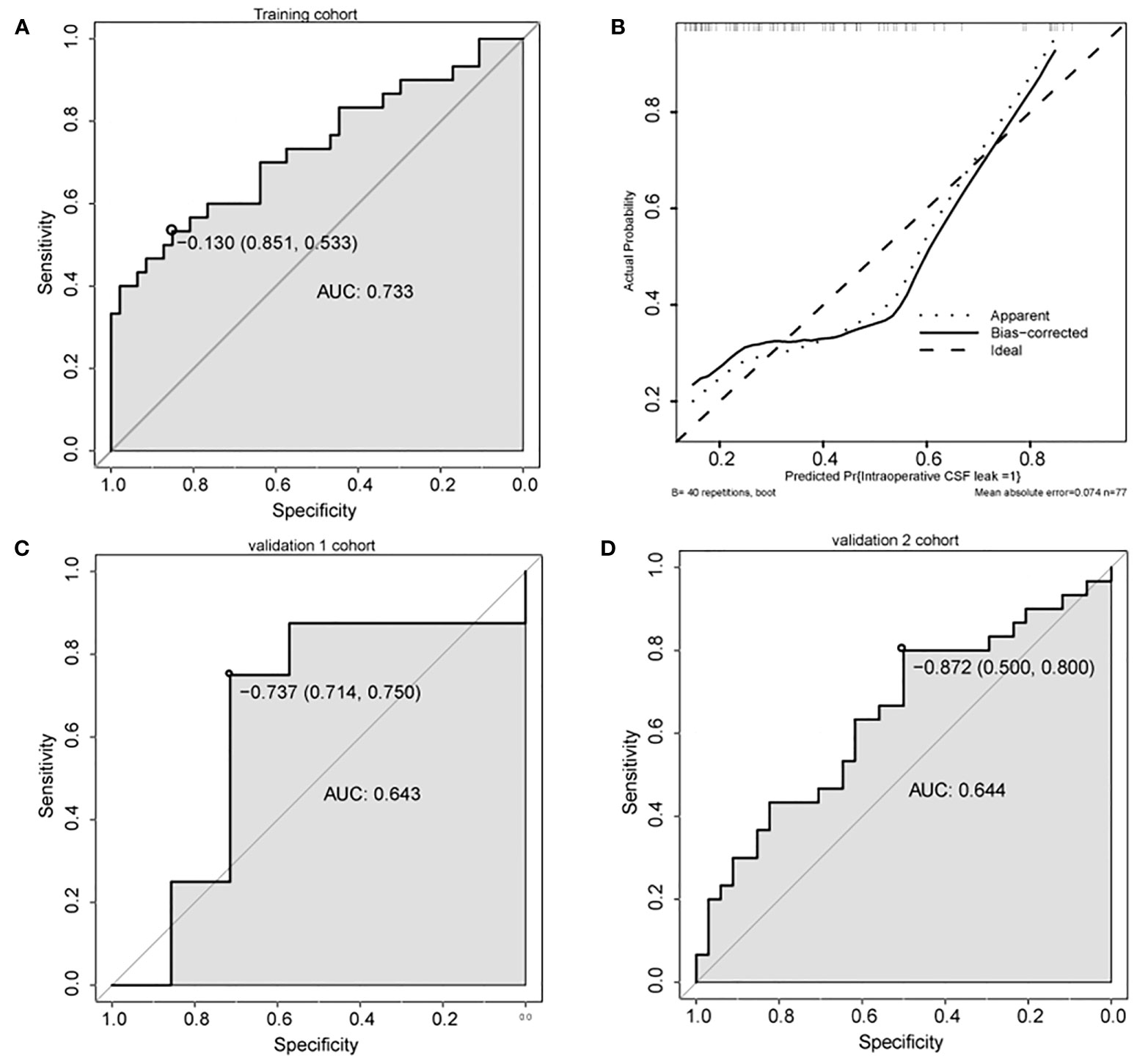
Figure 3 Predictive performance of the nomogram. (A, C, D) ROC analysis of nomogram in training cohort (A), validation cohort 1 (C), and validation cohort 2 (D). (B) Calibration plots of the nomogram. AUC, area under the curve.
Discussion
PAs account for approximately 15% of primary intracranial and central nervous system tumors (1). Endoscopic transsphenoidal surgery is the preferred treatment for patients with clear indications for surgery. iCSF leakage is one of the major complications of PA resection surgery (3, 4). Preoperative prediction of iCSF leakage could assist neurosurgeons in developing individualized surgery strategies for patients with PAs. However, no user-friendly predictive tool for preoperatively predicting iCSF leakage is available. In the current research, lengths of tumor height and albumin were identified as independent predictive factors for iCSF leakage. These two variables were incorporated into a nomogram model to preoperatively calculate the probability of iCSF leakage tailored to individual patients.
A series of risk factors for iCSF leakage have been identified. Karnezis et al. found that in endoscopic sellar surgery, craniopharyngioma, mild liver disease, and extension into the anterior cranial fossa are preoperative risk factors for iCSF leakage (17). Patel et al. revealed that body mass index, suprasellar extension, hydrocephalus, and craniopharyngioma are significant independent predictors for iCSF leakage in endoscopic transsphenoidal sellar surgery (18). Zhou et al. discovered that tumor consistency (p = 0.001; OR = 2.379) and tumor size (p = 0.026; OR = 1.032) were independent predictors for iCSF leakage in endoscopic transsphenoidal PA surgery (19). Wang et al. found that, in endoscopic transsphenoidal PA surgery, tumor diameter was the independent predictor for iCSF leakage (p = 0.004, OR = 1. 090) (20). Liu et al. proposed that applying intraoperative lumbar drainage (LD) significantly decreased the iCSF leakage rate (10.1% vs. 31.4%, p < 0.001) in endoscopic transsphenoidal surgery of pituitary macroadenomas (21).
The protective role of LD was supported by a meta-analysis conducted by Tan et al. (22). Xue et al. discovered that tumors with lobular or irregular contours and gonadotrophic-positive staining increased the risk of iCSF leakage in endoscopic transsphenoidal PA surgery (23). We also found that tumors with a lobulated shape are a risk factor for iCSF leakage in univariate logistic regression analysis (p = 0.0383; OR = 3.712; Supplementary Table S4). However, this variable was filtered out in the multivariate analysis. Villalonga et al. classified the sellar barrier into strong, mixed, and weak, based on the relationship between the pituitary gland, tumor, and CSF (12). They found that a strong sellar barrier significantly reduced the iCSF leakage rate (RR = 0.08; 95% CI 0.03-0.19; p < 0.0001), while a weak sellar barrier was associated with higher rates of iCSF leakage (RR = 8.54; 95% CI 5.4-13.5; p < 0.0001).
We also investigated the relationship between the sellar barrier and iCSF leakage. However, we only separated the sellar barrier into two types: strong or weak. Strong denoted normal pituitary tissue exists between PAs and CSF, which included strong and mixed sellar barriers, as described by Villalonga et al. Weak is the weak sellar barrier described by Villalonga et al. The protective effect of a strong sellar barrier for iCSF leakage was detected in the current research in a univariate logistic regression analysis (p = 0.0427; OR = 0.413; Supplementary Table S4). However, this effect disappeared in the multivariate logistic regression analysis.
Victor et al. proposed the only ML model for predicting iCSF leakage during endoscopic transsphenoidal surgery for PAs (4). Although they analyzed a series of clinical and radiological variables, no factor showed significant predictive value for iCSF leakage in traditional uni- or multivariate analyses. However, with the same variables, the ML model they constructed had an AUC of 0.84 in the validation dataset. In our work, we witnessed a range of variables with significant predictive effect in the comparison between groups with and without iCSF leakage [lengths of tumor height and thickness, tumor volume, T3, tumor shape 1, tumor shape 2, and sellar barrier (Table 1)] and univariate logistic regression analysis [Hardy grade for suprasellar extension, tumor shape 1, Knosp grade, tumor shape 2, sellar barrier, lengths of tumor maximum dimension, height, width and thickness, and tumor volume (Supplementary Table S4)] We combined these variables with other variables screened out from the LASSO analysis to conduct a multivariable logistic regression analysis.
The final model included two independent predictors for iCSF leakage, which were lengths of tumor height (OR, 95% CI: 1.1141, 1.0485–1.1839, p = 0.0005) and albumin (OR, 95% CI: 0.8698, 0.7576–0.9986, p = 0.0477). Compared with the ML model constructed by Victor et al., our model had relatively lower AUCs (0.643 and 0.644 for validation cohorts 1 and 2, respectively) but better interpretability. Clinicians can distinguish the risk predictor from the protective predictor based on the OR value. The application of the ML model remains a challenge in clinical practice because there is no easy-to-use platform for prediction based on the ML model (6). After the multivariate regression model was visualized into a nomogram, our model was clinically useful and easy to incorporate into clinical practice.
As mentioned above, some research has found a significant relationship between iCSF leakage and preoperative factors, including suprasellar extension (18), tumor size (19), tumor diameter (20), lobular tumors (23), and sellar barrier (12). These factors also showed significant predictive value in our univariate analysis (Supplementary Table S4). However, all of them were filtered out in the multivariate regression analysis, and only lengths of tumor height remained in the final model, which seems to be related to these filtered variables. We conducted correlation analyses between length of tumor height and some of these filtered variables (Supplementary Table S6 and Supplementary Figure S3). The results showed that length of tumor height was significantly correlated with tumor volume (R = 0.87; p < 0.01; Supplementary Figure S3B), length of tumor maximum dimension (R = 0.93; p < 0.01; Supplementary Figure S3A), length of tumor width (R = 0.83; p < 0.01; Supplementary Figure S3D), and length of tumor thickness (R = 0.88; p < 0.01; Supplementary Figure S3C). We also discovered that length of tumor height differed significantly between groups according to the Knosp grade (p < 0.001; Supplementary Table S6), Hardy grade for suprasellar extension (p < 0.001; Supplementary Table S6), tumor shape 1 (p < 0.001; Supplementary Table S6), tumor shape 2 (p = 0.017; Supplementary Table S6), and sellar barrier (p = 0.002; Supplementary Table S6). Based on these results, we suggest that there may be collinearity between length of tumor height and these factors.
We found that albumin was an independent protective predictor for iCSF leakage (OR, 95% CI: 0.8698, 0.7576–0.9986, p = 0.0477; Table 2). This result was supported by Karnezis et al. Their research examined 1,108 people with pituitary adenomas and 53 people with craniopharyngiomas who underwent endoscopic sellar surgery, and mild liver disease was revealed to be a risk factor for iCSF leakage (OR=3.636, p =0.046), which usually lead to low albumin levels. Based on the review by Wang et al., lower albumin levels function as a biomarker for immune dysfunction (24), and Zhang et al. discovered that invasive PAs showed higher infiltration of M2-like tumor-associated macrophages (25), which have an anti-inflammatory phenotype (26). We suggest that lower albumin levels may act as a biomarker for an anti-inflammatory immune environment in PAs, which is suggestive of invasive tumor behavior. However, this hypothesis needs to be verified in further research.
There were several limitations in this study. First, because of the lack of thyrotropinoma and gonadotropinoma patients in the center, we only included nonfunctioning, GH secreting, PRL secreting, and ACTH secreting PAs. This may have caused potential selection bias, which is unavoidable in a single-institution retrospective study. Therefore, further studies are needed to comprehensively evaluate the nomogram model. Second, the model’s predictive performance was unsatisfactory, however, this is the first nomogram for preoperative prediction of iCSF leakage in endoscopic pituitary surgery for PA patients. Its predictive performance in some subgroups was relatively sufficient, and we recommend that clinicians apply this model in carefully selected patients. For example, patients that are male (AUCs = 0.844, 0.705 for validation cohort 1 and 2, respectively), with GH secreting PAs (AUCs = 0.750, 0.714 for validation cohort 1 and 2, respectively), and with an invasive Knosp grade (AUCs = 0.800, 0.789 for validation cohort 1 and 2, respectively) are appropriate for this nomogram. Third, some variables extracted in this study were missing data. However, all of the variables processed into the LASSO and univariate regression analyses had less than 60% of their data missing. Furthermore, only 35.3% of data in the final model was missing, which is acceptable and provides an adequate sample size for the multivariate analysis. Finally, because the current work focused on all patients with PAs treated with endoscopic pituitary surgery, nomograms for particular subgroup populations were not computed in this research. Additional research is warranted to calculate the predictive nomogram model for iCSF leakage for various subgroup populations.
Conclusions
This study revealed that tumor height and albumin were independent risk factors associated with iCSF leakage. Albumin is found for the first time to be an independent predictor for iCSF leakage. This study developed and validated a feasible and stable novel nomogram for preoperative prediction of iCSF leakage, which could assist neurosurgeons in developing individualized operation plans for patients with PAs. This may optimize treatment results.
Data Availability Statement
The raw data of this article is available from the corresponding author upon reasonable request.
Ethics Statement
The studies involving human participants were reviewed and approved by Research ethics board of Jinling Hospital. The ethics committee waived the requirement of written informed consent for participation.
Author Contributions
CM conceived and designed the investigation. XC analyzed the data and drafted the manuscript. JZ, JY, CT, FY, and ZC conducted statistical analyses. All authors have read and approved the manuscript.
Conflict of Interest
The authors declare that the research was conducted in the absence of any commercial or financial relationships that could be construed as a potential conflict of interest.
Publisher’s Note
All claims expressed in this article are solely those of the authors and do not necessarily represent those of their affiliated organizations, or those of the publisher, the editors and the reviewers. Any product that may be evaluated in this article, or claim that may be made by its manufacturer, is not guaranteed or endorsed by the publisher.
Acknowledgments
We thank Department of Neurosurgery, Jinling Hospital for help during the research. And we express our special thanks to Professor Yuxiu Liu who gave us guidance about statistical analysis. Also, XC specially thank Sodagreen for their encouraging music.
Supplementary Material
The Supplementary Material for this article can be found online at: https://www.frontiersin.org/articles/10.3389/fonc.2021.719494/full#supplementary-material
Supplementary Figure 1 | Predictive performance for the nomogram in the subgroup analysis from validation cohort 1. (A, B) ROC analysis in the subgroup according to age (A for >51 years and B for ≤51 years); (C, D) ROC analysis in the subgroup according to gender (C for female and D for male); (E, F) ROC analysis in the subgroup according to clinical subtypes (E for nonfunctioning and F for GH secreting); (G, H) ROC analysis in the subgroup according to primary-recurrence subtypes (G for primary and H for recurrence subtypes); (I) ROC analysis in the subgroup according to the maximum dimension (I for macroadenoma); (J, K) ROC analysis in the subgroup according to Knosp grade (J for noninvasive and K for invasive); (L, M) ROC analysis in the subgroup according to Hardy grade (L for noninvasive and M for invasive); (N, O) ROC analysis in the subgroup according to length of tumor height (N for >23.6 mm and O for ≤23.6 mm); (P, Q) ROC analysis in the subgroup according to albumin (P for >41g/L and Q for ≤41g/L).
Supplementary Figure 2 | Predictive performance for the nomogram in the subgroup analysis from validation cohort 2. (A, B) ROC analysis in the subgroup according to age (A for >51 years and B for ≤51 years); (C, D) ROC analysis in the subgroup according to gender (C for female and D for male); (E–G) ROC analysis in the subgroup according to clinical subtypes (E for nonfunctioning, F for PRL secreting and G for GH secreting); (H, I) ROC analysis in the subgroup according to primary-recurrence subtypes (H for primary and I for recurrence subtypes); (J–L) ROC analysis in the subgroup according to the maximum dimension (J for microadenoma, K for macroadenoma and L for giant adenoma); (M, N) ROC analysis in the subgroup according to Knosp grade (M for noninvasive and N for invasive); (O, P) ROC analysis in the subgroup according to Hardy grade (O for noninvasive and P for invasive); (Q, R) ROC analysis in the subgroup according to length of tumor height (Q for >23.6 mm and R for ≤23.6 mm); (S, T) ROC analysis in the subgroup according to albumin (S for >41g/L and T for ≤41g/L).
Supplementary Figure 3 | Correlation analysis. Correlation between length of tumor height and length of tumor maximum dimension (A), tumor volume (B), length of tumor thickness (C), and length of tumor width (D), respectively.
References
1. Melmed S. Pituitary-Tumor Endocrinopathies. N Engl J Med (2020) 382:937–50. doi: 10.1056/NEJMra1810772
2. van Furth WR, de Vries F, Lobatto DJ, Kleijwegt MC, Schutte PJ, Pereira AM, et al. Endoscopic Surgery for Pituitary Tumors. Endocrinol Metab Clin North Am (2020) 49:487–503. doi: 10.1016/j.ecl.2020.05.011
3. Ivan ME, Iorgulescu JB, El-Sayed I, McDermott MW, Parsa AT, Pletcher SD, et al. Risk Factors for Postoperative Cerebrospinal Fluid Leak and Meningitis After Expanded Endoscopic Endonasal Surgery. J Clin Neurosci (2015) 22:48–54. doi: 10.1016/j.jocn.2014.08.009
4. Staartjes VE, Zattra CM, Akeret K, Maldaner N, Muscas G, Bas van Niftrik CH, et al. Neural Network-Based Identification of Patients at High Risk for Intraoperative Cerebrospinal Fluid Leaks in Endoscopic Pituitary Surgery. J Neurosurg (2019) 133:1–7. doi: 10.3171/2019.4.Jns19477
5. Jakimovski D, Bonci G, Attia M, Shao H, Hofstetter C, Tsiouris AJ, et al. Incidence and Significance of Intraoperative Cerebrospinal Fluid Leak in Endoscopic Pituitary Surgery Using Intrathecal Fluorescein. World Neurosurg (2014) 82:e513–23. doi: 10.1016/j.wneu.2013.06.005
6. Peiffer-Smadja N, Dellière S, Rodriguez C, Birgand G, Lescure FX, Fourati S, et al. Machine Learning in the Clinical Microbiology Laboratory: Has the Time Come for Routine Practice? Clin Microbiol Infect (2020) 26:1300–9. doi: 10.1016/j.cmi.2020.02.006
7. Iasonos A, Schrag D, Raj GV, Panageas KS. How to Build and Interpret a Nomogram for Cancer Prognosis. J Clin Oncol (2008) 26:1364–70. doi: 10.1200/jco.2007.12.9791
8. de Pablos-Velasco P, Venegas EM, Álvarez Escolá C, Fajardo C, de Miguel P, González N, et al. Diagnosis, Treatment and Follow-Up of Patients With Acromegaly in a Clinical Practice Setting in Spain: The ACROPRAXIS Program Delphi Survey. Pituitary (2020) 23:129–39. doi: 10.1007/s11102-019-01012-3
9. Gada JV, Sanamandra P, Barasara SA, Chauhan YV, Bhagwat NM. Current Status of Diagnosis and Management of Functioning Pituitary Tumors: Part Ii. Neurol India (2020) 68:S20–s7. doi: 10.4103/0028-3886.287672
10. Thakkar K, Sarathi V, Shah NS. Current Status of Diagnosis and Management for Functioning Pituitary Tumors: Part I. Neurol India (2020) 68:S13–s9. doi: 10.4103/0028-3886.287680
11. Bouthillier A, van Loveren HR, Keller JT. Segments of the Internal Carotid Artery: A New Classification. Neurosurgery (1996) 38:425–32; discussion 32-3. doi: 10.1097/00006123-199603000-00001
12. Villalonga JF, Solari D, Cavallo LM, Cappabianca P, Prevedello DM, Carrau R, et al. The Sellar Barrier on Preoperative Imaging Predicts Intraoperative Cerebrospinal Fluid Leak: A Prospective Multicenter Cohort Study. Pituitary (2021) 24:27–37. doi: 10.1007/s11102-020-01082-8
13. Knosp E, Steiner E, Kitz K, Matula C. Pituitary Adenomas With Invasion of the Cavernous Sinus Space: A Magnetic Resonance Imaging Classification Compared With Surgical Findings. Neurosurgery (1993) 33:610–7; discussion 7-8. doi: 10.1227/00006123-199310000-00008
14. Hardy J, Somma M. “Surgical Treatment by Transsphenoidal Microsurgical Removal of the Pituitary Adenoma”. In: Colins W, Tindall G, editors. Clinical Management of Pituitary Disorders. New York: Raven (1979). p. 209–17.
15. Moons KG, Altman DG, Reitsma JB, Ioannidis JP, Macaskill P, Steyerberg EW, et al. Transparent Reporting of a Multivariable Prediction Model for Individual Prognosis or Diagnosis (TRIPOD): Explanation and Elaboration. Ann Intern Med (2015) 162:W1–73. doi: 10.7326/m14-0698
16. Peduzzi P, Concato J, Kemper E, Holford TR, Feinstein AR. A Simulation Study of the Number of Events Per Variable in Logistic Regression Analysis. J Clin Epidemiol (1996) 49:1373–9. doi: 10.1016/s0895-4356(96)00236-3
17. Karnezis TT, Baker AB, Soler ZM, Wise SK, Rereddy SK, Patel ZM, et al. Factors Impacting Cerebrospinal Fluid Leak Rates in Endoscopic Sellar Surgery. Int Forum Allergy Rhinol (2016) 6:1117–25. doi: 10.1002/alr.21783
18. Patel PN, Stafford AM, Patrinely JR, Smith DK, Turner JH, Russell PT, et al. Risk Factors for Intraoperative and Postoperative Cerebrospinal Fluid Leaks in Endoscopic Transsphenoidal Sellar Surgery. Otolaryngol Head Neck Surg (2018) 158:952–60. doi: 10.1177/0194599818756272
19. Zhou Q, Yang Z, Wang X, Wang Z, Zhao C, Zhang S, et al. Risk Factors and Management of Intraoperative Cerebrospinal Fluid Leaks in Endoscopic Treatment of Pituitary Adenoma: Analysis of 492 Patients. World Neurosurg (2017) 101:390–5. doi: 10.1016/j.wneu.2017.01.119
20. Wang M, Peng Y, Jiang Y. Risk Factors of Cerebrospinal Fluid Leak in Endoscopic Endonasal Transsphenoidal Pituitary Surgery. Chin J Neurosurgery (2018) 34:277–81. doi: 10.3760/cma.j.issn.1001-2346.2018.03.013
21. Liu B, Wang Y, Zheng T, Liu S, Lv W, Lu D, et al. Effect of Intraoperative Lumbar Drainage on Gross Total Resection and Cerebrospinal Fluid Leak Rates in Endoscopic Transsphenoidal Surgery of Pituitary Macroadenomas. World Neurosurg (2020) 135:e629–e39. doi: 10.1016/j.wneu.2019.12.100
22. Tan J, Song R, Huan R, Huang N, Chen J. Intraoperative Lumbar Drainage can Prevent Cerebrospinal Fluid Leakage During Transsphenoidal Surgery for Pituitary Adenomas: A Systematic Review and Meta-Analysis. BMC Neurol (2020) 20:303. doi: 10.1186/s12883-020-01877-z
23. Xue H, Wang X, Yang Z, Bi Z, Liu P. Risk Factors and Outcomes of Cerebrospinal Fluid Leak Related to Endoscopic Pituitary Adenoma Surgery. Br J Neurosurg (2020) 34:447–52. doi: 10.1080/02688697.2020.1754336
24. Wang J, Maxwell CA, Yu F. Biological Processes and Biomarkers Related to Frailty in Older Adults: A State-Of-the-Science Literature Review. Biol Res Nurs (2019) 21:80–106. doi: 10.1177/1099800418798047
25. Zhang A, Xu Y, Xu H, Ren J, Meng T, Ni Y, et al. Lactate-Induced M2 Polarization of Tumor-Associated Macrophages Promotes the Invasion of Pituitary Adenoma by Secreting CCL17. Theranostics (2021) 11:3839–52. doi: 10.7150/thno.53749
Keywords: albumin, cerebrospinal fluid leakage, endoscopic pituitary surgery, nomogram, pituitary tumors, tumor height
Citation: Cai X, Zhu J, Yang J, Tang C, Yuan F, Cong Z and Ma C (2021) Development and Validation of Nomogram to Preoperatively Predict Intraoperative Cerebrospinal Fluid Leakage in Endoscopic Pituitary Surgery: A Retrospective Cohort Study. Front. Oncol. 11:719494. doi: 10.3389/fonc.2021.719494
Received: 02 June 2021; Accepted: 08 October 2021;
Published: 26 October 2021.
Edited by:
Alireza Mansouri, The Pennsylvania State University (PSU), United StatesReviewed by:
Lei Cao, Capital Medical University, ChinaJan Egger, Graz University of Technology, Austria
Copyright © 2021 Cai, Zhu, Yang, Tang, Yuan, Cong and Ma. This is an open-access article distributed under the terms of the Creative Commons Attribution License (CC BY). The use, distribution or reproduction in other forums is permitted, provided the original author(s) and the copyright owner(s) are credited and that the original publication in this journal is cited, in accordance with accepted academic practice. No use, distribution or reproduction is permitted which does not comply with these terms.
*Correspondence: Chiyuan Ma, machiyuan_nju@126.com