- 1Genomic Epidemiology Group, German Cancer Research Center (DKFZ), Heidelberg, Germany
- 2Medical Faculty Heidelberg, University of Heidelberg, Heidelberg, Germany
- 3Department of Biology, University of Pisa, Pisa, Italy
- 4Division of Gastroenterology and Research Laboratory, Fondazione Istituto di Ricovero e Cura a Carattere Scientifico (IRCCS) Casa Sollievo della Sofferenza, San Giovanni Rotondo, Italy
- 5Department of Basic Medical Sciences, Laboratory of Biology, Medical School, National and Kapodistrian University of Athens, Athens, Greece
- 6Pancreato-Biliary Endoscopy and Endosonography Division, Pancreas Translational and Clinical Research Center, San Raffaele Scientific Institute Istituto di Ricovero e Cura a Carattere Scientifico (IRCCS), Milan, Italy
- 7Department of Surgery, Medical School, University of Pécs, Pécs, Hungary
- 8Oncological Department, Oncological Unit of Massa Carrara, Azienda Unità Sanitaria Locale (USL) Toscana Nord Ovest, Carrara, Italy
- 9General Surgery, Department of Translational Research and New Technologies in Medicine and Surgery, University of Pisa, Pisa, Italy
- 10Endoscopic Surgery Department, Hippocratio General Hospital of Athens, Athens, Greece
- 11Department of Molecular and Clinical Cancer Medicine, University of Liverpool, Liverpool, United Kingdom
- 12Department of General, Visceral and Thoracic Surgery, University Medical Center Hamburg-Eppendorf, Hamburg, Germany
- 13Department of Gastroenterology, Institute for Digestive Research, Medical Academy, Lithuanian University of Health Sciences, Kaunas, Lithuania
- 14Department of Oncology, Faculty of Medicine and Dentistry, Palacky University Olomouc and University Hospital Olomouc, Olomouc, Czechia
- 15Department for Determinants of Chronic Diseases (DCD), National Institute for Public Health and the Environment (RIVM), Bilthoven, Netherlands
- 16Department of Molecular Biology of Cancer, Institute of Experimental Medicine of the Czech Academy of Sciences, Prague, Czechia
- 17Faculty of Medicine and Biomedical Center in Pilsen, Charles University, Pilsen, Czechia
- 18First Faculty of Medicine, Institute of Biology and Medical Genetics, Prague, Czechia
- 19Division of Clinical Epidemiology and Aging Research, German Cancer Research Center (DKFZ), Heidelberg, Germany
- 20Division of Preventive Oncology, German Cancer Research Center (DKFZ) and National Center for Tumor Diseases (NCT), Heidelberg, Germany
- 21German Cancer Consortium (DKTK), German Cancer Research Center (DKFZ), Heidelberg, Germany
- 22Department of General Surgery, University of Heidelberg, Heidelberg, Germany
- 23Department of Internal Medicine, San Carlo Hospital, Potenza, Italy
- 24Department of General and Pancreatic Surgery, The Pancreas Institute, University and Hospital Trust of Verona, Verona, Italy
- 25Institute for Translational Medicine, Medical School, University of Pécs, Pécs, Hungary
- 26Centre for Translational Medicine, Department of Medicine, University of Szeged, Szeged, Hungary
- 27Laboratory for Applied Science and Technology in Health, Carlos Chagas Institute, Curitiba, Brazil
- 28First Department of Medicine, University of Szeged, Szeged, Hungary
- 29Department of Surgery-Dipartimento di Scienze Chirurgiche Oncologiche e Gastroenterologiche (DiSCOG), Padua University Hospital, Padua, Italy
- 30Department of Hematology, Transplantation and Internal Medicine, Medical University of Warsaw, Warsaw, Poland
- 31Department of Radiology and Oncology, Institute of Cancer of São Paulo (ICESP), São Paulo, Brazil
- 32Faculty of Medicine, University of São Paulo, São Paulo, Brazil
- 33Department of Surgery, Faculty Hospital Kralovske Vinohrady and Third Faculty of Medicine, Charles University, Prague, Czechia
- 34Digestive and Liver Disease Unit, Sant’ Andrea Hospital, Rome, Italy
- 35Faculty of Medicine and Psychology, Sapienza University of Rome, Rome, Italy
- 36Blood Transfusion Service, Children’s Hospital, Azienda Ospedaliero-Universitaria Meyer, Florence, Italy
- 37Pándy Kálmán Hospital of Békés County, Gyula, Hungary
- 38Institute of Molecular and Translational Medicine, Faculty of Medicine and Dentistry, Palacky University Olomouc, Olomouc, Czechia
- 39Biomedical Center, Faculty of Medicine in Pilsen, Charles University, Pilsen, Czechia
- 40Division of Experimental Oncology, Gastroenterology and Gastrointestinal Endoscopy Unit, Vita-Salute San Raffaele University, Istituto di Ricovero e Cura a Carattere Scientifico (IRCCS) San Raffaele Scientific Institute, Milan, Italy
- 41First Propaedeutic University Surgery Clinic, Hippocratio General Hospital, Medical School, National and Kapodistrian University of Athens, Athens, Greece
- 42Department of Medical Oncology, Cancer Center Amsterdam, Amsterdam University Medical Centers, University of Amsterdam, Amsterdam, Netherlands
- 43Department of Medicine (DIMED), Padua University Hospital, Padua, Italy
- 44Department of Surgery, University Hospital Brno Bohunice, Faculty of Medicine, Masaryk University, Brno, Czechia
- 45Department of Surgery I, Faculty of Medicine and Dentistry, Palacky University Olomouc and University Hospital Olomouc, Olomouc, Czechia
- 46Division of Gastroenterology and Digestive Endoscopy, Department of Gastroenterology, Humanitas Clinical and Research Center Istituto di Ricovero e Cura a Carattere Scientifico (IRCCS), Milan, Italy
- 47Department of Surgery, Cancer Center Amsterdam, Amsterdam University Medical Centers, University of Amsterdam, Amsterdam, Netherlands
- 48Applied Research on Cancer (ARC)-Net Research Center, University and Hospital Trust of Verona, Verona, Italy
- 49Division of General and Transplant Surgery, Pisa University Hospital, Pisa, Italy
- 50Department of Digestive Tract Diseases, Medical University of Lodz, Lodz, Poland
- 51Centre for Surgical Oncology, Medias Klinikum Burghausen, Burghausen, Germany
Although 21 pancreatic cancer susceptibility loci have been identified in individuals of European ancestry through genome-wide association studies (GWASs), much of the heritability of pancreatic cancer risk remains unidentified. A recessive genetic model could be a powerful tool for identifying additional risk variants. To discover recessively inherited pancreatic cancer risk loci, we performed a re-analysis of the largest pancreatic cancer GWAS, the Pancreatic Cancer Cohort Consortium (PanScan) and the Pancreatic Cancer Case-Control Consortium (PanC4), including 8,769 cases and 7,055 controls of European ancestry. Six single nucleotide polymorphisms (SNPs) showed associations with pancreatic cancer risk according to a recessive model of inheritance. We replicated these variants in 3,212 cases and 3,470 controls collected from the PANcreatic Disease ReseArch (PANDoRA) consortium. The results of the meta-analyses confirmed that rs4626538 (7q32.2), rs7008921 (8p23.2) and rs147904962 (17q21.31) showed specific recessive effects (p<10−5) compared with the additive effects (p>10−3), although none of the six SNPs reached the conventional threshold for genome-wide significance (p < 5×10−8). Additional bioinformatic analysis explored the functional annotations of the SNPs and indicated a possible relationship between rs36018702 and expression of the BCL2L11 and BUB1 genes, which are known to be involved in pancreatic biology. Our findings, while not conclusive, indicate the importance of considering non-additive genetic models when performing GWAS analysis. The SNPs associated with pancreatic cancer in this study could be used for further meta-analysis for recessive association of SNPs and pancreatic cancer risk and might be a useful addiction to improve the performance of polygenic risk scores.
Introduction
Pancreatic cancer ranks fourth for cancer-related deaths in western countries and is projected to become the second by 2030 (1, 2). It is a very deadly disease with the mortality rate closely approaching to the incidence rate. The median survival is less than 18 months, and the 5-year survival rate remains as low as 3 ~ 15% (3–5). The poor prognosis is mainly due to the late onset of symptoms, diagnosis at an advanced stage and subsequent rapid progression. A comprehensive identification of the risk factors can be instrumental to a better understanding of the disease etiology and to the development of methods for risk stratification, that in turn could facilitate early detection, which at the moment remains elusive.
Genetic factors play an important role in the etiology of pancreatic cancer (6). Genome-wide association studies (GWAS) have identified various frequent genetic variants associated with pancreatic cancer risk. The two largest pancreatic cancer GWAS done in European populations are the Pancreatic Cancer Cohort Consortium (PanScan) and the Pancreatic Cancer Case-Control Consortium (PanC4), and a total of 21 susceptibility loci associated at genome-wide significance level have been discovered, and studied individually and in combination (7–15). However, the identified SNPs explain only 4.1% of the total phenotypic variance of pancreatic cancer, which do not fully account for the overall 21.2% estimated genetic heritability (16). This can be explained by the relatively small effect sizes of the individual risk loci, and by the strict multiple testing correction required for GWAS (typically p < 5x10-8), which is likely to result in a large number of false negatives.
Over the past decade, GWAS have achieved substantial success in discovering many common variants underlying the genetic architecture of complex diseases (17), including pancreatic cancer. Standard models for implying specific relationships between genotypes and phenotypes include additive, recessive and dominant models (18). The association of biallelic single nucleotide polymorphisms (SNPs) having alleles A/a with a given endpoint (e.g. disease risk) is typically analyzed with a logistic regression model logit(P) = α + β (X), where in an additive model X = 0, 1 or 2 depending on the genotype (homozygotes A/A, heterozygotes A/a and homozygotes a/a, respectively), thus the risk of disease is increased exp(β)-fold for subjects with genotype A/a and exp(2β)-fold for subjects with genotype a/a. A recessive model compares rare homozygotes a/a (who will have X=1) versus the rest (combining heterozygotes A/a and common homozygotes A/A, who will have X=0); a dominant model compares A/A (X=0) versus A/a + a/a (X=1). As most GWAS studies assume that allelic effects are additive, most of the associations reported in GWAS consider only the additive model of inheritance. But for variants which do not follow an intermediate model of inheritance, the recessive or the dominant genetic model can have more power to detect associations. Reanalysis of GWAS data with the recessive model of inheritance, considering homozygotes for the minor allele as the only “exposed” category could help to identify additional risk loci for non-negligible subsets of SNPs (19).
To discover novel recessively inherited pancreatic cancer risk loci, we performed a secondary analysis using genotyping data from all published pancreatic cancer GWAS conducted in subjects of European origin, and then replicated the most promising variants in cases and controls collected from the PANcreatic Disease ReseArch (PANDoRA) consortium. Better understanding the genetic background of the disease could be an invaluable tool to stratify the population by individual risk and increase our chances of early detection.
Materials and Methods
Study Populations
The following publicly available GWAS datasets on pancreatic cancer risk were used for this study: the Pancreatic Cancer Cohort Consortium (PanScan, comprising of PanScan I, PanScan II, and PanScan III) and the Pancreatic Cancer Case Control Consortium (PanC4). We obtained the genotype data from the NCBI database of genotypes and phenotypes (dbGaP) (study accession numbers phs000206.v5.p3 and phs000648.v1.p1; project reference #12644). We performed standard quality control and genotype imputation for the four datasets separately, using the Michigan Imputation Server (https://imputationserver.sph.umich.edu) (20) and the Haplotype Reference Consortium (HRC, V.r1.1) reference panel (21). Before imputation, we implemented individual- and SNP-level quality control steps as follows: individual and SNP missingness (call rate<0.9); sex discrepancy; heterozygosity (>3 SD from the mean); relatedness (PI_HAT>0.2, i.e., subjects related up to the second degree); ethnic outliers (population structure was captured by principal component analysis to remove non-European ancestry individuals); minor allele frequency (MAF) <0.005; and Hardy-Weinberg equilibrium (HWE) (p<1×10−6). After imputation, we removed SNPs with low imputation quality (INFO score r2<0.7, MAF<0.05 or call rate<0.9). Then, we merged the four imputed datasets and rechecked for the relatedness in the pooled dataset. At the end, a total of 5,056,279 SNPs in 8,769 cases and 7,055 controls (8,600 males and 7,224 females) remained for further analysis.
Additional samples belonging to the PANDoRA consortium, mostly from European populations, were selected for genotyping. Cases were diagnosed with pancreatic ductal adenocarcinoma (PDAC) and were all collected from the PANDoRA consortium (22). Controls were from the same geographical regions as the cases. A subset of the German controls (N=932) derived from ESTHER, a prospective cohort with 9,953 participants recruited in the Saarland region of Germany during a general health check-up in the period of July 2000 and December 2002. British and Dutch controls were collected from the European Prospective Investigation on Cancer (EPIC, http://epic.iarc.fr/), a prospective cohort study consisting of general population healthy volunteers from ten European countries (23). All subjects provided written informed consent. Approval for the PANDoRA study protocol (including for controls from ESTHER and EPIC cohorts) was received from the Ethics Commission of the Medical Faculty of the University of Heidelberg.
SNP Selection
We performed the association analysis on the pooled imputed PanScan+PanC4 GWAS data using both additive and recessive models. Association statistics (odds ratios (OR) and 95% confidence intervals (CI)) on PDAC risk were obtained with logistic regression adjusting for age, sex and the top ten principal components using PLINK version 1.9 (24). There were 268 SNPs that showed an association with p-value lower than 10-5, according to a recessive model (Supplementary Table 1). Most of them overlapped with previously reported pancreatic cancer risk loci (1q32.1, 2p14, 3q28, 5p15.33, 7p14.1, 7q32.3, 9q34, 13q12.2 and 16q23.1) from additive analyses. Among remaining SNPs which were over 1 Mb away from the closest known locus and showed no linkage disequilibrium (LD) with known loci (r2 < 0.01), ten SNPs at six loci, showed large differences in p-values using the two models (p < 10-5 using the recessive model, and p > 10-3 using the additive model). After filtering SNPs in LD (r2>0.8, N=3) and removing SNPs that showed p≥0.05 for association with PDAC risk in either PanScan or PanC4 (N=1), the top six promising SNPs were moved forward to genotyping.
Genotyping
DNA of PANDoRA samples was isolated from whole blood using QIAamp DNA extraction kit (Qiagen) and distributed in 384-well plates for genotyping. For quality control, 8% of the samples was randomly duplicated throughout the plates and no template controls (NTC) were used in each genotyping plate. Genotyping was performed using TaqMan (ABI, Applied Biosystems, Foster City, CA, USA) and KASP (KBioscence, Hoddesdon, UK) probes on the Real-Time PCR system. Since the genotyping assay for rs147904962 failed to work, rs12943205 was genotyped as a proxy SNP, in high LD (r2 = 0.99). Detection was done with a Viia7 instrument and Viia7 software (Applied Biosystems, Foster City, CA). After calling all the genotypes, samples with a call rate < 83.3% (i.e., missing more than one genotype) were removed. Duplicated samples with low concordance rate (>1 discordant genotype) were excluded. Discordance from HWE distribution was checked in controls, in the overall population and by country, and all the genotyped SNPs were in HWE (p>10-3). Dutch and British controls were genotyped in the context of a GWAS using the Human 660W-Quad BeadChip array (Illumina, San Diego, CA). Quality control steps were performed after TaqMan genotyping. Finally, 3,212 PDAC cases and 3,470 controls were included for further analysis. The characteristics of the study population are summarized in Table 1.
Statistical Analysis
To investigate the effect of the genotyped SNPs (rare allele vs. common allele; rare homozygous genotype vs. heterozygous plus common homozygous genotypes) in PANDoRA samples on the PDAC risk, we performed unconditional logistic regression adjusting for sex, age and country. Then we performed meta-analyses using R package “meta” by fixed-effects model (or random-effects model when p < 0.05 in the heterogeneity test) between phase one (reanalysis of the pancreatic cancer GWASs, PanScan and PanC4) and phase two (replication in samples collected from PANDoRA), with a final sample size of 11,981 PDAC cases and 10,525 controls. For the analysis with the genotyped SNPs in phase two, age, sex and genotypes had missing rates between 1% to 5%. Considering that missing data can have a significant effect on the conclusion, we applied multiple imputation which is a missing data method that provides valid statistical inferences under the missing at random condition (25). The R package “mice”, which imputes incomplete multivariate data by chained equations (26), was used to impute five times the variables involved in analysis, to analyze each of the imputed datasets separately based on the logistic regression model, then to automatically combine all the results together. Since the Brazilian population is known to be ethnically admixed, we performed additional statistical analyses with the PANDoRA Brazilian cases and controls. Meta-analyses were performed after multiple imputation as well. Analyses were carried out with R V3.6.
In addition, we performed gene-based analysis using MAGMA v1.08 to test the associations between all coding genes and PDAC risk based on the p-values under additive and recessive models respectively (27).
Bioinformatic Tools
We used the following tools/databases to explore the possible function of candidate SNPs: the Genotype-Tissue Expression (GTEx, 8th version) project portal (https://www.gtexportal.org, accessed on 30 June 2020), HaploReg v4.1 (https://pubs.broadinstitute.org/mammals/haploreg/haploreg.php) and RegulomedB (https://www.regulomedb.org/regulome-search/) (28–30). The Gene Expression Profiling Interactive Analysis (GEPIA2) database (http://gepia2.cancer-pku.cn) was applied to verify the expression levels and evaluate the prognostic value of genes of interest in pancreas tumor and normal tissues (31). Three-Dimensional-genome Interaction Viewer (3DIV, http://3div.kr), which collected all publicly available high-throughput chromatin conformation capture (Hi-C) data from human cell/tissue types, was used to explore the locus regulatory effects of the 3D genome (32). SNPnexus (https://www.snp-nexus.org/) and OpenTargets Genetics (https://genetics.opentargets.org) summarize the results of many different functional annotations (33, 34). The Functional Mapping and Annotation of Genome-Wide Association Studies platform (FUMA, https://fuma.ctglab.nl) was used to annotate the results of the recessive model GWAS (35).
Results
In the first phase, which was conducted at a genome-wide scale, we re-analyzed the data from the PanScan+PanC4 GWAS dataset according to a recessive model of inheritance, and we observed six SNPs that showed specific recessive associations with PDAC risk with p<10-5 while p>10-3 using the additive model (Figure 1, Supplementary Table 2). The correlated SNPs in these regions (r2>0.8 in LD) did not show evidence of stronger association under an additive model (Supplementary Table 3, Supplementary Figure 1). The associations of the genotyped SNPs with PDAC risk under the additive and recessive genetic models are shown in Figure 1 (Supplementary Table 2). In the validation phase in PANDoRA, no statistically significant associations (p<0.05) were observed, using the recessive model, except for rs2066357. However, this SNP showed high heterogeneity, with an opposite effect compared to the discovery phase under the recessive genetic model.
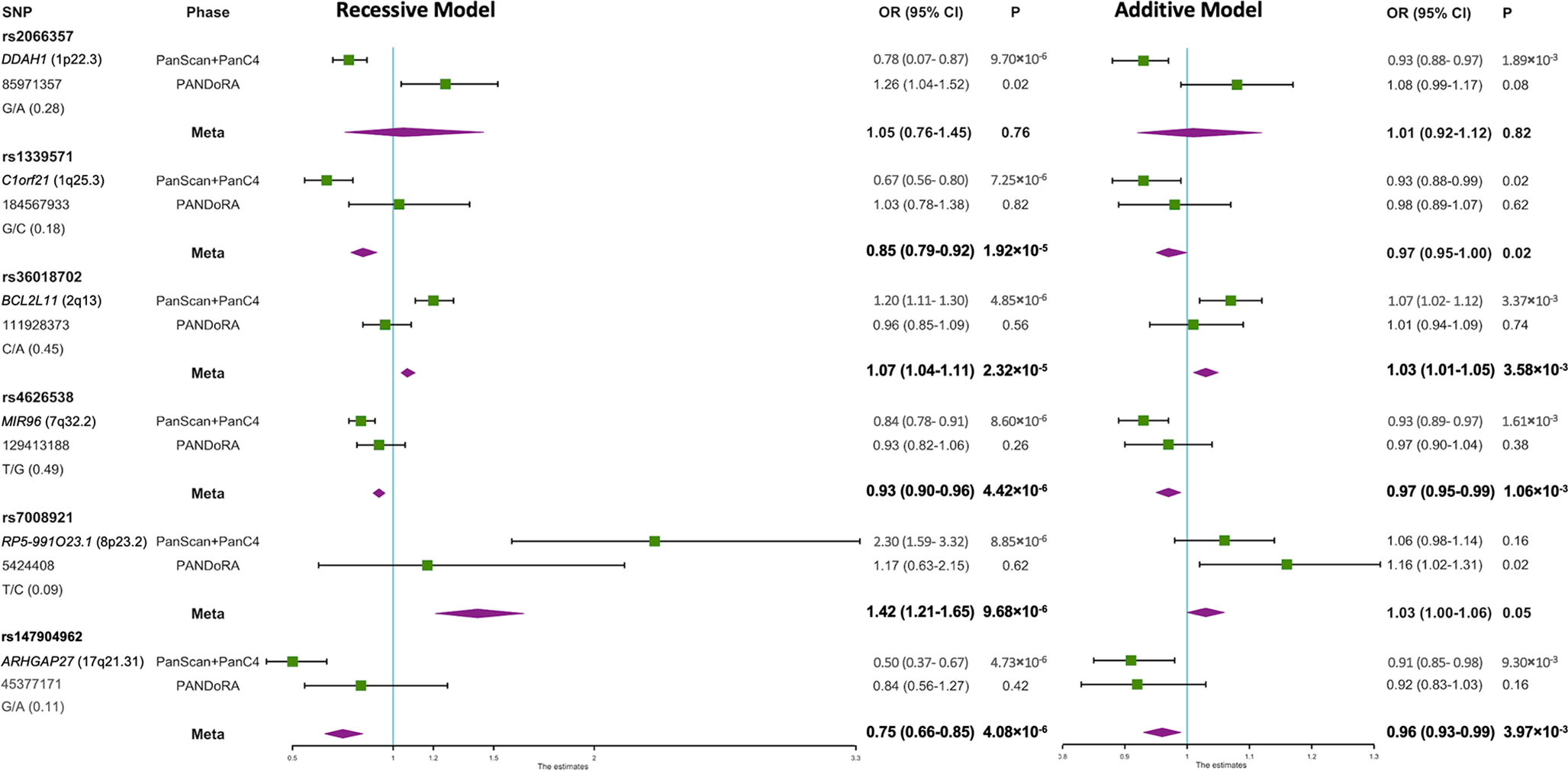
Figure 1 Forest plot of the associations of the 6 SNPs with PDAC risk under recessive and additive genetic models. A forest plot for the 6 SNPs and risk of PDAC is shown by two genetic models using data from discovery and replication analyses combined. Population specific odds ratios (OR) and 95% confidence intervals (CI) are denoted by green boxes and black lines. The combined OR estimates are represented by purple diamonds, where diamond width corresponds to 95% CI bounds. The position information (hg38) and minor allele frequency (MAF) for each SNP are shown on the left.
In meta-analyses, none of the six SNPs reached the conventional genome-wide significance threshold (p<5×10−8). However, MIR96 rs4626538 (OR=0.93; p=4.42×10−6), RP5-991O23.1 rs7008921 (OR=1.42; p=9.68×10−6) and ARHGAP27 rs147904962 (OR=0.75; p=4.08×10−6) maintained a specific recessive effect compared to the additively inherited effects (p=1.06×10−3, p=0.05 and p=3.97×10−3, respectively), and the p-values of rs4626538 and rs147904962 in the meta-analysis were slightly lower in comparison with those observed in the first phase. The results after multiple imputation were generally consistent with those without multiple imputation (Supplementary Table 2). Results did not change when we added the PANDoRA cases and controls from Brazil, who are ethnically admixed (Supplementary Tables 4, 5).
We used data from the GTEx consortium to investigate associations between genetic variants and RNA expression. We observed that the rs147904962-A allele was associated with increased LRRC37A4P RNA expression in adipose tissue (p=8.1×10−6). An expanded list of linked SNPs (in LD with our six candidate SNPs, r2>0.6) was also considered for the GTEx analysis; we found that the T allele of rs590097 (in LD with the A allele of rs36018702, r2 = 0.74, D’=1) was associated with higher expression of BCL2L11 in pancreas (p=5.64×10−6). No expression quantitative trait loci (eQTL) associations in pancreas were found for the other SNPs. Haploreg and RegulomeDB did not show evidence for functional effect for these variants.
Using a threshold of >2 for distance-normalized chromatin interaction frequency, 3DIV predicted C1orf21 and APOBEC4 to be interaction genes for rs1339571, BUB1 for rs36018702, MIR4423 for rs2066357, SPPL2C, SLC4A1, RUNDC3A, LOC100133991, TEX34, ITGA2B, and C17orf57 for rs147904962, respectively.
Additional analyses with SNPnexus and OpenTargets Genetics did not suggest any clear functional link between our candidate SNPs and pancreatic physiology or pathology. Likewise, when we reanalyzed with FUMA the results of the GWAS analysis according to the recessive model, we did not observe any noteworthy signal in the regions of the six candidate SNPs.
The gene-based analysis using MAGMA based on the p-values of the recessive model revealed that 14 genes were associated with PDAC risk at p < 0.001 (Supplementary Table 6). Two of these genes showed evidence for association at p < 0.001 under the recessive model (CTSG 14q12, p = 2.53x10-4; LEPROTL1 8p12, p = 4.34x10-4), but not with the additive one (p = 0.20 and p = 0.10, respectively). Then we verified the expression level of the two genes in pancreatic cancer patients using GEPIA2. We found that LEPROTL1 has increased expression in pancreatic cancer tissues compared to adjacent normal pancreatic tissues of the same patients (Figure 2).
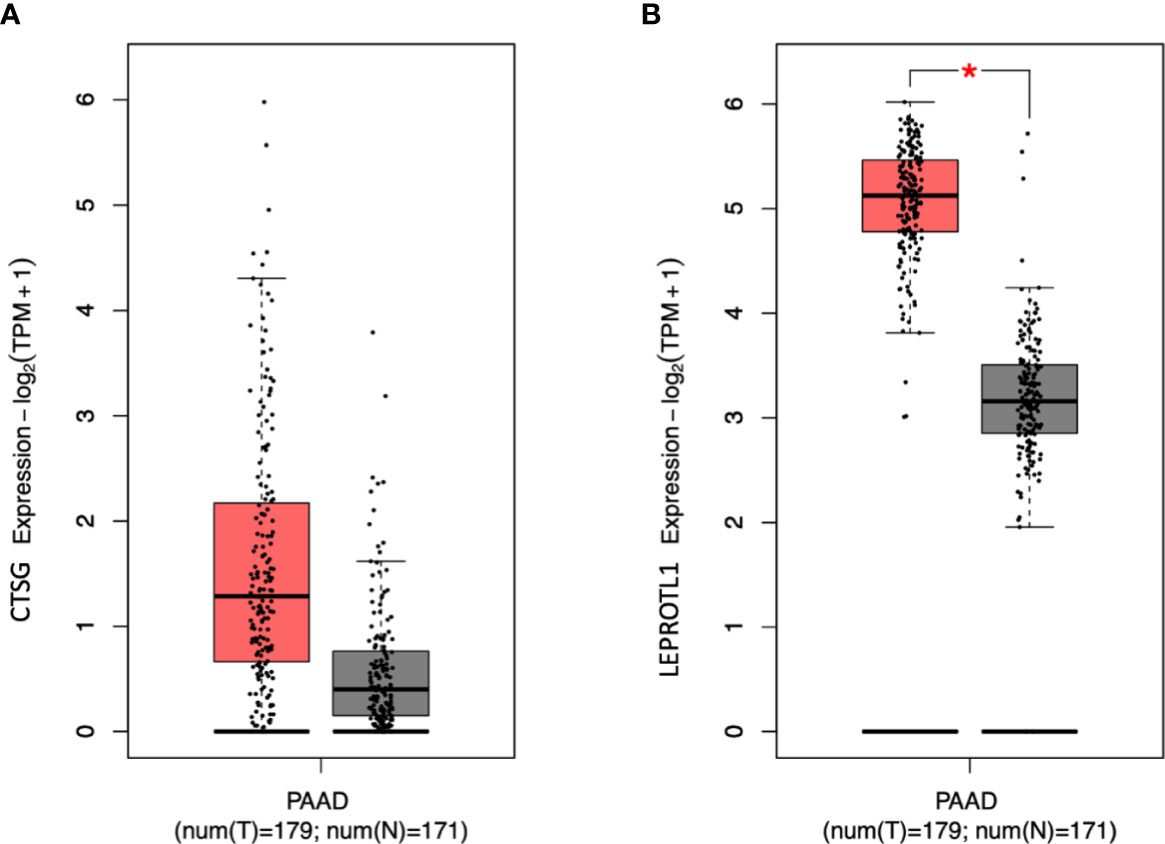
Figure 2 The expression level of CTSG and LEPROTL1 in PAAD patients. GEPIA2 generates box plot for comparing gene expression in pancreatic cancer and paired normal tissues (TCGA tumor versus TCGA normal + GTEx normal). (A, B) differential expression analysis. Peach and grey clusters represent tumor and normal samples; * genes with higher |log2FC| values (>1) and lower Q-values (<0.01) were considered differentially expressed genes.
Discussion
GWAS data are usually analyzed according to an additive genetic model, which is generally considered to be a good surrogate for other genetic models, except for the recessive one (19). Researchers have reported risk variants that showed specifically stronger evidence under a recessive model than an additive model, for type 2 diabetes (36), schizophrenia (37), high triglycerides (38), and other traits (39, 40), but not for PDAC yet. To identify recessive susceptibility loci for PDAC risk, we performed a secondary analysis with the largest currently available pancreatic cancer GWAS datasets (PanScan and PanC4) of European ancestry and attempted the replication of the six most promising variants in additional samples collected from the PANDoRA consortium, with a combined sample size of 11,981 PDAC cases and 10,525 controls. In this study, none of our results reached genome-wide statistical significance (p<5x10−8) in either phase, or in the meta-analyses, therefore our results are not conclusive. However, for five of the six selected SNPs the results of the meta-analysis do not exclude the possible recessive association with pancreatic cancer risk. In particular, rs4626538 (7q32.2), rs7008921 (8p23.2) and rs147904962 (17q21.31) maintained a large difference in significance between recessive effects compared with the additively inherited effects.
None of the previous studies indicated a link between these loci and pancreatic cancer risk. No variants in high LD (r2>0.8) have been previously associated with any trait or disease in GWAS, although variants in low to moderate LD (r2 = 0.14~0.60, D’=0.88~1 in Europeans) with rs147904962 have been reported to be associated with waist-to-hip ratio and with risk of developing allergic diseases. The minor G allele of rs7214661 (r2 = 0.19, D’=0.98) was associated with higher risk of allergic disease (41) while the corresponding A allele of rs147904962 was associated with lower risk of pancreatic cancer in our study. It is consistent with the protective effect of allergy for pancreatic cancer in epidemiologic studies (42).
Additionally, GTEx showed that rs590097 regulates BCL2L11 expression in pancreas tissue. BCL2L11 is a member of the BCL2 family and plays a role in neuronal and lymphocyte apoptosis. There is evidence shown that BCL2L11 is one of the major genes contributing to apoptosis, known to be important for pancreatic biology (http://www.genome.jp/kegg/pathway.html) (43). Moreover, the observed association that rs36018702-A (correlated with rs590097-T) showed increased risk of PDAC is consistent with the higher expression of BCL2L11 in pancreatic cancer tissues than in normal pancreas tissues found through GEPIA2.
BUB1 is the interaction gene of rs36018702 predicted by 3DIV. There is evidence that BUB1 is overexpressed in PDAC tissues, suggesting a role of BUB1 in PDAC progression, and therefore corroborating the association of rs36018702 and PDAC risk (44).
The lowest p-value we observed in the meta-analysis is 4.08x10-6 for the association of rs147904962 (17q21.31) with the risk of PDAC. rs147904962 is situated 17kb at the 3’ end of Rho GTPase Activating Protein 27 (ARHGAP27). This gene encodes a member of a large family of proteins that activate Rho-type guanosine triphosphate (GTP) metabolizing enzymes and are involved in cancer through the dysregulation of this mechanism. As ARHGAP27 mRNA is expressed in pancreatic cancer, we speculate that rs147904962 mediates regulation of cancer-associated ARHGAP27, promoting carcinogenesis through dysregulation of Rho/Rac/Cdc42-like GTPases (45). However, it has to be acknowledged that this SNP is not known to be located in a regulatory region of ARHGAP27.
Gene-based analyses based on the PanScan and PanC4 datasets (we were not able to replicate these analyses in PANDoRA, which does not have GWAS data) showed that SNPs in LEPROTL1 and CTSG were associated with PDAC risk according to the recessive, but not to the additive model. The bioinformatic analysis identified that LEPROTL1 was highly expressed in pancreatic cancer compared to matched normal pancreatic tissue of the same patients, suggesting a potential involvement in the etiopathology of PDAC. The leptin receptor overlapping transcript-like 1 gene (LEPROTL1) encodes a membrane protein, and may play a role in liver resistance by suppressing the growth hormone activity (46, 47), while the pancreatic cancer-related functions of LEPROTL1 remain unknown. The cathepsin G gene (CTSG) encodes a neutrophil serine protease of the chymotrypsin family, which was shown to affect neutrophil infiltration into the pancreas in a mouse model of pancreatitis (48). Based on this circumstantial evidence it is tempting to speculate a role for this gene and its polymorphisms in modulation of inflammation in the pancreas, which plays a role in the etiology of PDAC. However, to the best of our knowledge, a role for CTSG in pancreatic cancer has not been reported in the literature.
The lack of direct functional evidence for the SNPs of interest from bioinformatic analyses may at least in part reflect the fact that also bioinformatic tools/databases have not been designed to address effects of real recessive alleles. Ad hoc tools are needed to better understand the genetic architecture of complex genetic diseases.
It is hard to reach sufficient statistical power to detect variants with recessive effects, unless they are very frequent or have very large effects. Given the effective combined sample size of 11,981 PDAC cases and 10,525 controls, disease prevalence of 1.6%, and a significance cut-off of p<5×10−8, we had at least 80% power to detect a association with ORs equal to those observed in the discovery phase for the rare homozygote genotype for SNPs rs7008921 and rs147904962, whereas for the other SNPs power ranged between 54% to 69%. Thus, our study, in spite of the large sample size, lacked statistical power to confirm the risk with recessive model for some of the SNPs. It is worth noting that between PanScan, PanC4 and PANDoRA we have used the largest available resources for genetics of pancreatic cancer in populations of European origin. Our hypothesis that some variants may be associated with pancreatic cancer risk with a recessive model of inheritance was not disproved, but to prove it convincingly will require even larger datasets that will become available as more GWAS on pancreatic cancer risk are performed.
Identifying high-risk groups could contribute to focus surveillance and invasive screening measures, thereby improving the chance of early detection. Polygenic risk scores (PRS) approaches which could combine modest effect from each risk SNPs have demonstrated accuracies between 59% and 63% for predicting the risk of PDAC when including both non-genetic and genetic factors (14, 49–51). The accuracy of the existing PRS is not ready yet to be used in the clinical practice. It is necessary to expand the PRS with additional risk factors to improve its predictive power. For example, PRS including more SNPs that are not genome-wide significant but having noteworthy effects such as the ones we highlighted in this work may provide an additive contribution to the overall performance.
Conclusions
In conclusion, we propose some candidate SNPs as recessively inherited genetic variants for pancreatic cancer risk in European populations, which should be further confirmed by better powered investigations and/or meta-analysis of our results with those of other studies. Although none of the SNPs reached the genome-wide statistical significance, it is still worth to include these relevant SNPs into the PRS approach for risk stratification. A risk stratification approach with high predictive power could be used to identify subgroups at particularly increased risk of pancreatic cancer, either in the general population or in groups that are already known to have an elevated risk, such as diabetics.
Data Availability Statement
The datasets presented in this study can be found in online repositories. The names of the repository/repositories and accession number(s) can be found in the article/Materials and Methods, Study Populations section.
Ethics Statement
The studies involving human participants were reviewed and approved by the Ethics Commission of the Medical Faculty of the University of Heidelberg. The patients/participants provided their written informed consent to participate in this study.
Author Contributions
DC and FC conceived and designed the study. YL performed the lab work. YL performed data curation and analysis. YL drafted the manuscript. DC, MGe, and FC reviewed and edited the manuscript. All other authors provided samples and data. All authors critically read, commented and approved the final manuscript.
Funding
This work was supported by intramural funding of German Cancer Research Center (DKFZ); and by Fondazione Tizzi (www.fondazionetizzi.it); Fondazione Arpa (www.fondazionearpa.it); the Economic Development and Innovation Operative Programme Grant (Grant number GINOP-2.3.2-15-2016-00048); the Human Resources Development Operational Programme Grant (Grant number EFOP 3.6.2‐16‐2017‐0006); Associazione Italiana Ricerca Cancro (Grant number 5x1000, 12182, and IG 17177); Fondazione Italiana Malattie Pancreas – Ministero Salute (Grant number FIMPCUP_J38D19000690001); Fondazione Cariverona: Oncology Biobank Project “Antonio Schiavi” (Grant number 203885/2017); the Ministry of Health of the Czech Republic (Grant number NV19-03-00097, FNOL-00098892); student grant from Palacky University (Grant number IGA_LF_2020_005). The ESTHER study was funded by the Baden-Württemberg State Ministry of Science, Research and Arts (Stuttgart, Germany).
Conflict of Interest
The authors declare that the research was conducted in the absence of any commercial or financial relationships that could be construed as a potential conflict of interest.
Publisher’s Note
All claims expressed in this article are solely those of the authors and do not necessarily represent those of their affiliated organizations, or those of the publisher, the editors and the reviewers. Any product that may be evaluated in this article, or claim that may be made by its manufacturer, is not guaranteed or endorsed by the publisher.
Acknowledgments
We would like to thank Mrs. Angelika Stein for help with the lab work. The research used the genotyping data provided by the EPIC, we would like to thank the contributors from the UK and the Netherlands. The EPIC-Norfolk study (DOI 10.22025/2019.10.105.00004) has received funding from the Medical Research Council (MR/N003284/1 and MC-UU_12015/1) and Cancer Research UK (C864/A14136). We are grateful to all the participants who have been part of the project and to the many members of the study teams at the University of Cambridge who have enabled this research. The authors would also thank all the participants of the PanScan GWAS Study and Pancreatic Cancer Case Control Association Study, and dbGaP for providing cancer genotyping dataset.
Supplementary Material
The Supplementary Material for this article can be found online at: https://www.frontiersin.org/articles/10.3389/fonc.2021.771312/full#supplementary-material
References
1. Ferlay J, Colombet M, Soerjomataram I, Dyba T, Randi G, Bettio M, et al. Cancer Incidence and Mortality Patterns in Europe: Estimates for 40 Countries and 25 Major Cancers in 2018. Eur J Cancer (2018) 103:356–87. doi: 10.1016/j.ejca.2018.07.005
2. Rahib L, Smith BD, Aizenberg R, Rosenzweig AB, Fleshman JM, Matrisian LM. Projecting Cancer Incidence and Deaths to 2030: The Unexpected Burden of Thyroid, Liver, and Pancreas Cancers in the United States. Cancer Res (2014) 74:2913–21. doi: 10.1158/0008-5472.CAN-14-0155
3. Bray F, Ferlay J, Soerjomataram I, Siegel RL, Torre LA, Jemal A. Global Cancer Statistics 2018: GLOBOCAN Estimates of Incidence and Mortality Worldwide for 36 Cancers in 185 Countries. CA Cancer J Clin (2018) 68:394–424. doi: 10.3322/caac.21492
4. Karim-Kos HE, de Vries E, Soerjomataram I, Lemmens V, Siesling S, Coebergh JWW. Recent Trends of Cancer in Europe: A Combined Approach of Incidence, Survival and Mortality for 17 Cancer Sites Since the 1990s. Eur J Cancer (2008) 44:1345–89. doi: 10.1016/j.ejca.2007.12.015
5. Arnold M, Rutherford MJ, Bardot A, Ferlay J, Andersson TML, Myklebust TÅ, et al. Progress in Cancer Survival, Mortality, and Incidence in Seven High-Income Countries 1995–2014 (ICBP SURVMARK-2): A Population-Based Study. Lancet Oncol (2019) 20:1493–505. doi: 10.1016/S1470-2045(19)30456-5
6. Gentiluomo M, Canzian F, Nicolini A, Gemignani F, Landi S, Campa D. Germline Genetic Variability in Pancreatic Cancer Risk and Prognosis. Semin Cancer Biol (2020) S1044-579X(20)30174-7. doi: 10.1016/j.semcancer.2020.08.003
7. Amundadottir L, Kraft P, Stolzenberg-Solomon RZ, Fuchs CS, Petersen GM, Arslan AA, et al. Genome-Wide Association Study Identifies Variants in the ABO Locus Associated With Susceptibility to Pancreatic Cancer. Nat Genet (2009) 41:986–90. doi: 10.1038/ng.429
8. Petersen GM, Amundadottir L, Fuchs CS, Kraft P, Stolzenberg-Solomon RZ, Jacobs KB, et al. A Genome-Wide Association Study Identifies Pancreatic Cancer Susceptibility Loci on Chromosomes 13q22.1, 1q32.1 and 5p15.33. Nat Genet (2010) 42:224–8. doi: 10.1038/ng.522
9. Childs EJ, Mocci E, Campa D, Bracci PM, Gallinger S, Goggins M, et al. Common Variation at 2p13.3, 3q29, 7p13 and 17q25.1 Associated With Susceptibility to Pancreatic Cancer. Nat Genet (2015) 47:911–6. doi: 10.1038/ng.3341
10. Zhang M, Wang Z, Obazee O, Jia J, Childs EJ, Hoskins J, et al. Three New Pancreatic Cancer Susceptibility Signals Identified on Chromosomes 1q32.1, 5p15.33 and 8q24.21. Oncotarget (2016) 7:66328–43. doi: 10.18632/oncotarget.11041
11. Wolpin BM, Rizzato C, Kraft P, Kooperberg C, Petersen GM, Wang Z, et al. Genome-Wide Association Study Identifies Multiple Susceptibility Loci for Pancreatic Cancer. Nat Genet (2014) 46:994–1000. doi: 10.1038/ng.3052
12. Klein AP, Wolpin BM, Risch HA, Stolzenberg-Solomon RZ, Mocci E, Zhang M, et al. Genome-Wide Meta-Analysis Identifies Five New Susceptibility Loci for Pancreatic Cancer. Nat Commun (2018) 9:1–11. doi: 10.1038/s41467-018-02942-5
13. Campa D, Gentiluomo M, Obazee O, Ballerini A, Vodickova L, Hegyi P, et al. Genome-Wide Association Study Identifies an Early Onset Pancreatic Cancer Risk Locus. Int J Cancer (2020) 147:2065–74. doi: 10.1002/ijc.33004
14. Galeotti AA, Gentiluomo M, Rizzato C, Obazee O, Neoptolemos JP, Pasquali C, et al. Polygenic and Multifactorial Scores for Pancreatic Ductal Adenocarcinoma Risk Prediction. J Med Genet (2021) 58:369–77. doi: 10.1136/jmedgenet-2020-106961
15. Kim J, Yuan C, Babic A, Bao Y, Clish CB, Pollak MN, et al. Genetic and Circulating Biomarker Data Improve Risk Prediction for Pancreatic Cancer in the General Population. Cancer Epidemiol Biomarkers Prev (2020) 29:999–1008. doi: 10.1158/1055-9965.EPI-19-1389
16. Chen F, Childs EJ, Mocci E, Bracci P, Gallinger S, Li D, et al. Analysis of Heritability and Genetic Architecture of Pancreatic Cancer: A PANC4 Study. Cancer Epidemiol Biomarkers Prev (2019) 28:1238–45. doi: 10.1158/1055-9965.EPI-18-1235
17. Sud A, Kinnersley B, Houlston RS. Genome-Wide Association Studies of Cancer: Current Insights and Future Perspectives. Nat Rev Cancer (2017) 17:692–704. doi: 10.1038/nrc.2017.82
18. Clarke GM, Anderson CA, Pettersson FH, Cardon LR, Morris AP, Zondervan KT. Basic Statistical Analysis in Genetic Case-Control Studies. Nat Protoc (2011) 6:121–33. doi: 10.1038/nprot.2010.182
19. Dizier MH, Demenais F, Mathieu F. Gain of Power of the General Regression Model Compared to Cochran-Armitage Trend Tests: Simulation Study and Application to Bipolar Disorder. BMC Genet (2017) 18:24. doi: 10.1186/s12863-017-0486-6
20. Das S, Forer L, Schönherr S, Sidore C, Locke AE, Kwong A, et al. Next-Generation Genotype Imputation Service and Methods. Nat Genet (2016) 48:1284–7. doi: 10.1038/ng.3656
21. McCarthy S, Das S, Kretzschmar W, Delaneau O, Wood AR, Teumer A, et al. A Reference Panel of 64,976 Haplotypes for Genotype Imputation. Nat Genet (2016) 48:1279–83. doi: 10.1038/ng.3643
22. Campa D, Rizzato C, Capurso G, Giese N, Funel N, Greenhalf W, et al. Genetic Susceptibility to Pancreatic Cancer and Its Functional Characterisation: The PANcreatic Disease ReseArch (PANDoRA) Consortium. Dig Liver Dis (2013) 45:95–9. doi: 10.1016/j.dld.2012.09.014
23. Riboli E, Hunt K, Slimani N, Ferrari P, Norat T, Fahey M, et al. European Prospective Investigation Into Cancer and Nutrition (EPIC): Study Populations and Data Collection. Public Health Nutr (2002) 5:1113–24. doi: 10.1079/phn2002394
24. Purcell S, Neale B, Todd-Brown K, Thomas L, Ferreira MAR, Bender D, et al. PLINK: A Tool Set for Whole-Genome Association and Population-Based Linkage Analyses. Am J Hum Genet (2007) 81:559–75. doi: 10.1086/519795
25. Graham JW. Missing Data Analysis: Making It Work in the Real World. Annu Rev Psychol (2009) 60:549–76. doi: 10.1146/annurev.psych.58.110405.085530
26. van Buuren S, Groothuis-Oudshoorn K. Mice: Multivariate Imputation by Chained Equations in R. J Stat Softw (2011) 45:1–67. doi: 10.18637/jss.v045.i03
27. de Leeuw CA, Mooij JM, Heskes T, Posthuma D. MAGMA: Generalized Gene-Set Analysis of GWAS Data. PloS Comput Biol (2015) 11:1–19. doi: 10.1371/journal.pcbi.1004219
28. Lonsdale J, Thomas J, Salvatore M, Phillips R, Lo E, Shad S, et al. The Genotype-Tissue Expression (GTEx) Project. Nat Genet (2013) 45:580–5. doi: 10.1038/ng.2653
29. Ward LD, Kellis M. HaploReg V4: Systematic Mining of Putative Causal Variants, Cell Types, Regulators and Target Genes for Human Complex Traits and Disease. Nucleic Acids Res (2016) 44:D877–81. doi: 10.1093/nar/gkv1340
30. Boyle AP, Hong EL, Hariharan M, Cheng Y, Schaub MA, Kasowski M, et al. Annotation of Functional Variation in Personal Genomes Using RegulomeDB. Genome Res (2012) 22:1790–7. doi: 10.1101/gr.137323.112
31. Tang Z, Kang B, Li C, Chen T, Zhang Z. GEPIA2: An Enhanced Web Server for Large-Scale Expression Profiling and Interactive Analysis. Nucleic Acids Res (2019) 47:W556–60. doi: 10.1093/nar/gkz430
32. Kim K, Jang I, Kim M, Choi J, Kim MS, Lee B, et al. 3DIV Update for 2021: A Comprehensive Resource of 3D Genome and 3D Cancer Genome. Nucleic Acids Res (2021) 49:D38–46. doi: 10.1093/nar/gkaa1078
33. Oscanoa J, Sivapalan L, Gadaleta E, Dayem Ullah AZ, Lemoine NR, Chelala C. SNPnexus: A Web Server for Functional Annotation of Human Genome Sequence Variation (2020 Update). Nucleic Acids Res (2020) 48:W185–92. doi: 10.1093/NAR/GKAA420
34. Ghoussaini M, Mountjoy E, Carmona M, Peat G, Schmidt EM, Hercules A, et al. Open Targets Genetics: Systematic Identification of Trait-Associated Genes Using Large-Scale Genetics and Functional Genomics. Nucleic Acids Res (2021) 49:D1311–20. doi: 10.1093/nar/gkaa840
35. Watanabe K, Taskesen E, Van Bochoven A, Posthuma D. Functional Mapping and Annotation of Genetic Associations With FUMA. Nat Commun (2017) 8:1826. doi: 10.1038/s41467-017-01261-5
36. Grarup N, Moltke I, Andersen MK, Bjerregaard P, Larsen CVL, Dahl-Petersen IK, et al. Identification of Novel High-Impact Recessively Inherited Type 2 Diabetes Risk Variants in the Greenlandic Population. Diabetologia (2018) 61:2005–15. doi: 10.1007/s00125-018-4659-2
37. Zhu D, Yin J, Liang C, Luo X, Lv D, Dai Z, et al. CACNA1C (Rs1006737) may be a Susceptibility Gene for Schizophrenia: An Updated Meta-Analysis. Brain Behav (2019) 9:e01292. doi: 10.1002/brb3.1292
38. Hebbar P, Nizam R, Melhem M, Alkayal F, Elkum N, Elsa John S, et al. Genome-Wide Association Study Identifies Novel Recessive Genetic Variants for High TGs in an Arab Population. J Lipid Res (2018) 59:1951–96. doi: 10.1194/jlr.P080218
39. Power RA, Keller MC, Ripke S, Abdellaoui A, Wray NR, Sullivan PF, et al. A Recessive Genetic Model and Runs of Homozygosity in Major Depressive Disorder. Am J Med Genet Part B Neuropsychiatr Genet (2014) 165:157–66. doi: 10.1002/ajmg.b.32217
40. Camargo M, Rivera D, Moreno L, Lidral AC, Harper U, Jones M, et al. GWAS Reveals New Recessive Loci Associated With Non-Syndromic Facial Clefting. Eur J Med Genet (2012) 55:510–4. doi: 10.1016/j.ejmg.2012.06.005
41. Ferreira MA, Vonk JM, Baurecht H, Marenholz I, Tian C, Hoffman JD, et al. Shared Genetic Origin of Asthma, Hay Fever and Eczema Elucidates Allergic Disease Biology. Nat Genet (2017) 49:1752–7. doi: 10.1038/ng.3985
42. Midha S, Chawla S, Garg PK. Modifiable and Non-Modifiable Risk Factors for Pancreatic Cancer: A Review. Cancer Lett (2016) 381:269–77. doi: 10.1016/j.canlet.2016.07.022
43. Li D, Duell EJ, Yu K, Risch HA, Olson SH, Kooperberg C, et al. Pathway Analysis of Genome-Wide Association Study Data Highlights Pancreatic Development Genes as Susceptibility Factors for Pancreatic Cancer. Carcinogenesis (2012) 33:1384–90. doi: 10.1093/carcin/bgs151
44. Piao J, Zhu L, Sun J, Li N, Dong B, Yang Y, et al. High Expression of CDK1 and BUB1 Predicts Poor Prognosis of Pancreatic Ductal Adenocarcinoma. Gene (2019) 701:15–22. doi: 10.1016/j.gene.2019.02.081
45. Katoh Y, Katoh M. Identification and Characterization of ARHGAP27 Gene in Silico. Int J Mol Med (2004) 14:943–7. doi: 10.3892/ijmm.14.5.943
46. Huang Y, Ying K, Xie Y, Zhou Z, Wang W, Tang R, et al. Cloning and Characterization of a Novel Human Leptin Receptor Overlapping Transcript-Like 1 Gene (LEPROTL1). Biochim Biophys Acta Gene Struct Expr (2001) 1517:327–31. doi: 10.1016/S0167-4781(00)00266-9
47. Touvier T, Conte-Auriol F, Briand O, Cudejko C, Paumelle R, Caron S, et al. LEPROT and LEPROTL1 Cooperatively Decrease Hepatic Growth Hormone Action in Mice. J Clin Invest (2009) 119:3830–8. doi: 10.1172/JCI34997
48. Aghdassi AA, John DS, Sendler M, Storck C, van den Brandt C, Krüger B, et al. Absence of the Neutrophil Serine Protease Cathepsin G Decreases Neutrophil Granulocyte Infiltration But Does Not Change the Severity of Acute Pancreatitis. Sci Rep (2019) 9:16774. doi: 10.1038/s41598-019-53293-0
49. Zhang YD, Hurson AN, Zhang H, Choudhury PP, Easton DF, Milne RL, et al. Assessment of Polygenic Architecture and Risk Prediction Based on Common Variants Across Fourteen Cancers. Nat Commun (2020) 11:1–13. doi: 10.1038/s41467-020-16483-3
50. Torkamani A, Wineinger NE, Topol EJ. The Personal and Clinical Utility of Polygenic Risk Scores. Nat Rev Genet (2018) 19:581–90. doi: 10.1038/s41576-018-0018-x
Keywords: pancreatic cancer, susceptibility, genome-wide association study, recessive model, genetic polymorphisms
Citation: Lu Y, Gentiluomo M, Macauda A, Gioffreda D, Gazouli M, Petrone MC, Kelemen D, Ginocchi L, Morelli L, Papiris K, Greenhalf W, Izbicki JR, Kiudelis V, Mohelníková-Duchoňová B, Bueno-de-Mesquita B, Vodicka P, Brenner H, Diener MK, Pezzilli R, Ivanauskas A, Salvia R, Szentesi A, Aoki MN, Németh BC, Sperti C, Jamroziak K, Chammas R, Oliverius M, Archibugi L, Ermini S, Novák J, Kupcinskas J, Strouhal O, Souček P, Cavestro GM, Milanetto AC, Vanella G, Neoptolemos JP, Theodoropoulos GE, van Laarhoven HWM, Mambrini A, Moz S, Kala Z, Loveček M, Basso D, Uzunoglu FG, Hackert T, Testoni SGG, Hlaváč V, Andriulli A, Lucchesi M, Tavano F, Carrara S, Hegyi P, Arcidiacono PG, Busch OR, Lawlor RT, Puzzono M, Boggi U, Guo F, Małecka-Panas E, Capurso G, Landi S, Talar-Wojnarowska R, Strobel O, Gao X, Vashist Y, Campa D and Canzian F (2021) Identification of Recessively Inherited Genetic Variants Potentially Linked to Pancreatic Cancer Risk. Front. Oncol. 11:771312. doi: 10.3389/fonc.2021.771312
Received: 06 September 2021; Accepted: 16 November 2021;
Published: 03 December 2021.
Edited by:
Walter Hernán Pavicic, Consejo Nacional de Investigaciones Científicas y Técnicas (CONICET), ArgentinaReviewed by:
Phuong Cao Thi Ngoc, Lund University, SwedenFlorentia Fostira, National Centre of Scientific Research Demokritos, Greece
Copyright © 2021 Lu, Gentiluomo, Macauda, Gioffreda, Gazouli, Petrone, Kelemen, Ginocchi, Morelli, Papiris, Greenhalf, Izbicki, Kiudelis, Mohelníková-Duchoňová, Bueno-de-Mesquita, Vodicka, Brenner, Diener, Pezzilli, Ivanauskas, Salvia, Szentesi, Aoki, Németh, Sperti, Jamroziak, Chammas, Oliverius, Archibugi, Ermini, Novák, Kupcinskas, Strouhal, Souček, Cavestro, Milanetto, Vanella, Neoptolemos, Theodoropoulos, van Laarhoven, Mambrini, Moz, Kala, Loveček, Basso, Uzunoglu, Hackert, Testoni, Hlaváč, Andriulli, Lucchesi, Tavano, Carrara, Hegyi, Arcidiacono, Busch, Lawlor, Puzzono, Boggi, Guo, Małecka-Panas, Capurso, Landi, Talar-Wojnarowska, Strobel, Gao, Vashist, Campa and Canzian. This is an open-access article distributed under the terms of the Creative Commons Attribution License (CC BY). The use, distribution or reproduction in other forums is permitted, provided the original author(s) and the copyright owner(s) are credited and that the original publication in this journal is cited, in accordance with accepted academic practice. No use, distribution or reproduction is permitted which does not comply with these terms.
*Correspondence: Federico Canzian, f.canzian@dkfz.de
†These authors share last authorship