- 1Department of Radiotherapy, Second Hospital of Jilin University, Changchun, China
- 2Department of Breast Surgery, Second Hospital of Jilin University, Changchun, China
- 3Nuclear Medicine Department, Second Hospital of Jilin University, Changchun, China
- 4Department of Cardiovascular Surgery, Second Hospital of Jilin University, Changchun, China
- 5Medical Research Center, Second Hospital of Jilin University, Changchun, China
Next-generation sequencing and bioinformatics analyses have clearly revealed the roles of mitochondrial ribosomal genes in cancer development. Mitochondrial ribosomes are composed of three RNA components encoded by mitochondrial DNA and 82 specific protein components encoded by nuclear DNA. They synthesize mitochondrial inner membrane oxidative phosphorylation (OXPHOS)-related proteins and participate in various biological activities via the regulation of energy metabolism and apoptosis. Mitochondrial ribosomal genes are strongly associated with clinical features such as prognosis and foci metastasis in patients with cancer. Accordingly, mitochondrial ribosomes have become an important focus of cancer research. We review recent advances in bioinformatics research that have explored the link between mitochondrial ribosomes and cancer, with a focus on the potential of mitochondrial ribosomal genes as biomarkers in cancer.
1 Introduction
In human cells, the vast majority of mitochondria-associated proteins are encoded by nuclear genes and synthesized by cytoplasmic ribosomes. Mitochondria, as endosymbiotic organelles, retain the characteristics of the original genomic DNA of their bacterial ancestors and possess relatively independent gene expression mechanisms (1). Human mitochondrial DNA (mtDNA) encodes two ribosomal RNAs (rRNAs) and a group of transfer RNAs (tRNAs) as well as oxidative phosphorylation (OXPHOS)-related proteins. These mitochondrially encoded proteins are located within the inner mitochondrial membrane and are synthesized by mitochondrial ribosomes (2). The mitochondrial ribosome differs from bacterial and cytoplasmic mitochondria with respect to complexity, structure, and mechanisms of action. It is composed of three RNA components encoded by mitochondrial DNA and 82 specific protein components encoded by nuclear DNA (3, 4). Over the past decade, the roles of mitochondrial ribosomal proteins in apoptosis and cellular energetics have been revealed (5, 6). Importantly, the inhibition of cell death, proliferative signaling, and deregulation of cellular energetics are hallmarks of cancer (7).
Margaret Dayhoff (1925–1983), an American physical chemist known as “the father of bioinformatics”, was the first to apply computational methods to the biological realm (8). Relying on advances in both molecular biology and computer science, bioinformatics has been evolving over several decades. The application of microarray technology, next-generation sequencing, and big data analysis techniques has revealed the important roles of mitochondrial ribosomal proteins in cancer biology.
This review examines the link between mitochondrial ribosomes and cancer. In particular, the composition, structure, and function of the mitochondrial ribosome are described. Bioinformatics tools, including relevant databases and methods for sequencing and analysis, are described with a focus on their application to the mining of candidate mitochondrial ribosomal protein biomarkers for malignancy. This review adds a bioinformatics perspective to research on mitochondrial ribosomes in relation to malignancies.
2 Mitochondrial Ribosomes
2.1 Components and Structure
In all organisms, the mature mitochondrial ribosome is composed of a large subunit (LSU) responsible for catalyzing peptidyl transferase reactions and a small subunit (SSU) providing a platform for mRNA binding and decoding (Figure 1). The 28S mt-SSU of the mammalian mitochondrial ribosome consists of one 12S rRNA and 29 mitochondrial ribosomal proteins (MRPs), whereas the 39S mt-LSU consists of one 16S rRNA, 48 MRPs, and one structural tRNA (9, 10). Using deep RNA sequencing, Alan Brown identified this structural tRNA as mt-tRNA Val, a molecule whose position resembles that occupied by 5S rRNA in the cytoplasmic ribosome (11). In addition, 16 mt-SSU proteins and 28 mt-LSU proteins are homologous to their counterparts in Escherichia coli, and the rest are mitochondria-specific proteins (12, 13). These nuclear-encoded subunit proteins are synthesized within the cytosol and enter mitochondria for subsequent assembly, mediated by chaperones and the transmembrane transport complex including Translocase of the Outer mitochondrial Membrane (TOM) and Translocase of the Inner Membrane (TIM) (14, 15). Unlike cytoplasmic ribosomes, the majority of mitochondrial ribosomes are permanently anchored to the inner mitochondrial membrane by mL45 (MRPL45) of the large subunit (4). The large and small subunits are connected by three protein–protein and six protein–RNA inter-subunit bridges, whereas bridges in bacterial and eukaryotic cytoplasmic ribosomes mainly consist of conserved RNA–RNA interactions (16). In addition, mitochondrial ribosomes differ from cytoplasmic and bacterial ribosomes by a high RNA-to-protein ratio (17). Finally, two relevant publications are cited that provide the nomenclature of mitochondrial ribosomal proteins for readers to consult (18, 19).
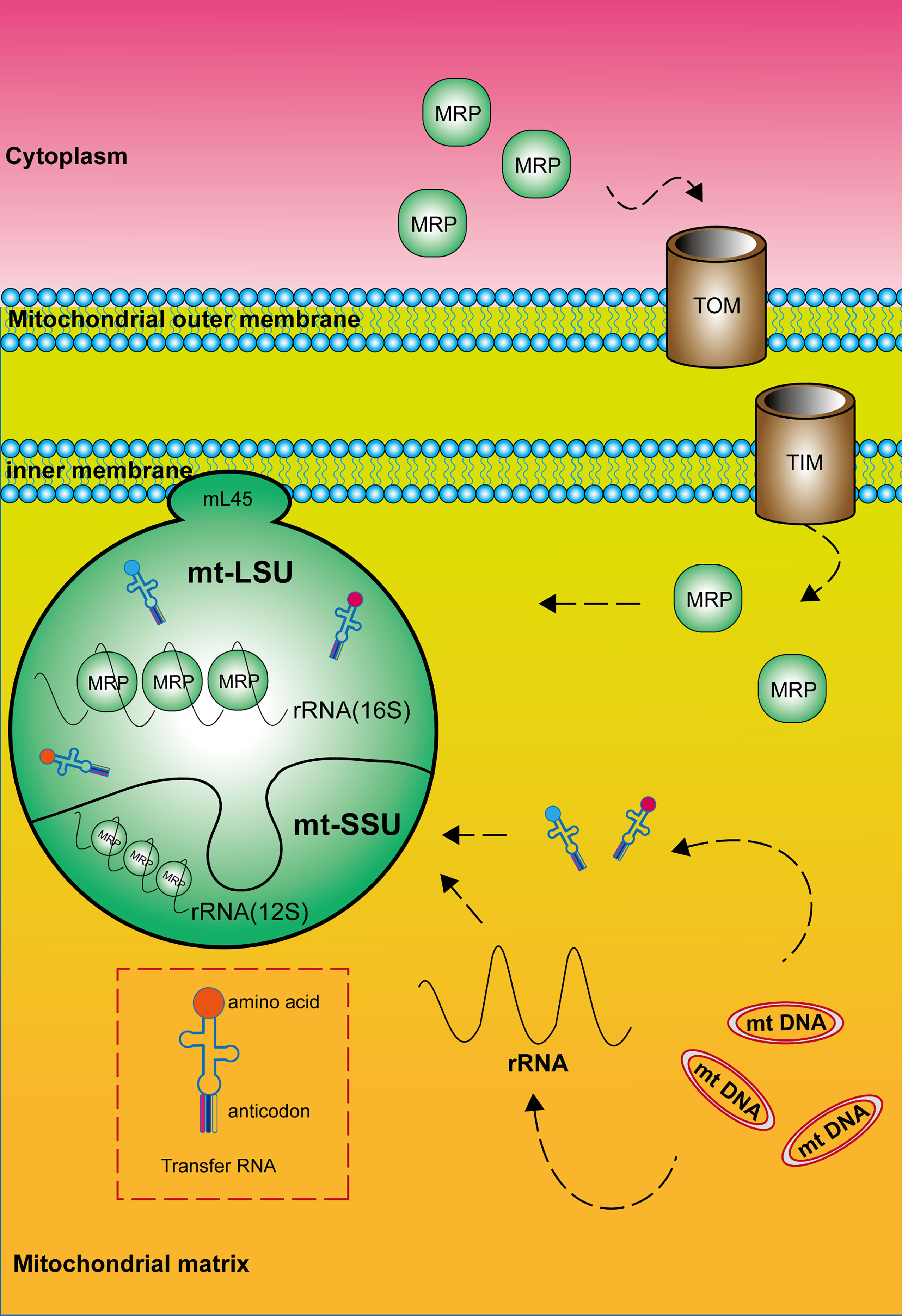
Figure 1 Composition and structure of the mitochondrial ribosome. The mature mitochondrial ribosome, which consists of a large and a small subunit, is permanently anchored to the inner mitochondrial membrane by MRPL45 (mL45) of the large subunit. Mitochondrial ribosomal proteins are synthesized within the cytosol and enter mitochondria mediated by the transmembrane transport complex including Translocase of the Outer mitochondrial Membrane (TOM) and Translocase of the Inner Membrane (TIM). RNA components are encoded by mitochondrial DNA.
2.2 MRPs and Energy Metabolism
Mitochondrial ribosomes are responsible for the translation of 13 subunit proteins of OXPHOS complex I, III, IV and V, which are encoded by the mitochondrial DNA. These proteins located in the inner mitochondrial membrane include seven subunits of complex I (NADH: ubiquinone oxidoreductase), one subunit of complex III (ubiquinone: cytochrome c oxidoreductase), three subunits of complex IV (cytochrome C: oxidoreductase), and two subunits of complex V (ATP synthase) (Figure 2) (2, 11, 20). Single-stranded mRNAs formed after mtDNA transcription are enriched at the SSU neck, where the pentatricopeptide repeat protein MRPS39 (mS39) recognizes and binds to the 5′ end of the mRNA and activates the translation machinery (21, 22).
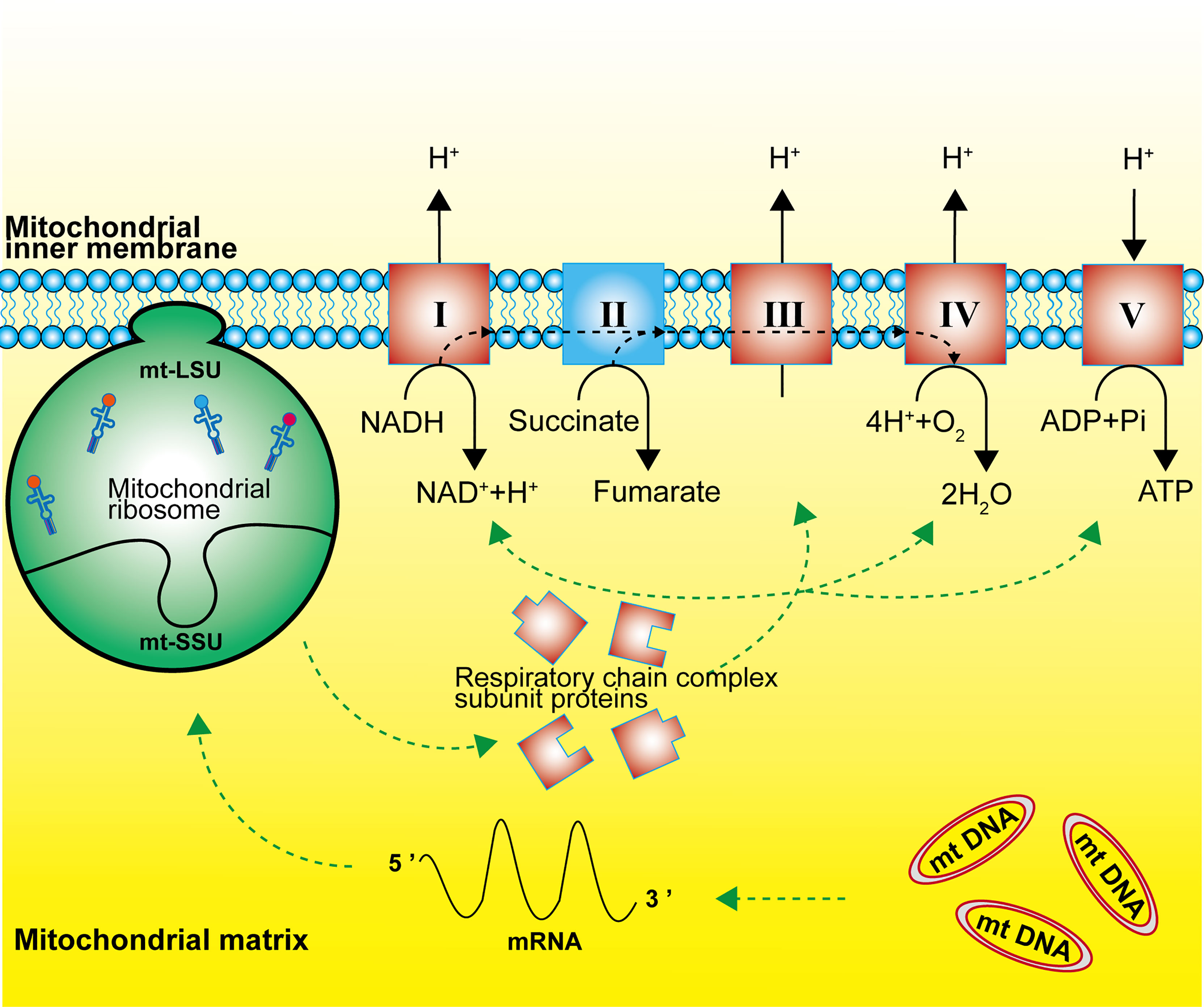
Figure 2 Function of the mitochondrial ribosome. Mitochondrial ribosomes synthesize the subunit proteins of OXPHOS complex I, III, IV and V, which are encoded by the mitochondrial DNA. The dashed black line represents the flow of electrons.
In mammalian cells under normal conditions, more than 80% of cellular ATP requirements are met by ATP generated by oxidative phosphorylation (OXPHOS). The OXPHOS metabolic pathway generates ATP by transporting electrons along a series of transmembrane protein complexes in the inner mitochondrial membrane, called the electron transport chain (ETC). Energy transport is achieved by the flow of electrons between complex I, complex II, coenzyme Q10, complex III, cytochrome c, and complex IV, with oxygen as the terminal electron acceptor (23). A methylation study has revealed a unique mechanism by which arginine and lysine methylation of MRPS23 promote breast cancer metastasis by regulating OXPHOS (24). Bioinformatics analyses have revealed that MRPS5 is closely related to the function of OXPHOS complex I and the acetylation status of MRPS5 is directly regulated by the NAD+-dependent deacetylase sirtuin-1 (SIRT1), indicating that the SIRT1/MRPS5 axis is involved in metabolic reprogramming and tumor progression (25). MRPL13 expression is reduced in OXPHOS-deficient hepatoma cells (SNU354 and SNU423 cell lines), and the specific inhibition of mitochondrial glycosomal translation by siRNA-mediated knockdown of MRPL13 decreases expression of the OXPHOS complex IV subunit protein COX2 (26).
Metabolic reprogramming in cancer cells confers the ability to adjust metabolic pathways to support heterogeneous biological processes. According to the Otto Warburg theory, glycolysis is upregulated in cancer cells compared to that in normal cells (7). However, this does not mean that oxidative phosphorylation is universally downregulated in cancer. A meta-analysis has suggested that the average contribution of OXPHOS to ATP production is 80% in normal cells and 83% in cancer cells (27), consistent with in vivo data from a study by Vaupel et al., who showed that the mitochondrial respiratory capacity is not always functionally impaired (28). Variation in the contribution of OXPHOS among cancer types may be explained by differences in the mtDNA content. In particular, the mtDNA content is higher in many cancer tissues than in normal tissues, including tissues from patients with endometrial cancer, colorectal cancer, ovarian cancer, prostate cancer, head and neck squamous cell carcinoma, lung adenocarcinoma, esophageal squamous cell carcinoma, and thyroid cancer (20, 29). There is growing evidence that some cancers are critically dependent on OXPHOS, and inhibiting OXPHOS can effectively target specific cancer subtypes. For example, patients with diabetes receiving the anti-diabetic drug metformin have a lower incidence of cancer and a better prognosis than patients not receiving metformin (30, 31). In vitro studies suggest the effect of metformin is mediated by the inhibition of OXPHOS complex I, resulting in decreased ATP production by cancer cells (32).
Since the expression analyses of genes encoding OXPHOS related proteins in mtDNA may not fully reflect the function of OXPHOS, proteomic or metabolomic approaches are required to fully characterize OXPHOS activity. Furthermore, given that mitochondrial ribosomes are responsible for the synthesis of OXPHOS related proteins, relevant studies targeting the expression of genes encoding mitochondrial ribosomal proteins and the function of MRPs should not be overlooked.
2.3 MRPs and Apoptosis
One of the hallmarks of tumorigenesis and tumor progression is the evasion of apoptosis in cancer cells, and traditional chemoradiotherapy largely relies on the induction of cancer cell apoptosis. The mitochondrial pathway is an important apoptosis pathway and is regulated by pro-apoptotic factors (such as Bax and Bak) as opposed to anti-apoptotic factors (such as Bcl-2 and Bcl-xL) from the Bcl-2 (B-cell Lymphoma 2) protein family (33, 34). Subsequent mitochondrial outer membrane permeabilization (MOMP) and the release of mitochondrial pro-apoptotic proteins (cytochrome c, SMAC, and OMI) into the cytoplasm results in the activation of the caspase family of proteases, which promote apoptosis via a cascade of reactions, leading to cell death (35, 36). Among the mitochondrial ribosomal proteins, MRPL41 (mL41), MRPS29 (mS29), and MRPS30 (mS30) have been implicated in the regulation of apoptosis.
In human cells, Bcl-2 blocks the apoptosis-inducing ability of MRPL41 (mL41) via multiple mechanisms, and amino acid residues 13–17 of mL41 are essential for binding to Bcl-2; accordingly, mL41 has also been named BCL-2-interacting mitochondrial ribosomal protein (BMRP). MRPS29 (mS29), originally also known as death-associated protein 3 (DAP3), is strongly associated with the Fas receptor-associated death-inducing signaling complex. MRPS30 (mS30) is also known as programmed cell death protein 9 (PDCD9) (37). Although the roles of these three MRPs in the regulation of apoptosis are well-established, studies of their roles in cancer biology are limited. We utilized the visualization tool UALCAN (http://ualcan.path.uab.edu/index.html (38).) to analyze the gene expression data for these three genes from The Cancer Genome Atlas (Figure 3). These three representative genes are highly expressed in many malignancies, such as lung and breast cancer (p < 0.01) and can be intensively studied as potential tumor biomarkers. The expression of gene MRPS29 is higher in TCGA gastric adenocarcinoma samples than in controls (normal n = 34; primary tumor n = 415), consistent with the published conclusion of Jia y (40). Interestingly, they are not always highly expressed in cancer. For example, MRPL41 and MRPS30 are under expressed in renal clear cell carcinoma (p < 0.01), which may be associated with tumor heterogeneity, but requires further investigation.
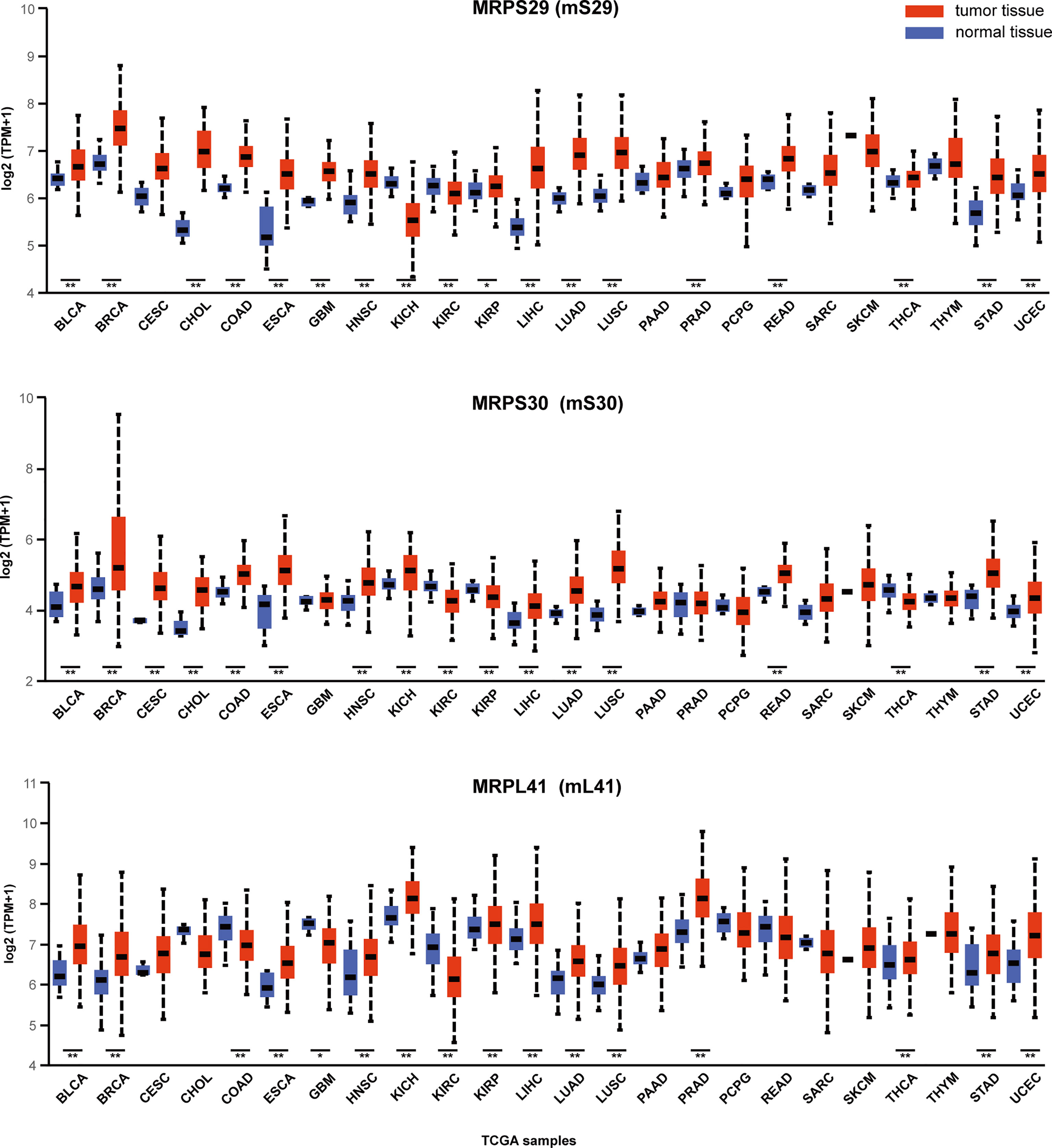
Figure 3 Expression of MRPS29, MRPS30, MRPL41 across TCGA cancers. Three representative mitochondrial ribosomal genes are differentially expressed in cancer. TPM is the normalization of gene reads derived from high-throughput sequencing (39). (*p < 0.05; **p < 0.01).TPM, Transcripts Per Kilobase Million; BLCA, Bladder urothelial carcinoma; BRCA, Breast invasive carcinoma; CESC, Cervical squamous cell carcinoma; CHOL, Cholangiocarcinoma; COAD, Colon adenocarcinoma; ESCA, Esophageal carcinoma; GBM, Glioblastoma multiforme; HNSC, Head and Neck squamous cell carcinoma; KICH, Kidney Chromophobe; KIRC, Kidney renal clear cell carcinoma; KIRP, Kidney renal papillary cell carcinoma; LIHC, Liver hepatocellular carcinoma; LUAD, Lung adenocarcinoma; LUSC, Lung squamous cell carcinoma; PAAD, Pancreatic adenocarcinoma; PRAD, Prostate adenocarcinoma; PCPG, Pheochromocytoma and Paraganglioma; READ, Rectum adenocarcinoma; SARC, Sarcoma; SKCM, Skin Cutaneous Melanoma; THCA, Thyroid carcinoma; THYM, Thymoma; STAD, Stomach adenocarcinoma; UCEC, Uterine Corpus Endometrial Carcinoma.
3 Bioinformatics, Mitochondrial Ribosomes, and Malignancy
In the early 1960s, bioinformatics was developed along with advances in molecular biology and computer science. In the 2000s, major innovations in sequencing technology as well as decreasing costs led to the arrival of the “big data” era, with bioinformatics data mining and management forming a new area of expertise (41). Bioinformatics analytics based on next-generation sequencing aim to apply advanced computational tools and databases to convert sequencing signals into data with interpretable information. The whole analysis process can be simplified into three steps. The first step consists of processing the raw sequencing instrument signal into nucleotide bases and short-read data (42). The second step consists of alignment to the reference sequence or de novo assembly of nucleotide reads and subsequent variant detection, etc. (43). The third step relates sample-specific genomic profiles to disparate descriptive annotations, followed by statistical analysis and visualization. A comprehensive review (with classification and description) of major DNA, RNA, and protein related bioinformatics databases with publicly available tools was performed by Chen etc. (44–47).
With respect to bioinformatics and cancer, valuable databases have been established. For example, the Gene Expression Omnibus (GEO http://www.ncbi.nlm.nih.gov/geo/summary/) is a widely used database supported by the National Center for Biotechnology Information (NCBI), which primarily hosts global gene expression data for access by the research community (48). The Cancer Genome Atlas (TCGA http://cancergenome.nih.gov/abouttcga) is the landmark cancer genomics program initiated and funded by the National Institutes of Health to molecularly characterize more than 20,000 primary cancers with the goal of improving cancer diagnosis, treatment, and prognosis prediction (49, 50). The massive genomic, epigenomic, transcriptomic, and proteomic data generated from sequencing are all available for subsequent bioinformatics analyses. TCGA data are based on microarrays (to test nucleic acids and proteins) and next-generation sequencing (for global analyses of nucleic acids), including but not limited to RNA sequencing, DNA sequencing, and reverse-phase protein arrays.
To meet the demand for multi-dimensional comprehensive analyses of massive genomic data, advanced visual analysis methods, such as weighted gene co-expression network analysis (WGCNA), have been developed. New advances in the field of mitochondrial ribosomes and malignancies using common high-throughput sequencing and bioinformatics techniques, coupled with in vitro and in vivo experimental validation, are described below. We have produced two Tables for readers’ access based on the findings mentioned below (Tables 1, 2).
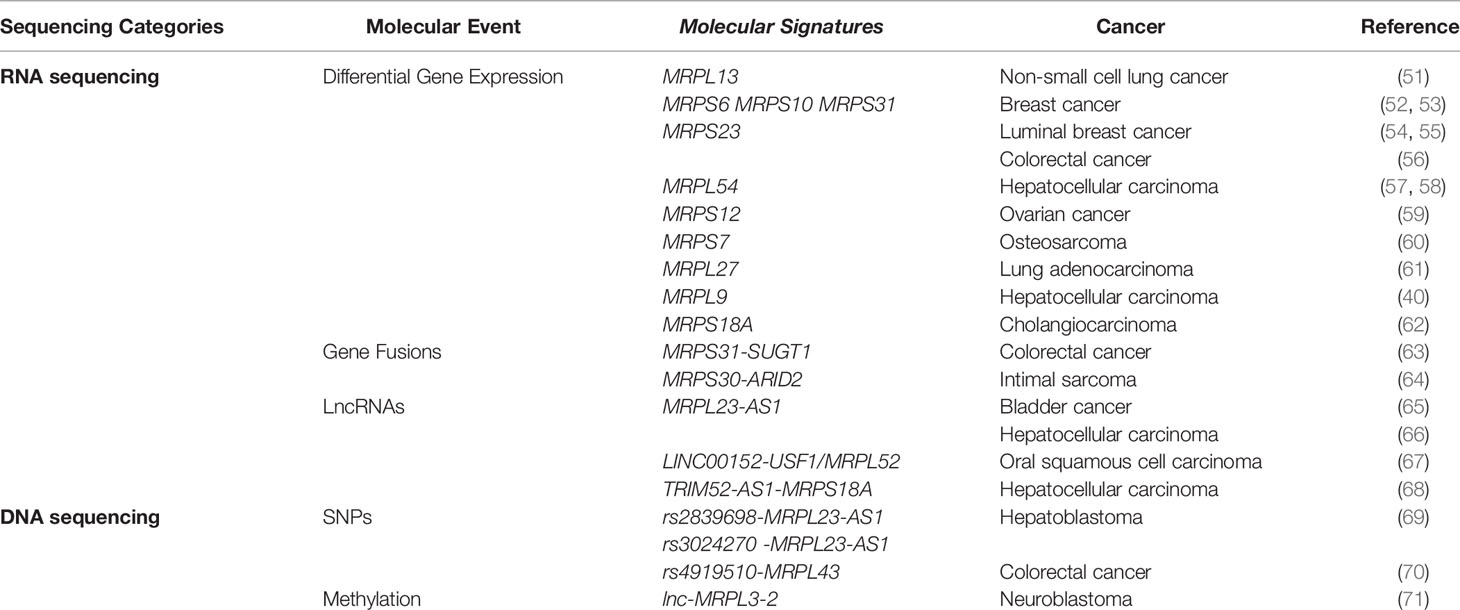
Table 1 Association between mitochondrial ribosomal genes and cancer revealed by high-throughput sequencing.
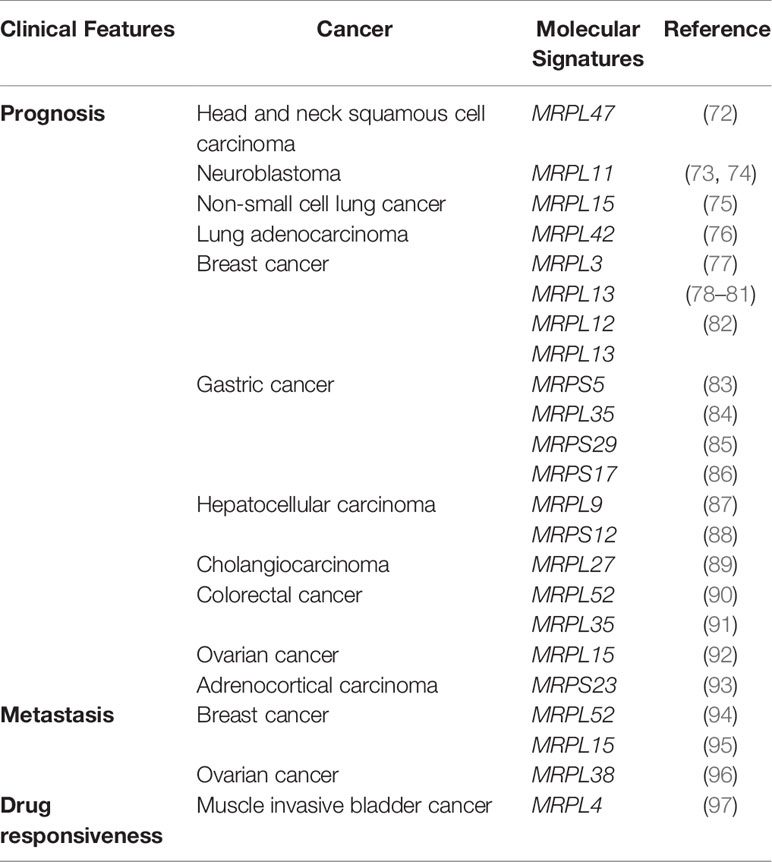
Table 2 Association between mitochondrial ribosomal genes and clinical features of cancer revealed by bioinformatics analyses.
3.1 RNA Sequencing
RNA sequencing (RNAseq) can be used to rapidly identify and quantify rare and common transcripts across a broad range of samples, providing information regarding gene expression, gene fusions, non-coding RNAs, exons, etc. (98).
3.1.1 Differential Gene Expression
Bioinformatic data analyses combined with in vitro and in vivo experimental results have revealed that MRPL13 is highly expressed in non-small cell lung cancer tissues and cell lines and can promote cancer cell proliferation; the locus is, therefore, an independent tumor marker and candidate therapeutic target (51). Expression levels of MRPS6, MRPS10, MRPS23, and MRPS31 are significantly elevated in breast cancer cells and tissues, and these findings have been corroborated by corresponding cell-based functional assay results (52, 53). In addition, MRPS23 has been identified as a driver of proliferation in luminal breast cancer, as supported by a series of in vitro and in vivo studies (54, 55). The depletion of MRPS29 functions via the β-Catenin/Lgr5/Bcl-2 axis to induce drug resistance in gastric cancer cells (99). MRPL54, EZH2, PPARGC1A, and EIF2AK4 were identified as hub genes in bioinformatics analysis of hepatocellular carcinoma (HCC). RNA sequence data extracted from TCGA, and the gene signatures based on these loci showed good predictive ability for HCC prognosis (57, 58). Using a similar data mining approach, 12 genes, including MRPS23, were identified as promising predictors with an important role in the pathogenesis of colorectal cancer (CRC) (56). MRPS12 expression is significantly higher in ovarian cancer than in normal ovarian tissues and is positively correlated with the infiltration of macrophages and neutrophils (59). Another bioinformatics analysis using GEO data revealed seven differentially expressed genes related to osteosarcoma metastasis, including MRPS7, but further experimental studies are still needed to elucidate the biological functions and mechanisms of action of these genes (60).
3.1.2 Gene Fusions
Structural chromosomal rearrangements may result in the exchange of coding or regulatory DNA sequences between genes, and such gene fusions are strong driver mutations in the formation of malignant tumors, providing fundamental insights into mechanisms underlying tumorigenesis (100).
Fusion genes and epigenetic regulators (microRNAs, long non-coding RNAs, etc.) constitute an important part of the genomic landscape of tumors, including roles in CRC occurrence and progression. A tumor-specific gene fusion named MRPS31-SUGT1, generated by an intrachromosomal translocation on chromosome 13 and whose sequence was 100% identical to that of the lncRNA MRPS31P5, was discovered. MRPS31P5 may be an important gene expression regulator and is a potential biomarker for detecting early CRC events (63). Intimal sarcoma is a rare, histologically heterogeneous tumor that usually originates from the pulmonary artery. Using data from the American Association for Cancer Research Project Genomics Evidence Neoplasia Information Exchange (AACRP GENIE) database, Roszik characterized genetic alterations in 13 patients with intimal sarcoma and their clinical value and identified genomic rearrangement events, especially the fusion of MRPS30-ARID2 and PDE4DIP-NOTCH2 (64).
3.1.3 Non-Coding RNAs
It is estimated that more than 90% of mammalian genomes are transcribed as non-coding RNAs. Long non-coding RNAs (lncRNAs) are broadly defined as non-coding RNA molecules longer than 200 nucleotides and lack the ability to encode proteins due to the lack of open reading frames (101, 102). LncRNAs located in nuclei regulate the expression of downstream target genes through epigenetic modification (103). The aberrant expression of lncRNAs leads to the development of many diseases such as cancer (104, 105).
lncRNAs have diverse functions in cancer immune responses and the tumor microenvironment. To investigate the immune-related lncRNA (IRlncRNA) signature for predicting bladder cancer (BLCA) prognosis and immunotherapy response, Wu extracted BLCA data from TCGA. Ultimately, eight IRlncRNAs with prognostic significance, including MRPL23-AS1, were identified as immunotherapy-related biomarkers (65). A recent study of oral squamous cell carcinoma has revealed a novel LINC00152-USF1/MRPL52 signaling axis that promotes tumor growth. The long intergenic non-protein-coding RNA 152 (LINC00152) was identified as an oncogenic lncRNA in multiple cancers, which physically interacts with upstream transcription factor 1 (USF1) and can bind to the promoter region of MRPL52 and activate its transcription (67). In another study, a risk score system based on five lncRNAs, including MRPL23-AS1, was used to predict the survival of patients with HCC with cirrhosis (66). Moreover, an in-vitro experimental study combined with a luciferase reporter assay revealed that the lncRNA TRIM52-AS1 sponged miR-514a-5p to facilitate HCC progression through increasing MRPS18A expression (68).
3.1.4 CeRNAs
Competing endogenous RNAs (ceRNAs) are transcripts that regulate each other at the post-transcriptional level by competing for shared miRNAs. ceRNA networks, which link the function of protein-coding mRNAs and noncoding RNAs, contribute to the pathogenesis of cancers (106). Furthermore, host microbiota contributes to many diseases, including cancer. Therefore, Xiangzhou Tan constructed a ceRNA network using data from TCGA and GEO databases to identify the mechanisms underlying microbiota-mediated CRC development and progression, among which four gene signatures (MRPL23-AS1, FRMD6-AS2, and LIFR-AS1) were identified as independent prognostic factors for CRC (107).
3.2 DNA Sequencing
DNA sequencing (DNAseq) is used to detect nucleotide alterations, providing DNA-level information, such as insertions, deletions, single nucleotide polymorphisms (SNPs), as well as methylation status (108).
3.2.1 Single Nucleotide Polymorphisms
SNPs are single bases in the genome with variation in a population. SNP-based DNA microarray technology can measure allele-specific copy numbers at many different SNP sites in the genome and can be used to analyze genome-wide cancer-related structural variation (49, 109). H19 is a 3.0 kb highly conserved lncRNA present on human chromosome 11; H19 polymorphisms are associated with increased susceptibility to a variety of cancers. A study of three H19 polymorphisms in 213 patients with hepatoblastoma demonstrated that the rs2839698 and rs3024270 polymorphisms are associated with decreased MRPL23 antisense RNA 1 (MRPL23-AS1) expression, whereas the rs217727 polymorphism is associated with increased MRPL23-AS1 expression (69). Many studies have evaluated the association between the miR-608 rs4919510 polymorphism and CRC susceptibility. The G allele of rs4919510 located in miR-608 is associated with increased expression of MRPL43 in colon tissues (70). Functional experiments have shown that the knockdown of MRPL43 could inhibit growth and promote apoptosis in the CRC HCT-116 cell line. In addition, SNP loci in MRPS30 are associated with breast cancer (110–112).
3.2.2 Methylation
DNA methylation is a covalent modification in which methyl groups are added to the cytosine of CpG islands in the genome by S-adenosylmethionine. As an epigenetic modification, methylation can regulate gene expression without altering the DNA sequence (113). DNA microarray-based high-throughput methylation sequencing provides information on epigenetic changes in the genome. In a study of neuroblastoma with the largest coverage to date, methylation-specific PCR assays for 78 differentially methylated regions revealed associations between event-free survival and five unique regions, including lnc-MRPL3-2; several novel prognostic biomarkers were identified and independently validated (71).
3.3 WGCNA
Weighted Gene Co-Expression Network Analysis (WGCNA) is a bioinformatics approach to determine intergenic associations in microarray samples, involving network construction, module detection, calculation of topological properties, and data simulation; this approach is useful for screening disease biomarkers or potential therapeutic targets (114). Co-expression is a commonly used strategy to analyze correlations. In the correlation network of genes, each node represents a gene, and lines between nodes are used to represent the correlation of the two genes. Traditional unweighted co-expression network calculates the correlation coefficient between two genes directly through a linear correlation function and judge whether there is a correlation between these two genes based on a given threshold of the correlation coefficient (115, 116). In fact, the correlation of two genes is a value that fluctuates from 0 to 1, and the traditional method ignores the originally changing trend, resulting in the unweighted co-expression network losing much information. Therefore, the development team of WGCNA optimized the algorithm. Pearson correlations are taken and then weighted by raising their absolute value to a power (“β” in adjacency function) (117). This operation guarantees the invariance of the correlation relationship and reinforces the level of variation of the correlation coefficient. Through this analysis, co-expressed gene modules can be identified, and furthermore, modules can be correlated with phenotypic data to mine potential mark genes through metrics such as connectivity, module membership (MM), and gene significance (GS) (117).
Using Dai’s study as an example, WGCNA was used to identify central genes associated with the incidence and prognosis of KRAS-mutant lung adenocarcinoma (LUAD). A total of 2590 DEGs were found between 184 LUAD samples of different pathological stages and 59 normal lung tissue samples from TCGA database, and 10 gene modules were identified. Functional analysis of the key modules revealed enrichment of ribosome biogenesis related terms. Survival analysis revealed that the expression of 8 genes, including MRPL27, was positively correlated with poor survival in patients with KRAS-mutant LUAD (61). Tumor mutational burden (TMB) is associated with the efficacy of immunotherapy, but the prognostic role of TMB-related genes in HCC has not been clearly defined. A WGCNA identified MRPL9 as a TMB-specific gene with good prognostic value. A cell-based functional study has shown that the knockdown of MRPL9 could significantly inhibit cell proliferation and migration in HCC (40). Cholangiocarcinoma (CCA), a highly malignant tumor found in biliary epithelial cells, is the second most common primary tumor of the liver. A WGCNA based on mRNA sequencing data and clinical information for patients with CCA (from TCGA) revealed that MRPS18A, CST1, and SCP2 are genes associated with clinical features, such as pathological stage, histological grade, and liver function, as well as overall survival (62).
3.4 Mitochondrial Ribosomes and Clinical Features
TCGA sample collection and processing involved multiple collaborating centers, including Tissue Source Sites (TSSs), Biospecimen Core Resource (BCR), and Data Coordinating Center (DCC) (http://cancergenome.nih.gov/abouttcga/overview). Biological samples (blood and tissue) are collected from eligible cancer patients, who have detailed clinical information. Potential biomarkers are screened by follow-up high-throughput sequencing and bioinformatics analyses related to clinical features, such as prognosis, lesion metastasis, and drug responsiveness.
3.4.1 Prognosis
Hu et al. identified 92 differentially expressed genes from TCGA and developed a gene signature and prognostic risk model based on MRPL47, NCBP2, MKRN3, AZGP1, IGF2BP2, and EZH2, providing a basis for personalized immunotherapy for patients with head and neck squamous cell carcinoma (72). MRPL11 is highly expressed in stage 4 neuroblastoma and is associated with a poor prognosis. Tigecycline, an FDA-approved broad-spectrum antibiotic, may be an indirect therapeutic strategy for neuroblastoma via the dysregulation of MRPL11 (73, 74).
Another study has demonstrated that high MRPL15 expression predicts a worse prognosis in non-small cell lung cancer; it revealed potential regulatory networks and demonstrated a negative correlation with immune infiltration (75). MRPL42 is highly expressed in early-stage lung adenocarcinoma (LUAD) tissues and cell lines and is significantly associated with prognosis. The knockdown of MRPL42 reduces cell proliferation, promotes cell cycle arrest at the G1/S phase, and attenuates the migration and invasion abilities of LUAD cells in vitro (76).
Breast cancer accounts for the largest number of datasets in TCGA. Yin et al. integrated several bioinformatics tools and RNA in situ detection to identify and validate breast cancer-related hub genes and found that the expression of four genes, including MRPL3, were upregulated in tumor tissues and correlated with cancer progression; these genes could serve as diagnostic and prognostic biomarkers (77). As a poor prognostic factor in breast cancer, MRPL13 expression is significantly higher in cancer tissues than in normal tissues (78–80). This gene can promote tumor cell proliferation, migration, and EMT via the PI3K-Akt-mTOR pathway and is, therefore, a candidate therapeutic target and prognostic marker (81). In breast cancer, a three-gene prognostic model based on MRPL12, MRPL13, and POP1 has significant predictive value for survival. Moreover, the downregulation of endogenous MRPL12, MRPL13, or POP1 expression could significantly inhibit the viability and migration of breast cancer cells in vitro (82).
Gastric cancer (GC) is a digestive system disease with high morbidity and mortality; early clinical screening and diagnosis are difficult, and treatment efficacy at a later stage is unsatisfactory. Therefore, it is imperative to find new tumor markers and therapeutic targets for GC. Based on GC data in TCGA and Genotype Tissue Expression (GTEX), aberrantly expressed proteins between normal and cancer tissues were identified, and hub genes, such as MRPS5, were found to be associated with disease-specific survival (83). MRPL35 expression is upregulated in GC and is associated with a poor prognosis. Cell functional studies have revealed that the knockdown of MRPL35 inhibits cell proliferation in GC via related proteins, including PICK1, Bcl-xL, and AGR2 (84). High expression of MRPS29 in GC is associated with a better prognosis, and the downregulation of MRPS29 expression could increase resistance to chemotherapy by inhibiting apoptosis and promoting cell migration, consistent with the pro-apoptotic function of MRPS29 (85). In another study on gastric adenocarcinoma, a model based on seven genes, such as MRPS17, was identified as a reliable prognostic biomarker (86).
HCC is one of the most prevalent neoplasms and the leading cause of cancer-related deaths worldwide. An mRNA expression-based stemness index revealed a three-gene signature, including PTDSS2, MRPL9, and SOCS, as a potential prognostic biomarker for HCC (87). Based on the differentially expressed genes between HBV-related HCC and control specimens, screened by univariate analyses, a signature model based on 11 genes, including MRPS12, was finally developed. This gene signature showed high sensitivity and accuracy for the prediction of 1-, 3-, and 5-year overall survival, disease-free survival, and progression-free interval, with predictive power superior to those of other clinical parameters (88).
Cholangiocarcinoma, a highly malignant tumor found in biliary epithelial cells, is the second most common primary tumor of the liver. A study of 36 patients with cholangiocarcinoma demonstrated that MRPL27 mRNA levels are significantly upregulated in tumor tissues, and patients with high MRPL27 expression had a poorer overall survival and disease-free survival than those of patients with low MRPL27 expression (89).
Additionally, 19 genes, including MRPL52, have been identified from microarray gene expression profiling data for 78 patients with CRC. Further validation using patient cohorts from Australia (n = 185), the United States (n = 114), Denmark (n = 37), and Norway (n = 95) revealed that this 19-gene signature has significantly better predictive value for survival in CRC than the traditional Dukes classification (90). Higher expression of MRPL35 in CRC cells and tissues is associated with shorter overall survival in CRC, and in vitro studies have shown that the downregulation of MRPL35 expression leads to increased production of reactive oxygen species (91).
Ovarian cancer (OC) is a leading cause of death among gynecological cancers, and unique tissue and genetic heterogeneity are huge obstacles to their diagnosis and treatment. Xu et al. screened six MRPs (uL10m, uL15m, bL36m, mL39, bS16m, and mS31) associated with OC, among which MRPL15 (uL15m) was highly expressed and amplified in OC and associated with a poor prognosis. A mechanistic analysis revealed that MRPL15 (uL15m) plays a role in OC via various pathways, including cell cycle, DNA repair, and mTOR 1 signaling (92).
Adrenocortical carcinoma (ACC) is an extremely rare disease, and the current prognostic markers have limitations in identifying patients with poor prognosis. In a previous study, 45 formalin-fixed paraffin-embedded (FFPE) tissues of adrenal tumors were analyzed by liquid chromatography-tandem mass spectrometry (LC-MS/MS) to screen differentially expressed proteins by machine learning algorithms. MRPS23 was found to be significantly associated with survival in ACC, and this finding was validated using the TCGA database; thus, MRPS23 could be considered as a potential prognostic marker (93).
3.4.2 Metastasis
Xinyan Li identified differentially expressed mRNAs in breast tumors with and without metastasis by high-throughput RNA sequencing, revealing that the expression of MRPL52 was upregulated in breast cancer and was significantly associated with aggressive clinicopathological features and a higher risk of metastasis (94). Sotgia combined four mitochondrial proteins (uL15m, HSPD1, UQCRB, and COX17) to generate a compact mitochondrial gene signature that successfully predicts distant metastasis in breast cancer, suggesting that mitochondrial biogenesis should be considered a novel therapeutic target to overcome tumor recurrence, distant metastasis, and treatment failure in breast cancer (95). Studies targeting human OC cell lines SKOV3 and SKOV3.ip1 with different metastatic potential revealed differential expression in 11 genes, such as MRPL38, suggesting that high MRPL38 expression is associated with invasion and metastasis (96). Consistent with these findings, evaluation of data from the Human Protein Atlas revealed that the protein abundance of MRPL38 (mL38) is highly elevated in most tumor types (1).
3.4.3 Drug Responsiveness
Cisplatin-based neoadjuvant chemotherapy (NAC) prior to radical cystectomy is recommended for patients with muscle invasive bladder cancer (MIBC). However, clinically approved biomarkers for predicting the response to NAC are lacking. A study involving 30 MIBC cases reported a new signature gene set, including MRPL4, and was able to select NAC responders with 100% prediction accuracy; this gene set could serve as a promising biomarker for predicting chemo-responsiveness in patients with MIBC (97).
Concluding Remarks
Mitochondrial ribosomes are involved in biological activities via the regulation of energy metabolism and apoptosis. Next-generation sequencing and novel bioinformatics analyses have revealed that mitochondrial ribosomal genes are closely related to malignancy. Given the potential of mitochondrial ribosomal genes as cancer biomarkers, the detection of abnormally expressed mitochondrial ribosomal coding genes and non-coding RNAs is expected to facilitate the early diagnosis of malignant tumors. In the future, therapies that specifically target mitochondrial ribosomes may improve the prognosis of patients with cancers.
Author Contributions
SB, XW, and ML: induction, analysis, writing, and financial support. ZG, DZ, and DS: literature search and curation. LL: guidance and modifications. All authors contributed to the article and approved the submitted version.
Funding
This work was supported by a grant from the National Natural Science Foundation of China (No. 81773523).
Conflict of Interest
The authors declare that the research was conducted in the absence of any commercial or financial relationships that could be construed as a potential conflict of interest.
Publisher’s Note
All claims expressed in this article are solely those of the authors and do not necessarily represent those of their affiliated organizations, or those of the publisher, the editors and the reviewers. Any product that may be evaluated in this article, or claim that may be made by its manufacturer, is not guaranteed or endorsed by the publisher.
Abbreviations
mtDNA, mitochondrial DNA; rRNA, ribosomal RNA; tRNA, transfer RNA; LSU, Large Subunit; SSU, Small Subunit; MRPs, Mitochondrial Ribosomal Proteins; OXPHOS, Oxidative Phosphorylation; DAP3, Death-Associated Protein 3; GEO, Gene Expression Omnibus; TCGA, The Cancer Genome Atlas; WGCNA, Weighted Gene Co-Expression Network Analysis; RNAseq, RNA sequencing; ceRNAs, competing endogenous RNAs; DNAseq, DNA sequencing; HCC, Hepatocellular Carcinoma; CRC, Colorectal Cancer; OC, Ovarian Cancer; BLCA, Bladder Cancer; SNP, Single Nucleotide Polymorphisms.
References
1. Kim HJ, Maiti P, Barrientos A. Mitochondrial Ribosomes in Cancer. Semin Cancer Biol (2017) 47:67–81. doi: 10.1016/j.semcancer.2017.04.004
2. De Silva D, Tu YT, Amunts A, Fontanesi F, Barrientos A. Mitochondrial Ribosome Assembly in Health and Disease. Cell Cycle (2015) 14(14):2226–50. doi: 10.1080/15384101.2015.1053672
3. Bogenhagen DF, Ostermeyer-Fay AG, Haley JD, Garcia-Diaz M. Kinetics and Mechanism of Mammalian Mitochondrial Ribosome Assembly. Cell Rep (2018) 22(7):1935–44. doi: 10.1016/j.celrep.2018.01.066
4. Gopisetty G, Thangarajan R. Mammalian Mitochondrial Ribosomal Small Subunit (MRPS) Genes: A Putative Role in Human Disease. Gene (2016) 589(1):27–35. doi: 10.1016/j.gene.2016.05.008
5. Wazir U, Orakzai MM, Khanzada ZS, Jiang WG, Sharma AK, Kasem A, et al. The Role of Death-Associated Protein 3 in Apoptosis, Anoikis and Human Cancer. Cancer Cell Int (2015) 15:39. doi: 10.1186/s12935-015-0187-z
6. Conde JA, Claunch CJ, Romo HE, Benito-Martin A, Ballestero RP, Gonzalez-Garcia M. Identification of a Motif in BMRP Required for Interaction With Bcl-2 by Site-Directed Mutagenesis Studies. J Cell Biochem (2012) 113(11):3498–508. doi: 10.1002/jcb.24226
7. Hanahan D, Weinberg RA. Hallmarks of Cancer: The Next Generation. Cell (2011) 144(5):646–74. doi: 10.1016/j.cell.2011.02.013
8. Masic I. The Most Influential Scientists in the Development of Medical Informatics (13): Margaret Belle Dayhoff. Acta Inform Med (2016) 24(4):299. doi: 10.5455/aim.2016.24.299-299
9. Christian BE, Spremulli LL. Mechanism of Protein Biosynthesis in Mammalian Mitochondria. Biochim Biophys Acta (2012) 1819(9-10):1035–54. doi: 10.1016/j.bbagrm.2011.11.009
10. Amunts A, Brown A, Toots J, Scheres SHW, Ramakrishnan V. Ribosome. The Structure of the Human Mitochondrial Ribosome. Science (2015) 348(6230):95–8. doi: 10.1126/science.aaa1193
11. Brown A, Amunts A, Bai XC, Sugimoto Y, Edwards PC, Murshudov G, et al. Structure of the Large Ribosomal Subunit From Human Mitochondria. Science (2014) 346(6210):718–22. doi: 10.1126/science.1258026
12. Greber BJ, Bieri P, Leibundgut M, Leitner A, Aebersold R, Boehringer D, et al. Ribosome. The Complete Structure of the 55S Mammalian Mitochondrial Ribosome. Science (2015) 348(6232):303–8. doi: 10.1126/science.aaa3872
13. Greber BJ, Boehringer D, Leibundgut M, Bieri P, Leitner A, Schmitz N, et al. The Complete Structure of the Large Subunit of the Mammalian Mitochondrial Ribosome. Nature (2014) 515(7526):283–6. doi: 10.1038/nature13895
14. Papa S, Martino PL, Capitanio G, Gaballo A, De Rasmo D, Signorile A, et al. The Oxidative Phosphorylation System in Mammalian Mitochondria. Adv Exp Med Biol (2012) 942:3–37. doi: 10.1007/978-94-007-2869-1_1
15. Horten P, Colina-Tenorio L, Rampelt H. Biogenesis of Mitochondrial Metabolite Carriers. Biomolecules (2020) 10(7):1008. doi: 10.3390/biom10071008
16. Chen J, Tsai A, O'Leary SE, Petrov A, Puglisi JD. Unraveling the Dynamics of Ribosome Translocation. Curr Opin Struct Biol (2012) 22(6):804–14. doi: 10.1016/j.sbi.2012.09.004
17. Greber BJ, Boehringer D, Leitner A, Bieri P, Voigts-Hoffmann F, Erzberger JP, et al. Architecture of the Large Subunit of the Mammalian Mitochondrial Ribosome. Nature (2014) 505(7484):515–9. doi: 10.1038/nature12890
18. Ban N, Beckmann R, Cate JH, Dinman JD, Dragon F, Ellis SR, et al. A New System for Naming Ribosomal Proteins. Curr Opin Struct Biol (2014) 24:165–9. doi: 10.1016/j.sbi.2014.01.002
19. Amunts A, Brown A, Bai XC, Llacer JL, Hussain T, Emsley P, et al. Structure of the Yeast Mitochondrial Large Ribosomal Subunit. Science (2014) 343(6178):1485–9. doi: 10.1126/science.1249410
20. Yu M. Generation, Function and Diagnostic Value of Mitochondrial DNA Copy Number Alterations in Human Cancers. Life Sci (2011) 89(3-4):65–71. doi: 10.1016/j.lfs.2011.05.010
21. Yin P, Li Q, Yan C, Liu Y, Liu J, Yu F, et al. Structural Basis for the Modular Recognition of Single-Stranded RNA by PPR Proteins. Nat (2013) 504(7478):168–71. doi: 10.1038/nature12651
22. Manavski N, Guyon V, Meurer J, Wienand U, Brettschneider R. An Essential Pentatricopeptide Repeat Protein Facilitates 5' Maturation and Translation Initiation of Rps3 mRNA in Maize Mitochondria. Plant Cell (2012) 24(7):3087–105. doi: 10.1105/tpc.112.099051
23. Ashton TM, McKenna WG, Kunz-Schughart LA, Higgins GS. Oxidative Phosphorylation as an Emerging Target in Cancer Therapy. Clin Cancer Res (2018) 24(11):2482–90. doi: 10.1158/1078-0432.CCR-17-3070
24. Liu L, Zhang X, Ding H, Liu X, Cao D, Liu Y, et al. Arginine and Lysine Methylation of MRPS23 Promotes Breast Cancer Metastasis Through Regulating OXPHOS. Oncogene (2021) 40(20):3548–63. doi: 10.1038/s41388-021-01785-7
25. Wei Z, Jia J, Heng G, Xu H, Shan J, Wang G, et al. Sirtuin-1/Mitochondrial Ribosomal Protein S5 Axis Enhances the Metabolic Flexibility of Liver Cancer Stem Cells. Hepatology (2019) 70(4):1197–213. doi: 10.1002/hep.30622
26. Lee YK, Lim JJ, Jeoun UW, Min S, Lee EB, Kwon SM, et al. Lactate-mediated mitoribosomal defects impair mitochondrial oxidative phosphorylation and promote hepatoma cell invasiveness. J Biol Chem. (2017) 292(49):20208–17. doi: 10.1074/jbc.M117.809012
27. Zu XL, Guppy M. Cancer Metabolism: Facts, Fantasy, and Fiction. Biochem Biophys Res Commun (2004) 313(3):459–65. doi: 10.1016/j.bbrc.2003.11.136
28. Vaupel P, Mayer A. Availability, Not Respiratory Capacity Governs Oxygen Consumption of Solid Tumors. Int J Biochem Cell Biol (2012) 44(9):1477–81. doi: 10.1016/j.biocel.2012.05.019
29. Reznik E, Miller ML, Senbabaoglu Y, Riaz N, Sarungbam J, Tickoo SK, et al. Mitochondrial DNA Copy Number Variation Across Human Cancers. Elife (2016) 5:e10769. doi: 10.7554/eLife.10769
30. Koritzinsky M. Metformin: A Novel Biological Modifier of Tumor Response to Radiation Therapy. Int J Radiat Oncol Biol Phys (2015) 93(2):454–64. doi: 10.1016/j.ijrobp.2015.06.003
31. Pernicova I, Korbonits M. Metformin–mode of Action and Clinical Implications for Diabetes and Cancer. Nat Rev Endocrinol (2014) 10(3):143–56. doi: 10.1038/nrendo.2013.256
32. Wheaton WW, Weinberg SE, Hamanaka RB, Soberanes S, Sullivan LB, Anso E, et al. Metformin Inhibits Mitochondrial Complex I of Cancer Cells to Reduce Tumorigenesis. Elife (2014) 3:e02242. doi: 10.7554/eLife.02242
33. Warren CFA, Wong-Brown MW, Bowden NA. BCL-2 Family Isoforms in Apoptosis and Cancer. Cell Death Dis (2019) 10(3):177. doi: 10.1038/s41419-019-1407-6
34. Edlich F. BCL-2 Proteins and Apoptosis: Recent Insights and Unknowns. Biochem Biophys Res Commun (2018) 500(1):26–34. doi: 10.1016/j.bbrc.2017.06.190
35. Dadsena S, King LE, Garcia-Saez AJ. Apoptosis Regulation at the Mitochondria Membrane Level. Biochim Biophys Acta Biomembr (2021) 1863(12):183716. doi: 10.1016/j.bbamem.2021.183716
36. Bock FJ, Tait SWG. Mitochondria as Multifaceted Regulators of Cell Death. Nat Rev Mol Cell Biol (2020) 21(2):85–100. doi: 10.1038/s41580-019-0173-8
37. Cavdar Koc E, Ranasinghe A, Burkhart W, Blackburn K, Koc H, Moseley A, et al. A New Face on Apoptosis: Death-Associated Protein 3 and PDCD9 are Mitochondrial Ribosomal Proteins. FEBS Lett (2001) 492(1-2):166–70. doi: 10.1016/S0014-5793(01)02250-5
38. Chandrashekar DS, Bashel B, Balasubramanya SAH, Creighton CJ, Ponce-Rodriguez I, Chakravarthi B, et al. UALCAN: A Portal for Facilitating Tumor Subgroup Gene Expression and Survival Analyses. Neoplasia (2017) 19(8):649–58. doi: 10.1016/j.neo.2017.05.002
39. Vera Alvarez R, Pongor LS, Marino-Ramirez L, Landsman D. TPMCalculator: One-Step Software to Quantify mRNA Abundance of Genomic Features. Bioinformatics (2019) 35(11):1960–2. doi: 10.1093/bioinformatics/bty896
40. Tang B, Zhu J, Zhao Z, Lu C, Liu S, Fang S, et al. Diagnosis and Prognosis Models for Hepatocellular Carcinoma Patient's Management Based on Tumor Mutation Burden. J Adv Res (2021) 33:153–65. doi: 10.1016/j.jare.2021.01.018
41. Gauthier J, Vincent AT, Charette SJ, Derome N. A Brief History of Bioinformatics. Brief Bioinform (2019) 20(6):1981–96. doi: 10.1093/bib/bby063
42. Moorthie S, Hall A, Wright CF. Informatics and Clinical Genome Sequencing: Opening the Black Box. Genet Med (2013) 15(3):165–71. doi: 10.1038/gim.2012.116
43. Horner DS, Pavesi G, Castrignano T, De Meo PD, Liuni S, Sammeth M, et al. Bioinformatics Approaches for Genomics and Post Genomics Applications of Next-Generation Sequencing. Brief Bioinform (2010) 11(2):181–97. doi: 10.1093/bib/bbp046
44. Hong M, Tao S, Zhang L, Diao LT, Huang X, Huang S, et al. RNA Sequencing: New Technologies and Applications in Cancer Research. J Hematol Oncol (2020) 13(1):166. doi: 10.1186/s13045-020-01005-x
45. Kukurba KR, Montgomery SB. RNA Sequencing and Analysis. Cold Spring Harb Protoc (2015) 2015(11):951–69. doi: 10.1101/pdb.top084970
46. Chen C, Huang H, Wu CH. Protein Bioinformatics Databases and Resources. Methods Mol Biol (2017) 1558:3–39. doi: 10.1007/978-1-4939-6783-4_1
47. Oliver GR, Hart SN, Klee EW. Bioinformatics for Clinical Next Generation Sequencing. Clin Chem (2015) 61(1):124–35. doi: 10.1373/clinchem.2014.224360
48. Clough E, Barrett T. The Gene Expression Omnibus Database. Methods Mol Biol (2016) 1418:93–110. doi: 10.1007/978-1-4939-3578-9_5
49. Tomczak K, Czerwinska P, Wiznerowicz M. The Cancer Genome Atlas (TCGA): An Immeasurable Source of Knowledge. Contemp Oncol (Pozn) (2015) 19(1A):A68–77. doi: 10.5114/wo.2014.47136
50. Chin L, Andersen JN, Futreal PA. Cancer Genomics: From Discovery Science to Personalized Medicine. Nat Med (2011) 17(3):297–303. doi: 10.1038/nm.2323
51. Jing C, Fu R, Wang C, Li X, Zhang W. MRPL13 Act as a Novel Therapeutic Target and Could Promote Cell Proliferation in Non-Small Cell Lung Cancer. Cancer Manag Res (2021) 13:5535–45. doi: 10.2147/CMAR.S316428
52. Revathi Paramasivam O, Gopisetty G, Subramani J, Thangarajan R. Expression and Affinity Purification of Recombinant Mammalian Mitochondrial Ribosomal Small Subunit (MRPS) Proteins and Protein-Protein Interaction Analysis Indicate Putative Role in Tumourigenic Cellular Processes. J Biochem (2021) 169(6):675–92. doi: 10.1093/jb/mvab004
53. Oviya RP, Gopal G, Shirley SS, Sridevi V, Jayavelu S, Rajkumar T. Mitochondrial Ribosomal Small Subunit Proteins (MRPS) MRPS6 and MRPS23 Show Dysregulation in Breast Cancer Affecting Tumorigenic Cellular Processes. Gene (2021) 790:145697. doi: 10.1016/j.gene.2021.145697
54. Klaestad E, Opdahl S, Engstrom MJ, Ytterhus B, Wik E, Bofin AM, et al. MRPS23 Amplification and Gene Expression in Breast Cancer; Association With Proliferation and the non-Basal Subtypes. Breast Cancer Res Treat (2020) 180(1):73–86. doi: 10.1007/s10549-020-05532-6
55. Gao Y, Li F, Zhou H, Yang Y, Wu R, Chen Y, et al. Down-Regulation of MRPS23 Inhibits Rat Breast Cancer Proliferation and Metastasis. Oncotarget (2017) 8(42):71772–81. doi: 10.18632/oncotarget.17888
56. Fan X, Liu L, Shi Y, Guo F, Wang H, Zhao X, et al. Integrated Analysis of RNA-Binding Proteins in Human Colorectal Cancer. World J Surg Oncol (2020) 18(1):222. doi: 10.1186/s12957-020-01995-5
57. Wang M, Jiang F, Wei K, Wang J, Zhou G, Wu C, et al. Development and Validation of a RNA Binding Protein-Associated Prognostic Model for Hepatocellular Carcinoma. Technol Cancer Res Treat (2021) 20:15330338211004936. doi: 10.1177/15330338211004936
58. Huang Y, Chen S, Qin W, Wang Y, Li L, Li Q, et al. A Novel RNA Binding Protein-Related Prognostic Signature for Hepatocellular Carcinoma. Front Oncol (2020) 10:580513. doi: 10.3389/fonc.2020.580513
59. Qiu X, Guo D, Du J, Bai Y, Wang F. A Novel Biomarker, MRPS12 Functions as a Potential Oncogene in Ovarian Cancer and is a Promising Prognostic Candidate. Med (Baltimore) (2021) 100(8):e24898. doi: 10.1097/MD.0000000000024898
60. Liu J, Wu S, Xie X, Wang Z, Lei Q. Identification of Potential Crucial Genes and Key Pathways in Osteosarcoma. Hereditas (2020) 157(1):29. doi: 10.1186/s41065-020-00142-0
61. Dai D, Shi R, Han S, Jin H, Wang X. Weighted Gene Coexpression Network Analysis Identifies Hub Genes Related to KRAS Mutant Lung Adenocarcinoma. Med (Baltimore) (2020) 99(32):e21478. doi: 10.1097/MD.0000000000021478
62. Tian A, Pu K, Li B, Li M, Liu X, Gao L, et al. Weighted Gene Coexpression Network Analysis Reveals Hub Genes Involved in Cholangiocarcinoma Progression and Prognosis. Hepatol Res (2019) 49(10):1195–206. doi: 10.1111/hepr.13386
63. Panza A, Castellana S, Biscaglia G, Piepoli A, Parca L, Gentile A, et al. Transcriptome and Gene Fusion Analysis of Synchronous Lesions Reveals Lncmrps31p5 as a Novel Transcript Involved in Colorectal Cancer. Int J Mol Sci (2020) 21(19):7120. doi: 10.3390/ijms21197120
64. Roszik J, Khan A, Conley AP, Livingston JA, Groisberg R, Ravi V, et al. Unique Aberrations in Intimal Sarcoma Identified by Next-Generation Sequencing as Potential Therapy Targets. Cancers (Basel) (2019) 11(9):1283. doi: 10.3390/cancers11091283
65. Wu Y, Zhang L, He S, Guan B, He A, Yang K, et al. Identification of Immune-Related LncRNA for Predicting Prognosis and Immunotherapeutic Response in Bladder Cancer. Aging (Albany NY) (2020) 12(22):23306–25. doi: 10.18632/aging.104115
66. Ye J, Li H, Wei J, Luo Y, Liu H, Zhang J, et al. Risk Scoring System Based on lncRNA Expression for Predicting Survival in Hepatocellular Carcinoma With Cirrhosis. Asian Pac J Cancer Prev (2020) 21(6):1787–95. doi: 10.31557/APJCP.2020.21.6.1787
67. Zou X, Hu X, He F, Zhang M, Kong X, Rui S, et al. LncRNA LINC00152 Promotes Oral Squamous Cell Carcinoma Growth via Enhancing Upstream Transcription Factor 1 Mediated Mitochondrial Ribosomal Protein L52 Transcription. J Oral Pathol Med (2021). doi: 10.1111/jop.13253
68. Zhou C, Chen Z, Peng C, Chen C, Li H. Long Noncoding RNA TRIM52-AS1 Sponges miR-514a-5p to Facilitate Hepatocellular Carcinoma Progression Through Increasing MRPS18A. Cancer Biother Radiopharm (2021) 36(2):211–9. doi: 10.1089/cbr.2019.3271
69. Tan T, Li J, Wen Y, Zou Y, Yang J, Pan J, et al. Association Between lncRNA-H19 Polymorphisms and Hepatoblastoma Risk in an Ethic Chinese Population. J Cell Mol Med (2021) 25(2):742–50. doi: 10.1111/jcmm.16124
70. Zhu X, Liu Y, Xu J, Cheng Z, Yu Y, Chu M, et al. miR-608 Rs4919510 Polymorphism May Affect Susceptibility to Colorectal Cancer by Upregulating MRPL43 Expression. DNA Cell Biol (2020) 39(11):2017–27. doi: 10.1089/dna.2020.5689
71. Decock A, Ongenaert M, Cannoodt R, Verniers K, De Wilde B, Laureys G, et al. Methyl-CpG-Binding Domain Sequencing Reveals a Prognostic Methylation Signature in Neuroblastoma. Oncotarget (2016) 7(2):1960–72. doi: 10.18632/oncotarget.6477
72. Hu G, Jiang Q, Liu L, Peng H, Wang Y, Li S, et al. Integrated Analysis of RNA-Binding Proteins Associated With the Prognosis and Immunosuppression in Squamous Cell Carcinoma of Head and Neck. Front Genet (2020) 11:571403. doi: 10.3389/fgene.2020.571403
73. Bell JL, Hagemann S, Holien JK, Liu T, Nagy Z, Schulte JH, et al. Identification of RNA-Binding Proteins as Targetable Putative Oncogenes in Neuroblastoma. Int J Mol Sci (2020) 21(14):5098. doi: 10.3390/ijms21145098
74. Hu B, Guo Y. Inhibition of Mitochondrial Translation as a Therapeutic Strategy for Human Ovarian Cancer to Overcome Chemoresistance. Biochem Biophys Res Commun (2019) 509(2):373–8. doi: 10.1016/j.bbrc.2018.12.127
75. Zeng Y, Shi Y, Xu L, Zeng Y, Cui X, Wang Y, et al. Prognostic Value and Related Regulatory Networks of MRPL15 in Non-Small-Cell Lung Cancer. Front Oncol (2021) 11:656172. doi: 10.3389/fonc.2021.656172
76. Jiang W, Zhang C, Kang Y, Yu X, Pang P, Li G, et al. MRPL42 is Activated by YY1 to Promote Lung Adenocarcinoma Progression. J Cancer (2021) 12(8):2403–11. doi: 10.7150/jca.52277
77. Yin J, Lin C, Jiang M, Tang X, Xie D, Chen J, et al. CENPL, ISG20L2, LSM4, MRPL3 are Four Novel Hub Genes and may Serve as Diagnostic and Prognostic Markers in Breast Cancer. Sci Rep (2021) 11(1):15610. doi: 10.1038/s41598-021-95068-6
78. Tao Z, Suo H, Zhang L, Jin Z, Wang Z, Wang D, et al. MRPL13 is a Prognostic Cancer Biomarker and Correlates With Immune Infiltrates in Breast Cancer. Onco Targets Ther (2020) 13:12255–68. doi: 10.2147/OTT.S263998
79. Xu YH, Deng JL, Wang LP, Zhang HB, Tang L, Huang Y, et al. Identification of Candidate Genes Associated With Breast Cancer Prognosis. DNA Cell Biol (2020) 39(7):1205–27. doi: 10.1089/dna.2020.5482
80. Zhou X, Xiao C, Han T, Qiu S, Wang M, Chu J, et al. Prognostic Biomarkers Related to Breast Cancer Recurrence Identified Based on Logit Model Analysis. World J Surg Oncol (2020) 18(1):254. doi: 10.1186/s12957-020-02026-z
81. Cai M, Li H, Chen R, Zhou X. MRPL13 Promotes Tumor Cell Proliferation, Migration and EMT Process in Breast Cancer Through the PI3K-AKT-mTOR Pathway. Cancer Manag Res (2021) 13:2009–24. doi: 10.2147/CMAR.S296038
82. Liu Y, Sun H, Li X, Liu Q, Zhao Y, Li L, et al. Identification of a Three-RNA Binding Proteins (RBPs) Signature Predicting Prognosis for Breast Cancer. Front Oncol (2021) 11:663556. doi: 10.3389/fonc.2021.663556
83. Zhou L, Wu Y, Xin L, Zhou Q, Li S, Yuan Y, et al. Development of RNA Binding Proteins Expression Signature for Prognosis Prediction in Gastric Cancer Patients. Am J Transl Res (2020) 12(10):6775–92.
84. Yuan L, Li JX, Yang Y, Chen Y, Ma TT, Liang S, et al. Depletion of MRPL35 Inhibits Gastric Carcinoma Cell Proliferation by Regulating Downstream Signaling Proteins. World J Gastroenterol (2021) 27(16):1785–804. doi: 10.3748/wjg.v27.i16.1785
85. Jia Y, Ye L, Ji K, Zhang L, Hargest R, Ji J, et al. Death-Associated Protein-3, DAP-3, Correlates With Preoperative Chemotherapy Effectiveness and Prognosis of Gastric Cancer Patients Following Perioperative Chemotherapy and Radical Gastrectomy. Br J Cancer (2014) 110(2):421–9. doi: 10.1038/bjc.2013.712
86. Li J, Zhou W, Wei J, Xiao X, An T, Wu W, et al. Prognostic Value and Biological Functions of RNA Binding Proteins in Stomach Adenocarcinoma. Onco Targets Ther (2021) 14:1689–705. doi: 10.2147/OTT.S297973
87. Liu J, Lu J, Li W. A Comprehensive Prognostic and Immunological Analysis of a New Three-Gene Signature in Hepatocellular Carcinoma. Stem Cells Int (2021) 2021:5546032. doi: 10.1155/2021/5546032
88. Li M, Liu Z, Wang J, Liu H, Gong H, Li S, et al. Systematic Analysis Identifies a Specific RNA-Binding Protein-Related Gene Model for Prognostication and Risk-Adjustment in HBV-Related Hepatocellular Carcinoma. Front Genet (2021) 12:707305. doi: 10.3389/fgene.2021.707305
89. Zhuang L, Meng Z, Yang Z. MRPL27 Contributes to Unfavorable Overall Survival and Disease-Free Survival From Cholangiocarcinoma Patients. Int J Med Sci (2021) 18(4):936–43. doi: 10.7150/ijms.50782
90. Abdul Aziz NA, Mokhtar NM, Harun R, Mollah MM, Mohamed Rose I, Sagap I, et al. A 19-Gene Expression Signature as a Predictor of Survival in Colorectal Cancer. BMC Med Genomics (2016) 9(1):58. doi: 10.1186/s12920-016-0218-1
91. Zhang L, Lu P, Yan L, Yang L, Wang Y, Chen J, et al. MRPL35 Is Up-Regulated in Colorectal Cancer and Regulates Colorectal Cancer Cell Growth and Apoptosis. Am J Pathol (2019) 189(5):1105–20. doi: 10.1016/j.ajpath.2019.02.003
92. Xu H, Zou R, Li F, Liu J, Luan N, Wang S, et al. MRPL15 is a Novel Prognostic Biomarker and Therapeutic Target for Epithelial Ovarian Cancer. Cancer Med (2021) 10(11):3655–73. doi: 10.1002/cam4.3907
93. Jang HN, Moon SJ, Jung KC, Kim SW, Kim H, Han D, et al. Mass Spectrometry-Based Proteomic Discovery of Prognostic Biomarkers in Adrenal Cortical Carcinoma. Cancers (Basel) (2021) 13(15):3890. doi: 10.3390/cancers13153890
94. Li X, Wang M, Li S, Chen Y, Wang M, Wu Z, et al. HIF-1-Induced Mitochondrial Ribosome Protein L52: A Mechanism for Breast Cancer Cellular Adaptation and Metastatic Initiation in Response to Hypoxia. Theranostics (2021) 11(15):7337–59. doi: 10.7150/thno.57804
95. Sotgia F, Fiorillo M, Lisanti MP. Mitochondrial Markers Predict Recurrence, Metastasis and Tamoxifen-Resistance in Breast Cancer Patients: Early Detection of Treatment Failure With Companion Diagnostics. Oncotarget (2017) 8(40):68730–45. doi: 10.18632/oncotarget.19612
96. Wang Y, Dong L, Cui H, Shen DH, Wang Y, Chang XH, et al. Up-Regulation of Mitochondrial Antioxidation Signals in Ovarian Cancer Cells With Aggressive Biologic Behavior. J Zhejiang Univ Sci B (2011) 12(5):346–56. doi: 10.1631/jzus.B1000192
97. Hepburn AC, Lazzarini N, Veeratterapillay R, Wilson L, Bacardit J, Heer R. Identification of CNGB1 as a Predictor of Response to Neoadjuvant Chemotherapy in Muscle-Invasive Bladder Cancer. Cancers (Basel) (2021) 13(15):3903. doi: 10.3390/cancers13153903
98. Stark R, Grzelak M, Hadfield J. RNA Sequencing: The Teenage Years. Nat Rev Genet (2019) 20(11):631–56. doi: 10.1038/s41576-019-0150-2
99. Jia Y, Li Z, Cheng X, Wu X, Pang F, Shi J, et al. Depletion of Death-Associated Protein-3 Induces Chemoresistance in Gastric Cancer Cells Through the Beta-Catenin/LGR5/Bcl-2 Axis. J Investig Med (2019) 67(5):856–61. doi: 10.1136/jim-2018-000934
100. Mertens F, Johansson B, Fioretos T, Mitelman F. The Emerging Complexity of Gene Fusions in Cancer. Nat Rev Cancer (2015) 15(6):371–81. doi: 10.1038/nrc3947
101. Spizzo R, Almeida MI, Colombatti A, Calin GA. Long Non-Coding RNAs and Cancer: A New Frontier of Translational Research? Oncogene (2012) 31(43):4577–87. doi: 10.1038/onc.2011.621
102. Derrien T, Johnson R, Bussotti G, Tanzer A, Djebali S, Tilgner H, et al. The GENCODE V7 Catalog of Human Long Noncoding RNAs: Analysis of Their Gene Structure, Evolution, and Expression. Genome Res (2012) 22(9):1775–89. doi: 10.1101/gr.132159.111
103. Fatica A, Bozzoni I. Long non-Coding RNAs: New Players in Cell Differentiation and Development. Nat Rev Genet (2014) 15(1):7–21. doi: 10.1038/nrg3606
104. Xing C, Sun SG, Yue ZQ, Bai F. Role of lncRNA LUCAT1 in Cancer. BioMed Pharmacother (2021) 134:111158. doi: 10.1016/j.biopha.2020.111158
105. Wang J, Su Z, Lu S, Fu W, Liu Z, Jiang X, et al. LncRNA HOXA-AS2 and its Molecular Mechanisms in Human Cancer. Clin Chim Acta (2018) 485:229–33. doi: 10.1016/j.cca.2018.07.004
106. Qi X, Zhang DH, Wu N, Xiao JH, Wang X, Ma W. ceRNA in Cancer: Possible Functions and Clinical Implications. J Med Genet (2015) 52(10):710–8. doi: 10.1136/jmedgenet-2015-103334
107. Tan X, Mao L, Huang C, Yang W, Guo J, Chen Z, et al. Comprehensive Analysis of lncRNA-miRNA-mRNA Regulatory Networks for Microbiota-Mediated Colorectal Cancer Associated With Immune Cell Infiltration. Bioengineered (2021) 12(1):3410–25. doi: 10.1080/21655979.2021.1940614
108. Shendure J, Balasubramanian S, Church GM, Gilbert W, Rogers J, Schloss JA, et al. DNA Sequencing at 40: Past, Present and Future. Nat (2017) 550(7676):345–53. doi: 10.1038/nature24286
109. Van Loo P, Nilsen G, Nordgard SH, Vollan HK, Borresen-Dale AL, Kristensen VN, et al. Analyzing Cancer Samples With SNP Arrays. Methods Mol Biol (2012) 802:57–72. doi: 10.1007/978-1-61779-400-1_4
110. O'Brien KM, Cole SR, Poole C, Bensen JT, Herring AH, Engel LS, et al. Replication of Breast Cancer Susceptibility Loci in Whites and African Americans Using a Bayesian Approach. Am J Epidemiol (2014) 179(3):382–94. doi: 10.1093/aje/kwt258
111. Quigley DA, Fiorito E, Nord S, Van Loo P, Alnaes GG, Fleischer T, et al. The 5p12 Breast Cancer Susceptibility Locus Affects MRPS30 Expression in Estrogen-Receptor Positive Tumors. Mol Oncol (2014) 8(2):273–84. doi: 10.1016/j.molonc.2013.11.008
112. Ellingjord-Dale M, Grotmol T, Lee E, Van Den Berg DJ, Hofvind S, Couto E, et al. Breast Cancer Susceptibility Variants and Mammographic Density Phenotypes in Norwegian Postmenopausal Women. Cancer Epidemiol Biomarkers Prev (2014) 23(9):1752–63. doi: 10.1158/1055-9965.EPI-13-1212
113. Li XQ, Guo YY, De W. DNA Methylation and microRNAs in Cancer. World J Gastroenterol (2012) 18(9):882–8. doi: 10.3748/wjg.v18.i9.882
114. Langfelder P, Horvath S. WGCNA: An R Package for Weighted Correlation Network Analysis. BMC Bioinf (2008) 9:559. doi: 10.1186/1471-2105-9-559
115. Zhang B, Horvath S. A General Framework for Weighted Gene Co-Expression Network Analysis. Stat Appl Genet Mol Biol (2005) 4:Article17. doi: 10.2202/1544-6115.1128
116. Horvath S, Dong J. Geometric Interpretation of Gene Coexpression Network Analysis. PloS Comput Biol (2008) 4(8):e1000117. doi: 10.1371/journal.pcbi.1000117
Keywords: mitochondrial ribosome, bioinformatics, cancer, biomarker, apoptosis, energy metabolism
Citation: Bao S, Wang X, Li M, Gao Z, Zheng D, Shen D and Liu L (2022) Potential of Mitochondrial Ribosomal Genes as Cancer Biomarkers Demonstrated by Bioinformatics Results. Front. Oncol. 12:835549. doi: 10.3389/fonc.2022.835549
Received: 14 December 2021; Accepted: 27 April 2022;
Published: 26 May 2022.
Edited by:
Matiullah Khan, AIMST University, MalaysiaReviewed by:
Sven Dennerlein, University Medical Center Göttingen, GermanyHalina Abramczyk, Lodz University of Technology, Poland
Copyright © 2022 Bao, Wang, Li, Gao, Zheng, Shen and Liu. This is an open-access article distributed under the terms of the Creative Commons Attribution License (CC BY). The use, distribution or reproduction in other forums is permitted, provided the original author(s) and the copyright owner(s) are credited and that the original publication in this journal is cited, in accordance with accepted academic practice. No use, distribution or reproduction is permitted which does not comply with these terms.
*Correspondence: Linlin Liu, 1299935176@qq.com