- 1The First Clinical Medical College, Lanzhou University, Lanzhou, China
- 2Department of Gastroenterology, The First Hospital of Lanzhou University, Lanzhou, China
- 3Gansu Key Laboratory of Gastroenterology, Lanzhou University, Lanzhou, China
- 4Digestive Endoscopic Center, The First Hospital of Lanzhou University, Lanzhou, China
Background: Different matrisomal patterns are shared across carcinomas. However, little is known about whether there exists a unique tumor matrisome that modulates GC progression and immune regulation.
Methods: We conducted a genome-wide analysis based on matrisomal-related lncRNAs (MRLs) in 375 patients with GC from the Cancer Genome Atlas (TCGA) database. Patients were split into the training set and validation set at a ratio of 1:1 using the R package cart. Pearson correlation analysis (PCA) was performed to identify lncRNAs that correlated with matrisome based on differential expression genes. Subsequently, we performed univariate Cox regression analyses and lasso Cox analysis on these lncRNAs to construct a risk model. Considering the primary effect of GRASLND on the GC prognosis, we chose it for further validation in an experimental setting.
Results: We identified a 15-MRL signature to predict overall survival and immune cell infiltration of patients with GC. The AUC values to predict 5-year outcome in three sets were 0.89, 0.65, and 0.78, respectively. Further analyses suggested that the high-risk group showed more obvious immune cell infiltration, and demonstrated an immunologically “cold” profile. In vitro, knockdown of GRASLND could inhibit the invasion capability of GC cells, and downregulate the protein expression of crucial matrisomal-related gene MMP9.
Conclusions: The 15-MRL gene signature might serve as a relatively good predictive tool to manage patients with GC.
Introduction
Gastric cancer (GC) represents one of the most common digestive malignancies worldwide, particularly in East Asia. The number of deaths was 768,793 in 2020 (1). Despite recent advances in GC treatment, a significant proportion of GC patients are diagnosed at the time of later disease progression (2). The outcomes of GC patients still need to be improved. Chemotherapy has been widely applied as a clinical treatment for advanced GC (3). However, these drugs show few satisfactory therapeutic effects due to chemoresistance or other adverse events (4), which limited their further application in some cases. As a promising innovative therapy, immunotherapy provides an effective strategy for the treatment of GC (5). Seeking effective immune therapeutic indicators for GC remains a unique challenge (6). Therefore, we aimed to establish a novel risk model associated with immune infiltration and survival outcome of GC patients based on large cancer datasets.
The extracellular matrix (ECM) consists of a complex network of cross-linked proteins and has been extensively investigated in recent years. Alterations in the composition, ultrastructure, and mechanical properties of ECM elements could have an impact on the phenotype of the cell, thus participating in the tumorigenesis and development of cancers. Long non-coding RNAs (lncRNAs) exert a variety of biological functions through various gene-regulatory mechanisms (7). Aberrant expression of lncRNAs contribute to the progression of cancers, including GC (8). Several lines of evidence have demonstrated that lncRNAs are mainly involved in the regulation of ECM through several crucial ECM-related regulators. Previous studies revealed that TGFBI, as an important player in the ECM, was negatively regulated by lncRNA H19 via the lncRNA H19/miR-675 axis (9, 10). Gu et al. indicated that lncRNA CTD-2589M5.4 could inhibit ovarian cancer progression via regulation of ECM remodeling (11). LINC01089 is a novel conserved lncRNA, which could function as the inhibitor of ECM invasion in breast cancer (Sas-12). Moreover, a recent study has also found that the miR-150-related regulatory axis was involved in biological processes that relied on the ECM in hepatocellular carcinoma (13). Therefore, ECM-related lncRNAs might play crucial roles in tumor progression and immune response. Nevertheless, direct lines of evidence about matrisomal-related lncRNAs in GC are still lacking and need further study.
In this study, we conducted a genome-wide analysis about matrisomal-related lncRNAs and developed a new and robust gene signature based on the TCGA database. This new classification could accurately predict survival outcome and was associated with immune infiltration. Furthermore, several experiments in vitro showed that knockdown of GRASLND could regulate matrisomal-related gene MMP9. This might provide new insights into exploring the regulatory roles of matrisomal-related lncRNAs in GC.
Methods
Data Source
We downloaded high-throughput sequencing profile data of 375 GC patients from the TCGA public database and removed four samples missing clinical follow-up information. LncRNAs and protein-coding RNAs were annotated based on the GENCODE project (https://www.gencodegenes.org/) (14). A total of 1,068 matrisomal-related genes were obtained from the M.I.T. “Matrisome Project” website (www.matrisomeproject.mit.edu/other-resources/human-matrisome). The workflow is illustrated in Figure 1.
Identification of Matrisomal-Related LncRNAs
The Pearson coefficient was used to investigate links between the potential lncRNAs and the matrisomal-related genes. The absolute value of a correlation coefficient of more than 0.4 and a p-value of less than 0.001 were set as threshold values (15–17). The candidate matrisomal-related lncRNAs were considered for subsequent analyses.
Construction of a Matrisomal-Related LncRNA Risk Model
Differentially expressed matrisomal-related lncRNAs (DE-MRLs) between the cancer and normal tissues were firstly obtained based on R package “limma”. Our criteria were set as |log2 fold change (FC)| > 1 and FDR < 0.05. Based on the survival information for patients with GC, we screened out prognostic matrisomal-related lncRNAs (p < 0.001) using univariate Cox regression analysis. Then, GC patients were randomly split into the training and validation set at a ratio of 1:1 using the R package “caret.” We applied lasso analysis in the training set using the R package “glmnet”. A coefficient for each prognostic matrisomal-related lncRNAs was generated accordingly. Multivariate Cox regression analysis was performed to propose the following formula:. GC patients were classified into high- and low-risk groups using the threshold of median value.
Evaluation of the Prognostic Signature
Kaplan–Meier survival analysis was employed to investigate overall survival discrepancy between the different risk groups. Subsequently, receiver operating characteristic (ROC), decision curve analysis (DCA), and concordance index (C-index) were used to evaluate the performance of this prognostic model. Additionally, we constructed a nomogram to predict 1-, 3-, and 5-year survival status. Meanwhile, the calibration curve was performed to evaluate the predictive power of the nomogram.
Immune Cell Infiltration Analysis
Seven immune infiltration prediction algorithms based on RNA-seq were utilized to compare tumor-infiltrating immune cell (TIC) infiltration between the different risk groups. Subsequently, we examined the relationship between the proportions of TICs and candidate matrisomal-related lncRNAs using the CIBERSORT algorithm. Additionally, we performed ssGSEA analysis to quantify the immune regulatory roles. Finally, several common immune checkpoint genes and TIDE score were selected and evaluated between two groups.
Cell Lines and Transfection
Human GC cell lines, AGS and MKN45, were obtained from the Cell Bank of the Chinese Academy of Science. Two cell lines were cultured in RPMI 1640 medium containing 10% fetal bovine serum (FBS: Gibco). GenePharma (Shanghai) synthesized small interfering RNA (siRNA) specific for GRASLND. siRNA was diluted into 100 μl of Opti-MEM Medium, and then transfected into AGS and MKN45 cells using 100 μl of Opti-MEM Medium with Lipofectamine 3000 (Invitrogen, CA, USA). Knockdown efficiency of the siRNA was assessed using quantitative PCR. The sequence was as follows (18):
GRASLND sense-1 GCUUUGACUUAGACUUCUAGC
GRASLND antisense-1 UAGAAGUCUAAGUCAAAGCUU
GRASLND sense-2 CUGUGAUGGUUAAUGUUAAGU
GRASLND antisense-2 UUAACAUUAACCAUCACAGGG
Quantitative Real-Time PCR
TransScript One step gDNA kit (TransGen, China) was utilized to achieve complementary DNA (cDNA) synthesis following the manufacturer’s instructions. RT-PCR was performed using TransStart Top Green qPCR SuperMix (TransGen, China). The genetic expression level was normalized to the internal control GAPDH. The standard 2−ΔΔCt method was utilized to analyze the relative lncRNA abundances. Specific primers utilized in this study are as follows (18):
GRASLND Forward AGGATTCAGGGGATGCACAG
GRASLND Reverse TGGGCTGAAGATGAGACGTT
Invasion Assays
Cell invasion assays were conducted as previously described (19). Matrigel was diluted at a ratio of 1:8 with PBS. After transfection, 1 × 105 cells were added to the upper chamber with 200 μl of serum-free medium. Complete cell culture medium (600 μl) was added to the lower chamber. Then, they were incubated for 48 h at 37°C. Finally, 4% PFA was used to fix all the inserts for 20 min. The inserts were immersed in 0.5% crystal violet for 20 min and quantified using a microscope.
Western Blot Analysis
The procedures of Western blotting were followed as described in previous reports (20). The primary antibody used was MMP9 (1:1,000, CST). The endogenous protein GAPDH (1:1,000, CST) was utilized for normalization.
Results
Identification of Matrisomal-Related LncRNAs in Gastric Cancer
Firstly, we identified 2,256 matrisomal-related lncRNAs (Table S1). Subsequently, we carried out differential gene expression analysis between the tumor and normal tissues, and obtained 912 differential expression non-coding RNAs. Finally, 54 lncRNAs were found to be significantly associated with the OS of GC patients using univariate Cox regression analysis (Figure 2A).
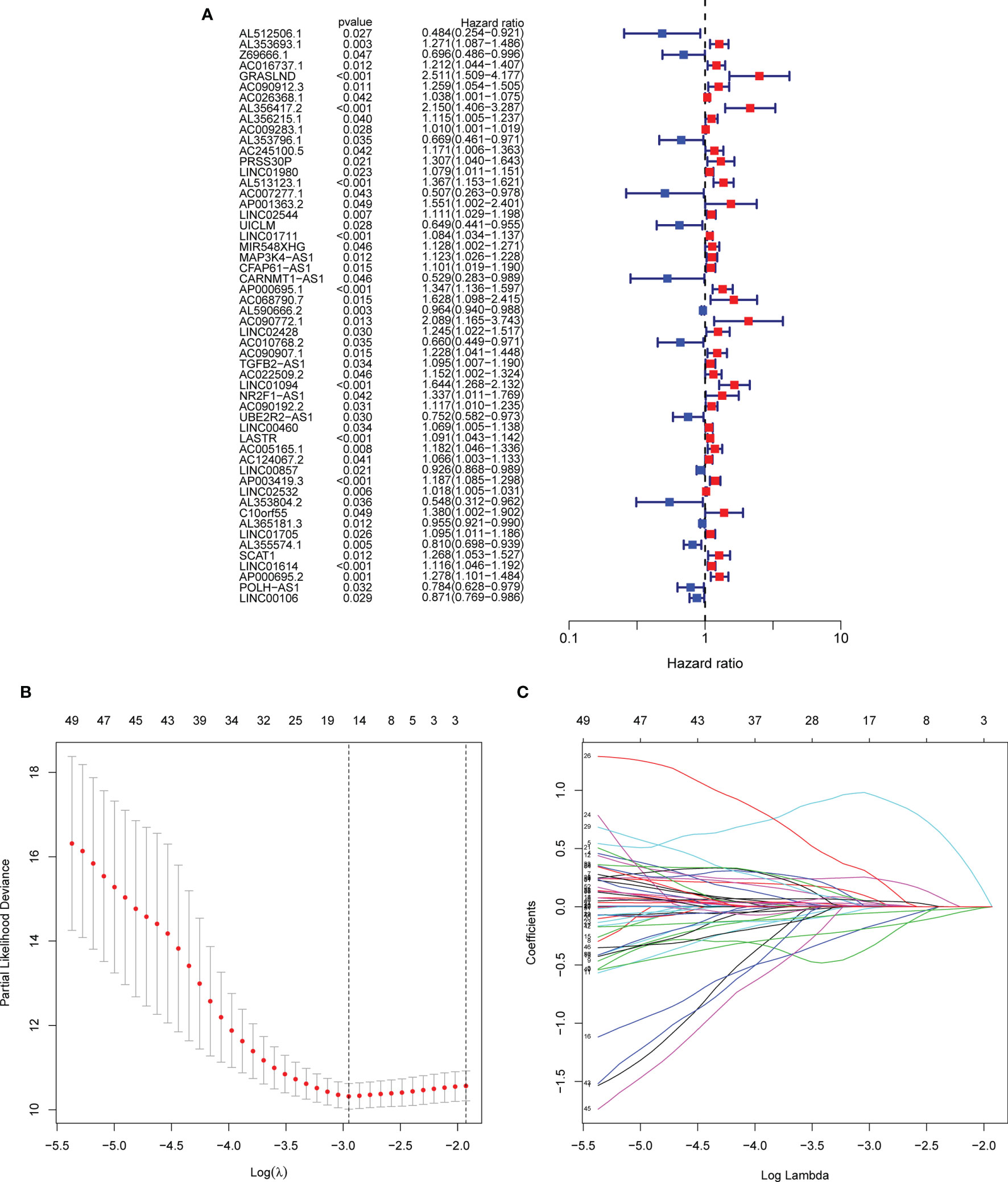
Figure 2 Screening of matrisomal-related lncRNAs and construction of a risk model. (A) Forest plot shows the p-value and the hazard ratio of 54 matrisomal-related lncRNAs via univariate Cox regression analysis. (B). The change trajectory of each independent candidate variable. (C). The optimal model was eventually determined when lambda = 15.
Development and Validation of the Matrisomal-Related LncRNA Risk Model
A total of 371 GC patients were randomly segregated into two sets at a ratio of 1:1. Lasso Cox analysis was further performed in the training set, and a coefficient for each prognostic matrisomal-related lncRNA was generated accordingly. A 15 matrisomal-related lncRNAs model was eventually developed using the coefficient value (Figures 2B, C). The risk score of each sample was as follows:
GC patients were split into two different risk groups based on the median risk value. Kaplan–Meier analysis was employed to investigate OS discrepancy between two groups. As shown in Figures 3A–C, GC patients in the high-risk group exhibit significantly shorter median OS (p < 0.001). Furthermore, we found that patients with high grade, metastasis, and immune score were associated with higher risk scores, suggesting that there exists a potential relationship between the risk score and the immune status (Figures 3D–K).
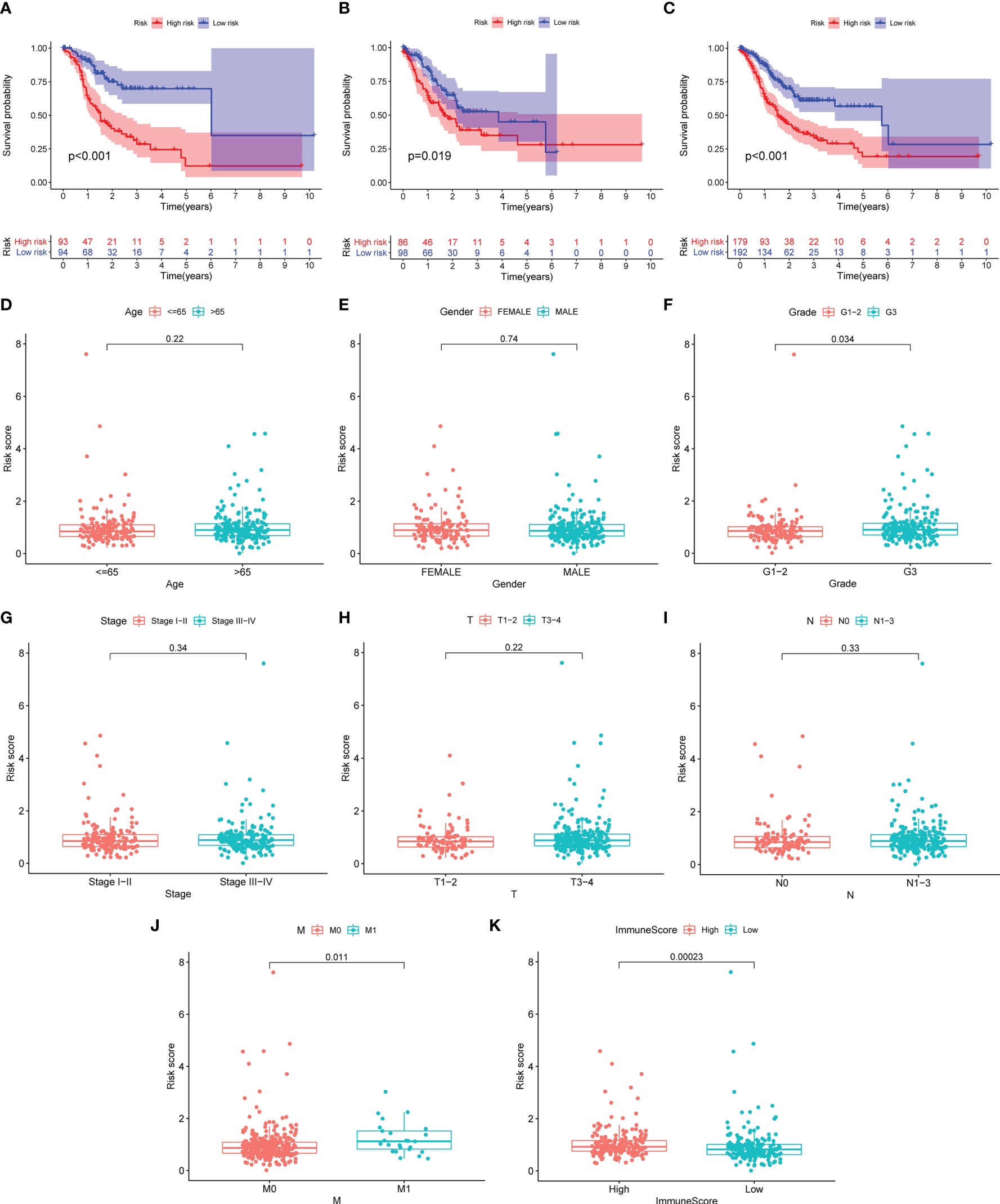
Figure 3 The risk score correlated with overall survival and clinicopathological features. (A–C) Kaplan–Meier curves of GC patients between the high- and low-risk group in the training (A), validation (B), and total (C) set. (D–K) Comparison of risk score distribution between the two groups: age (D), gender (E), G1–2/G3 (F), stage I–II/III–IV (G), T1–2/T3–4 (H), N0/N1–3 (I), M0/M1 (J), and high and low immunescore (K)
Kaplan–Meier Survival Analysis Based on Clinicopathological Characteristics
To assess the prognostic value for established MRL signature, GC patients were split into multiple subgroups based on their clinicopathological characteristics to perform Kaplan–Meier analysis. As shown in Figures 4A–N, the results demonstrated that GC patients in the high-risk group were correlated with worse survival in most groups.
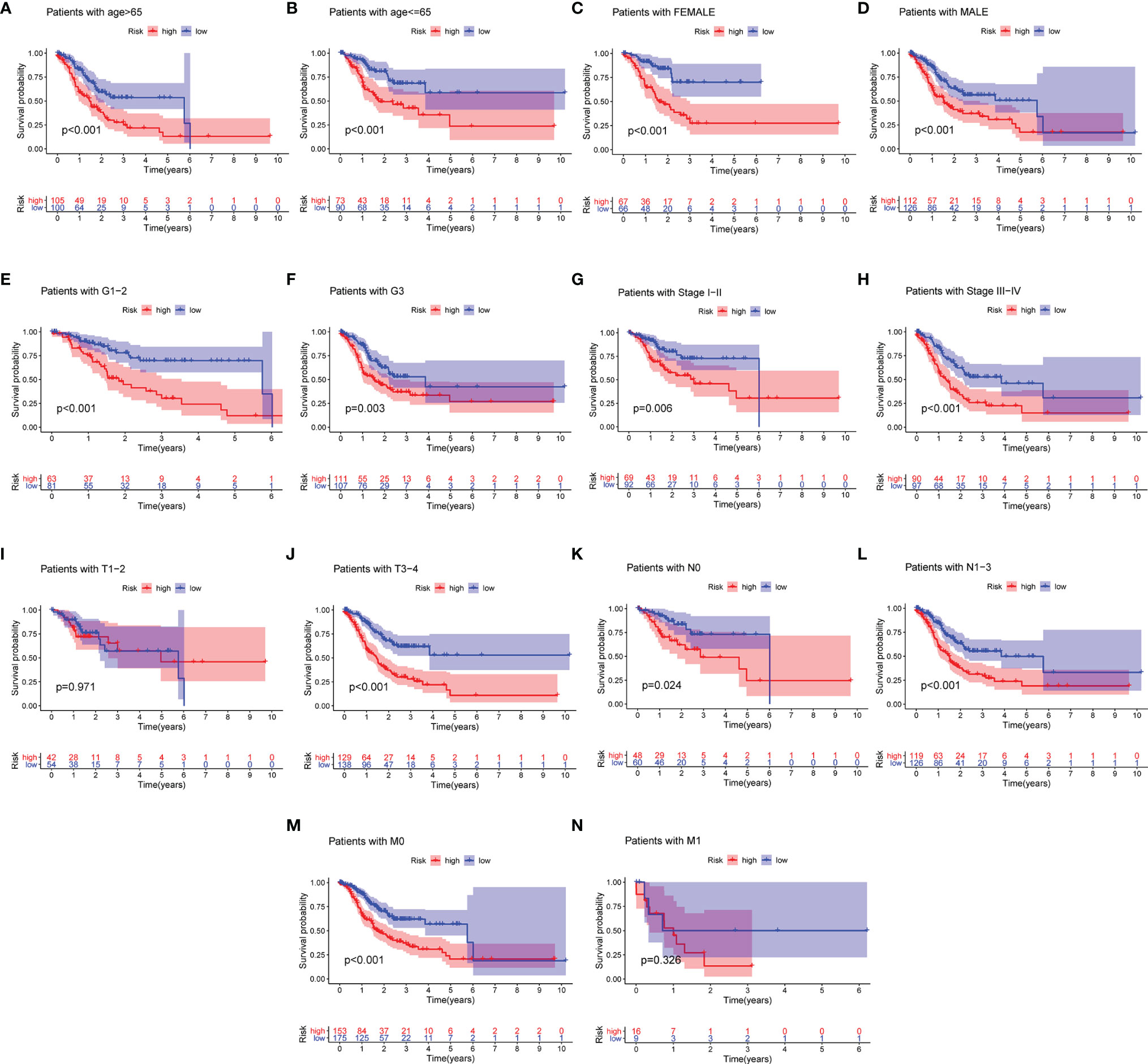
Figure 4 Differential survival outcomes of GC in the clinical subgroup. (A–N) Kaplan–Meier curves for age (A, B), gender (C, D), grade (E, F), stage (G, H), T (I, J), N (K, L), and M (M, N).
Independent Prognostic Value of the Matrisomal-Related LncRNA Signature
Next, we visualized the distribution of risk score, overall survival status, and matrisomal-related lncRNA expression in multiple datasets. We observed that higher risk scores appeared depending on the increasing risk in the training (Figure 5A), validation (Figure 5B), and total (Figure 5C) set. Moreover, the results from two regression analyses indicated that the risk score was closely linked to OS (p < 0.001) after adjusting for age, gender, grade, and stage in the training (Figure 5D), validation (Figure 5E), and total sets (Figure 5F), suggesting that the risk score could be used as a potential independent prognostic variable.
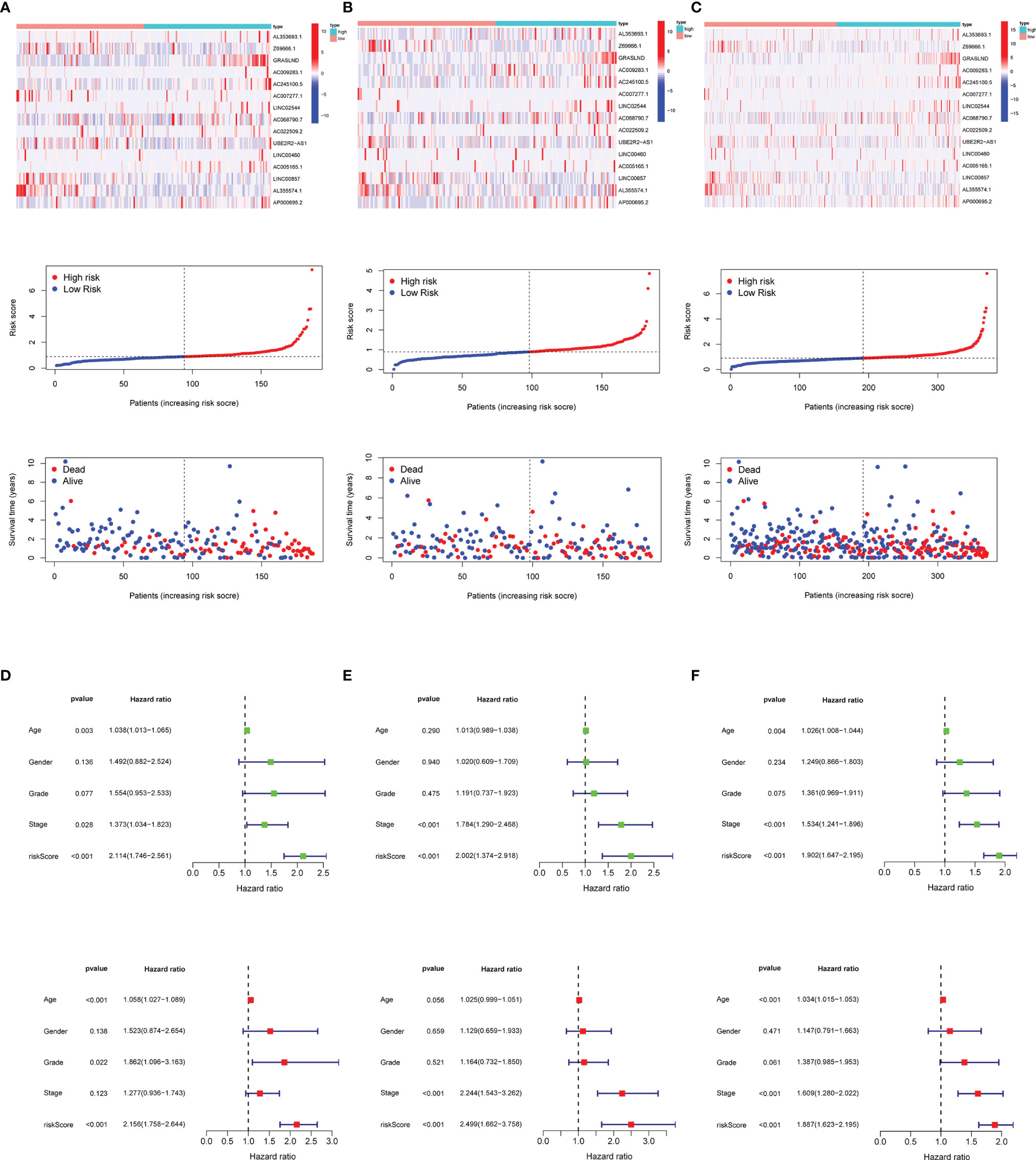
Figure 5 Risk score distribution, survival status, and 15 matrisomal-related lncRNA expression. (A) Training set. (B) Validation set. (C) Total set. Risk score function as an independent prognostic factor. (D) Training set. (E) Validation set. (F) Total set.
Evaluation of the Validity of the Model
The results in Figure 6 showed the accuracy of this signature based on time-dependent ROC curves in three sets. The AUC value for 1, 3, and 5 years was 0.773, 0.794, and 0.893 in the training set (Figure 6A), 0.666, 0.624, and 0.648 in the validation set (Figure 6B), and 0.718, 0.712, and 0.784 (Figure 6C) in the total set, respectively. Moreover, we compared the risk score with multiple clinicopathological features (Figures 6D–F). The risk score in three sets was more effective than other factors in predicting GC prognosis. Finally, we employed C-index (Figure 6G) and DCA (Figure 6H) to assess the performance of the risk model. PCA was further performed to reflect the distribution of the overall samples in different statuses (Figures 7A–D). The results showed that GC samples were distributed more obviously in distinct directions, indicating a good discrimination for the risk model.
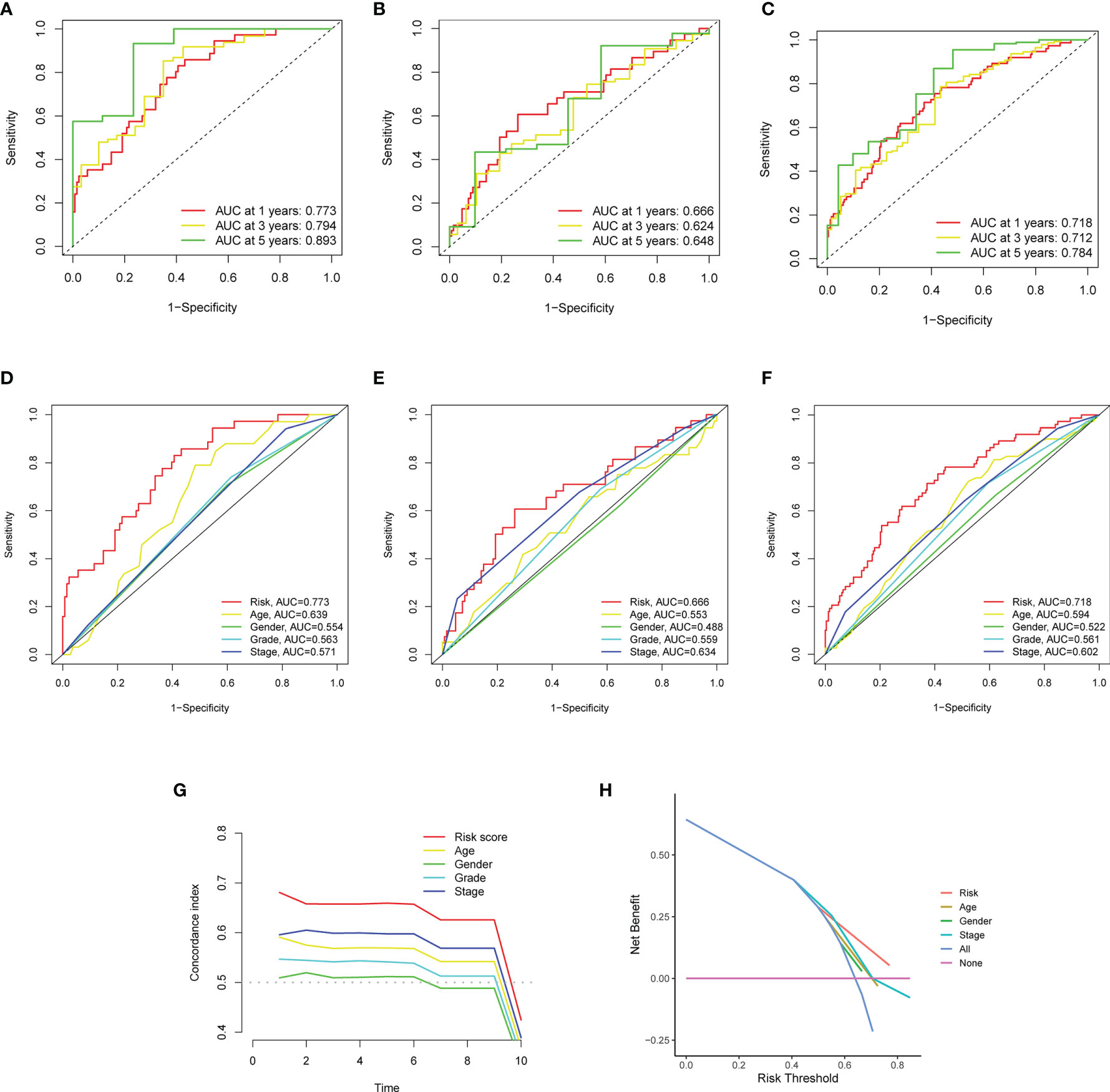
Figure 6 The performance of the matrisomal-related lncRNAs in prognostic prediction. (A–C) Time-dependent ROC analysis for evaluating the sensitivity and specificity of this prognostic model in the training (A), validation (B), and total sets (C). (D–F) Comparison of clinicopathological features and the risk score in the training (D), validation (E), and total sets (F). (G, H) Comparison of clinicopathological features and the risk score in the total sets based on concordance index (G) and decision curve analysis (H).
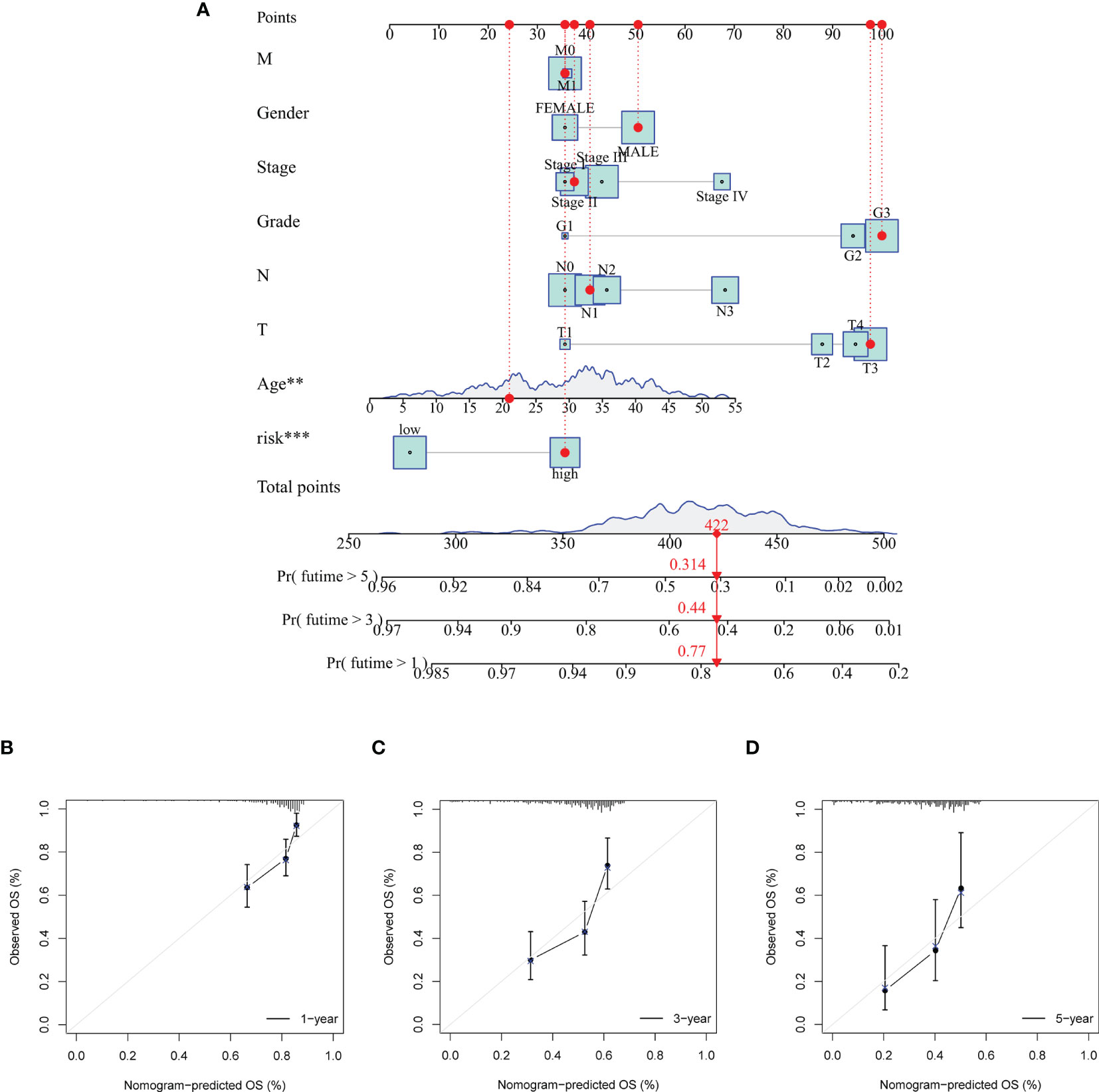
Figure 7 Principal component analysis (PCA) to visualize gene expression patterns in the different groups. The groups defined by all genes (A), matrisomal-related genes (B), matrisomal-related lncRNAs (C), and risk score based on matrisomal-related lncRNAs (D). **p < 0.01, ***p < 0.001
Construction and Validation of the Nomogram
In order to promote the clinical application of the risk model, the nomogram was eventually built to predict 1-, 3-, and 5-year OS. Several clinical variables, including age, gender, grade, stage, T, N, M, and risk score, were used to establish the nomogram (Figure 8A). Moreover, calibration curves were introduced to predict 1-, 3-, and 5-year OS (Figures 8B–D). The stability and accuracy of this hybrid nomogram could plausibly function in the areas of GC management.
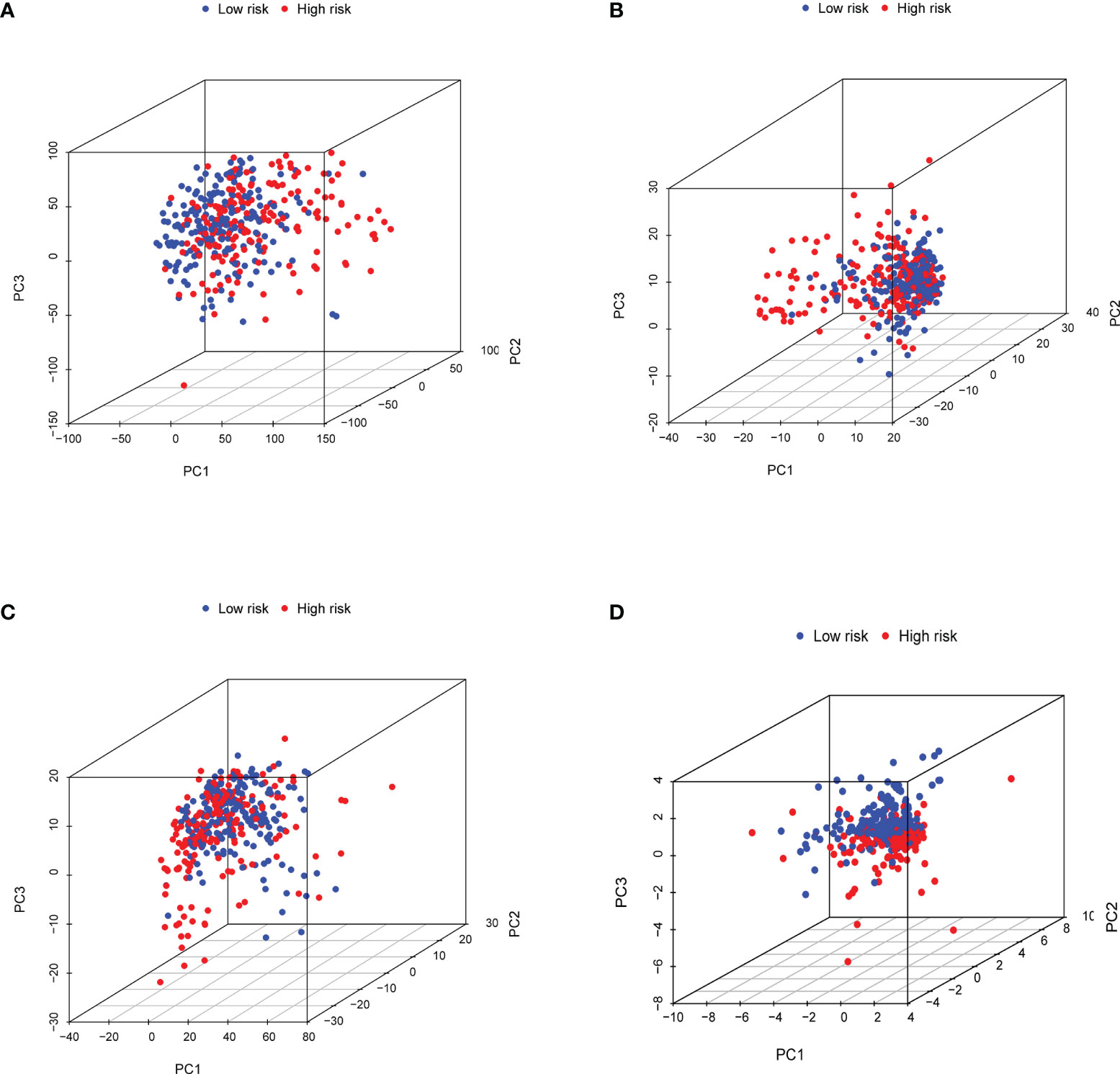
Figure 8 Establishment and validation of a nomogram for predicting the overall survival of GC patients. (A) The nomogram was used for predicting the 1-, 3-, and 5-year OS of patients in the total set. Calibration curves of the nomogram predicting OS of 1 year (B), 3 years (C), and 5 years (D).
Functional Enrichment Analysis of the Risk Signature
To determine potential biological roles that were correlated with risk signature, we performed functional enrichment analysis for DEGs between two groups. A total of 241 DEGs were further identified between the low- and high-risk subgroups. Gene Ontology (GO) analysis consisted of cellular component (CC), molecular function (MF), and biological process (BP). The CC GO terms showed that the top 4 enriched pathways were collagen-containing ECM, contractile fiber, contractile fiber part, and myofibril (Figure 9). The MF GO terms indicated that the top 4 enriched pathways were ECM structural constituent, glycosaminoglycan binding, heparin binding, and sulfur compound binding (Figure 9). The BP GO terms demonstrated that the top 4 enriched pathways were muscle system process, muscle contraction, extracellular structure organization, and ECM organization (Figure 9). These results indicated that the risk signature might be functionally implicated in the regulation of ECM networks.
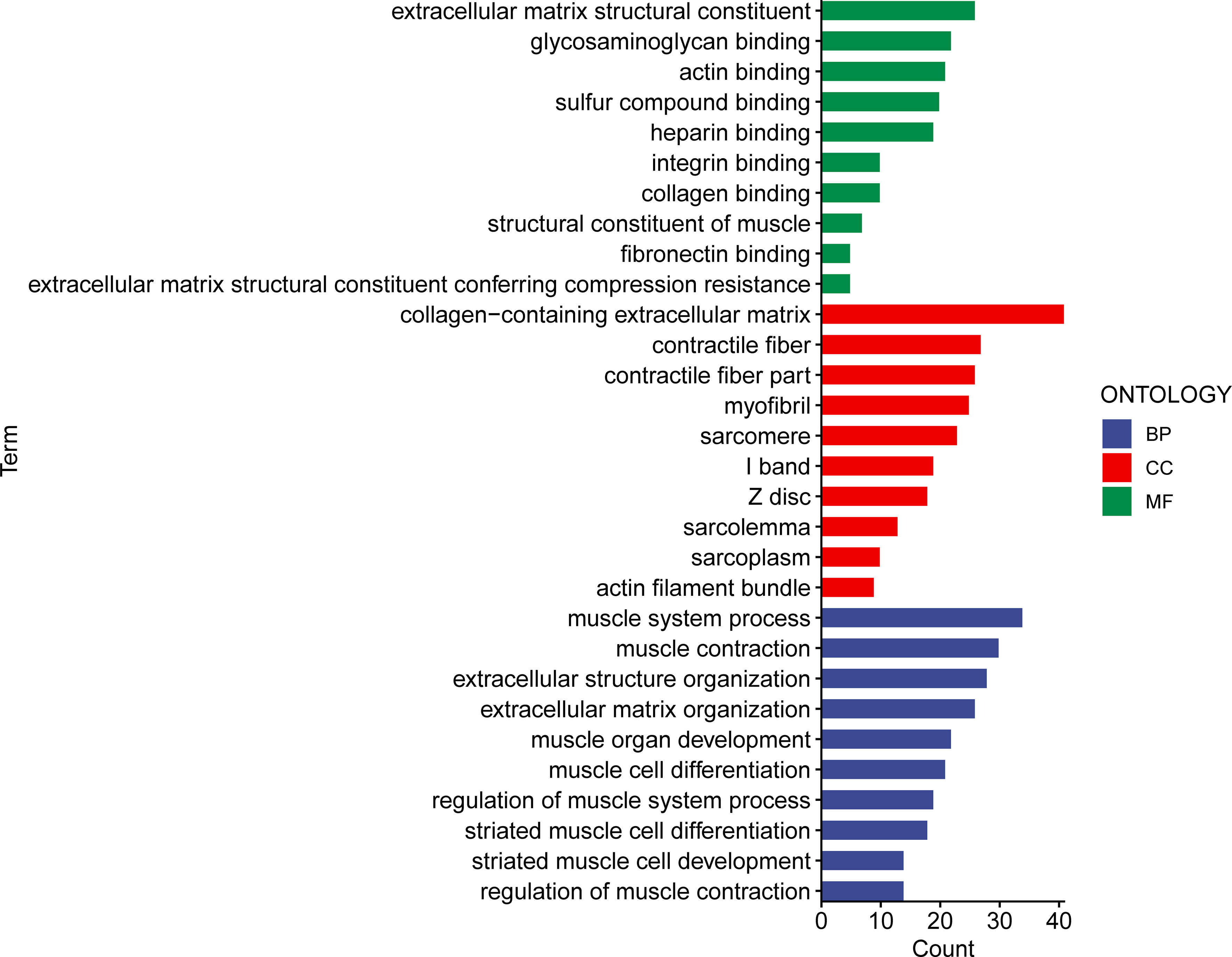
Figure 9 The GO enriched pathways in the different risk subsets obtained from the 15 matrisomal-related lncRNA-based signature.
Functional Enrichment Analysis of the Risk Signature
To confirm the association between the risk score and infiltration of immune cells, we conducted further analysis based on seven algorithms (Figure 10A). The heatmap was used to display the tumor-infiltrating cell proportions in the different groups. As shown in Figure 10A, GC patients in the high-risk group showed higher tumor-infiltrating cell proportions. Macrophage infiltration has been mostly observed in the high-risk group using six algorithms. We further showed the results based on CIBERSORT algorithm as an example, M2 macrophage infiltration was easier to detect in the high-risk group (Figure 10B). The results indicated that the proportions of M2 macrophage in the high-risk group might contribute to immune evasion or tumor-promoting effects. Afterwards, we analyzed the potential correlation between the risk score and immune function. As is shown Figures 10C, D, the contents of the primary antigen presentation process showed higher proportions in the high-risk group. However, lower MHC_class_I was observed in the high-risk group. The results indicated that this might be associated with the immunosuppressive microenvironment. As is seen in Figure 10E, most of the immune checkpoint genes (CD86, CD200, LAIR1, CD44, TNFSF9, PDCD1LG2, NRP1CD276, CD48, and HAVCR2) were dramatically upregulated in the high-risk group. The abnormal expression of genes might contribute to the immunosuppressive microenvironment. To confirm this speculation, we further examined the TIDE score in the different groups. As we expected, our high-risk group showed a higher TIDE score, suggesting that the risk score could discriminate subgroups of GC patients with different immune responses (Figure 10F).
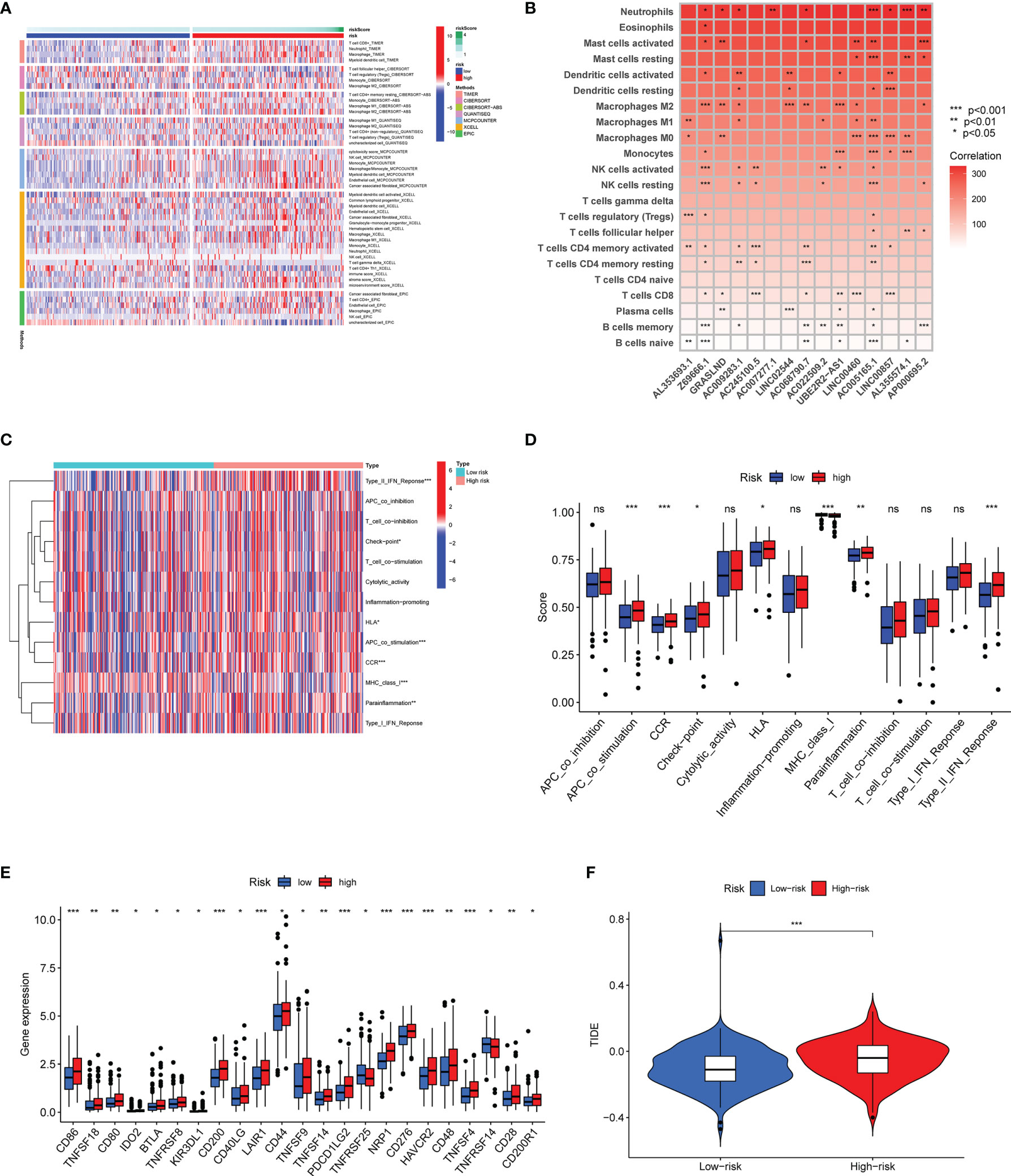
Figure 10 Differential immune features in the high-risk and low-risk subsets. (A) The association between infiltrating immune cells and risk scores using seven algorithms. (B) The correlation between the expression of 15 matrisomal-related lncRNA-based signature and infiltrating immune cells using the CIBERSORT algorithm. (C, D) The heatmap and boxplot show the correlation analysis between immune functions and risk score. (E) Box plots revealed the differential expression of the immune checkpoint genes between two groups. (F) Correlation of risk score and TIDE score in different risk subgroups. ns no statistical significance, *p < 0.05, **p < 0.01, and ***p < 0.001.
Effects of LncRNA GRASLND on the Regulation of Matrisome in Gastric Cancer
Furthermore, we sought to prove that these lncRNAs in the risk model contribute to the regulation of ECM. Since lncRNA GRASLND showed the highest coefficient and hazard ratio, we chose it to evaluate the potential effects on the regulation of ECM in GC. AGS and MKN45 cell lines were transfected with two si-GRASLNDs, respectively. Subsequently we performed qRT-PCR analysis to confirm the knockdown efficiency (Figure 11A). Afterwards, GRASLND depletion significantly decreased the invasive capacity of AGS and MKN45 (Figures 11B, C). Furthermore, we analyzed the protein expression of the key enzyme of ECM, MMP9. We found that MMP9 was significantly downregulated after lncRNA GRASLND knockdown in GC (Figure 11D). Together, our results suggest that there is a strong correlation between lncRNA GRASLND and the regulation of ECM in GC.
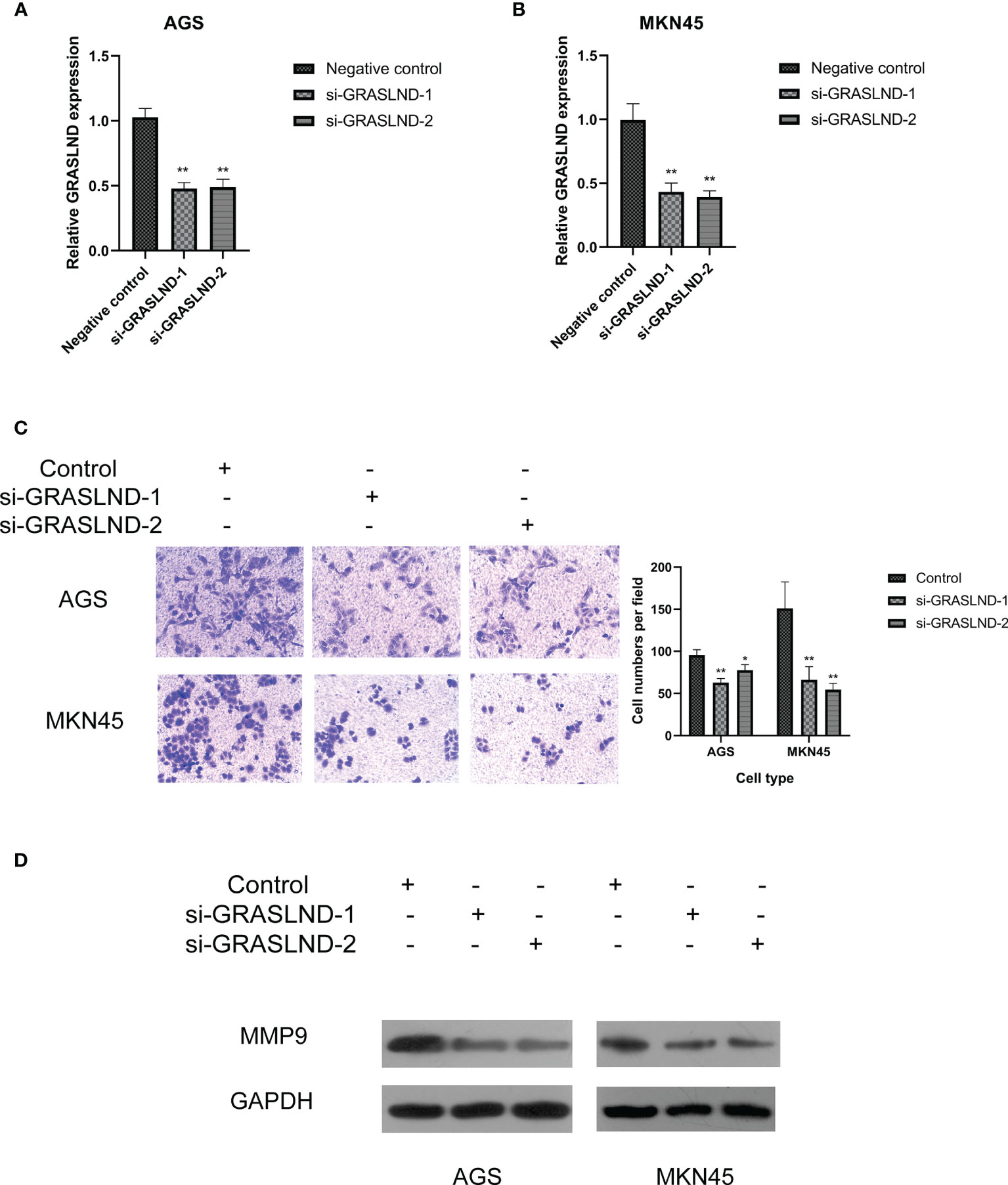
Figure 11 Effects of lncRNA GRASLND on the regulation of extracellular matrix in GC cells. (A) AGS and MKN45 cell lines were transfected with si-GRASLND, and qRT-PCR was performed to confirm the knockdown efficiency. (B) Knockdown of lncRNA GRASLND decreased the invasive ability of MKN45 cells. (C) Knockdown of lncRNA GRASLND decreased the invasive ability of AGS cells. (D) Knockdown of lncRNA GRASLND decreased the protein level of MMP9. *p < 0.05, **p < 0.01.
Discussion
Although surgical resection has been proven to improve the curative effect of GC, the therapeutic effect of advanced and metastatic GC is still not fully satisfactory. Several remarkable advances and breakthroughs have been witnessed in the management of advanced cancers, especially immunotherapy (21, 22). In recent years, researchers have pointed out the dominant roles of tumor heterogeneity in drug resistance and treatment failure (23). Moreover, the complexity of the tumor microenvironment has also exerted a distinct effect on these processes (24, 25). Considering the roles of ECM during tumor progression and immunotherapy resistance, we sought to explore the potential roles of matrisomal-related genes in prognosis prediction and immune regulation.
In this study, we constructed the risk model with a good performance based on matrisomal-related lncRNAs. This model could achieve the robustness of prognosis prediction and assess the robustness of prognosis prediction. Firstly, we obtained 1,068 matrisomal-related genes from the M.I.T. and identified matrisomal-related lncRNAs by Pearson correlation analysis. Among these candidate matrisomal-related lncRNAs, 54 prognostic-related lncRNAs were firstly selected and introduced to the lasso regression. Ultimately, 15 optimal matrisomal-related lncRNAs were obtained for risk model construction. The 1-, 3-, and 5-year AUCs were 0.718, 0.712, and 0.784, respectively, suggesting that the model showed good predictive power for the prognosis of GC patients. The predictive efficiency of the risk model was better than common clinicopathological variables. Multivariate analysis further demonstrated that the risk score was an independent prognostic factor for GC. In addition, to better guide clinical application, a nomogram model was established to predict the prognosis of GC patients. This nomogram model showed a better performance in the prediction of OS. Moreover, we uncovered a positive correlation between risk score and M2 macrophage infiltration. Finally, the experimental validation presented in this study suggested the strong correlation between lncRNA GRASLND and the regulation of ECM in GC.
Recent studies have demonstrated that specific matrix gene sets were predictors of immunosuppression, and thus might predict the efficacy of anti-PD1 therapy (26). Furthermore, Erkan et al. have also identified several stromal signatures that served as predictive and prognostic indicators for patients with pancreatic cancer (12). These results suggested the potential link between matrisome and anti-tumor immunity. Tumor immune-infiltrating cells not only exert an impact on tumor progression, but also induced immune responses to the anti-tumor therapy. Therefore, we explored tumor immune-infiltrating cells to further reveal the underlying mechanisms of immune evasion. In this study, we explored the correlation between risk score and the immune microenvironment. Our results showed that M2 macrophage infiltration was significantly altered in the high-risk group. Several studies demonstrated that M2 macrophage was associated with poor prognosis and disease processes (27, 28). Increasing lines of evidence suggested that different patterns of tumor-associated macrophages (TAMs) played a crucial role in immune escape. M2 macrophages have been proven to be associated with inhibitory cytokine secretion and immune cell infiltration, further attracting negative regulatory factors to the favorable immunosuppressive TME (29, 30). The mechanisms on how M2 macrophages mediated tumor immune escape was complex according to previous studies. Several cells like Th2, Tregs, and MDSCs could achieve M2 macrophage polarization and enhance its infiltration via different pathways (31). Conversely, cross-talk among these cells could also lead to a consequent immunosuppressive effect. These findings highlight the crucial roles of M2 macrophage in immune escape. As such, our risk model shows the close relation to M2 macrophage infiltration. Therefore, this model might offer important and unique advantages in the future of cancer immunotherapy.
Subsequently, we studied the interaction between risk score and immune functions. The results of ssGSEA showed that the contents of the antigen presentation process significantly differed in the high-risk group. The above findings further explained the reasons for the tumor-promoting status in the high-risk group and revealed that the immunosuppressive microenvironment exists in this group. These results supported findings from immune cell infiltration analysis. Due to the significance of ICIs, we further assessed the genetic basis of expression of ICI genes, and found the difference between two groups. Interestingly, we found that CD86, CD200, LAIR1, CD44, TNFSF9, PDCD1LG2, NRP1, CD276, CD48, and HAVCR2 were significantly higher in the high-risk group. Finally, the TIDE score was introduced to predict immune response. We found that the high-risk score was associated with a high TIDE score. Collectively, these observations suggested that these patients demonstrated an immunologically “cold” profile not to gain from immunotherapy.
Considering that GRASLND showed the primary effect on the prognosis of GC patients and had a larger coefficient in the risk model, we finally chose GRASLND to conduct functional experiments and explore the correlation with matrisome in GC cells. Our results indicated that knockdown of lncRNA GRASLND could decrease the protein expression of the key enzyme of ECM, MMP9. MMP9 plays a crucial role in the degradation of the ECM (32–34). Moreover, the upregulation of MMP9 was related to metastasis in GC (35, 36). Thus, our experiments confirmed that lncRNA GRASLND was the potential contributor towards regulation of ECM. However, there are still several limitations to our study. External validation of the risk model would be beneficial for its wide use. Hence, clinical samples would also be collected for further verification despite being time-consuming. Furthermore, further experiments are also needed to clarify the unknown functions and the potential mechanism of these lncRNAs.
Conclusion
In conclusion, we first constructed the 15 matrisomal-related lncRNAs model with a good performance in predicting the prognosis of GC patients. The risk signature might serve as a relatively good predictive tool to manage patients with GC.
Data Availability Statement
The original contributions presented in the study are included in the article/Supplementary Material Further inquiries can be directed to the corresponding authors.
Author Contributions
Conception and design: YY, LS, and JZ. Administrative support: YNZ and QG. Provision of study materials or patients: YZ, GW, and ML. Collection and assembly of data: JS, ZC, YW, and RJ. Data analysis and interpretation: YY, LS, and JZ. Manuscript writing: All authors. Final approval of manuscript: All authors.
Funding
This study was supported by the National Natural Science Foundation of China (71964021 and 81960430) and the Natural Science Foundation of Gansu province (21JR1RA117 and 21JR11RA099).
Conflict of Interest
The authors declare that the research was conducted in the absence of any commercial or financial relationships that could be construed as a potential conflict of interest.
Publisher’s Note
All claims expressed in this article are solely those of the authors and do not necessarily represent those of their affiliated organizations, or those of the publisher, the editors and the reviewers. Any product that may be evaluated in this article, or claim that may be made by its manufacturer, is not guaranteed or endorsed by the publisher.
Acknowledgments
We acknowledge the TCGA database for providing their data.
Supplementary Material
The Supplementary Material for this article can be found online at: https://www.frontiersin.org/articles/10.3389/fonc.2022.926404/full#supplementary-material
References
1. Sung H, Ferlay J, Siegel RL, Laversanne M, Soerjomataram I, Jemal A, et al. Global Cancer Statistics 2020: GLOBOCAN Estimates of Incidence and Mortality Worldwide for 36 Cancers in 185 Countries. CA Cancer J Clin (2021) 71(3):209–49. doi: 10.3322/caac.21660
2. Viale PH. The American Cancer Society's Facts & Figures: 2020 Edition. J Adv Pract Oncol (2020) 11(2):135–6. doi: 10.6004/jadpro.2020.11.2.1
3. Wang FH, Zhang XT, Li YF, Tang L, Qu XJ, Ying JE, et al. The Chinese Society of Clinical Oncology (CSCO): Clinical Guidelines for the Diagnosis and Treatment of Gastric Cance. Cancer Commun (Lond) (2021) 41(8):747–95. doi: 10.1002/cac2.12193
4. Wang X, Wang SS, Huang H, Cai L, Zhao L, Peng RJ, et al. Effect of Capecitabine Maintenance Therapy Using Lower Dosage and Higher Frequency vs Observation on Disease-Free Survival Among Patients With Early-Stage Triple-Negative Breast Cancer Who Had Received Standard Treatment: The SYSUCC-001 Randomized Clinical Trial. Jama (2021) 325(1):50–8. doi: 10.1001/jama.2020.23370
5. Zhao Q, Cao L, Guan L, Bie L, Wang S, Xie B, et al. Immunotherapy for Gastric Cancer: Dilemmas and Prospect. Brief Funct Genomics (2019) 18(2):107–12. doi: 10.1093/bfgp/ely019
6. Lordick F, Shitara K, Janjigian YY. New Agents on the Horizon in Gastric Cancer. Ann Oncol (2017) 28(8):1767–75. doi: 10.1093/annonc/mdx051
7. Wu P, Mo Y, Peng M, Tang T, Zhong Y, Deng X, et al. Emerging Role of Tumor-Related Functional Peptides Encoded by lncRNA and circRNA. Mol Cancer (2020) 19(1):22. doi: 10.1186/s12943-020-1147-3
8. Xie S, Chang Y, Jin H, Yang F, Xu Y, Yan X, et al. Non-Coding RNAs in Gastric Cancer. Cancer Lett (2020) 493:55–70. doi: 10.1016/j.canlet.2020.06.022
9. Nummela P, Lammi J, Soikkeli J, Saksela O, Laakkonen P, Hölttä E. Transforming Growth Factor Beta-Induced (TGFBI) is an Anti-Adhesive Protein Regulating the Invasive Growth of Melanoma Cells. Am J Pathol (2012) 180(4):1663–74. doi: 10.1016/j.ajpath.2011.12.035
10. Zhu M, Chen Q, Liu X, Sun Q, Zhao X, Deng R, et al. lncRNA H19/miR-675 Axis Represses Prostate Cancer Metastasis by Targeting TGFBI. FEBS J (2014) 281(16):3766–75. doi: 10.1111/febs.12902
11. Gu Y, Huang K, Zhang M, Teng F, Ge L, Zhou J, et al. Long Noncoding RNA CTD-2589m5.4 Inhibits Ovarian Cancer Cell Proliferation, Migration, and Invasion Via Downregulation of the Extracellular Matrix-Receptor Interaction Pathway. Cancer Biother Radioph (2021). doi: 10.1089/cbr.2020.4429
12. Erkan M, Michalski CW, Rieder S, Reiser-Erkan C, Abiatari I, Kolb A, et al. The Activated Stroma Index Is a Novel and Independent Prognostic Marker in Pancreatic Ductal Adenocarcinoma. Clin Gastroenterol Hepatol (2008) 6(10):1155–61. doi: 10.1016/j.cgh.2008.05.006
13. Li T, Xie J, Shen C, Cheng D, Shi Y, Wu Z, et al. Amplification of Long Noncoding RNA ZFAS1 Promotes Metastasis in Hepatocellular Carcinoma. Cancer Res (2015) 75(15):3181–91. doi: 10.1158/0008-5472.Can-14-3721
14. Frankish A, Diekhans M, Ferreira AM, Johnson R, Jungreis I, Loveland J, et al. GENCODE Reference Annotation for the Human and Mouse Genomes. Nucleic Acids Res (2019) 47(D1):D766–d773. doi: 10.1093/nar/gky955
15. Wu L, Wen Z, Song Y, Wang L. A Novel Autophagy-Related lncRNA Survival Model for Lung Adenocarcinoma. J Cell Mol Med (2021) 25(12):5681–90. doi: 10.1111/jcmm.16582
16. Xu Q, Wang Y, Huang W. Identification of Immune-Related lncRNA Signature for Predicting Immune Checkpoint Blockade and Prognosis in Hepatocellular Carcinoma. Int Immunopharmacol (2021) 92:107333. doi: 10.1016/j.intimp.2020.107333
17. Liu ZK, Wu KF, Zhang RY, Kong LM, Shang RZ, Lv JJ, et al. Pyroptosis-Related LncRNA Signature Predicts Prognosis and Is Associated With Immune Infiltration in Hepatocellular Carcinoma. Front Oncol (2022) 12:794034. doi: 10.3389/fonc.2022.794034
18. Li Z, Shi L, Li X, Wang X, Wang H, Liu Y. RNF144A-AS1, A TGF-β1- and Hypoxia-Inducible Gene That Promotes Tumor Metastasis and Proliferation via Targeting the miR-30c-2-3p/LOX Axis in Gastric Cancer. Cell Biosci (2021) 11(1):177. doi: 10.1186/s13578-021-00689-z
19. Jiang L, Liu JY, Shi Y, Tang B, He T, Liu JJ, et al. MTMR2 Promotes Invasion and Metastasis of Gastric Cancer via Inactivating Ifnγ/STAT1 Signaling. J Exp Clin Cancer Res (2019) 38(1):206. doi: 10.1186/s13046-019-1186-z
20. Yuan H, Chen Z, Bai S, Wei H, Wang Y, Ji R, et al. Molecular Mechanisms of lncRNA SMARCC2/miR-551b-3p/TMPRSS4 Axis in Gastric Cancer. Cancer Lett (2018) 418:84–96. doi: 10.1016/j.canlet.2018.01.032
21. Iwai Y, Terawaki S, Honjo T. PD-1 Blockade Inhibits Hematogenous Spread of Poorly Immunogenic Tumor Cells by Enhanced Recruitment of Effector T Cells. Int Immunol (2005) 17(2):133–44. doi: 10.1093/intimm/dxh194
22. Sharma P, Allison JP. The Future of Immune Checkpoint Therapy. Science (2015) 348(6230):56–61. doi: 10.1126/science.aaa8172
23. Burrell RA, McGranahan N, Bartek J, Swanton C. The Causes and Consequences of Genetic Heterogeneity in Cancer Evolution. Nature (2013) 501(7467):338–45. doi: 10.1038/nature12625
24. Junttila MR, de Sauvage FJ. Influence of Tumour Micro-Environment Heterogeneity on Therapeutic Response. Nature (2013) 501(7467):346–54. doi: 10.1038/nature12626
25. Quail DF, Joyce JA. Microenvironmental Regulation of Tumor Progression and Metastasis. Nat Med (2013) 19(11):1423–37. doi: 10.1038/nm.3394
26. Chakravarthy A, Khan L, Bensler NP, Bose P, De Carvalho DD. TGF-β-Associated Extracellular Matrix Genes Link Cancer-Associated Fibroblasts to Immune Evasion and Immunotherapy Failure. Nat Commun (2018) 9(1):4692. doi: 10.1038/s41467-018-06654-8
27. Chung W, Eum HH, Lee HO, Lee KM, Lee HB, Kim KT, et al. Single-Cell RNA-Seq Enables Comprehensive Tumour and Immune Cell Profiling in Primary Breast Cancer. Nat Commun (2017) 8:15081. doi: 10.1038/ncomms15081
28. Melcher V, Graf M, Interlandi M, Moreno N, de Faria FW, Kim SN, et al. Macrophage-Tumor Cell Interaction Promotes ATRT Progression and Chemoresistance. Acta Neuropathol (2020) 139(5):913–36. doi: 10.1007/s00401-019-02116-7
29. Noy R, Pollard JW. Tumor-Associated Macrophages: From Mechanisms to Therapy. Immunity (2014) 41(1):49–61. doi: 10.1016/j.immuni.2014.06.010
30. Zhang M, Hutter G, Kahn SA, Azad TD, Gholamin S, Xu CY, et al. Anti-CD47 Treatment Stimulates Phagocytosis of Glioblastoma by M1 and M2 Polarized Macrophages and Promotes M1 Polarized Macrophages In Vivo. PloS One (2016) 11(4):e0153550. doi: 10.1371/journal.pone.0153550
31. Ricketts TD, Prieto-Dominguez N, Gowda PS, Ubil E. Mechanisms of Macrophage Plasticity in the Tumor Environment: Manipulating Activation State to Improve Outcomes. Front Immunol (2021) 12:642285. doi: 10.3389/fimmu.2021.642285
32. Van Doren SR. Matrix Metalloproteinase Interactions With Collagen and Elastin. Matrix Biol (2015) 44-46:224–31. doi: 10.1016/j.matbio.2015.01.005
33. Bassiouni W, Ali MAM, Schulz R. Multifunctional Intracellular Matrix Metalloproteinases: Implications in Disease. FEBS J (2021) 288(24):7162–82. doi: 10.1111/febs.15701
34. Sokolova O, Naumann M. Matrix Metalloproteinases in Helicobacter Pylori-Associated Gastritis and Gastric Cancer. Int J Mol Sci (2022) 23(3):1883. doi: 10.3390/ijms23031883
35. Huang Q, Lan F, Wang X, Yu Y, Ouyang X, Zheng F, et al. IL-1β-Induced Activation of P38 Promotes Metastasis in Gastric Adenocarcinoma via Upregulation of AP-1/C-Fos, MMP2 and MMP9. Mol Cancer (2014) 13:18. doi: 10.1186/1476-4598-13-18
Keywords: matrisomal pattern, gastric cancer, overall survival (OS), immune regulation, risk model
Citation: Yang Y, Shi L, Zhang J, Zheng Y, Wu G, Sun J, Liu M, Chen Z, Wang Y, Ji R, Guo Q and Zhou Y (2022) A Novel Matrisomal-Related LncRNA Signature Associated With Survival Outcome and Immune Evasion in Patients With Gastric Cancer. Front. Oncol. 12:926404. doi: 10.3389/fonc.2022.926404
Received: 22 April 2022; Accepted: 23 May 2022;
Published: 24 June 2022.
Edited by:
Palmiro Poltronieri, Italian National Research Council, ItalyReviewed by:
Macrina Beatriz Silva Cázares, Autonomous University of San Luis Potosí, MexicoZhigang Jie, The First Affiliated Hospital of Nanchang University, China
Daniele Vergara, University of Salento, Italy
Copyright © 2022 Yang, Shi, Zhang, Zheng, Wu, Sun, Liu, Chen, Wang, Ji, Guo and Zhou. This is an open-access article distributed under the terms of the Creative Commons Attribution License (CC BY). The use, distribution or reproduction in other forums is permitted, provided the original author(s) and the copyright owner(s) are credited and that the original publication in this journal is cited, in accordance with accepted academic practice. No use, distribution or reproduction is permitted which does not comply with these terms.
*Correspondence: Qinghong Guo, gqh@lzu.edu.cn; Yongning Zhou, zhouyn@lzu.edu.cn
†These authors have contributed equally to this work