- 1The First Clinical School of Shandong University of Traditional Chinese Medicine, Jinan, China
- 2Innovation Research Institute of Traditional Chinese Medicine, Shandong University of Traditional Chinese Medicine, Jinan, China
- 3Department of Earth Sciences, Kunming University of Science and Technology, Kunming, China
- 4Department of Breast and Thyroid Surgery, Affiliated Hospital of Shandong University of Traditional Chinese Medicine, Jinan, China
Breast cancer, as one of the most common malignancies in women, exhibits complex and heterogeneous pathological characteristics across different subtypes. Triple-negative breast cancer (TNBC) and HER2-positive breast cancer are two common and highly invasive subtypes within breast cancer. The stability of the breast microbiota is closely intertwined with the immune environment, and immunotherapy is a common approach for treating breast cancer.Tertiary lymphoid structures (TLSs), recently discovered immune cell aggregates surrounding breast cancer, resemble secondary lymphoid organs (SLOs) and are associated with the prognosis and survival of some breast cancer patients, offering new avenues for immunotherapy. Machine learning, as a form of artificial intelligence, has increasingly been used for detecting biomarkers and constructing tumor prognosis models. This article systematically reviews the latest research progress on TLSs in breast cancer and the application of machine learning in the detection of TLSs and the study of breast cancer prognosis. The insights provided contribute valuable perspectives for further exploring the biological differences among different subtypes of breast cancer and formulating personalized treatment strategies.
Introduction
Since 2019, breast cancer has surpassed lung cancer as the malignant tumor with the highest global incidence (1), and its incidence is closely related to socioeconomic development, with the highest risk of disease in economically transformed regions and the lowest survival rates in economically underdeveloped regions (2). Underdiagnosis, misdiagnosis and lack of effective treatments are the most important reasons for the huge differences in prevalence and survival rates worldwide (3). The current treatment options for breast cancer include various modalities such as surgery, radiation, immunization, endocrine, targeted,chemotherapy and Chinese medicine. Immunotherapy has made significant breakthroughs in recent years, with immune checkpoint inhibitors in particular boosting survival in many patients. However, this approach has shown significant results in only a subset of patients, with relatively low success rates. Objective remission rates in the vast majority of clinical studies were less than 20%, while median progression-free survival and overall survival were less than 3 and 24 months (4). Therefore, there is a need for in-depth research on new methods and ideas for the treatment of breast cancer. TLSs are a class of ectopic lymphoid organs that form in non-lymphoid tissues and are common in conditions such as tumors, chronic inflammation, and autoimmune diseases. Because TLSs are formed in non-lymphoid tissues, they have a more flexible ability to respond to local abnormal lesions, thus playing an important immunomodulatory role in chronic inflammation and tumor development and contributing to the efficiency of immune response and local therapeutic efficacy. The presence of TLSs has been observed in a variety of malignant tumors such as non-small cell lung cancer, colorectal cancer, breast cancer, melanoma, sarcoma, and renal cell carcinoma (5). Recent findings indicate that researchers are gradually recognizing the positive role of TLSs in treating breast cancer patients. These insights have provided clinicians with new perspectives to reformulate breast cancer treatment protocols from an immune perspective (6). Machine learning belongs to a kind of artificial intelligence, with the arrival of the era of big data, the ability of computers to quickly process complex and huge data is increasingly dominant, and in recent years the increasing popularity of high-throughput histological data and the success of artificial intelligence technology has made machine learning in a variety of fields widely used (7–9). Machine learning is more efficient than humans in predicting and prognosticating treatment for some cancers. This review summarizes the current understanding of TLSs in breast cancer and the potential application of machine learning in the study of TLSs and breast cancer.
Detecting TLSs through machine learning
The most common techniques for detecting TLSs are multiple immunofluorescence, hematoxylin and eosin (HE) staining, of which multiple immunofluorescence is difficult to generalize in research due to its high cost, small field of view, and high complexity. In contrast, HE staining is easier and remains the clinical standard in histopathology. TLSs have been detected by pathologists on HE slides, but manual detection is time-consuming and laborious, and results vary according to the level of expertise.Li, Z et al. (10) developed a machine-learning-based computational tool for the automatic detection and quantitative assessment of TLSs on routine HE slides. They confirmed its independent prognostic value in an international multicenter cohort of 1924 patients with six common gastrointestinal cancers. An important advantage of this method is the automated enumeration and quantitative characterization of TLSs. This study, the largest to date, confirms the association of TLSs with the survival of patients with gastrointestinal cancers.
Composition of TLSs
TLSs contain within them T cells, B cells, dendritic cells, and high endothelial venules (HEV) (11). Similar to SLOs, both share the components of B cells, T cells, and dendritic cells. However, compared to SLOs, TLSs have a simpler organization with scattered lymphocyte aggregates and lack the connective tissue membrane of SLOs (12). The non-enveloped structure of TLSs allows the immune cells to fully interact with the surrounding microenvironment, and, unlike SLOs, TLSs do not persist in a specific location, but rather are formed under specific pathologic conditions, independent of secondary lymphoid organ regions. TLSs are formed under specific pathological conditions, independent of secondary lymphoid organ regions, and trigger immune responses under the regulation of clear inflammatory signals. However, the mechanism that triggers the formation of TLSs remains unclear (13).
T cells, B cells and dendritic cells
The T-cell zone is located in the periphery of TLSs and consists mainly of clusters of T cells and mature dendritic cells; the B-cell zone is located in the central region of TLSs and consists of a large number of B cells and some T cells, follicular dendritic cells, and macrophages. In the T-cell zone, T cells are activated by stimulation with specific antigens, and dendritic cells capture and present antigens so that T cells can recognize and bind to them. Activated T cells proliferate rapidly to form effector T cells, which subsequently migrate to the site of infection to execute an immune attack (14–16). In the B-cell region, B cells are activated and differentiate into plasma cells that produce specific antibodies against antigens. Similarly, dendritic cells capture and present foreign antigens to B cells in this process, initiating antibody production. This coordinated immune response mechanism ensures the body’s immune balance (17–21). Researchers comprehensively analyzed 69 studies covering 19 types of cancers and showed a positive correlation between tumor-infiltrating B cells (including plasma cells) and clinical outcome in half of the cases, while the rest showed either a negative correlation (9.3%) or a neutral effect (40.7%) (22). Helmink and others investigated the density and distribution of B cells and their relationship with TLSs and found that the same properties of memory B cells and plasma cells required for the acquired immune response may contribute to an effective T cell response after neoadjuvant immune checkpoint blockade. Importantly, these B cells may act by altering T cell activation and function and through other mechanisms with key immune components of TLSs (23). Evidence suggests that B cells predict a better prognosis and a higher response rate to immunotherapy only if they form TLSs, and that B cells outside of TLSs may suppress antitumor immunity and promote tumor growth (24). These studies reveal that B cells in the tumor microenvironment not only correlate with clinical outcomes, but may also play a critical role in T cell responses in the context of immunotherapy.
HEV
HEV is a unique vascular structure in the lymphatic system, which helps the formation of TLSs through its unique morphology and function, and contributes to lymphocyte colonization and immunoregulation in the lymphatic system. Most of the current opinions believe that the formation of TLSs is highly dependent on SLOs and HEV (25–28). Some of the lymphocytes in SLOs spread to tumor tissues through lymphatic vessels or HEV when stimulated by inflammatory factors for a long period of time, thus initiating the formation of TLSs (29). In a retrospective cohort study, Martinet analyzed data containing 146 patients with invasive breast cancer and showed that the density of HEV was positively correlated with patients’ disease-free survival, metastasis-free survival, and overall survival, demonstrating their important role in the formation of TLSs (30). Therefore, tumor HEV may be potential therapeutic targets in cancer diagnosis and treatment. For example, Colbeck and his team effectively killed tumor cells in the Foxp3DTR mouse model by depleting Treg cells to promote the self-expanding circuit of T-cell activation as well as the formation of HEV in the tumor, which in turn induced the formation and maturation of TLSs in the tumor (31).
The formation process of TLSs
CXCL13 and IL-7 are involved in the formation of TLSs
The body undergoes a complex series of reactions in response to damaged tissues or pathogens in an inflammatory state. This process involves the release of signaling molecules (e.g., inflammatory cytokines, chemokines, growth factors) from damaged tissues at the site of inflammation, which then attracts surrounding immune cells toward the damaged area (32, 33). Chemokine C-X-C motif ligand 13 (CXCL13) and interleukin (IL)-7 are key chemokines. They are released near the site of inflammation and can direct the migration of lymphoid tissue inducer cells (LTi) (Figure 1). CXCL13 recruits B cells to the tumor region to form TLSs, and the investigators revealed that, in the tumor microenvironment, Th-CXCL13 cells clustered in the central B-cell region and formed within the follicular TLSs. co-localization. Notably, they may attract B cells through chemotaxis as a way to promote the formation and maturation of follicular TLSs (34). CXCL13 has been identified as one of the most potent predictors of improved survival in human cancers (35–38), and the production of CXCL13 by T cells infiltrating inflammatory tissues may be a critical step in the initiation of TLSs formation (39). Some studies have confirmed the presence of dense TLSs and tumor-infiltrating lymphocytes (TILs) in clear cell renal cell carcinoma and demonstrated the oncogenic role of CXCL13 expression in clear cell renal cell carcinoma (40), and Hsieh analyzed the disease-free survival of 794 breast cancer patients (Disease-Free Survival, DFS) in 794 breast cancer patients and the clinical correlation between CXCL13 showed that CXCL13 expression was positively correlated with DFS in breast cancer patients, especially in the HER2 group. Researchers went on to investigate 996 breast cancer patients receiving neoadjuvant chemotherapy and showed that CXCL13 expression was highly correlated with complete remission in HER2 patients (41), a finding that could help in the development of new immunotherapies. These studies have confirmed that CXCL13 expression induces the formation of TLSs.IL-7 is a pluripotent cytokine that maintains the homeostasis of the immune system and plays a crucial role in the development, proliferation, and differentiation of T cells as well as in the promotion of B-cell maturation through the activation of the IL-7 receptor (42–46). The expansion of the lymphatic vessels associated with TLSs occurs in two distinct phases. The first stage of expansion is dependent on IL-7. the second stage is responsible for the drainage of leukocytes from the gland and is regulated by lymphotoxin (LT) βR signaling. Maria Iolyeva have demonstrated that autocrine signaling for IL-7 on lymphatic endothelial cells in SLOs regulates lymphatic vessel remodeling and expansion (47). In addition, fibroblast-derived IL-7 may support lymphangiogenesis in a paracrine manner (48), and all of these findings could suggest that IL-7 is a key regulatory molecule for lymphatic vessel expansion in TLSs (49). Indeed, prophylactic blockade of IL-7 affects lymphatic endothelial cell proliferation, which determines the formation of smaller caliber lymphatic vessels. Although this defect is not complete, it could still indicate that IL-7 plays a role in the early stages of lymphangiogenesis associated with TLSs.
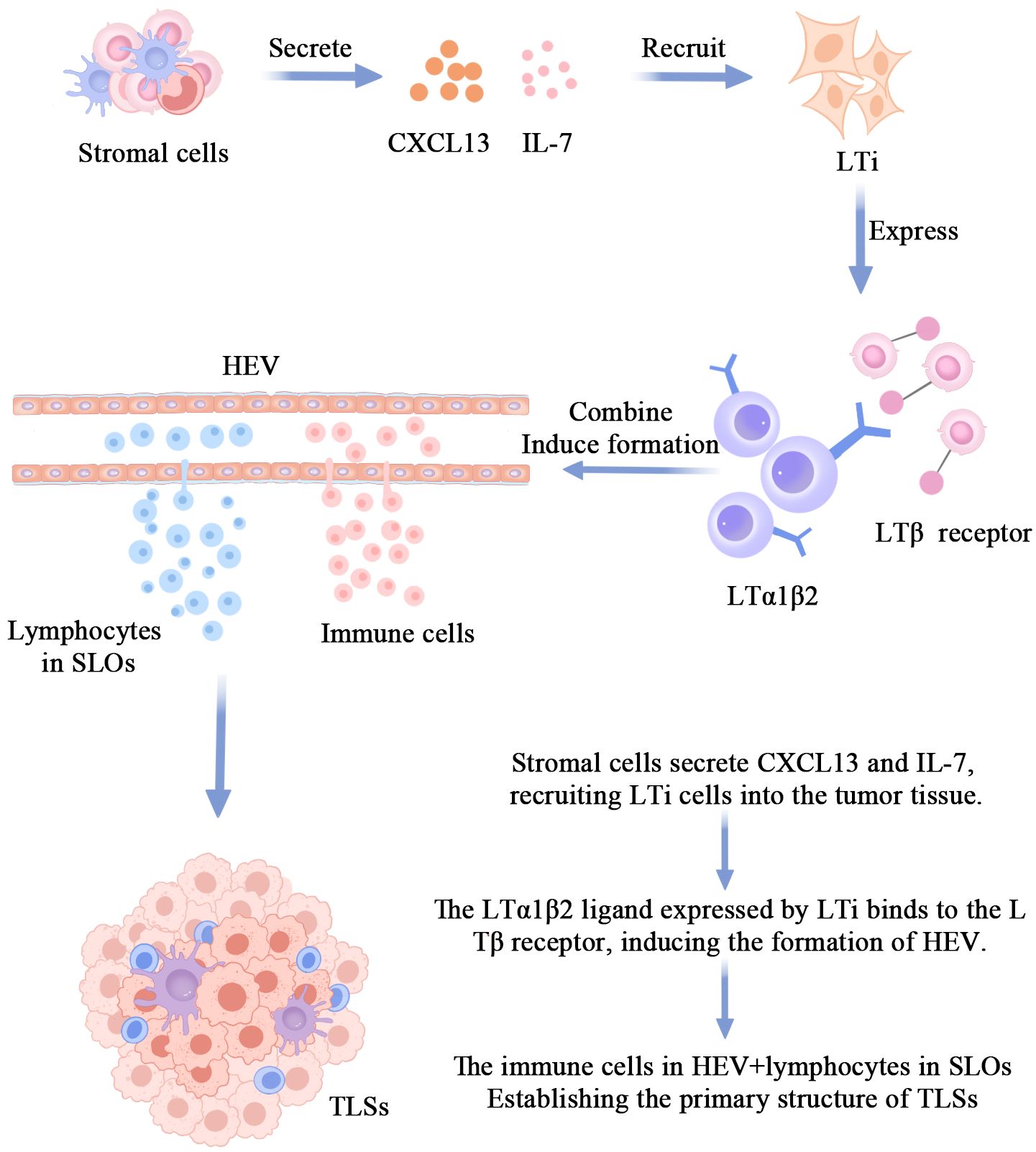
Figure 1 The mechanism of TLS formation.The primary pathway for TLSs formation involves stromal cells secreting CXCL13 and IL-7 to recruit LTi cells into the tumor tissue. The interaction between the LTa1β2 ligand on LTi cell surfaces and the LTβ receptor induces the formation of HEV. Immunocytes within HEV and some lymphocytes in SLO, under prolonged stimulation by inflammatory factors, disseminate into the tumor tissue, giving rise to the initial morphology of TLSs.
LTi’s involvement in the formation of TLSs
LTi is a special class of lymphocytes that plays an important role in induction during embryonic development and lymphoid organogenesis.LTi expresses many molecules related to lymphoid organogenesis, such as tumor necrosis factor-associated activation-inducing cytokines, nuclear factor kappa B receptor activator (50–54). Besides these proteins, LTi expresses molecules that bind to the LTβR receptor on the surface of stromal cells. LTi also secretes IL-17, which stimulates stromal cells to release a variety of chemokines, including CXCL12, CXCL13, CCL19, and CCL21 (55–58), which in turn attracts more lymphocytes to accumulate in the region where TLSs form. Cupedo and his team found that intradermal injection of nascent LTi induced TLSs (59). Ectopic expression of LTα in pancreatic islets also induces the formation of TLSs, and Picarella found that LTα-deficient mice completely lacked peripheral lymphoid organs (60); in contrast, specific expression of the LTα transgene in the kidney and pancreas triggered severe chronic inflammation with concomitant formation of TLSs that had the ability to promote antigen-specific responses and antibody class switching.In addition, in contrast to overexpression of only LTα, simultaneous overexpression of both LTα and LTβ leads to more pronounced formation of TLSs compared to overexpression of only LTα (61).
Application of machine learning in breast cancer
Existing research suggests that machine learning is increasingly used for clinical cancer diagnosis, grading, genetic alteration prediction, and disease prognosis, and that it can identify molecular markers for cancer treatment (62–67), predict surgical outcomes (68), and interpret electrocardiograms. Liu, X et al. developed a novel breast cancer recurrence and metastasis risk assessment framework from histopathology images using image features and machine learning techniques (69), The study is expected to reduce the workload of pathologists and improve the chances of survival for breast cancer patients. Sammut, S.-J. analyzed breast tumors from patients with primary invasive cancers who participated in the TransNEO study at Cambridge University Hospitals NHS Foundation Trust between 2013 and 2017, and found that machine-learning models combining clinical, molecular, and numerical pathology data for predicting response to treatment significantly outperformed models based on clinical variables, emphasizing the The importance of data integration for response prediction and can be used to generate similar predictors for other cancers (70). Machine learning can not only diagnose and assess the prognosis of breast cancer from a pathologic perspective, but also help clinicians choose breast cancer treatments from an imaging perspective. Yu, Y. et al. (71) then developed an efficient preoperative magnetic resonance imaging imaging histology for assessing axillary lymph node status in breast cancer using machine learning techniques, which can identify patients with axillary lymph node metastasis in early-stage invasive breast cancer preoperatively. While machine learning has made significant progress in the diagnosis, treatment, and prognosis assessment of breast cancer, it also has limitations. The performance of machine learning algorithms highly depends on the quality and quantity of input data. Therefore, incomplete or biased datasets may affect the accuracy of the model.
Microbiota and Immunological Research in Breast Cancer
The human microbiota refers to the collection of microorganisms that inhabit the human body. While most microorganisms are beneficial to the human body, an imbalance in the microbial community, where harmful bacteria outnumber beneficial ones, can lead to various diseases, including cancer. In recent years, researchers have uncovered previously unrecognized connections between immune microenvironment dysregulation and breast cancer (72–76). There is limited knowledge about the microbial composition associated with normal breast tissue and breast-related diseases. Human breasts are not sterile; they harbor diverse bacterial communities. Studies have confirmed that, besides the skin, some microbial communities in breast tissue can also translocate from the gastrointestinal tract through the nipple, possibly facilitated by breastfeeding and/or oral contact through sexual activity (77). This breast microbiota stimulates resident immune cells to maintain healthy breast tissue. Additionally, the types of bacteria present and their metabolic activities, such as their ability to degrade carcinogenic substances, may also contribute. Xuan, C. observed that the baseline expression of antimicrobial response genes in tumors is lower than in healthy breast tissue. Microbial DNA is present in the breast, suggesting that bacteria or their components may influence the local immune microenvironment (78). Banerjee et al. examined the specific and common viral, bacterial, fungal, and parasitic features of each breast cancer subtype. They identified distinct patterns in triple-negative and triple-positive breast cancer samples, while ER-positive and HER2-positive samples exhibited similar microbial characteristics. These features, unique or shared among different breast cancer types, offer a new research avenue for gaining further insights into the treatment and prognosis of breast cancer. This provides a novel understanding of the role of the microbiota in breast cancer (79). Research has found that postmenopausal women newly diagnosed with breast cancer exhibit less diversity and compositional differences in their fecal microbiota compared to women without breast cancer. This discovery suggests that the gut microbiota may influence the risk of breast cancer occurrence and could potentially do so through estrogen-independent pathways (80). The stability of the microbiota in the breast is closely intertwined with the immune environment. Clinical practitioners can develop tailored treatment strategies based on the mechanisms of action of the microbiota in the breast in improving the prognosis of breast cancer.
Mechanisms of TLSs formation involving other factors
Inflammation-related necrosis and macrophage infiltration may be associated with the formation of TLSs. Tumor necrosis is often accompanied by the onset of an inflammatory response in which damaged tissue releases inflammatory factors and cytokines in response to external aggression. In some cases, cell necrosis within the tumor results in the formation of foci of inflammatory necrosis. These necrotic foci become one of the underlying conditions for the formation of TLSs, which attract immune cells (especially macrophages) by releasing a large number of signaling molecules that accumulate in the damaged area (81). The high degree of plasticity of macrophages can adapt to various microenvironmental changes in tissues, and M1-type macrophages, which are usually activated by toll-like receptors, mostly exhibit anti-pro-inflammatory effects in the immune response (82–85). Olson demonstrated that modulation of phagocytosis in macrophages effectively inhibits tumor progression and improves the prognosis of cancer patients (86). Under inflammatory conditions, macrophages activate and release more inflammatory mediators and chemokines, which further direct immune cells to migrate to the region of TLSs, contributing to the structural formation and maintenance of TLSs (87).
Expression of TLSs in different breast cancer subtypes
TLSs have been shown to have a favorable prognostic function in a variety of malignancies, but have been less well studied in breast cancer. In the available studies, significant differences were found between TLSs-positive and TLSs-negative subgroups within the same molecular subtype of breast cancer, with significant effects on breast cancer recurrence, lymphovascular infiltration and perineural infiltration (88). TLSs in the breast cancer stroma have been associated with activation of tumor angiogenesis, suggesting that this may be a factor favoring breast cancer metastasis, but most of these studies have been performed in animal models and there are no data on human tissues (89).TLSs were detected in 60% of breast cancer tumors and correlated with higher infiltration of TILs by Buisseret. PD-1 and PD-L1 expression were also associated with higher density of TILs and TLSs, in addition TILs density, TLSs and PD-L1 expression were associated with more aggressive tumor characteristics (90), on the basis of this study, researchers found a positive correlation between the expression of immune checkpoint molecules and baseline TILs and TLSs suggesting that assessing these parameters in breast cancer patients may identify immunomodulatory therapies in tumors that are responsive to them (91).
TLSs and TNBC
With the in-depth study of TLSs in various types of malignant tumors, more and more researchers are finding TLSs in TNBC (92–94). TNBC refers to a subtype of breast cancer that lacks ER, PR, and HER2, which accounts for 15%-20% of breast cancer cases, and more than 50% of patients recur within the first 3-5 years after diagnosis, making it the most malignant subtype of breast cancer (95, 96). Current treatment options are limited to surgery, adjuvant chemotherapy and radiotherapy (97–101). However, these treatments have certain drawbacks: 1. Surgery may result in changes in breast appearance, including reduction or loss of breast size, which may have an impact on the patient’s psychological and emotional well-being. 2. Adjuvant chemotherapy and radiotherapy may cause damage to the immune system. In recent years, immunotherapy has emerged as an innovative treatment option that significantly reduces damage to healthy cells by activating the patient’s own immune system to fight cancer cells (102–105). Available immunotherapies such as PD-1/PD-L1 inhibitors, CTLA-4 inhibitors, and immune-checkpoint combination therapies have dramatically improved the condition of patients with breast cancer and the adverse effects of the treatment.
TLSs have been reported to be present in approximately 60% of breast cancers (106), and most papers aimed at determining the presence of TLSs or their impact on breast cancer prognosis have been reported mainly in the TNBC subtype.Figenschau demonstrated that tumors with higher levels of TILs were associated with the formation of intra-tumoral TLSs, higher tumor grade, and higher degree of inflammation, which led to a poorer prognosis (107). In addition, TLSs were found to correlate with DFS and overall survival in some TNBC patients, providing new ideas for the treatment of TNBC.Schmid found that PD-L0 expression was moderately correlated with TILs in TNBC (r = 45.0-59.8) (108). Researchers showed a positive correlation with TILs in TNBC when using HE-stained slides and CD3/CD20 immunohistochemistry (IHC)-stained slides, by which they found that, compared to TNBC with low plasma cell density, the presence of TLSs in TNBC with high plasma cell density significantly higher numbers (109), in addition they confirmed that TNBC with higher plasma cell density were also associated with higher B-cell density. The investigators collected 108 patients with TNBC treated with neoadjuvant chemotherapy and measured the amount of TILs and TLSs on histopathology using HE-stained slides. HEV densities and subpopulations of TILs by IHC measuring MECA79, CD3, CD8, and CD20, and the amount of TLSs in core needle biopsies from neoadjuvant chemotherapy prior to TNBC using a digital computer analyzer were found to be higher levels of TLSs as represented by MECA79-positive HEV densities, and higher levels of TILs in the HE slides were pathologic complete remission predictors. On the basis of this finding, it was concluded that the approach of increasing HEV may be beneficial for cancer immunotherapy and is of great clinical therapeutic relevance. They also evaluated the percentage of TLSs positivity in all molecular subtypes of breast cancer.Luminal B breast cancer subtype had a high percentage of TLSs positive cases among Luminal subtypes 27.58% followed by Luminal A-BC (24.13%) and Luminal B-HER2 (10.34%) (109).
TLSs and HER2-positive breast cancer
Studies have shown that the presence of TLSs may be an important good prognostic indicator for patients with HER2-positive breast cancer, regardless of the level of TILs (110). The HER2 receptor belongs to a family of receptors consisting of four cell surface receptors (HER1-4). When expressed at normal levels, HER2 regulates cell growth, differentiation and survival. However, in pathological conditions where HER2 is overexpressed, it leads to aggressive tumor growth. Therefore, the prognosis associated with HER2-positive breast cancer is usually poor and most patients with HER2-positive breast cancer are resistant to targeted hormonal therapy.The most common drugs for treating HER2-positive breast cancer include trastuzumab, lapatinib, pertuzumab, and ado-trastuzumab emtansine. However, there are still questions regarding the optimal sequence, duration, and combination (with or without chemotherapy) of anti-HER2 targeted therapies, both in advanced and adjuvant settings (111–114). Since the presence of TLSs is associated with anti-tumor immune responses and prolonged patient survival, the study of TLSs in HER2-positive breast cancer is of great clinical guidance. It has been shown in the literature that the favorable outcome of many HER2-positive breast cancer patients treated with chemotherapy and/or HER2-targeted therapy is attributed to active anti-tumor immunity, passive immunotherapy (115). Therefore, the presence of TLSs may be an indicator of treatment response in HER2-positive breast cancer patients. It has been found that in HER2-positive patients, the presence of TLSs at the infiltration margins and/or peritumor was associated with better DFS, but not with overall survival (110). Tumor TLSs are surrounded by a specific vascular system, including peripheral lymph node address-positive vessels, which may allow direct migration of peripheral blood lymphocytes into the TLSs. Indeed, primitive T cells and B cells can be found in tumor TLSs, which escape the local immunosuppressive effects of the tumor environment and thus promote more effective antitumor immunity. A significant correlation was found between the extent of TLSs and HER2 IHC score or HER2 gene copy number, as well as a strong correlation between the percentage of precancerous lesions in the ducts and the extent of TLSs (116). It can therefore be hypothesized that increased HER2 protein expression or associated mutations may act as immunogenic factors that attract lymphocytes to the tissue and promote TLSs. Another possible explanation is that the acne necrosis commonly seen in HER2-positive intraductal precancerous lesions may be associated with increased macrophage infiltration, which also plays an important role in the immune response as antigen-presenting cells (117).
Conclusions
In summary, the in-depth investigation of the relationship between breast cancer and TLSs has brought new ideas and prospects for the clinical diagnosis and treatment of this type of malignant tumor. Machine learning demonstrates high efficiency in the detection of TLSs and the diagnosis and prognosis of breast cancer.Immune checkpoint inhibitors based on TLSs have become an important breakthrough in breast cancer treatment, but not all patients can benefit from them. In the future, we should focus on researching the distribution and activity of TLSs, making effective use of machine learning to identify beneficiary populations, and ensuring more precise selection before treatment. Furthermore, by exploring the molecular signaling pathways influencing the formation and function of TLSs, as well as the microbial characteristics of each subtype of breast cancer, identifying intervention targets, and developing novel treatment strategies, it is possible to formulate personalized treatment plans based on individual patients. This approach has the potential to significantly enhance treatment efficacy and reduce unnecessary medical interventions.
Author contributions
XinL: Writing – review & editing, Writing – original draft, Validation, Supervision, Methodology, Investigation, Formal analysis, Conceptualization. HX: Writing – review & editing, Visualization, Validation, Supervision, Conceptualization. ZD: Writing – review & editing, Validation, Supervision, Investigation. QC: Writing – review & editing, Supervision, Resources, Investigation, Conceptualization. XiaL: Writing – review & editing, Writing – original draft, Supervision, Resources, Investigation, Funding acquisition.
Funding
The author(s) declare that no financial support was received for the research, authorship, and/or publication of this article.
Conflict of interest
The authors declare that the research was conducted in the absence of any commercial or financial relationships that could be construed as a potential conflict of interest.
Publisher’s note
All claims expressed in this article are solely those of the authors and do not necessarily represent those of their affiliated organizations, or those of the publisher, the editors and the reviewers. Any product that may be evaluated in this article, or claim that may be made by its manufacturer, is not guaranteed or endorsed by the publisher.
References
1. Burstein H, Curigliano G, Thürlimann B, Weber W, Poortmans P, Regan M, et al. Customizing local and systemic therapies for women with early breast cancer: the st. gallen international consensus guidelines for treatment of early breast cancer 2021. Ann Oncol (2021) 32(10):1216–35. doi: 10.1016/j.annonc.2021.06.023
2. Sung H, Ferlay J, Siegel RL, Laversanne M, Soerjomataram I, Jemal A, et al. Global cancer statistics 2020: GLOBOCAN estimates of incidence and mortality worldwide for 36 cancers in 185 countries. CA Cancer J Clin (2021) 71(3):209–49. doi: 10.3322/caac.21660
3. Wilkinson L, Gathani T. Understanding breast cancer as a global health concern. Br J Radiol (2022) 95(1130):20211033. doi: 10.1259/bjr.20211033
4. Tai Y, Jiang J. PD-1/PD-L1 inhibitors for breast cancer: current status, problems and countermeasures. Chin J Cancer (2023) 30(2):99–107. doi: 10.3872/j.issn.1007-385x.2023.02.001
5. Chen J, Chen J, Wang L. Tertiary lymphoid structures as unique constructions associated with the organization, education, and function of tumor-infiltrating immunocytes. J Zhejiang Univ Sci B (2022) 23(10):812–2. doi: 10.1016/j.immuni.2017.10.009
6. Zou ZW, Li W, Zhang F, Zhang Q, Liu GW. Tertiary lymphoid structure and breast cancer treatment progress. Anti-Tumor Pharm (2015) 6):414–9. doi: 10.3969/j.issn.2095-1264.2015.06.04
7. Lee CH, Yoon HJ. Medical big data: promise and challenges. Kidney Res Clin Pract (2017) 36(1):3–11. doi: 10.23876/j.krcp.2017.36.1.3
8. Bulten W, Pinckaers H, Van Boven H, Vink R, De Bel T, Van Ginneken B, et al. Automated deep-learning system for gleason grading of prostate cancer using biopsies: a diagnostic study. Lancet Oncol (2020) 21(2):233–41. doi: 10.1016/S1470-2045(19)30739-9
9. Kather JN, Pearson AT, Halama N, Jäger D, Krause J, Loosen SH, et al. Deep learning can predict microsatellite instability directly from histology in gastrointestinal cancer. Nat Med (2019) 25(7):1054–6. doi: 10.1038/s41591-019-0462-y
10. Li Z, Jiang Y, Li B, Han Z, Shen J, Xia Y, et al. Development and validation of a machine learning model for detection and classification of tertiary lymphoid structures in gastrointestinal cancers. JAMA Netw Open (2023) 6(1):e2252553. doi: 10.1001/jamanetworkopen.2022.52553
11. Schumacher TN, Thommen DS. Tertiary lymphoid structures in cancer. Science (2022) 375(6576):eabf9419. doi: 10.1126/science.abf9419
12. Han N, Gao XC, Yao RR, Nie YZ. Progress in the study of tertiary lymphoid structures for malignant solid tumors. Electron J Compr Cancer Treat (2023) 9(2):119–28. doi: 10.12151/JMCM.2023.02-10
13. Pitzalis C, Jones GW, Bombardieri M, Jones SA. Ectopic lymphoid-like structures in infection, cancer and autoimmunity. Nat Rev Immunol (2014) 14(7):447–62. doi: 10.1038/nri3700
14. Altmann DM. Regulatory t-cells: receptors, repertoires and roles in disease. Immunology (2018) 155(2):153–4. doi: 10.1111/imm.12999
15. Wu X, Zhou Z, Cao Q, Chen Y, Gong J, Cao G. Reprogramming of treg cells in the inflammatory microenvironment during immunotherapy: a literature review. Front Immunol (2023) 14:1268188. doi: 10.3389/fimmu.2023.1268188
16. Cao Q, Wu X, Chen Y, Wei Q, You Y, Qiang Y, et al. The impact of concurrent bacterial lung infection on immunotherapy in patients with non-small cell lung cancer: a retrospective cohort study. Front Cell Infect Microbiol (2023) 13:1257638. doi: 10.3389/fcimb.2023.1257638
17. Huse M. Mechanical forces in the immune system. Nat Rev Immunol (2017) 17(11):679–90. doi: 10.1038/nri.2017.74
18. Ganeshan K, Chawla A. Metabolic regulation of immune responses. Annu Rev Immunol (2014) 32:609–34. doi: 10.1146/annurev-immunol-032713-120236
19. Janssen LM, Ramsay EE, Logsdon CD, Overwijk WW. The immune system in cancer metastasis: friend or foe. J Immunother Cancer (2017) 5:1–14. doi: 10.1186/s40425-017-0283-9
20. Carvalho LA, Gerdes JM, Strell C, Wallace GR, Martins JO. Interplay between the endocrine system and immune cells. BioMed Res Int (2015) 2015:986742. doi: 10.1155/2015/986742
21. Prestwich RJ, Errington F, Hatfield P, Merrick A, Ilett EJ, Selby PJ, et al. The immune system–is it relevant to cancer development, progression and treatment. Clin Oncol (2008) 20(2):101–12. doi: 10.1016/j.clon.2007.10.011
22. Wouters MC, Nelson BH. Prognostic significance of tumor-infiltrating b cells and plasma cells in human cancer. Clin Cancer Res (2018) 24(24):6125–35. doi: 10.1158/1078-0432.CCR-18-1481
23. Helmink BA, Reddy SM, Gao J, Zhang S, Basar R, Thakur R, et al. B cells and tertiary lymphoid structures promote immunotherapy response. Nature (2020) 577(7791):549–55. doi: 10.1038/s41586-019-1922-8
24. Calderaro J, Petitprez F, Becht E, Laurent A, Hirsch TZ, Rousseau B, et al. Intra-tumoral tertiary lymphoid structures are associated with a low risk of early recurrence of hepatocellular carcinoma. J Hepatol (2019) 70(1):58–65. doi: 10.1016/j.jhep.2018.09.003
25. Blanchard L, Girard JP. High endothelial venules (HEVs) in immunity, inflammation and cancer. Angiogenesis (2021) 24(4):719–53. doi: 10.1007/s10456-021-09792-8
26. Vella G, Guelfi S, Bergers G. High endothelial venules: a vascular perspective on tertiary lymphoid structures in cancer. Front Immunol (2021) 12:736670. doi: 10.3389/fimmu.2021.736670
27. Mueller CG, Nayar S, Gardner D, Barone F. Cellular and vascular components of tertiary lymphoid structures. Methods Mol Biol (2018) 1845:17–30. doi: 10.1007/978-1-4939-8709-2_2
28. Jones E, Gallimore A, Ager A. Defining high endothelial venules and tertiary lymphoid structures in cancer. Methods Mol Biol (2018) 1845:99–118. doi: 10.1007/978-1-4939-8709-2_7
29. Cabrita R, Lauss M, Sanna A, Donia M, Skaarup Larsen M, Mitra S, et al. Tertiary lymphoid structures improve immunotherapy and survival in melanoma. Nature (2020) 577(7791):561–5. doi: 10.1038/s41586-019-1914-8
30. Martinet L, Garrido I, Filleron T, Le Guellec S, Bellard E, Fournie JJ, et al. Human solid tumors contain high endothelial venules: association with t-and b-lymphocyte infiltration and favorable prognosis in breast cancer. Cancer Res (2011) 71(17):5678–87. doi: 10.1158/0008-5472.CAN-11-0431
31. Colbeck EJ, Jones E, Hindley JP, Smart K, Schulz R, Browne M, et al. Treg depletion licenses t cell-driven HEV neogenesis and promotes tumor destruction. Cancer Immunol Res (2017) 5(11):1005–15. doi: 10.1158/2326-6066.CIR-17-0131
32. Jing F, Choi EY. Potential of cells and cytokines/chemokines to regulate tertiary lymphoid structures in human diseases. Immune Netw (2016) 16(5):271. doi: 10.4110/in.2016.16.5.271
33. Pimenta EM, Barnes BJ. Role of tertiary lymphoid structures (TLS) in anti-tumor immunity: potential tumor-induced cytokines/chemokines that regulate TLS formation in epithelial-derived cancers. Cancers (2014) 6(2):969–97. doi: 10.3390/cancers6020969
34. Li JP, Wu CY, Chen MY, Liu SX, Yan SM, Kang YF, et al. PD-1+ CXCR5- CD4+ th-CXCL13 cell subset drives b cells into tertiary lymphoid structures of nasopharyngeal carcinoma. J Immunother Cancer (2021) 9(7):e002101. doi: 10.1136/jitc-2020-002101
35. Rubio AJ, Porter T, Zhong X. Duality of b cell-CXCL13 axis in tumor immunology. Front Immunol (2020) 11:521110. doi: 10.3389/fimmu.2020.521110
36. Finch DK, Ettinger R, Karnell JL, Herbst R, Sleeman MA. Effects of CXCL 13 inhibition on lymphoid follicles in models of autoimmune disease. Eur J Clin Invest (2013) 43(5):501–9. doi: 10.1111/eci.12063
37. Razis E, Kalogeras KT, Kotsantis I, Koliou GA, Manousou K, Wirtz R, et al. The role of CXCL13 and CXCL9 in early breast cancer. Clin Breast Cancer (2020) 20(1):e36–53. doi: 10.1016/j.clbc.2019.08.008
38. Wei Y, Lin C, Li H, Xu Z, Wang J, Li R, et al. CXCL13 expression is prognostic and predictive for postoperative adjuvant chemotherapy benefit in patients with gastric cancer. Cancer Immunol Immunother (2018) 67:261–9. doi: 10.1007/s00262-017-2083-y
39. Havenar-Daughton C, Lindqvist M, Heit A, Wu JE, Reiss SM, Kendric K, et al. CXCL13 is a plasma biomarker of germinal center activity. Proc Natl Acad Sci USA (2016) 113(10):2702–7. doi: 10.1073/pnas.1520112113
40. Xu W, Ma C, Liu W, Anwaier A, Tian X, Shi G, et al. Prognostic value, DNA variation and immunologic features of a tertiary lymphoid structure-related chemokine signature in clear cell renal cell carcinoma. Cancer Immunol Immunother (2022) 71(8):1923–935. doi: 10.1007/s00262-021-03123-y
41. Hsieh CH, Jian CZ, Lin LI, Low GS, Ou PY, Hsu C, et al. Potential role of CXCL13/CXCR5 signaling in immune checkpoint inhibitor treatment in cancer. Cancers (2022) 14(2):294. doi: 10.3390/cancers14020294
42. Barata JT, Durum SK, Seddon B. Flip the coin: IL-7 and IL-7R in health and disease. Nat Immunol (2019) 20(12):1584–93. doi: 10.1038/s41590-019-0479-x
43. Coppola C, Hopkins B, Huhn S, Du Z, Huang Z, Kelly WJ. Investigation of the impact from IL-2, IL-7, and IL-15 on the growth and signaling of activated CD4+ t cells. Int J Mol Sci (2020) 21(21):7814. doi: 10.3390/ijms21217814
44. Alpdogan ON, Van Den Brink MR. IL-7 and IL-15: therapeutic cytokines for immunodeficiency. Trends Immunol (2005) 26(1):56–64. doi: 10.1016/j.it.2004.11.002
45. Ceredig R, Rolink AG. The key role of IL-7 in lymphopoiesis. Semin Immunol (2012) 24(3):159–64. doi: 10.1016/j.smim.2012.02.004
46. Mackall CL, Fry TJ, Gress RE. Harnessing the biology of IL-7 for therapeutic application. Nat Rev Immunol (2011) 11(5):330–42. doi: 10.1038/nri2970
47. Iolyeva M, Aebischer D, Proulx ST, Willrodt AH, Ecoiffier T, Häner S, et al. Interleukin-7 is produced by afferent lymphatic vessels and supports lymphatic drainage. Blood (2013) 122(13):2271–81. doi: 10.1182/blood-2013-01-478073
48. Onder L, Narang P, Scandella E, Chai Q, Iolyeva M, Hoorweg K, et al. IL-7-producing stromal cells are critical for lymph node remodeling. Blood (2012) 120(24):4675–83. doi: 10.1182/blood-2012-03-416859
49. Nayar S, Campos J, Chung MM, Navarro-Nunez L, Chachlani M, Steinthal N, et al. Bimodal expansion of the lymphatic vessels is regulated by the sequential expression of IL-7 and lymphotoxin α1β2 in newly formed tertiary lymphoid structures. J Immunol (2016) 197(5):1957–67. doi: 10.4049/jimmunol.1500686
50. Van De Pavert SA. Lymphoid tissue inducer (LTi) cell ontogeny and functioning in embryo and adult. BioMed J (2021) 44(2):123–32. doi: 10.1016/j.bj.2020.12.003
51. Cherrier M, Eberl G. The development of LTi cells. Curr Opin Immunol (2012) 24(2):178–83. doi: 10.1016/j.coi.2012.02.003
52. Strober W. The LTi cell, an immunologic chameleon. Immunity (2010) 33(5):650–2. doi: 10.1016/j.immuni.2010.11.016
53. Ruddle NH. Basics of inducible lymphoid organs. Curr Top Microbiol Immunol (2020) 426:1–19. doi: 10.1007/82_2020_218
54. Crellin NK, Trifari S, Kaplan CD, Satoh-Takayama N, Di Santo JP, Spits H. Regulation of cytokine secretion in human CD127+ LTi-like innate lymphoid cells by toll-like receptor 2. Immunity (2010) 33(5):752–64. doi: 10.1016/j.immuni.2010.10.012
55. Vondenhoff MF, Greuter M, Goverse G, Elewaut D, Dewint P, Ware CF, et al. LTbetaR signaling induces cytokine expression and up-regulates lymphangiogenic factors in lymph node anlagen. J Immunol (2009) 182(9):5439–45. doi: 10.4049/jimmunol.0801165
56. Ciccia F, Guggino G, Rizzo A, Saieva L, Peralta S, Giardina A, et al. Type 3 innate lymphoid cells producing IL-17 and IL-22 are expanded in the gut, in the peripheral blood, synovial fluid and bone marrow of patients with ankylosing spondylitis. Ann Rheum Dis (2015) 74(9):1739–47. doi: 10.1136/annrheumdis-2014-206323
57. Comerford I, Harata-Lee Y, Bunting MD, Gregor C, Kara EE, Mccoll SR. A myriad of functions and complex regulation of the CCR7/CCL19/CCL21 chemokine axis in the adaptive immune system. Cytokine Growth Factor Rev (2013) 24(3):269–83. doi: 10.1016/j.cytogfr.2013.03.001
58. Van De Pavert SA, Olivier BJ, Goverse G, Vondenhoff MF, Greuter M, Beke P, et al. Chemokine CXCL13 is essential for lymph node initiation and is induced by retinoic acid and neuronal stimulation. Nat Immunol (2009) 10(11):1193–9. doi: 10.1038/ni.1789
59. Cupedo T, Jansen W, Kraal G, Mebius RE. Induction of secondary and tertiary lymphoid structures in the skin. Immunity (2004) 21(5):655–67. doi: 10.1016/j.immuni.2004.09.006
60. Picarella DE, Kratz A, Li CB, Ruddle NH, Flavell RA. Insulitis in transgenic mice expressing tumor necrosis factor beta (lymphotoxin) in the pancreas. Proc Natl Acad Sci USA (1992) 89(21):10036–40. doi: 10.1073/pnas.89.21.10036
61. Drayton DL, Ying X, Lee J, Lesslauer W, Ruddle NH. Ectopic LTαβ directs lymphoid organ neogenesis with concomitant expression of peripheral node addressin and a HEV-restricted sulfotransferase. J Exp Med (2003) 197(9):1153–63. doi: 10.1084/jem.20021761
62. Lee SI, Celik S, Logsdon BA, Lundberg SM, Martins TJ, Oehler VG, et al. A machine learning approach to integrate big data for precision medicine in acute myeloid leukemia. Nat Commun (2018) 9(1):42. doi: 10.1038/s41467-017-02465-5
63. Handelman GS, Kok HK, Chandra RV, Razavi AH, Lee MJ, Asadi H. eDoctor: machine learning and the future of medicine. J Intern Med (2018) 284(6):603–19. doi: 10.1111/joim.12822
64. Lee YW, Choi JW, Shin EH. Machine learning model for predicting malaria using clinical information. Comput Biol Med (2021) 129:104151. doi: 10.1016/j.compbiomed.2020.104151
65. Sultan AS, Elgharib MA, Tavares T, Jessri M, Basile JR. The use of artificial intelligence, machine learning and deep learning in oncologic histopathology. J Oral Pathol Med (2020) 49(9):849–56. doi: 10.1111/jop.13042
66. Kan A. Machine learning applications in cell image analysis. Immunol Cell Biol (2017) 95(6):525–30. doi: 10.1038/icb.2017.16
67. Erickson BJ. Machine learning: discovering the future of medical imaging. J Digit Imaging (2017) 30(4):391. doi: 10.1007/s10278-017-9994-1
68. Merali ZG, Witiw CD, Badhiwala JH, Wilson JR, Fehlings MG. Using a machine learning approach to predict outcome after surgery for degenerative cervical myelopathy. PloS One (2019) 14(4):e0215133. doi: 10.1371/journal.pone.0215133
69. Liu X, Yuan P, Li R, Zhang D, An J, Ju J, et al. Predicting breast cancer recurrence and metastasis risk by integrating color and texture features of histopathological images and machine learning technologies. Comput Biol Med (2022) 146:105569. doi: 10.1016/j.compbiomed.2022.105569
70. Sammut SJ, Crispin-Ortuzar M, Chin SF, Provenzano E, Bardwell HA, Ma W, et al. Multi-omic machine learning predictor of breast cancer therapy response. Nature (2022) 601(7894):623–9. doi: 10.1038/s41586-021-04278-5
71. Yu Y, He Z, Ouyang J, Tan Y, Chen Y, Gu Y, et al. Magnetic resonance imaging radiomics predicts preoperative axillary lymph node metastasis to support surgical decisions and is associated with tumor microenvironment in invasive breast cancer: A machine learning, multicenter study. Ebiomedicine (2021) 69:103460. doi: 10.1016/j.ebiom.2021.103460
72. Dieci MV, Miglietta F, Guarneri V. Immune infiltrates in breast cancer: recent updates and clinical implications. Cells (2021) 10(2):223. doi: 10.3390/cells10020223
73. Mehraj U, Dar AH, Wani NA, Mir MA. Tumor microenvironment promotes breast cancer chemoresistance. Cancer Chemother Pharmacol (2021) 87(2):147–58. doi: 10.1158/0008-5472.Can-10-0538
74. Wang S, Xiong Y, Zhang Q, Su D, Yu C, Cao Y, et al. Clinical significance and immunogenomic landscape analyses of the immune cell signature based prognostic model for patients with breast cancer. Brief Bioinform (2021) 22(4):bbaa311. doi: 10.1093/bib/bbaa311
75. Tzeng A, Sangwan N, Jia M, Liu CC, Keslar KS, Downs-Kelly E, et al. Human breast microbiome correlates with prognostic features and immunological signatures in breast cancer. Genome Med (2021) 13:60. doi: 10.1186/s13073-021-00874-2
76. Sadeghalvad M, Mohammadi-Motlagh HR, Rezaei N. Immune microenvironment in different molecular subtypes of ductal breast carcinoma. Breast Cancer Res Treat (2021) 185:261–79. doi: 10.1007/s10549-020-05954-2
77. Hieken TJ, Chen J, Hoskin TL, Walther-Antonio M, Johnson S, Ramaker S, et al. The microbiome of aseptically collected human breast tissue in benign and malignant disease. Sci Rep (2016) 6(1):30751. doi: 10.1038/srep30751
78. Xuan C, Shamonki JM, Chung A, Dinome ML, Chung M, Sieling PA, et al. Microbial dysbiosis is associated with human breast cancer. PloS One (2014) 9(1):e83744. doi: 10.1371/journal.pone.0083744
79. Banerjee S, Tian T, Wei Z, Robertson ES. Distinct microbial signatures associated with different breast cancer types. Front Microbiol (2018) 9:951. doi: 10.3389/fmicb.2018.00951
80. Goedert JJ, Jones G, Hua X, Xu X, Yu G, Flores R, et al. Investigation of the association between the fecal microbiota and breast cancer in postmenopausal women: a population-based case-control pilot study. J Natl Cancer Inst Monogr (2015) 107(8):djv147. doi: 10.1093/jnci/djv147
81. Rouanne M, Arpaia N, Marabelle A. CXCL13 shapes tertiary lymphoid structures and promotes response to immunotherapy in bladder cancer. Eur J Cancer (2021) 151:245–8. doi: 10.1016/j.ejca.2021.03.054
82. Li J, Yan S, Xu J, Li Y. Progess of IL-37 inhibiting inflammatory diseases by regulating macrophage polarization. Mod Med Health (2023) 39(20):3527–31. doi: 10.3969/j.issn.1009-5519.2023.20.023
83. Locati M, Curtale G, Mantovani A. Diversity, mechanisms, and significance of macrophage plasticity. Annu Rev Pathol (2020) 15:123–47. doi: 10.1146/annurev-pathmechdis-012418-012718
84. Wu K, Lin K, Li X, Yuan X, Xu D. Redefining tumor-associated macrophage subpopulations and functions in the tumor microenvironment. Front Immunol (2020) 11:1731. doi: 10.3389/fimmu.2020.01731
85. Zhou D, Huang C, Lin Z, Zhan S, Kong L, Fang C, et al. Macrophage polarization and function with emphasis on the evolving roles of coordinated regulation of cellular signaling pathways. Cell Signal (2014) 26(2):192–7. doi: 10.1016/j.cellsig.2013.11.004
86. Olson OC, Kim H, Quail DF, Foley EA, Joyce JA. Tumor-associated macrophages suppress the cytotoxic activity of antimitotic agents. Cell Rep (2017) 19(1):101–3. doi: 10.1016/j.celrep.2017.03.038
87. Wang QY, Zhao Y, Shen XF, Du JF. Progress on the role of tertiary lymphatic structure in colorectal cancer. Chin J Pract Surg (2021) 41(7):820–4. doi: 10.19538/j.cjps.issn1005-2208.2021.07.20
88. Barb AC, Pasca Fenesan M, Pirtea M, Margan MM, Tomescu L, Melnic E, et al. Tertiary lymphoid structures (TLSs) and stromal blood vessels have significant and heterogeneous impact on recurrence, lymphovascular and perineural invasion amongst breast cancer molecular subtypes. Cells (2023) 12(8):1176. doi: 10.3390/cells12081176
89. Mustapha R, Ng K, Monypenny J, Ng T. Insights into unveiling a potential role of tertiary lymphoid structures in metastasis. Front Mol Biosci (2021) 8:661516. doi: 10.3389/fmolb.2021.661516
90. Buisseret L, Garaud S, De Wind A, Van Den Eynden G, Boisson A, Solinas C, et al. Tumor-infiltrating lymphocyte composition, organization and PD-1/PD-L1 expression are linked in breast cancer. Oncoimmunology (2017) 6(1):e1257452. doi: 10.1080/2162402X.2016.1257452
91. Solinas C, Garaud S, De Silva P, Boisson A, Van den Eynden G, De Wind A, et al. Immune checkpoint molecules on tumor-infiltrating lymphocytes and their association with tertiary lymphoid structures in human breast cancer. Front Immunol (2017) 8:291667. doi: 10.3389/fimmu.2017.01412
92. Seow DYB, Yeong JPS, Lim JX, Chia N, Lim JCT, Ong CCH, et al. Tertiary lymphoid structures and associated plasma cells play an important role in the biology of triple-negative breast cancers. Breast Cancer Res Treat (2020) 180(6):369–77. doi: 10.1007/s10549-020-05548-y
93. Yin L, Duan JJ, Bian XW, Yu SC. Triple-negative breast cancer molecular subtyping and treatment progress. Breast Cancer Res (2020) 22:1–13. doi: 10.1186/s13058-020-01296-5
94. Garrido-Castro AC, Lin NU, Polyak K. Insights into molecular classifications of triple-negative breast cancer: improving patient selection for treatment. Cancer Discov (2019) 9(2):176–98. doi: 10.1158/2159-8290.CD-18-1177
95. Derakhshan F, Reis-Filho JS. Pathogenesis of triple-negative breast cancer. Ann Rev Pathol Mech Dis (2022) 17:181–204. doi: 10.1146/annurev-pathol-042420-093238
96. Li Y, Zhang H, Merkher Y, Chen L, Liu N, Leonov S, et al. Recent advances in therapeutic strategies for triple-negative breast cancer. J Hematol Oncol (2022) 15(1):121. doi: 10.1186/s13045-022-01341-0
97. He MY, Rancoule C, Rehailia-Blanchard A, Espenel S, Trone JC, Bernichon E, et al. Radiotherapy in triple-negative breast cancer: Current situation and upcoming strategies. Crit Rev Oncol Hematol (2018) 131:96–101. doi: 10.1016/j.critrevonc.2018.09.004
98. Gadi VK, Davidson NE. Practical approach to triple-negative breast cancer. J Oncol Pract (2017) 13(5):293–300. doi: 10.1200/JOP.2017.022632
99. Guney Eskiler G, Cecener G, Egeli U, Tunca B. Triple negative breast cancer: new therapeutic approaches and BRCA status. Apmis (2018) 126(5):371–9. doi: 10.1111/apm.12836
100. Papa A, Caruso D, Tomao S, Rossi L, Zaccarelli E, Tomao F. Triple-negative breast cancer: investigating potential molecular therapeutic target. Expert Opin Ther Targets (2015) 19(1):55–75. doi: 10.1517/14728222.2014.970176
101. Khosravi-Shahi P, Cabezón-Gutiérrez L, Custodio-Cabello S. Metastatic triple negative breast cancer: optimizing treatment options, new and emerging targeted therapies. Asia Pac J Clin Oncol (2018) 14(1):32–9. doi: 10.1111/ajco.12748
102. Zuo ZJ, Tian TL, Luo T. Progress in immunotherapy of breast cancer. West China Med J (2023) 38(11):1766–9. doi: 10.7507/1002-0179.202212167
103. Szeto GL, Finley SD. Integrative approaches to cancer immunotherapy. Trends Cancer (2019) 5(7):400–10. doi: 10.1016/j.trecan.2019.05.010
104. Cha JH, Chan LC, Song MS, Hung MC. New approaches on cancer immunotherapy. Cold Spring Harb Perspect Med (2020) 10(8):a036863. doi: 10.1101/cshperspect.a036863
105. Hong WX, Haebe S, Lee AS, Westphalen CB, Norton JA, Jiang W, et al. Intratumoral immunotherapy for early-stage solid tumors. Clin Cancer Res (2020) 26(13):3091–9. doi: 10.1158/1078-0432.CCR-19-3642
106. Lee HJ, Park IA, Song IH, Shin SJ, Kim JY, Yu JH, et al. Tertiary lymphoid structures: prognostic significance and relationship with tumour-infiltrating lymphocytes in triple-negative breast cancer. J Clin Pathol (2016) 69(5):422–30. doi: 10.1136/jclinpath-2015-203089
107. Figenschau SL, Fismen S, Fenton KA, Fenton C, Mortensen ES. Tertiary lymphoid structures are associated with higher tumor grade in primary operable breast cancer patients. BMC Cancer (2015) 15:1–11. doi: 10.1186/s12885-015-1116-1
108. Schmid P, Cortes J, Pusztai L, McArthur H, Kümmel S, Bergh J, et al. Pembrolizumab for early triple-negative breast cancer. N Engl J Med (2020) 382(9):810–21. doi: 10.1056/NEJMoa1910549
109. Song IH, Heo SH, Bang WS, Park HS, Park IA, Kim YA, et al. Predictive value of tertiary lymphoid structures assessed by high endothelial venule counts in the neoadjuvant setting of triple-negative breast cancer. Cancer Res Treat (2017) 49(2):399–407. doi: 10.4143/crt.2016.215
110. Liu X, Tsang JY, Hlaing T, Hu J, Ni YB, Chan SK, et al. Distinct tertiary lymphoid structure associations and their prognostic relevance in HER2 positive and negative breast cancers. Oncologist (2017) 22(11):1316–24. doi: 10.1634/theoncologist.2017-0029
111. Arab A, Yazdian-Robati R, Behravan J. HER2-positive breast cancer immunotherapy: a focus on vaccine development. Arch Immunol Ther Exp (Warsz) (2020) 68(1):2. doi: 10.1007/s00005-019-00566-1
112. Schettini F, Prat A. Dissecting the biological heterogeneity of HER2-positive breast cancer. Breast (2021) 59:339–50. doi: 10.1016/j.breast.2021.07.019
113. Connell CM, Doherty GJ. Activating HER2 mutations as emerging targets in multiple solid cancers. ESMO Open (2017) 2(5):e000279. doi: 10.1136/esmoopen-2017-000279
114. Zhang H, Moisini I, Turner BM, Wang X, Dhakal A, Yang Q, et al. Significance of HER2 in microinvasive breast carcinoma: A single academic institution experience. Am J Clin Pathol (2021) 156(1):155–65. doi: 10.1093/ajcp/aqaa222
115. Gaibar M, Beltrán L, Romero-Lorca A, Fernández-Santander A, Novillo A. Somatic mutations in HER2 and implications for current treatment paradigms in HER2-positive breast cancer. J Oncol (2020) 2020:6375956. doi: 10.1155/2020/6375956
116. Lee HJ, Kim JY, Park IA, Song IH, Yu JH, Ahn JH, et al. Prognostic significance of tumor-infiltrating lymphocytes and the tertiary lymphoid structures in HER2-positive breast cancer treated with adjuvant trastuzumab. Am J Clin Pathol (2015) 144(2):278–88. doi: 10.1309/AJCPIXUYDVZ0RZ3G
Keywords: breast cancer, tertiary lymphoid structures, microorganisms, immunity, machine learning
Citation: Li X, Xu H, Du Z, Cao Q and Liu X (2024) Advances in the study of tertiary lymphoid structures in the immunotherapy of breast cancer. Front. Oncol. 14:1382701. doi: 10.3389/fonc.2024.1382701
Received: 13 February 2024; Accepted: 18 March 2024;
Published: 02 April 2024.
Edited by:
Gang Ye, Sichuan Agricultural University, ChinaReviewed by:
Lingxuan An, Ludwig Maximilian University of Munich, GermanyLifang Liu, Hunan University of Chinese Medicine, China
Copyright © 2024 Li, Xu, Du, Cao and Liu. This is an open-access article distributed under the terms of the Creative Commons Attribution License (CC BY). The use, distribution or reproduction in other forums is permitted, provided the original author(s) and the copyright owner(s) are credited and that the original publication in this journal is cited, in accordance with accepted academic practice. No use, distribution or reproduction is permitted which does not comply with these terms.
*Correspondence: Xiaofei Liu, drliuxf@126.com
†These authors have contributed equally to this work