- 1Key Laboratory of Oral Diseases Research of Anhui Province, College and Hospital of Stomatology, Anhui Medical University, Hefei, China
- 2Department of Physiology, School of Basic Medical Sciences, Anhui Medical University, Hefei, China
Background: Circular RNA (circRNA) has an important influence on oral squamous cell carcinoma (OSCC) progression as competing endogenous RNAs (ceRNAs). However, the link between ceRNAs and the OSCC immune microenvironment is unknown. The research aimed to find circRNAs implicated in OSCC carcinogenesis and progression and build a circRNA-based ceRNA network to create a reliable OSCC risk prediction model.
Methods: The expression profiles of circRNA in OSCC tumors and normal tissues were assessed through RNA sequencing. From the TCGA database, clinicopathological data and expression patterns of microRNAs (miRNAs) and mRNAs were obtained. A network of circRNA-miRNA-mRNA ceRNA was prepared according to these differentially expressed RNAs and was analyzed through functional enrichment. Subsequently, based on the mRNA in the ceRNA network, the influence of the model on prognosis was then evaluated using a risk prediction model. Finally, considering survival, tumor-infiltrating immune cells (TICs), clinicopathological features, immunosuppressive molecules, and chemotherapy efficacy were analyzed.
Results: Eleven differentially expressed circRNAs were found in cancer tissues relative to healthy tissues. We established a network of circRNA-miRNA-mRNA ceRNA, and the ceRNA network includes 123 mRNAs, six miRNAs, and four circRNAs. By the assessment of Genomes pathway and Kyoto Encyclopedia of Genes, it is found that in the cellular senescence, PI3K-AKT and mTOR signaling pathway mRNAs were mainly enrichment. An immune-related signature was created utilizing seven immune-related genes in the ceRNA network after univariate and multivariate analysis. The receiver operating characteristic of the nomogram exhibited satisfactory accuracy and predictive potential. According to a Kaplan-Meier analysis, the high-risk group’s survival rate was signally lower than the group with low-risk. In addition, risk models were linked to clinicopathological characteristics, TICs, immune checkpoints, and antitumor drug susceptibility.
Conclusion: The profiles of circRNAs expression of OSCC tissues differ significantly from normal tissues. Our study established a circRNA-associated ceRNA network associated with OSCC and identified essential prognostic genes. Furthermore, our proposed immune-based signature aims to help research OSCC etiology, prognostic marker screening, and immune response evaluation.
Introduction
Oral squamous cell carcinoma (OSCC) is a group of malignant diseases that develop on the surfaces of the lips, gums, tongue, cheeks, and palate (Wong and Wiesenfeld, 2018). In 2020, the International Agency for Research on Cancer (IARC) reported that there were approximately 3,77,713 new cases of lip and oral cancer worldwide, accounting for 2.1% of all cancers (Kalogirou et al., 2021). OSCC is three times more common in men than women, and the average age of diagnosis is 50, although it also occurs in younger populations (Conway et al., 2018; Mello et al., 2018; Jehn et al., 2019). The occurrence and development of OSCC result from a combination of factors. Its main risk factors include tobacco and alcohol consumption and other possible risk factors, such as chronic irritation, poor oral hygiene, human papillomavirus (HPV), poor nutrition, and suppressed immune system (Petersen, 2009; Oji and Chukwuneke, 2012; Spence et al., 2016). These risk factors cause various genetic instabilities and molecular alterations, including loss of heterozygosity on chromosomes 3, 4, 7, 8, 11, 17, and 19, and downregulation of tumor suppressor genes such as TP53, RB, CDKN2A, and cancer upregulation of genes such as cyclin D1 (Leemans et al., 2011; Schaal and Chellappan, 2014; Alsahafi et al., 2019). Over the past few decades, there has been considerable progress in comprehensive treatment modalities for OSCC, with current treatments including radical surgery, radiation therapy, and chemotherapy (Oosting and Haddad, 2019). However, due to its late detection and high recurrence rate, mortality remains high, and the prognosis is relatively poor (Chang et al., 2021). Therefore, it is essential to explore the molecular mechanism of OSCC and the development of its malignant biological behavior and find effective targets for OSCC treatment.
At present, factors such as immune system disorders and tumor microenvironment (TME) have been confirmed to affect tumors’ occurrence, development, invasion, and drug resistance (Wu and Dai., 2017; Hinshaw and Shevde, 2019). The TME consists of tumor cells, tumor-associated stromal cells, and the extracellular matrix (Gao et al., 2019). TME can enhance or inhibit therapeutic effects and may have variable activation states (Vuong et al., 2019). Modifying or regulating specific factors or cells in the TME is particularly beneficial for treating tumors, such as immune checkpoint inhibitors (ICIs) (Liu et al., 2017). However, the distribution and mechanism of action of complex TMEs in OSCC have not been elucidated. In addition, it is necessary to explore methods further to accurately predict the efficacy of OSCC immunotherapy and find immunotherapy-related markers.
The circular RNA (circRNA) genome was discovered in a virus in 1976, but its role in gene regulation and cancer formation has only recently been discovered (Li et al., 2015). CircRNAs are a new type of non-protein-coding RNAs that are single-stranded with the head 3′ and tail 5′ ends covalently bound together to form a circular form (Geng et al., 2018). Due to the special covalently closed circular molecular structure, circRNAs are more resistant to degradation and more stable than traditional linear RNAs (Chen et al., 2016). In addition, circRNAs are suggested to be the universal molecules distributed in human cells; and in some circumstances, circRNAs are way more abundant than their linear isoforms. Many studies have shown that circRNAs can competitively adsorb miRNAs, thereby regulating gene expression at the post-transcriptional level (Gao et al., 2016). The circRNA hsa_circ_0009128 has been reported to be associated with the malignant progression of OSCC by targeting MMP9 to activate epithelial-mesenchymal transition (EMT) to stimulate OSCC cell proliferation and migration (Zhang et al., 2021). Cui et al. (2021) demonstrated that CircCDR1as elevates SLC7A11 as an oncogene for OSCC progression by targeting miR-876-5p. Shi et al. (2021) discovered that circGDI2 is a tumor suppressor that plays a role in OSCC pathogenesis by regulating FOXF2 expression through miR-454-3p. Furthermore, studies have shown that key nodal factors in the ceRNA network affect the proportion of tumor infiltrating immune cells (TICs) in the TME and the efficacy of immunotherapy. Wang et al. (2020) found that circRNA-002178 induced PD1 expression by sponging miR-34, thereby inducing T cell depletion. Ou et al. (2019) showed that circ_0000977 promoted the accumulation of hypoxia-inducible factor 1-alpha (HI1FA), inhibited NK cell lysis and led to immune escape of pancreatic cancer cells. However, there are few studies on the immune infiltration patterns of ceRNA and OSCC. At present, most of these studies use lncRNAs as the starting point to establish ceRNA networks and perform immune infiltration analysis. There is still a lack of related research on circRNAs.
In this study, we analyzed differentially expressed genes, including circRNAs, miRNAs, and mRNAs, based on high-throughput sequencing and the TCGA database. Public databases were used to examine interactions between circRNAs, miRNAs, and mRNAs, and to construct ceRNA networks. Genes within the ceRNA network were profiled by Gene Ontology (GO) and Kyoto Encyclopedia of Genes and Genomes (KEGG) pathway analysis. A prognostic mRNA signature associated with OSCC was established using univariate, Least Absolute Shrinkage and Selection Operator (LASSO) and multivariate Cox proportional hazards regression analysis and validated using time-dependent Receiver Operating Characteristic (ROC) curve analysis. Finally, we also assessed the association of this risk model with tumor-infiltrating immune cells or immune-related molecules, and the relationship of this model with the efficacy of chemotherapy in OSCC. Our study will contribute to a better understanding of the regulatory ceRNA network and provide a reliable reference for developing therapeutic targets for OSCC.
Materials and methods
Patient tissue samples and cell lines
For high-throughput sequencing, three pairs of quickly frozen OSCC tissue and surrounding healthy tissue were obtained from patients through the operation. Then, twenty pairs of samples were used for circRNA validation by quantitative real-time polymerase chain reaction (qRT-PCR), including samples for high-throughput sequencing analysis. Before the procedure, all experimental patient samples received no other therapies, and a thorough pathologic analysis validated all oral squamous cell carcinoma tissues. Each patient signed a written informed permission form, and the Medical Ethics Committee approved the study at Anhui Medical University’s Affiliated Stomatological Hospital. Each method was conducted as per appropriate regulations and guidelines.
HNSCC cell lines SCC9, CAL27, HN4, HN6, and human normal oral epithelial keratinocytes (HOK) were purchased from Ninth People’s Hospital Affiliated with Shanghai Jiaotong University School of Medicine in Shanghai (China). All cell lines were subjected to STR profiling and tested for Mycoplasma contamination every 3 months. These cell lines were saved in DMEM (BI, Israel) supplemented with 10% fetal bovine serum (BI, Israel), 1% penicillin, and streptomycin (NCM, Suzhou, China). Moreover, these cell lines were cultured at 37°C in a humidified incubator containing 5% CO2.
Extraction of RNA and assessment of quality
Total RNA was extracted from frozen tumor tissues (C group) and matched non-cancerous tissues (N group) using Thermo Fisher Scientific’s TRIzol reagent (United States), according to the manufacturer’s instructions. Genomic DNA (gDNA) was extracted from tissues using PureLink Genomic DNA Mini Kit according to the manufacturer’s instructions (Thermo Fisher Scientific, K182001). A NanoDrop Spectrophotometer (Thermo Fisher Scientific, United States) was used to determine the concentration and purity of RNA. The OD value was measured after taking 1 μl RNA sample 50 times diluted, and the ratio of OD260/OD280 was greater than 1.8, indicating that the prepared RNA was pure and free from protein contamination. RNA samples were taken 1 μl, 1% agarose gel electrophoresis at 80 V × 20 min, EB staining for 10 min, observed and photographed with gel imaging system, the total RNA extraction was proved to be complete if the three bands were complete. RNA samples were stored at −80°C.
Library preparation and sequencing
Libraries for the sequencing of were prepared from 2 μg of total RNA with the following modification. We removed ribosomal RNA using the Epicentre Ribo-Zero™ rRNA Removal Kit (Human/Mouse/Rat). Linear RNA was removed using RNase R (Epicentre, lnc). Magnetic beads with attached poly-T oligos were used to remove residual poly-A RNA. For fragmentation, divalent cations were used under increased temperature in an proprietary fragmentation buffer from Illumina. Using RNA as a template and primers of random oligonucleotides, synthesize cDNA first strand, then RNaseH was used to degrade the strand of RNA, and dNTP with dUTP instead of dTTP was used as the raw material to synthesize the cDNA second strand with in the DNA polymerase I system. Purify the cDNA, then perform double-end repair and introduce the “A” base at the 3′end and connect the sequencing adapter. At this time, USER enzyme (NEB, United States) was included in the system for the degradation of cDNA second strand of containing U. To select cDNA fragments of the preferred 0.4–0.5 Kbp length, purification of the library fragments was conducted using the AMPure XP system (Beckman Coulter, United States). Fragments of DNA having ligated adaptor molecules on the two ends were specifically enriched using the PCR Primer Cocktail from Illumina in a PCR reaction (15 cycle). After product purification (AMPure XP system) they were quantified through the Agilent high sensitivity DNA assay on Agilent’s Bioanalyzer 2100 system. This was followed by sequencing the library on Illumina’s NovaSeq 6000 platform (Shanghai Personal Biotechnology Cp. Ltd.).
Differential expression analysis
To determine differentially expressed CricRNAs, DESeq (1.30.0) was used, and transcripts having |log2FoldChange| > 1 and p-value < 0.05 were considered differentially expressed CricRNAs. A heatmap package in R was used for the visual assessment of differentially expressed RNA. CSCD (http://gb.whu.edu.cn/CSCD/) is an online tool to study circRNAs specific to cancer and obtain probable structures of circRNA structures.
Quantitative real-time polymerase reaction
By using the Prime Script RT Master Mix from Takara (Cat. #RR047A), the RNA (total) which were isolated from 20 samples were reversely transcribed. qRT-PCR was conducted in CFX96 Touch Real-Time PCR Detection System (Bio-Rad, Hercules, CA, United States) per the manufacturer’s protocol. For internal reference, GAPDH was used, and the reaction was conducted in two steps. The conditions for the reaction were: pre-denaturation at 95°C for 30 s; denaturation at 95°C for 5 s, and annealing/extension at 60°C for 30 s for 50 cycles. The threshold cycle (Ct) approach was used to estimate expression, and the 2−ΔΔCt method calculated relative expression levels. Information about primers is shown in Table 1.
Competing endogenous RNA network construction
Based on the ceRNA theory, a circRNA-miRNA-mRNA regulatory network was prepared. The circBase database (http://www.circbase.org/cgi-bin/getseq.cgi) provided CircRNA information. Then, CircInteractome (https://circinteractome.nia.nih.gov/index.html) was used to determine DEcircRNA target miRNAs. These target miRNAs were crossed with DEmiRNAs in the OSCC patient dataset of the TGCA database using the Venn package, overlapping miRNAs were found, and finally, circRNA-miRNA pairs were established. We predicted the target mRNAs for intersecting miRNAs using Starbase (https://starbase.sysu.edu.cn) databases, miRDB (http://www.mirdb.org/mirdb/index.html), and TargetScan (http://www.targetscan.org/vert_72/), and only the genes that were found in all three databases at the same time were considered as likely mRNA targets. To obtain miRNA-mRNA pairs, the TCGA dataset was again used to intersect these target mRNAs with DEmRNAs. CirRNAs operate as miRNA molecular sponges, according to the ceRNA theory, and regulate mRNA expression. CircRNA expression is negatively connected with its target miRNA, while miRNA expression is adversely correlated with its target mRNA. A ceRNA regulatory network was prepared according to this principle using the above-identified circRNAs, miRNAs, and mRNAs. Finally, visualization of the ceRNA network was done using Cytoscape 3.8.2 software.
Gene ontology and kyoto encyclopedia of genes and genomes enrichment analysis
On all mRNAs in the ceRNA network, KEGG (http://www.kegg.jp/) and GO (http://geneontology.org/) enrichment analyses were performed. Each of the genes was mapped to each term in the GO database, and for each term, the numbers of mRNAs were calculated using the hypergeometric distribution. GO terms with p-value corrected to ≤0.05 were deemed enriched significantly. KEGG automatic annotation server (KAAS) was applied for pathway annotation using the complete genome as the background. The hypergeometric distribution was applied to estimate the significant mRNAs enrichment pathway; a p-value ≤ 0.05 were deemed significantly enriched.
Construction and validation of prognostic models
The association of DEmRNAs with overall survival (OS) and univariate Cox regression analysis were examined for the association between prognosis in patients with OSCC genes in the ceRNA network. Each DEmRNAs with p-value < 0.05 were finally chosen as a candidate gene for further analysis. To avoid overfitting, LASSO analysis was used, and the most appropriate prognostic DEmRNA was identified. After that, a multivariate Cox regression analysis was used to determine an optimal risk score. The risk score for patients with OSCC was estimated as follows: Risk score =
A chi-square test was used to verify the practicability of the generative model for clinical application and assess the association between clinicopathological features and risk scores. Band plots were drawn for visualization. The Wilcoxon signed-rank test was also used to see any differences in risk ratings across groups depending on clinical features, with the results displayed in box plots.
Assessment of tumor-infiltrating immune cells
To examine if there was a link between immune cells and risk scores in the tumor microenvironment, we employed well-established approaches such as TIMER, XCELL, EPIC, QUANTISEQ, CIBERSORT, MCPCOUNTER, and CIBERSORT-ABS to analyze TICs in samples. The relationship between risk scores and TICs was estimated by Spearman correlation analysis, and the correlation coefficient is shown in the lollipop plot (significance threshold p < 0.05). The R ggplot two package uses this operation.
Investigation of the expression of ICI-Related molecules
The “limma,” “reshape2,” “ggplot2,” and “ggpubr” packages in R were used to examine the expression of immune checkpoint genes in high-risk and low-risk patients, and immunotherapy score data was received from TCIA. To further evaluate the prognostic function of our risk model, sensitivity to immunotherapy was assessed for patients in high-risk and low-risk groups.
Chemotherapeutic drug sensitivity analysis
To assess the value of features in predicting treatment effect in OSCC, we calculated IC50s for standard chemotherapeutics and molecularly targeted drugs per sample applying pRRophetic. Guidelines recommend using antitumor drugs such as gefitinib, doxorubicin, gemcitabine, and rapamycin to treat OSCC. The Wilcoxon signed-rank test compared IC50 differences between high-risk and low-risk groups, and we used the “ggplot2” R package to show the results as box plots.
Cell transfection
We employed a negative control (NC) oligonucleotide and short interfering RNA (siRNA) to target hsa_circ_0005991. For cell culture, CAL27 cells were seeded in 6-well plates. Then, using Liposome 2000 (Invitrogen, United States) by the manufacturer’s instructions, siRNAs or controls (General, Anhui, China) were added to the cells at a final concentration of 50 nM.
Cell counting kit-8 proliferation assay
In each well of a 96-well plate, a total of 3,000 cells were implanted. 10 ul of CCK-8 reagent was added to the culture medium at 0, 24, 48, and 72 h after transfection. Then, the cells were incubated at 37°C for 1 h, and the absorbance was measured at 450 nm with a microplate reader.
Wound healing assay
Eighty percent fusion was achieved after transfected CAL27 cells were injected onto a 12-well plate. With the tip of a 10 ul pipette, single-cell layers were scraped. After three PBS washes to remove cell debris, fresh media containing serum was added. Three high magnification fields were taken at 0 h and 24 h after scratching to obtain typical images of cell migration. Using ImageJ, the scratch width was estimated.
Transwell migration and invasion assays
In the migration assay, transfected CAL27 cells were inoculated in the upper chamber of the Transwell system (BD Biosciences, San Jose, CA, United States), and the lower chamber was filled with 500 μl of medium containing 10% FBS. 24 h later, the cells remaining on the surface of the filter membrane were gently wiped off with a cotton swab, and the cells passing through the membrane were fixed with methanol and then stained with crystal violet solution. Under an inverted microscope, three randomly selected fields of view (including the center and periphery of the membrane) were used to count the number of cells. Matrigel (BD Biosciences, San Jose, CA, United States) was used to coat the filters of the Transwell system in the invasion assay, and the other steps were the same as for the migration assay.
Statistical assessments
Each experiment was repeated thrice, independently. Data are presented as the mean ± SD (standard deviation). For data analysis, the two-tailed Student’s t-test was applied. p < 0.05 was deemed a statistically significant difference.
Results
CircRNA profiles differentially expressed in OSCC
We discovered many circRNA in the neighboring normal tissue and three pairs of OSCC samples. These samples revealed a total number of targets of 3,738 circRNAs, including 2,421 known circRNAs and 1,317 new circRNAs. The lengths of candidate circRNAs were mostly <2,000 nucleotides (nt) (Figure 1A). The majority of circRNA is transcribed from exons that code for proteins. Some are intragenic and antisense and come from introns (Figure 1B). Candidate circRNAs are found on all chromosomes but primarily concentrate on the first and second (Figure 1C). CircRNAs that were differentially expressed with a statistical significance among the two groups were identified with |log2FoldChange| > 1 and p < 0.05. 11 circRNAs were significantly differentially expressed and visualized by a clustered heatmap (Figure 1D). In these samples, ten molecules of circRNA were up-regulated, and one was down-regulated (Table 2). We visualized the structure of circRNAs according to the CSCD database, and the results showed that all 11 circRNAs have miRNA response elements (MREs) (Supplementary Figure S1).
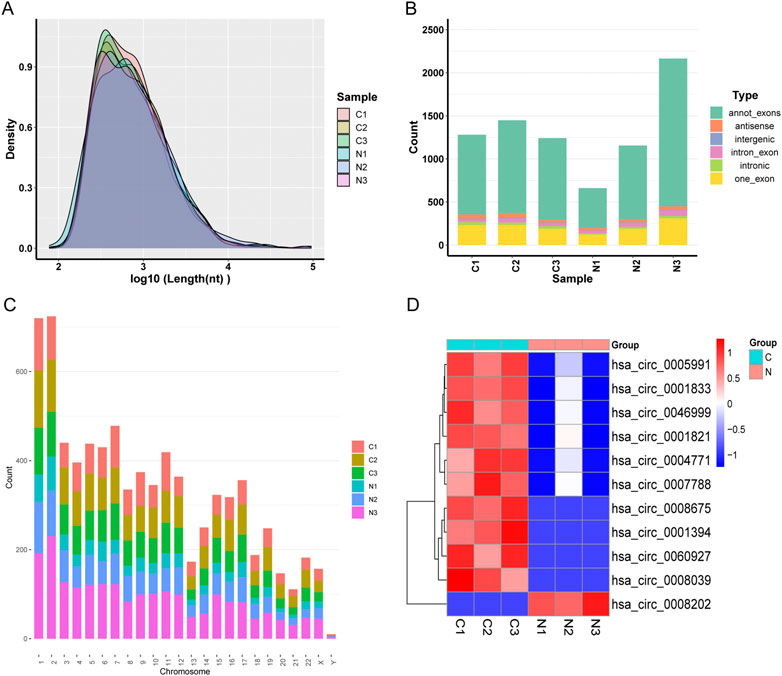
FIGURE 1. (A) Distribution of the lengths of the detected circRNAs. The length of the identified circRNAs is shown on the x-axis, and the abundance of circRNAs sorted by length is shown on the y-axis. (B) The circRNA category is depicted as a bar diagram. (C) In human chromosomes, the distribution of differently expressed circRNAs. (D) Analysis of all target circRNAs using a heat map and hierarchical clustering. High relative expression is shown by the red strip, whereas low relative expression is represented by the blue strip.
Differentially expressed circRNAs validation by qRT-PCR
We conducted qRT-PCR in 20 sample pairs that included the analysis of tissues for high-throughput sequencing. The results showed that five circRNA molecules were significantly upregulated, and one circRNA molecule was significantly downregulated in tumor tissues compared to normal tissues (Figure 2A). Figure 2B shows the validation results of the above six circRNA molecules in OSCC cell lines.
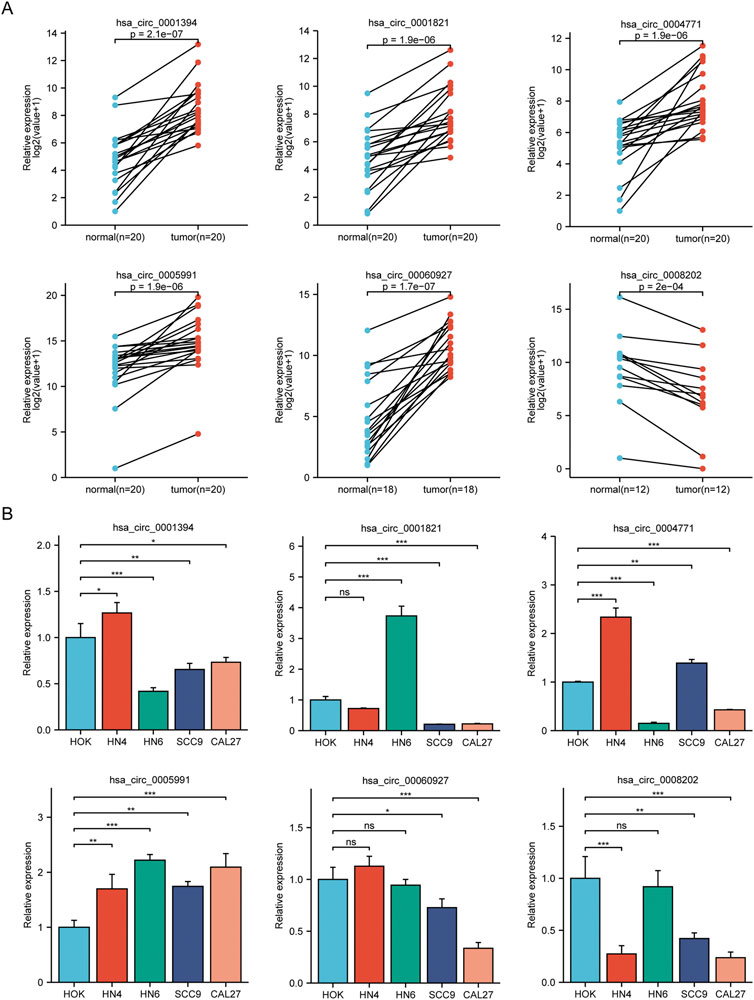
FIGURE 2. Analysis of circRNAs in cell lines and OSCC tissues. (A) Results of qRT-PCR in clinical specimens for six circRNAs. Five circRNA molecules were significantly upregulated, and one circRNA molecule was significantly downregulated in tumor tissues compared to normal tissues. (B) The expression of six circRNAs in OSCC cell lines (HN4, HN6, SCC9, CAL27) compared with HOK. *p < 0.05, **p < 0.01, ***p < 0.001. NS, no significance.
Prediction of the circRNA–miRNA–mRNA interaction network
To learn more about the functions of mRNAs and circRNAs in OSCC incidence, we built a ceRNA network. The ceRNA network will only include genes that match the following criteria: 1) All genes should be expressed differentially; 2) circRNAs and mRNAs should have an association with miRNAs through binding at the same time; and 3) RNAs (circRNAs, mRNAs) and miRNAs must be negatively regulated. We finally identified four circRNAs (3 upregulated and one downregulated), six overlapping miRNAs (2 upregulated and four downregulated), and 123 overlapping mRNAs (61 upregulated and 62 downregulated) to construct a ceRNA network (Figure 3A). Multiple circRNAs can operate as ceRNAs, capturing downstream miRNAs and influencing phenotypes through mRNA regulation. In addition, we performed a differential analysis of the nodes in the network and drew a heat map based on the expression data in the TCGA database. The results showed significant differences between the node molecules in cancer and paracancerous tissues (Figures 3B,C). Then, Kaplan-Meier survival analysis was used to validate further the association between genes involved in the ceRNA network and OSCC prognosis. The results showed that a total of 65 genes were significantly associated with overall survival in OSCC (p < 0.05, Supplementary Table S2).
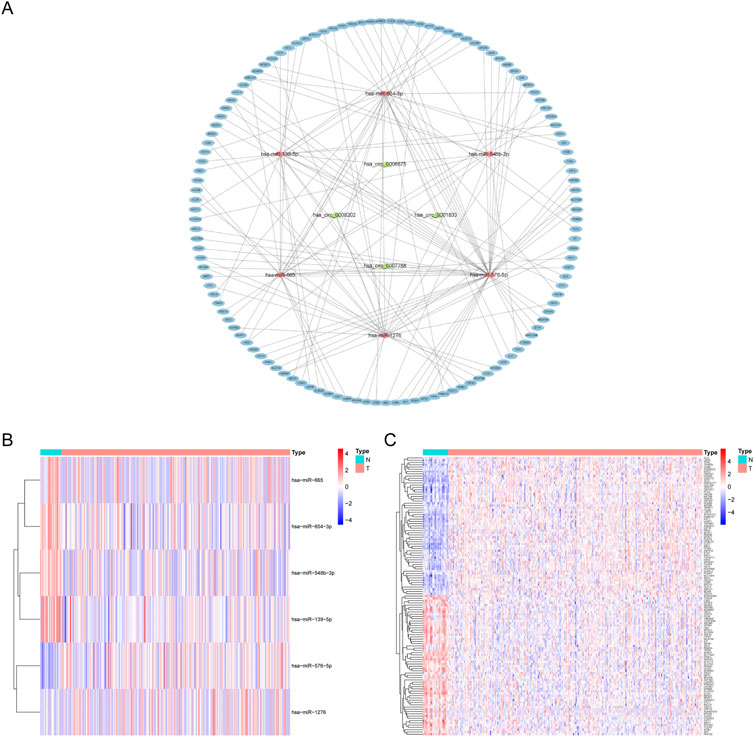
FIGURE 3. CeRNA network construction and node differential expression. (A) The ellipse represents 123 mRNAs, the diamond six microRNAs, the triangle four circular RNAs. (B,C) The differential expression of miRNAs and mRNAs in the ceRNA network in OSCC tissues and adjacent tissues in the TCGA database. N, adjacent non-tumor tissues; T, tumor tissues.
Analysis of kyoto encyclopedia of genes and genomes pathway and annotation of gene ontology function
We conducted KEGG and GO functional enrichment analysis on mRNAs (n = 123) in the ceRNA network (Supplementary Table S3). The top five enriched CC (Cellular Component), BP (Biological Process), MF (Molecular Function) terms, and KEGG pathways are presented in Figures 4A,B. They are enriched mainly in positive regulation of cell adhesion, positive regulation of SMAD protein signal transduction (BPs), the cell-cell junction (CCs), and transmembrane receptor protein kinase activity (MFs). Analysis of the KEGG pathway shows enrichment in the PI3K-Akt signaling pathway, mTOR signaling pathway, cellular senescence, and proteoglycans in cancer. These findings suggest that the differentially expressed genes are linked to tumor signaling pathways and OSCC progression.
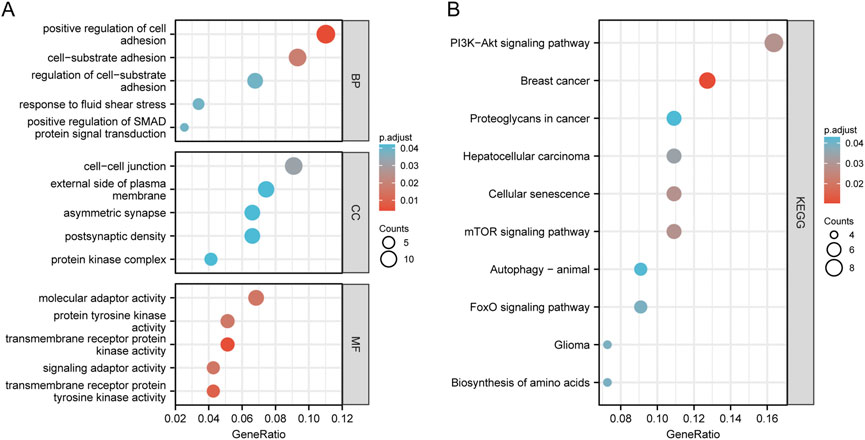
FIGURE 4. Enrichment analysis of function. Bubble plot of target gene GO enrichment analysis: (A) BP, CC, and MF in the ceRNA network; (B) The bubble chart of the signal pathway focused by the KEGG enrichment analysis of genes in the ceRNA network.
Construction and evaluation of risk assessment model
We found that seven DEmRNAs substantially impacted patients’ overall survival by applying univariate Cox regression (p < 0.05, Supplementary Table S4), suggesting that mRNAs in the ceRNA prognostic subnet might affect the survival and prognosis of OSCC patients. Following that, LASSO Cox regression analyses were utilized to establish a risk model for the above seven genes, and the LASSO regression analysis results kept the above seven genes (Supplementary Figures S2A,B). Finally, a multivariate Cox regression analysis was performed using the stepwise regression approach, and seven DEmRNAs were screened out to establish an OSCC prognostic model (Supplementary Figures S2C,D).
To verify the model’s accuracy, ROC curves (1-, 2-, and 3-year) were drawn, and could successfully prognosticate OSCC patients because all values of areas under curve (AUC) were more than 0.65 (Figure 5A). Next, with the help of multi-metric ROC curves, we plotted the ROC curves of the risk model together with the ROC curves of clinical characteristics such as age, sex, grade, and stage in the same graph for comparison. The results showed that the AUC values of the risk model were significantly better than the clinical parameters, indicating the model’s high performance (Figure 5B). We also used the AIC value for the identification of the maximum inflection point as the cutoff point for the ROC curve for 1-year (Figure 5C).
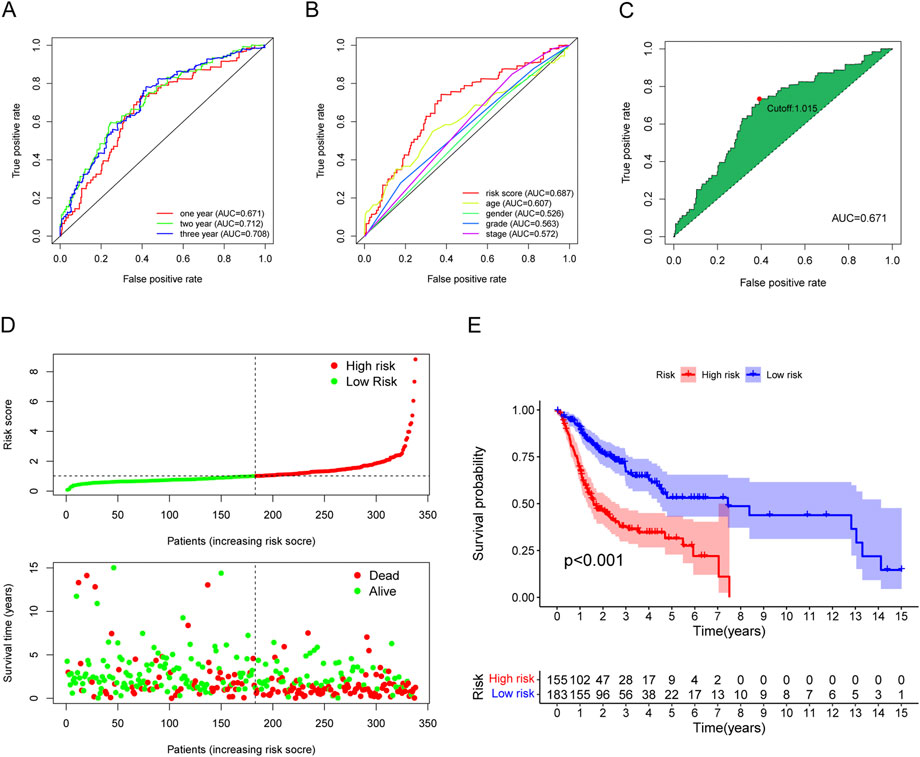
FIGURE 5. Evaluation of the risk assessment model for prognosis prediction. (A) The AUCs of the 1-, 2-, and 3-year ROC curves. (B) The 1-year ROC curve of the risk model and other clinicopathological characteristics. (C) The cut-off risk score identified by Youden Index. (D) The distribution of risk score and survival time and status. (E) The overall survival of the low-risk groups was higher based on K-M analysis.
Model for clinical evaluation through risk assessment
Based on the earlier determined cut-off points, 155 cases were classified as a high-risk group, and 183 were classified as a low-risk group. The risk score distribution for each OSCC case is presented in Figure 5D; these data indicate better clinical outcomes for low-risk group patients than those in the high-risk group. Patients with OSCC in the high-risk group exhibited a significant decrease in survival than low-risk OSCC patients, according to a Kaplan-Meier analysis and associated survival curve (p < 0.001; Figure 5E). In addition, the analysis showed that nearly all patients with high-risk scores lived under 8 years, while in the low-risk group, about 50% were still alive. We used chi-square tests to assess whether the risk model is associated with the clinicopathological traits of OSCC patients. Bar graphs show overall results, with tumor grade and the stage being exceptionally closely related to risk (Figure 6A). The proportion of each clinicopathological feature in the high- or low-risk group is shown in Supplementary Figure S3. In addition, we analyzed the differences in risk scores between groups stratified by different clinicopathological factors. As shown in Figures 6B–D, statistically significant high-risk scores were more common in patients with higher tumor grades and more advanced clinical stages. However, there were no differences in risk scores between patients by gender (Figure 6E). Finally, we performed univariate and multivariate cox analyses and found that the risk model could be used as an independent prognostic predictor (Figures 6F,G). In conclusion, a risk assessment model based on DEmRNAs can be used as a reliable predictor of survival outcomes and tumor aggressiveness in OSCC.
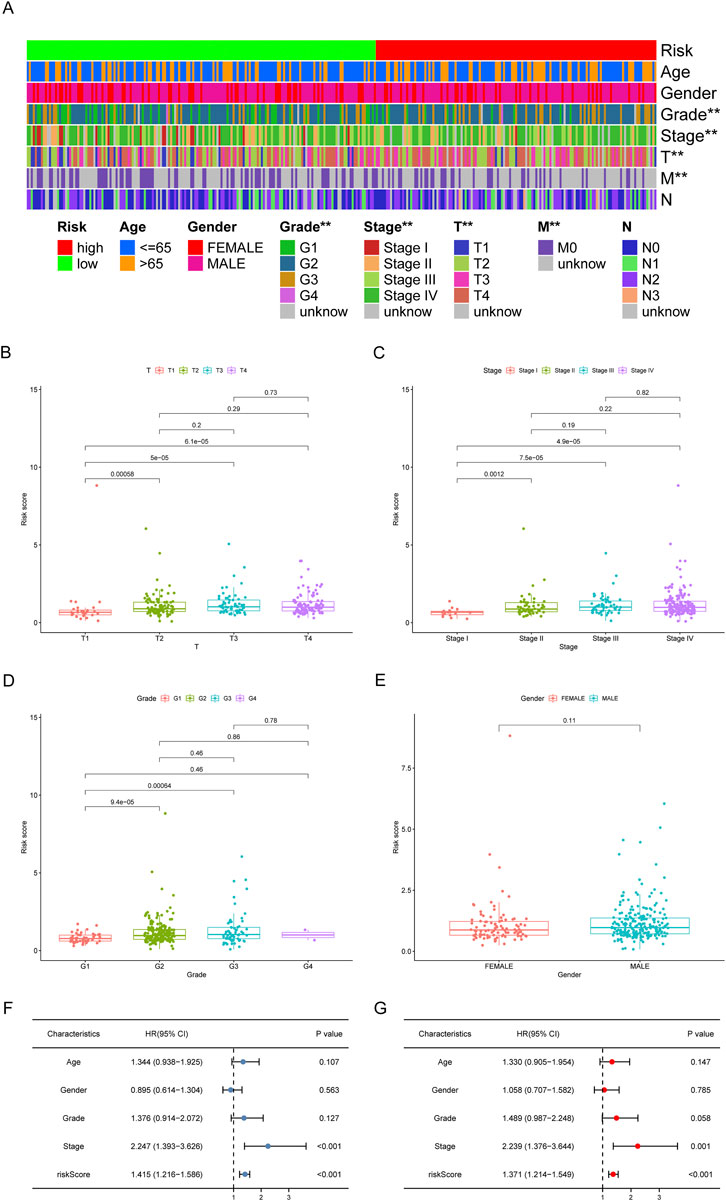
FIGURE 6. Clinical evaluation of the risk assessment model. (A) The strip chart and the scatter diagram demonstrated that T stage (B), clinical stage (C), tumor grade (D), and gender (E) were significantly correlated with the risk score. *p < 0.05, **p < 0.01. (F,G) The risk model could be used as an independent prognostic predictor by the univariate and multivariate Cox regression analysis.
Correlation between tumor-infiltrating immune cells or immunosuppressive molecules and the risk model
We concentrated on immune cell infiltration and looked at the model’s connection with the tumor immunological microenvironment. According to Spearman correlation analysis, a high-risk group in the model was correlated positively with TICs, including common lymphoid progenitors, macrophages, and NK cells. In contrast, CD4+ T cells, CD8+ T cells, neutrophils, mast cells, and B cells had a negative correlation (Figure 7A). In addition, compared to the low expression group, the low-risk group was significantly higher in the stromal score, immune score, and ESTIMATE score (Figures 7B–D). We further investigated whether the model was associated with ICI and found that the high-risk group in the model was negatively associated with various immune checkpoint molecules, such as BTLA, CD27, CD244, and CTLA4 (Figures 8A–D).
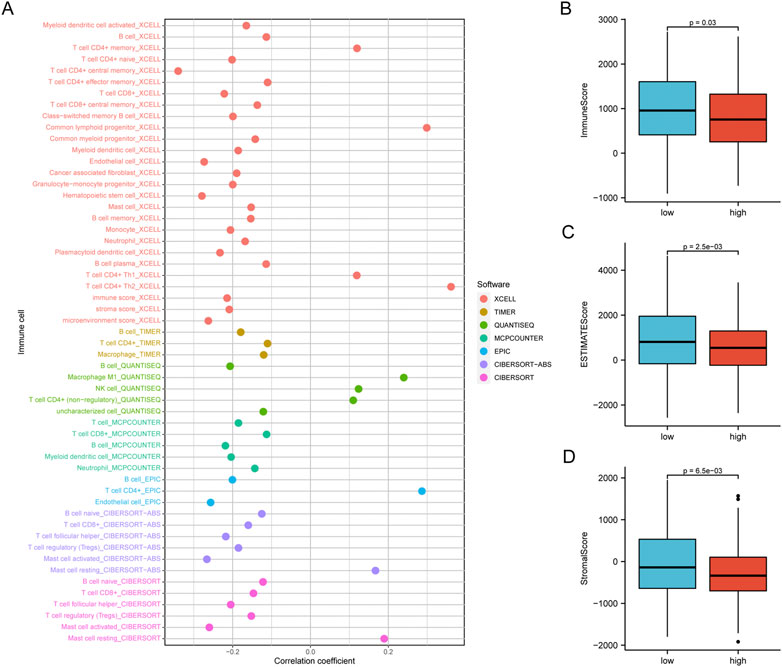
FIGURE 7. The Risk assessment model was used to estimate tumor-infiltrating cells. (A) According to Spearman correlation analysis, patients in the high-risk group were more positively associated with tumor-infiltrating immune cells like ommon lymphoid progenitor, macrophages, NK cells, while they were negatively associated with CD4+ T cells, B cells, CD8+ T cells, mast cells, and neutrophils. (B) The low-risk group has higher TME scores.
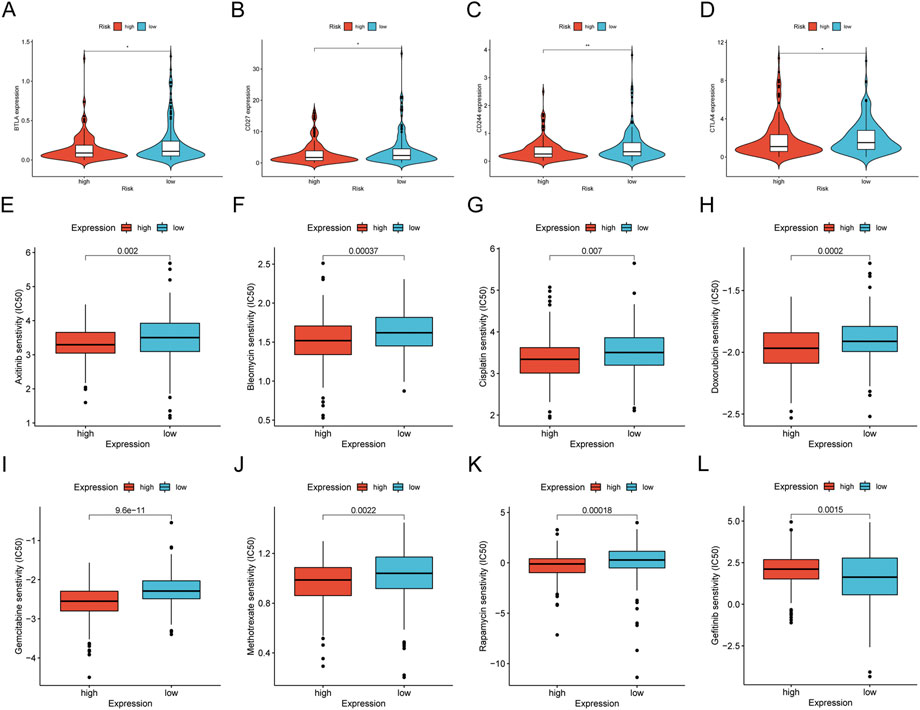
FIGURE 8. Differences of checkpoint-related gene expression and chemotherapeutic sensitivity in high- and low-risk groups. (A–D) The high-risk group in the model was negatively associated with BTLA, CD27, CD244, and CTLA4. The high-risk group in the model was related to lower IC50 of chemotherapeutics of axitinib (E), bleomyci (F), cisplatin (G), doxorubicin (H), gemcitabine (I), methotrexate (J), rapamycin (K), and the low-risk group was related to lower IC50 of chemotherapeutics of gefitinib (L).
Analysis of the correlation between chemosensitivity and the risk mode
We investigated common medication sensitivities (expressed as IC50s) in individuals with low and high-risk ratings to see if the efficacy of several regularly used chemotherapeutic medicines is associated with risk. The patients with high-risk had a lower IC_(50) for axitinib (p = 0.002; Figure 8E), bleomycin (p = 0.00037; Figure 8F), cisplatin (p = 0.007; Figure 8G), doxorubicin (p = 0.0002; Figure 8H), gemcitabine (p < 0.0001; Figure 8I), methotrexate (p = 0.0022; Figure 8J), rapamycin (p = 0.00018; Figure 8K), higher IC_(50) for gefitinib (p = 0.0015; Figure 8L). Therefore, this model can potentially predict sensitivity to chemotherapeutic drugs.
Hsa_circ_0005991 promotes HNSCC cells proliferation, migration, and invasion in vitro
In HOK and five HNSCC cell lines, the expression of hsa_circ_0005991 was identified by qRT-PCR, and the results revealed that four HNSCC cells expressed hsa_circ_0005991 at a higher level than HOK cells (Figure 9A). Ultimately, the CAL27 cell lines were selected by us to represent the knockdown cells of hsa_circ_0005991. In CAL27 cells treated with siRNA fragments, according to qRT-PCR data, hsa_circ_0005991 expression was dramatically down-regulated. The si-hsa_circ_0005991-3 was chosen for further investigation among the three siRNAs because it showed the best silencing effectiveness in CAL27 cells (Figure 9B). The downregulation of hsa_circ_0005991 inhibited the proliferative activity of HNSCC cells according to the CCK-8 assay (Figure 9C). Then, the influences of hsa_circ_0005991 on HNSCC cell invasion and migration were examined by wound healing and transwell assays. The results showed that downregulation of the hsa_circ_0005991 gene inhibited CAL27 cells invasion and migration ability (Figures 9D,E). These experiments proved that in HNSCC cells, hsa_circ_0005991 could promote proliferation, migration, and invasion.
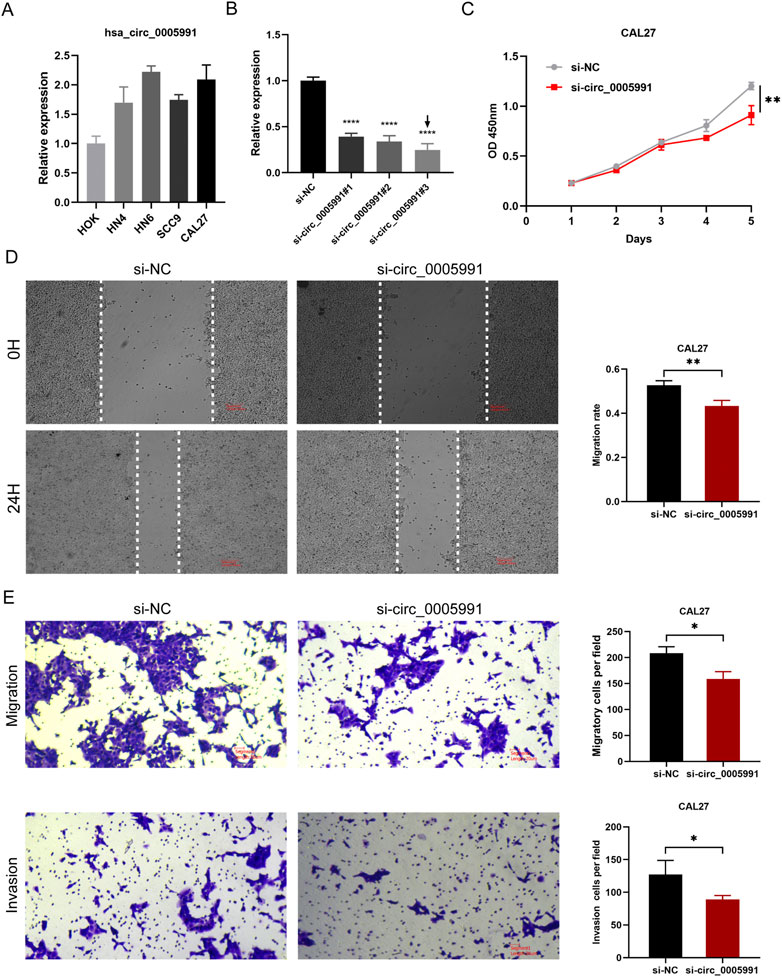
FIGURE 9. Hsa_circ_0005991 promotes the proliferation, migration and invasion of HNSCC cells in vitro. (A) The qRT-PCR results revealed that HOK cells expressed hsa_circ_0005991 at a lower level than five HNSCC cells. (B) qRT-PCR analysis of hsa_circ_0005991 expression in CAL27 cells treated with siRNAs. (C) The proliferation ability of CAL27 cells transfected with NC or si-hsa_circ_0005991 was examined by CCK-8 assay. (D,E) The migration and invasion ability of the cells were detected by wound healing and transwell assays. Data were showed as mean ± SD,*p < 0.05, **p < 0.01, ***p < 0.001, ****p < 0.0001.
Discussion
Researchers in different countries have made significant progress in OSCC research in recent years, especially in developing molecularly targeted drugs and antibody-drug conjugates, which have greatly benefited OSCC patients (Nagaya et al., 2017; Ketabat et al., 2019; Zhang et al., 2020). Current treatments for OSCC include surgery, chemotherapy, molecularly targeted therapy, and radiation therapy. Although there are various treatment methods, most OSCC patients have entered the advanced stage, and the overall treatment effect is not ideal due to factors such as drug resistance, distant metastasis, and poor typing. The pathogenesis and progression of cancer are closely related to genetic mutations and genetic diseases. Studies have shown that dysregulation of circular RNA expression plays a vital role in the pathogenesis and progression of many tumors. CircRNAs are suggested to express in a tissue-specific and developmental stage-specific manner, which makes them potential biomarkers of specific diseases (Wang et al., 2021). CircRNA-associated ceRNA regulatory networks play critical roles in the pathogenesis and progression of OSCC, bladder cancer, colorectal cancer, and other cancers (Song and Fu, 2019; Wu et al., 2019; Liu et al., 2020). Importantly, mRNAs, miRNAs, and circRNAs in ceRNA networks can serve as potential targets for OSCC therapy and molecular targets for assessing prognosis. However, little is known in the OSCC field about the combined analysis of circRNA-based ceRNA networks and the immune microenvironment. Therefore, we constructed a circRNA-miRNA-mRNA network and analyzed target genes and prognostic, TIC, and immune checkpoints to identify novel molecular mechanisms and prognostic biomarkers of OSCC occurrence. We also aimed to explore new targets that could mediate specific tumor immunotherapy.
We found abundant expression of circRNAs in three pairs of samples, including 2421 existing circRNA molecules and 1,317 new circRNA molecules. Most of the circRNAs were derived from exons. Exonic circRNAs are generated by a process called reverse slicing, which is a disorderly arrangement of exons (Zhang et al., 2013). This study identified ten up-regulated and one down-regulated circRNA molecule, and six selected circRNAs were consistent with RNA-seq data after validation using qRT-PCR.
Hsa_circ_0004771, hsa_circ_0005991, and hsa_circ_0060927 are spliced by nuclear receptor interacting protein 1 (NRIP1), amyloid beta precursor protein binding family B member 2 (APBB2), and cytochrome P450 family 24 subfamily A member 1 (CYP24A1), respectively, which play essential roles in tumor proliferation, migration, and apoptosis. NRIP1, a coregulator of several nuclear receptors and transcription factors, can act as a co-activator or corepressor and is upregulated in a variety of tumor types, including gastric cancer (Liang and Li, 2020), gastric adenocarcinoma (Fang and Lu., 2020), esophageal squamous cell carcinoma (Ni et al., 2018), and breast cancer (Binato et al., 2021). APBB2 is an articulated protein characterized for its function in amyloid precursor protein processing (Tanahashi and Tabira, 2002). APBB2 plays a dual regulatory role in bladder cancer, mediating the cell cycle through the CDK6 and MET pathways (Li et al., 2019). CYP24A1 is a mitochondrial enzyme responsible for the inactivation of vitamin D. Fazeli et al. (2020) demonstrated for the first time that hsa_circ_0060927 is ectopically expressed in uterine smooth muscle tumors compared to adjacent tissues.
In addition, a ceRNA network consisting of four circRNAs, six miRNAs, and 123 mRNAs was constructed based on DEcircRNA. The biological functions of DEmRNA were analyzed by GO functional annotation and KEGG pathway enrichment. The results of GO analysis revealed that cell adhesion participates in the functions of these DEmRNAs, and the role of cell adhesion in the progression of OSCC has been extensively studied (de Campos et al., 2017; Kita et al., 2017; Aseervatham and Ogbureke., 2020). KEGG analysis showed that DEmRNAs are widely involved in the PI3K-AKT signaling pathway, mTOR signaling pathway, and Cellular senescence. These pathways play a crucial role in the development of OSCC. The PI3K-AKT-mTOR signaling pathway is associated with several cellular functions, controlling protein synthesis, cell growth, apoptosis, proliferation, and angiogenesis, and may regulate the progression of OSCC (Martins et al., 2016). Wu et al. (2022) found that HPRT1 could improve cisplatin (CDDP) resistance in OSCC patients by promoting the MMP1/PI3K/Akt axis. Liu et al. (2022) showed that LHPP promotes apoptosis in OSCC by decreasing the transcriptional activity of p-PI3K and p-Akt.
A prognostic risk model with seven genes was developed by integrating LASSO regression and Cox regression analysis. Kaplan-Meier curve analysis showed that our prognostic model could accurately distinguish between high- and low-risk populations. Furthermore, the AUC of the ROC plots for 1-year, 2-year, and 3-year overall survival were 0.671, 0.712, and 0.708, respectively. The results confirmed the excellent predictive value of our risk profile. Of these seven genes, six are potential risk genes, and one is a potential protective gene. Six genes are involved in the development and progression of various cancers. LIM and SH3 protein 1 (LASP1) is a member of the LIM family of proteins, initially identified from a cDNA library of human breast cancer tissue (Tomasetto et al., 1995). LASP1 expression was reported to be increased in OSCC patients and significantly correlated with primary tumor size. Further studies have shown that LASP1 promotes OSCC cell proliferation by accelerating cell cycle progression (Shimizu et al., 2013). DEP domain containing 1 (DEPDC1) is a highly conserved protein in many species, from Cryptobacterium hidradenum to mammals (Kanehira et al., 2007). Guo et al. (2020) showed that DEPDC1 exerts its oncogenic activity by suppressing CYP27B1 protein expression, while NNK promotes DEPDC1 upregulation by stimulating DNMT1 expression in OSCC. Similarly, DNA damage-inducible transcript 4 (DDIT4) and glutamic-oxaloacetic transaminase 1 (GOT1) as oncogenes are associated with poor prognosis in OSCC (Busso-Lopes et al., 2021; Han et al., 2021). Basal cell adhesion molecule (BCAM), also known as Lutheran, is widely expressed in various tissues and involves many biological processes, such as cell adhesion, migration, and invasion (De Grandis et al., 2013; Bartolini et al., 2016). Emerging research suggests that BCAM plays a vital role in tumor progression, including skin tumors, hepatocellular carcinoma, colorectal cancer, and bladder cancer (Bartolini et al., 2016; Chang et al., 2017; Jin et al., 2020). Choi et al. (2020) identified a novel tumor suppressor, KLHL14, a subunit of ubiquitin ligase, associated with the endoplasmic reticulum-associated protein degradation (ERAD) machinery and is recurrently mutated in mature B cell malignancies. However, the roles of KLHL14, BCAM, and HAUS augmin like complex subunit 6 (HAUS6) in OSCC, are unknown.
Since a single gene may predict OS instability, a signature integrating the efficacy of seven immune-related genes would show strong predictive power. To establish a relatively accurate prognostic model in OSCC patients, this study proposes a novel prognostic line chart, including immune-related risk scores and clinicopathological features, which showed good predictive power. To explore the relationship between risk scores and tumor-infiltrating immune cells, we used seven commonly accepted methods to estimate immune infiltrating cells, including XCELL (Aran et al., 2017; Aran, 2020), TIMER (Li et al., 2017; Li et al., 2020), QUANTISEQ (Finotello et al., 2019; Plattner et al., 2020), MCPCOUNTER (Dienstmann et al., 2019), EPIC (Racle et al., 2017), CIBERSORT ABS (Tamminga et al., 2020), and CIBERSORT (Chen et al., 2018; Zhang et al., 2020). Analyzing these results, we found that the high-risk population in the model was significantly and negatively associated with immune infiltration of CD8+ T cells, neutrophils, mast cells, and B cells. The role of B cells has been controversial: some studies have shown that B cell infiltration in tumors is associated with poor prognosis, while others have shown the opposite (Tsou et al., 2016; Sarvaria et al., 2017). CD8+ T cells are the major lymphocyte subpopulation that kills cancer cells with major histocompatibility class I molecules (Shen and Ren., 2018). The frequency of CD8+ T cells was positively correlated with survival in patients with lung cancer, melanoma, and breast cancer (Reiser and Bamerjee., 2016; van der Leun et al., 2020). In addition, infiltration of CD8+ T cells in TME was associated with improved response in patients with ICIS-treated cancers. Wong et al. (2019) found that melanoma patients with high CD8+ T cell counts survived longer on anti-PD-1 therapy. Theoretically, neutrophils may be a potent antitumor effector cell because neutrophil granules contain various antimicrobial and cytotoxic compounds that can destroy malignant cells (Ocana et al., 2017). However, many studies have shown that tumor-associated neutrophils may promote tumor progression. N2 Polarized neutrophils morphologically resemble granulocytes or polymorphonuclear myeloid-derived suppressor cells and thus may exert tumor suppressive effects (Rakic et al., 2018). Although the signature is associated with checkpoint-associated biomarkers such as BTLA, CD27, CD244, and CTLA4, we believe that the specific mechanisms and biomarkers are yet to be confirmed and validated due to differences in different immune cells and immune-associated phenotypes. To further evaluate the value of risk models for clinical application in the treatment of OSCC, we calculated the IC50 of several commonly used antitumor drugs and compared the differences in drug sensitivity between patients in the high-risk and low-risk subgroups. We found that high-risk OSCC patients had higher sensitivity (lower IC50) to antitumor drugs such as cisplatin, gemcitabine, and rapamycin. The relationship between the risk model and drug sensitivity can be used to guide the selection and administration of clinical antitumor drugs and needs to be further investigated. Because our conclusions are based on computational predictions, molecular biology tests are required to ascertain the role of CircRNA in OSCC formation. Tumor metastasis is considered a significant factor in OSCC based on biological processes and potential mechanisms of occurrence. As a result, we carried out pertinent research and discovered that in vitro downregulation of hsa_circ_0005991 prevented CAL27 cells from migrating and invading.
In this study, first, we performed high-throughput sequencing and qRT-PCR validation based on collected clinical tissue samples, which means this study has better clinical relevance. Second, we are the first to construct a circRNA-based OSCC-related prognostic model, which shows a good prognostic predictive ability for OSCC patients. Third, the model we constructed can also guide clinicians in choosing appropriate chemotherapy and immunotherapy approaches. However, we also recognize some shortcomings and limitations of this study. First, a circRNA-miRNA-mRNA network was established by bioinformatics analysis. Our study only showed at the in vitro level that circRNA can promote the malignant progression of HNSCC cells. Therefore, future studies should validate the current findings in animal models. CircRNAs are found evolutionally conserved in different species, which means some circRNA biomarkers identified in murine modes hold the potential to be translated to clinical application for human beings (Ma et al., 2020). Second, the original dataset for the initial analysis was relatively insufficient, as it was only downloaded from TCGA. While we used various methods to validate the accuracy and validity of our predictive model, more external cohorts are needed in the future to confirm it. Finally, the clinical utility of our risk model, such as its relationship to antitumor drug susceptibility, has not been clinically validated. Therefore, we plan to collect additional clinical samples for RNA-seq to validate our risk model in future experiments and establish more robust clinical links for this new signature.
Conclusion
In this study, we found that circRNAs were significantly expressed in OSCC compared to normal tissues. We identified four key circRNAs and constructed a circRNA-related ceRNA network associated with OSCC, which will help to elucidate the molecular mechanism of OSCC occurrence and development. In addition, we established an OSCC-related risk model, which may improve OSCC patient survival prediction and reflect immune status, and provide a new reference for personalized treatment.
Data availability statement
The raw sequence data obtained have been deposited at the NCBI in the Sequence Read Archive (SRA) database under bioproject PRJNA904235.
Ethics statement
The studies involving human participants were reviewed and approved by the Medical Ethics Committee of the Affiliated Stomatological Hospital of Anhui Medical University. The patients/participants provided their written informed consent to participate in this study.
Author contributions
YH and DY worked together to design the study, analyze the data, and write the paper. YH carried out the research and wrote the paper. The experimental validation was carried out by DY. YL and JX participated with study design and manuscript revision. The project was overseen by LW and YW, who also revised the paper. The final manuscript was read and approved by all of the authors.
Funding
This study was supported by Anhui Medical University School of Stomatology Discipline Construction Follow-up Project (2020kqsy02) and Scientific research projects in Anhui universities (YJS20210291).
Conflict of interest
The authors declare that the research was conducted in the absence of any commercial or financial relationships that could be construed as a potential conflict of interest.
Publisher’s note
All claims expressed in this article are solely those of the authors and do not necessarily represent those of their affiliated organizations, or those of the publisher, the editors and the reviewers. Any product that may be evaluated in this article, or claim that may be made by its manufacturer, is not guaranteed or endorsed by the publisher.
Supplementary material
The Supplementary Material for this article can be found online at: https://www.frontiersin.org/articles/10.3389/fphar.2022.949713/full#supplementary-material
References
Alsahafi, E., Begg, K., Amelio, I., Raulf, N., Lucarelli, P., Sauter, T., et al. (2019). Clinical update on head and neck cancer: Molecular biology and ongoing challenges. Cell Death Dis. 10 (8), 540. doi:10.1038/s41419-019-1769-9
Aran, D. (2020). Cell-type enrichment analysis of bulk transcriptomes using xCell. Methods Mol. Biol. 2120, 263–276. doi:10.1007/978-1-0716-0327-7_19
Aran, D., Hu, Z., and Butte, A. J. (2017). xCell: digitally portraying the tissue cellular heterogeneity landscape. Genome Biol. 18 (1), 220. doi:10.1186/s13059-017-1349-1
Aseervatham, J., and Ogbureke, K. (2020). Effects of DSPP and MMP20 silencing on adhesion, metastasis, angiogenesis, and epithelial-mesenchymal transition proteins in oral squamous cell carcinoma cells. Int. J. Mol. Sci. 21 (13), E4734. doi:10.3390/ijms21134734
Bartolini, A., Cardaci, S., Lamba, S., Oddo, D., Marchio, C., Cassoni, P., et al. (2016). BCAM and LAMA5 mediate the recognition between tumor cells and the endothelium in the metastatic spreading of KRAS-mutant colorectal cancer. Clin. Cancer Res. 22 (19), 4923–4933. doi:10.1158/1078-0432.CCR-15-2664
Binato, R., Correa, S., Panis, C., Ferreira, G., Petrone, I., Da, C. I., et al. (2021). NRIP1 is activated by C-JUN/C-FOS and activates the expression of PGR, ESR1 and CCND1 in luminal A breast cancer. Sci. Rep. 11 (1), 21159. doi:10.1038/s41598-021-00291-w
Busso-Lopes, A. F., Carnielli, C. M., Winck, F. V., Patroni, F., Oliveira, A. K., Granato, D. C., et al. (2021). A reductionist approach using primary and metastatic cell-derived extracellular vesicles reveals hub proteins associated with oral cancer prognosis. Mol. Cell. Proteomics. 20, 100118. doi:10.1016/j.mcpro.2021.100118
Chang, H. Y., Chang, H. M., Wu, T. J., Chaing, C. Y., Tzai, T. S., Cheng, H. L., et al. (2017). The role of Lutheran/basal cell adhesion molecule in human bladder carcinogenesis. J. Biomed. Sci. 24 (1), 61. doi:10.1186/s12929-017-0360-x
Chang, J., Tseng, C. H., Lu, P. H., and Wang, Y. P. (2021). Contemporary molecular analyses of malignant tumors for precision treatment and the implication in oral squamous cell carcinoma. J. Pers. Med. 12 (1), 12. doi:10.3390/jpm12010012
Chen, B., Khodadoust, M. S., Liu, C. L., Newman, A. M., and Alizadeh, A. A. (2018). Profiling tumor infiltrating immune cells with CIBERSORT. Methods Mol. Biol. 1711, 243–259. doi:10.1007/978-1-4939-7493-1_12
Chen, Y., Li, C., Tan, C., and Liu, X. (2016). Circular RNAs: A new frontier in the study of human diseases. J. Med. Genet. 53 (6), 359–365. doi:10.1136/jmedgenet-2016-103758
Choi, J., Phelan, J. D., Wright, G. W., Haupl, B., Huang, D. W., Shaffer, A. R., et al. (2020). Regulation of B cell receptor-dependent NF-κB signaling by the tumor suppressor KLHL14. Proc. Natl. Acad. Sci. U. S. A. 117 (11), 6092–6102. doi:10.1073/pnas.1921187117
Conway, D. I., Purkayastha, M., and Chestnutt, I. G. (2018). The changing epidemiology of oral cancer: Definitions, trends, and risk factors. Br. Dent. J. 225 (9), 867–873. doi:10.1038/sj.bdj.2018.922
Cui, L., Huang, C., and Zhou, D. (2021). Overexpression of circCDR1as drives oral squamous cell carcinoma progression. Oral Dis. doi:10.1111/odi.14085
de Campos, P. S., Matte, B. F., Diel, L. F., Jesus, L. H., Bernardi, L., Alves, A. M., et al. (2017). Low doses of curcuma longa modulates cell migration and cell-cell adhesion. Phytother. Res. 31 (9), 1433–1440. doi:10.1002/ptr.5872
De Grandis, M., Cambot, M., Wautier, M. P., Cassinat, B., Chomienne, C., Colin, Y., et al. (2013). JAK2V617F activates Lu/BCAM-mediated red cell adhesion in polycythemia vera through an EpoR-independent Rap1/Akt pathway. Blood 121 (4), 658–665. doi:10.1182/blood-2012-07-440487
Dienstmann, R., Villacampa, G., Sveen, A., Mason, M. J., Niedzwiecki, D., Nesbakken, A., et al. (2019). Relative contribution of clinicopathological variables, genomic markers, transcriptomic subtyping and microenvironment features for outcome prediction in stage II/III colorectal cancer. Ann. Oncol. 30 (10), 1622–1629. doi:10.1093/annonc/mdz287
Fang, D., and Lu, G. (2020). Expression and role of nuclear receptor-interacting protein 1 (NRIP1) in stomach adenocarcinoma. Ann. Transl. Med. 8 (20), 1293. doi:10.21037/atm-20-6197
Fazeli, E., Piltan, S., Sadeghi, H., Gholami, M., Azizi-Tabesh, G., Yassaee, F., et al. (2020). Ectopic expression of CYP24A1 circular RNA hsa_circ_0060927 in uterine leiomyomas. J. Clin. Lab. Anal. 34 (4), e23114. doi:10.1002/jcla.23114
Finotello, F., Mayer, C., Plattner, C., Laschober, G., Rieder, D., Hackl, H., et al. (2019). Molecular and pharmacological modulators of the tumor immune contexture revealed by deconvolution of RNA-seq data. Genome Med. 11 (1), 34. doi:10.1186/s13073-019-0638-6
Gao, L., Yang, X., Yi, C., and Zhu, H. (2019). Adverse events of concurrent immune checkpoint inhibitors and antiangiogenic agents: A systematic review. Front. Pharmacol. 10, 1173. doi:10.3389/fphar.2019.01173
Gao, Y., Wang, J., Zheng, Y., Zhang, J., Chen, S., and Zhao, F. (2016). Comprehensive identification of internal structure and alternative splicing events in circular RNAs. Nat. Commun. 7, 12060. doi:10.1038/ncomms12060
Geng, Y., Jiang, J., and Wu, C. (2018). Function and clinical significance of circRNAs in solid tumors. J. Hematol. Oncol. 11 (1), 98. doi:10.1186/s13045-018-0643-z
Guo, J., Zhou, S., Huang, P., Xu, S., Zhang, G., He, H., et al. (2020). NNK-mediated upregulation of DEPDC1 stimulates the progression of oral squamous cell carcinoma by inhibiting CYP27B1 expression. Am. J. Cancer Res. 10 (6), 1745–1760.
Han, Y., Wang, X., Xia, K., and Su, T. (2021). A novel defined hypoxia-related gene signature to predict the prognosis of oral squamous cell carcinoma. Ann. Transl. Med. 9 (20), 1565. doi:10.21037/atm-21-4990
Hinshaw, D. C., and Shevde, L. A. (2019). The tumor microenvironment innately modulates cancer progression. Cancer Res. 79 (18), 4557–4566. doi:10.1158/0008-5472.CAN-18-3962
Jehn, P., Dittmann, J., Zimmerer, R., Stier, R., Jehn, M., Gellrich, N. C., et al. (2019). Survival rates according to tumour location in patients with surgically treated oral and oropharyngeal squamous cell carcinoma. Anticancer Res. 39 (5), 2527–2533. doi:10.21873/anticanres.13374
Jin, J., Xie, S., Sun, Q., Huang, Z., Chen, K., Guo, D., et al. (2020). Upregulation of BCAM and its sense lncRNA BAN are associated with gastric cancer metastasis and poor prognosis. Mol. Oncol. 14 (4), 829–845. doi:10.1002/1878-0261.12638
Kalogirou, E. M., Tosios, K. I., and Christopoulos, P. F. (2021). The role of macrophages in oral squamous cell carcinoma. Front. Oncol. 11, 611115. doi:10.3389/fonc.2021.611115
Kanehira, M., Harada, Y., Takata, R., Shuin, T., Miki, T., Fujioka, T., et al. (2007). Involvement of upregulation of DEPDC1 (DEP domain containing 1) in bladder carcinogenesis. Oncogene 26 (44), 6448–6455. doi:10.1038/sj.onc.1210466
Ketabat, F., Pundir, M., Mohabatpour, F., Lobanova, L., Koutsopoulos, S., Hadjiiski, L., et al. (2019). Controlled drug delivery systems for oral cancer treatment-current status and future perspectives. Pharmaceutics 11 (7), E302. doi:10.3390/pharmaceutics11070302
Kita, A., Kasamatsu, A., Nakashima, D., Endo-Sakamoto, Y., Ishida, S., Shimizu, T., et al. (2017). Activin B regulates adhesion, invasiveness, and migratory activities in oral cancer: A potential biomarker for metastasis. J. Cancer 8 (11), 2033–2041. doi:10.7150/jca.18714
Leemans, C. R., Braakhuis, B. J., and Brakenhoff, R. H. (2011). The molecular biology of head and neck cancer. Nat. Rev. Cancer 11 (1), 9–22. doi:10.1038/nrc2982
Li, J., Yang, J., Zhou, P., Le, Y., Zhou, C., Wang, S., et al. (2015). Circular RNAs in cancer: Novel insights into origins, properties, functions and implications. Am. J. Cancer Res. 5 (2), 472–480.
Li, J., Ying, Y., Xie, H., Jin, K., Yan, H., Wang, S., et al. (2019). Dual regulatory role of CCNA2 in modulating CDK6 and MET-mediated cell-cycle pathway and EMT progression is blocked by miR-381-3p in bladder cancer. Faseb J. 33 (1), 1374–1388. doi:10.1096/fj.201800667R
Li, T., Fan, J., Wang, B., Traugh, N., Chen, Q., Liu, J. S., et al. (2017). Timer: A web server for comprehensive analysis of tumor-infiltrating immune cells. Cancer Res. 77 (21), e108–e110. doi:10.1158/0008-5472.CAN-17-0307
Li, T., Fu, J., Zeng, Z., Cohen, D., Li, J., Chen, Q., et al. (2020). TIMER2.0 for analysis of tumor-infiltrating immune cells. Nucleic Acids Res. 48 (W1), W509–W514. doi:10.1093/nar/gkaa407
Liang, L., and Li, L. (2020). Down-regulation of circNRIP1 promotes the apoptosis and inhibits the migration and invasion of gastric cancer cells by miR-182/ROCK1 Axis. Onco. Targets. Ther. 13, 6279–6288. doi:10.2147/OTT.S221633
Liu, F., Zhang, H., Xie, F., Tao, D., Xiao, X., Huang, C., et al. (2020). Hsa_circ_0001361 promotes bladder cancer invasion and metastasis through miR-491-5p/MMP9 axis. Oncogene 39 (8), 1696–1709. doi:10.1038/s41388-019-1092-z
Liu, Q., Liao, Q., and Zhao, Y. (2017). Chemotherapy and tumor microenvironment of pancreatic cancer. Cancer Cell Int. 17, 68. doi:10.1186/s12935-017-0437-3
Liu, S., Gao, W., Lu, Y., Zhou, Q., Su, R., Hasegawa, T., et al. (2022). As a novel tumor suppressor, LHPP promotes apoptosis by inhibiting the PI3K/AKT signaling pathway in oral squamous cell carcinoma. Int. J. Biol. Sci. 18 (2), 491–506. doi:10.7150/ijbs.66841
Ma, S., Kong, S., Wang, F., and Ju, S. (2020). CircRNAs: Biogenesis, functions, and role in drug-resistant tumours. Mol. Cancer 19 (1), 119. doi:10.1186/s12943-020-01231-4
Martins, F., de Sousa, S. C., Dos, S. E., Woo, S. B., and Gallottini, M. (2016). PI3K-AKT-mTOR pathway proteins are differently expressed in oral carcinogenesis. J. Oral Pathol. Med. 45 (10), 746–752. doi:10.1111/jop.12440
Mello, F. W., Miguel, A., Dutra, K. L., Porporatti, A. L., Warnakulasuriya, S., Guerra, E., et al. (2018). Prevalence of oral potentially malignant disorders: A systematic review and meta-analysis. J. Oral Pathol. Med. 47 (7), 633–640. doi:10.1111/jop.12726
Nagaya, T., Nakamura, Y., Okuyama, S., Ogata, F., Maruoka, Y., Choyke, P. L., et al. (2017). Syngeneic mouse models of oral cancer are effectively targeted by anti-CD44-based NIR-PIT. Mol. Cancer Res. 15 (12), 1667–1677. doi:10.1158/1541-7786.MCR-17-0333
Ni, X. F., Zhao, L. H., Li, G., Hou, M., Su, M., Zou, C. L., et al. (2018). MicroRNA-548-3p and MicroRNA-576-5p enhance the migration and invasion of esophageal squamous cell carcinoma cells via NRIP1 down-regulation. Neoplasma 65 (6), 881–887. doi:10.4149/neo_2018_171206N803
Ocana, A., Nieto-Jimenez, C., Pandiella, A., and Templeton, A. J. (2017). Neutrophils in cancer: Prognostic role and therapeutic strategies. Mol. Cancer 16 (1), 137. doi:10.1186/s12943-017-0707-7
Oji, C., and Chukwuneke, F. (2012). Poor oral hygiene may be the sole cause of oral cancer. J. Maxillofac. Oral Surg. 11 (4), 379–383. doi:10.1007/s12663-012-0359-5
Oosting, S. F., and Haddad, R. I. (2019). Best practice in systemic therapy for head and neck squamous cell carcinoma. Front. Oncol. 9, 815. doi:10.3389/fonc.2019.00815
Ou, Z. L., Luo, Z., Wei, W., Liang, S., Gao, T. L., and Lu, Y. B. (2019). Hypoxia-induced shedding of MICA and HIF1A-mediated immune escape of pancreatic cancer cells from NK cells: Role of circ_0000977/miR-153 axis. RNA Biol. 16 (11), 1592–1603. doi:10.1080/15476286.2019.1649585
Petersen, P. E. (2009). Oral cancer prevention and control-the approach of the World Health Organization. Oral Oncol. 45 (4-5), 454–460. doi:10.1016/j.oraloncology.2008.05.023
Plattner, C., Finotello, F., and Rieder, D. (2020). Deconvoluting tumor-infiltrating immune cells from RNA-seq data using quanTIseq. Methods Enzymol. 636, 261–285. doi:10.1016/bs.mie.2019.05.056
Racle, J., de Jonge, K., Baumgaertner, P., Speiser, D. E., and Gfeller, D. (2017). Simultaneous enumeration of cancer and immune cell types from bulk tumor gene expression data. Elife 6, e26476. doi:10.7554/eLife.26476
Rakic, A., Beaudry, P., and Mahoney, D. J. (2018). The complex interplay between neutrophils and cancer. Cell Tissue Res. 371 (3), 517–529. doi:10.1007/s00441-017-2777-7
Reiser, J., and Banerjee, A. (2016). Effector, memory, and dysfunctional CD8(+) T cell fates in the antitumor immune response. J. Immunol. Res. 2016, 8941260. doi:10.1155/2016/8941260
Sarvaria, A., Madrigal, J. A., and Saudemont, A. (2017). B cell regulation in cancer and anti-tumor immunity. Cell. Mol. Immunol. 14 (8), 662–674. doi:10.1038/cmi.2017.35
Schaal, C., and Chellappan, S. P. (2014). Nicotine-mediated cell proliferation and tumor progression in smoking-related cancers. Mol. Cancer Res. 12 (1), 14–23. doi:10.1158/1541-7786.MCR-13-0541
Shen, M., and Ren, X. (2018). New insights into the biological impacts of immune cell-derived exosomes within the tumor environment. Cancer Lett. 431, 115–122. doi:10.1016/j.canlet.2018.05.040
Shi, D., Li, H., Zhang, J., and Li, Y. (2021). CircGDI2 regulates the proliferation, migration, invasion and apoptosis of OSCC via miR-454-3p/FOXF2 Axis. Cancer Manag. Res. 13, 1371–1382. doi:10.2147/CMAR.S277096
Shimizu, F., Shiiba, M., Ogawara, K., Kimura, R., Minakawa, Y., Baba, T., et al. (2013). Overexpression of LIM and SH3 Protein 1 leading to accelerated G2/M phase transition contributes to enhanced tumourigenesis in oral cancer. Plos One 8 (12), e83187. doi:10.1371/journal.pone.0083187
Song, W., and Fu, T. (2019). Circular RNA-associated competing endogenous RNA network and prognostic nomogram for patients with colorectal cancer. Front. Oncol. 9, 1181. doi:10.3389/fonc.2019.01181
Spence, T., Bruce, J., Yip, K. W., and Liu, F. F. (2016). HPV associated head and neck cancer. Cancers (Basel) 8 (8), E75. doi:10.3390/cancers8080075
Tamminga, M., Hiltermann, T., Schuuring, E., Timens, W., Fehrmann, R. S., and Groen, H. J. (2020). Immune microenvironment composition in non-small cell lung cancer and its association with survival. Clin. Transl. Immunol. 9 (6), e1142. doi:10.1002/cti2.1142
Tanahashi, H., and Tabira, T. (2002). Characterization of an amyloid precursor protein-binding protein Fe65L2 and its novel isoforms lacking phosphotyrosine-interaction domains. Biochem. J. 367 (3), 687–695. doi:10.1042/BJ20020562
Tomasetto, C., Moog-Lutz, C., Regnier, C. H., Schreiber, V., Basset, P., and Rio, M. C. (1995). Lasp-1 (MLN 50) defines a new LIM protein subfamily characterized by the association of LIM and SH3 domains. FEBS Lett. 373 (3), 245–249. doi:10.1016/0014-5793(95)01040-l
Tsou, P., Katayama, H., Ostrin, E. J., and Hanash, S. M. (2016). The emerging role of B cells in tumor immunity. Cancer Res. 76 (19), 5597–5601. doi:10.1158/0008-5472.CAN-16-0431
van der Leun, A. M., Thommen, D. S., and Schumacher, T. N. (2020). CD8(+) T cell states in human cancer: Insights from single-cell analysis. Nat. Rev. Cancer 20 (4), 218–232. doi:10.1038/s41568-019-0235-4
Vuong, L., Kotecha, R. R., Voss, M. H., and Hakimi, A. A. (2019). Tumor microenvironment dynamics in clear-cell renal cell carcinoma. Cancer Discov. 9 (10), 1349–1357. doi:10.1158/2159-8290.CD-19-0499
Wang, J., Zhao, X., Wang, Y., Ren, F., Sun, D., Yan, Y., et al. (2020). circRNA-002178 act as a ceRNA to promote PDL1/PD1 expression in lung adenocarcinoma. Cell Death Dis. 11 (1), 32. doi:10.1038/s41419-020-2230-9
Wang, S., Zhang, K., Tan, S., Xin, J., Yuan, Q., Xu, H., et al. (2021). Circular RNAs in body fluids as cancer biomarkers: The new frontier of liquid biopsies. Mol. Cancer 20 (1), 13. doi:10.1186/s12943-020-01298-z
Wong, P. F., Wei, W., Smithy, J. W., Acs, B., Toki, M. I., Blenman, K., et al. (2019). Multiplex quantitative analysis of tumor-infiltrating lymphocytes and immunotherapy outcome in metastatic melanoma. Clin. Cancer Res. 25 (8), 2442–2449. doi:10.1158/1078-0432.CCR-18-2652
Wu, J., Liu, S., Xiang, Y., Qu, X., Xie, Y., and Zhang, X. (2019). Bioinformatic analysis of circular RNA-associated ceRNA network associated with hepatocellular carcinoma. Biomed. Res. Int. 2019, 8308694. doi:10.1155/2019/8308694
Wu, T., and Dai, Y. (2017). Tumor microenvironment and therapeutic response. Cancer Lett. 387, 61–68. doi:10.1016/j.canlet.2016.01.043
Wu, T., Jiao, Z., Li, Y., Su, X., Yao, F., Peng, J., et al. (2022). HPRT1 promotes chemoresistance in oral squamous cell carcinoma via activating MMP1/PI3K/akt signaling pathway. Cancers (Basel) 14 (4), 855. doi:10.3390/cancers14040855
Zhang, H., Li, R., Cao, Y., Gu, Y., Lin, C., Liu, X., et al. (2020). Poor clinical outcomes and immunoevasive contexture in intratumoral IL-10-producing macrophages enriched gastric cancer patients. Ann. Surg. 275, e626–e635. doi:10.1097/SLA.0000000000004037
Zhang, H., Wang, Z., and Zhang, Z. (2021). Hsa_circ_0009128 mediates progression of oral squamous cell carcinoma by influencing MMP9. Oral Dis. doi:10.1111/odi.14019
Zhang, M., Liang, J., Yang, Y., Liang, H., Jia, H., and Li, D. (2020). Current trends of targeted drug delivery for oral cancer therapy. Front. Bioeng. Biotechnol. 8, 618931. doi:10.3389/fbioe.2020.618931
Keywords: oral squamous cell carcinoma, high-throughput sequencing, circular RNA, ceRNA network, prognostic model
Citation: He Y, Yang D, Li Y, Xiang J, Wang L and Wang Y (2022) Circular RNA-related CeRNA network and prognostic signature for patients with oral squamous cell carcinoma. Front. Pharmacol. 13:949713. doi: 10.3389/fphar.2022.949713
Received: 21 May 2022; Accepted: 15 November 2022;
Published: 01 December 2022.
Edited by:
Cheng Chen, Hefei Institutes of Physical Science (CAS), ChinaReviewed by:
Yan Li, Shandong Jiaotong University, ChinaJin-Hai Ye, Nanjing Medical University, China
Copyright © 2022 He, Yang, Li, Xiang, Wang and Wang. This is an open-access article distributed under the terms of the Creative Commons Attribution License (CC BY). The use, distribution or reproduction in other forums is permitted, provided the original author(s) and the copyright owner(s) are credited and that the original publication in this journal is cited, in accordance with accepted academic practice. No use, distribution or reproduction is permitted which does not comply with these terms.
*Correspondence: Liecheng Wang, wangliecheng@ahmu.edu.cn; Yuanyin Wang, wyy1970548@sohu.com
†These authors have contributed equally to this work and share first authorship