- 1Department of Psychology, University of Haifa, Haifa, Israel
- 2McLean Hospital and Harvard Medical School, Boston, MA, United States
Hofmann et al. argued that “[w]hile the clinical field has produced a dizzying number of treatment models and treatment protocols for virtually every psychiatric and psychological problem imaginable, increases in understanding of the processes of change in psychotherapy has been slow to arrive.” We propose that one of the reasons for the slow progress is that prior psychotherapy research conflates trait-like and state-like components of mechanisms of change. Trait-like components can serve as prescriptive or prognostic variables, whereas state-like components reflect within-client processes of change, and may highlight active ingredients of successful treatment. Distinguishing between the two is essential for clarifying the underlying processes of change in psychotherapy, and ultimately identifying empirically-derived individualized treatment targets. We review studies that implement methodological and statistical approaches for disentangling the two. These studies clarified particular mechanisms of change that may operate in a given treatment, highlighted differences in the processes of change between different treatments, and explored the within-individual interplay between different mechanisms of change during treatment. Examples include studies investigating the therapeutic role of behavioral, cognitive, and interpersonal skills, as well as emotional processing. We conclude with suggestions for future research, including attention to diversity, improved measurement to facilitate a reliable and valid estimation of trait-like and state-like components, the use of appropriate statistical approaches to adequately disentangle the two components, integration of theory-driven and data-driven methods of analysis, and the need to experimentally manipulate the state-like changes in a given mechanism of change to strengthen causal inferences.
Introduction
Theoretical conceptualizations of the mechanisms underlying psychotherapeutic change refer to dynamic, multivariable processes which unfold over the course of treatment (1). Within-client state-like changes in theory-specified mechanisms of change are assumed to contribute to reductions in symptoms and improvements in well-being. Researchers in many fields of science have shown that the trait-like qualities of a construct and state-like changes in it over time are meaningfully distinct entities and critical to disaggregate (2). One commonly used example illustrating the importance of disentangling trait-like and state-like components of the same construct is the association between typing speed (number of words typed per minute) and the percentage of typing errors made (3). At the between-individual or trait-like level, there is an inverse association: individuals who type faster tend to make fewer mistakes than those who type more slowly. In contrast, at the state-like level (i.e., within individuals), the association between typing speed and typos is positive: the faster one types, the more errors one is likely to make. As another example of the need to disentangle trait vs. state level effects, individuals who exercise more are, on average, at decreased risk of a heart attack relative to those who do not. However, at the individual level, one is at a higher risk of a heart attack during intensive exercise relative to at rest (4). A third example in which trait-like and state-like effects show opposite directions is of the effect of self-efficacy on performance. Whereas the trait-like effect of self-efficacy on performance is positive [individuals with higher self-efficacy show better performance; (5)], the state-like effect is negative [a state-like boost in self-efficacy may result in poorer performance; (6)], due, perhaps, to overconfidence in one's abilities.
As these three examples demonstrate, effects examined at the trait-like vs. state-like level can not only be inconsistent, but even opposite in direction. In addition, as described in more details below, trait-like level characteristics may moderate state-like effects (e.g., the within-individual association of exercise on heart attack risk is moderated by pre-existing cardiovascular risk factors). Below, we discuss the importance of disentangling trait-like and state-like effects to clarify the mechanism of change in CBT and for informing treatment selection and targets.
Key Challenge in the Study of Mechanisms of Change in CBT: Conflating Trait-Like and State-Like Effects
Reviews and meta-analyses on the mechanisms of change in CBT for depression suggest that CBT may improve dysfunctional thinking (7), and in turn, that cognitive change is associated with better treatment outcomes (8). However, most studies on the mechanism of change in CBT, including those focused on the core question of the role of cognitive change, are constrained in the causal inferences they can draw due to methodological limitations and have yielded mixed findings (9). For example, in the treatment of depression, a meta-analysis suggested that adherence and competence are, on average, not significantly associated with treatment outcome, with mixed findings across the included studies (10). Mixed results have also been obtained for treatments of anxiety disorders. For example, Foa and Kozak's emotional processing theory (11) was supported by some studies (12, 13) but not by others (14, 15). Similarly, the inhibitory learning theory of Craske et al. (16) produced mixed results, with some studies supporting it (14, 17) and others describing a more complex picture (18). The mixed results are so profound that in their systematic review of the literature on common factors across psychotherapies, Cuijpers, Reijnders, and Huibers (19) concluded that: “It is as if we have been in the pilot phase of research for five decades without being able to dig deeper” (p. 224).
An important factor that may help account, at least in part, for the mixed results is that most studies conflate trait-like and state-like components. As others have emphasized, it is critical to disentangle trait-like (between-individuals variance) and state-like (within-individual variance) components (2, 20), especially with the type of data generated in psychotherapy research (21). Inferences drawn from studies that do not disaggregate trait-like and state-like components can be strikingly different relative to those that do. As has been argued by Fisher and colleagues (21): “… conclusions drawn from aggregated data may be worryingly imprecise” (p. 6106). Trait-like variability refers to any variance between individuals in their traits or relatively stable characteristics. For example, within psychotherapy, trait-like characteristics may describe relatively enduring, automatic pre-treatment patterns of thoughts, feelings, and behaviors that are consistent across similar situations. Trait-like components may refer to relatively fixed entities (like many demographic variables) or to a recurring, dynamic pattern that characterizes the individual [i.e., predicable diurnal cycles in anxiety; (22)]. State-like changes may include reductions or increases in a trait-like characteristic (e.g., reduction in previously stable levels of anxiety) or deviations from a previously stable dynamic pattern (e.g., attenuation of a strong diurnal pattern of anxiety), potentially as the result of treatment. The trait-like components may serve as (a) “prognostic” (i.e., treatment non-specific) predictors – stable client characteristics that influence one's ability to benefit from any treatment (e.g., cognitive impairment or interpersonal pathology) or as (b) “prescriptive” variables (i.e., moderators) – variables that predict differential response to one treatment vs. another (e.g., CBT vs. antidepressants). In contrast, state-like components refer to within-individual variation in a construct that occur over time, such as in a mechanism of change as a result of implementing therapeutic techniques that target those mechanisms. State-like changes in those mechanisms are in turn expected to bring about changes in symptoms. The trait-like vs. state-like distinction may shed light on inconsistent earlier findings.
Benefits of Disaggregating Trait-Like and State-Like Components
Clarifying the Mechanisms of Change
A core feature of CBT is the focus on the acquisition of cognitive (e.g., identifying and interrogating negative automatic thoughts) and behavioral (e.g., behavioral activation) skills. To what extent does client use of cognitive and/or behavioral skills in fact contribute to depressive symptom change? To adequately address this question, the state-like component (i.e., variance in cognitive and behavioral skills within clients over the course of treatment) needs to be isolated from the trait-like component (i.e., stable, between-client differences in the tendency to report generally high vs. low levels of cognitive or behavioral skills) (see Figure 1 for a simulated example to illustrate state-like vs. trait-like effects). In a recent study, Webb et al. (23) found that client-reported use of behavioral – but not cognitive – skills predicted symptom change in CBT for depressed adolescents. The latter finding emerged when using conventional analyses (i.e., not disaggregating state-like and trait-like components). However, when disaggregating these two components, only the state-like components were significant. Specifically, and consistent with a causal interpretation, greater state-like within-individual levels of behavioral skills predicted greater depressive symptom change. The same pattern of findings emerged whether client skills were assessed from the perspective of the client themselves or from the therapist. Importantly, and similar to the abovementioned typing speed and heart attack examples, one could certainly imagine how trait-like and state-like effects could operate in opposite directions. For example, those individuals with trait-like deficits in behavioral activation (BA) skills may be more likely than those with relatively high levels of skills to benefit from BA therapy, given that the latter treatment specifically targets that skill set (i.e., lower trait-like skills predicts relatively enhanced response to BA). In contrast, greater state-like within-individual increases in BA skills may predict better outcomes within treatment (i.e., the opposite relation for the state-like effect). An example for such opposite directions of trait-like and state-like components comes from a study by Rubel and colleagues (24). In their study, higher levels of state-like in-session affective experiences and involvement were associated with a greater subsequent reduction in symptoms. However, the trait-like effects were in the opposite direction: higher overall levels of affective experiences were associated with higher overall symptom severity.
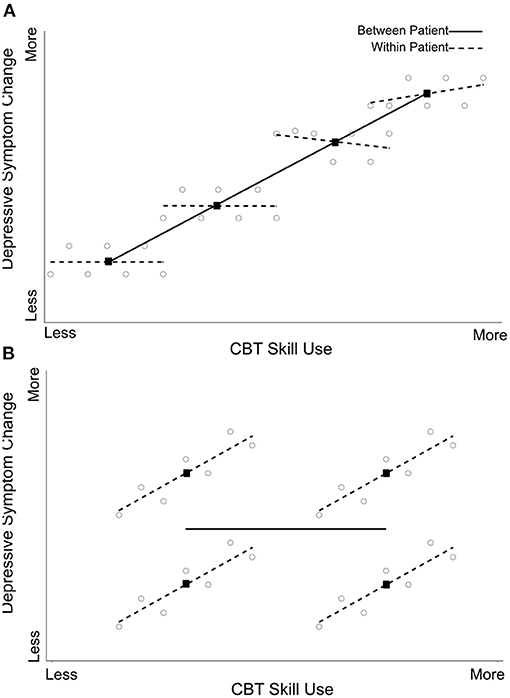
Figure 1. Webb et al. (23) American Psychological Association. Reprinted with permission. The figure displays simulated data from four clients (seven time points per client) showing a between-client (dark line), but no within-client (dotted lines), effect of skills on symptom improvement (A) vs. a within-client, but no between-client, effect of skills on outcome (B). Circles represent skill scores for each client at each time points, and black squares refer to each client's mean skill score.
Disentangling trait-like and state-like components is also important for identifying which techniques bring about changes within individuals. For example, when using conventional analyses (i.e., not disentangling trait-like and state-like components), both adherence to identifying and evaluating automatic thoughts and adherence to negotiating therapy content with the client and structuring the session were significant predictors of treatment outcome (25). In contrast, with the trait-like vs. state-like distinction, only state-like changes in adherence to identifying and evaluating automatic thoughts predicted next-session symptom change. Such findings may help inform which techniques therapists should consider implementing in a session to bring about better treatment outcomes (26). Another example for distinct effects at the trait-like and state-like levels comes from the research on non-verbal synchrony. Recent findings suggest that at the between-individual level, trait-like non-verbal synchrony was not associated with either problem actuation or motivational clarification. However, at the within-client level, state-like non-verbal synchrony was associated with both problem actuation and motivational clarification (27).
Identifying Differences in Mechanisms of Change Between Treatments
One of the most replicated findings in psychotherapy research is that treatments conceptualized as working via different mechanisms often show similar outcomes at the end of treatment [commonly referred to as the Dodo Bird Verdict, (28, 29)]. Based on this finding, many scholars have argued that all treatments work through the same mechanisms, and consequently questioned the claim that different treatments have unique mechanisms of change. We argue that the trait-like vs. state-like distinction may have the potential to reveal different mechanisms of change underlying distinct treatments. Of relevance, a recent study (30) comparing exposure-based cognitive therapy (EBCT) and cognitive-behavioral therapy (CBT) for depression found that although EBCT augments CBT by the addition of exposure-based strategies, no significant differences between the two conditions emerged in their treatment outcomes. After making the trait-like vs. state-like distinction (using a centering approach), however, EBCT was found to result in greater state-like increases in emotional processing during treatment and higher self-efficacy during follow-up relative to CBT, both of which were associated with better long-term depression outcome (31). One potentially fruitful avenue for future research is to identify individuals who may benefit most from integrating emotional processing strategies. For those individuals, EBCT may result in better outcomes than CBT, given that the former treatment directly targets emotional processing. Another example comes from research on the working alliance, which is commonly referred to as a non-specific common factor. Studies suggest that trait-like differences in the alliance between patients are indeed associated with treatment outcome across different treatments, with stronger alliances being linked to better outcomes (32). However, in treatments that directly focus on the alliance as a mechanism of change (e.g., brief relational treatment), SL changes in alliance were stronger predictors of subsequent treatment outcome, than in treatments where the alliance is typically not considered a main mechanism of change (33, 34).
The Longitudinal Interplay Between Different Mechanisms of Change
Although studies commonly focus on a single mechanism of change, the reality of clinical practice teaches us that for a given individual a variety of factors – and complex interactions among them – are contributing to symptom change. For example, state-like changes in one mechanism may be moderated by trait-like levels of another, suggesting that the processes or mechanisms of change may differ as a function of identifiable client characteristics, and thus answering the question for whom a given therapeutic procedure may be most beneficial. For example, Fitzpatrick et al. (35) explored the question of who benefits most from cognitive change in cognitive therapy for depression. After disaggregating state-like and trait-like components of cognitive change, using a centering approach (20), the authors found that clients with poorer trait-like interpersonal skills and greater trait-like interpersonal problems exhibited a stronger relation between state-like changes in cognition and symptom improvement.
Moreover, state-like changes in one mechanism may be moderated by state-like changes in another. This type of interaction may suggest how two mechanisms of change interact to bring about therapeutic change. Interactions between state-like components of two or more mechanisms or other process variables may also guide clinical decisions on when to target a specific mechanism. Specifically, state-like changes in process variables may provide useful and actionable information about the optimal timing for implementing procedures that target a specific mechanism of change. In this case, the interaction may suggest when (i.e., at which levels of the process variable) state-like changes in a particular mechanism of change are most beneficial in bringing about therapeutic change. For example, Zilcha-Mano (36) found that state-like improvements in alliance at a given session result in subsequent reduction in symptoms only in the case of higher sense of life satisfaction at that session, thus suggesting when it may be most therapeutically beneficial to implement techniques for strengthening the alliance.
Interactions between trait-like and state-like components of the same construct may be of particular interest, because they may contribute to progress toward precision medicine (37). Such interactions serve as a test of two contrasting hypotheses: building on clients' relative weaknesses vs. capitalizing on their strengths (38). A recent meta-analysis based on individual level data from 5,350 individuals suggested that the effect of state-like changes in alliance on outcome was stronger for individuals with stronger trait-like alliance (39). This finding supports the capitalizing on the clients' strengths hypothesis: those with stronger trait-like alliance are the ones who derive the most therapeutic benefit from state-like gains in the alliance. Building on the BA example above, individuals with relatively higher baseline competency in BA skills may be more likely to take advantage of a BA treatment that capitalizes on their pre-existing strengths (40, 41). Whereas the latter example is consistent with a “capitalization” model, one could also imagine a “compensatory” model [i.e., individuals with trait-like deficits in BA skills benefit the most from a treatment (BA) that directly targets their deficit].
The examples so far focused on moderation. However, another way in which state-like changes in two variables can relate to each other is by one preceding the other, in a within-client mediation model, to delineate the temporal process of how therapeutic change occurs. For example, Schmidt et al. (42) found that immediate state-like cognitive changes predicted sustained cognitive changes, which in turn predicted treatment outcome.
What Does the Future Hold?
Below we highlight several promising directions for future treatment research disentangling state-like within-person vs. trait-like between-person effects.
Better Measurement Will Facilitate a More Reliable and Valid Estimation of the Trait-Like and State-Like Components
When distinguishing between trait-like and state-like components, the ability to capture dynamic patterns is critical. Based on an accurate assessment of baseline trait-like dynamics, it is possible to investigate not only whether the individual's average values of a construct have changed, but also whether the trait-like dynamics have changed. For example, mean level of negative affect (NA) may change as a result of effective treatment, and the dynamic pattern of the individual may change as well (e.g., attenuated fluctuations in NA; Figure 2). Capturing this dynamic before, during the course of, and after treatment requires frequent sampling of NA in the daily lives of individuals. Given the omnipresence of smartphones, ecological momentary assessment (EMA) has become increasingly popular in psychological research and holds promise for psychotherapy studies investigating relevant state-like within-person vs. trait-like between-person processes. In addition to EMA, the expansion of “passive” (i.e., no user input required) measurement methods also holds promise for examining predictors and processes of change, including sensor data (e.g., activity levels and movement from accelerometer and GPS, proxies of social interaction from call and text meta-data) from smartphones and wearables (43), as well as other markers based on motion (44, 45), acoustic and language style (46–48) and physiology (49). The extent to which biological variables, such as hormones (50), neuroimaging (51–53) and inflammatory biomarkers (54), provide incremental predictive validity above conventional (and less costly and time-consuming) self-report measures is also an important area of research (55).
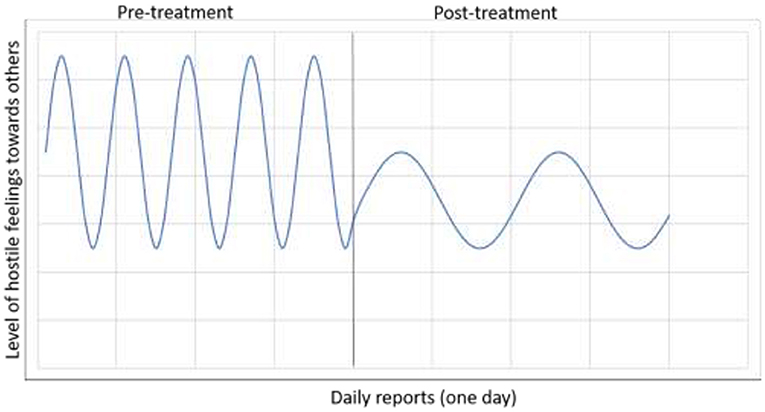
Figure 2. A demonstration of change in both the overall mean level of a construct and in its dynamic pattern as the result of treatment. The client started treatment with a generally high level of negative affect, and specifically, higher levels of hostile feelings toward others, characterized by a pattern of frequent and extreme daily reports of hostility. In the course of treatment, the overall mean level of hostile feelings was reduced. The dynamic pattern changed as well, the client displaying less frequent and less extreme hostility toward others.
The Use of Appropriate Statistical Analyses for Disentangling Trait-Like and State-Like Components
The trait-like vs. state-like distinction requires specialized statistical approaches to disaggregate and analyze these two components. First, it is important to use the appropriate methods to make the distinction, which fit the type of the data collected (20), and it is equally important to use appropriate statistical methods in analyzing each of the two components. Many statistical methods currently being used to analyze psychotherapy data are suitable for handling the trait-like components of mechanisms of change, but not the state-like components. For example, the analyses conducted to identify factors at the basis of the majority of available self-report scales are appropriate for trait-like components, but not for state-like components, and yet the same scales are often used to assess within-subject, should be within-individual. Factor structures of a scale for trait-like and state-like components of the same construct may differ (56). It is essential, therefore, to use the factor analyses that are suitable for state-like data. As another example, Group Iterative Multiple Model Estimation [GIMME; (57)], based on a unified structural equation modeling [uSEM; (58)] framework, integrates within-individual (idiographic) and conventional between-individual (nomothetic) modeling. Specifically, GIMME estimates subject-specific associations, as well searches for commonalities between individuals in those relations (59). With sufficient data points per individual (e.g., repeated EMA of relevant mechanism of change variables and outcome assessments), GIMME may allow psychotherapy researchers to estimate common patterns in mechanisms of change across clients, while simultaneously capturing individual-level heterogeneity in those variable relations (i.e., client-specific patterns). A detailed discussion of GIMME, and related approaches, is beyond the scope of this review. However, it is important to note that there are a number of assumptions that should be met with such time series data (e.g., stationarity, approximately equal time intervals between assessments, continuous variables; see (59, 60).
Integration of Theory-Driven and Data-Driven Methods of Analysis
Data-driven approaches have been increasingly common in psychotherapy research in recent years and may have fruitful applications for research focused on trait-like vs. state-like distinctions (40, 61, 62). As one example from relationship science, a team of researchers recently sought to predict the construct of relationship quality (63). Using a machine learning approach with a total sample of 11,196 and 2,413 potential predictors, the researchers discovered a similar pattern of findings to those that have accumulated in many fields of science: the trait-like and state-like components of relationship quality produce distinct patterns. Up to about half the variance in the trait-like component of relationship quality can be explained by the individual's baseline trait-like predictors (e.g., attachment avoidance). By contrast, the variance of the state-like component of relationship quality (that is, relationship quality change) was largely unpredictable. Similar results demonstrating the differences in predicting trait-like vs. state-like components were obtained regarding other constructs [e.g., (64–66)]. With regards to research investigating mechanisms of change in psychotherapy research, recent work has revealed the challenges in identifying predictors of the state-like effect of alliance on outcome (62), although some promising results have been obtained when a variety of potential interpersonal predictors were used (67). Promising results have also been obtained in a recent study using a machine learning approach to predict client-specific skill-affect associations based on baseline clinical and demographic characteristics (59). These preliminary findings on the implementation of machine leaning approaches to identifying predictors of state-like effects stress the importance of thoughtful selection of relevant predictors in future trial designs, as well as consideration of a variety of machine leaning-related analytical approaches. It is also worth noting that computational models of psychological change and recovery that attempt to directly emulate the psychological mechanisms occurring within each individual client may contribute to progress in psychotherapy research toward precision medicine (68).
Demonstrating Causality
Establishing a correct temporal relationship between state-like changes in a mechanism of change and subsequent symptomatic change is important in progress toward inferring causality, but a more direct (experimental) test of the effect of state-like manipulation is needed. Examples of direct manipulation of mechanisms of change include the administration of D-cycloserine and hydrocortisone as facilitators of inhibitory learning in exposure therapy (18), as well as the direct modulation of brain function connectivity using approaches such as transcranial magnetic stimulation (TMS) of the cerebellar midline (69).
Attention to Diversity
Different mechanisms of change may be at play for different populations. As Hollon (70) has argued, moderated mediation models can improve the precision of the tested mediation model because they take into account the different processes that may come into play for different individuals. Potential moderators may include clinical symptoms [e.g., therapists adherence to cognitive techniques may play a relatively more prominent role in contributing to symptom change among clients with more severe depressive symptoms, (71); whereas the reverse may be the case with regards to the alliance, (39)] and socio-demographic variables [e.g., adherence to cognitive techniques may be more critical for women than men (72)]. Attention to such diversity may contribute to more contextually appropriate implementations of therapeutic procedures to bring about state-like changes in specific mechanisms of change.
Summary
Although studies focused on the mechanisms of change in psychotherapy have been published at a rapid rate, our understanding of underlying processes of change has made slow progress and produced contradictory results. In the present article, we propose that one contributing factor to the slow advance and the mixed results is the conflation of trait-like and state-like components of individual mechanisms of change. As has been demonstrated before, the two components have distinct meanings and play different roles in treatment (37), and studies can yield very different findings depending on whether these two components are conflated or disaggregated (21, 32).
As reviewed, studies leveraging methodological and statistical approaches to disaggregate trait-like and state-like components can yield important findings on the processes of psychotherapeutic change, including: (a) clarifying within-client mechanisms of change in CBT (as in the example of state-like changes in behavioral skills predicting a reduction in depressive symptoms, Figure 1); (b) identifying differences between treatments in putative mechanisms of change (as in the example of the mechanisms targeted in ECBT vs. CBT); and (c) exploring the interplay between mechanisms of change in the process of bringing about therapeutic change, with the aim of clarifying the optimal circumstances and timing for targeting any given mechanism or a series of mechanisms (such as interactions between trait-like and state-like components of multiple mechanisms of change to answer the questions for whom, when, and how to implement given therapeutic procedures). It is of course important to note that disaggregating state-like and trait-like effects is relevant to psychotherapy research more broadly, and not just CBT (37).
We are optimistic about the future of psychotherapy science implementing the trait-like vs. state-like distinction using interdisciplinary approaches. The accumulation of data making this distinction will be instrumental in building clear and detailed links between evidence-based procedures (e.g., exposure, mindfulness practices) and evidence-based mechanisms and processes (e.g., cognitive flexibility and diffusion/distancing)(1).
Data Availability Statement
The original contributions presented in the study are included in the article/supplementary materials, further inquiries can be directed to the corresponding author/s.
Author Contributions
SZ-M and CW contributed equality to conceiving the presented ideas and to the writing of the manuscript. All authors contributed to the article and approved the submitted version.
Funding
The writing of this article was supported by two grants from the Israeli Science Foundation (Grant 186/15; 395/19 to SZ-M). CW was partially supported by R01 MH116969, the Tommy Fuss Fund, and a NARSAD Young Investigator Grant from the Brain & Behavior Research Foundation. The opinions and assertions contained in this article should not be construed as reflecting the views of the sponsors.
Conflict of Interest
The authors declare that the research was conducted in the absence of any commercial or financial relationships that could be construed as a potential conflict of interest.
References
1. Hofmann SG, Hayes SC. The future of intervention science: process-based therapy. Clin Psychol Sci. (2019) 7:37–50. doi: 10.1177/2167702618772296
2. Curran PJ, Bauer DJ. The disaggregation of within-person and between-person effects in longitudinal models of change. Annu Rev Psychol. (2011) 62:583–619. doi: 10.1146/annurev.psych.093008.100356
3. Hamaker EL. Why researchers should think “within-person”: a paradigmatic rationale. In: Mehl MR, Conner TS, editors. Handbook of Research Methods for Studying Daily Life. New York, NY: The Guilford Press (2012). p. 43–61.
5. Bandura A. Self-efficacy mechanism in human agency. Am Psychol. (1982) 37:122. doi: 10.1037/0003-066X.37.2.122
6. Vancouver JB, Thompson CM, Tischner EC, Putka DJ. Two studies examining the negative effect of self-efficacy on performance. J Appl Psychol. (2002) 87:506. doi: 10.1037/0021-9010.87.3.506
7. Cristea IA, Kok RN, Cuijpers P. Efficacy of cognitive bias modification interventions in anxiety and depression: meta-analysis. Br J Psychiatry. (2015) 206:7–16. doi: 10.1192/bjp.bp.114.146761
8. Garratt G, Ingram RE, Rand KL, Sawalani G. Cognitive processes in cognitive therapy: evaluation of the mechanisms of change in the treatment of depression. Clin Psychol Sci Pract. (2007) 14:224–39. doi: 10.1111/j.1468-2850.2007.00081.x
9. Lorenzo-Luaces L, German RE, DeRubeis RJ. It's complicated: the relation between cognitive change procedures, cognitive change, and symptom change in cognitive therapy for depression. Clin Psychol Rev. (2015) 41:3–15. doi: 10.1016/j.cpr.2014.12.003
10. Webb CA, DeRubeis RJ, Barber JP. Therapist adherence/competence and treatment outcome: a meta-analytic review. J Consult Clin Psychol. (2010) 78:200. doi: 10.1037/a0018912
11. Foa EB, Kozak MJ. Emotional processing of fear: exposure to corrective information. Psychol Bull. (1986) 99:20. doi: 10.1037/0033-2909.99.1.20
12. Harned MS, Ruork AK, Liu J, Tkachuck MA. Emotional activation and habituation during imaginal exposure for PTSD among women with borderline personality disorder. J Trauma Stress. (2015) 28:253–7. doi: 10.1002/jts.22013
13. Sripada RK, Rauch SAM. Between-session and within-session habituation in prolonged exposure therapy for posttraumatic stress disorder: a hierarchical linear modeling approach. J Anxiety Disord. (2015) 30:81–7. doi: 10.1016/j.janxdis.2015.01.002
14. Culver NC, Stoyanova M, Craske MG. Emotional variability and sustained arousal during exposure. J Behav Ther Exp Psychiatry. (2012) 43:787–93. doi: 10.1016/j.jbtep.2011.10.009
15. Meuret AE, Seidel A, Rosenfield B, Hofmann SG, Rosenfield D. Does fear reactivity during exposure predict panic symptom reduction? J Consult Clin Psychol. (2012) 80:773. doi: 10.1037/a0028032
16. Craske MG, Liao B, Brown L, Vervliet B. Role of inhibition in exposure therapy. J Exp Psychopathol. (2012) 3:322–45. doi: 10.5127/jep.026511
17. Rothbaum BO, Price M, Jovanovic T, Norrholm SD, Gerardi M, Dunlop B, et al. A randomized, double-blind evaluation of D-cycloserine or alprazolam combined with virtual reality exposure therapy for posttraumatic stress disorder in Iraq and Afghanistan War veterans. Am J Psychiatry. (2014) 171:640–8. doi: 10.1176/appi.ajp.2014.13121625
18. de Kleine RA, Smits JAJ, Hendriks G-J, Becker ES, van Minnen A. Extinction learning as a moderator of d-cycloserine efficacy for enhancing exposure therapy in posttraumatic stress disorder. J Anxiety Disord. (2015) 34:63–7. doi: 10.1016/j.janxdis.2015.06.005
19. Cuijpers P, Reijnders M, Huibers MJH. The role of common factors in psychotherapy outcomes. Annu Rev Clin Psychol. (2019) 15:207–31. doi: 10.1146/annurev-clinpsy-050718-095424
20. Wang LP, Maxwell SE. On disaggregating between-person and within-person effects with longitudinal data using multilevel models. Psychol Methods. (2015) 20:63. doi: 10.1037/met0000030
21. Fisher AJ, Medaglia JD, Jeronimus BF. Lack of group-to-individual generalizability is a threat to human subjects research. Proc Natl Acad Sci. (2018) 115:E6106–15. doi: 10.1073/pnas.1711978115
22. Fisher AJ, Newman MG. Reductions in the diurnal rigidity of anxiety predict treatment outcome in cognitive behavioral therapy for generalized anxiety disorder. Behav Res Ther. (2016) 79:46–55. doi: 10.1016/j.brat.2016.02.006
23. Webb CA, Stanton CH, Bondy E, Singleton P, Pizzagalli DA, Auerbach RP. Cognitive versus behavioral skills in CBT for depressed adolescents: disaggregating within-patient versus between-patient effects on symptom change. J Consult Clin Psychol. (2019) 87:484. doi: 10.1037/ccp0000393
24. Rubel JA, Rosenbaum D, Lutz W. Patients' in-session experiences and symptom change: session-to-session effects on a within-and between-patient level. Behav Res Ther. (2017) 90:58–66. doi: 10.1016/j.brat.2016.12.007
25. Strunk DR, Brotman MA, DeRubeis RJ. The process of change in cognitive therapy for depression: predictors of early inter-session symptom gains. Behav Res Ther. (2010) 48:599–606. doi: 10.1016/j.brat.2010.03.011
26. Sasso KE, Strunk DR, Braun JD, DeRubeis RJ, Brotman MA. A re-examination of process–outcome relations in cognitive therapy for depression: disaggregating within-patient and between-patient effects. Psychother Res. (2016) 26:387–98. doi: 10.1080/10503307.2015.1026423
27. Prinz J, Boyle K, Ramseyer F, Kabus W, Bar-Kalifa E, Lutz W. Within and between associations of nonverbal synchrony in relation to Grawe's general mechanisms of change. Clin Psychol Psychother. (2020). doi: 10.1002/cpp.2498
28. Rosenzweig S. Some implicit common factors in diverse methods of psychotherapy. Am J Orthopsychiatry. (1936) 6:412. doi: 10.1111/j.1939-0025.1936.tb05248.x
29. Luborsky L, Singer B, Luborsky L. Comparative studies of psychotherapies: is it true that everyone has won and all must have prizes? Arch Genl Psychiatry. (1975) 32:995–1008. doi: 10.1001/archpsyc.1975.01760260059004
30. Grosse Holtforth M, Krieger T, Zimmermann J, Altenstein-Yamanaka D, Dörig N, Meisch L, et al. A randomized-controlled trial of cognitive–behavioral therapy for depression with integrated techniques from emotion-focused and exposure therapies. Psychother Res. (2019) 29:30–44. doi: 10.1080/10503307.2017.1397796
31. Gómez Penedo JM, Coyne AE, Constantino MJ, Krieger T, Hayes AM. Theory-specific patient change processes and mechanisms in different cognitive therapies for depression. J Consult Clin Psychol. (2020) 88:774. doi: 10.1037/ccp0000502
32. Zilcha-mano S. Is the alliance really therapeutic? Revisiting this question in light of recent methodological advances. Am Psychol. (2017) 72:311–25. doi: 10.1037/a0040435
33. Zilcha-Mano S, Muran JC, Hungr C, Eubanks CF, Safran JD, Winston A. The relationship between alliance and outcome: Analysis of a two-person perspective on alliance and session outcome. J Consult Clin Psychol. (2016) 84:484. doi: 10.1037/ccp0000058
34. Zilcha-Mano S, Eubanks CF, Muran JC. Sudden gains in the alliance in cognitive behavioral therapy versus brief relational therapy. J Consult Clin Psychol. (2019) 87:501. doi: 10.1037/ccp0000397
35. Fitzpatrick S, Bailey K, Rizvi SL. Changes in emotions over the course of dialectical behavior therapy and the moderating role of depression, anxiety, and posttraumatic stress disorder. Behav Ther. (2019) 51:946–57. doi: 10.1016/j.beth.2019.12.009
36. Zilcha-Mano S, Lipsitz I, Errázuriz P. When is it effective to focus on the alliance? Analysis of a within-client moderator. Cognit Ther Res. (2018) 42:159–71. doi: 10.1007/s10608-017-9867-4
37. Zilcha-Mano S. Toward personalized psychotherapy: the importance of the trait-like/state-like distinction for understanding therapeutic change. Am Psychol. (2020). doi: 10.1037/amp0000629
38. Cheavens JS, Strunk DR, Lazarus SA, Goldstein LA. The compensation and capitalization models: a test of two approaches to individualizing the treatment of depression. Behav Res Ther. (2012) 50:699–706. doi: 10.1016/j.brat.2012.08.002
39. Flückiger C, Rubel J, del Re AC, Horvath AO, Wampold BE, Crits-Christoph P, et al. The reciprocal relationship between alliance and early treatment symptoms: a two-stage individual participant data meta-analysis. J Consult Clin Psychol. (2020) 88:829. doi: 10.1037/ccp0000594
40. Webb CA, Cohen ZD, Beard C, Forgeard M, Peckham AD, Björgvinsson T. Personalized prognostic prediction of treatment outcome for depressed patients in a naturalistic psychiatric hospital setting: a comparison of machine learning approaches. J Consult Clin Psychol. (2020) 88:25. doi: 10.1037/ccp0000451
41. Forbes CN. New directions in behavioral activation: using findings from basic science and translational neuroscience to inform the exploration of potential mechanisms of change. Clin Psychol Rev. (2020) 79:101860. doi: 10.1016/j.cpr.2020.101860
42. Schmidt ID, Pfeifer BJ, Strunk DR. Putting the “cognitive” back in cognitive therapy: sustained cognitive change as a mediator of in-session insights and depressive symptom improvement. J Consult Clin Psychol. (2019) 87:446. doi: 10.1037/ccp0000392
43. Jacobson NC, Chung YJ. Passive sensing of prediction of moment-to-moment depressed mood among undergraduates with clinical levels of depression sample using smartphones. Sensors. (2020) 20:3572. doi: 10.3390/s20123572
44. Lutz W, Prinz JN, Schwartz B, Paulick J, Schoenherr D, Deisenhofer A-K, et al. Patterns of early change in interpersonal problems and their relationship to nonverbal synchrony and multidimensional outcome. J Couns Psychol. (2020) 67:449. doi: 10.1037/cou0000376
45. Ramseyer FT. Motion energy analysis (MEA): a primer on the assessment of motion from video. J Couns Psychol. (2020) 67:536. doi: 10.1037/cou0000407
46. Aafjes-van Doorn K, Porcerelli J, Müller-Frommeyer LC. Language style matching in psychotherapy: an implicit aspect of alliance. J Couns Psychol. (2020) 67:509. doi: 10.1037/cou0000433
47. Reich CM, Berman JS, Dale R, Levitt HM. Vocal synchrony in psychotherapy. J Soc Clin Psychol. (2014) 33:481–94. doi: 10.1521/jscp.2014.33.5.481
48. Goldberg SB, Flemotomos N, Martinez VR, Tanana MJ, Kuo PB, Pace BT, et al. Machine learning and natural language processing in psychotherapy research: alliance as example use case. J Couns Psychol. (2020) 67:438. doi: 10.1037/cou0000382
49. Kleinbub JR, Talia A, Palmieri A. Physiological synchronization in the clinical process: a research primer. J Couns Psychol. (2020) 67:420. doi: 10.1037/cou0000383
50. Zilcha-Mano S, Shamay-Tsoory S, Dolev-Amit T, Zagoory-Sharon O, Feldman R. Oxytocin as a biomarker of the formation of therapeutic alliance in psychotherapy and counseling psychology. J Couns Psychol. (2020) 67:523. doi: 10.1037/cou0000386
51. Webb CA, Weber M, Mundy EA, Killgore WDS. Reduced gray matter volume in the anterior cingulate, orbitofrontal cortex and thalamus as a function of mild depressive symptoms: a voxel-based morphometric analysis. Psychol Med. (2014) 44:2833. doi: 10.1017/S0033291714000348
52. Webb CA, Olson EA, Killgore WDS, Pizzagalli DA, Rauch SL, Rosso IM. Rostral anterior cingulate cortex morphology predicts treatment response to internet-based cognitive behavioral therapy for depression. Biol Psychiatry Cogn Neurosci Neuroimaging. (2018) 3:255–62. doi: 10.1016/j.bpsc.2017.08.005
53. Pizzagalli DA, Webb CA, Dillon DG, Tenke CE, Kayser J, Goer F, et al. Pretreatment rostral anterior cingulate cortex theta activity in relation to symptom improvement in depression: a randomized clinical trial. JAMA Psychiatry. (2018) 75:547–54. doi: 10.1001/jamapsychiatry.2018.0252
54. Moriarity DP, Kautz MM, mac Giollabhui N, Klugman J, Coe CL, Ellman LM, et al. Bidirectional associations between inflammatory biomarkers and depressive symptoms in adolescents: potential causal relationships. Clin Psychol Sci. (2020) 8:690–703. doi: 10.1177/2167702620917458
55. Zilcha-Mano S, Ramseyer FT. Innovative approaches to exploring processes of change in counseling psychology: insights and principles for future research. J Couns Psychol. (2020) 67:409. doi: 10.1037/cou0000426
56. McAleavey AA, Castonguay LG, Hayes JA, Locke BD. Multilevel vs. single-level factor analysis: differentiating within-person and between-person variability using the CCAPS-34. J Consult Clin Psychol. (2020) 88:907–22. doi: 10.1037/ccp0000529
57. Gates KM, Molenaar PCM. Group search algorithm recovers effective connectivity maps for individuals in homogeneous and heterogeneous samples. NeuroImage. (2012) 63:310–9. doi: 10.1016/j.neuroimage.2012.06.026
58. Kim J, Zhu W, Chang L, Bentler PM, Ernst T. Unified structural equation modeling approach for the analysis of multisubject, multivariate functional MRI data. Hum Brain Mapp. (2007) 28:85–93. doi: 10.1002/hbm.20259
59. Webb CA, Forgeard M, Israel E, Lovell-Smith NM, Björgvinsson T, Beard C. Personalized prescriptions of therapeutic skills from patient characteristics: an ecological momentary assessment approach. J Consult Clin Psychol. (In press).
60. Beltz AM, Gates KM. Network mapping with GIMME. Multivariate Behav Res. (2017) 52:789–804. doi: 10.1080/00273171.2017.1373014
61. Cohen ZD, DeRubeis RJ. Treatment selection in depression. Annu Rev Clin Psychol. (2018) 14:209–36. doi: 10.1146/annurev-clinpsy-050817-084746
62. Rubel JA, Zilcha-Mano S, Giesemann J, Prinz J, Lutz W. Predicting personalized process-outcome associations in psychotherapy using machine learning approaches—A demonstration. Psychother Res. (2020) 30:300–9. doi: 10.1080/10503307.2019.1597994
63. Joel S, Eastwick PW, Allison CJ, Arriaga XB, Baker ZG, Bar-Kalifa E, et al. Machine learning uncovers the most robust self-report predictors of relationship quality across 43 longitudinal couples studies. Proc Natl Acad Sci. (2020) 117:19061–71. doi: 10.1073/pnas.1917036117
64. Salganik MJ, Lundberg I, Kindel AT, Ahearn CE, Al-Ghoneim K, Almaatouq A, et al. Measuring the predictability of life outcomes with a scientific mass collaboration. Proc Natl Acad Sci. (2020) 117:8398–403.
65. Santangelo PS, Holtmann J, Hosoya G, Bohus M, Kockler TD, Koudela-Hamila S, et al. Within-and between-persons effects of self-esteem and affective state as antecedents and consequences of dysfunctional behaviors in the everyday lives of patients with borderline personality disorder. Clin Psychol Sci. (2020) 8:428–49. doi: 10.1177/2167702620901724
66. Jacobson NC, Evey KJ, Wright AGC, Newman MG. Integration of discrete and global building blocks of affect: specific affect within-persons and global affect between-persons. (2020). doi: 10.31234/osf.io/gb5up
67. Zilcha-Mano S, Muran JC, Eubanks CF, Safran JD, Winston A. Not just a non-specific factor: Moderators of the effect of within-and between-clients alliance on outcome in CBT. Cogn Ther Res. (2018) 42:146–58. doi: 10.1007/s10608-017-9866-5
68. Mansell W, Huddy V. Why do we need computational models of psychological change and recovery, and how should they be designed and tested? Front Psychiatry. (2020) 11:624. doi: 10.3389/fpsyt.2020.00624
69. Brady RO Jr, Gonsalvez I, Lee I, Öngür D, Seidman LJ, Schmahmann JD, et al. Cerebellar-prefrontal network connectivity and negative symptoms in schizophrenia. Am J Psychiatry. (2019) 176:512–20. doi: 10.1176/appi.ajp.2018.18040429
70. Hollon SD. Moderation, mediation, and moderated mediation. World Psychiatry. (2019) 18:288. doi: 10.1002/wps.20665
71. Webb TL, Miles E, Sheeran P. Dealing with feeling: a meta-analysis of the effectiveness of strategies derived from the process model of emotion regulation. Psychol Bull. (2012) 138:775. doi: 10.1037/a0027600
Keywords: personalized treatment, mechanisms of change, process of change, between-individual effects, within-individual effect, State-like, Trait-like
Citation: Zilcha-Mano S and Webb CA (2021) Disentangling Trait-Like Between-Individual vs. State-Like Within-Individual Effects in Studying the Mechanisms of Change in CBT. Front. Psychiatry 11:609585. doi: 10.3389/fpsyt.2020.609585
Received: 23 September 2020; Accepted: 11 December 2020;
Published: 21 January 2021.
Edited by:
Nikolaos Kazantzis, Institute for Social Neuroscience Psychology, AustraliaReviewed by:
Warren Mansell, The University of Manchester, United KingdomKate Bentley, Massachusetts General Hospital and Harvard Medical School, United States
Copyright © 2021 Zilcha-Mano and Webb. This is an open-access article distributed under the terms of the Creative Commons Attribution License (CC BY). The use, distribution or reproduction in other forums is permitted, provided the original author(s) and the copyright owner(s) are credited and that the original publication in this journal is cited, in accordance with accepted academic practice. No use, distribution or reproduction is permitted which does not comply with these terms.
*Correspondence: Sigal Zilcha-Mano, sigalzil@gmail.com