- Department of Microbiology and Biotechnology, Sister Nivedita University, Kolkata, West Bengal, India
The integration of mathematical modelling in different scientific domains has increased dramatically in recent years. In general, modelling involves using programming languages, manipulating matrices, designing algorithms, and tracking functions and data to gain new insights and more quantitative and qualitative information about systems. These strategies have motivated researchers to investigate numerous approaches to accurately solve a variety of problems. In this direction, modelling and simulation have been used to create sensitive and focused detection methods for a variety of applications, including environmental control. New pollutants, including pesticides, heavy metals, and medications, are endangering wildlife by poisoning water supplies. As a result, numerous biosensors that use modelling for effective environmental monitoring have been documented in the literature. The most current model-inspired biosensors used for environmental monitoring will be discussed in this review study. Additionally, each analytical biosensor’s capabilities and degree of success will be discussed. Finally, present difficulties in this area will be highlighted.
1 Introduction
Due to the harmful impacts of pollutants on ecosystems and human health, environmental monitoring is one of the global issues that is becoming more and more of a worry (Justino et al., 2015). Unfortunately, the majority of these pollutants have a long half-life, high toxicity, and are non-biodegradable, which increases the risk to living things by causing bioaccumulation (Bonanno and Giudice, 2010; Teniou et al., 2021). Therefore, mitigating the harmful impacts of these toxins requires constant environmental monitoring. For the past few decades, heavy metals have been a significant contributor to environmental degradation (Majumdar et al., 2008). The food chain incorporates heavy metals, which then participate in bioaccumulation and biomagnification (Volesky and Naja, 2007). Long-term exposure to the heavy metals can result in cancer, organ failure, nervous system impairment, and, in severe cases, even death. Therefore, the bulk of environmental protection authorities worldwide are quite concerned with heavy metal removal (Abdel Baki et al., 2011). According to reports, the biological elimination of heavy metals is a safe, effective, and risk-free method. The approach can be used to treat drinking water since it is more effective at removing heavy metals from diluted solutions than other methods. Additionally, biosorbents are easily regenerative and versatile (Wang and Chen, 2009).
Over the past 10 years, artificial intelligence (AI) has advanced significantly in a wide range of applications. It has been applied to address challenging, non-linear, and dynamic issues. Additionally, modelling, prediction, simulation, and optimization at high speed is thought of as an alternative to conventional techniques or as a part of integrated systems (Teniou et al., 2021). Analytical chemistry uses chemometric procedures frequently, particularly in environmental investigations, demonstrating the effectiveness of data processing methods in this area. Chemometric approaches are one of the AI-based advanced analysis techniques that are frequently employed in analytical chemistry, particularly in environmental investigations, demonstrating the effectiveness of data processing methods in this area.
The main areas of interest in chemometric environmental studies are quantitative chemical analysis, environmental quality assessment monitoring, modelling, and the prediction of toxicological consequences (Gros et al., 2006). Chemometric techniques are widely employed in environmental monitoring because they make it possible to identify and describe how different environmental elements interact. They also make clear how these factors might affect the environment (Chapman et al., 2020). Analysis by Principal Components PCA provides the foundation for many other chemometric techniques and is thought to be the most effective and well-liked one. This strategy is an exploratory data analysis using a multivariate statistical method (Teniou et al., 2021).
2 Emerging contaminants and sources
In recent years, it has become well known that wastewater, groundwater, and surface waters can contain emerging contaminants (ECs), such as pesticides, personal care products, x-ray contrast media, endocrine disruptors, and medications (Naidu et al., 2016; Wilson and Aqeel-Ashraf, 2018; Rasheed et al., 2019; Keerthanan et al., 2021). Disruptive endocrine chemicals (EDCs) are one class of EC that has drawn a lot of attention recently. EDCs are “an exogenous (non-natural) chemical, or a mixture of chemicals, that interferes with any aspect of hormone action,” according to the Endocrine Society. These substances alter the body’s hormonal balance through a variety of mechanisms, including hormone production disruption, hormone mimicry, hormone receptor development, hormone antagonist activity, and hormone binding modification.
EDCs are a diverse class of molecules known as “endocrine disruptors” that have been shown to have physiological activity. Through a variety of pathways, including point sources (including municipal sewage, industrial wastewaters, and landfills) and non-point sources (such as landfills), EDCs accumulate in the environment, particularly in waters (for example, agricultural runoff and underground contamination). There have been reports of ECs in wastewater, groundwater, and surface water. Only a small number have, however, been found in the natural world. Volatilization, photolysis, biodegradation, sorption, or a combined action of these processes usually results in a higher concentration of these substances at the outflow of wastewater and sewage treatment plants.
When measured in surface waters, on the other hand, it is usually lower because of volatilization, sorption, biodegradation, photolysis, or a combined action of these processes (Gurr and Reinhard, 2006). whereas effluents from wastewater treatment plants are typically discharged into surface waters. The amplitudes and concentration levels measured in surface waters are generally much higher than in groundwaters since residence times for surface water are shorter than groundwater (Barnes et al., 2008). The characteristics of the substance present are influenced by the source of the ECs. Additionally, physicochemical properties and environmental factors like altitude, latitude, organic matter content, pH, polarity, precipitation, water solubility, temperature, and volatility affect the transport and conversion of ECs. When estimating an EC’s lifespan in the environment, these factors must be taken into account. There are many different EC sources, both in terms of quantity and type. The two main categories are point and diffuse pollution sources (Lapworth et al., 2012).
According to Edelstein and Ben-Hur (2018), heavy metals (HMs) are a class of metals and metalloids distinguished by their high density (greater than 4,000 kg/m3) and high toxicity, even at low concentrations. This group of emerging pollutants includes cadmium (Cd), lead (Pb), mercury (Hg), and arsenic (As). Other trace elements that are regarded as heavy metals include copper (Cu), selenium (Se), and zinc (Zn) (Pandey and Madhuri, 2014). The majority of heavy metals are created during industrial activities such as automobiles, electroplating, industrial processing, mining, organic chemicals, pharmaceuticals, and other industrial wastewater processes (Huang et al., 2007).
By binding to particular proteins and enzymes to form “free radicals,” heavy metals disrupt the body’s systems and inhibit the absorption of nutritional minerals by competing with them. Therefore, HMs have the ability to change certain metabolic processes, cellular processes, and other substances that are vital to preserving the equilibrium of the organism (Sharaf, 2019). Heavy metals are mutagenic, carcinogenic, and immunosuppressive when exposed repeatedly. The kidneys, reproductive, nervous, and immune systems are all impacted by high levels of Pb (Cîrtînă et al., 2019). Heavy metal pollution is a serious issue for farming soil pollution and product quality because it causes plants to grow in ways that are not natural (Edelstein and Ben-Hur, 2018).
It has been demonstrated that oxidative stress and reactive oxygen species are mainly produced by the high concentrations of Cu in plants (Filetti et al., 2018). A significant rate of Pb in soil has also been associated with the altered morphology of different plant species (Kushwaha et al., 2018). Liberation of heavy metals into aquatic systems may result in various physical, chemical, and biological processes (Guo et al., 2018). For example, shifts in physical conditions could affect the pH of the water, the organic content of the substrate, and the size of the water’s particles, which would have an impact on plants by lowering species diversity and densities (Teniou et al., 2021).
3 Source of heavy metals
Heavy metals can be found in our environment from a number of different sources, including 1) household waste; 2) industrial sources; 3) agricultural sources; 4) power plants; 5) electronic waste; 6) mining; 7) natural resources; and 8) other resources. In Scheme 1, the sources of heavy metals are represented schematically. Many of the categories discussed below may be debatable when it comes to separating the source and treatment of heavy metal pollution; however, some categories, like the remains of manufacturing facilities and mines, could undoubtedly be combined within a larger industrial section. Therefore, even though they are part of a larger whole, we do want to emphasize that we have tried to address each issue in a specific way.
3.1 Simulation-based approach towards treatment of emerging contaminants
3.1.1 Heavy metal analysis based on chemometrics
In order to obtain reliable results on the presence of HMs in soil and food, research in these areas requires not only cutting-edge analytical techniques with high levels of sensitivity, specificity, and accuracy but also sophisticated statistical techniques that give an overall picture of the problem at hand. For some matrices of complex data, multivariate statistical techniques are the best tool for viewing and analysis. We can infer how specific variables (metal concentration, other soil or plant parameters) that characterize objects (soil, plants) determine their association by using Principal Component Analysis (PCA) and cluster analysis (CA), two unsupervised methods. The PCA method estimates the correlation structure of the variables by identifying hypothetical new variables (principal components, PC) that account for as much as possible of the variance (or correlation) in a multidimensional data set if the CA method is used for sample grouping the original variables.
The original variables have been linearly combined to create these new variables (Nayik and Nanda, 2016). With the aid of this technique, we can group samples (soil or vegetable species) and variables (such as heavy metal concentrations or even other soil or plant parameters) based on loadings and scores. The chemometric technique PCA was used to comprehend the intricate relationship between soil or plant samples and the contents of heavy metals. It is founded on the covariance or correlation matrix’s eigen analysis. Each variable has a loading that indicates how well the model components are taking that variable into account. They serve to interpret the relationship between the variables by demonstrating how significantly each variable contributes to the meaningful variation (or correlation) in the data.
The size of a variable’s residual variance in a PC model serves as an indicator for that variable’s significance. This is helpful for the variable selection process because it allows the removal of a variable with very little explained variance without making further adjustments to the PC model. The rule for multiple regressions that the number of variables must be less than the number of objects does not apply in PCA cases, so there is no restriction on the number of variables. PCA can be used to reduce the data set to only two variables in order to simplify plotting (the first two components).
Chemometrics has developed into a crucial tool for sample classification and pollution source identification through the use of various environmental techniques. The application can reveal intricate relationships in the field of environmental science and lower the sample collection and analysis costs (Chuan et al., 2022). In order to further clarify the source of metals, the raw data for sediment particle size, percentage of total organic carbon, and metal concentration were computed using principal component analysis. A method for reducing the multivariate problem dimensions is Principal Component Analysis (PCA) (Iwamori et al., 2017). By removing the data dimensions, this analysis defines the correlation of the variable’s principal components (PCs), which is very similar to the correlation or regression analysis method. Due to its significant reduction in the number of variables and ability to identify structure in the relationships between various variables, PCA is frequently used by researchers in a variety of fields. The following equation can be used to generate the PCs for variables:
Analytical chemistry uses chemometric approaches frequently, particularly in environmental studies, demonstrating the effectiveness of data processing methods in this area. The main areas of interest in chemometric approaches are modelling, quantitative chemical analysis, prediction of toxicological effects, and environmental quality assessment monitoring (Otto, 2016; Teniou et al., 2021). Chemometric techniques are widely used in environmental monitoring because they make it possible to identify and describe how different environmental factors interact. They also make clear how these factors might affect the environment (Chapman et al., 2020).
To gain a deeper understanding of trace metal patterns, a chemometric approach was often applied. Both inductively coupled plasma atomic emission spectrometry and flame atomic absorption and emission spectrometry were used to examine the concentrations of Cu, Zn, Mn, Fe, K, Ca, Mg, Al, Ba, and B in 26 herbal drugs. A PCA was then used to emphasize the connection between the elements. Four important factors were found, and some of them were attributed to important influencing sources and the high mobility of some elements, which also alluded to possible anthropogenic contamination. Chemometric techniques come in a variety of forms, including cluster analysis (CA), principal component analysis (PCA), linear discriminant analysis (LDA), partial least squares regression (PLSR), random forest (RF), etc. These methods are classified as either supervised or unsupervised (Teniou et al., 2021).
To rapidly categorize freshwater bacteria that have been exposed to heavy metals, chemometric approaches are also used. Chemometric analysis was used by Sajdak and Pieszko (2012) to determine the concentration of heavy metals like Cd, Cu, Fe, Mn, Ni, Pb, and Zn. The relationship between element quantity, matrix type, and sampling location has been identified based on the concentration assessment, and it has been confirmed and described that definite element group co-existence exists. Kurniawan et al., 2022 reported biosorption of heavy metals from aqueous solutions using Aeromasss hydrophyla, and Branhamella spp. based on modelling with GEOCHEM.
3.1.2 Environmental monitoring based on artificial neural networks (ANNs)
A neuron is a type of cell that uses biochemical processes to receive, manage, process, and transmit information. More than 10 billion interconnected neurons make up the human brain’s network (Teniou et al., 2021). Artificial neural networks (ANNs) were first developed as computational models of the nervous system. Neural networks were created as a result of McCulloh and Pitts’ initial use of simplified neurons (Teniou et al., 2021). ANNs are computer programs with biological influences that try to imitate how the human brain processes information. According to Hassani et al. (2016), they are viewed as a potential modelling technique, particularly for data sets with complex non-linear relationships between dependent and independent variables.
In contrast to other techniques that perform specific tasks through simple computer implementation (Zurada, 1992), ANNs are trained through the use of experience-based knowledge as inputs. Following training, new patterns can be applied directly to specific tasks like prediction and classification without the need for programming. Although a single neuron is capable of carrying out basic information processing, the main benefit of ANNs, which makes them a potent computational tool, is the ability to connect neurons in a network. ANNs are easily able to recognize a wide range of input patterns due to the billion connections between neurons (Zurada, 1992).
ANN systems enable high-quality, quick, and high-capacity detection, claim Ferentinos et al. (2012). They have also been used as a potential technique for tracking and rating environmental pollution (Ferentinos et al., 2012). A single layer of an ANN can result in a simple neural network, while multiple layers can result in a multilayer neural network (MNN). The multilayer neural network is primarily used for more complex processes, while the simple neural network is particularly well-suited for straightforward issues (Figure 1).
According to the following expression, the mean square error (MSE) as a percentage between the predicted and measured values was used to assess the neural network prediction performance:
Environmental monitoring is very difficult when it comes to heavy metal biosensing. Environmental monitoring is greatly hampered by the difficulty of heavy metal biosensing. The classification of soil, pollutant infiltration properties, organic content and macronutrient measurements, and soil contamination prediction have all been studied using ANNs (El Tabach et al., 2007; Wilson et al., 2015; Emamgholizadeh et al., 2017; De Souza et al., 2020; Teniou et al., 2021). Anagu et al., 2009 developed a sorption model for estimating heavy metal sorption from fundamental soil properties and assessing the dangers associated with their appearance. Nine heavy metals have been studied in this work: Cd, Cr, Cu, Mo, Ni, Pb, Sb, and Tl (Table 1).
The ANN models performed well, with modelling efficiency (EF) ranging from 0.79 (Cr) to 0.94 and a root mean square error (RMS) of 0.04 g/kg (Cd) to 0.1 g/kg (Cr) (Cd, Zn). Electrochemical biosensing platforms that use different types of electrodes have also been combined with ANNs for HM detection in the environment. For instance, a polyvinyl chloride (PVC) membrane-based electronic tongue approach has been used in the quick and easy on-site monitoring of a number of heavy metals (Mimendia et al., 2010a; Mimendia et al., 2010b). In this case, an artificial tongue (ANNs) combined with a membrane-selective electrode has been used to resolve a mixture of three heavy metals (Pb2+, Cd2+, and Cu2+). For the three targets, a limit of detection of ∼1 mg/L was attained with good reproducibility.
Furthermore, the correlation for the three ions exceeded 0.975 and was significant. Finally, when used on contaminated soil sample analysis, this system demonstrated good prediction capability. Even though the results are intriguing, this strategy is only modified for three HMs. As a result, another team used the same potentiometric e-tongue and PVC membranes using ANNs to investigate the simultaneous detection of a mixture of four HMs. With low root mean squared error values of 1 mmol/L, the method was successfully used to quantify low levels of Cu2+, Pb2+, Zn2+, and Cd2+ ions from quaternary mixtures in rivers, open-air waste streams, and soil. Talib et al., 2019 reported the Cr removal efficiency of Acinetobacter radioresistens and the application of Artificial Neural Networks (ANNs) and Response Surface Methodology (RSM) for modelling the optimization of Cr (VI) removal from agricultural soil. Altowayti et al., 2020 reported the potential of mixed dried biomass of three bacterial strains Bacillus thuringiensis strain WS3, Pseudomonas stutzeri strain WS9 and Micrococcus yunnanensis strain WS11 in the removal of As (V) and As(III) and optimization of process parameters using ANN model. Artificial neural networks (ANN) were conducted by Elsayed et al., 2022 for modelling the biosorption of Co(II) by Pseudomonas alcaliphilaNEWG-2.
Other electrodes besides PVC membranes were used in ANN-based sensing. The simultaneous detection of EP mixtures in the environment at the ultra-trace level appears to have been successfully accomplished using electrochemical biosensing technologies based on ANNs. Additionally, these systems offer a number of benefits like high specificity, quick response times, portability, ease of use, and low cost, making them trustworthy EP monitoring devices (Hernandez-Vargas et al., 2018; Honeychurch and Piano, 2018).
3.1.3 Heavy metal removal from industrial wastewater using a response surface methodological approach
Numerous conventional methods, including chemical precipitation, electrochemical treatment, electrodialysis, ion exchange, membrane filtration, reverse osmosis, and adsorption, have been used to remove heavy metal ions from wastewater. The adsorption method is superior to the others due to its flexibility in design, ease of operation, and ease of handling. It is also thought to be more efficient and cost-effective (EPA, 1990; Ince and Ince, 2019). However, the majority of these methods have high operational costs for the treatment process as well as high capital costs.
Since the adsorption process’ dynamic characteristics are intricate, ideal working conditions are necessary to achieve the highest level of pollution removal effectiveness. To achieve the best obtained response level, which is the value of the design parameters, process optimization is essential. One of the most popular techniques is the response surface methodology (RSM), which develops, enhances, and optimizes processes, particularly when there are complex interactions present. It is employed to identify the optimum points of independent variables that function optimally and to assess how these variables interact (Wantala et al., 2012).
The fact that fewer experimental trials are needed to interpret multiple parameters is its biggest benefit. Because of this, the RSM optimization process consists of three main steps: 1) choosing an appropriate experimental design; 2) estimating the model coefficients using analysis of variance (ANOVA); and 3) validating the final model based on experimental runs and predictions (Myers et al., 2009; Ince and Ince, 2019). The ability to track and interpret interactions between variables as well as describe the overall impact of the parameters on the process makes this experimental design method for an adsorption process more useful than alternative methods.
The relationship between the response surface and the independent variables is typically unknown in RSM analyses (Suliman et al., 2017). As a result, one of the initial steps in RSM involves locating an appropriate approximation for the true and efficient relationship that exists between the response variable and a set of independent variables. The central composite design is the response surface design that many researchers favour over other designs. The response surface designs result in a good fit of the model to the data, give enough details to test for a lack of fit, and provide an estimate of the error due to pure experimentation. The experiment can be carried out in segments thanks to the design, which reduces costs.
The effects of the control variables on the relevant outcome variable are also described using linear, quadratic, or polynomial functions. Utilizing particular measures and independent variables supplied by the user, certain computer packages, including R, Design-Expert, and JMP, are available and offer optimal designs (Montgomery, 2017; Suliman et al., 2017). Regarding the number of design points and blocks as well as the experimental runs selected, each design is distinct. The model is established during data collection, and the model’s coefficients are hypothesized.
The RSM has been implemented effectively, and its most significant uses have been in industrial research (Prabhakaran et al., 2010). According to Jain et al. (2011), there have been numerous studies, and various adsorbents have yielded different results. Using chemically treated Helianthus annuus flowers, they investigated the removal of Cr (VI) from aqueous solutions by using the Box-Behnken model and a combined RSM approach. Esmaeili and Khoshnevisan (2016) used an alginate-coated chitosan nanoparticle to remove heavy metals from industrial effluents (Esmaeili and Khoshnevisan, 2016). An RSM approach was taken in order to carry out the optimization of the process of using biomass for the removal of heavy metals from synthetic and industrial effluents that contained nickel (Table 1).
With an RSM-based Box-Behnken experimental strategy, Ighalo and Eletta (2020) optimized the loading of Zn (II) and Pb (II) onto the scales of Micropogonias undulatus. The operating factors (Ni (II) initial concentration, adsorbent dose, and solution pH) affecting Ni (II) removal by dried Bacillus cereus were analyzed using the RSM based on CCD (Zhang et al., 2016). In order to optimize the biosorption process for the removal of Zn (II), Ni (II), and Cr (VI) ions by immobilized bacterial biomass sp. Bacillus brevis, Kumar et al. (2009) used the response surface methodology approach. Afraz et al., 2021, reported the potential of Lactobacillus acidophilus for biosorption of Pb2+ and Cd2+ and the design of experiment RSM was interpreted to acquire the optimum condition for the independent operational factors affecting the process. A statistical design of experiments was used by Banerjee et al. (2016a) to optimize the As (v) biosorption by immobilized bacterial biomass. Ibrahim et al., 2022 studied the biosorption of heavy metals by Alcaligenes faecalis strain isolated from soil based on optimization and simulation of process parameters using RSM. Afolabi et al., 2021 applied response surface methodology for the removal of heavy metals using biosorbent. Their investigation contributed to the search for environment-friendly and sustainable explanations towards heavy metal-contaminated (Cu2+ and Pb2+) water bodies. The bacterial mechanism of biosorption is shown in Figure 2. The application of different simulation based approach towards removal of emerging contaminants with special reference to heavy metals has been shown in Figure 3.
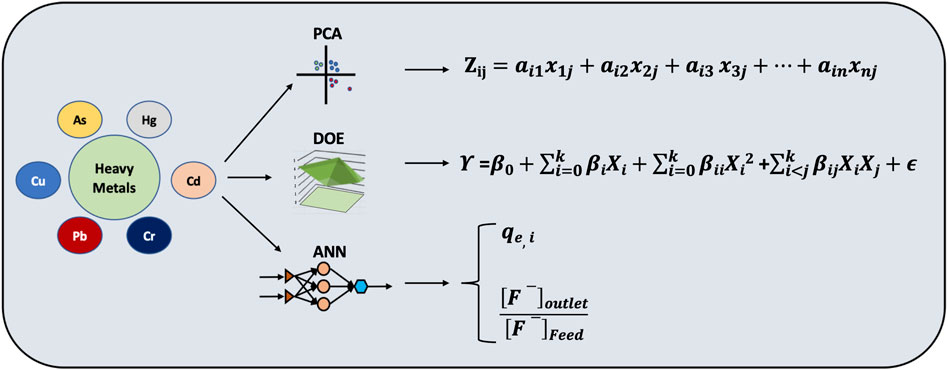
FIGURE 3. Illustration showing the application of ANN, RSM and PCA in the removal of emerging contaminants (heavy metals) from wastewater.
Plackett-Burman and Response Surface Methodological Approaches were used by Reddy et al. (2008) to optimize alkaline protease production using batch cultures of Bacillus sp. RKY3. Using a statistical design of experiments based on RSM and BBD, Banerjee et al. (2016b) optimized the maximum arsenate reductase production by Kocuria palustris (RJB-6) and immobilization parameters in polymer beads. Using RSM modelling, Djinni and Djoudi, 2022 showed enhanced Cu (II) removal by Streptomyces sp. WR1L1S8, a powerful endophytic marine strain.
In addition to allowing for the effective estimation of the linear and quadratic terms of the model, Box and Behnken (Box and Behnken, 1960) proposed a method for choosing design points from the three-level factorial arrangement. Because of the large number of factors, the designs are essentially more effective and economical than their corresponding 3K designs. The BBD for three-factor optimization with 13 experimental points is also shown. The analysis of variance (ANOVA) is used to analyze the outcomes of the experimental trial runs, and the second-order polynomial model (Eq. 1) is used to fit the observations:
Where Y is the anticipated response, or the biomass’s ability to absorb pollutants, b0 is the constant coefficient, bi is the ith linear coefficient of input factor xi, bii is the ith quadratic coefficient of input factor xii, and bij is the various interaction coefficient between input factors xi and xj. Fisher’s F-test for the analysis of variance (ANOVA) is used to determine the interactions between the process variables and response in order to assess the statistical appropriateness and significance of the model. The second order polynomial model equation’s quality of fit is shown by the correlation coefficient (R2) and adjusted R2. The three-dimensional contour plots are used to illustrate the relationship between the experimental levels of each parameter and the resultant response from the model (Banerjee et al., 2016b).
According to Ghoniem et al., 2020, the Box-Behnken design matrix controlled the interactions between the independent operational factors of initial concentration of Cu2+, initial culture pH, and incubation times in the culture medium growth to achieve the highest copper removal percentage by Azotobacter nigricans NEWG-1. The data show variations in the percentage of Cu2+ removed, which ranged from 12.12% to 80.56%. These variations suggested that the optimization process was crucial for maximizing A. nigricans NEWG-1’s ability to remove copper. The data also demonstrate the greatest removal of Cu2+ (80.56%).
4 Conclusion and future perspective
Heavy metals, drugs, biotoxins, and pesticides are examples of environmental pollutants that are extremely dangerous for all different aspects of being an organism, which would include health, food, energy, etc. Traditional monitoring methods for these contaminants are constrained by their poor effectiveness, high cost, and time-intensive nature. In order to model environmental monitoring, this paper reviewed the key applications and recent developments in sensing strategies incorporating artificial intelligence. AI biosensors are thought to be an effective analytical tool for quickly and accurately identifying one or more pollutants in challenging samples.
For in situ operations and analytical performance, there are a few cutting-edge artificial intelligence for environmental monitoring based on chemometrics, multivariate process optimization based on artificial neural networks (ANN), and response surface methodology (RSM). Therefore, creating more sophisticated and sensitive AI tools to find pollutants presents a new challenge. And finally, AI biosensing will offer a fresh foundation for upcoming innovation. Additionally, significant work is needed to design dependable and durable tools that will improve pollutant detection.
Author contributions
SS acquired resources and prepared figures. SS and AB developed the concept. AB and SS wrote the manuscript with the help of AG. AB and SS revised, edited and formatted the manuscript. All authors contributed to the article and approved the submitted version.
Acknowledgments
The authors would like to thank Sister Nivedita University for providing the Article Processing Fee as financial support. The authors also thank the reviewers for their contribution.
Conflict of interest
The authors declare that the research was conducted in the absence of any commercial or financial relationships that could be construed as a potential conflict of interest.
Publisher’s note
All claims expressed in this article are solely those of the authors and do not necessarily represent those of their affiliated organizations, or those of the publisher, the editors and the reviewers. Any product that may be evaluated in this article, or claim that may be made by its manufacturer, is not guaranteed or endorsed by the publisher.
References
Abdel-Baki, A. S., Dkhil, M. A., and Al-Quraishy, S. African bioaccumulation of some heavy metals in tilapia fish relevant to their concentration in water and sediment of Wadi Hanifah. J. Biotechnol. (2011) 10:2541–2547. doi:10.5897/AJB10.1772
Afolabi, F. O., Musonge, P., and Bakare, B. F. Application of the response surface methodology in the removal of Cu2+ and Pb2+ from aqueous solutions using orange peels. Sci. Afr. (2021) 13:e00931. doi:10.1016/j.sciaf.2021.e00931
Afraz, V., Younesi, H., Bolandi, M., and Hadiani, M. R. Assessment of resistance and biosorption ability ofLactobacillus paracaseito remove lead and cadmium from aqueous solution. Water Environ. Res. (2021) 93:1589–1599. doi:10.1002/wer.1540
Altowayti, W. A. H., Haris, S. A., Almoalemi, H., Shahir, S., Zakaria, Z., and Ibrahim, S. The removal of arsenic species from aqueous solution by indigenous microbes: Batch bioadsorption and artificial neural network model. Environ. Technol. Innovation (2020) 19:100830. doi:10.1016/j.eti.2020.100830
Anagu, I., Ingwersen, J., Utermann, J., and Streck, T. Estimation of heavy metal sorption in German soils using Artificial neural networks. Geoderma (2009) 152:104–112. doi:10.1016/j.geoderma.2009.06.004
Ariza-Avidad, M., Cuellar, M., Salinas-Castillo, A., Pegalajar, M., Vukovi´c, J., and Capitan-Vallvey, L. Feasibility of the use of disposable optical tongue based on neural networks for heavy metal identification and determination. Anal. Chim. Acta. (2013) 783:56–64. doi:10.1016/j.aca.2013.04.035
Banerjee, A., Sarkar, P., and Banerjee, S. Application of statistical design of experiments for optimization of as (v) biosorption by immobilized bacterial biomass. Ecol. Eng. (2016a) 86:13–23. doi:10.1016/j.ecoleng.2015.10.015
Banerjee, A., Banerjee, S., and Sarkar, P. Statistical design of experiments for optimization of arsenate reductase production by Kocuria palustris (RJB-6) and immobilization parameters in polymer beads. RSC Adv. (2016b) 6:49289–49297. doi:10.1039/c6ra00030d
Barnes, K. K., Kolpin, D. W., Furlong, E. T., Zaugg, S. D., Meyer, M. T., and Barber, L. B. A national reconnaissance of pharmaceuticals and other organic wastewater contaminants in the United States — I) groundwater. Sci. Total Environ. (2008) 402:192–200. doi:10.1016/j.scitotenv.2008.04.028
Bhateria, R., and Renu, D. Optimization and statistical modelling of cadmium biosorption process in aqueous medium by Aspergillus niger using response surface methodology and principal component analysis. Ecol. Eng. (2019) 135:127–138. doi:10.1016/j.ecoleng.2019.05.010
Boeris, P. S., Liffourrena, A. S., and Lucchesi, G. I. Aluminum biosorption using non-viable biomass of Pseudomonas putida immobilized in agar–agar: Performance in batch and in fixed-bed column. Environ. Technol. &Innovation (2018) 11:105–115. doi:10.1016/j.eti.2018.05.003
Bonanno, G., and Giudice, R. L. Heavy metal bioaccumulation by the organs of Phragmites australis (common reed) and their potential use as contamination indicators. Ecol. Indic. (2010) 10:639–645. doi:10.1016/j.ecolind.2009.11.002
Box, G. E. P., and Behnken, D. W. Some new three level designs for the study of quantitative variables. Technometrics (1960) 2:455–475. doi:10.1080/00401706.1960.10489912
Cai, C. X., Xu, J., Deng, N. F., Dong, X. W., Tang, H., Liang, Y., et al. A novel approach of utilization of the fungal conidia biomass to remove heavy metals from the aqueous solution through immobilization. Sci. Rep. (2013) 6:36546. doi:10.1038/srep36546
Chapman, J., Truong, V. K., Elbourne, A., Gangadoo, S., Cheeseman, S., Rajapaksha, P., et al. Combining chemometrics and sensors: Toward new applications in monitoring and environmental analysis. Chem. Rev. (2020) 120:6048–6069. doi:10.1021/acs.chemrev.9b00616
Chuan, O. M., Chuen, Y. J., Shaari, H., Bidai, J., Shazili, N. A. M., Pradit, S., et al. The application of chemometrics in metals source of identification in Brunei Bay surface sediment. Environ. Geochem Health (2022). doi:10.21203/rs.3.rs-2053109/v1
Cîrtînă, D., Mecu, R., and Nănescu, V. The considerations relating to the effects of toxic substances from the environment on the organism. Romania: Constantin Brancusi” University of Targu Jiu: Targiu Jiu (2019).
Cui, L., Meng, Q., Bi, H., Zhou, L., and Ye, Z. Simultaneous removal of Pb(II) and chemical oxygen demand from aqueous solution using immobilized microorganisms on polyurethane foam carrier. Korean J. Chem. Eng. (2016) 30:1729–1734. doi:10.1007/s11814-013-0095-7
De Souza, W. M., Ribeiro, A. J., and Da Silva, C. A. Use of ANN and visual-manual classification for prediction of soil properties for paving purposes. Int. J. Pavement Eng. (2020) 23:1482–1490. doi:10.1080/10298436.2020.1807546
Djinni, I., and Djoudi, W. Streptomyces sp. WR1L1S8 a potent endophytic marine strain for heavy metal resistance and copper removal enhanced by RSM modeling. Acta Ecol. Sin. (2022) 42:80–89. doi:10.1016/j.chnaes.2021.04.004
Edelstein, M., and Ben-Hur, M. Heavy metals and metalloids: Sources, risks and strategies to reduce their accumulation in horticultural crops. Sci. Hortic. (2018) 234:431–444. doi:10.1016/j.scienta.2017.12.039
El Tabach, E., Lancelot, L., Shahrour, I., and Najjar, Y. Use of artificial neural network simulation metamodelling to assess groundwater contamination in a road project. Math. Comput. Model. (2007) 45:766–776. doi:10.1016/j.mcm.2006.07.020
Elsayed, A., Moussa, Z., Alrdahe, S. S., Alharbi, M. M., Ghoniem, A. A., El-Khateeb, A. Y., et al. Optimization of heavy metals biosorption via artificial neural network: A case study of cobalt (II) sorption by Pseudomonas alcaliphila NEWG-2. Front. Microbiol. (2022) 13:893603. doi:10.3389/fmicb.2022.893603
Emamgholizadeh, S., Shahsavani, S., and Eslami, M. A. Comparison of artificial neural networks, geographically weighted regression and Cokriging methods for predicting the spatial distribution of soil macronutrients (N, P, and K). Chin. Geogr. Sci. (2017) 27:747–759. doi:10.1007/s11769-017-0906-6
Environmental Protection Agency (EPA). Environmental pollution control alternatives. Cincinnati, US: Environmental Protection Agency (1990).EPA/625/5-90/025, EPA/625/4-89/023.
Esmaeili, A., and Khoshnevisan, N. Optimization of process parameters for removal of heavy metals by biomass of Cu and Co-doped alginate-coated chitosan nanoparticles. Biores Technol. (2016) 218:650–658. doi:10.1016/j.biortech.2016.07.005
Ferentinos, K., Yialouris, C., Blouchos, P., Moschopoulou, G., Tsourou, V., and Kintzios, S. The use of artificial neural networks as a component of a cell-based biosensor device for the detection of pesticides. Procedia Eng. (2012) 47:989–992. doi:10.1016/j.proeng.2012.09.313
Filetti, F. M., Vassallo, D. V., Fioresi, M., and Simões, M. R. Reactive oxygen species impair the excitation-contraction coupling of papillary muscles after acute exposure to a high copper concentration. Toxicol. Vitr. (2018) 51:106–113. doi:10.1016/j.tiv.2018.05.007
Ghoniem, A. A., El-Naggar, N. E. A., Saber, W. I., El-Hersh, M. S., and El-Khateeb, A. Y. Statistical modeling-approach for optimization of Cu2+ biosorption by Azotobacter nigricans NEWG-1; characterization and application of immobilized cells for metal removal. Sci. Rep. (2020) 10, 9491. doi:10.1038/s41598-020-66101-x
González-Calabuig, A., Cetó, X., and Del Valle, M. A. A voltammetric electronic tongue for the resolution of ternary nitrophenol mixtures. Sensors (2018) 18:216. doi:10.3390/s18010216
Gros, M., Petrovi´c, M., and Barceló, D. Development of a multi-residue analytical methodology based on liquid chromatography– tandem mass spectrometry (LC–MS/MS) for screening and trace level determination of pharmaceuticals in surface and wastewaters. Talanta (2006) 70:678–690. doi:10.1016/j.talanta.2006.05.024
Guo, Q., Li, N., Bing, Y., Chen, S., Zhang, Z., Chang, S., et al. Denitrifier communities impacted by heavy metal contamination in freshwater sediment. Environ. Pollut. (2018) 242:426–432. doi:10.1016/j.envpol.2018.07.020
Gurr, C. J., and Reinhard, M. Harnessing natural attenuation of pharmaceuticals and hormones in rivers. Environ. Sci. Technol. (2006) 40:2872–2876. doi:10.1021/es062677d
Hassani, S., Momtaz, S., Vakhshiteh, F., Maghsoudi, A. S., Ganjali, M. R., Norouzi, P., et al. Biosensors and their applications in detection of organophosphorus pesticides in the environment. Arch. Toxicol. (2016) 91:109–130. doi:10.1007/s00204-016-1875-8
Hernandez-Vargas, G., Sosa-Hernández, J. E., Saldarriaga-Hernandez, S., Villalba-Rodríguez, A. M., Parra-Saldivar, R., and Iqbal, H. Electrochemical biosensors: A solution to pollution detection with reference to environmental contaminants. Biosensors (2018) 8:29. doi:10.3390/bios8020029
Honeychurch, K. C., and Piano, M. Electrochemical (bio) sensors for environmental and food analyses. Biosens. (Basel). (2018) 8:57. doi:10.3390/bios8030057
Huang, S., Liao, Q., Hua, M., Wu, X., Bi, K., Yan, C., et al. Survey of heavy metal pollution and assessment of agricultural soil in Yangzhong district, Jiangsu Province, China. Chemosphere (2007) 67:2148–2155. doi:10.1016/j.chemosphere.2006.12.043
Ibrahim, U. B., Yahaya, S., Yusuf, I., and Kawo, A. H. Optimization and simulation of process parameters in biosorption of heavy metals by Alcaligenes faecalis strain UBI (MT107249) isolated from soil of local mining area in North-West Nigeria. Soil Sediment Contam. Int. J. (2022) 31:438–455. doi:10.1080/15320383.2021.1963211
Ighalo, J. O., and Eletta, O. A. A. Response surface modelling of the biosorption of Zn(II) and Pb(II) ontoMicropogonias undulatus scales: Box–Behnken experimental approach. Appl. Water Sci. (2020) 10:197. doi:10.1007/s13201-020-01283-3
Ince, M., and Ince, O. K. Heavy metal removal techniques using response surface methodology: Water/wastewater treatment. In: M. Ince, O. K. Ince, and G. Ondrasek, editors. Biochemical toxicology - heavy metals and nanomaterials. London: IntechOpen (2019). doi:10.5772/intechopen.88915
Iwamori, H., Yoshida, K., Nakamura, H., Kuwatani, T., Hamada, M., Haraguchi, S., et al. Classification of geochemical data based on multivariate statistical analyses: Complementary roles of cluster, principal component, and independent component analyses. Geochem. Geophys. Geosyst. (2017) 18:994–1012. doi:10.1002/2016gc006663
Jaafari, J., and Kamyar, Y. Optimization of heavy metal biosorption onto freshwater algae (Chlorella coloniales) using response surface methodology (RSM). Chemosphere (2019) 217:447–455. doi:10.1016/j.chemosphere.2018.10.205
Jain, M., Garg, V. K., and Kadirvelu, K. Investigation of Cr(VI) adsorption onto chemically treated Helianthus annuus: Optimization using response surface methodology. Biores Technol. (2011) 102:600–605. doi:10.1016/j.biortech.2010.08.001
Jaiswal, V., Saxena, S., Kaur, I., Dubey, P., Nand, S., Naseem, M., et al. Application of four novel fungal strains to remove arsenic from contaminated water in batch and column modes. J. Hazard. Mater. (2018) 356:98–107. doi:10.1016/j.jhazmat.2018.04.053
Justino, C. I., Freitas, A. C., Pereira, R., Duarte, A. C., and Santos, T. A. R. Recent developments in recognition elements for chemical sensors and biosensors. Trac. Trends Anal. Chem. (2015) 68:2–17. doi:10.1016/j.trac.2015.03.006
Keerthanan, S., Jayasinghe, C., Biswas, J. K., and Vithanage, M. Pharmaceutical and personal care products (PPCPs) in the environment: Plant uptake, translocation, bioaccumulation, and human health risks. Crit. Rev. Environ. Sci. Technol. (2021) 51:1221–1258. doi:10.1080/10643389.2020.1753634
Kemper, T., and Sommer, S. Estimate of heavy metal contamination in soils after a mining accident using reflectance spectroscopy. Environ. Sci. Technol. (2002) 36:2742–2747. doi:10.1021/es015747j
Kumar, R., Singh, R., Kumar, N., Bishnoi, K., and Bishnoi, N. R. Response surface methodology approach for optimization of biosorption process for removal of Cr (vi), Ni (ii) and Zn (ii) ions by immobilized bacterial biomass sp. Bacillus brevis. Chem. Eng. J. (2009) 146:401–407. doi:10.1016/j.cej.2008.06.020
Kurniawan, T. A., Lo, W., Othman, M. H. D., Goh, H. H., and Chong, K. K. Biosorption of heavy metals from aqueous solutions using activated sludge, Aeromasss hydrophyla, and Branhamella spp based on modeling with GEOCHEM. Environ. Res. (2022) 214:114070. doi:10.1016/j.envres.2022.114070
Kushwaha, A., Hans, N., Kumar, S., and Rani, R. A critical review on speciation, mobilization and toxicity of lead in soil-microbe-plant system and bioremediation strategies. Ecotoxicol. Environ. Saf. (2018) 147:1035–1045. doi:10.1016/j.ecoenv.2017.09.049
Lapworth, D. J., Baran, N., Stuart, M. E., and Ward, R. S. Emerging organic contaminants in groundwater: A review of sources, fate and occurrence. Environ. Pollut. (2012) 163:287–303. doi:10.1016/j.envpol.2011.12.034
Majumdar, S. S., Das, S. K., Saha, T., Panda, G. C., Bandyopadhyoy, T., and Guha, A. K. Adsorption behavior of copper ions on Mucor rouxii biomass, through microscopic and FTIR analysis. Colloids Surfaces B Biointerfaces (2008) 63:138–145. doi:10.1016/j.colsurfb.2007.11.022
Milojković, J., Pezo, L., Stojanović, M., Mihajlović, M., Lopičić, Z., Petrović, J., et al. Selected heavy metal biosorption by compost of Myriophyllum spicatum—A chemometric approach. Ecol. Eng. (2016) 93:112–119. doi:10.1016/j.ecoleng.2016.05.012
Mimendia, A., Gutiérrez, J., Leija, L., Hernández, P. R., Favari, L., Muñoz, R., et al. A review of the use of the potentiometric electronic tongue in the monitoring of environmental systems. Environ. Model. Softw. (2010a) 25:1023–1030. doi:10.1016/j.envsoft.2009.12.003
Mimendia, A., Legin, A., Merkoçi, A., and Del Valle, M. Use of Sequential Injection Analysis to construct a potentiometric electronic tongue: Application to the multidetermination of heavy metals. Sens. Actuators B Chem. (2010b) 146:420–426. doi:10.1016/j.snb.2009.11.027
Mortensen, J., Legin, A., Ipatov, A., Rudnitskaya, A., Vlasov, Y., and Hjuler, K. A flow injection system based on chalcogenide glass sensors for the determination of heavy metals. Anal. Chim. Acta. (2000) 403:273–277. doi:10.1016/s0003-2670(99)00544-9
Mourzina, Y. G., Schubert, J., Zander, W., Legin, A., Vlasov, Y. G., Lüth, H., et al. Development of multisensor systems based on chalcogenide thin film chemical sensors for the simultaneous multicomponent analysis of metal ions in complex solutions. Electrochim. Acta. (2001) 47:251–258. doi:10.1016/s0013-4686(01)00563-1
Myers, R. H., Montgomery, D. C., and Anderson-Cook, C. M. Response surface methodology: Process and product optimization using designed experiments. Hoboken, New Jersey: John Wiley & Sons (2009).
Naidu, R., Arias Espana, V. A., Liu, Y., and Jit, J. Emerging contaminants in the environment: Risk-based analysis for better management. Chemosphere (2016) 154:350–357. doi:10.1016/j.chemosphere.2016.03.068
Nayik, G. A., and Nanda, V. A chemometric approach to evaluate the phenolic compounds, antioxidant activity and mineral content of different unifloral honey types from Kashmir, India. LWT (2016) 74:504–513. doi:10.1016/j.lwt.2016.08.016
Otto, M. Chemometrics: Statistics and computer application in analytical chemistry. Hoboken, NJ, USA: John Wiley & Sons (2016).
Pandey, G., and Madhuri, S. Heavy metals causing toxicity in animals and fishes. Res. J. Anim. Vet. Fish. Sci. (2014) 2:17–23.
Piccirillo, C., Pereira, S. I. A., Marques, A. P. G. C., Pullar, R. C., Tobaldi, D. M., Pintado, M. E., et al. Bacteria immobilisation on hydroxyapatite surface for heavy metals removal. J. Environ. Manag. (2013) 121:87–95. doi:10.1016/j.jenvman.2013.02.036
Prabhakaran, D., Basha, C. A., Kannadasan, T., and Aravinthan, P. Removal of hydroquinone from water by electrocoagulation using flow cell and optimization by response surface methodology. J. Environ. Sci. Health, Part A. (2010) 45:400–412. doi:10.1080/10934520903540174
Rasheed, T., Bilal, M., Nabeel, F., Adeel, M., and Iqbal, H. M. N. Environmentally-related contaminants of high concern: Potential sources and analytical modalities for detection, quantification, and treatment. Environ. Int. (2019) 122:52–66. doi:10.1016/j.envint.2018.11.038
Reddy, L. V. A., Wee, Y. J., Yun, J. S., and Ryu, H. W. Optimization of alkaline protease production by batch culture of Bacillus sp. RKY3 through plackett–burman and response surface methodological approaches. Bioresour. Technol. (2008) 99:2242–2249. doi:10.1016/j.biortech.2007.05.006
Sajdak, M., and Pieszko, C. Chemometric analysis of heavy metal content for various environmental matrices in terms of their use in biomass thermal processing. Open Chem. (2012) 10:1696–1706. doi:10.2478/s11532-012-0096-0
Sharaf, S. Comparative study of heavy metals residues and histopathological alterations in large ruminants from selected areas around industrial waste drain. Pak. Veter J. (2019) 40:55–60. doi:10.29261/pakvetj/2019.111
Shi, X., Zhou, G., Liao, S., Shan, S., Wang, G., and Guo, Z. Immobilization of cadmium by immobilized Alishewanella sp. WH16-1 with alginate-lotus seed pods in pot experiments of Cd-contaminated paddy 1059 soil. J. Hazard. Mater. (2018) 357:431–439. doi:10.1016/j.jhazmat.2018.06.027
Suliman, R., Mitul, A. F., Mohammad, L., Djira, G., Pan, Y., and Qiao, Q. Modeling of organic solar cell using response surface methodology. Results Phys. (2017) 7:2232–2241. doi:10.1016/j.rinp.2017.04.037
Talib, N. S. R., Halmi, M. I. E., Abd Ghani, S. S., Zaidan, U. H., and Shukor, M. Y. A. Artificial neural networks (ANNs) and response surface methodology (RSM) approach for modelling the optimization of chromium (VI) reduction by newly isolated Acinetobacter radioresistens strain NS-MIE from agricultural soil. BioMed Res. Int. (2019) 2019:5785387. doi:10.1155/2019/5785387
Teniou, A., Rhouati, A., and Marty, J. L. Mathematical modelling of biosensing platforms applied for environmental monitoring. Chemosensors (2021) 9:50. doi:10.3390/chemosensors9030050
Volesky, B., and Naja, G. Biosorption technology: Starting up an enterprise. Int. J. Technol. Transf. Commer. (2007) 6:196–211. doi:10.1504/ijttc.2007.017806
Wang, J., and Chen, C. Biosorbents for heavy metals removal and their future. Biotechnol. Adv. (2009) 27:195–226. doi:10.1016/j.biotechadv.2008.11.002
Wantala, K., Khongkasem, E., Khlongkarnpanich, N., Sthiannopkao, S., and Kim, K. W. Optimization of as (V) adsorption on Fe-RH-MCM-41- immobilized GAC using box–Behnken design: Effects of pH, loadings, and initial concentrations. Appl. Geochem. (2012) 27:1027–1034. doi:10.1016/j.apgeochem.2011.11.014
Wilson, M., and Aqeel-Ashraf, M. Study of fate and transport of emergent contaminants at waste water treatment plant. Environ. Contam. Rev. (2018) 1:01–12. doi:10.26480/ecr.01.2018.01.12
Wilson, D., Alegret, S., and Del Valle, M. Simultaneous titration of ternary mixtures of Pb(II), Cd(II) and Cu(II) with potentiometric electronic tongue detection. Electroanalysis (2015) 27:336–342. doi:10.1002/elan.201400480
Yin, Y., Hu, J., and Wang, J. Removal of Sr2+, Co2+, and Cs+ from aqueous solution by immobilized Saccharomyces cerevisiae with magnetic chitosan beads. Environ. Prog. Sustain. Energy (2017) 36:989–996. doi:10.1002/ep.12531
Zhang, J., Yang, T., Wang, H., and Yang, K. Optimization of process variables by dried Bacillus cereus for biosorption of nickel(II) using response surface method. Desalination Water Treat. (2016) 57:16096–16103. doi:10.1080/19443994.2015.1091995
Zhang, W., Yin, K., Li, B., and Chen, L. A glutathione S-transferase from Proteus mirabilis involved in heavy metal resistance and its potential application in removal of Hg2⁺. J. Hazard Mater (2018) 261, 646–652. doi:10.1016/j.jhazmat.2013.08.023
Zouboulis, A. I., Matis, K. A., Loukidou, M., and Šebesta, F. Metal biosorption by PAN-immobilized fungal biomass in simulated wastewaters. Colloids Surfaces A Physicochem. Eng. Aspects (2003) 212:185–195. doi:10.1016/s0927-7757(02)00304-7
Keywords: ANN, artificial neural networks, bacteria, chemometrics, emerging contaminants (ECs), PCA, RSM
Citation: Banerjee A, Singh S and Ghosh A (2023) Detection and removal of emerging contaminants from water bodies: A statistical approach. Front. Anal. Sci. 3:1115540. doi: 10.3389/frans.2023.1115540
Received: 04 December 2022; Accepted: 16 March 2023;
Published: 04 April 2023.
Edited by:
Marcos Tascon, National University of General San Martín, ArgentinaReviewed by:
Juan Padró, YPF Tecnología S.A. (Y-TEC), ArgentinaCopyright © 2023 Banerjee, Singh and Ghosh. This is an open-access article distributed under the terms of the Creative Commons Attribution License (CC BY). The use, distribution or reproduction in other forums is permitted, provided the original author(s) and the copyright owner(s) are credited and that the original publication in this journal is cited, in accordance with accepted academic practice. No use, distribution or reproduction is permitted which does not comply with these terms.
*Correspondence: Surjit Singh, c3Vyaml0LnNAc251bml2LmFjLmlu