- 1Université Paris-Saclay, UVSQ, CNRS, LATMOS/IPSL, Guyancourt, France
- 2LEGOS, CNRS, Toulouse, France
- 3LAERO, Univ Toulouse, CNRS, Toulouse, France
- 4Jet Propulsion Laboratory, Pasadena, CA, United States
- 5NASA GSFC, Greenbelt, MD, United States
- 6GESTARII, Morgan State University, Baltimore, MD, United States
- 7CNRM, Université de Toulouse, Météo-France, CNRS, Toulouse, France
- 8Stony Brook University, Stony Brook, NY, United States
Convective clouds serve as a primary mechanism for the transfer of thermal energy, moisture, and momentum through the troposphere. Arguably, satellite observations are the only viable way to sample the convective updrafts over the oceans. Here, the potential of temporal derivatives of measurements performed in H2O lines (183GHz and 325 GHz) to infer the deep convective vertical air motions is assessed. High-resolution simulations of tropical convection are combined with radiative transfer models to explore the information content of time-derivative maps (as short as 30 s) of brightness temperatures (dTb/dt). The 183-GHz Tb signal from hydrometeors is used to detect the location of convective cores. The forward simulations suggest that within growing convective cores, the dTb/dt is related to the vertically integrated ice mass flux and that it is sensitive to the temporal evolution of microphysical properties along the life cycle of convection. In addition, the area-integrated dTb/dt, is related to the amount, size, and density of detrained ice, which are controlled by riming and aggregation process rates. These observations, particularly in conjunction with Doppler velocity measurements, can be used to refine these assumptions in ice microphysics parameterizations. Further analyses show that a spectral sampling of the 183 GHz absorbing line can be used to estimate the maximum in-cloud vertical velocity that is reached as well as its altitude with reasonable uncertainties.
Introduction
Importance of Convective Transport for Weather and Climate
Tropical convection plays a fundamental role in the climate system by transporting air, water, and momentum from the lower layers of the atmosphere to the free troposphere and has been the subject of numerous field experiments and modeling studies for decades (Houze, 2018). Despite these comprehensive efforts, observations of vertical transport in deep convection over the tropical oceans are simply not available. The lack of understanding of the convective updraft properties and their relationship to environmental factors limits our ability to represent deep convection and its feedbacks in large scale circulation models.
Efficient vertical transport occurs in deep convective cells embedded in organized meso-scale convective cloud structures (Houze and Betts, 1981; Schumacher and Rasmussen, 2020). The two-way relationship between deep convection and its large-scale environment is hence complicated owing to this intermediate agent, that both influences and is influenced by deep convection, and its environment as well. This complexity is perhaps the reason for sustained research despite half a century of dedicated efforts (Tomassini, 2021).
Vertical transport of water permits the formation of large upper level cloud decks that interact with the radiation, the thermodynamics and the dynamics of the large-scale environment in which they form. In return, the cloud mass deposited aloft can feedback onto the initial causes that triggered deep convection in the first place. In the simplest conceptual models, the cloud deck, also known as the stratiform anvil cloud, is associated with a mesoscale circulation that can perturb the surface conditions and help release instability for new deep convective cells to form and contribute to feeding the cloud decks again (Wang et al., 2020). In this simple view, deep convection is a process strongly coupled with its cloud structure. Understanding of this coupling has remained particularly stubborn to unravel despite significant progress over the last decades (Houze, 2018). In particular, the reasons for the observed duration of these convective systems are still debated. From the earlier cold pools-deep convection dynamical coupling theory (Rotunno et al., 1988), the stratiform-cold pools connection (Lafore and Moncrieff, 1989) to the role of radiation in sustaining the system duration (Roca et al., 2020; Gasparini et al., 2021) to the aerosol invigoration process (Seigel and Van Den Heever, 2013), a large suite of candidate lead-processes are at hands. What these considerations all have in common, is the need to couple the deep convection to the cloud deck through, in short, an articulated water budget of the anvil cloud (Redelsperger, 1997). The pathway from deep convection to stratiform anvil mass can be quantified with the convective mass flux. Elsaesser et al. (2021) recently proposed a simple formulation of this relationship. Noting A the surface of the cloud shield of the convective system, Ac the surface of the convective part of cloud shield, Mc the convective mass flux and τ the characteristic decay time, then the growth rate of the surface of the cloud shield of the convective system can be linked to the growth rate of the convective surface Ac and the vertical convergence of the convective mass flux and reads:
with Mc, the convective mass flux over an area that can be spelled out
where ρ is the air density, σ the surface occupied by deep convective cells and wc the areal-averaged vertical velocity over the surface σ; the major difficulty is to specify the relative contribution of σ and wc to Mc (Schubert et al., 2018). This illustrates the importance of both the knowledge of the convective surface and the convective vertical velocity to the cloud budget. While recent investigations seem to favor convective surface variability to explain the variability of cloud mass flux over that of the vertical velocity in the tropics (Feng et al., 2012; Kumar et al., 2015; Giangrande et al., 2016; Masunaga and Luo, 2016; Wang et al., 2020) strong scale dependence is also found and a thorough assessment at the global scale is much needed. Relative humidity in the troposphere also is impacted differently by deep convection with different aggregated states (Bony et al., 2020; Romps, 2021).
Observing the Dynamics of Deep Convection
The role of field campaigns in the investigation of the properties of storm and their control is unquestionable. From the GATE experiment in 1974 that targeted oceanic convection over the tropical Atlantic and its predictability, extensive multi-instrumental campaigns have been conducted. Such campaigns include (cf Houze, 2018): TOGA-COARE in 1992–1993 for the documentation on ocean/atmosphere coupling, AMMA in 2006–2007 for the study of the West African Monsoon, TWPICE in 2006 focusing on the tropical Warm Pool and the Australian Monsoon, DYNAMO in 2011–2012 that deployed over the equatorial Indian Ocean and looked at the Madden-Julian Oscillation. The tremendous deployment of active, passive and in-situ instruments during these 2-to-6 months field experiments has been extensively used to develop a better understanding on the micro- and macro-physical properties of convection. Field campaigns are major opportunities to measure vertical motion intensity using airborne instrumentation (Zipser and LeMone 1980; LeMone et al., 1998), in spite of limitations due to aircraft safety.
More recently, using profiling and scanning radars installed on well-instrumented ground-based sites, population of convective updrafts and downdrafts has been statistically characterized at different locations (May and Rajopadhyaya 1999; Ray et al., 2012; Giangrande et al., 2013, 2016; Kumar et al., 2015; North et al., 2017; Kollias et al., 2018; Ovchinnikov et al., 2019; Wang et al., 2020). These studies show that vertical velocity increases with altitude in spite of a competition between entrainment and mixing with the environment and hydrometeor loading that tends to slow the vertical velocity on the one hand and latent heat release on the other (Zipser 2003). They also demonstrate that the vertical mass flux is mainly controlled by the updraft and downdraft core width. These long time series also allow to study the sensitivity of the vertical mass flux to environmental parameters (convective inhibition - CIN, convective available potential energy—CAPE, for instance). Updraft size seems to be strongly related to large scale vertical velocity and to the CIN, whereas higher vertical velocities are observed in relatively dry conditions in the low levels (Kumar et al., 2015; Giangrande et al., 2016).
The quantitative documentation of the properties of embedded deep convection and convective mass flux would help to understand the coupling between deep convection and the associated cloud system. Some works already rely on observations with short revisit time to investigate cloud dynamics. Adler and Fenn (1979) demonstrated the use of infra-red (IR, GOES satellite) imagers and their rapid-scan modes (5min) onboard geostationary platforms to infer the cloud top vertical velocity from the decreasing rate of the IR measurements, assuming a moist adiabatic lapse rate, for a few thunderstorms. This approach was expanded to MTSAT-1R measurements by Hamada and Takayabu (2016) to study cloud top vertical velocity during the growing phase of convection. Low earth orbit (LEO) satellites have also been analyzed in a similar method by Luo et al. (2014) who used the close configuration (1–2min) of the infra-red imagers of the A-Train to study the vertical velocity of convective tops.
As noted, this approach has been applied to IR imagers, which limits the interpretations to cloud tops. In order to reach in-cloud vertical velocities, microwave radiometers and radars in LEO are the instruments to use. However, owing to their long wavelengths (relative to visible light), such instruments must be placed on LEO to achieve the necessary spatial resolution, which makes their temporal sampling quite limited. Indeed, a single LEO instrument will very rarely observe a weather system more than once during the lifetime of the system. On the rare occasion that a single instrument may revisit a storm on two consecutive orbits, the visits are nevertheless separated by the typical amount of time it takes the satellite to complete one orbit, i.e., ∼ 90 min. During this re-visit gap, the cloud will typically have undergone dramatic changes, observed only by geostationary satellites orbiting at much higher altitudes.
Recent technological advances have enabled the design of miniaturized microwave instruments that are quite capable and, at the same time, inexpensive enough to consider the formation of a convoy of identical sensors in low-Earth orbit.
Several missions aiming at looking the fast changes of clouds have been proposed in recent years with, for some of them, launch of the demonstrator. For instance, the TEMPEST mission (TEMPoral Experiment for Storms and Tropical systems, JPL/NASA-Colorado State University; Reising et al., 2015; Padmanabhan et al., 2020) proposes to deploy five Smallsats flying 5 min apart to observe the global clouds and their transition to precipitation with microwave sensors. Its demonstrator, TEMPEST-D, successfully deployed from the ISS and operated over 05/2018–07/2021. Rotation maneuvers of the spacecraft allowed to approach the feasibility of the exploitation of measurements of a same scene with a very short revisit time (Schulte et al., 2020). The TROPICS constellation (Time-Resolved Observations of Precipitation structure and storm Intensity with a Constellation of Smallsats, GFSC/NASA-MIT Lincoln Laboratory; Blackwell et al., 2018) will combine six CubeSats distributed into three orbital planes for a 30min revisit time and aims at providing microwave measurements on the lifecycles of extreme meteorological events like storms and cyclones. The test satellite TROPICS-Pathfinder was launched on June 2020 and will be joined in 2022 by the rest of the constellation. One can also mention the C3IEL mission (Cluster for Cloud evolution, ClImatE and Lightning, CNES and ISA; Rosenfeld et al., 2019), that focused on the 3D envelope of clouds from stereocameras onboard two to three nanosats, taking snapshots of the same scene every 20s at two to three different viewing angles. Finally, the 10th Earth Explorer Mission of ESA called Harmony will be a convoy of satellites carrying a multi-beam IR instrument that will measure height-resolved cloud-top movements.
A rather closer formation of satellites, separated in time by Δt ∼ 1 min, would reach the temporal scale required to observe the highly nonlinear cloud dynamics present in convective updrafts (Haddad et al., 2017; Sy et al., 2017; Stephens et al., 2019). For instance, Sy et al. (2017) have shown that pairs of Ka-band profiling radars 90s apart would be able to resolve the dry air mass flux and condensed-water flux above the melting level (∼5 km in tropical storms). The INCUS mission (“Investigation of Convective UpdraftS”) relies on such a constellation of 3 Ka-band radars accompanied by a TEMPEST-D passive radiometer and has been selected recently by NASA as part of its Earth Venture Program.
Inspired by these studies, the proposed C2OMODO mission consists of a tandem of identical passive microwave radiometers separated by less than 3min, that would provide the scientific community with measurements of the convective mass flux Mc and, thanks to the swath of the radiometer, to the surface of the convective cells σ (Eqs. 1, 2).
In Section 2 we describe further the C2OMODO mission. Section 3 is dedicated to unravelling the information content of such an observing system thanks to several case studies, idealized (3.1) or nature-like (3.2), to the promising synergies if a Doppler radar is aligned with the tandem (3.3). Section 4 presents the main lines of retrieval algorithms, from the question of the detection of a convectively active column from d/dt measurements (4.1) to operational “Level-2” products (4.2). Section 5 draws the main implications of the C2OMODO tandem.
The C2OMODO “Mini-Train”: Overview of the Instruments
C2OMODO stands for “Convective Core Observations through MicrOwave Derivatives in the trOpics”. This project has been conceptualized in 2018, following a round-table meeting that focused on distributed small instruments (such as radiometers or radars) as an emerging strategy to observe atmospheric dynamics of clouds and storms at very fine temporal scales (Haddad et al., 2017; Sy et al., 2017; Stephens et al., 2019).
The time-delayed observations are conceptually similar to those obtained from ground weather radars, as well as geostationary imagery, which readily show the evolution of precipitation (in the radar case) or cloud tops (in the imagery case) over minutes. The satellite convoys overcome the limitations of geostationary images (sensitive only to the very top of the clouds), and those of ground radar (not available over the tropical oceans). While passive microwave radiometers tend to be more sensitive to the total amount of condensed water in the column (Crewell et al., 2009), multi-channel microwave radiometry, with adequately selected frequencies, may be used to provide vertical information on hydrometeors (Evans et al., 2012; Birman et al., 2017). Then if each satellite instrument is sensitive to the 3-dimensional distribution of condensed water within its field of view, the convoy is sensitive to the change in this condensed water over the minute(s) separating the convoy members.
The C2OMODO mission concept explores this time-difference approach with passive microwave radiometers flying 30–180s apart. The time-lag between the two radiometers is part of ongoing sensitivity studies and is not yet fixed. This is illustrated on Figure 1 using simulations of the weather forecast model AROME (Application of Research to Operations at MEsoscale) of Météo-France. For this illustration, the two passive radiometers provide the horizontal map of the time difference in brightness temperatures (henceforth Tb and dTb/dt) measured in a 183.31 ± 11 GHz channel, and surround a 94 GHz radar looking at nadir, reflectivity providing vertically resolved distribution of cloud hydrometeors. The convective core sampled for this schematic, associated with the highest values of reflectivities, is clearly visible on the time-difference map and its horizontal structure reveals patterns associated to the convective activity.
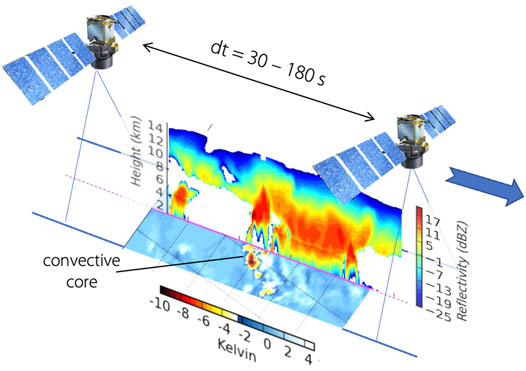
FIGURE 1. schematic of a convoy of satellites carrying passive microwave instruments providing the horizontal map. The map reveals the intensity of in-cloud upward motion associated to condensed water from time differences of the brightness temperature (in K, over dt = 30s) measured at 183 ± 11 GHz. The vertical cross-section is the vertically-resolved concentration of hydrometeors (reflectivity) as provided by a radar (in the present case, the CloudSat radar at 94 GHz).
The two passive microwave radiometers inherit from the SAPHIR moisture sounder on Megha-Tropiques (Brogniez et al., 2013; Roca et al., 2015), which underwent technical improvements to have a more compact (more receivers for the same volume) and less energy-consuming instrument (Puech et al., 2021), as well as from the ICI sounder onboard the upcoming MetOP-SG (Thomas et al., 2012). This so-called SAPHIR-New Generation (SAPHIR-NG) sounder will observe the 183.31 GHz and 325.15 GHz strong H2O absorption lines and will be completed by a window channel at 89 GHz (Puech et al., 2021).
At these frequencies, the upwelling radiation from the low troposphere is quite large, and the interaction with the icy hydrometeors that accumulate in the clouds is mostly by scattering. Therefore 183 GHz measurements are generally used very successfully to detect deep convection and overshoots (Burns et al., 1997; Greenwald and Christopher, 2002; Rysman et al., 2016; Chen and Bennartz, 2020; among many others). Since the scattering is strongly dependent to the size of the particle, measurements at 325 GHz will be sensitive to smaller ice particles, thus providing complementary observations to 183 GHz measurements during the formation and dissipation of convection. Hence in presence of hydrometeors the intensity of the depression in the Tb with respect to the Tb of the surrounding clear sky is modulated by the concentration of hydrometeors in the column.
A hyperspectral configuration is also currently considered as an option for the 183 and 325 GHz channels. A reinforced spectral sampling would be valuable for the vertical profiling both for the water vapor estimates in clear sky and the profiling of hydrometeors (Birman et al., 2017). Note that the added-value of this hyperspectral sampling is not considered in the present study.
Preliminary Information Content Studies
This section presents information content studies based on numerical models. An idealized simulation of a single convective cell is first studied and used to test sensitivities to microphysical properties, followed by nature-like simulations covering large domains that convey a wide range of convective activity. The complementarity of the C2OMODO tandem with a Doppler radar is finally addressed with a case study from a large-eddy simulation.
Idealized Study of Individual Convective Cells
The Goddard Cumulus Ensemble (GCE) model was used to conduct two idealized simulations, one with strong and one with weak convection. Warm bubbles are set-up to trigger the convection: a 10-km diameter bubble and peak temperature perturbation of 1K for the strong case and a 3-km diameter bubble and peak temperature perturbation of 3K for the weak case. The characteristics of the GCE model are provided in Appendix A.1. For each of the two idealized situations, four simulations have been conducted: a “control” simulation, a “graupel” simulation that produces less and smaller graupel; a “+cloud ice” simulation that produces more pristine ice particles; and a “graupel and +ice” simulation that combines the changes in “graupel” and “+cloud ice”.
Time series of several parameters from these simulations are shown in Figure 2.
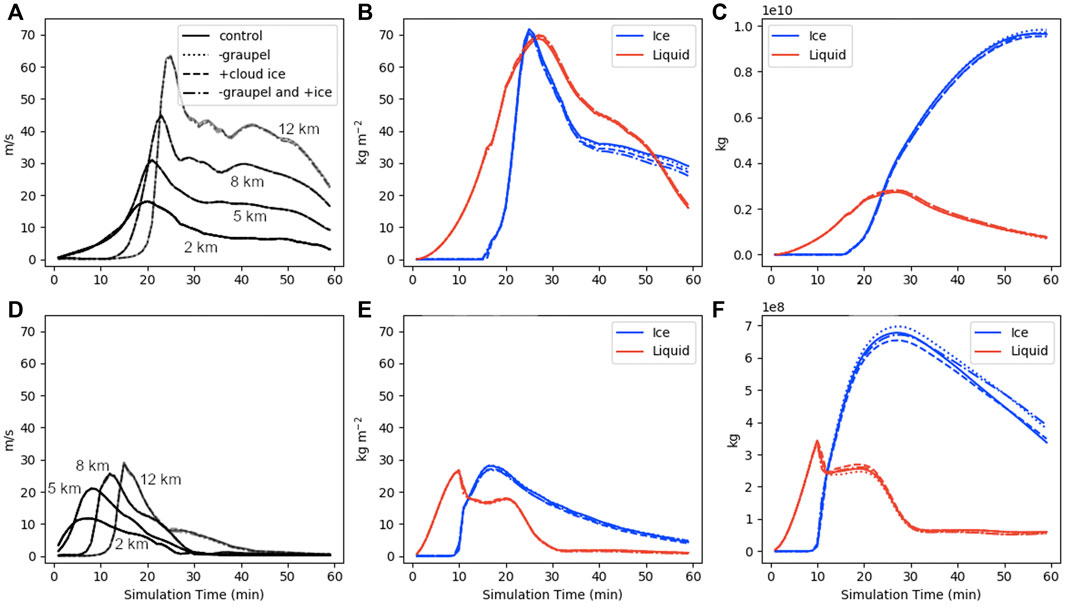
FIGURE 2. Time series of the maximum updraft speed at four altitudes [in m/s, subplots (A,C)], maximum ice and liquid water paths (in kg/m2, subplots (B,E), and total ice and liquid condensate mass [in kg, subplots (C,F)] for the strong (top) and weak (bottom) convection cell simulations and for the four microphysical situations.
Despite the perturbations to the microphysics there are only minor (<10%) differences in the maximum and total condensate at any given time step (Figure 2 b-c-e-f). This is because the instability-driven updraft dominates the condensation process; changes in microphysical process rates affect the partitioning among species, but not the total condensate. There is some separation between experiments after the initial updraft (t > 30 min), where the general effect of the perturbations is to reduce the maximum of ice water path (via reduction of riming, henceforth IWP in kg/m2), but increase the total ice condensate (by reducing the fall speed as more ice is present in the slower-falling aggregates and cloud ice categories).
The eight GCE simulations were used as input to a radiative transfer model to forward simulate the SAPHIR-NG channels at the radiometer resolution and at 1-min time steps (cf Appendix A.1 for details). Two variables were examined for each channel: the minimum brightness temperature (Tbmin, spatial minimum) and the Integrated Scattering Depression (ISD). The ISD (in K.km2) is defined as the inverse area integral of the Tb at time step t (Tb(t)) subtracted from the mean background Tb at the first time step of the simulation, prior to any condensation (Tb(t=0)):
The ISD is computed for each frequency. The time series of Tbmin and its derivative dTbmin/dt are shown in Figure 3 for two selected channels in the 183 and 325 GHz bands. These channels were chosen for their similar weighting functions and clear-sky Tb. Differences are therefore due to the frequency dependence of the scattering properties of the various hydrometeor species.
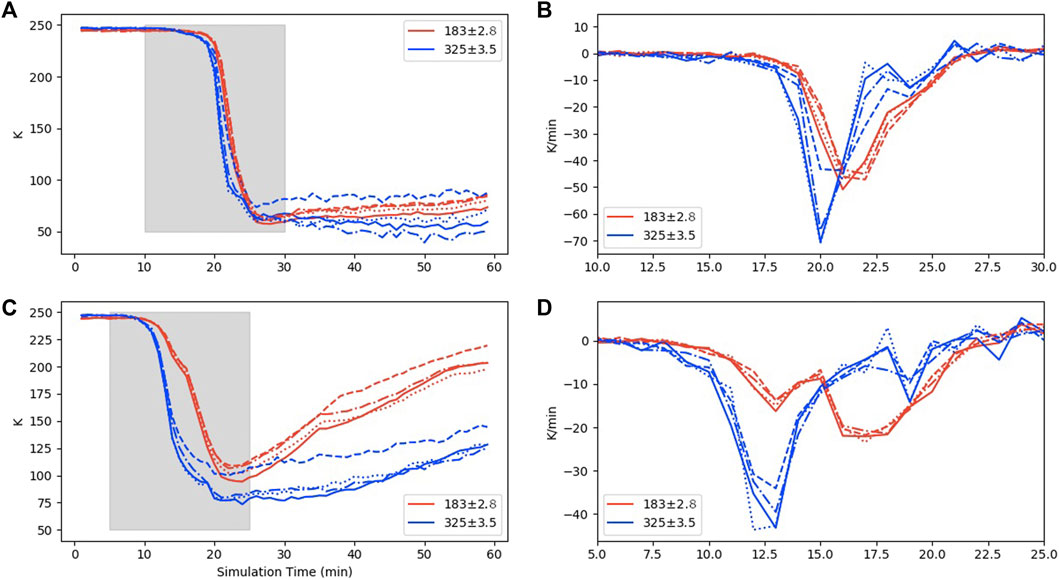
FIGURE 3. Time series of minimum brightness temperature (Tbmin in K, subplots (A,C) and its time derivative (dTbmin/dt in K/min, subplots (B,D); note the change in time scale) for the C3 (183 ± 2.8 GHz in red) and C8 (325 ± 3.5 GHz in blue) SAPHIR-NG channels. The right panels are temporal zooms of the left panels, covering the period in grey shadings. Top row: strong convection; bottom row: weak convection. The line styles indicate the microphysics perturbation experiments (see Figure 2).
The initial pattern, for both frequencies and convective cell strengths, is a rapid decline of Tbmin caused by the formation of large quantities of condensate. Tbmin decreases sharply during the initial period when IWP is increasing. There are some differences between the 183 and 325 GHz frequencies: The magnitude of the dTbmin/dt is higher and occurs earlier in time at the 325 ± 3.5 GHz channel compared to that of the 183 ± 2.8 GHz channel. This is due to the contribution of supercooled liquid water, which absorbs more strongly at 325 than 183 GHz, which damps the scattering signal more strongly at the higher frequency until the cloud fully glaciates. Thus, dTbmin/dt can be used to infer the glaciation state of a convective plume. There is relatively little sensitivity of Tbmin or of dTbmin/dt to the microphysics perturbations, although the “+cloud ice” experiment did consistently increase Tbmin at 325 GHz after the initial updraft stage, due to the change in particle size distribution (smaller cloud ice particles have a lower single scatter albedo than larger aggregate or graupel particles).
The ISD and its time derivative d (ISD)/dt, can also be readily estimated from C2OMODO simulated swath measurements. The time series of these parameters for two selected channels with similar clear-sky weighting functions so the differences can again be attributed to the frequency-dependent scattering properties of the condensed water species that are shown in Figure 2.
The ISD is, to the first order, a proxy for the total condensed ice water (Figure 4), although some notable differences between frequencies and microphysics experiments can be observed. First, the ISD at 325 GHz exceeds that at 183 GHz, due to the increased scattering optical depth with frequency (Buehler et al., 2007). For the two cases, after the initial updraft (∼40min for the strong convective case, ∼30min for the weak convective case) the 325 GHz ISD continues to grow or reaches a steady state as the anvil expands, even as the 183 GHz ISD begins to decrease. This is a consequence of the nonlinear dependence of the Tb on IWP. At 325 GHz, the scattering signal saturates at a lower IWP than at 183 GHz and the size of the anvil (not the average IWP) is the dominant factor in determining the ISD.
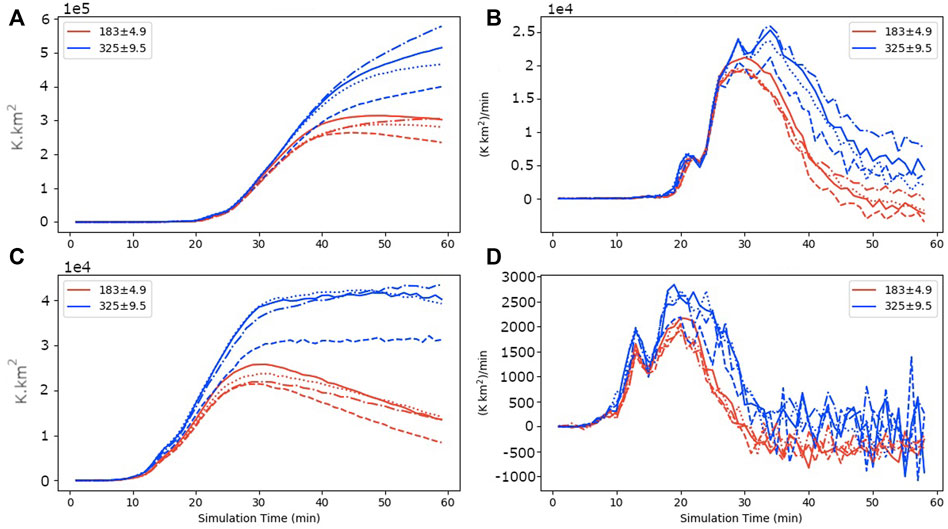
FIGURE 4. Time series of Integrated Scattering Depression (ISD, in K.km2, subplots (A,C) and its time derivative dISD/dt (in K.km2/min, subplots (B,D) for the C4 (183 ± 4.9 GH, in red) and the C9 (325 ± 9.5 GHz, in blue) SAPHIR-NG channels. Top row: strong convection; bottom row: weak convection. The line styles indicate the microphysics perturbation experiments (see Figure 2).
As with the Tbmin, the increase cloud ice experiment (”+cloud ice”) had the most consistent effect in reducing the ISD by partitioning the condensed ice into smaller particles with lower single scattering albedos, without a compensating increase in the areal coverage of the anvil. The time derivatives of ISD appear to be more sensitive to the microphysics perturbations at 325 GHz than 183 GHz and are rather noisy on the 1-min scale, especially for the weak convection, suggesting that a longer separation time (∼5 min) may be optimal for discerning the microphysical processes that govern anvil evolution than the short (∼1 min) timescales that capture the processes in the initial updraft.
Nature-Like Situations Over a Large Domain
In addition to the two idealized simulations described previously, convective-scale simulations from the non-hydrostatic model Meso-NH are used to complete the information content study of the C2OMODO concept. One situation considers the thunderstorm Hector that develops almost on a daily basis during the period September - April over the Tiwi Islands North of Darwin, Australia (henceforth HEC, initialized by a radiosounding launched on Nov. 30th 2005 at 0000 UTC, Dauhut et al., 2015) while the other situation is a radiative-convective equilibrium ocean case (RCE) from the RCEMIP exercise (Wing et al., 2020). The characteristics of Meso-NH and the details of the simulations are provided in Appendix A.2. Again, the SAPHIR-NG channels are simulated for each set of simulations. Figure 5 presents a snapshot of the Meso-NH HEC set of simulations in the Tb space for two channels (C1 at 183.31 ± 0.2 GHzand C6 at 183.31 ± 11 GHz) and the corresponding time derivative dTb/dt, for a 1 min time step (dt = 1 min).
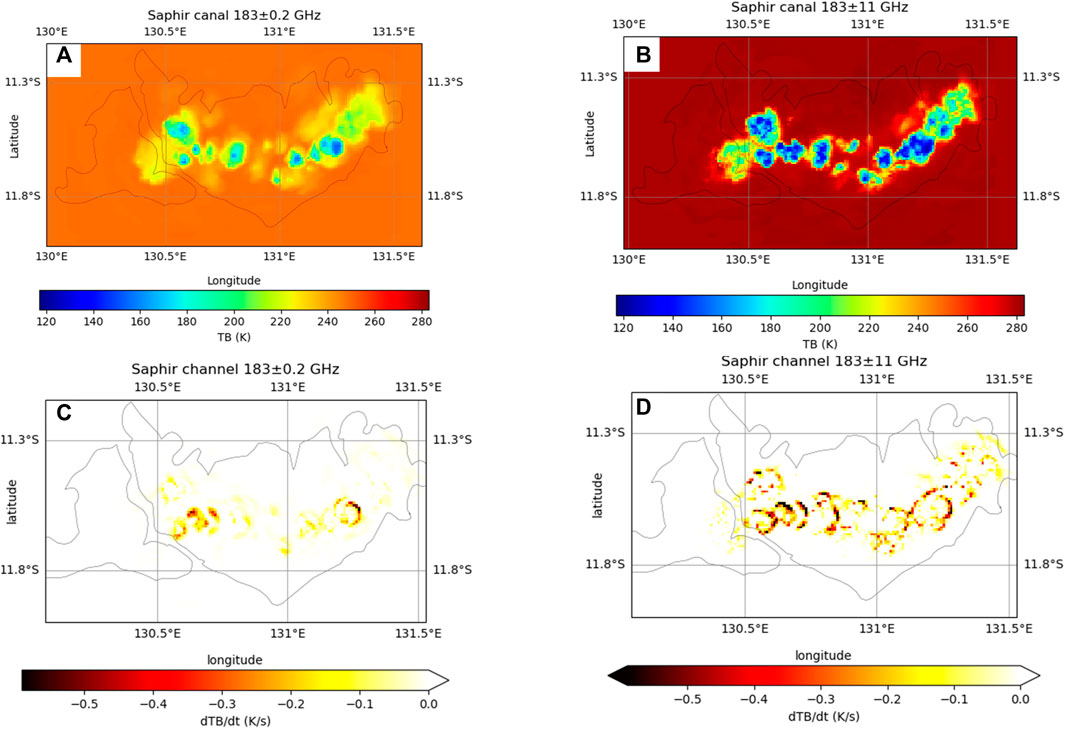
FIGURE 5. Snapshots from the Meso-NH HEC simulations (model resolution at 1 km). Top panels show the Tb (K) for (A) C1 and (B) C6 channels, and bottom panels show the dTb/dt for (c) C1 (183.31 ± 0.2 GHz) and (d) C6 (183.31 ± 11 GHz) channels, for a time interval of dt = 1min. On figures (C) and (D), the white areas are masked using a deep convection criterion (following Rysman et al, 2016) as well as dTb/dt < 0 (see text for details).
The minimum Tbs for channels C1 (183.31 ± 0.2 GHz, sounding in the upper troposphere) and C6 (183.31 ± 11 GHz, reaching the top of the boundary layer) are colocated as expected, the intensity of the depression with respect to the surrounding clear sky is much stronger for channel C6 than for channel C1. The maps of the corresponding dTb/dt seem to show that the largest temporal variations are on the edges of the minimum of the Tbs, linking to the anvil evolution as mentioned previously (Section 3.1). It should be noted, however, that the true radiometer resolution (5 km at 183 GHz at nadir, see Table 1) will reduce the amplitude of the differences.
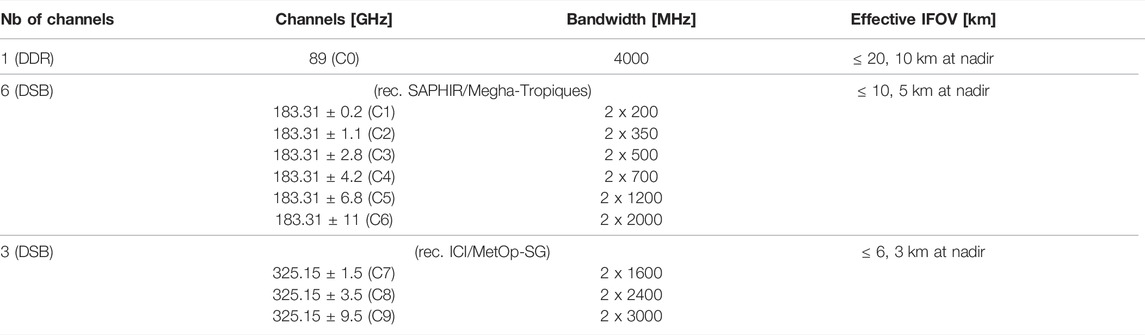
TABLE 1. Main characteristics of the SAPHIR-NG radiometer. DDR: Direct Detection Radiometer. DSB: Double-Sided Band.
The relationship between the simulated Tbs and the model variables is examined for both the HEC and RCE simulations. The focus is on the center of two absorption bands defined from the optional hyperspectral configuration at [183.31; 183.31 + 0.2] = 183.41 GHz and [325.15; 325.15 + 0.2] = 325.25 GHz and for a 1-min delay of the satellite tandem.
To match the expected observational pixel resolution (see IFOV on Table 1), the simulation outputs were averaged at the 6 km resolution at 183.31 GHz and 3 km at 325.15 GHz. As underlined in the previous section, the Tbs are strongly sensitive to IWP in deep convection. Two model variables for which a relationship with the observations of the C2OMODO concept is expected are thus examined: the time derivative of IWP (dIWP/dt), and the IWP-weighted vertical velocity (wice). More precisely, wice is computed from the vertically integrated momentum of ice (VIM, in kg/m/s) following
rice is the mixing ratio of the total ice (kg/kg), including cloud ice, graupel and snow, ρ is the density of air (kg/m3), and w is the vertical velocity (m/s). The variable wice thus characterizes the vertical wind speed within the icy cloud weighted by the ice content and integrated over the atmospheric column.
Results for the 183.41 GHz channel are shown in Figure 6. The grey shading delineates the impact of a 1-K uncertainty on the Tb, which can be considered as a best-case scenario. It is indeed expected that the intercalibration and geolocalization between the two radiometers will add noise to the dTb/dt. Only grid points where the criterion Tb183.31-Tb193.31 > 0 is satisfied are kept, a criterion successfully used by Rysman et al. (2016) among others to detect deep convection. Due to stronger deep convection activity for HEC than for RCE, the dTb/dt reaches larger negative values in the former simulations (-0.2 K/s) than in the later (-0.1 K/s). Consistently, the median value of dIWP/dt extends up to about 40 g/m2/s (HEC) and 30 g/m2/s (RCE). For both simulations, a linear relationship between dTb/dt and dIWP/dt is found. The slope of the regression line taken as the median is equal to -200 g/m2/K and the interquartile range of the distribution remains close to the median. The good agreement between HEC and RCE suggests that this relationship is weakly dependent on meteorological conditions, at least under tropical situations.
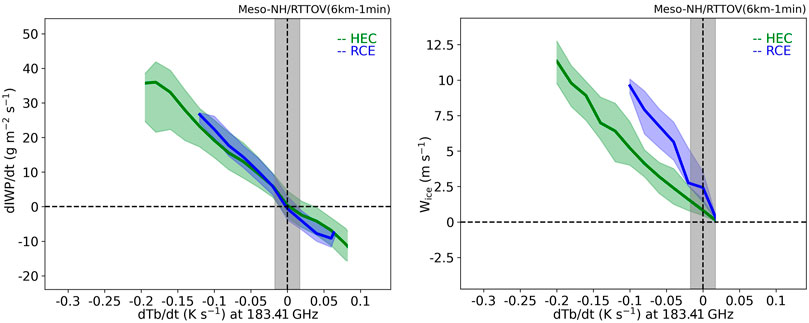
FIGURE 6. Histogram of dIWP/dt (left, in g/m2/s) and wice (right, in m/s) as a function of dTb/dt for the 183.41 GHz channel, at 6 km resolution and with a time delay of the satellite tandem of 1 min. The bin interval is 0.02 K/s. The median (bold lines) and the interquartile ranges (shadings) are shown for HECTOR (HEC/green) and RCE (blue). The grey band delimits a dTb/dt uncertainty of 0.017 K/s, corresponding to a 1 K uncertainty in the Tb measurement for a 1 min time-delay between the satellites. Results are shown for gridpoints verifying the deep convection criterion at 183 GHz (see text), restricted to growing cores for wice (right).
The variation of wice with dTb/dt is analyzed for a subset of situations, keeping only the deep convective cores in growing stage (Figure 6, right). For this, we consider only the grid points where deep convection occurs (Tb183.31-Tb193.31 > 0, as above) and local minima of Tb and dTb/dt in the horizontal space for the 183.41 GHz channel are found. These additional filters are important because the temporal variation in IWP is due to ice transport in both the horizontal and vertical direction and to microphysical changes in the ice. Therefore, a relationship between wice and dTb/dt is expected only in the deep convective cores where the vertical transport of ice may be the dominant contributor. As previously, a quasi-linear relationship is found between dTb/dt and wice (Figure 6, right). This time, the slope of the linear regression differs between the simulations: 200 m/K for HEC and -100 m/K for RCE. This difference may be due to differences in the characteristics of deep convection (strength, size, lifetime, … ) over land (HEC) and over ocean (RCE). Further work is needed to evaluate this hypothesis.
Figure 7 presents the evolution of the same variables dIWP/dt and wice with respect to dTb/dt for the 325.25 GHz channel. The criterion for the detection of deep convection is adapted at this channel such as only gridpoints where Tb325.15-Tb335.15 > 0 are kept. The range of dTb/dt goes down to -0.3 K/s. This value is larger than that at 183 GHz because the 3 km resolution at 325 GHz allows to capture more spatial variability in the Tb than the 6-km resolution. The median value of dIWP/dt ranges to about 30 g/m2/s for both RCE and HEC. This contrasts with the 40 g/m2/s found for HEC at 183.41 GHz. The stronger absorption of water vapor at 325.25 GHz could explain a lower sensitivity to change in IWP. The time derivative of IWP, dIWP/dt, also varies quasi-linearily with dTb/dt, with an interquartile range closely following the median, but with a slope of the linear regression at median value of about -100 g/m2/K. This value of the slope is half the value obtained for the 183.41 GHz channel indicating less sensitivity of the 325.25 GHz channel to the amplitude in dIWP/dt. Obtaining information on the time change of the IWP at 3 km resolution is interesting however, to get fine-scale variability.
The variation of wice with dTb/dt for the 325.25 GHz channel is also shown (Figure 7, right). In these cases, a quasi-linear relationship between the two variables can be found with a slope around -40 m/K for HEC and -70 m/K for RCE. Again, the difference in slope for HEC could be due to the contrast of deep convection between land and sea, which requires further study.
Synergy With Doppler Radar
As briefly mentioned previously, the C2OMODO tandem may potentially fly in train with active instruments, in particular a Doppler cloud radar. This section aims at exploring the information content complementarity of both instruments. While the major limitation of a spaceborne Doppler radar is its limited swath, its main strength is its ability to resolve the whole cloud vertical profile. The synergy with the C2OMODO concept and its capability of characterising vertical mass flux at fine resolution over a wide swath is then of particular interest.
This synergy is studied here by simulating a spaceborne 35.5 GHz Doppler radar track. This simulated transect is computed from the Meso-NH RCE runs at 200 m horizontal resolution (large-eddy simulation, see Appendix A.2) The spaceborne Doppler radar simulator (Kollias et al., 2014; Kollias et al., 2018) estimates the total backscatter (unattenuated radar reflectivity factor, dBZ), gaseous and hydrometeor signal extinction (dBZ/km) and mean Doppler velocity. T-matrix scattering is used for the cloud, drizzle, and rain hydrometeor species, and the Self-Similar Rayleigh-Gans Approximation (SSRGA, Hogan and Westbrook, 2014) is used for ice and snow particles. Hail and graupel particles are assumed to have spherical shape with different densities (0.9 and 0.4 g/cm3 respectively). A realistic Earth’s surface echo (Lamer et al., 2020; Burns et al., 1997) is introduce to account for missed detections near the Earth’s surface and for estimating PIA estimates. These radar observables are used as input to a comprehensive spaceborne Doppler simulator that estimates the raw simulated spaceborne radar signals. The radar simulator accounts for the instrument sampling geometry (antenna and range weighting function, along track integration), receiver noise and platform motion.
Figure 8 shows the simulated 35.5 GHz reflectivity and the corresponding Doppler vertical velocity, as well as the associated 183 GHz Tb and their 1-min temporal derivatives centred over the radar observations. This configuration would correspond to two radiometers flying respectively 30s before and after a 35.5 GHz radar. All these simulated instruments have been averaged on a common 2.4 km resolution corresponding to the radar resolution and a sample is provided every kilometre.
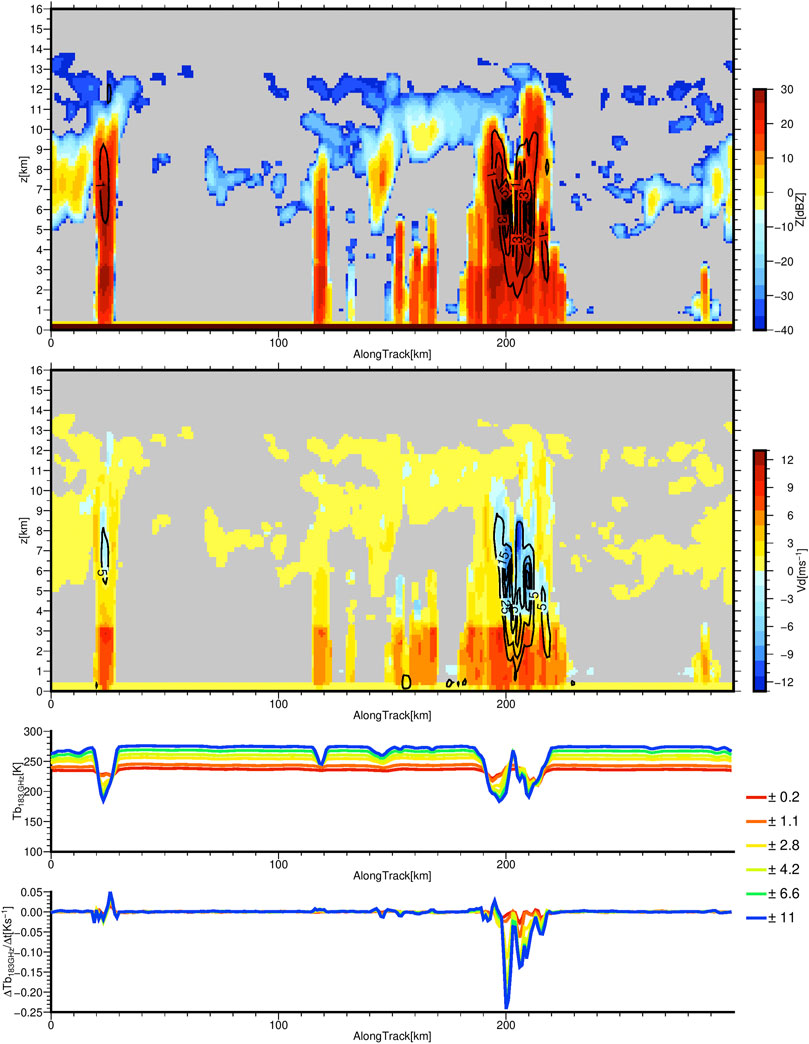
FIGURE 8. Attenuated radar reflectivity (Z, in dBZ, top panel) and corresponding mean Doppler velocity (Vd in m/s, middle panel) without satellite motion effects at 35.5 GHz emulated from the 200 m resolution RCE simulation. Upward vertical velocity (in m/s) and water mass flux (in g/m2/s) are respectively superimposed on the two top panels as contour levels. The two lower panels show the Tbs and dTb/dt of the six channels sampling the 183.31 GHz line.
This simple version of a C2OMODO/Doppler radar simulator shows that the depression in the Tb with respect to clear sky is of the same order of magnitude whatever the intensity of convection (compare at x = 20 km and x = 200 km). This saturation effect limits the retrieval of the intense vertical transport of hydrometeors by convective motions. As underlined in the previous sections, the dTb/dt encompasses convective strength, which allows for accurately locating the convective and intensifying cores in a wider swath than what is possible with a radar. Indeed, the lower values in dTb/dt are associated to the atmospheric columns where the more intense vertical motions and condensed mass fluxes are observed. At each altitude, during this short 1-min time interval, hydrometeors are produced through microphysical processes or transported from below (modulus their fall speed) analogous to a Doppler velocity (Stephens et al., 2019).
Figure 9 shows the correlation at each altitude of the radar Doppler velocity Vd with the dTb/dt for the six 183 GHz channels for the situations of deep convection identified using the detection criteria described in Section 3.3. The highest values of correlation (that can be higher than 0.8) are reached at different altitude according to the channel because of their different weighting functions. Under clear sky situations, the peaks of 183 GHz channels are between 7 (183 ± 1 GHz) and 2 km (183 ± 7 GHz) altitude, the actual altitude depending on the water vapor content (Chen and Bennartz, 2020), with upward shifts in cloudy situations. The correlations displayed in Figure 8 reach the highest values close to the altitude of the peak of the weighting functions. This suggests that the signal that is contained in the Doppler velocity is also contained in the dTb/dt of the passive instruments.
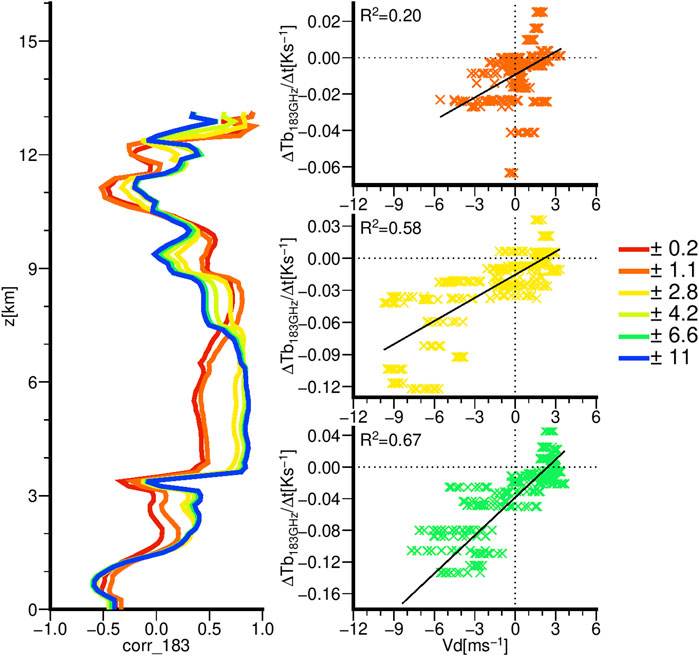
FIGURE 9. Correlation coefficient computed at each altitude between radar Doppler velocity and temporal derivatives of the six SAPHIR bands sampling the 183 GHz water vapor absorbing band for convective profiles (first vertical panel). Brightness temperature temporal derivatives (dTb/dt in K/s) as a function of Doppler velocity Vd (in m/s) for convective profiles in 1 km-depth altitude layer centered on the peaks of the weighting functions (second vertical panel) for SAPHIR channels C2, C3 and C5. The black lines are linear regressions and the associated R2 are provided.
The scatter plots of Figure 9 show the dTB/dt of the C2 (183 ± 1.1 GHz), the C3 (183 ± 2.8 GHz) and the C5 (183 ± 6.8 GHz) SAPHIR-NG channels as function of the radar Doppler velocity vd averaged in 1 km-depth layers roughly centered around the peaks of the weighting functions. For these three layers, a linear relationship is found between the dTB/dt and the layer-averaged Vd: a reinforcement of the upward mass flux translates into a higher IWP and thus a larger reduction of the Tb (Chen and Bennartz, 2020).
This is exactly where the synergy between the two sets of observations lies: the vertically-resolved profiles of Doppler vertical velocity provided by the radar can be extrapolated to the swath of the C2OMODO passive radiometers to obtain layer-averaged upward motion of ice particles as well as their horizontal extent.
Towards a Retrieval Algorithm
The previous sections have shown that there is a link between the vertical mass flux in a convective atmosphere and passive microwave observations spaced in time by a short delay dt. Here we draw the main lines of the data processing involving both the observations and their time-derivatives. Such data processing needs to quantify the extent to which the vertical transport in a cloudy column over a discrete time interval dt can be characterized from a set of measurements O = [ Tb1(t),…, TbN(t), Tb1 (t + dt), …, TbN(t + dt) ] measured in N channels at the initial and final times t and t + dt.
A straightforward approach is to proceed in two steps:
1. Quantify how well the observational setup can detect if the column has any significant vertical transport in the first place—this is the detection step;
2. Then, and only for those columns where vertical transport is detected, quantify how well the coarse vertical characteristics of the transport can be retrieved. The characteristics will necessarily be coarse, because the passive measurements have already been shown to be sensitive to the coarse-scale vertical distribution of condensed water (Jiang et al., 2017; Chen and Bennartz, 2020) without the ability to resolve changes at resolutions on the order of 1,000 m or finer.
Detection of a Convectively Active Column
The first step requires the derivation and evaluation of a detector. Starting with a set of convection-permitting model simulations (CPMs), conducted at horizontal resolution sufficiently fine to represent the vertical transport reasonably accurately (i.e., on the order of 100 m), one can try to derive the joint distribution pup of one’s observations O = [ Tb1(t), …, TbN(t), Tb1 (t + dt), …, TbN(t + dt) ] conditioned on there being a significant vertical transport in the column (subscript “up”), and quantify how different this distribution is from the joint distribution pnot when there is no significant vertical transport in the column (subscript “not”). For simplicity, one can define “there is a significant vertical transport in the column” to mean that there is a height h in the column where the vertical velocity w(h) exceeds a threshold wmin and where the condensed water content q(h) also exceeds a threshold qmin. Each distribution can be approximated by a Gaussian, so that one only needs to compute the two conditional means Om,up = E{O | updraft} and Om,not = E{O | no updraft}, and the two corresponding conditional covariance matrices Cup = Cov{O | updraft} and Cnot = Cov{O | no updraft}.
Here our CPMs is the WRF model which was set-up to simulate Hurricane Isabel at the expected radiometer resolution. The details of the model are provided in Appendix A.3. One half of the simulations obtained during the first 10 min of the run was used as the reference and one half of the simulations in the latter half of the run was used to evaluate the retrieval errors. The columns produced by our CPM have been analyzed for different combinations of wmin = 1, two or 3 m/s and qmin = 0.05 or 0.2 g/m3. Only three channels are retained for simplicity: at 166 GHz (a window channel very similar to the C6 channel of SAPHIR-NG), 184 GHz (close to C2), and 190 GHz (close to C5). The results are summarized in Table 2.

TABLE 2. Probabilities of detection/false-alarm of non-shallow convective core from measurements of a pair of radiometers, according to different minimum thresholds in condensed water qmin and vertical velocities wmin.
This observational configuration allows to detect correctly the presence of a significant updraft in the column more than 70% of the time. In the case of the lower detection threshold (qmin = 0.05 g/m3) the probabilities of detection reach higher values (>80%). The false-alarm rates seem to stay the same regardless of what is chosen for qmin at a given vertical velocity wmin. These results highlight that passive microwave radiometers aligned in a convoy separated by a short time (∼1min) can achieve a success rate in excess of 80% for the identification of convective updrafts.
The approach to evaluate the detection can be used to quantify the sensitivity of the observations to the coarse vertical characteristics of the underlying updraft. To the extent that the prototypical updraft, as a function of height, should start with w = 0 at the lowest level (by definition) increasing to a maximum value wmax somewhere in the column and then decreasing down to 0 past the top of the cloud, it is not unreasonable to try to determine the value wmax along with the height hmax at which it is achieved. To determine how sensitive the observation vector O is to the pair (wmax, hmax), one can start by partitioning the two-dimensional (wmax, hmax)-space into a set of contiguous tiles, indexed by a pair of indices (i,j) where i indicates the discrete interval of values of wmax and j the discrete interval of values of hmax. The CPMs columns that fall in each tile can then be used to approximate the conditional distribution pi,j of O in that tile, namely by considering that pi,j is normal and hence completely determined by the conditional mean mi,j = E{O | the underlying column is in tile (i,j)}and the conditional covariance Mi,j = Cov{O | the underlying column is in tile (i,j)}, which can be readily computed given the columns in each tile. Armed with these distributions, i.e. the conditional-mean vectors mi,j and conditional-covariance matrices Mi,j, one can easily determine which tile a given arbitrary observation O “belongs” to: indeed, O is most likely to be from the population (i,j) for which pi,j(O) > pi’,j’(O) for all other (i’,j’). In other words, for a given observation O one can compute the values of all the conditional distributions pi,j(O) and then choose the one with the largest value as the distribution that O most likely belongs to—and thereby attribute to O the value of (wmax, hmax) in that maximum-likelihood tile. Rather than stopping at the mean value of (wmax, hmax) in that tile, we derived a linear regression for (wmax, hmax) in terms of the entries of O, for each tile. The resulting estimate of wmax(O) and hmax(O) can then be compared with the true values for the column, to quantify the error in this simple characterization.
In a nutshell, we first compile a reference database D of stormy columns and we partition the database D into tiles Di,j according to the true values of (wmax, hmax), so that in real time, given an observation vector O, we can calculate the different probabilities pi,j(O) of observing O if the truth was in either one of the tiles and then select the tile for which this probability is the largest: that is the tile to which O most likely “belongs” and therefore the mean of wmax and hmax in that tile are the values that we associate as the retrieval for the observed O. Table 3 summarizes the results with root-mean-square errors (rmse).
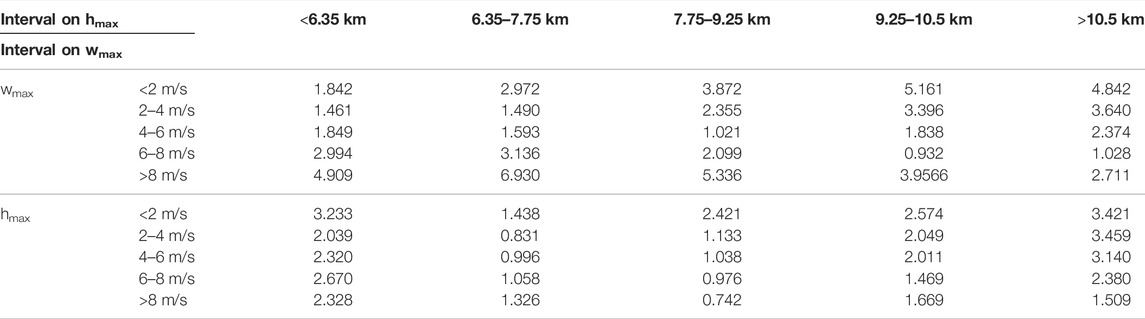
TABLE 3. Root-mean-square errors on wmax (in m/s) and hmax (in km) for different tiles of the space (wmax, hmax).
The values of rmse for wmax are highest for the slowest (<2 m/s) and fastest (>8 m/s) updrafts, whatever their altitude in the column. In between, the linear regression established from the observation vector O allows to estimate the maximum vertical velocity wmax for each of the pre-defined atmospheric layers with reasonably small uncertainties. In parallel, this detector can also attribute with a good accuracy the altitude hmax of wmax for each layer, thus enabling to determine vertically the altitude of maximum velocity within the column.
The estimates of wmax and hmax, together with the estimate of the cloud top height (CTH), defined as the maximum height for which q > qmin, provide a coarse description of the vertical shape of the vertical transport of mass. Hence, using wmax, hmax and CTH as well as the top three principal components of the profiles computed from a Principal Component Analysis onto the reference database D (gathering the stormy columns only), one can reconstruct the profile of vertical transport. Examples of reconstructed profiles of mass flux are presented on Figure 10 and compared with the original ones. As illustrated the retrieved profiles are not perfect replicas of the originals, but the discrepancies follow the errors summarized in Table 3.
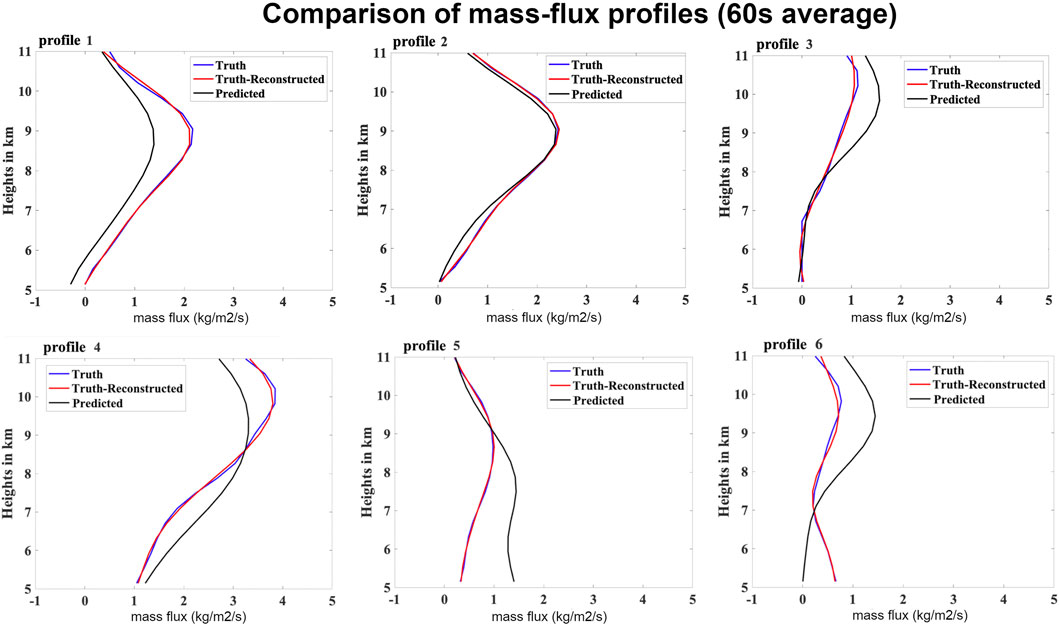
FIGURE 10. Six examples of profiles of vertical mass flux retrieved the Tbs obtained at t and t + dt: the Tbs are used to estimate wmax and hmax, which are combined to the cloud top height (CTH) and the top three principal components of each profile obtained from a PCA (see text for details). The blue curve is the original profile, and the red curve is the reconstruction of that profile using only its top three principal components. The black curves are the profiles retrieved from wmax, hmax and CTH.
Development of “Level-2” Geophysical Products
These information content studies make it possible to fully explore the swath of the C2OMODO concept to infer the upward motion of ice within convection, through the development of a retrieval method. Here we present an insight of such retrieval, as a first stage of future Level-2 products derived from time-differences of microwave Tb.
Both machine learning or deep learning methods are well suited for multi-variate retrievals (Aires et al., 2011; Sivira et al., 2015). For the sake of simplicity in this overview paper on the C2OMODO mission, we focus on a retrieval based on full convolutional neural networks (also called U-Net, Ronneberger et al., 2015). Such approaches, adapted to image detection and classification, have been recently applied very successfully to highly resolved satellite images and the retrieval of parameters like surface winds (Shen et al., 2019) or rain rates (Veillette et al., 2018; Choi and Kim, 2019; Sadeghi et al., 2020; Duan et al., 2021). Basically, the architecture of U-Net is made of a series of blocks of convolutional functions that detect the spatial structures of the input image and encode them into feature representations at different spatial resolutions. The decoder part of the U-Net projects the features that have been detected into the original image. The advantage of deep learning methods over traditional (e.g. Bayesian) methods lies a lot on the learning of the spatial structures, not just on the signal itself.
The baseline simulation of HEC discussed above underwent slight perturbations to increase the size of available simulations (increases of 10% RH to 40% RH with or without wind, yielding to 10 different versions of HEC). Each one of the simulations underwent a procedure of data augmentation with a random horizontal and vertically flipping to prevent the U-Net from just learning the position of the spatial structures within the maps. The training and prediction stages use these 10 available versions of HEC, through cross-validation procedure: nine out of 10 are used for the training (80%)/validation (20%) steps while the last one is dedicated to test the retrieval. A gradient descent is used to update the weight during the training while the optimization method is ADAM (Kingma and Ba, 2014). The deep convection criteria defined above (Tb183.31-Tb193.31 > 0, see Sect 3.3.2) is applied to all the simulations, in order to focus on the learning of deep convective structures and not the surrounding clear air.
For the present case, which is purely a demonstration, we make use of the (Tb, d Tb/dt) variables of the six channels at 183 GHz and the three channels at 325 GHz, without the hyperspectral option. A U-Net is thus trained on this subset of 18 variables (dt = 1min) to learn their non-linear relationships with the vertically integrated ice mass flux (VIM, Eq. (4)). The results of the VIM retrieved from this U-Net are illustrated in Figure 11 as a demonstration. The map (Figure 11A) shows the structure of the convective systems as defined from the VIM variable for one time step of HEC.
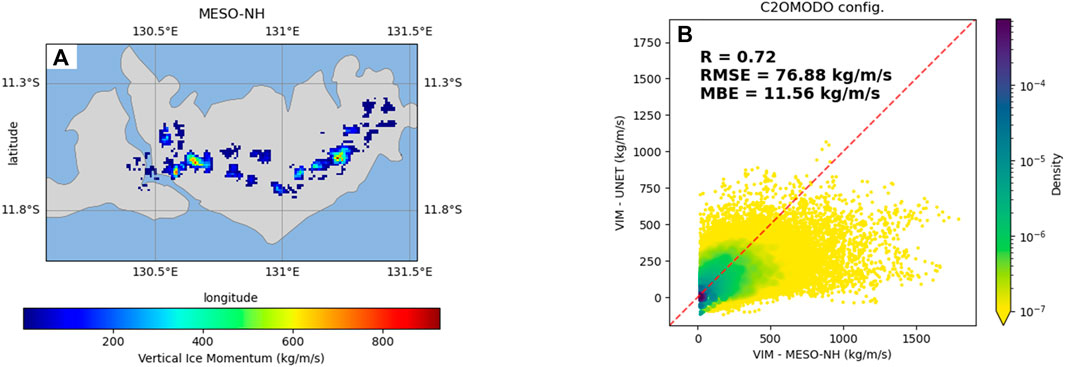
FIGURE 11. (A) Vertically integrated ice mass flux (VIM, kg/m/s) as simulated by MESO-NH for a snapshot of the HEC set of simulations. (B) Meso-NH VIM (x-axis) versus predicted VIM (y-axis) for the filtered deep convective situations within the entire validation dataset. The Pearson correlation coefficient (R), root-mean-square error (RMSE) and mean bias (MBE) are indicated. The color scale for the scatter plot represents the normalized density of data, in log scale.
The comparison of the VIM provided by MESO-NH and the predicted VIM from the U-Net for the full set of the validation dataset is presented on Figure 11B. The U-Net algorithm performs already really well for the retrieval of the VIM parameter with a good correlation (0.72), a small bias (11.56 kg/m/s) and a quite reasonable RMSE (76.88 kg/m/s). One can also notice a slight tendency to underestimate the large values of VIM. This most certainly comes from the definition of VIM which can lead to values near 0 kg/m/s when there are both downdrafts and updrafts in the column, even if there is a large amount of ice. In such situations the U-Net model learns the relationship between the Tbs and VIM with some complicated situations where the Tb are low (near 130 K), associated to large amount of ice in the column, whereas the VIM is small.
Of course, the retrieval approach can be refined and better tuned to the information content of the C2OMODO tandem. Several factors can improve the estimations and are currently under study: the use of the hyperspectral option mentioned above; the refinement of the criteria to detect deep convection; an increase of the dataset used for the U-Net training; a more sophisticated architecture than U-Net; the retrieval of the vertical ice mass flux profile instead the integrated column in order to separate downdraft and updraft regions. The retrieval of the pairs (wmax; hmax) is also under study.
Summary and Way Forward
Measurement from passive radiometers, organized in a convoy with very short revisit-time Δt ∼ 1-min, can be smartly used to look at the fast changes that occur within the updrafts that characterize deep convection.
Within the current trend in miniaturized instruments (active/passive) on Smallsats, the C2OMODO mission proposes to exploit the information content of microwave measurements at 183 and 325 GHz and their time-derivatives. Numerical models were used to perform idealized simulations of a single convective cell as well as nature-like simulations covering large domains to look at a wide range of convective activity. These simulations were used to infer the information content of the C2OMODO mission. A third simulation involved a Doppler nadir-viewing radar (35.5 GHz). From these preliminary studies, several aspects can be drawn from the set of observations (Tb, dTB/dt) provided by the C2OMODO payload:
- the time-derivative dTb/dt at both 183 and 325 GHz can be used to infer the glaciation state of convection via the signature of IWP at these two frequencies that reflects the microphysical changes during the development of convection;
- the relationships between upward ice mass flux and dTb/dt seem weakly dependent on the weather conditions, at least in our set of tropical experiments;
- the maximum vertical velocity reached within a convective atmospheric column, as well as its height can be estimated with a small error, depending on the range of velocity (smaller error for higher speeds) and on the height (more or less close to the ground).
- the vertical information included within the Tb and dTb/dt, via the weighting functions of the channels can be related to the vertically-resolved profiles of vertical velocity from nadir-viewing Doppler radar, thus showing a path of very strong synergy between C2OMODO and a Doppler radar.
Such new set of observations can be explored to dig further into the physics of deep convection and its place within the energy and water cycle. Emerging global kilometer-scale models are now anticipated for both climate and forecast applications (Neumann et al., 2019; Bauer et al., 2021). The C2OMODO observations together with the various innovative satellite missions under development will bring an invaluable and much needed observational constraint to help improving these models that suffers from long enduring uncertainty on the vertical mass flux and vertical velocity (Varble et al., 2011; Marinescu et al., 2021).
Data Availability Statement
The raw data supporting the conclusions of this article will be made available by the authors, without undue reservation.
Author Contributions
HB and RR designed the paper and all authors contributed to the writing of the paper. Authors contributed to writing specific parts: FA and J-PC conducted the Meso-NH simulations and analyzed its results, SM and XL conducted the GCE simulations and analyzed its results, ZH performed the CPM simulations and designed the detection approach, PK ran the radar simulator and DB analyzed the radar/radiometer results, AD developed the U-Net model and HB analyzed the results.
Funding
Part of this work was performed thanks to the Megha-Tropiques and C2OMODO projects supported by the CNES French space agency. SM. work was performed within the ACCP (now AOS) Decadal. Survey Study Team supported by NASA HQ under Lead Program Scientist Dr. Hal Maring. XL was supported by PMM funding 80NSSC19K0738 under program manager Gail Skofonick-Jackson. Computer resources for running Meso-NH were allocated by GENCI through Project 90569. The ESPRI-IPSL center is also acknowledged for the computing facilities and data server.
Conflict of Interest
The authors declare that the research was conducted in the absence of any commercial or financial relationships that could be construed as a potential conflict of interest.
The handling editor declared a shared affiliation with one of the authors ZH at time of review.
Publisher’s Note
All claims expressed in this article are solely those of the authors and do not necessarily represent those of their affiliated organizations or those of the publisher, the editors, and the reviewers. Any product that may be evaluated in this article, or claim that may be made by its manufacturer, is not guaranteed or endorsed by the publisher.
Acknowledgments
Thanks are also due to James Hocking from the MetOffice for the RTTOV coefficients of the SAPHIR-NG hyperspectral configuration. Finally the two reviewers are thanked for their comments that greatly helped to improve the manuscript.
Supplementary Material
The Supplementary Material for this article can be found online at: https://www.frontiersin.org/articles/10.3389/frsen.2022.854735/full#supplementary-material
References
Adler, R. F., and Fenn, D. D. (1979). Thunderstorm Vertical Velocities Estimated from Satellite Data. J. Atmos. Sci. 36, 1747–1754. doi:10.1175/1520-0469(1979)036<1747:tvvefs>2.0.co;2
Bauer, P., Stevens, B., and Hazeleger, W. (2021). A Digital Twin of Earth for the green Transition. Nat. Clim. Chang. 11, 80–83. doi:10.1038/s41558-021-00986-y
Birman, C., Mahfouf, J.-F., Milz, M., MendrokBuehler, J. S. A., and Brath, S. A. M. (2017). Information Content on Hydrometeors from Millimeter and Sub-millimeter Wavelengths. Tellus A: Dynamic Meteorology and Oceanography 69 (1), 1271562. doi:10.1080/16000870.2016.1271562
Blackwell, W. J., Braun, S., Bennartz, R., Velden, C., DeMaria, M., Atlas, R., et al. (2018). An Overview Of The Tropics Nasa Earth Venture Mission. Q.J.R. Meteorol. Soc. 144, 16–26. doi:10.1002/qj.3290
Bony, S., Semie, A., Kramer, R. J., Soden, B., Tompkins, A. M., and Emanuel, K. A. (2020). Observed Modulation of the Tropical Radiation Budget by Deep Convective Organization and Lower-Tropospheric Stability. AGU Adv. 1, e2019AV000155. doi:10.1029/2019av000155
Brath, M., Ekelund, R., Eriksson, P., Lemke, O., and Buehler, S. A. (2020). Microwave and Submillimeter Wave Scattering of Oriented Ice Particles. Atmos. Meas. Tech. 13, 2309–2333. doi:10.5194/amt-13-2309-2020
Brogniez, H., Kirstetter, P.-E., and Eymard, L. (2013). Expected Improvements in the Atmospheric Humidity Profile Retrieval Using the Megha-Tropiques Microwave Payload. Q.J.R. Meteorol. Soc. 139, 842–851. doi:10.1002/qj.1869
Buehler, S. A., Jiménez, C., Evans, K. F., Eriksson, P., Rydberg, B., Heymsfield, A. J., et al. (2007). A Concept for a Satellite mission to Measure Cloud Ice Water Path, Ice Particle Size, and Cloud Altitude. Q.J.R. Meteorol. Soc. 133, 109–128. doi:10.1002/qj.143
Buehler, S. A., Mendrok, J., Eriksson, P., Perrin, A., Larsson, R., and Lemke, O. (2018). ARTS, the Atmospheric Radiative Transfer Simulator - Version 2.2, the Planetary Toolbox Edition. Geosci. Model. Dev. 11 (4), 1537–1556. doi:10.5194/gmd-11-1537-2018
Burns, B. A., Wu, X., and Diak, G. R. (1997). Effects of Precipitation and Cloud Ice on Brightness Temperatures in AMSU Moisture Channels. IEEE Trans. Geosci. Remote Sensing 35, 1429–1437. doi:10.1109/36.649797
Chen, R., and Bennartz, R. (2020). Sensitivity of 89-190-GHz Microwave Observations to Ice Particle Scattering. J. Clim. 59, 1195–1215. doi:10.1175/JAMC-D-19-0293.1
Choi, Y., and Kim, S. (2021). Rain-Type Classification from Microwave Satellite Observations Using Deep Neural Network Segmentation. IEEE Geosci. Remote Sensing Lett. 18, 2137–2141. doi:10.1109/LGRS.2020.3016001
Crewell, S., Ebell, K., Löhnert, U., and Turner, D. D. (2009). Can Liquid Water Profiles Be Retrieved from Passive Microwave Zenith Observations? Geophys. Res. Lett. 36, L06803. doi:10.1029/2008GL036934
Cuxart, J., Bougeault, P., and Redelsperger, J.-L. (2000). A Turbulence Scheme Allowing for Mesoscale and Large-Eddy Simulations. Q.J R. Met. Soc. 126, 1–30. doi:10.1002/qj.49712656202
Dauhut, T., Chaboureau, J. P., Escobar, J., and Mascart, P. (2015). Large‐eddy Simulations of Hector the Convector Making the Stratosphere Wetter. Atmos. Sci. Lett. 16, 135–140. doi:10.1002/asl2.534
Davis, C., Emde, C., and Harwood, R. (2005). A 3-D Polarized Reversed Monte Carlo Radiative Transfer Model for Millimeter and Submillimeter Passive Remote Sensing in Cloudy Atmospheres. IEEE Trans. Geosci. Remote Sensing 43 (5), 1096–1101. doi:10.1109/TGRS.2004.837505
Duan, M., Xia, J., Yan, Z., Han, L., Zhang, L., Xia, H., et al. (2021). Reconstruction of the Radar Reflectivity of Convective Storms Based on Deep Learning and Himawari-8 Observations. Remote Sensing 13, 3330. doi:10.3390/rs13163330
Elsaesser, G., Roca, R., Fiolleau, T., Del Genio, A. D., and Wu, J. (2021). A Simple Model for Tropical Convective Cloud Shield Area Growth and Decay Rates Informed by Geostationary IR, GPM, and Aqua/AIRS Satellite Data. Earth Sp. Sci. Open Arch., 1–39. doi:10.1002/essoar.10507613.2
Eriksson, P., Ekelund, R., Mendrok, J., Brath, M., Lemke, O., and Buehler, S. A. (2018). A General Database of Hydrometeor Single Scattering Properties at Microwave and Sub-millimetre Wavelengths. Earth Syst. Sci. Data 10, 1301–1326. doi:10.5194/essd-10-1301-2018
Evans, K. F., Wang, J. R., O'C Starr, D., Heymsfield, G., Li, L., Tian, L., et al. (2012). Ice Hydrometeor Profile Retrieval Algorithm for High-Frequency Microwave Radiometers: Application to the CoSSIR Instrument during TC4. Atmos. Meas. Tech. 5, 2277–2306. doi:10.5194/amt-5-2277-2012
Feng, Z., Dong, X., Xi, B., McFarlane, S. A., Kennedy, A., Lin, B., et al. (2012). Life Cycle of Midlatitude Deep Convective Systems in a Lagrangian Framework. J. Geophys. Res. 117, a–n. doi:10.1029/2012JD018362
Fouquart, Y., and Bonnel, B. (1980). Computations of Solar Heating of the Earth’s Atmosphere – A New Parameterization. Beitraege zur Physik der Atmosphaere 53, 35–62.
Gasparini, B., Rasch, P. J., Hartmann, D. L., Wall, C. J., and Dütsch, M. (2021). A Lagrangian Perspective on Tropical Anvil Cloud Lifecycle in Present and Future Climate. Geophys. Res. Atmos. 126, e2020JD033487. doi:10.1029/2020JD033487
Giangrande, S. E., Collis, S., Straka, J., Protat, A., Williams, C., and Krueger, S. (2013). A Summary Of Convective-Core Vertical Velocity Properties Using Arm Uhf Wind Profilers In Oklahoma. J. Appl. Meteorol. Climat. 52, 2278–2295. doi:10.1175/Jamc-d-12-0185.1
Giangrande, S. E., Toto, T., Jensen, M. P., Bartholomew, M. J., Feng, Z., Protat, A., et al. (2016). Convective Cloud Vertical Velocity and Mass‐flux Characteristics from Radar Wind Profiler Observations during GoAmazon2014/5. J. Geophys. Res. Atmos. 121 (12), 12891–12913. doi:10.1002/2016JD025303
Greenwald, T. J., and Christopher, S. (2002). Effect of Cold Clouds on Satellite Measurements Near 183 GHz. J. Geophys. Res. 107, 4170. doi:10.1029/2000jd000258
Haddad, Z. S., Sy, O. O., Hristova-Veleva, S., and Stephens, G. L. (20172017). Derived Observations from Frequently Sampled Microwave Measurements of Precipitation-Part I: Relations to Atmospheric Thermodynamics. IEEE Trans. Geosci. Remote Sensing 55 (6), 3441–3453. doi:10.1109/TGRS.2017.2671598
Hamada, A., and Takayabu, Y. N. (2016). Convective Cloud Top Vertical Velocity Estimated from Geostationary Satellite Rapid‐scan Measurements. Geophys. Res. Lett. 43, 5435–5441. doi:10.1002/2016GL068962
Houze, R. A. (2018). 100 Years of Research on Mesoscale Convective Systems. Meteorol. Monogr. 59, 1–117. 53. doi:10.1175/AMSMONOGRAPHS-D-18-0001.1
Houze, R. A., and Betts, A. K. (1981). Convection in GATE. Rev. Geophys. 19 (4), 541–576. doi:10.1029/rg019i004p00541
Jiang, J. H., Yue, Q., Su, H., Reising, S. C., Kangaslahti, P. P., Deal, W. R., et al. (2017). A Simulation of Ice Cloud Particle Size, Humidity, and Temperature Measurements from the TWICE CubeSat. Earth Space Sci. 4, 574–587. doi:10.1002/2017EA000296
Kingma, P. D., and Ba, J. (2014) Adam: A Method for Stochastic Optimization. arXiv preprint arXiv:1412.6980
Kollias, P., Battaglia, A., Tridon, F., Tatarevic, A., Pfitzenmaier, L., and Lamer, K. (2018). The EarthCARE Cloud Profiling Radar (CPR) Doppler Measurements in Deep Convection: Challenges, post-processing, and Science Applications. Remote Sensing of the Atmosphere, Clouds, and Precipitation VII. doi:10.1117/12.2324321
Kollias, P., Tanelli, S., Battaglia, A., and Tatarevic, A. (2014). Evaluation of EarthCARE Cloud Profiling Radar Doppler Velocity Measurements in Particle Sedimentation Regimes. J. Atmos. Oceanic Technol. 31, 366–386. doi:10.1175/JTECH-D-11-00202.1
Kumar, V. V., Jakob, C., Protat, A., Williams, C. R., and May, P. T. (2015). Mass-flux Characteristics of Tropical Cumulus Clouds from Wind Profiler Observations at Darwin, Australia. J. Atmos. Sci. 72 (5), 1837–1855. doi:10.1175/JAS-D-14-0259.1
Lac, C., Chaboureau, J.-P., Masson, V., Pinty, J.-P., Tulet, P., Escobar, J., et al. (2018). Overview of the Meso-NH Model Version 5.4 and its Applications. Geosci. Model. Dev. 11, 1929–1969. doi:10.5194/gmd-11-1929-2018
Lafore, J-P., and Moncrief, R. (1989). A Numerical Investigation of the Organization and Interaction of the Convective and Stratiform Regions of Tropical Squall Lines. J. Atmos. Sci. 46 (4), 521–544. doi:10.1175/1520-0469(1989)046<0521:anioto>2.0.co;2
LeMone, M. A., Zipser, E. J., and Trier, S. B. (1998). The Role of Environmental Shear and Thermodynamic Conditions in Determining the Structure and Evolution of Mesoscale Convective Systems during TOGA COARE. J. Atmos. Sci. 55, 3493–3518. doi:10.1175/1520-0469(1998)055<3493:troesa>2.0.co;2
Luo, Z. J., Jeyaratnam, J., Iwasaki, S., Takahashi, H., and Anderson, R. (2014). Convective Vertical Velocity and Cloud Internal Vertical Structure: An A-Train Perspective. Geophys. Res. Lett. 41, 723–729. doi:10.1002/2013GL058922
Marinescu, P. J., Van Den Heever, S. C., Heikenfeld, M., Barrett, A. I., Barthlott, C., Hoose, C., et al. (2021). Impacts of Varying Concentrations of Cloud Condensation Nuclei on Deep Convective Cloud Updrafts-A Multimodel Assessment. J. Atmos. Sci. 78 (4), 1147–1172. doi:10.1175/JAS-D-20-0200.1
Masson, V., Le Moigne, P., Martin, E., Faroux, S., Alias, A., Alkama, R., et al. (2013). The SURFEXv7.2 Land and Ocean Surface Platform for Coupled or Offline Simulation of Earth Surface Variables and Fluxes. Geosci. Model. Dev. 6, 929–960. doi:10.5194/gmd-6-929-2013
Masunaga, H., and Luo, Z. J. (2016). Convective and Large‐scale Mass Flux Profiles over Tropical Oceans Determined from Synergistic Analysis of a Suite of Satellite Observations. J. Geophys. Res. Atmos. 121, 7958–7974. doi:10.1002/2016JD024753
May, P. T., and Rajopadhyaya, D. K. (1999). Vertical Velocity Characteristics of Deep Convection over Darwin, Australia. Mon. Wea. Rev. 127, 1056–1071. doi:10.1175/1520-0493(1999)127<1056:vvcodc>2.0.co;2
Mlawer, E. J., Taubman, S. J., Brown, P. D., Iacono, M. J., and Clough, S. A. (1997). Radiative Transfer for Inhomogeneous Atmospheres: RRTM, a Validated Correlated-K Model for the Longwave. J. Geophys. Res. 102, 16663–16682. doi:10.1029/97jd00237
Morrison, H., Curry, J. A., and Khvorostyanov, V. I. (2005). A New Double-Moment Microphysics Parameterization for Application in Cloud and Climate Models. Part I: Description. J. Atmos. Sci. 62 (6), 1665–1677. doi:10.1175/jas3446.1
Neumann, P., Düben, P., Adamidis, P., Bauer, P., Brück, M., KornBlueh, L., et al. (2019). Assessing the Scales in Numerical Weather and Climate Predictions: Will Exascale Be the rescue? Phil. Trans. R. Soc. A. 377, 20180148. doi:10.1098/rsta.2018.0148
North, K. W., Oue, M., Kollias, P., Giangrande, S. E., Collis, S. M., and Potvin, C. K. (2017). Vertical Air Motion Retrievals in Deep Convective Clouds Using the ARM Scanning Radar Network in Oklahoma during MC3E. Atmos. Meas. Tech. 10, 2785–2806. doi:10.5194/amt-10-2785-2017
Ovchinnikov, M., Giangrande, S., Larson, V. E., Protat, A., and Williams, C. R. (2019). Dependence of Vertical Alignment of Cloud and Precipitation Properties on Their Effective Fall Speeds. J. Geophys. Res. Atmos. 124, 2079–2093. doi:10.1029/2018JD029346
Padmanabhan, S., Gaier, T. C., Tanner, A. B., Brown, S. T., Lim, B. H., Reising, S. C., et al. (2021). TEMPEST-D Radiometer: Instrument Description and Prelaunch Calibration. IEEE Trans. Geosci. Remote Sensing 59, 10213–10226. doi:10.1109/TGRS.2020.3041455
Pergaud, J., Masson, V., Malardel, S., and Couvreux, F. (2009). A Parameterization of Dry Thermals and Shallow Cumuli for Mesoscale Numerical Weather Prediction. Boundary-layer Meteorol. 132, 83–106. doi:10.1007/s10546-009-9388-0
Pinty, J.-P., and Jabouille, P. (1998). “A Mixed-Phased Cloud Parameterization for Use in a Mesoscale Non-hydrostatic Model: Simulations of a Squall Line and of Orographic Precipitation,” in Conf. On Cloud Physics (WA: Everett), 217–220.
Puech, J., Hermozo, L., Brogniez, H., Chambon, P., Roca, R., Cipolla, V., et al. (2021). SAPHIR-NG High Resolution Microwave Sounder: Towards an Enhanced Observation of the Atmosphere. IEEE Int. Geosci. Remote Sensing Symp. IGARSS, 7689–7692. doi:10.1109/IGARSS47720.2021.9554426
Ray, P. S., Ziegler, C. L., Bumgarner, W., and Serafin, R. J. (2012). Multiple-doppler Radar Observations of Tornadic Storms. Mon. Wea. Rev. 108 (10), 1607–1625. doi:10.1175/1520-0493
Redelsperger, J.-L. (1997). “The Mesoscale Organization of Deep Convection,” in The Physics and Parameterization of Moist Atmospheric Convection. NATO ASI Series (Series C: Mathematical and Physical Sciences). Editor R. K. Smith (Dordrecht: Springer), Vol 505, 59–98. doi:10.1007/978-94-015-8828-7_3
Roca, R., Bouniol, D., and Fiolleau, T. (2020). “On the Duration and Life Cycle of Precipitation Systems in the Tropics,” in Satellite Precipitation Measurement. Advances in Global Change Research. Editors V. Levizzani, C. Kidd, D. Kirschbaum, C. Kummerow, K. Nakamura, and F. Turk (Cham: Springer), Vol 69, 729–744. doi:10.1007/978-3-030-35798-6_14
Roca, R. m., Brogniez, H. l. n., Chambon, P., Chomette, O., Cloché, S., Gosset, M. E., et al. (2015). The Megha-Tropiques mission: a Review after Three Years in Orbit. Front. Earth Sci. 3, 3–17. doi:10.3389/feart.2015.00017
Romps, D. M. (2021). Ascending Columns, WTG, and Convective Aggregation. J. Atmos. Sci. 78, 497–508. doi:10.1175/JAS-D-20.0041.110.1175/jas-d-20-0041.1
Ronneberger, O., Fischer, P., and Brox, T. (2015). “U-net: Convolutional Networks for Biomedical Image Segmentation,” in Medical Image Computing and Computer-Assisted Intervention – MICCAI 2015. MICCAI 2015. Lecture Notes in Computer Science. Editors N. Navab, J. Hornegger, W. Wells, and A. Frangi (Cham: Springer), Vol 9351, 234–241. doi:10.1007/978-3-319-24574-4_28
Rotunno, R., Klemp, J. B., and Weisman, M. L. (1988). A Theory for strong, Long-Lived Squall Lines. J. Atmos. Sci. 45 (3), 463–485. doi:10.1175/1520-0469(1988)045<0463:atfsll>2.0.co;2
Sadeghi, M., Nguyen, P., Hsu, K., and Sorooshian, S. (2020). Improving Near Real-Time Precipitation Estimation Using a U-Net Convolutional Neural Network and Geographical Information. Environ. Model. Softw. 134, 104856. doi:10.1016/j.envsoft.2020.104856
Saunders, R., Hocking, J., Turner, E., Rayer, P., Rundle, D., Brunel, P., et al. (2018). An Update on the RTTOV Fast Radiative Transfer Model (Currently at Version 12). Geosci. Model. Dev. 11, 2717–2737. doi:10.5194/gmd-11-2717-2018
Schubert, W. H., Ciesielski, P. E., and Johnson, R. H. (2018). “Heat and Moisture Budget Analysis with an Improved Form of Moist Thermodynamics,” in Atmospheric and Oceanic Physics, 1–14. arXiv: :1810.11119 [physics].
Schulte, R. M., Kummerow, C. D., Berg, W., Reising, S. C., Brown, S. T., Gaier, T. C., et al. (2020). A Passive Microwave Retrieval Algorithm with Minimal View-Angle Bias: Application to the TEMPEST-D CubeSat Mission. J. Atmos. Ocean. Technol. 37 (2), 197–210. doi:10.1175/JTECH-D-19-0163.1
Schumacher, R. S., and Rasmussen, K. L. (2020). The Formation, Character and Changing Nature of Mesoscale Convective Systems. Nat. Rev. Earth Environ. 1 (6), 300–314. doi:10.1038/s43017-020-0057-7
Seigel, R. B., and van den Heever, S. C. (2013). Squall-line Intensification via Hydrometeor Recirculation. J. Atmos. Sci. 70 (7), 2012–2031. doi:10.1175/JAS-D-12-0266.1
Shen, D., Liu, B., and Li, X. (2019). Sea Surface Wind Retrieval from Synthetic Aperture Radar Data by Deep Convolutional Neural Networks. IEEE Int. Geosci. Remote Sensing Symp., 8035–8038. doi:10.1109/IGARSS.2019.8898542
Skamarock, W. C., Klemp, J. B., Dudhia, J., Gill, D. O., Liu, Z., Berner, J., et al. (2019) A Description of the Advanced Research WRF Version 4. NCAR Tech. Note NCAR/TN-556+STR, 145 pp. doi:10.5065/1dfh-6p97
Stephens, G. L., van den Heever, S. C., Haddad, Z. S., Posselt, D. J., Storer, R. L., Grant, L. D., et al. (2020). A Distributed Small Satellite Approach for Measuring Convective Transports in the Earth's Atmosphere. IEEE Trans. Geosci. Remote Sensing 58, 4–13. doi:10.1109/TGRS.2019.2918090
Sy, O. O., Haddad, Z. S., Stephens, G. L., and Hristova-Veleva, S. (2017). Derived Observations from Frequently Sampled Microwave Measurements of Precipitation. Part II: Sensitivity to Atmospheric Variables and Instrument Parameters. IEEE Trans. Geosci. Remote Sensing 55 (5), 2898–2912. doi:10.1109/TGRS.2017.2656061
Tao, W.-K., Lang, S., Zeng, X., Li, X., Matsui, T., Mohr, K., et al. (2014). The Goddard Cumulus Ensemble Model (GCE): Improvements and Applications for Studying Precipitation Processes. Atmos. Res. 143, 392–424. doi:10.1016/j.atmosres.2014.03.005
Tao, W.-K., and Simpson, J. (1993). Goddard Cumulus Ensemble Model. Part I: Model Description. Terr. Atmos. Oceanic Sci. 4, 35–72. doi:10.3319/tao.1993.4.1.35(a)
Thomas, B., Brandt, M., Walber, A., Philipp, M., Gibson, H., Czekala, H., et al. (2012). Submillimetre-wave Receiver Developments for ICI Onboard MetOP-SG and Ice Cloud Remote Sensing Instruments. IEEE Int. Geosci. Remote Sensing Symp., 1278–1281. doi:10.1109/IGARSS.2012.6351306
Tomassini, L. (2020). The Interaction between Moist Convection and the Atmospheric Circulation in the Tropics. Bull. Am. Meteorol. Soc. 101 (8), E1378–E1396. doi:10.1175/BAMS-D-19-0180.1
Varble, A., Fridlind, A. M., Zipser, E. J., Ackerman, A. S., Chaboureau, J.-P., Fan, J., et al. (2011). Evaluation of Cloud-Resolving Model Intercomparison Simulations Using TWP-ICE Observations: Precipitation and Cloud Structure. J. Geophys. Res. 116 (12), 1–22. doi:10.1029/2010JD015180
Veillette, M. S., Hassey, E. P., Mattioli, C. J., Iskenderian, H., and Lamey, P. M. (2018). Creating Synthetic Radar Imagery Using Convolutional Neural Networks. J. Atmos. Ocean. Technol. 35, 2323–2338. doi:10.1175/jtech-d-18-0010.1
Wang, D., Giangrande, S. E., Feng, Z., Hardin, J. C., and Prein, A. F. (2020). Updraft and Downdraft Core Size and Intensity as Revealed by Radar Wind Profilers: MCS Observations and Idealized Model Comparisons. J. Geophys. Res. 125 (11). doi:10.1029/2019JD031774
Wing, A. A., Stauffer, C. L., Becker, T., Reed, K. A., Ahn, M. S., Arnold, N. P., et al. (2020). Clouds and Convective Self‐Aggregation in a Multimodel Ensemble of Radiative‐Convective Equilibrium Simulations. J. Adv. Model. Earth Syst. 12, e2020MS002138. doi:10.1029/2020MS002138
Zipser, E. J., and LeMone, M. A. (1980). Cumulonimbus Vertical Velocity Events in GATE. Part II: Synthesis and Model Core Structure. J. Atmos. Sci. 37, 2458–2469. doi:10.1175/1520-0469(1980)037<2458:cvveig>2.0.co;2
Keywords: microwave radiometry, time-derivative, convective mass flux, deep convection, detection of convective updrafts, synergy Doppler radar, passive microwave radiometer
Citation: Brogniez H, Roca R, Auguste F, Chaboureau J-P, Haddad Z, Munchak SJ, Li X, Bouniol D, Dépée A, Fiolleau T and Kollias P (2022) Time-Delayed Tandem Microwave Observations of Tropical Deep Convection: Overview of the C2OMODO Mission. Front. Remote Sens. 3:854735. doi: 10.3389/frsen.2022.854735
Received: 14 January 2022; Accepted: 17 February 2022;
Published: 27 April 2022.
Edited by:
Matthew Lebsock, NASA Jet Propulsion Laboratory (JPL), United StatesReviewed by:
Rick Schulte, Colorado State University, United StatesCatherine M. Naud, Columbia University, United States
Copyright © 2022 Brogniez, Roca, Auguste, Chaboureau, Haddad, Munchak, Li, Bouniol, Dépée, Fiolleau and Kollias. This is an open-access article distributed under the terms of the Creative Commons Attribution License (CC BY). The use, distribution or reproduction in other forums is permitted, provided the original author(s) and the copyright owner(s) are credited and that the original publication in this journal is cited, in accordance with accepted academic practice. No use, distribution or reproduction is permitted which does not comply with these terms.
*Correspondence: Hélène Brogniez, aGVsZW5lLmJyb2duaWV6QGxhdG1vcy5pcHNsLmZy