A Global Analysis of the Spatial and Temporal Variability of Usable Landsat Observations at the Pixel Scale
- 1Department of Earth and Environment, Boston University, Boston, MA, United States
- 2Department of Natural Resources and the Environment, University of Connecticut, Storrs, CT, United States
The Landsat program has the longest collection of moderate-resolution satellite imagery, and the data are free to everyone. With the improvements of standardized image products, the flexibility of cloud computing platforms, and the development of time series approaches, it is now possible to conduct global-scale analyses of time series using Landsat data over multiple decades. Efforts in this regard are limited by the density of usable observations. The availability of usable Landsat Tier 1 observations at the scale of individual pixels from the perspective of time series analysis for land change monitoring is remarkably variable both in space (globally) and time (1985–2020), depending most immediately on which sensors were in operation, the technical capabilities of the mission, and the acquisition strategies and objectives of the satellite operators (e.g., USGS, commercial company) and the international ground receiving stations. Additionally, analysis of data density at the pixel scale allows for the integration of quality control data on clouds, cloud shadows, and snow as well as other properties returned from the atmospheric correction process. Maps for different time periods show the effect of excluding observations based on the presence of clouds, cloud shadows, snow, sensor saturation, hazy observations (based on atmospheric opacity), and lack of aerosol optical depth information. Two major discoveries are: 1) that filtering saturated and hazy pixels is helpful to reduce noise in the time series, although the impact may vary across different continents; 2) the atmospheric opacity band needs to be used with caution because many images are removed when no value is given in this band, when many of those observations are usable. The results provide guidance on when and where time series analysis is feasible, which will benefit many users of Landsat data.
Introduction
The provision of free and standardized data, with consistent characteristics since the beginning of the 1980s, has greatly advanced the monitoring of environmental change using remote sensing. Time series analysis of Landsat data that stretches over decades enables a more comprehensive investigation of changes on the land surface—an investigation that is more accurate, more informative, more timely, and that includes information on the timing of change. The result is a shift away from traditional, retrospective change detection based on data acquired over the same area at two or a few points in time to continuous monitoring of the land surface (Woodcock et al., 2020). Previous obstacles related to data storage, preprocessing, and computing power have been largely overcome with the emergence of powerful cloud-computing platforms that provide direct access to the data (Gorelick et al., 2017). Of importance is the availability of decades-long time series of usable reflectance measurements at the level of individual pixels.
Several noteworthy examples exist in the literature of how the availability of satellite data and computing power has advanced our understanding of environmental change. One of the first and perhaps most illustrative examples is the map of global forest change by Hansen et al. (2013). The map was produced by classifying spectral metrics (statistics of surface reflectance such as percentile, mean of percentiles, and slope of linear regression of reflectance) created from time series of Landsat data on a Google computing platform. The ability to create a global 30-m product was made possible only by systematic global image acquisitions of high geometric and radiometric quality available at no direct cost. Another illustrative example is the MapBiomas project (The Brazilian Annual Land Use and Land Cover Mapping Project), which produces annual maps of land use and land cover across Brazil from 1985 onwards by processing large quantities of Landsat data on Google Earth Engine (Souza et al., 2020; MapBiomas, 2021). In addition, many other studies and products have leveraged time series of satellite data and cloud computing to characterize the global land surface, including water and coastlines (Pekel et al., 2016; Mentaschi et al., 2018), land cover and land cover change (Jin et al., 2019; Arévalo et al., 2020; Brown et al., 2020; Homer et al., 2020), forest disturbance (Bullock et al., 2020), agriculture (Deines et al., 2019; Wang et al., 2020), and impervious surfaces (Gong et al., 2020).
Central to the provision of global land-cover products are algorithms that use a combination of time series analysis and machine learning to detect and label changes on the land surface. Time series-based algorithms either operate on annually composited data (e.g., Kennedy et al., 2010), or directly on all the available pixel-level data (e.g., Continuous Change Detection and Classification (CCDC); Zhu and Woodcock, 2014). In either case, all observations not contaminated by clouds and cloud shadows are analyzed. These data-driven approaches to monitoring has enabled the shift away from rudimentary change detection toward a characterization of dynamic ecosystem processes (Kennedy et al., 2014). Consequently, our ability to achieve continuous and comprehensive monitoring depends on the amount of data available for analysis; insufficient amounts of usable observations undermine effective land monitoring.
The Global Land Cover Estimation (GLanCE) mapping process, funded by NASA MEaSURES and implemented at Boston University by the authors, aims to provide a data record of 21st-century global land cover and land-cover change at 30-m resolution (https://sites.bu.edu/measures/). Global maps of land cover and land-cover change from 2000 onwards are currently being produced by applying the CCDC algorithm to Landsat time series data on Google Earth Engine. While the data density is sufficient across most of Earth’s landmasses, we have noticed a marked spatial and temporal variability in our ability to do time series analysis. This variability is a direct result of varying density of usable Landsat observations, which is often limited by the presence of clouds, cloud shadows, and snow but not always—global acquisition strategies and capabilities, the proximity to and history of international receiving stations and the number of operational Landsat sensors in orbit, which are sometimes more important to pixel-level data density than clouds (Ju and Roy, 2008; Kovalskyy and Roy, 2013; Wulder et al., 2015, 2016).
Characterization of the Landsat archive in terms of spatio-temporal coverage and quality of observations (e.g., clouds, atmospheric opacity, and radiometric saturation) is important for scientific studies of the Earth’s surface and global environmental change. The spatio-temporal distribution of Landsat imagery has been previously characterized in several studies (Arvidson et al., 2006; Goward et al., 2006; Ju & Roy, 2008; Kovalskyy & Roy, 2013; Wulder et al., 2016). However, to the best of our knowledge, there have been no recent and systematic global studies of the availability of usable observations in the Landsat archive at the pixel scale, and of the impact of different filtering strategies on data density in space and time.
Here, we present results from a global analysis of usable Landsat Tier 1 observations in Collection 1 at the scale of individual pixels between 1985 and 2020 from the perspective of time series analysis for land change monitoring. We aim to provide the remote sensing community information about when and where time series analysis based on Landsat data is feasible, given the high data variability at the global scale. Further, we intend to raise awareness of the issues of data density variability, and in turn, to call upon the community to highlight, investigate, and publish potential approaches to augment and gapfill time series. We entertain a few ideas in the Discussion Section that can potentially mitigate the problem, and although we are actively researching approaches to filling gaps in coverage, it is outside the scope of this article to present solutions to the problem.
Methods
Landsat Data
We analyzed all available Tier 1 data from 1985 to 2020 in Landsat Collection 1 (C1) for the entire globe produced by the U.S. Geological Survey (USGS) and hosted on Google Earth Engine (GEE) to evaluate the availability and variability of usable observations. The Tier 1 data products analyzed include surface reflectance from Landsat 4 and 5 Thematic Mapper (TM), Landsat 7 Enhanced Thematic Mapper Plus (ETM+), and Landsat 8 Operational Land Imager (OLI).
Landsat 4-7 C1 surface reflectance products were generated using Landsat Ecosystem Disturbance Adaptive Processing System (LEDAPS) (version 3.4.0) (Masek et al., 2006). The C Version of Function of Mask (CFmask) was applied to define the attributes of the pixel qualities, which identified each pixel as clear (land/water), snow, cloud, adjacent to cloud, or cloud shadow (along with levels of confidence) (Zhu and Woodcock, 2012; Zhu et al., 2015). Landsat 8 OLI C1 Surface Reflectance was generated using the Land Surface Reflectance Code (LaSRC) (version 1.4.1) (Vermote et al., 2016). Although Landsat 8 allows for better detection of high-altitude, thin cirrus and wispy clouds (Shi et al., 2021), the structure of the Quality Assessments (QA) bands of the two products are identical. In addition to the pixel QA, both products are accompanied with radiometric saturation and atmospheric opacity layers.
The USGS assigns Landsat data with the highest quality to Tier 1, and to Tier 2 if not meeting Tier 1 criteria, which is primarily based on the geometric accuracy of the image registration (Townshend et al., 1992). All analyses presented in this paper were based on Tier 1 data as they are considered more suitable for time series analysis (https://www.usgs.gov/landsat-missions/landsat-collection-1). To investigate the data availability within certain time intervals at the pixel-scale, we followed the product guides provided by USGS and evaluated each pixel based on its 1) Pixel Quality Assessment Bit Index (pixel_qa); 2) Radiometric Saturation Quality Assessment (radsat_qa) Bit Index, and 3) Atmospheric Opacity (sr_atmos_opacity) band/aerosol attributes.
Filtering Scenarios
To explore the effects of multiple filtering strategies on the global availability of usable Landsat observations at an interannual scale, we produced maps of the annual number-of-observations from 1985 to 2020 for the entire globe based on the following scenarios at the pixel scale: 1) no filters; 2) Pixel QA filter; 3) Radiometric saturation and unrealistic reflectance (out-of-range reflectance values) filter; 4) Atmospheric opacity filter. The three filters were applied sequentially to assess the impact of each on data availability.
First, we retrieved all available surface reflectance images from 1985 to 2020 hosted on GEE and counted the total number of images for each year. At this step, no filter was applied to the pixels. In essence, this result is what one gets when doing the analysis at the scale of entire images rather than pixels.
Second, the pixels were filtered using the pixel QA bands. Each Landsat image has a QA band, which allows users to filter the data based on the attributes, including fill pixel, clear, water, snow/ice, cloud, cloud shadow, and cloud extent in the imagery (with indicators of confidence). Landsat 8 data also has an indicator for cirrus clouds. Dilated clouds are initially included in the cloud category by marking a few pixels at the edge of the high confidence clouds as clouds (USGS, 2020a; USGS, 2020b; USGS, 2020c). Our objective was to discard all pixels contaminated by cloud, cloud shadow, and snow/ice, and only use the remaining “clear” observations. We therefore constructed a filter that identified pixels labeled as “clear” or “water” with low-confidence cloud in the QA band (Table 1).

TABLE 1. Pixel_qa values of “clear” pixels for Landsat 4, 5, 7, and 8 [extracted from Landsat 8 Collection 1 (C1) Land Surface Reflectance Code (LaSRC) Product Guide (USGS, 2020a; USGS, 2020b; USGS, 2020c)].
Third, we also investigated the impact of radiometric saturation. The Radiometric Saturation Quality Assessment (radsat_qa) band indicates whether observations are saturated in any bands when collected. Saturation was expected to influence Landsat 4, 5, and 7 data more due to limited radiometric resolution (0–255 DN range; 8-bit) compared to Landsat 8 OLI with a 12-bit radiometric resolution (Irons et al., 2012; Roy et al., 2016).
A value of 0 in the radsat_qa band indicates valid data, and 1 indicates saturation. We discarded pixels flagged as saturated; however, saturation sometimes occurs in very bright pixels even if flagged as valid. The surface reflectance values of such pixels are set to a filled value of 20,000 (scale factor of 0.0001). Note that the “filled saturated value” is set to the spectral band, not the radsat_qa band.
In addition, valid surface reflectance values are theoretically in the range of 0–1, but overestimation of aerosols can lead to negative surface reflectance values (Masek et al., 2006). Other causes of out-of-range values include Landsat calibration errors and instrument artifacts not accommodated for by the Landsat calibration (Roy et al., 2014). The Lambertian surface assumption is one of the explanations for surface reflectance greater than 1 (Ju and Roy, 2008; Roy et al., 2014). Thus, we also removed pixels outside the valid range for surface reflectance (0–1).
Finally, we investigated filtering based on atmospheric aerosols. One of the major differences between LEDAPS and LaSRC was the method used to retrieve aerosol optical thickness (AOT). For Landsat 4, 5, and 7, the atmospheric opacity band is generated by LEDAPS and stored as a number between 0 and 10. The greater the atmospheric opacity, the higher the AOT. We followed the product guide (<0.1 = clear; 0.1–0.3 = average; > 0.3 = hazy) and removed the pixels with sr_atmos_opacity greater than 0.3. For Landsat 8, the aerosol QA band represents the state of the atmosphere retrieved by LaSRC (Table 2). We excluded pixels classified as high aerosol content and kept low- and medium-level aerosol pixels as recommended by the product guide.
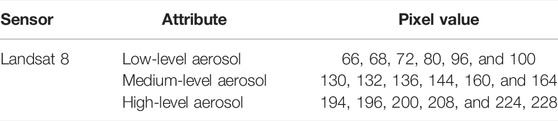
TABLE 2. Landsat 8 internal surface reflectance aerosol quality assessment (sr_aerosol) bit index (USGS, 2020b).
Caution is needed when using the sr_atmos_opacity band because LEDAPS may fail to generate atmospheric opacity values due to no Dark Dense Vegetation (DDV) (USGS, 2022c). If there is no atmospheric opacity retrieval available, the value for sr_atmos_opacity band is set to “Not Available” (or NA). However, on GEE, instead of having “NA” value for the sr_atmos_opacity band, pixels are simply “masked”, which could cause problems when the implementation applies only a threshold without proper handling.
In addition to annual maps of usable observations, we also produced the average annual number of observations globally for the entire Landsat archive in four time periods to investigate the impact of Landsat eras and the historical data acquisition strategy: 1) 1985–1999 focuses on the data density before the 21st-century with Landsat 4 and 5 in operation; 2) 2000–2003 shows the data availability before the failure of the Scan Line Corrector (SLC) on Landsat 7 (i.e., SLC-on); 3) 2004–2012 captures the effect of SLC-off data from Landsat 7; and 4) 2013–2020 when Landsat 8 is available, which was expected to largely improve data availability.
The counting of usable observations was done at both 30-m and 900-m spatial resolutions with the latter calculated as the mean value of the 30-m pixels inside each 900-m pixel. For better visualization, only the 900-m results are shown in this paper.
Results
Figure 1 shows the number of images acquired by the satellites before any filtering. Only small amounts of data were collected for Australia, Oceania, Southern Africa in the late 1980s (SupplementaryFigure S3 for annual maps). Limited numbers of images are available for Siberia, Central and West Africa, and the southernmost areas of South America until the launch of Landsat 7 in 1999. Since 2000, there are 20 or more images collected per year for most of the globe, except for Central and West Africa, Russia, Kazakhstan, and India, where less than five images were collected annually. After the launch of Landsat 8 in 2013, 20 or more images per year were collected for most locations on Earth. Notable exceptions are the northern part of South America and West and Central Africa that are still experiencing low data acquisition density. These areas exhibit high cloud cover which limits the ability for images to be registered within the requirements for Tier 1.
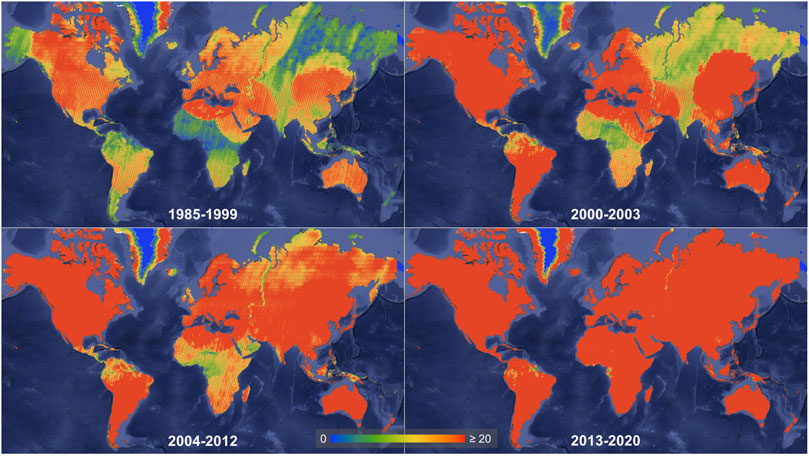
FIGURE 1. The global average value of the annual number of observations for all images in Landsat archive (TM, ETM+, and OLI data) in four time periods 1) 1985–1999 (upper-left); 2) 2000–2003 (upper-right); 3) 2004–2012 (bottom-left); 4) 2013–2020 (bottom-right).
Filtering by pixel quality removes observations contaminated by cloud (also dilated cloud), cloud shadows, and snow/ice. After pixel-quality filtering, large amounts of data are lost at high latitudes because of the presence of snow and ice, while tropical regions such as the Amazon basin, Southeast Asia, and West Africa lose data due to the frequent presence of clouds (Figure 2A). Overall, 1985–1999 has the lowest data availability, and the Landsat-8 era has the highest. Spatially, hotspots of high data density vary little across different periods; in places like Ecuador, Western Colombia, and the North region of Brazil, and West and Central Africa the data density remained low even in the Landsat-8 era. Figure 2B shows the number of images removed by the pixel-quality filter. Except for the Sahara Desert, Saudi Arabia, and parts of Australia, the pixel quality filter reduces the data density by at least 30% for most of the world (Supplementary Figure S1). The large numbers of observations filtered at this stage in the Landsat 8 era are largely due to the increased number of total images collected.
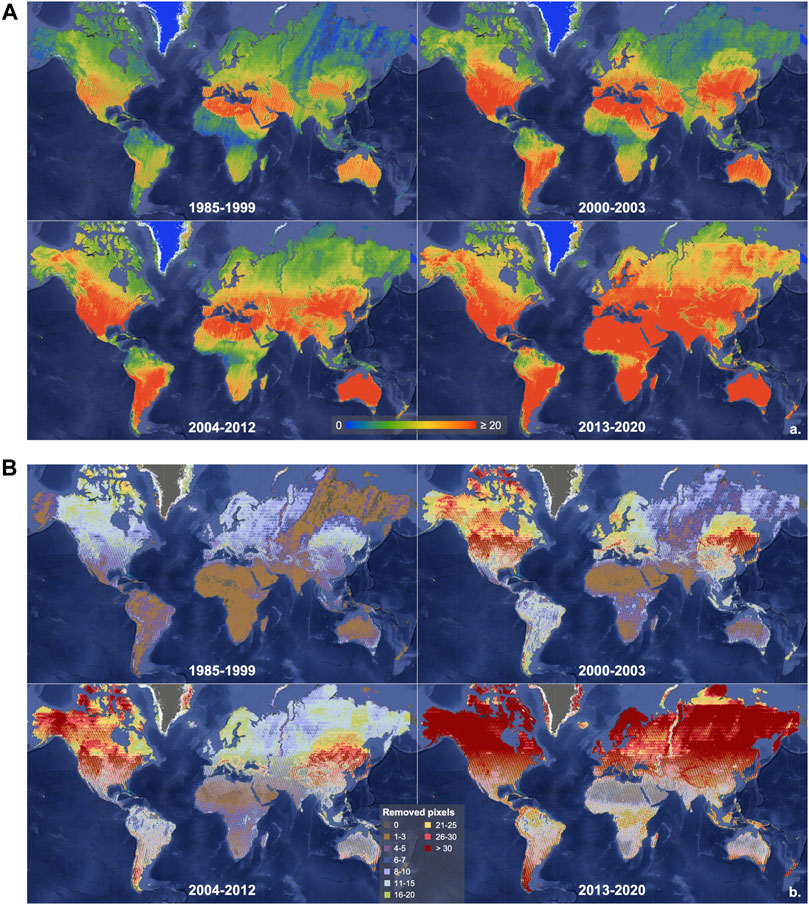
FIGURE 2. (A)Average number of clear observations per year in four time periods 1) 1985–1999 (upper-left); 2) 2000–2003 (upper-right); 3) 2004–2012 (bottom-left); 4) 2013–2020 (bottom-right). (B) Average number of observations removed per year by applying the pixel quality filter. 1) 1985–1999 (upper-left); 2) 2000–2003 (upper-right); 3) 2004–2012 (bottom-left); 4) 2013–2020 (bottom-right).
Investigation of the data availability after filtering by radiometric saturation followed the investigation of pixel-quality filtering. Figure 3A shows the number of observations that remained after both radiometric saturation and pixel quality filtering. Very few images were removed by the radiometric saturation filter (Figure 3B). China, Saudi Arabia, and South America have more saturated/out-of-range pixels found between 2000 and 2003 than other periods, but still less than 10% of observations were removed (Supplementary Figure S2).
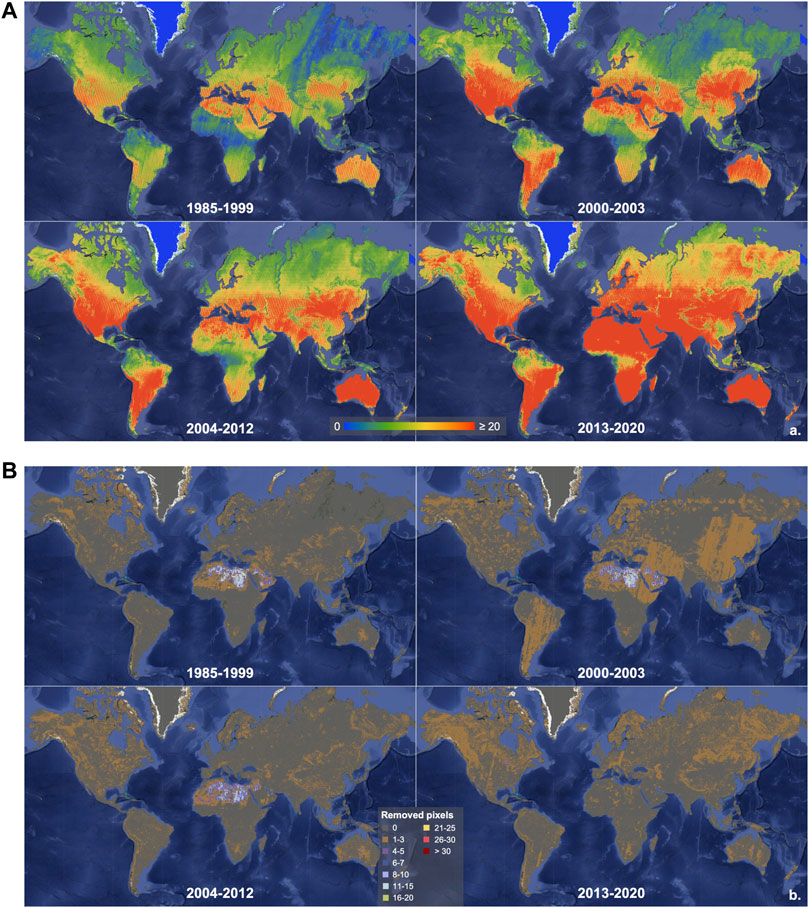
FIGURE 3. (A)Average number of observations per year, filtered out cloud, cloud shadow, snow, saturated, and unrealistic SR pixels. (B) Average number of observations removed per year by filtering pixels with unrealistic SR and saturation. The difference is between maps in Figures 2A, 3A.
High AOT can be the result of missed clouds or haze which, if present in the data, complicates time series analysis. Figures 4A,B show that the AOT filter has a very limited impact in arid environments whereas Southeast China and India exhibited a lot of haze in 2013–2020, which reduced the data availability by 30%. Another hotspot was found in the Amazon Basin, where organic aerosol serve as the condensation nuclei for water vapor and trigger the formation of fog and clouds (Pöschl et al., 2010). Some of those clouds were missed by Fmask. Similarly, warm and humid air above Gabon and Congo rising from the forest cools in the upper air and causes a cloudy and hazy atmosphere (NASA Earth Observatory, 2019). Consequently, the data availability in these regions worsen when applying the AOT filter on top of the previous filters. Overall, Western Canada, Southeast China, India, and Southeast Asia lost over 30% of images from the AOT filter (Supplementary Figure S4).
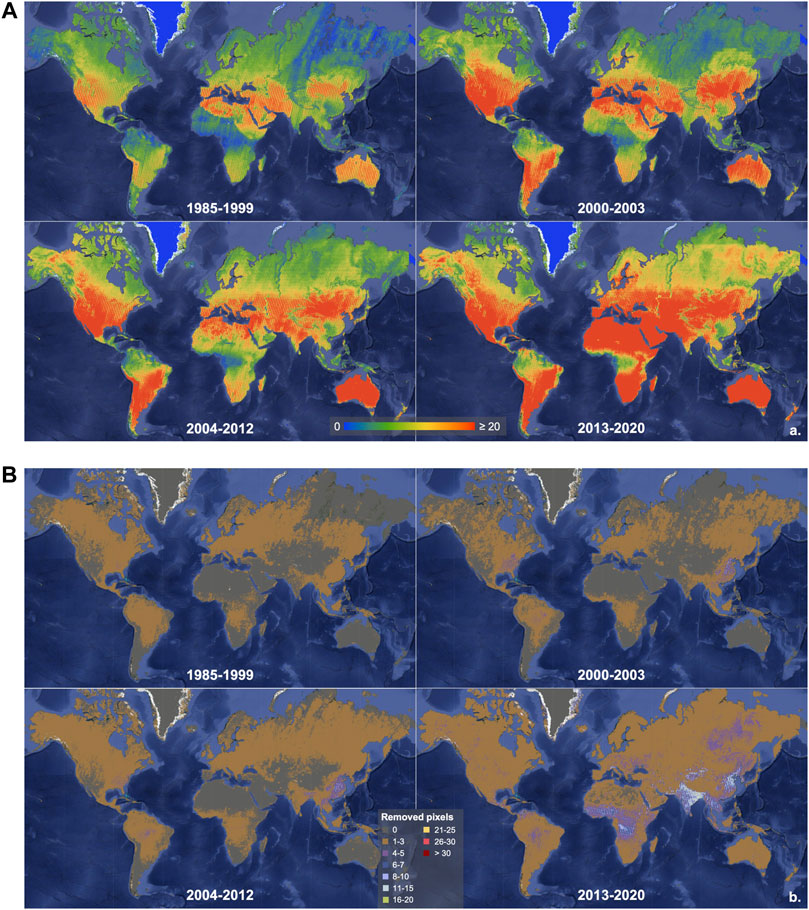
FIGURE 4. (A) Average number of annual observations after filtering cloud, cloud shadow, snow, saturated, unrealistic SR, and hazy atmospheric pixels. (B) Average number of observations removed per year by filtering pixels with a hazy atmosphere. The difference is between maps in Figures 3A, 4A.
The atmospheric opacity band was helpful for identifying hazy pixels. However, on GEE, simply applying a threshold to the sr_atmos_opacity band without consideration of the pixels missing atmospheric opacity information (AOT = “masked”) caused over-filtering. Figures 5A,B show that the Sahara region and Saudi Arabia lost about 20 images per year because of improper use of the AOT filter. The result is that no surface reflectance data exist between 1985 and 2012. Other dry regions such as South Australia, the Xinjiang Province in China, Mongolia, and the Middle East, lost more than 30% of available data because of the AOT filter.
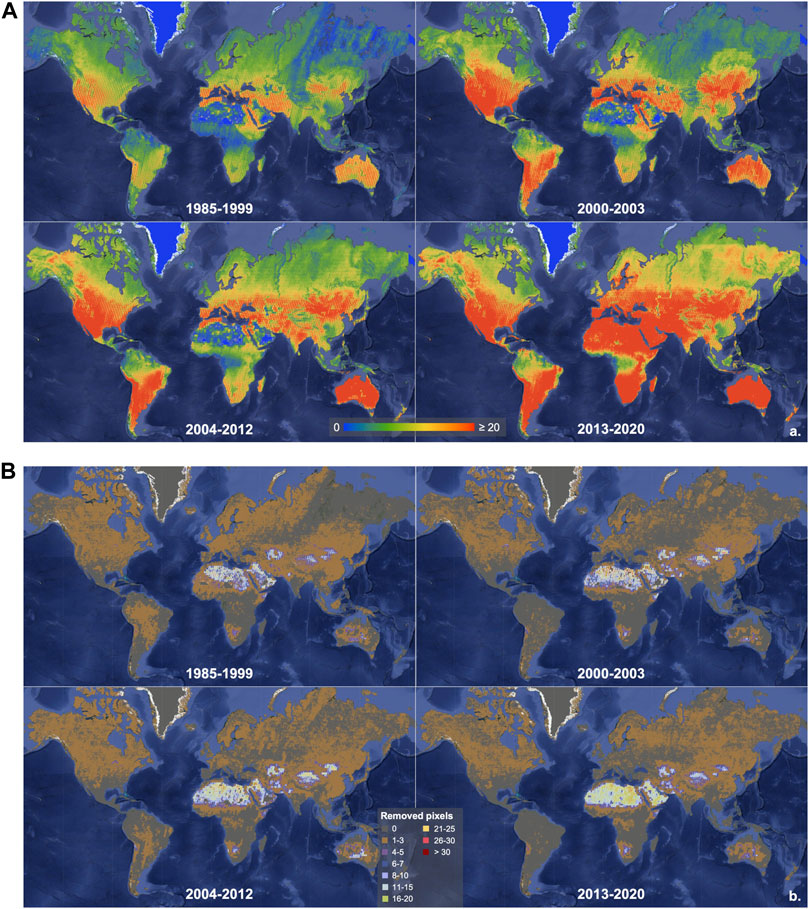
FIGURE 5. (A) Average number of annual observations when using the sr_atmos_opacity band without considering the LEDAPS failing pixels. (B) Average number of observations removed per year when no AOT information is available. The difference is between maps in Figures 4A, 5A.
All the 900-m results can be accessed through the link to the Google Earth Engine [Asset ID: projects/GLANCE/RESULTS/NOBS/Global_900_C1_S0 (Figure 1), /Global_900_C1_S1 (Figure 2), Global_900_C1_S3 (Figure 3), Global_900_C1_S4 (Figure 4)]. Due to the large size of results in 30-m resolution, only number of observation maps of the last filtering strategy shown in Figure 4 are available on GEE at: projects/glance-public-data/assets/FILTERING_S3, projects/glance-public-dataX/assets/FILTERING_S3 (X = 1,2,...,7).
Discussion
Spatio-Temporal Variation of Usable Data Density
Open access to historical Landsat data has enabled a paradigm shift away from static change detection based on two or a few points in time to continuous monitoring of the land surface. The advantages of such monitoring are many: information on the timing, magnitude, and duration of land-surface activities (White et al., 2022; Zhang et al., 2022); an unprecedented ability to inform on more subtle changes in ecosystem condition and land-use dynamics (Bullock et al., 2020; Myers-Smith et al., 2020); and a general increase in the accuracy and precision of remote sensing-based products (Woodcock et al., 2020). At the heart this paradigm shift is time series analysis, which are techniques concerned with analyzing the dependency of adjacent observations taken sequentially in time (Box et al., 2015). In this study we found that the density of historical Landsat observations in pixel-level time series vary greatly across the globe both in space and in time. That the amount of usable observations collected by an optical sensor varies with cloud cover is obvious, but the combined impact of the operation of receiving stations, sensor longevity, and acquisition plans on data availability is substantial and surprising. Adding to the impact is the lack of estimates of the aerosol optical depth over deserts, which results in insufficient information for atmospheric correction, and in turn, a marked reduction of imagery in mostly cloud-free areas. Similar spatial patterns were found when applied the same filtering strategy to Landsat Collection 2 data. We believe that knowledge of the global variability of data density will inform users of Landsat data about the feasibility of data-intensive approaches to monitoring. We also want to highlight the importance of global data collection when designing acquisition plans and satellite missions.
For the first era shown in Figure 1, only Landsat 5 was operating for the entire period from 1985 to 1999, with Landsat 4 operation ending in 1993. It had compromised communications capabilities because of the failure of the Tracking and Data Relay Satellite System (TDRSS) link. As a result, images could only be acquired and downloaded when they were in direct view of an international ground station. It is also important to note that most of this era was during the commercialization period. The provider did not acquire images on a routine basis for the purpose of building an archive but rather acquired images when there were sales potential. From 2000 to 2012, the USGS started building the global archive and acquired Landsat 5 based on that policy. With Landsat 7 launched in 1999, there were two satellites that were operating, which allowed the possibility of 8-day coverage. In addition, Landsat 7 used the long-term acquisition plan (LTAP) developed by Arvidson et al. (2006) that pursued the goal of collecting a clear scene for every season across the globe every year. From 2013 forward there were two satellites collecting imagery and both with strong acquisition plans that have been pushed to the limits by the USGS.
Implication for Time Series Analysis
We used CCDC to investigate the impact of the different filtering strategies on the time series analysis because we wanted to study the impact of data variability on change detection results generated by time series analysis. The challenges of using the various algorithms available are not identical but we tried to make the results as generic as possible. In short, we used the observations filtered by the QA band as a baseline, and the scenario of using other filters as a test case (e.g., QA versus QA and AOT filters; QA versus QA and saturation filters), and computed the Root Mean Square Error (RMSE) from different CCDC outputs, as adding noise to a time series should increase the RMSE.
The variability of usable observation may cause significantly different results for time series analysis. Taking the images filtered by the QA band as a baseline, and the scenario of using AOT>0.3 filter (only QA and AOT filters) as a test case, the time series shows that more noisy observations exist if no AOT filter is applied, mainly in highly vegetated areas. Figure 6 is an example of agricultural fields in China and Figure 7 is an example of a dense forest in the Amazon. In these two figures, the inclusion of noisy observations triggered spurious breaks detected by CCDC with the higher RMSE, which could be avoided by using a stricter filtering strategy. According to the high-resolution images on Google Earth, the example pixel in Figure 6 has been agricultural land since 1985. However, without AOT filtering, the model reached “three times the RMSE” and detected a break in 2013 (shown in red and blue curves in Figure 6), but no break when using AOT filtering (shown in black curve). Another example in Figure 7 shows that the time series after 2008 was split into three short segments without AOT filtering, even though a clear-cut once occurred and there was no land cover change during the vegetation regrowth. Therefore, using the AOT>0.3 can remove noisy observations and result in more reliable change detection results.
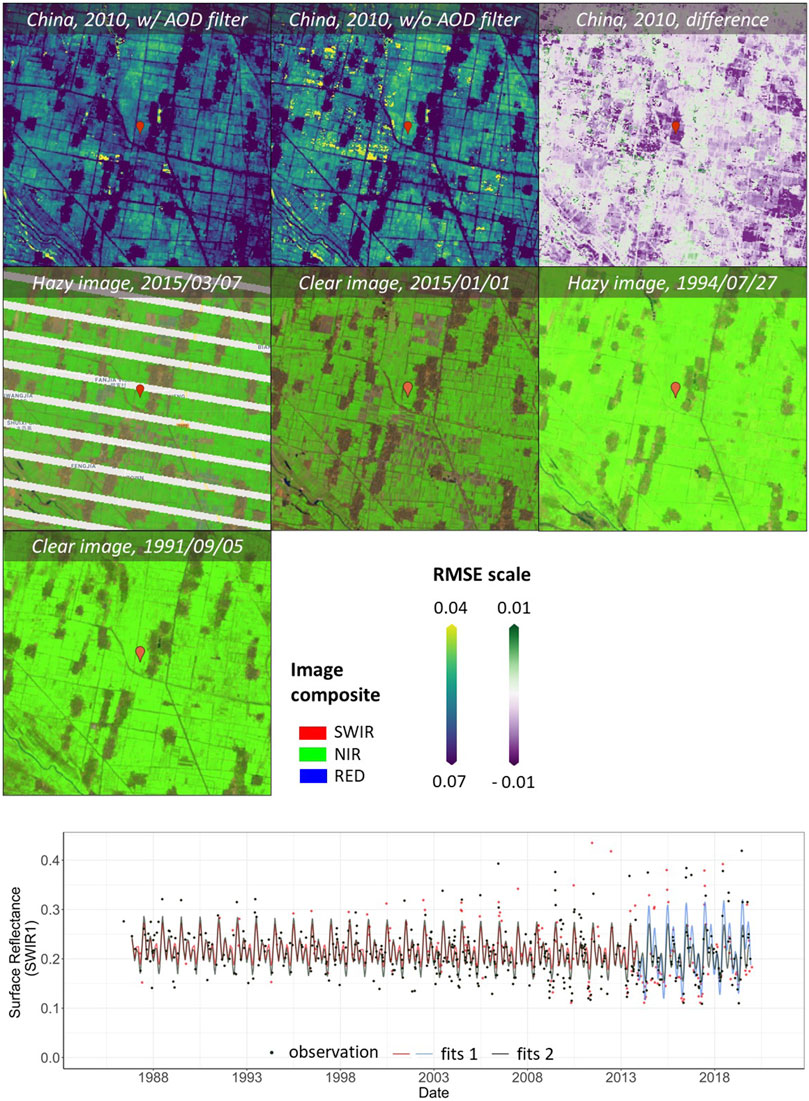
FIGURE 6. The top row are Root Mean Square Error (RMSE) maps from CCDC for 2010 in Henan Province, China. From the left to right, they are RMSE maps with an AOT>0.3 filter, without any AOT filtering, and the difference between the two results. The remaining thumbnails are Landsat images on specific dates. The first two are during the winter and the other two are from the summer. The time series plot includes two cases: black dots and black curves correspond to CCDC model fits using AOT filter; red dots and red/blue curves correspond to CCDC model fits without using AOT filter (Lat: 34.2023; Lon: 114.3525).
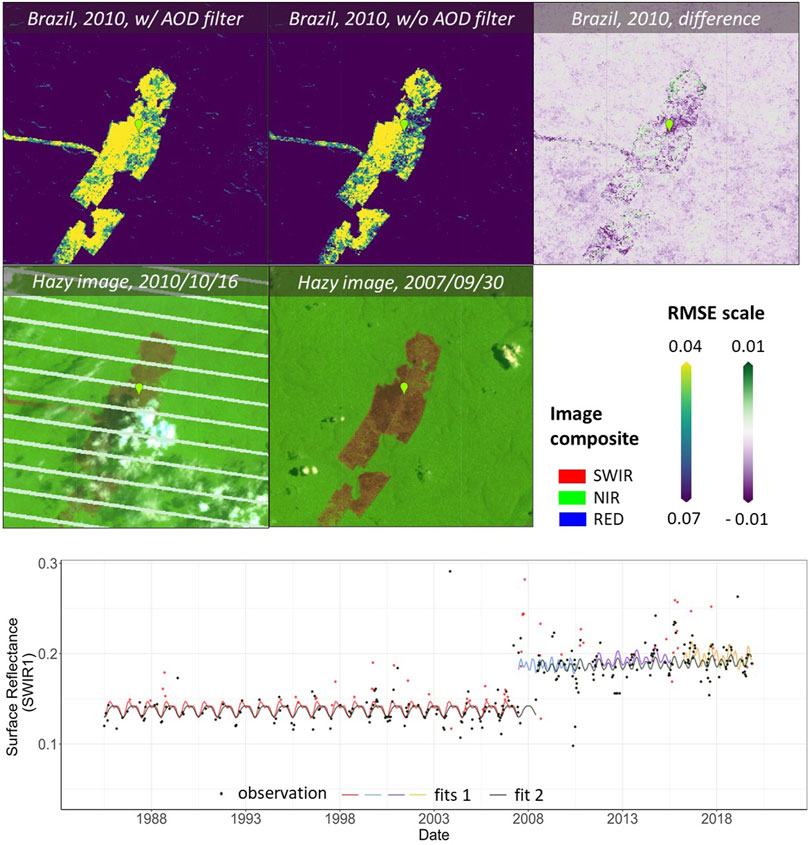
FIGURE 7. The first row is the Root Mean Square Error (RMSE) map of the CCDC model for 2010 near the Amazon Forest. From the left to right, they are RMSE maps with AOT filter, without AOT filter, and the difference between using AOT filter or not. The two image chips are Landsat imageries on specific dates. The time series plot includes two cases: black dots and black curves correspond to CCDC model fits using AOT filter; red dots and red/blue/purple/yellow curves correspond to CCDC model fits without using AOT filter (Lat: −5.7818; Lon: −59.8746).
Figure 5A shows that almost no observations were left for arid regions before the Landsat 8 era without including images where LEDAPS failed to run, although they could still contain useful data for time series analysis (examples in Figure 8). The filtering of no AOT pixels eliminated many usable observations, which either resulted in no model fits due to very sparse observations, or inaccurate model predictions (Figures 8, 9). The other test was to investigate the impact of including saturated observations or irregular surface reflectance values, and Figure 10 illustrates the necessity of excluding those observations at least in arid regions.
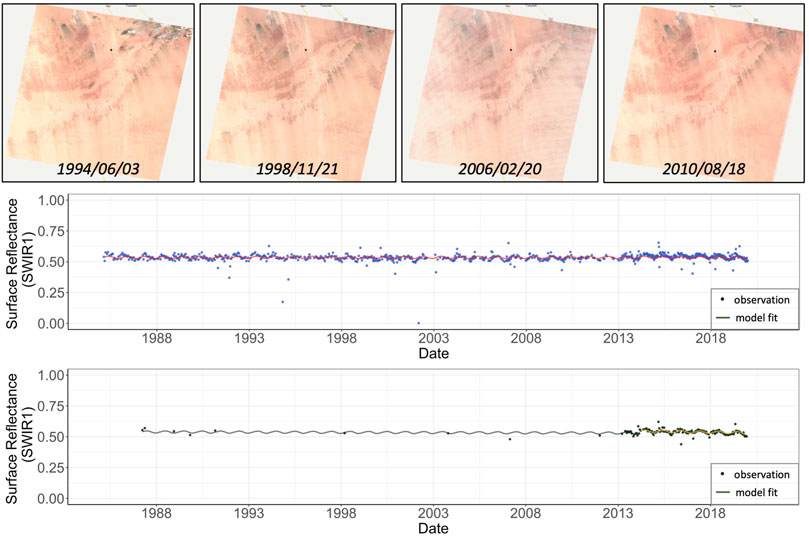
FIGURE 8. The time series plot of using over-filtering AOT observations (lower panel) versus the regular AOT filtering strategy (middle panel) and example of usable Landsat images (upper panel). No saturation filtering was applied here.
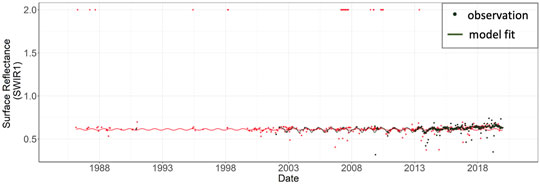
FIGURE 9. The time series plot of using over-filtering AOT observations (in black) versus the regular AOT filtering strategy (in red) (Lat: 18.9903; Lon: −14.7481). Note that no saturation filtering was applied here.
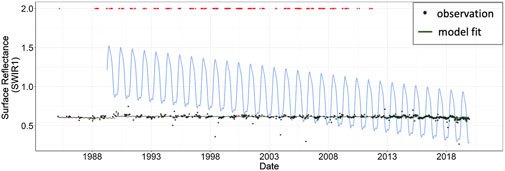
FIGURE 10. The time series plot of using the baseline observations to fit the model (only QA band filtering; red dots with red/blue curves) versus the saturation filtering (black dots and black curves) (Lat: 21.7886; Lon: 31.8935).
However, given the great variability of the data density, this is still a balance between applying the filters and considering available images. We suggest putting the data availability as the top priority, since some low data density places may be at the edge of having insufficient observations to obtain a reliable land cover type or even detect a land cover change.
Alternative Approaches When Data Density is Insufficient for Time Series Analysis
The results of this study, which make evident that the density of Landsat observations are highly variable, raise the question: how to effectively perform time series analysis and continuous monitoring in areas of limited data density? Algorithm development and testing is outside the scope of this paper but the issue is important. Also, there is a wide variety in the necessary number of observations needed for time series analysis. For example, Zhang et al., 2022) recommended a density of at least 7 clear observations per year to perform a good time series analysis result. However, except for the United States, most of the world does not meet the requirements until Landsat 8 was launched. Some countries such as Ecuador, Colombia, and Gabon have less than 7 clear observations per year even after 2014. If we lower the recommendation to 4 clear observations per year, only parts of Siberia and West Africa do not reach the threshold from 2000 to 2013. If data are simply lacking, there is nothing obvious that can be done. Fortunately, such places are rare. In such cases, creating pixel-level composites using available historical Landsat data is an option (Potapov et al., 2012). (Qiu, 2021) investigated a range of different approaches to compositing and found that no single compositing scheme is optimal in all situations but that the data application in combination with the spectral and spatial conditions dictate the choice of compositing algorithm. In general, Qiu et al. (2022) found the best-available-pixel (BAP; White et al., 2014) and maximum NDVI compositing schemes to perform better in most situations.
Still, even if compositing is possible in areas of low data density, it is unlikely that the composites would be dense enough to enable monitoring algorithms such as LandTrendr and CCDC. This fact in turn raises the question: what should users do in cases where the data density is insufficient only in parts of the study area? For example, time series-based algorithms including CCDC (Arévalo et al., 2020), CODED (Bullock et al., 2020), and LandTrendr (Murillo-Sandoval et al., 2021) have been successfully applied in the Colombian Amazon to study environmental change. However, none of these algorithms can be used along the Pacific coast of Colombia, where not a single usable Landsat observation is available for several years. Should users who need to study the entire country apply time series-based approaches in parts of the country and resort to alternatives such as multi-temporal classification of composites in other parts? Or instead aim for consistency by using the same mapping approach across the entire study area? These are outstanding questions that need answering.
Finally, while we have shown that acquisition policies and plans, satellite capabilities or limitations, and receiving station activities impact data availability, clouds are still a major obstacle to achieving sufficient data density, especially in the Landsat-8/Sentinel-2 era. Spaceborne radar instruments offer a solution as radar data are largely unaffected by clouds. Traditionally, the collection of radar data was not uniform and the data not free, which have hampered the use of radar data for monitoring. The situation changed with the launch of Sentinel-1A and B in 2014 and 2016. While the frequency, polarization, and mode of the data collected by Sentinel-1 vary in space and time, the collection is more uniform and frequent than previous radar missions—and importantly, the data is free and preprocessed. However, using radar data alone for monitoring environmental change has proven challenging for a number of technical reasons, which is why the remote sensing community is striving to combine optical and radar data (Reiche et al., 2018; Holden, 2019). Attempts to achieve “interoperability” between optical and radar data have largely failed because the two types of instruments are in essence measuring different phenomena (reflected sunlight vs. backscattered radio waves). An approach more likely to succeed is data fusion where the data streams are analyzed separately but used to provide information on a common score or metric (Tang et al., 2022). Successful fusion of optical and radar data would alleviate much of the observed variability in Landsat-data density after 2014.
Conclusion
Overall, the current acquisition status and strategies of the Landsat program enable sufficient observations for time series analysis at the global scale, even though studies of land cover change face some significant challenges. With multiple filtering strategies, we presented the usable observations in the Landsat archive and how that varies in space and time. The question of how much of the globe has coverage that is suitable for time series analysis versus areas that are deficient were discussed in the previous section. Answering this question depends on the data density, the spatial-temporal scale of the study, and combining multiple time series analysis approaches.
In this paper, we have reported the following key findings:
• The combined impact of the operation of receiving stations, satellite capabilities, sensor longevity, and acquisition plans and policies on the amount of usable Landsat data is substantial. This impact was more pronounced during the 1980s and 90s when only Landsat-5 was in orbit and less after the launch of Landsat-7 and −8 when we had consistent 8-day repeat cycles.
• Clouds, cloud shadows, and snow/ice impact data availability across Earth but more so in the tropics. Of importance is the impact of cloud contamination in addition to other factors: data availability is sufficient for time series analysis in cloudy regions such as Amazon and Southeast Asia where much historical data have been collected, as opposed to regions where the compound effect of clouds, receiving stations, and acquisition plans have created a situation where time series analysis is difficult or even impossible (e.g., West Africa).
• Radiometric saturation or unrealistic values of surface reflectance (i.e., less than zero or greater than one) resulted in additional loss of data, especially between 2000 and 2003, but the impact on data availability was considerably less than the impact described in the two bullet points above.
• Missing data is also a consequence of very high values of AOT as witnessed in certain parts of the world, primarily in India and China. Failure of the atmospheric correction algorithm over many arid areas leads to no value for AOT in the metadata. If observations with No AOT value are filtered, large amounts of data are lost over arid regions. Conversely, missing data is also a consequence of very high values of AOT as witnessed in certain parts of the world, primarily in India and China.
Data Availability Statement
The original contributions presented in the study are included in the article/Supplementary Material, further inquiries can be directed to the corresponding author.
Author Contributions
YZ, CW, and MF conceived the research. YZ did the bulk of the analysis, with contributions from PA. YZ and PO did most of the writing, and all authors contributed text.
Funding
This research was supported by the NASA Making Earth System Data Records for Use in Research Environments (MEaSUREs) program (grant number 80NSSC18K0994), and Landsat Science Team funding (grant number 140G118C0006).
Conflict of Interest
The authors declare that the research was conducted in the absence of any commercial or financial relationships that could be construed as a potential conflict of interest.
Publisher’s Note
All claims expressed in this article are solely those of the authors and do not necessarily represent those of their affiliated organizations, or those of the publisher, the editors and the reviewers. Any product that may be evaluated in this article, or claim that may be made by its manufacturer, is not guaranteed or endorsed by the publisher.
Acknowledgments
The authors would like to thank Thomas R. Loveland and Christopher P. Barber for their contribution of insights regarding observed patterns of data availability.
Supplementary Material
The Supplementary Material for this article can be found online at: https://www.frontiersin.org/articles/10.3389/frsen.2022.894618/full#supplementary-material
References
Arévalo, P., Bullock, E. L., Woodcock, C. E., and Olofsson, P. (2020). A Suite of Tools for Continuous Land Change Monitoring in Google Earth Engine. Front. Clim. 2, 576740. doi:10.3389/fclim.2020.576740
Arvidson, T., Goward, S., Gasch, J., and Williams, D. (2006). Landsat-7 Long-Term Acquisition Plan. Photogramm. Eng. remote Sens. 72 (10), 1137–1146. doi:10.1016/j.rse.2019.01.01310.14358/pers.72.10.1137
Box, G. E., Jenkins, G. M., Reinsel, G. C., and Ljung, G. M. (2015). Time Series Analysis: Forecasting and Control. John Wiley & Sons.
Brown, J. F., Tollerud, H. J., Barber, C. P., Zhou, Q., Dwyer, J. L., Vogelmann, J. E., et al. (2020). Lessons Learned Implementing an Operational Continuous United States National Land Change Monitoring Capability: The Land Change Monitoring, Assessment, and Projection (LCMAP) Approach. Remote Sens. Environ. 238, 111356. doi:10.1016/j.rse.2019.111356
Bullock, E. L., Woodcock, C. E., Souza, C., and Olofsson, P. (2020). Satellite‐based Estimates Reveal Widespread Forest Degradation in the Amazon. Glob. Change Biol. 26 (5), 2956–2969. doi:10.1111/gcb.15029
Deines, J. M., Kendall, A. D., Crowley, M. A., Rapp, J., Cardille, J. A., and Hyndman, D. W. (2019). Mapping Three Decades of Annual Irrigation across the US High Plains Aquifer Using Landsat and Google Earth Engine. Remote Sens. Environ. 233, 111400. doi:10.1016/j.rse.2019.111400
Gong, P., Li, X., Wang, J., Bai, Y., Chen, B., Hu, T., et al. (2020). Annual Maps of Global Artificial Impervious Area (GAIA) between 1985 and 2018. Remote Sens. Environ. 236, 111510. doi:10.1016/j.rse.2019.111510
Gorelick, N., Hancher, M., Dixon, M., Ilyushchenko, S., Thau, D., and Moore, R. (2017). Google Earth Engine: Planetary-Scale Geospatial Analysis for Everyone. Remote Sens. Environ. 202, 18–27. doi:10.1016/j.rse.2017.06.031
Goward, S., Arvidson, T., Williams, D., Faundeen, J., Irons, J., and Franks, S. (2006). Historical Record of Landsat Global Coverage: Mission Operations, NSLRSDA, and International Cooperator Stations. Photogramm. Eng. 15, 1155–1169. doi:10.14358/PERS.72.10.1155
Hansen, M. C., Potapov, P. V., Moore, R., Hancher, M., Turubanova, S. A., Tyukavina, A., et al. (2013). High-resolution Global Maps of 21st-Century Forest Cover Change. Science 342 (6160), 850–853. doi:10.1126/science.1244693
Holden, C. E. (2019). Enhancing Landsat Time Series through Multi-Sensor Fusion and Integration of Meteorological Data. Doctoral dissertation, Boston University. Boston: Boston University. Available at: https://open.bu.edu/handle/2144/34913.
Homer, C., Dewitz, J., Jin, S., Xian, G., Costello, C., Danielson, P., et al. (2020). Conterminous United States Land Cover Change Patterns 2001-2016 from the 2016 National Land Cover Database. ISPRS J. Photogrammetry Remote Sens. 162, 184–199. doi:10.1016/j.isprsjprs.2020.02.019
Irons, J. R., Dwyer, J. L., and Barsi, J. A. (2012). The Next Landsat Satellite: The Landsat Data Continuity Mission. Remote Sens. Environ. 122, 11–21. doi:10.1016/j.rse.2011.08.026
Jin, S., Homer, C., Yang, L., Danielson, P., Dewitz, J., Li, C., et al. (2019). Overall Methodology Design for the United States National Land Cover Database 2016 Products. Remote Sens. 11 (24), 2971. doi:10.3390/rs11242971
Ju, J., and Roy, D. P. (2008). The Availability of Cloud-free Landsat ETM+ Data over the Conterminous United States and Globally. Remote Sens. Environ. 112 (3), 1196–1211. doi:10.1016/j.rse.2007.08.011
Kennedy, R. E., Andréfouët, S., Cohen, W. B., Gómez, C., Griffiths, P., Hais, M., ., , Healey, S. P., Helmer, E. H., Hostert, P., Lyons, M. B., Meigs, G. W., Pflugmacher, D., Phinn, S. R., Powell, S. L., Scarth, P., Sen, S., Schroeder, T. A., Schneider, A., Sonnenschein, R., Vogelmann, J. E., Wulder, M. A., and Zhu, Z. (2014). Bringing an Ecological View of Change to Landsat-Based Remote Sensing. Front. Ecol. Environ. 12 (6), 339–346. doi:10.1890/130066
Kennedy, R. E., Yang, Z., and Cohen, W. B. (2010). Detecting Trends in Forest Disturbance and Recovery Using Yearly Landsat Time Series: 1. LandTrendr - Temporal Segmentation Algorithms. Remote Sens. Environ. 114 (12), 2897–2910. doi:10.1016/j.rse.2010.07.008
Kovalskyy, V., and Roy, D. P. (2013). The Global Availability of Landsat 5 TM and Landsat 7 ETM+ Land Surface Observations and Implications for Global 30m Landsat Data Product Generation. Remote Sens. Environ. 130, 280–293. doi:10.1016/j.rse.2012.12.003
MapBiomas, (2021). Collection 6 of the Annual Land Use Land Cover Maps of Brazil. Availableat: https://mapbiomas.org/(Accessed on October 12, 2021).
Masek, J. G., Vermote, E. F., Saleous, N. E., Wolfe, R., Hall, F. G., Huemmrich, K. F., ., , Gao, F., Kutler, J., and Lim, T.-K. (2006). A Landsat Surface Reflectance Dataset for North America, 1990-2000. IEEE Geosci. Remote Sens. Lett. 3 (1), 68–72. doi:10.1109/LGRS.2005.857030
Mentaschi, L., Vousdoukas, M. I., Pekel, J.-F., Voukouvalas, E., and Feyen, L. (2018). Global Long-Term Observations of Coastal Erosion and Accretion. Sci. Rep. 8 (1), 12876. doi:10.1038/s41598-018-30904-w
Murillo-Sandoval, P. J., Gjerdseth, E., Correa-Ayram, C., Wrathall, D., Van Den Hoek, J., Dávalos, L. M., et al. (2021). No Peace for the Forest: Rapid, Widespread Land Changes in the Andes-Amazon Region Following the Colombian Civil War. Glob. Environ. Change 69, 102283. doi:10.1016/j.gloenvcha.2021.102283
Myers-Smith, I. H., Kerby, J. T., Phoenix, G. K., Bjerke, J. W., Epstein, H. E., Assmann, J. J., et al. (2020). Complexity Revealed in the Greening of the Arctic. Nat. Clim. Chang. 10 (2), 106–117. doi:10.1038/s41558-019-0688-1
NASA Earth Observatory (2019). Cloudy Congo River Basin. Availableat: https://earthobservatory.nasa.gov/images/144608/cloudy-congo-river-basin.
Pekel, J.-F., Cottam, A., Gorelick, N., and Belward, A. S. (2016). High-resolution Mapping of Global Surface Water and its Long-Term Changes. Nature 540 (7633), 418–422. doi:10.1038/nature20584
Pöschl, U., Martin, S. T., Sinha, B., Chen, Q., Gunthe, S. S., Huffman, J. A., et al. (2010). Rainforest Aerosols as Biogenic Nuclei of Clouds and Precipitation in the Amazon. science 329 (5998), 1513–1516. doi:10.1126/science.1191056
Potapov, P. V., Turubanova, S. A., Hansen, M. C., Adusei, B., Broich, M., Altstatt, A., et al. (2012). Quantifying Forest Cover Loss in Democratic Republic of the Congo, 2000-2010, with Landsat ETM+ Data. Remote Sens. Environ. 122, 106–116. doi:10.1016/j.rse.2011.08.027
Qiu, S., Zhu, Z., and Woodcock, C. E. (2020). Cirrus Clouds that Adversely Affect Landsat 8 Images: what Are They and How to Detect Them? Remote Sens. Environ. 246, 111884. doi:10.1016/j.rse.2020.111884
Reiche, J., Hamunyela, E., Verbesselt, J., Hoekman, D., and Herold, M. (2018). Improving Near-Real Time Deforestation Monitoring in Tropical Dry Forests by Combining Dense Sentinel-1 Time Series with Landsat and ALOS-2 PALSAR-2. Remote Sens. Environ. 204, 147–161. doi:10.1016/j.rse.2017.10.034
Roy, D. P., Kovalskyy, V., Zhang, H. K., Vermote, E. F., Yan, L., Kumar, S. S., et al. (2016). Characterization of Landsat-7 to Landsat-8 Reflective Wavelength and Normalized Difference Vegetation Index Continuity. Remote Sens. Environ. 185, 57–70. doi:10.1016/j.rse.2015.12.024
Roy, D. P., Wulder, M. A., Loveland, T. R., C.E., W., Allen, R. G., Anderson, M. C., et al. (2014). Landsat-8: Science and Product Vision for Terrestrial Global Change Research. Remote Sens. Environ. 145, 154–172. doi:10.1016/j.rse.2014.02.001
Souza, C. M., Z. Shimbo, J., Parente, L. L., A. Alencar, A., Rudorff, B. F. T. A., Hasenack, H., et al. (2020). Reconstructing Three Decades of Land Use and Land Cover Changes in Brazilian Biomes with Landsat Archive and Earth Engine. Remote Sens. 12 (17), 2735. doi:10.3390/rs12172735
Tang, X., Bratley, K. H., Cho, K., Bullock, E. L., Olofsson, P., and Woodcock, C. E. (2022). Near Real-Time Monitoring of Tropical Forest Disturbance by Fusion of Landsat, Sentinel-2, and Sentinel-1 Data. Remote Sensing of Environment.
Townshend, J. R. G., Justice, C. O., Gurney, C., and McManus, J. (1992). The Impact of Misregistration on Change Detection. IEEE Trans. Geosci. Remote Sens. 30 (5), 1054–1060. doi:10.1109/36.175340
U.S. Geological Survey USGS (2020a). Landsat 4-7 Collection 1 (C1) Surface Reflectance (LEDAPS) Product Guide. Availableat: https://d9-wret.s3.us-west-2.amazonaws.com/assets/palladium/production/s3fs-public/atoms/files/LSDS-1370_L4-7_C1-SurfaceReflectance-LEDAPS_ProductGuide-v3.pdf.
U.S. Geological Survey USGS (2022b). Landsat 8 Collection 1 (C1) Land Surface Reflectance Code (LaSRC) Product Guide. Availableat: https://d9-wret.s3.us-west-2.amazonaws.com/assets/palladium/production/s3fs-public/atoms/files/LSDS-1368_L8_C1-LandSurfaceReflectanceCode-LASRC_ProductGuide-v3.pdf.
U.S. Geological Survey USGS (2022c). Landsat Collection 2 Known Issues. Availableat: https://www.usgs.gov/landsat-missions/landsat-collection-2-known-issues.
Wang, S., Di Tommaso, S., Deines, J. M., and Lobell, D. B. (2020). Mapping Twenty Years of Corn and Soybean across the US Midwest Using the Landsat Archive. Sci. Data 7 (1), 307. doi:10.1038/s41597-020-00646-4
White, J. C., Hermosilla, T., Wulder, M. A., and Coops, N. C. (2022). Mapping, Validating, and Interpreting Spatio-Temporal Trends in Post-disturbance Forest Recovery. Remote Sens. Environ. 271, 112904. doi:10.1016/j.rse.2022.112904
White, J. C., Wulder, M. A., Hobart, G. W., Luther, J. E., Hermosilla, T., Griffiths, P., ., , Coops, N. C., Hall, R. J., Hostert, P., Dyk, A., and Guindon, L. (2014). Pixel-based Image Compositing for Large-Area Dense Time Series Applications and Science. Can. J. Remote Sens. 40 (3), 192–212. doi:10.1080/07038992.2014.945827
Woodcock, C. E., Loveland, T. R., Herold, M., and Bauer, M. E. (2020). Transitioning from Change Detection to Monitoring with Remote Sensing: A Paradigm Shift. Remote Sens. Environ. 238, 111558. doi:10.1016/j.rse.2019.111558
Wulder, M. A., Hilker, T., White, J. C., Coops, N. C., Masek, J. G., Pflugmacher, D., et al. (2015). Virtual Constellations for Global Terrestrial Monitoring. Remote Sens. Environ. 170, 62–76. doi:10.1016/j.rse.2015.09.001
Wulder, M. A., White, J. C., Loveland, T. R., Woodcock, C. E., Belward, A. S., Cohen, W. B., et al. (2016). The Global Landsat Archive: Status, Consolidation, and Direction. Remote Sens. Environ. 185, 271–283. doi:10.1016/j.rse.2015.11.032
Zhang, Y., Woodcock, C. E., Chen, S., Wang, J. A., Sulla-Menashe, D., Zuo, Z., et al. (2022). Mapping Causal Agents of Disturbance in Boreal and Arctic Ecosystems of North America Using Time Series of Landsat Data. Remote Sens. Environ. 272, 112935. doi:10.1016/j.rse.2022.112935
Zhu, Z., Wang, S., and Woodcock, C. E. (2015). Improvement and Expansion of the Fmask Algorithm: Cloud, Cloud Shadow, and Snow Detection for Landsats 4-7, 8, and Sentinel 2 Images. Remote Sens. Environ. 159, 269–277. doi:10.1016/j.rse.2014.12.014
Zhu, Z., and Woodcock, C. E. (2014). Continuous Change Detection and Classification of Land Cover Using All Available Landsat Data. Remote Sens. Environ. 144, 152–171. doi:10.1016/j.rse.2014.01.011
Keywords: landsat, time series, data density, pixel quality, land change, land cover
Citation: Zhang Y, Woodcock CE, Arévalo P, Olofsson P, Tang X, Stanimirova R, Bullock E, Tarrio KR, Zhu Z and Friedl MA (2022) A Global Analysis of the Spatial and Temporal Variability of Usable Landsat Observations at the Pixel Scale. Front. Remote Sens. 3:894618. doi: 10.3389/frsen.2022.894618
Received: 11 March 2022; Accepted: 26 May 2022;
Published: 21 June 2022.
Edited by:
Eric Vermote, National Aeronautics and Space Administration (NASA), United StatesReviewed by:
Sergii Skakun, University of Maryland, United StatesAndrii Shelestov, Kyiv Polytechnic Institute, Ukraine
Copyright © 2022 Zhang, Woodcock, Arévalo, Olofsson, Tang, Stanimirova, Bullock, Tarrio, Zhu and Friedl. This is an open-access article distributed under the terms of the Creative Commons Attribution License (CC BY). The use, distribution or reproduction in other forums is permitted, provided the original author(s) and the copyright owner(s) are credited and that the original publication in this journal is cited, in accordance with accepted academic practice. No use, distribution or reproduction is permitted which does not comply with these terms.
*Correspondence: Yingtong Zhang, zhangyt@bu.edu