- 1Earth Observation Group, Payne Institute for Public Policy, Colorado School of Mines, Golden, CO, United States
- 2Cooperative Institute for Research in the Environmental Sciences, University of Colorado, Boulder, Boulder, CO, United States
- 3Space Research Institute, Moscow, Russia
- 4Colorado School of Mines, Payne Institute for Public Policy, Golden, CO, United States
- 5Department of Geography, University of Denver, Denver, CO, United States
- 6Institut de Recherche Pour le Développement (IRD), Marseille, France
- 7New Light Technologies, Incorporated, Washington D.C., United States
The history of satellite remote sensing of nighttime lights traces it is roots back the U.S. Air Force Defense Meteorological Satellite Program (DMSP) which began flying polar orbiting satellites with cloud imaging sensors in the 1970’s. The meteorologists found that they could improve weather predictions with visible and thermal band imagery. The visible band channels on early DMSP satellites worked well in the daytime—but were unable to see clouds at nights. Visible band low light imaging was added in the mid-1970’s using photomultiplier tubes to amplify the signal, enabling the detection of moonlit clouds. In 1992, NOAA established a digital archive for DMSP data at the National Geophysical Data Center in Boulder, Colorado. The Earth Observation Group (EOG) was formed in 1994 and dedicated it is efforts to making global nighttime light data products for uses by the science and policy communities. Many of the shortcomings of DMSP data were addressed with the NASA/NOAA Visible Infrared Imaging Radiometer Suite (VIIRS) day/night band (DNB), which opened a new era in nighttime light studies. In total, EOG has produced 65 annual global nighttime light products and over 650 monthly products.
Introduction
Satellite observed nighttime lights (NTL) are among the most widely recognized satellite data products, providing a visual expression of humanity’s presence across the Earth’s surface (Figure 1). Over the years, NTL have emerged as a core data product for scientific communities—meteorologists (Miller et al., 2012), economists (Chen and Nordhaus, 2019), biologists (Gaston, 2018), astronomers (Falchi et al., 2016), and others. The utilization of satellite observed nighttime lights in the analysis of landscape dynamics may seem incongruous since the sensor is observing the radiant emissions of electric lighting rather than a traditional landscape component. However, researchers have utilized the location and brightness of lights, along with their changes through time, as proxies for a range of landscape related phenomena that would be difficult to map directly on a global basis (Zhao et al., 2019; Levin et al., 2020). The concept is that lighting will only be installed and maintained for sites having built infrastructure. New infrastructure brings new lighting and abandoned or demolished infrastructure no longer merits the lighting it previously had.
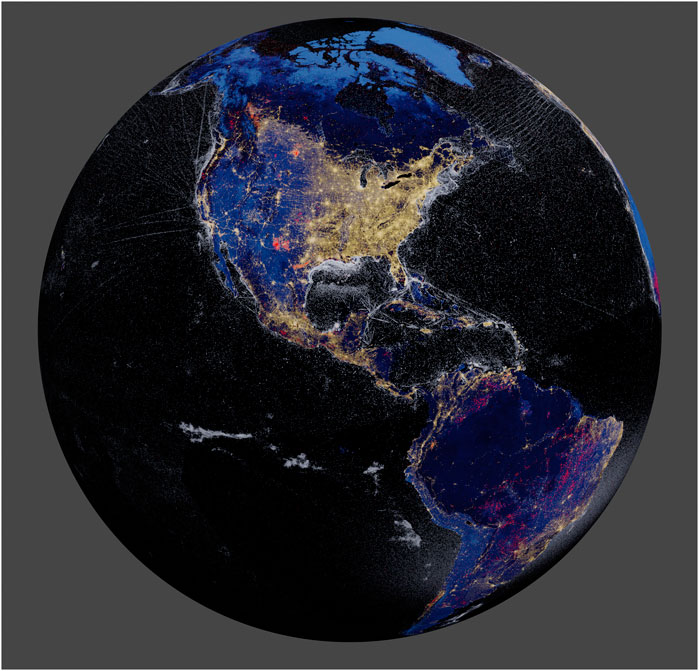
FIGURE 1. EOG’s 2015 v. 1 annual VIIRS nighttime lights projected on a sphere. Golden, electric lighting; Red, fires and flares; Pale gray, boats.
Some caution should be applied to using lights for landscape studies in conflict zones, immediately after natural disasters or during power grid failures. For example, Figure 2 shows the loss of lighting in Lebanon on 9 October 2021 associated with the collapse of the country’s power grid after its two main power plants ran out of fuel (Chulov, 2021). Such outages tend to get “averaged out” in the monthly and annual cloud-free composites, which are the preferred product types for landscape related studies.
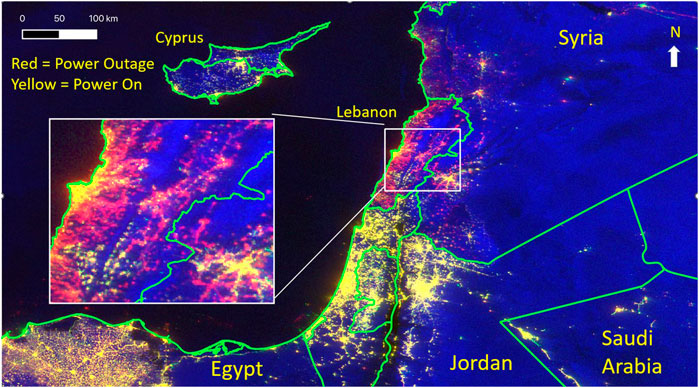
FIGURE 2. A VIIRS observation of power outages in Lebanon the night of 9 October 2021. The image is a color composite of the 2020 average as red, October 9 DNB as green, and October 9 thermal as blue (inverted so clouds look bright).
A classic example of landscape analysis based on nighttime lights is modeling of the spatial distribution and density of constructed materials (Elvidge et al., 2007a; Sutton et al., 2009). This type of analysis relies on an empirical calibration for estimating the percent cover of constructed materials based on the brightness of satellite observed lights. The calibration invariably points to small levels of constructed surfaces from dim lighting and high levels of constructed surfaces when lighting is bright. Other examples of such proxy uses for nighttime lights have been published for urban heat island effects (Gallo and Owen, 1998; Zhang et al., 2010; Peng et al., 2012), urban and exurban mapping (Small et al., 2013; Goldblatt et al., 2018; Xie et al., 2019), habitat fragmentation (Crooks et al., 2017; Jacobson et al., 2019) and the global human footprint (Sanderson et al., 2002; Venter et al., 2016).
The underlying low-light imaging data collections stretch back to the early 1970’s, making them one of the longest records of Earth observations from space. This paper describes the origin of the collection capabilities from the U.S. Air Force, the state of preservation, the resulting nighttime lights time series, and recent findings from the NASA/NOAA Visible Infrared Imaging Radiometer Suite (VIIRS) day/night band (DNB).
Origins of low light imaging on meteorological sensors and phenomenology
The origins of nightly global low-light imaging traces its roots back to the mid-1970’s when photomultiplier tubes (PMTs) became integrated with traditional thermal and visible sensors on cloud-imaging instruments flown in polar orbits by the U.S. Air Force Defense Meteorological Satellite Program (DMSP) (Nichols, 1975; Lieske, 1981; Westinghouse Electric Corporation, 1989; Goyette, 1992; Spangler, 2003). Prior to this, DMSP flew ten weather satellites from 1965 to 1971 carrying short (0.2–4 µm) and long wavelength thermal (7–12 µm) video cameras—with no low-light imaging capabilities. Air Force meteorologists made extensive use of the video camera data, doing their analyses with side-by-side images of the short wavelength and thermal images. Clouds are generally dark (cold) in the thermal and bright in short wavelength channel in daylit passes due to reflected sunlight. The detection limits of the short wavelength channel worked well during the day, but was unable to detect clouds at night. Thus, only the thermal video camera produced useable data at night. The value of simultaneously acquired short and longwave data was clear to the Air Force meteorologists, which led to the formulation of a requirement for nighttime visible imagery of clouds.
Engineers determined that with sufficiently low detection limits, clouds could be imaged at night in the visible and near infrared (VNIR) using moonlight instead of sunlight as the illumination source. This would require vastly lower detection limits, since moonlight is 400,000 times dimmer than sunlight. To address this requirement, the next generation DMSP cloud-imager included a photomultiplier tube (PMT) for VNIR signal intensification. The first PMT equipped instruments collecting nightly global low-light imaging data were the DMSP Block 5A, 5B, and 5C. This early low light imaging instrument’s name was “Sensor Aerospace Vehicle Equipment Package” (SAP), which were flown multiple times from 1970 to 1976 (Spangler, 2003). Unfortunately, none of the digital SAP data were preserved. SAP data were written to ten-inch-wide film for interpretation by the meteorologists. It is possible to find limited numbers of reproductions of the SAP film images showing nighttime lights, aurora, and gas flares.
In the mid-1970’s, DMSP developed a new cloud-imaging sensor known as the Operational Linescan System (OLS), which have flown in a series on the DMSP block 5D satellites (Goyette, 1992), from 1976 to the present—more than 45 years. The OLS instruments had the same two spectral bands as the SAP, including the PMT for low-light imaging and featured several new design features to improve data quality. The OLS is an oscillating telescope collecting individual lines of data as it swings from left to right and back again, collecting data in both scan directions. The swath width is 3,000 km and with fourteen orbits per day, global coverage is acquired twice per day. The OLS features a sinusoidal scan motion, which keeps the scanlines parallel to each other and perpendicular to the satellite’s track. The PMT’s electron beam is magnetically switched for first and final quarter of each scanline to constrain the size of the edge-of-scan pixel footprints. The switching results in a brief signal jump, leaving a pair of vertical lines marking the outer quarter panels (Figure 3). Figure 3 includes several typical nighttime OLS phenomena, such as city lights, lightning, and lighting blur induced by optically thin clouds. Lightning results in horizontal streaks of light that in some cases persist for several scanlines. In addition, OLS images record stray light contaminated data when the spacecraft is sunlit while collecting on a dark Earth below, a phenomena referred to as glare (Figure 4). The extremely low detection limits makes it possible for the OLS to record atmospheric glow associated with aurora (Figure 5). Early descriptions of the OLS’ low-light imaging phenomenology are describe in a series of paper by Croft (Croft, 1978; Croft and Colvocoresses, 1979; Croft, 1981).
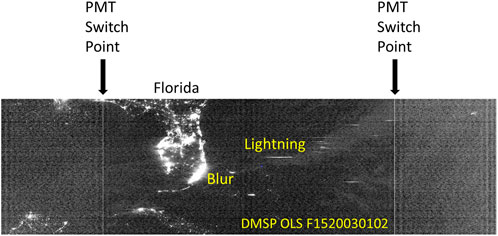
FIGURE 3. Nighttime visible band OLS data collected over Florida in 2003. There are a pair of vertical lines marking the PMT switch points. The switch point pixels are slightly brighter than pixels to either side. Data outside the switch points have larger pixel footprints and are noisier than the data near nadir. EOG’s v.4 DMSP time series exclude the switch point and outer panel pixels.
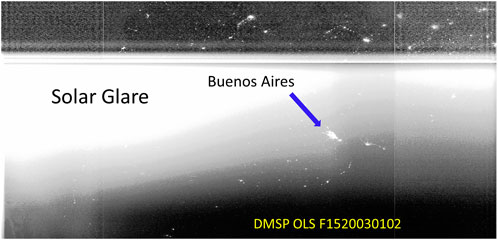
FIGURE 4. Solar glare occurs when the DMSP spacecraft is sunlit and the Earth surface is in night. EOG discards the glare contaminated data for nighttime lights product generation.
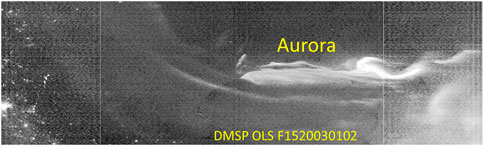
FIGURE 5. Aurora features in nighttime OLS visible band data. As with glare—EOG marks and excludes aurora contaminated orbital segments from nighttime lights product generation.
While the OLS collected improved low-light imaging data as compared to the SAP—there are several shortcomings that affect the quality of DMSP nighttime lights data products (Elvidge et al., 2013): 1) Coarse spatial resolution and overlapping pixel footprints. The OLS collects data with a nominal ground-sample distance (GSD) of 0.5 km, the so-called “fine” resolution data. The fine data are “smoothed” by on-board aggregation of 5 × 5 pixel blocks, resulting in pixels spaced 2.7 km apart (ground sample distance). Limited quantities of fine resolution data were brought down—focused on regions of interest to the Department of Defense. It is important to note that the OLS visible band pixel footprints are much larger than the GSD, meaning that there is substantial overlap in the ground footprints of adjacent pixels. 2) Limited dynamic range. The OLS VIS band is limited to 6-bit quantization, recording digital numbers ranging from 0 to 63.3) Saturation on bright lights. Since the operational mission is as a cloud-imager, the OLS’ gain runs high to enable the detection of moonlit clouds. This results in frequent saturation on the bright cores of urban centers, with DN = 63.4) Lack of in-flight calibration. While the thermal band data are calibrated with an on-board blackbody, there is no in-flight calibration for the VNIR band. There are pre-flight radiance calibrations for the VNIR—but these are of limited value since the gain states are difficult to determine and sensor degradations are not systematically tracked.
The standard DMSP constellation has two satellites—one in a day-night orbit and the other in a dawn-dusk orbit. Figure 6 shows the equatorial crossing time of the SAP and OLS sensors versus time. Note that over time the initial DMSP orbits degrade, creating trace sinusoidal tracks. This contrasts with the stability of the VIIRS overpass times, attributable to the precision orbital control of the SNPP and NOAA-20 satellites. Our experience is that satellites with equatorial crossing times between 19:30 and 04:30 local times collect enough nighttime data in a year to generate annual nighttime light products.
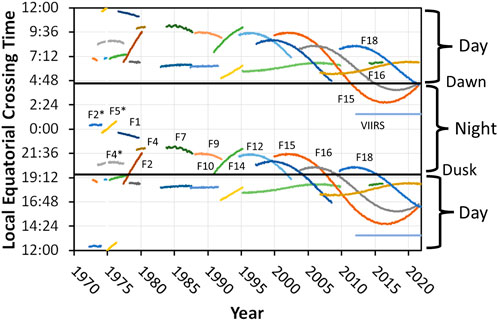
FIGURE 6. DMSP and VIIRS equatorial crossing times over time. Gradually, DMSP orbits decay, resulting in sinusoidal curves over time. In contrast—the VIIRS maintains a constant 01:30 and 13:30 equatorial crossing. The DMSP satellites are named with “F” or flight numbers. The three earliest flights labeled on the chart are from DMSP block 5B, collecting SAP data (F2*, F4* and F5*). The other flights (F1-F18) are from DMSP block 5D, collecting OLS data. The early DMSP satellites had short lifespans. Starting with DMSP F12, lifespans extended—resulting in both early evening and pre-dawn nighttime OLS collections from the F15, F16, and F18 satellites.
Many of the shortcoming of the OLS are addressed with the day/night band (DNB) collecting VNIR data on the NASA/NOAA Visible Infrared Imaging Radiometer Suite. The DNB collects low, medium and high gain VNIR data simultaneously from separate focal planes (Baker, 2011). The gain stages are known as LGS, MGS and HGS respectively. Time delay integration (TDI) is used to intensify the signal in the high gain setting. The DNB maintains a fixed pixel footprint of 742 m on a side from nadir to edge-of-scan by gradually reducing the number of detectors aggregated to form pixels. An onboard algorithm examines the pixels from the three gain settings and records the one having the best signal. In general, LGS pixels are selected in daytime, MGS pixels are selected across the terminator (transition from night to day), and HGS pixels are selected at night. The DNB is calibrated in-flight using a solar diffuser. The DNB’s dark current is set via space looks. These changes vastly improve the quality of the VIIRS low-light imaging data as compared to DMSP. The DNB HGS has lower detection limits than the OLS PMT, making it possible to detect smaller and dimmer light sources. The DNB pixel footprints are 42 times smaller than the OLS’ PMT pixel footprints (Elvidge et al., 2013), which results in a vast increase in the spatial detail for lighting present in urban areas.
The OLS and DNB have similar detection limits and share many of the same detection phenomena: lights, fires, stray light, and aurora. The sensors have a remarkable capability for the detection of combustion sources above 1000 K that traces back to their extremely low detection limits (Figure 7). In addition, with biomass burning there is a prominent Potassium emission centered at 767 nm (Vodacek et al., 2002)—near the transmission peak for both systems. The Potassium line (Figure 8) provides a 5–10% boost in the radiance that cannot be accounted for based on the size and temperature of the fire.
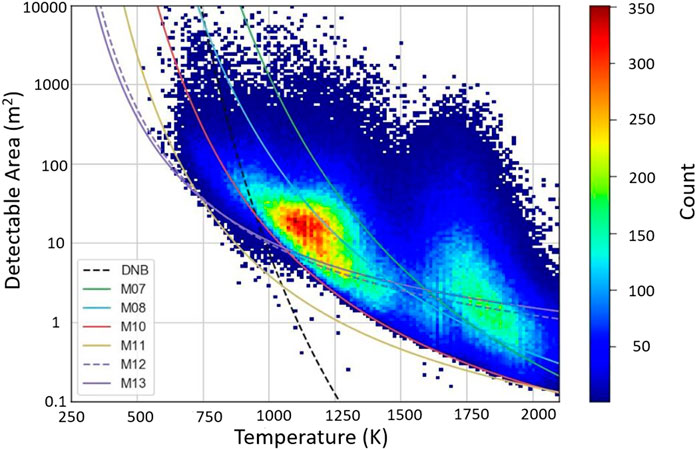
FIGURE 7. Detection limits of nighttime VIIRS M-bands used in the VIIRS nightfire (VNF) data product plus the DNB. The M-band detection limit lines are derived from the minimal detected radiances found in VNF and Planck’s Law. By varying the temperature, Planck’s Law (Planck, 1901) calculates the source size required to meet the detection limit. The colored data cloud are actual VNF temperatures and sources sizes from 1 month of VNF data. The DNB detection limit is derived from the VIIRS boat detection product. Figure is from (Elvidge et al., 2019).
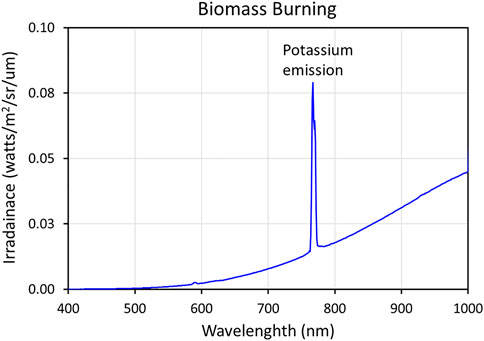
FIGURE 8. Spectrum from a wood fire collected with an Analytical Spectral Devices spectroradiometer. Across the DNB bandpass (500–900 nm) the fire exhibits the leading edge of a Planck curve. Biomass burning near-infrared radiant emissions invariably feature a prominent Potassium emission at 767 nm (Vodacek et al., 2002).
History of the DMSP-OLS archive
There is no official long-term archive for the DMSP-OLS data. The U.S. Air Force has no requirement for an archive, but kindly provided OLS data to NOAA since the mid-1970’s. NOAA’s official archive for satellite data, the Comprehensive Large Array-data Stewardship System (CLASS), contains DMSP Special Sensor Microwave Imager—but not OLS data. From the 1970’s to mid-1992, DMSP SAP and OLS data were written to 10-inch-wide film, with no preservation of the digital data. In the early 1970’s the low-light imaging capability was classified and was not disclosed outside of the Department of Defense. Since the low-light imaging was designed for cloud observations, there was considerable surprise when city lights were seen in the earliest data. In addition, there were intermittent high-latitude lighting features that perplexed Air Force scientists. A decision was made to disclose sample images to an internationally recognized expert at the University of Alaska, who lacked a security clearance. Professor Syun-Ichi Akasofu immediately recognized the features as aurora. Based on this disclosure, the DMSP low-light imaging data were declassified, making it possible for Akasofu to publish a paper on his findings in 1974 (Akasofu, 1974). OLS declassification led to the development of a film archive established jointly by NOAA’s National Geophysical Data Center (NGDC) and the National Snow and Ice Data Center at the University of Colorado. The archive received periodic shipments of DMSP OLS film for nearly 20 years. The first global map of nighttime lights was produced by Professor Woody Sullivan, who mosaiced hand selected film segments, produced a poster and published a paper (Sullivan, 1991).
From 1999 to 2010 EOG was able to have a large portion of the OLS film archive digitally scanned through NOAA’s Climate Data Modernization Program (CDMP). Figure 9 shows an example of an OLS film scan from 1989 covering India. Copies of the film scan archive are held by NOAA’s National Centers for Environmental Information in Boulder, Colorado and by EOG at the Colorado School of Mines. There is a limited set of OLS orbits from 1992 where both film scans and digital OLS data are available. Under CDMP, EOG developed a Film Scan Geolocation Program (FGSP) and demonstrated the geolocation of DMSP film scans. It is also possible to use image to image control points to geolocate sections of individual film scans.
With the fall of the Soviet Union in 1991, there were several years where the U.S. Department of Defense loosened its rules on science data access and distribution. In 1992, the Air Force shifted from film to digital analysis of the OLS data. That meant that the DMSP film archive would no longer receive shipment from the Air Force. NGDC’s Dr. Herbert W. Kroehl spoke out for establishing a digital OLS archive at the Air Force’s annual DMSP data user workshop. About 2 weeks later, Kroehl received a call from the DMSP program office indicating that they agreed with him that there should be an archive. The Air Force provided the seed funding for NGDC to establish an archive for the digital data—which were delivered to NGDC on 8 mm tapes via Federal Express—at the rate of one cassette per day. The data consisted of readout files, primarily from the ground station in Thule, Greenland. NGDC wrote software to orbitize the data, with orbits starting at the ascending node’s equatorial crossing. Each scanline contained the VIS and TIR data plus the satellite ephemeris calculated from bevel vector sighting of the spacecraft calculated using the Special Ephemeris (SPEPH) orbital model developed by the Air Force for DMSP satellites. The ephemeris consists of the scanline’s nadir latitude/longitude, satellite altitude, and heading. NGDC could not afford a tape archive—so the original day tapes and tapes containing the orbitized data were labeled and kept on racks. Accessing the data involved manual selection of 8 mm tapes for extraction via a single unit tape drive.
EOG had concerns about long term storage of the OLS archive on 8 mm tapes, which are not designed for long term data storage. In 2001, NGDC installed a robotic tape library system and invited EOG to store the OLS data there. By this time the OLS collection contained more than ten thousand 8 mm tapes, which are not designed for long term data storage. To accomplish the migration to the NGDC tape library, EOG purchased a refurbished Exabyte 8 mm tape with 40 slots. Ed Erwin took on the task to read all the 8 mm tapes and transferring the data to the NGDC tape library. Since that time the OLS data collection has continued to migrate as NGDC’s tape library system evolved.
Monthly and annual nighttime lights
The Earth Observation Group traces its origins to 1994 when a DMSP fire detection project funded at NGDC by the U.S. Department of Defense Strategic Environmental Research and Development Program (SERDP). The original EOG team at NGDC consisted of Chris Elvidge, Kimberly Baugh, Ethan Davis and Edward Erwin. The first task in the SERDP project was to develop a map of stable lights that could be used as a mask to detect biomass burning in rural areas. Kim and Ethan wrote software to geolocated OLS data. Then Kim and Chris developed methods to take a year’s worth of data to make global maps of nighttime lights. Initially cloud identification was performed visually by EOG analysts, who selected thermal band radiances for horizontal orbit segments—200 lines at a time (Elvidge et al., 1997). This made it possible for the thermal threshold to vary through an orbit, from arctic to tropical climates. Later an automatic cloud identification method was developed based on the thermal band differences from NCEP surface temperature grids (Baugh et al., 2010). EOG’s approach to nighttime lights generation features a cascading series of filtration steps to exclude sunlit, moonlit, stray light, lightning, and cloudy pixels. The annual sets are then filtered to remove outliers (biomass burning, High Energy Particle (HEP) detections, some aurora) and finally the background is zeroed out. What remains are primarily surface lighting features. In 2015 this approach was extended to nighttime lights product generation with VIIRS day/night band (DNB) data (Elvidge et al., 2017).
Over the past 30 years, EOG has produced a total of 67 annual and 690 monthly global nighttime light products. The DMSP products are 30 arc second grids and the DNB products are 15 arc second. The annual compositing process makes it possible to fill in usable nighttime data across seasonal fluctuations in solar contamination in mid-to-high latitude ranges, to find cloud-free pixels in the tropics, and to isolate seasonal biomass burning for outlier removal. The monthly products are truncated latitudinally during summer months and have data gaps in zones with heavy cloud cover. The annual product sets from both DMSP and VIIRS consists of: 1) A coverage grid—which tallies the number of dark night observations (sunlit and moonlit pixels excluded). 2) Cloud-free coverages. 3) Average cloud-free radiance (or average DN in the DMSP case). 4) An outlier removed radiance (or DN). 4) A lit grid cell mask. And 5) Average radiance (or DN) with background grid cells (where no surface lighting could be detected) zeroed out. Figure 10 shows examples of key products from the 2020 VIIRS nighttime lights. The DMSP and VIIRS nighttime light product sets are available on an open access basis from: https://payneinstitute.mines.edu/eog/nighttime-lights/.
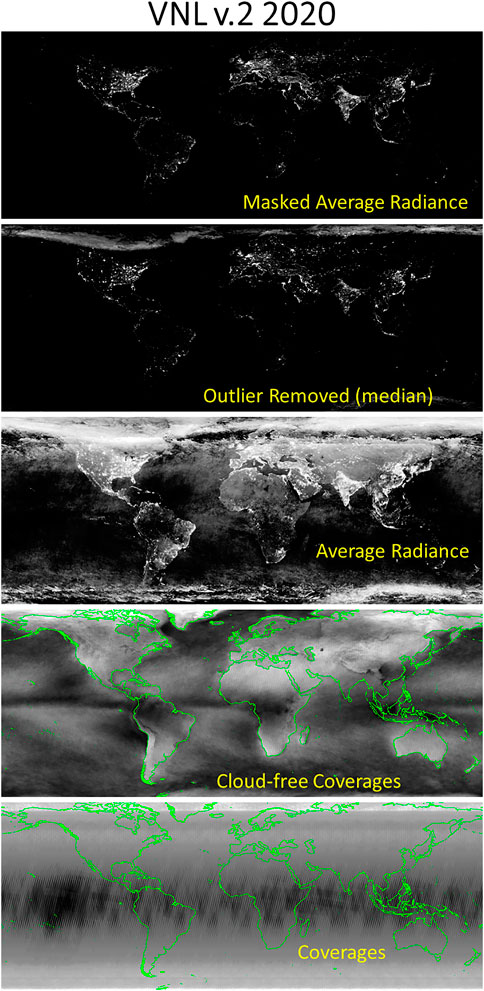
FIGURE 10. Each annual VIIRS nighttime lights product sets contains global grids of coverages, cloud-free coverages, average radiance, outlier removed radiance, lit grid cell mask (not shown), and masked average radiance. The DMSP sets report out DN (digital numbers) instead of radiances.
DMSP nighttime lights v.4 series
EOG began producing global DMSP-OLS monthly and annual nighttime lights in the late-1990’s (Baugh et al., 2010). Early version used nearly the full swath, with trimming to remove the PMT switch point and a set of noisy pixels at the edge of scans. Moonlit data are excluded based on a U.S. Navy lunar illumination model. Manual screening is used to select clip out sunlit data, aurora, and stray light glare. Initially analysists selected thermal band thresholds to mark cloudy pixels 200 lines at a time. In v.4, only the central portion of the swaths are utilized, between the two PMT switch points. Excluding the outer panels of the OLS orbits results in improved geolocation accuracy and sharper lighting features as pixel footprints expand rapidly towards the edges-of-scan. Also in v.4, clouds are automatically identified by histogram analysis of thermal band brightness temperatures minus surface temperature grids supplied by NOAA’s National Center for Environmental Prediction (NCEP). The DMSP v.4 monthly and annual nighttime lights extend from mid-1992 through December 2013. Production on the v.4 series ended in 2013 due to losses in usable nighttime data due the satellite F-18’s orbit degradation (Figure 6).
Lacking in-flight calibration, researchers rely on intercalibrations to produce more consistent results across the DMSP v.3 time series. The EOG in-house intercalibration used the DMSP F-12 1999 image of Sicily as a reference (Elvidge et al., 2009). Each satellite year was paired to F-12 1999 with gains and offsets derived from 2nd order regressions. Over the years quite a few papers have been published on alternate methods for intercalibration of the DMSP nighttime lights.
DMSP radiance series
In the mid-1990’s Elvidge contacted the OLS engineer, Theodore Bland of the Northrop Grumman Corporation, to discuss the PMT gain control on the OLS. Gain commands are uploaded to the OLS monthly to reduce the PMT gain under high lunar illuminance and to incrementally raise the gain as moonlight declined. The highest gain settings occur during the new Moon. Even with the gain turned to its highest monthly level under new Moon night, clouds cannot be detected. However, the high gain settings results in saturation in urban centers and bright emission sources such as gas flares. This led to the formulation of a plan to collect PMT data at reduced gain levels under new Moon conditions (Elvidge et al., 1999). The Air Force agreed to a set of tests where the PMT gain would be turned down to avoid saturation on bright light sources. The data would be collected with the Automatic Gain Control (AGC) onboard algorithm turned off—hence these data are referred to as “fixed gain” collections. On the initial 24 h of testing—the PMT was alternated through a wide range of fixed gain settings in a step wise schedule to provide data for devising a fixed gain strategy for collecting unsaturated data from the bright cores of urban centers out to the dim lighting present in suburbs and rural communities. Based on the stepped gain testing from the first 24 h—a strategy was developed to collect data at low, medium and high gain setting worldwide for a full week centered on the new Moon. EOG assembled this data into the first global nighttime lights product with units of radiance instead of DN. The DN’s had been converted to radiances based on the preflight PMT calibration. The fixed gain collection were repeated for several years making it possible to produce a limited time series. Later EOG’s Feng Chi Hsu (Hsu et al., 2015) reprocessed the fixed gain data to better address sub-pixel saturation and to blend the products with the operational annual nighttime lights to further extend the radiance lights into the dimmer outer edges of cities and rural lighting areas. The resultant Radiance Calibrated series is able to fully restore the detailed brightness changes in heavily lit area. As shown in Figure 11, the Tokyo metropolitan area being one of the most heavily lit area in the world, DMSP stable lights product has most of the Tokyo saturated at 63 DN. Whereas, the radiance calibrated product was able to capture the fine variation of brightness even at the core of the Tokyo agglomeration.
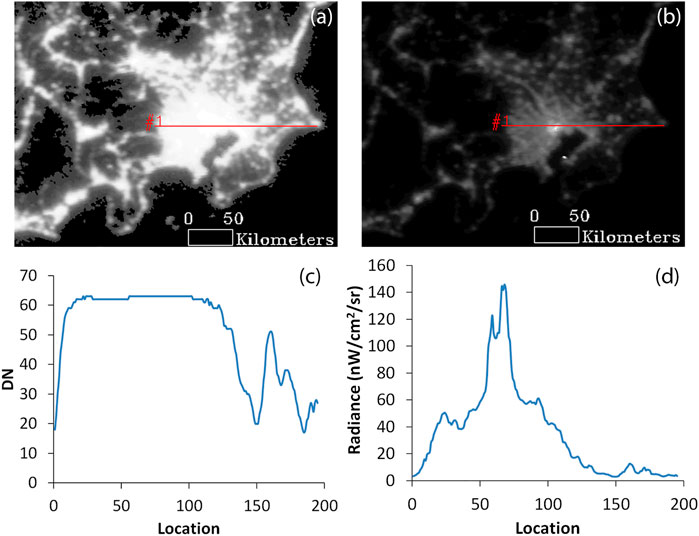
FIGURE 11. Comparison of DMSP stable light product (F16_2006) and radiance calibrated product (F16_20051128–20061224) over Tokyo, Japan. (A) DMSP v. 4 stable lights product of Tokyo; (B) Radiance calibrated DMSP product of Tokyo; (C) Transect of stable lights product across the center of Tokyo; (D) Transect of radiance calibrated product over the center of Tokyo. Note that the stable lights are saturated (DN = 63) across more than half of the transect.
DMSP extension series
The original DMSP v. 4 series was built using early evening OLS data. The original series ended in 2013, when the F-18 orbit gradually degraded to a point where the overpass time dropped below the 19:30 overpass time, which resulted in an insufficient quantity of nighttime data for assembly of an annual global product. All the DMSP satellites follow a similar orbital degradation pattern (Figure 6). The OLS instrument on DMSP satellite F15 has been particularly long lived. The original F15 v.4 products span 2000 to 2007. F-15 transitioned to a dawn-dusk orbit in 2008—with insufficient nighttime data collections for making a global nighttime lights product. Five years later, the F-15 orbit had degraded to a point where pre-dawn nighttime OLS data began to be collected. A similar shift to pre-dawn OLS data collection occurred for satellite F-16. With support from the AFD (Agence Française de Dévelopement), EOG produced an DMSP nighttime lights extension series with pre-dawn nighttime data using the v.4 methods (Ghosh et al., 2021). In addition, VNL v.2 data were resampled to 30 arc second grids and transformed to match the spatial resolution and digital number range of DMSP (Nechaev et al., 2021).
VIIRS nighttime lights (VNL) v.1 series
The VNL v.1 algorithms were developed with support from NASA’s VIIRS science team from 2015 through 2017. The basic steps from the DMSP v.4 methods were adapted to work with VIIRS data (Elvidge et al., 2017). The grid resolution is shifted from 30 to 15 arc seconds. Cloud identifications are based on the VIIRS cloud mask (VCM) (Kopp et al., 2014). No screening is done to remove data contaminated by aurora. Fire detections are filtered out based on the grid cell scattergram outlier removal from DMSP v. 4. VNL v.1 differs from the DMSP v.4 methods in several ways. Cloud identification is based on NOAA’s VIIRS cloud mask (VCM). Sunlit data are filtered based on the solar zenith angle instead of visual inspection. Pixels contaminated with stray light are excluded—except where the stray light correction (Mills et al., 2013; Lee and Cao, 2016) has been applied. Also, thanks to the near constant pixel footprint of the DNB—only the outer edges of the swath are excluded. Background areas are identified based on low data range values. Only two annual VNL v.1 global nighttime lights were produced—for 2015 and 2016. While EOG was not able to continue the annual product series for VNL v.1, the algorithms continue in use to this day for the generation of the monthly DNB cloud free composites. Both or the annual VNL v.1 and all the monthly products are available at: https://eogdata.mines.edu/products/vnl/.
VIIRS nighttime lights v.2 series
The VNL v.2 series includes only annual products, with monthly product generation continued using the VNL v.1 algorithms. The VNL v.2 global nighttime lights products are made by fusing data from twelve monthly DNB products (Elvidge et al., 2021). Radiance averaging applies weighting of the monthly averages based in the number of monthly cloud-free observations. Outlier removal is achieved with the median monthly cloud-free radiance. VNL v.2 uses the temporal leverage found with the full time series to remove infrequent sources of noise. Particular attention has been paid int the VNL v.2 time series to use the same thresholds and settings—so that the radiances and spatial patterns can be quantitatively compared. With the completion of the 2021 product in early 2022—the time series spans a full decade from 2012 to 2021. Figure 12 shows an example of the spatial expansion of VIIRS detected lighting in a portion of Northeastern India. VNL v.2 products are available at: https://eogdata.mines.edu/products/vnl/.
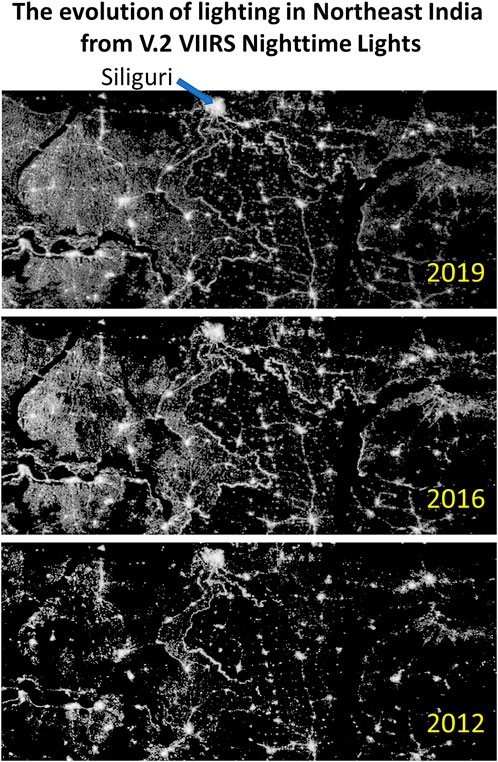
FIGURE 12. Expansion of lighting in a portion of Northeastern India from the VNL v.2 time series. Figure is from (Elvidge et al., 2021).
VIIRS nighttime lights v.3 series—nightly temporal profiles
Most studies conducted with VIIRS nighttime lights make use of the EOG’s monthly and annual summary grids, reporting the mean radiance levels. These have an advantage over nightly products in that data gaps from clouds are filled in and the averaging process stabilizes the brightness level by including large numbers of samples from a wide range of satellite zenith angles. In addition, the annual and monthly DNB products are convenient because they are readily available; compact; and relieve the researcher from having to ingest, filter, and compose vast quantities of raw data. However, some information related to lighting changes and electric power reliability is lost due to the averaging process. Figure 13 shows an example of a nightly temporal profile from Tucson, Arizona where the city replace high-pressure sodium streetlights with LEDs. The date range of the transition is shown as a step down in radiance values in the second half of 2016. LED streetlights are quite low in flicker, which results in a reduction in variance in the temporal record from 2017 to present (Elvidge et al., 2020). There are distinct advantages to working with the full-resolution temporal profiles for the characterization and removal of view angle effects and reflected moonlight. In addition, lighting phenomenology can be analyzed in greater detail, such as the advent of detectable lighting, power outages, growth and recovery, and annual cycling (Hsu et al., 2021). The other advantage of working with the full temporal resolution DNB profiles is the option to work with a broader range of indices, beyond just the mean.
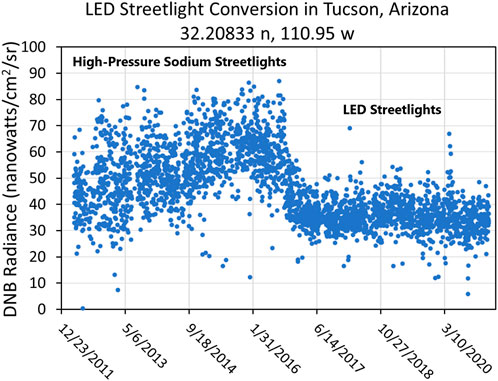
FIGURE 13. Example of a VNL v.3 DNB temporal profile with radiance adjustments for satellite zenith angle antd the subtraction of lunar reflectance. This example shows the effects of streetlight conversions from high-pressure sodium to LED sources, along with voltage reductions after midnight.
VNL v.3 DNB temporal profiles grids have been assembled for seventy cities across the North America, Asia, the Middle East and Africa (Figure 14). Several studies based on VNL v.3 temporal profiles have already been published (Ghosh et al., 2021; Nechaev et al., 2021). Spatially extended DNB temporal profile grids have been assembled for VNL v.2 lit grid cells for Kenya, Ghana, and Texas. EOG plans to expand the spatial extent of VNL v.3 to all lit grid cells from VNL v.2 over the next several years. Ideally, the temporal profiles should be updated on at least a monthly basis. The assembly of global VNL v.3 will be key to the development of global VNL v.4, described below.
VNL v.4 monthly and annual nighttime lights
All previous global monthly and annual nighttime lights products have focused on the average brightness of lights. Recent studies indicate that there is substantial information content on lighting and changes in lighting over time present in the VNL v.3 temporal profiles that are lost in the averaging process (Elvidge et al., 2020; Elvidge et al., 2019). This includes the statistical moments in addition to the mean or average: variance, skew and kurtosis. Also, there are weekly and annual cycling patterns that can be identified from autocorrelation analyses (Hsu et al., 2021). EOG is already prototyping VNL v.4, which will be derived from the VNL v.3 nightly temporal profiles and are planned to include the four statistical moments (mean, variance, skew and kurtosis), the temporal lag for cycling patterns, annual amplitude of cycling, and specifying the crests and troughs of the cycling. In addition, EOG has identified several areas for improving the quality of VIIRS nighttime lights: DNB specific cloud detection, atmospheric correction, improved removal of lunar reflectance, flattening of glow surround bright sources, and flattening of aurora.
Expansion of groups actively producing and using nighttime lights data
Until the advent of VIIRS DNB data—EOG was largely alone in the development and production of global nighttime lights products. In recent years there has been an expansion in the number of groups actively engaged in producing and providing access to nighttime lights data, plus products for specific applications. The NASA Black Marble project is producing nightly VIIRS mosaics with atmospheric, lunar illuminance, and plant canopy obscuration corrections (Román et al., 2018). These data products are provided in ten-by-ten-degree tiles, with ocean areas zeroed out. While the Standard product (500 m) is being processed on a daily basis within 3–5 h after acquisition, a new suite of higher spatial resolution data is also being developed, integrating data acquired from other Earth observing satellites (e.g., Landsat- 8, Sentinel-2) and ancillary data sources (street, building, and other GIS layers). Recently, NASA initiated a CARD4L working group to focus on improving the “Night Light Radiance” (NLR). The World Bank, NOAA and the University of Michigan recently launched an “Open Lights” service, providing access to both DMSP and DNB sub-orbits (World Bank Open Database License (ODbL), 2021). A commercial company, New Light Technologies Incorporated (NLT Inc.) has been contracted by the World Bank to develop a tool for inter-calibration of DMSP-OLS and VIIRS data. The procedure and tool, currently under development, will allow users to calculate and generate monthly and annual consistent composites for a desired Area of Interest (AOI) spanning 1992 to present. In this respect, it should be mentioned that the Socioeconomic Data and Applications Center (SEDAC) has developed the VIIRS Plus DMSP Change in Lights (VIIRS + DMSP dLIGHT) product (MacManus et al., 2021), which fuses DMSP-OLS and VIIRS DNB data to map the spatial distribution and temporal evolution of global nighttime lights between 1992 and 2015. Further, NLT Inc. has been involved for many years in multiple projects with and for the World Bank that utilize VIIRS data, for example, to support the World Bank’s Systematic Country Diagnostics, to estimate trends in economic activity of developing countries, to guide sustainable development of developing countries, for impact evaluation (e.g., the economic impacts of flood protection projects, local and regional economic trends around transportation corridors) and recently—to map the economic impacts of COVID-19 by generating VIIRS DNB composites, corrected for seasonal variations. VIIRS data are also used extensively for disaster management and disaster response. For example, NASA’s Short‐term Prediction Research and Transition (SPoRT) has developed workflows to create composite imagery to help detect power outages and restoration during disasters to support disaster management agencies prioritize disaster relief operations (these mosaics are disseminated during disasters via web mapping service (WMS)) (Molthan and Zavodsky, 2018). VIIRS data products are increasingly being stored, managed and disseminated through cloud-based platforms, including Google Earth Engine and ESRI`s Living Atlas of the World. The “Light Every Night” data catalog, an open access archive of all VIIRS DNB data from 2012–2020 and DMSP-OLS data from 1992–2013. The World Bank has also developed, together with NLT, the “Big Data Nighttime Lights Socioeconomic Observatory” (Bank Group, 2018), a platform that provides monthly nighttime light data for national and subnational levels, visualization of EOG v.1 suite of average radiance composites, time series visualizations for representation of nighttime light patterns and behavior across time, metrics indicating the amount of useful pixels (i.e., non-clouded-pixels or non-data pixels) utilized to calculate the statistics of interest, animated timeline of nighttime light data, CSV data download for given AOI and time period and more.
Concepts for future nighttime lights satellite sensors
Up until now, there have not been satellite sensors specifically designed for global mapping of nighttime lights, though the concept has been proposed (Elvidge et al., 2007b). There have been several satellite sensors capable of collecting low light imaging data at high spatial resolution, though none of these have capability to generate global cloud-free products. There will always be a role for nightly global low light imaging sensors, such as VIIRS, for mapping lights at the Earth’s surface and detecting lighting events such as power outages. But sensors designed specifically for nighttime lights could add to the information content regarding lighting by recording detail that is missed by coarse resolution sensors with panchromatic low light imaging.
Multispectral low light imaging would make it possible to distinguish lighting types and conversions of lighting types (Elvidge et al., 2010). A serious shortcoming of DMSP and VIIRS low light imaging is that their spectral bandpasses have no sensitivity to blue wavelengths of light. Several researchers (Falchi et al., 2011; Gaston et al., 2013) have pointed out that blue light scatters further in the atmopshere and have stronger biological effects than land wavelength visible light. Thus, having a separate blue spectral band would open up a new arenas in nighttime remote sensing of light pollution.
Finer spatial resolution would make it possible to improve the mapping of functional zones within human settlements. The trade-off for higher spatial resolution nighttime imaging is that such systems, such as Landsat, lack nightly global collection capabilities.
Low-light imaging sensors able to stare at lighting from space would make it possible to record alternating current flicker levels present in lighting. A recent study found the high intensity discharge lamps, such as high-pressure sodium and metal halides are high in flicker while modern LED lights have extremely low levels of flicker (Elvidge et al., 2022). Flicker rates range from 100 to 120 cycles per second—so an instrument would need to have a refresh rate in the 1–2 ms range and stare for about 10–20 ms to accomplish the flicker measurement. The VIIRS DNB pixel aggregation time is in the range of 2–3 ms, short enough to be affected by flicker, which contributes to the variance in the temporal profiles. Flicker, even at rates above the limit of human vision, can result in symptoms such as eye strain and irritability in humans. Biological impacts of exposure to flicker continue to be researched. Having an ability to measure flicker reliably from space would open up new areas of research on flicker effects on organisms.
There is a growing body of evidence that there are view angle effects on the brightness of lighting observed from space (Li et al., 2022; Tan et al., 2022; Wang et al., 2022). The view angle effects arise from building and shielding geometries that have yet to be fully explored. Elvidge et al. (Elvidge et al., 2020) developed a method for flattening view angel effects in VIIRS DNB temporal profiles and found that there are four main styles of view angle effects: 1) Flat with little-or-no view angle effects, 2) Brighter when viewed from the side, 3) Brighter when viewed at nadir, and 4) Peak-at-nadir. NASA’s Black Marble team has begun producing multi-angle cloud-free temporal composites to further explore the information content associated with view angle variations in the brightness of lights observed from space (Wang et al., 2022). Recent studies have pointed out the potential value in having consistently collected variable view angle nighttime lights data collected on urban areas (Kyba et al., 2021).
A final area where new low-light imaging satellite sensors could open up new areas of research is in the diurnal remote sensing of surface lighting. Recently, hourly drone collected over urban areas confirmed that diurnal patterns do exist in lighting (Li et al., 2020). Ghosh et al. (Ghosh et al., 2021) examined 2013 nighttime lights data from early-evening DMSP F18 data, after-midnight SNPP data and pre-dawn DMSP F15 data and found in most areas lighting dimmed as the evening progresses. The 2013 analysis is semi-quantitative due to the lack of calibration on the DMSP nighttime lights. A constellation of low-light imaging sensors could collect radiance calibrated nighttime lights for global analysis of diurnal patterns of lighting (Stokes et al., 2021) and how these change over time.
Conclusion
Nighttime lights have emerged as one of the most widely used global satellite data products. Google scholar lists 69,700 papers mentioning “nighttime lights”, attesting to the value scientists find in the data. Nighttime lights user communities span a wide swath of scientific disciplines from economics, biology, sociology, ecology, human health and astronomy. In 2021 EOG’s online open access service tallied forty two thousand file downloads totaling 560 TB of nighttime lights data.
In 2022 it is possible for researchers to work with a 30-years record of global nighttime lights, spanning four overlapping time series: DMSP v.4 (1992–2013), DMSP radiance lights (1996–2011), DMSP extension series (2013–2021), and VIIRS VNL v.2 (2012–2021). Scientists conducting cross-series studies should take into consideration the differences in the series (Figure 15). The DMSP series are constructed as 30 arc second grids while the VIIRS products are 15 arc second grids. The DMSP v.4 and extension series report brightness levels as uncalibrated digital numbers ranging from 0 to 63 (six-bit data). VNL and the DMSP radiance series report average brightness levels in radiance units (nanoWatts/cm2/sr). However, the DMSP radiance lights trace back to the pre-flight OLS calibrations and the VNL series has the benefit of in-flight radiance calibration. There are also diurnal differences between the series due to different overpass times. The best year to study diurnal patterns of lighting detection and brightness levels is 2013, where nighttime lights from three overpass times are available (Figure 15): evening, after midnight, and pre-dawn. A recent study (Elvidge et al., 2019) found that in general lights are brighter in the early evening collections present in the DMSP v.4 series, a bit dimmer after midnight, and dimmest in the pre-dawn hours.
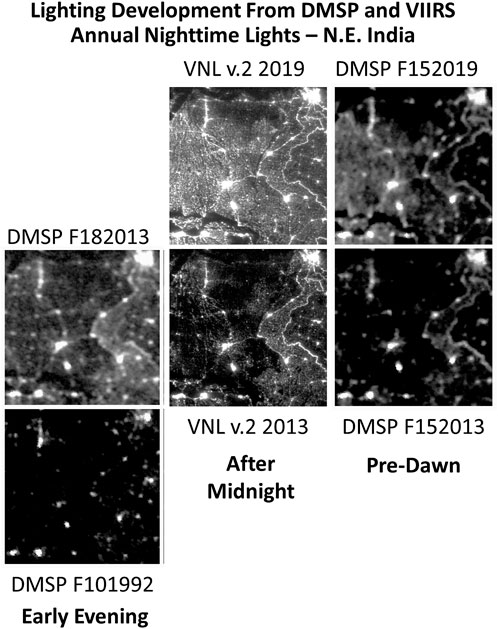
FIGURE 15. The combined DMSP v.4, VNL v.2 and DMSP extension series spans 30 years—from 1992 to 2021.
In some years there are products from multiple sensors and in 2013 there are products from three different time periods in the night: evening, after midnight, and pre-dawn. While low-light imaging sensors capable of detecting lighting have been recording nightly global data since the mid-1970’s, the early sensors were primitive and the historic record was poorly preserved until the 1992 founding of the DMSP archive. The first 17 years of DMSP low light imaging data was recorded on film, with the original records lost. While NOAA was successful in digital scanning much of the film exhibiting nighttime lights—these data are of low quality compared to the digital DMSP data. EOG has produced monthly and annual DMSP nighttime lights spanning 1992 through 2021 and while widely used by researchers—these data should be considered semi-quantitative due to the lack of inflight radiance calibration and saturation on bright lights. The VIIRS DNB data are vastly improved over DMSP and has opened a new era for nighttime lights studies.
Data availability statement
Publicly available datasets were analyzed in this study. This data can be found here: https://payneinstitute.mines.edu/eog/nighttime-lights/.
Author contributions
CE lead author, KB algorithms and product development, TG product generation, MZ algorithms and product development, FH algorithms and product development, TS graphics and text review, MB text review and editing, PS data product reviews, RG writing, KH funding and product development.
Funding
Over the years EOG received financial support to develop algorithms and to produce data products. NASA supported the development of the v.1 VIIRS Nighttime Lights, the Development Agency of France supported the DMSP extension series production, and the Rockefeller Foundation supported the development of VIIRS Nighttime Lights v.3 nightly temporal profiles.
Acknowledgments
The DMSP and VIIRS nighttime lights are only possible thanks to the many years of effort to build, fly and collect global nightly low light imaging data by the U.S. Air Force Defense Meteorological Satellite Program (DMSP), the National Aeronautics and Space Administration (NASA), and the National Oceanic and Atmospheric Administration (NOAA) Joint Polar Satellite System (JPSS).
Conflict of interest
RG is employed by New Light Technologies, Incorporated.
The remaining authors declare that the research was conducted in the absence of any commercial or financial relationships that could be construed as a potential conflict of interest.
Publisher’s note
All claims expressed in this article are solely those of the authors and do not necessarily represent those of their affiliated organizations, or those of the publisher, the editors and the reviewers. Any product that may be evaluated in this article, or claim that may be made by its manufacturer, is not guaranteed or endorsed by the publisher.
Supplementary material
The Supplementary Material for this article can be found online at: https://www.frontiersin.org/articles/10.3389/frsen.2022.919937/full#supplementary-material
References
Akasofu, S-I. (1974). A study of auroral displays photographed from the DMSP-2 satellite and from the alaska meridian chain of stations. Space Sci. Rev. 16 (5), 617–725. doi:10.1007/bf00182598
Baker, N. (2011). Joint polar satellite system (JPSS) visible infrared imaging radiometer Suite (VIIRS) sensor data records (SDR) algorithm theoretical basis document (ATBD). Goddard Space Flight Centre, Greenbelt Maryland, Ground Project Code. Available at: https://www.star.nesdis.noaa.gov/jpss/documents/ATBD/D0001-M01-S01-004_JPSS_ATBD_VIIRS-Geolocation_A.pdf(accessed on 7 March 2022) .
Bank Group, W (2018). Seizing a brighter future for all: Former yugoslav republic of Macedonia systematic country diagnostic. World Bank.
Baugh, K., Elvidge, C. D., Ghosh, T., and Ziskin, D. (2010). Development of a 2009 stable lights product using DMSP-OLS data. APAN. Proc. 30 (0), 114. doi:10.7125/apan.30.17
Chen, X., and Nordhaus, W. D. (2019). VIIRS nighttime lights in the estimation of cross-sectional and time-series GDP. Remote Sens. 11 (9), 1057. doi:10.3390/rs11091057
Chulov, M. (2021). Lebanon hit by electricity outage expected to last several days. Available at: https://www.theguardian.com/world/2021/oct/09/lebanon-hit-by-electricity-outage-expected-to-last-several-days.
Croft, T. A. (1978). Nighttime images of the Earth from space. Sci. Am. 239 (1), 86–98. doi:10.1038/scientificamerican0778-86
Croft, T. A. (1981). Radiometry with nighttime DMSP images in digital form. Photog. Engr. Remote Sens. 47, 1319–1325.
Croft, T. A., and Colvocoresses, A. P. The brightness of lights on Earth at night, digitally recorded by DMSP satellite. USGS Open File Report R290. number 80-167, 1979Available at: https://pubs.usgs.gov/of/1980/0167/report.pdf.
Crooks, K. R., Burdett, C. L., Theobald, D. M., King, S. R. B., Di Marco, M., Rondinini, C., et al. (2017). Quantification of habitat fragmentation reveals extinction risk in terrestrial mammals. Proc. Natl. Acad. Sci. U. S. A. 114 (29), 7635–7640. doi:10.1073/pnas.1705769114
Elvidge, C. D., Baugh, K. E., Zhizhin, M., and Hsu, F. C. (2013). Why VIIRS data are superior to DMSP for mapping nighttime lights. APAN. Proc. 35 (0), 62. doi:10.7125/apan.35.7
Elvidge, C. D., Baugh, K. E., Kroehl, E. A., and Davis., E R. (1997). Mapping city lights with nighttime data from the DMSP Operational Linescan System. Photogrammetric Eng. Remote Sens. 63 (6), 727–734.
Elvidge, C. D., Baugh, K. E., Theodore Bland, J. B. D., Sutton, P. C., Kroehl, H. W., and Kroehl, H. W. (1999). Radiance calibration of DMSP-OLS low-light imaging data of human settlements. Remote Sens. Environ. 68 (1), 77–88. doi:10.1016/s0034-4257(98)00098-4
Elvidge, C.r D., Baugh, K., Zhizhin, M., Hsu, F. C., and Ghosh, T. (2017). VIIRS night-time lights. Int. J. Remote Sens. 38 (21), 5860–5879. doi:10.1080/01431161.2017.1342050
Elvidge, C. D., Benjamin, T., Baugh, K., Howard, A., Milesi, C., et al. (2007). Global distribution and density of constructed impervious surfaces. Sensors 7 (9), 1962–1979. doi:10.3390/s7091962
Elvidge, C. D., Hsu, F.-C., Zhizhin, M., Ghosh, T, Taneja, J., and Bazilian, M. (2020). Indicators of electric power instability from satellite observed nighttime lights. Remote Sens. 1219, 3194. doi:10.3390/rs12193194
Elvidge, C. D., Keith, D., Baugh, K. E., and Baugh, K. E. (2010). Spectral identification of lighting type and character. Sensors 10 (4), 3961–3988. doi:10.3390/s100403961
Elvidge, C. D., Pierantonio Cinzano, D. R., Arvesen, P.S., Small, C., Nemani, R., Small, C., et al. (2007). The nightsat mission concept. Int. J. Remote Sens. 28 (12), 2645–2670. doi:10.1080/01431160600981525
Elvidge, C. D., Zhizhin, M., Baugh, K., Hsu, F. C., and Ghosh, T. (2019). Extending nighttime combustion source detection limits with short wavelength VIIRS data. Remote Sens. 11 (4), 395. doi:10.3390/rs11040395
Elvidge, C. D., Zhizhin, M., Ghosh, T., Hsu, F.-C.i, and Taneja, J. (2021). Annual time series of global VIIRS nighttime lights derived from monthly averages: 2012 to 2019. Remote Sens. 13 (5), 922. doi:10.3390/rs13050922
Elvidge, C. D., Zhizhin, M. K., Miller, S. D., Hsu, F. C., Ghosh, T., SharolynAnderson, J., et al. (2022). The VIIRS day/night band: A flicker meter in space? Remote Sens. 14 (6), 1316. doi:10.3390/rs14061316
Elvidge, C. D., Ziskin, D.l, Baugh, K. E., Tilottama Ghosh, T., Pack, D, and Zhizhin, M. (2009). A fifteen year record of global natural gas flaring derived from satellite data. Energies 2 (3), 595–622. doi:10.3390/en20300595
Falchi, F., Cinzano, P., Duriscoe, D., Kyba, C. M., Elvidge, C. D., Baugh, K., et al. (2016). The new world atlas of artificial night sky brightness. Sci. Adv. 2 (6), e1600377. doi:10.1126/sciadv.1600377
Falchi, Fabio, Cinzano, Pierantonio, Elvidge, Christopher D., Keith, D. M., and Abraham, H. (2011). Limiting the impact of light pollution on human health, environment and stellar visibility. J. Environ. Manag. 92 (10), 2714–2722. doi:10.1016/j.jenvman.2011.06.029
Gallo, K. P., and Owen, T. W. (1998). Assessment of urban heat islands: A multi‐sensor perspective for the dallas-ft. Worth, USA region. Geocarto Int. 13 (4), 35–41. doi:10.1080/10106049809354662
Gaston, K. J., Bennie, J., Davies, T. W., and Hopkins, J. (2013). The ecological impacts of nighttime light pollution: a mechanistic appraisal. Biol. Rev. 88 (4), 912–927. doi:10.1111/brv.12036
Gaston, K. J. (2018). Lighting up the nighttime. Science 362, 6416744–6416746. doi:10.1126/science.aau8226
Ghosh, T., Baugh, K., Zhizhin, M., Poyda, A., Hsu, F.-C., and Hsu, F. C. (2021). Extending the DMSP nighttime lights time series beyond 2013. Remote Sens. 13 (24), 5004. doi:10.3390/rs13245004
Goldblatt, R., Stuhlmacher, M. F., Tellman, B., Clinton, N., Hanson, G., Georgescu, M., et al. (2018). Using Landsat and nighttime lights for supervised pixel-based image classification of urban land cover. Remote Sens. Environ. 205, 253–275. doi:10.1016/j.rse.2017.11.026
Goyette, John A. (1992). The Defense meteorological satellite program sensors: An historical overview” U.S. Air Force Technology development and plans system engineering division air Force Technology development and plans system. Available at: https://eogdata.mines.edu/wwwdata/dmsp/document_scans/The%20DMSP_sensors_AnHistoricalOverview_March1992.pdf (Accessed July 21, 2022).
Hsu, F.-C., Baugh, K. E., Ghosh, T., Zhizhin, M., and Elvidge, C. D. (2015). DMSP-OLS radiance calibrated nighttime lights time series with intercalibration. Remote Sens. 7 (2), 1855–1876. doi:10.3390/rs70201855
Hsu, F., Zhizhin, M., Ghosh, T., Elvidge, C., and Taneja, J. (2021). The annual cycling of nighttime lights in India. Remote Sens. 13 (6), 1199. doi:10.3390/rs13061199
Jacobson, A. P., Riggio, J., Alexander, M. T.t, and Baillie, J. E. M. (2019). Global areas of low human impact (‘Low Impact Areas’) and fragmentation of the natural world. Sci. Rep. 9 (1), 14179. doi:10.1038/s41598-019-50558-6
Kopp, T. J., Thomas, W., Heidinger, A. K., Botambekov, D., Frey, R. A., Hutchison, K. D., et al. (2014). The VIIRS cloud mask: progress in the first year of S-NPP toward a common cloud detection scheme. J. Geophys. Res. Atmos. 119 (5), 2441–2456. doi:10.1002/2013jd020458
Kyba, C. M., Aubé, M., Bará, S., Bertolo, A., Bouroussis, C. A., Cavazzani, S., et al. Commentary: multiple angle observations would benefit visible band remote sensing using night lights. J. Geophys. Res. Atmos., e2021JD036382.
Lee, S., and Cao, C. (2016). Soumi NPP VIIRS day/night band stray light characterization and correction using calibration view data. Remote Sens. 8 (2), 138. doi:10.3390/rs8020138
Levin, N., Kyba, C. M., Zhang, Q., Román, M. O., Li, Xi., et al. (2020). Remote sensing of night lights: a review and an outlook for the future. Remote Sens. Environ. 237, 111443. doi:10.1016/j.rse.2019.111443
Li, X., Levin, N., Xie, J., and Li, D. (2020). Monitoring hourly night-time light by an unmanned aerial vehicle and its implications to satellite remote sensing. Remote Sens. Environ. 247, 111942. doi:10.1016/j.rse.2020.111942
Li, X., Shang, X., Zhang, Q., Li, D., Chen, F., Jia, M., et al. (2022). Using radiant intensity to characterize the anisotropy of satellite-derived city light at night. Remote Sens. Environ. 271, 112920. doi:10.1016/j.rse.2022.112920
Lieske, R. W. (1981). DMSP primary sensor data acquisition” international foundation for telemetering. Available at: https://eogdata.mines.edu/wwwdata/dmsp/document_scans/DMSP_primary_sensor.pdf.
MacManus, K., Balk, D., Engin, H., Gordon, M., and Inman, R. (2021). Estimating population and urban areas at risk of coastal hazards, 1990–2015: how data choices matter. Earth Syst. Sci. Data 13 (12), 5747–5801. doi:10.5194/essd-13-5747-2021
Miller, S. D., Mills, S. P., Hawkins, J. D., Lindsey, D. T., Lee, T. F., and Hawkins, J. D. (2012). Suomi satellite brings to light a unique frontier of nighttime environmental sensing capabilities. Proc. Natl. Acad. Sci. U. S. A. 109 (39), 15706–15711. doi:10.1073/pnas.1207034109
Mills, S., Weiss, S., and Liang, C. (2013). VIIRS day/night band (DNB) stray light characterization and correction. Earth Obs. Syst. XVIII, 8866, 549–566.
Molthan, A., and Zavodsky, B. (2018). “NASA's short-term prediction research and transition (SPoRT) center: a paradigm for transitioning research into operations,” in 2018 AMS annual meeting, no (MSFC-E-DAA-TN51195).
Nechaev, D., Zhizhin, M., Poyda, A., Ghosh, T., Hsu, F.-C., Elvidge, C., et al. (2021). Cross-sensor nighttime lights image calibration for DMSP/OLS and SNPP/VIIRS with residual U-net. Remote Sens. 13 (24), 5026. doi:10.3390/rs13245026
Nichols, David A. (1975). DMSP compendium of early satellites U.S. Air Force space and missile systems organization. Available at: https://eogdata.mines.edu/wwwdata/dmsp/document_scans/DMSP_compedium_of_early_satellites.pdf.
Peng, S., Piao, S., Ciais, P., Friedlingstein, P., Ottle, C., Nan, Huijuan, et al. (2012). Surface urban heat island across 419 global big cities. Environ. Sci. Technol. 46 (2), 696–703. doi:10.1021/es2030438
Planck, Max. (1901). On the law of distribution of energy in the normal spectrum. Ann. Phys. 4, 1–553.
Román, M. O., Wang, Z., Sun, Q., Kalb, V., Miller, S. D., Molthan, A., et al. (2018). NASA's Black Marble nighttime lights product suite. Remote Sens. Environ. 210, 113–143. doi:10.1016/j.rse.2018.03.017
Sanderson, E. W., Malanding JaitehRedford, M H., Wannebo, A. V., Woolmer, G., Wannebo, A. V., and Woolmer, G. (2002). The human footprint and the last of the wild: The human footprint is a global map of human influence on the land surface, which suggests that human beings are stewards of nature, whether we like it or not. BioScience 52 (10), 891. doi:10.1641/0006-3568(2002)052[0891:thfatl]2.0.co;2
Small, C., Elvidge, C. D., and Baugh, K. (2013). “Mapping urban structure and spatial connectivity with VIIRS and OLS night light imagery,” in Joint urban remote sensing event (IEEE), 230–233.
Spangler, M. J. The DMSP block 5 program history at Westinghouse and Northrop grumman - as remembered by some participants” Westinghouse corporation. Available at: https://eogdata.mines.edu/wwwdata/dmsp/document_scans/DMSP_Block5_program_history_at_Westinghouse_Oct2003.pdf.
Stokes, E. C., Román, M. O., Wang, Z., Kyba, C. M., Miller, S. D., Tobias, S., et al. (2021). Retired satellites: a chance to shed light. Science 373, 65621451–65621452. doi:10.1126/science.abl9965
Sullivan, W. T. (1991). Earth at night: An image of the nighttime earth based on cloud-free satellite photographs. Int. Astron. Union Colloq. 112, 11–17.
Sutton, P. C., SharolynAndersonElvidge, J., Ghosh, T., Tuttle, B. T., and Ghosh, T. (2009). Paving the planet: impervious surface as proxy measure of the human ecological footprint. Prog. Phys. Geogr. Earth Environ. 33 (4), 510–527. doi:10.1177/0309133309346649
Tan, X., Zhu, X., Chen, J., and Chen, R. (2022) Modeling the direction and magnitude of angular effects in nighttime light remote sensing. Remote Sens. Environ. 269, 112834. doi:10.1016/j.rse.2021.112834
Venter, O., Sanderson, E. W., Magrach, A., Allan, J. R., Beher, J., Jones, K. R., et al. (2016). Sixteen years of change in the global terrestrial human footprint and implications for biodiversity conservation. Nat. Commun. 7 (1), 12558. doi:10.1038/ncomms12558
Vodacek, A., Kremens, R. L., Fordham, A. J., VanGorden, S. C., Luisi, D., Schott, J. R., et al. (2002). Remote optical detection of biomass burning using a potassium emission signature. Int. J. Remote Sens. 23 (13), 2721–2726. doi:10.1080/01431160110109633
Wang, Zhuosen, Shrestha, Ranjay M., Román, Miguel O., and Kalb, Virginia L. (2022). NASA’s Black Marble multi-angle nighttime lights temporal composites. IEEE Geosci. Remote Sens. Lett. 19, 1–5. doi:10.1109/lgrs.2022.3176616
Westinghouse Electric Corporation, (1989). Technical operating report block 5D-3 operational linescan system (OLS)” CDRL item 054A2 1989. Available at: https://eogdata.mines.edu/wwwdata/dmsp/document_scans/Technical_Operating_Report_OLS_Apr1989-v2.pdf.
World Bank Open Database License (ODbL), (2021). World Bank - light Every night. https://registry.opendata.aws/wb-light-every-night/.
Xie, Y., Weng, Q., and Fu, P. (2019). Temporal variations of artificial nighttime lights and their implications for urbanization in the conterminous united states, 2013–2017. Remote Sens. Environ. 225, 160–174. doi:10.1016/j.rse.2019.03.008
Zhang, P., Imhoff, M. L., Wolfe, R. E., and Bounoua, L. (2010). Characterizing urban heat islands of global settlements using MODIS and nighttime lights products. Can. J. Remote Sens. 36 (3), 185–196. doi:10.5589/m10-039
Keywords: Nighttime lights, DMSP, VIIRS, DNB, Low-light imaging
Citation: Elvidge CD, Baugh K, Ghosh T, Zhizhin M, Hsu FC, Sparks T, Bazilian M, Sutton PC, Houngbedji K and Goldblatt R (2022) Fifty years of nightly global low-light imaging satellite observations. Front. Remote Sens. 3:919937. doi: 10.3389/frsen.2022.919937
Received: 14 April 2022; Accepted: 12 July 2022;
Published: 26 August 2022.
Edited by:
Eric Vermote, National Aeronautics and Space Administration (NASA), United StatesReviewed by:
Ali Mohammadzadeh, K. N. Toosi University of Technology, IranZhuosen Wang, University of Maryland, United States
Copyright © 2022 Elvidge, Baugh, Ghosh, Zhizhin, Hsu, Sparks, Bazilian, Sutton, Houngbedji and Goldblatt. This is an open-access article distributed under the terms of the Creative Commons Attribution License (CC BY). The use, distribution or reproduction in other forums is permitted, provided the original author(s) and the copyright owner(s) are credited and that the original publication in this journal is cited, in accordance with accepted academic practice. No use, distribution or reproduction is permitted which does not comply with these terms.
*Correspondence: Christopher D. Elvidge, Y2VsdmlkZ2VAbWluZXMuZWR1