Assessing the impact of wildfires on water quality using satellite remote sensing: the Lake Baikal case study
- 1Institute for Electromagnetic Sensing of the Environment, National Research Council, Milan, Italy
- 2European Space Agency Climate Office, ECSAT, Didcot, Oxfordshire, United Kingdom
Lakes have been observed as sentinels of climate change. In the last decades, global warming and increasing aridity has led to an increase in both the number and severity of wildfires. This has a negative impact on lake catchments by reducing forest cover and triggering cascading effects in freshwater ecosystems. In this work we used satellite remote sensing to analyse potential fire effects on lake water quality of Lake Baikal (Russia), considering the role of runoff and sediment transport, a less studied pathway compared to fire emissions transport. The main objectives of this study were to analyse time series and investigate relationships among fires (i.e., burned area), meteo-climatic parameters and water quality variables (chlorophyll-a, turbidity) for the period 2003–2020. Because Lake Baikal is oligotrophic, we expected detectable changes in water quality variables at selected areas near the three mains tributaries (Upper Angara, Barguzin, Selenga) due to river transport of fire-derived burned material and nutrients. Time series analysis showed seasonal (from April to June) and inter-annual fire occurrence, precipitation patterns (high intensity in summer) and no significant temporal changes for water quality variables during the studied periods. The most severe wildfires occurred in 2003 with the highest burned area detected (36,767 km2). The three lake sub-basins investigated have shown to respond differently according to their morphology, land cover types and meteo-climatic conditions, indicating their importance in determining the response of water variables to the impact of fires. Overall, our finding suggests that Lake Baikal shows resilience in the medium-long term to potential effects of fires and climate change in the region.
1 Introduction
Lakes provide crucial ecosystem services (e.g., food and fresh water supply, aquatic recreation) for human society (Mueller et al., 2016; McCullough et al., 2019) and they are sentinels, integrators and regulators of climate (Adrian et al., 2009; Subin et al., 2012). It has been evident for more than a decade (e.g., Williamson et al., 2009) that climate change is generating complex responses in aquatic ecosystems that vary according to their geographic distribution, magnitude, and timing across the global landscape. Climate change, in particular global warming and increasing aridity, is leading to an increase of fires and fire severity (Brown et al., 2021). At the same time wildfires are also a cause of the continuing process of global climate change, by reducing forest cover and emitting greenhouse gases (e.g., CO2 and CO) (Liu et al., 2022). Landscapes exposed to fire often include stream, river and lake catchments leading to cascade effects up to the loss of ecosystem services they provide to humans. In fact, lakes are naturally downstream recipients and integrator of transport from land to water and of processes that occur in their watersheds (Williamson et al., 2008). A large part of the literature focused on the fire impacts on water quality, mainly streams and rivers, and less attention was paid to lake water effects (McCullough et al., 2019). Recently a review article by McCullough et al., 2019 proposed a conceptual model of hypothesized physical, chemical, and biological responses of lakes to fire events. In synthesis, they considered the interaction and feedbacks between meteorological, catchment, and limnological processes with weather, lake, landscape, and fire characteristics. Fire effects such as vegetation loss, increase in soil erosion and sediments runoff and transport, might lead to an increase in water turbidity in lake waters, reducing light penetration and affecting lake primary production. Variation in soil nitrogen (N) and phosphorus (P) content due to burning may affect post-fire concentrations and loads of these nutrients in runoff, rivers and ultimately in lakes (Smith et al., 2011). Murphy et al. (2006a, b) reported that fires (combustion and volatilization) can cause a significant reduction of N (92%, NH4-N increase from combustion and subsequent NO3–N increases from nitrification) and P (76%; particulate P bioavailable) stored in forest floor (organic material), with minor decline (N 31%–51%) recorded under lower fire intensity. The magnitude and duration of hydrological and geomorphological activity following wildfires depends on the complex interplay of factors including site (e.g., catchment size) and fire characteristics (e.g., burned area severity and extent) as well as post-fire rainfall patterns (Pacheco and Fernandes, 2021). Changes in post-wildfire runoff and erosion are most severe during high-intensity rainfall (Moody et al., 2013) especially when occurring after fire events, since vegetation has little time to recover from fire. Indeed, the magnitude, intensity and frequency of post-fire rainfall and associated flow events are key drivers of erosion and sediment delivery in many burned catchments (Malmon et al., 2007; Robichaud et al., 2007; Cannon et al., 2008; Moody and Martin, 2009).
Changes induced by wildfires can last from 1 month to several years depending on the type of fire event and site characteristics (“window of disturban” as defined by Prosser and Williams, 1998). Smith et al. (2011) reviewed the effects of wildfires on the quality of water supply in forest catchments, with a focus on suspended sediments transport, reporting that post-fire turbidity in streams and reservoirs are limited. The quantification of the impact of wildfires on river and reservoir water quality is hampered by the unpredictable nature of fires and the distribution of monitoring network and/or the sampling regimes. The use of satellite images for fire monitoring has a long history: since early 70 s, a wide range of data sources and methods have been utilized (Chuvieco et al., 2019) with highly variable results in terms of product characteristics and accuracy. Long- and short-term impacts of wildfires on the quality of lakes water can be investigated with the use of datasets produced from remotely sensing techniques, which provide consistent, global and objective information and have been increasingly used for monitoring the state of the earth systems (Zhao et al., 2022). earth Observation (EO) data can support systematic delivery of active fires, burned area and water quality (e.g., chlorophyll-a, turbidity) products depicting their spatio-temporal variation within fire affected areas worldwide (Warren et al., 2021; Chuvieco et al., 2022; Lizundia-Loiola et al., 2020; Giglio et al., 2009. While detecting burned areas relies on the persistence of the burned signal, detecting changes in water constituents concentration due to fires (or any other factor) depends on sampling and/or observations frequency. Although remote sensing can certainly improve observations and monitoring frequency of water quality variables compared to in situ measurements, there is still uncertainty on the likelihood of detecting changes. This uncertainty is a function of the variability of each constituent and of the hydrological characteristics of the lake and its catchment (Smith et al., 2011).
Boreal regions represent a sensitive biome where wildfires are the major source of climate-driven impacts (Whitman et al., 2019). The Baikal region, in particular, has been identified as one of the most vulnerable areas in Russia (Popovicheva et al., 2021) where deforestation rapidly increased in the last decade. According to Safronov (2020), the Baikal basin lost more than 12% of its forests between 2013 and 2018. Severe fires contributed to the degradation of both terrestrial and aquatic ecosystems by increasing watershed loading of sediments, nutrients and pollutants as well as by introducing smoke pollutants into the atmosphere (Brown et al., 2021). Consequently, lake Baikal, the deepest and largest lake (by volume) on earth, currently in oligotrophic state, might be receptive to wildfires effects, such as sediments and nutrients runoff to river and lake waters (Brown et al., 2021).
The main objectives of this study are to: i) analyse patterns and trends of consistent time series of satellite products on both burned-areas and water quality variables (chlorophyll-a, turbidity) along with modelled precipitations; and ii) investigate the potential relationship between wildfires (i.e., burned area) via terrestrial pathways and meteo-climatic data on Lake Baikal water quality and its catchment. To address this aim, we performed data analyses considering the main tributaries of Lake Baikal and their corresponding sub-basins. We expected changes in water quality variables, such as turbidity and chlorophyll-a, to occur at the river inflow into the lake because of the transport of suspended sediments and nutrients from the lake catchment through the river network. Hence, water quality analyses focused on Regions Of Interest (ROIs) selected close to inflow area of the major rivers and on the catchment area where water is collected through the river network.
2 Materials and methods
2.1 Study area
The ancient Lake Baikal (Siberia) has a volume of 23,000 km3, with a maximum depth of 1,642 m (Figure 1) with a high number of endemic species and it is a hotspot of biodiversity (Naumenko et al., 2017; Brown et al., 2021). The lake is about 660 km long and 80 km wide. The average residence time of the lake is of 300–400 years (Colman, 1998; Moore et al., 2009). There are three major tributaries of Lake Baikal, the Upper Angara (annual water discharge of 8.5 km3 y−1) in the north, and Barguzin (annual water discharge of 3.9 km3 y−1) and Selenga (annual water discharge of 29.3 km3 y-1) on the eastern lake shore with over 500 minor rivers flowing into the lake (Popovicheva et al., 2021). The Baikal catchment includes mainly mountains, boreal forests, tundra and steppe of high conservation value and extends over 551,000 km2 (Lake Baikal excluded, 31,500 km2) between Siberia and Mongolia territories. New activities in the catchment, include deforestation, agriculture, and tourism to sustain the local economy, with a reduction of the Russian heavy industrial sector (e.g., mining) (Brown et al., 2021).
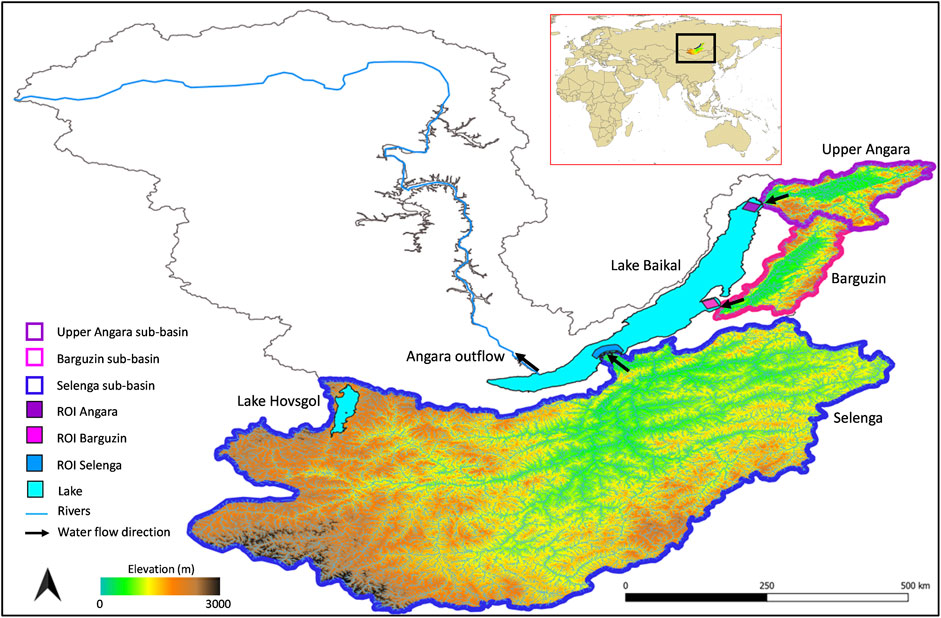
FIGURE 1. The Lake Baikal catchment (grey line; from HydroBASINS database, Siberia region level 4, Lehner and Grill, 2013), the three sub-basins corresponding to the major rivers (Upper-Angara, Barguzin, Selenga) and the Regions of Interest (ROIs) selected over the lake’s surface (ROI Angara, ROI Barguzin, ROI Selenga). In the small box the location of the study area into the globe.
Lake Baikal is covered by ice for several months of the year, but due to high transparency of the ice, phytoplankton and zooplankton can grow under ice cover. The lake is ultra-oligotrophic and well oxygenated due to water mixing (Shimaraev et al., 1994). The deep waters are pristine, but since the last century, pollution of anthropogenic origin (i.e., industrial pollution and cultural eutrophication) has started to threaten this ecosystem (Moore et al., 2009). Sources include industry, agriculture, forest fires, civil waste waters from villages and small towns along the coast and from the numerous tributaries, both from surface and groundwaters. The Selenga River is the main tributary of lake Baikal loading half of the water volume of the lake and the higher quantity of pollutants, as well as both micro and macronutrients, which are successfully filtered by the delta of the river before inflowing the lake (Khodzher et al., 2017; Roberts et al., 2020). In the last decade, the increase of phosphorus and nitrogen loads favoured a massive proliferation of filamentous algae (Spirogyra genus) mainly in autumn at the end of the touristic season, and along the lake shoreline (Mincheva et al., 2020). In the boreal zone of southern Siberia, forests are mainly composed by larch mixed with spruce and pine populations. The tree density and closeness of the canopy are low, which contributes to the drying of dead wood, fallen wood and bedding. Consequently, ground fires are more common in larch forests. Scarce rainfall and high summer air temperatures due to the harsh continental climate contribute to a high risk of large-scale forest fires. Forest fires in the region are mainly caused by anthropogenic activity and thunderstorms (Solovyev et al., 2009). Long time-series data show that forest fires in Siberia usually occur from May to September, with a maximum in the second part of the summer season (Solovyev and Budishchev, 2010; Solovyev et al., 2009).
2.2 Datasets
Since the objective of this study is the analysis of terrestrial pathway of the nutrient and sediment transport to lake water due to fire occurrence, we identified major tributaries of Lake Baikal and their catchments (Swiercz, 2007; Figure 1): Upper Angara (called Angara hereafter; 22,393 km2), Barguzin (21,249 km2) and Selenga (450,196 km2). The sub-basins considered covered the 90% of the Lake Baikal catchment and they were used to spatially aggregate daily burned areas and precipitation data. In both burned area and precipitation we retained the information at a daily time interval.
In order to better identify changes in lake water conditions and to reduce the impact of the diluting effect of the deepest open waters, we selected ROIs on the lake close to the tributaries’ inflow point. (Figure 1). In both cases of land and water variables, we built time series data with a daily step over the 2003–2020 period.
All input datasets (Table 1) were derived from EO products that can support long term monitoring of the relevant variables and, in particular.
Wildfires: Fire_cci burned area datasets (Lizundia-Loiola et al., 2020; CEDA, 2022a)
Water quality: Lakes_cci quality variables (Carrea et al. (2023); CEDA, 2022b),
Meteo-climatic data:ERA5 climate reanalysis products by ECMWF
For this study case we decided to use a burned area (BA) product as an input EO datasets depicting the spatial distribution of wildfires rather than active fires products. A burned area is the area affected by a fire that is characterized by the post-fire signal due to removal of vegetation and changes in vegetation structure and the deposit of combustion products (charcoal and ash) (Pereira et al., 1997). EO burned area products can better depict the amount of areas affected by fires compared to active fires that, by observing burning simultaneous to satellite overpass, can significantly underestimate the extent of the area burned (Roy et al., 2008). The persistence of the burned area signal, that is a function of several factors among which fire characteristics and land cover characteristics and conditions, guarantees a more accurate and reliable assessment of the area affected by fires.
2.2.1 Satellite-derived variables
The burned area (BA) product (Table 1) depicts spatio-temporal distribution of the global BA generated from MODIS satellite observations (Lizundia-Loiola et al., 2020) and it comprises maps of global burned area covering the period 2001–2020. The BA maps are obtained with a hybrid algorithm that combines MODIS highest resolution near-infrared (NIR) surface reflectance (∼250 m) and active fire information from the thermal channels. The FireCCI51 BA product, for each pixel identified as burned, provides information on the land cover from the year before fire detection as derived from the Land Cover (LC) v2.1.1 distributed by the Copernicus Climate Change Service. The detailed description of the algorithm and of validation are described in Lizundia-Loiola et al. (2020).
Turbidity and Chlorophyll-a (Chl-a) (Table 1), derived from satellite products provided by the Lakes_cci project (Carrea et al., 2023) were selected as indicators of biogeochemical processes and of water quality changes to investigate their correlation with fires. Ice cover, also provided by Lakes_cci, was additionally used as ancillary information to limit our analysis to ice-free days. The lake water quality products were obtained from satellite observations, namely, from MERIS-ENVISAT (15 spectral bands) for the period 2002–2012 and OLCI-Sentinel-3 A/B (21 spectral bands) for the period 2016–2020. The instruments have a spatial resolution of 250–300 m and an overpass frequency of 1–2 days. The description of the different algorithms used to create water quality products and their validation are reported in the “Algorithm Theoretical Basis Document—ATBD” and in the “Product Validation and Algorithm Selection Report (PVASR) and Algorithm Development Plan (ADP)” available on the project website.
The satellite products cover the period 2003 to 2020 for all variables considered, expect for Chl-a and Turbidity products which has a time gap of 4 years (2012–2015). For this reason, the two datasets are presented for the two different time periods 2003–2011 (p1) and 2016–2020 (p2). The datasets are available with a daily frequency, and they were aggregated temporally to weekly values to enhance peaks/anomalies and to resolve discontinuity in daily values due to missing observations. This dataset was previously used in Liu et al. (2021) to retrieve Chl-a concentration and associated uncertainty in optically diverse inland waters. EO-derived Chl-a and Turbidity products might be affected by uncertainties stemming from atmospheric correction as well as a lack of algorithms that can be universally applied to waterbodies spanning several orders of magnitude in non-covarying substance concentrations (Simis et al., 2020; Liu et al., 2021). To improve data quality, Chl-a and Turbidity values with an uncertainty <60% and <70% were hence used for analysis, as previously applied by Free et al., 2021, 2022).
2.2.2 Meteo-climatic data
The ancillary meteo-climatic data were derived from ERA5, which is the fifth-generation reanalysis for the global climate and weather for the past decades carried out by the European Centre for Medium-Range Weather Forecasts (ECMWF). ERA5 provides hourly estimates for a large number of atmospheric, ocean-wave and land-surface climate variables. Data is regridded to a regular latitude-longitude grid of 0.25° for the reanalysis and 0.5° for the uncertainty estimate. In particular, the hourly data downloaded for this analysis were: mean snowmelt rate, snow depth, 10 m v-component of wind (south-north direction), 10 m u-component of wind (west-east direction), 2 m temperature, total precipitation (Table 1). Hourly values were aggregated to daily and weekly syntheses. Data are for the three focus sub-basins (Angara, Barguzin, Selenga).
2.3 Trend and statistical analysis
The input time series extract for the variables of interest were processed and analyzed using R and RStudio open-source environment (R Core Team, 2013; Hijmans and van Etten, 2012) and QGIS (QGIS Development Team, 2020). First, we analysed time series individually by observing one variable at a time for each sub-basin (i.e., BA, precipitation) and lake ROI (i.e., Chl-a, Turbidity) for the period covered by the datasets (2003–2020). Since the CCI Chl-a and Turbidity products have a 4-year gap and were derived from different EO source data, water quality variables were analyzed separately for 2003–2011 and 2016–2020. Moreover, only summer months, June to September, were considered to retain observations from ice-free days. The normalized Z-score statistic was used to identify anomalous events for Chl-a and Turbidity based on a weekly standardized difference. The formula used to calculate it is:
Where xw,i is the weekly average of Chl-a or Turbidity in a given year, μw and σw are, respectively, the mean and the standard deviation of the parameter in the week w and computed separately for the two periods (p1: 2003–2011, p2: 2016–2020). Z-scores allow us to visualize the difference, in terms of standard deviation, relative to the period mean for a given week, highlighting values above and below the average and providing information on the direction of the phenomenon; in our case, we are looking at extreme values greater than the average (positive Z-scores). We calculated also the burned area proportion as the ratio between weekly burned area and sub-basin area.
We investigated the correlation between input variables and, in particular, the correlation between occurrence of significant wildfires and precipitation events that could trigger the increase of Chl-a and Turbidity as a consequence of sediments transport from the river network. First, Non-Parametric Multiplicative Regression (NPMR) (McCune, 2006a) was used to estimate the response of the Chl-a and Turbidity to the meteorological variables (ERA5; listed in Table 1) and to burned area (cumulative BA) for the two periods 2003–2011 and 2016–2020 due to the 4-year gap in the EO derived water quality data. The months included in the analyses were June-September. NPMR can define response surfaces using predictors in a multiplicative rather than in an additive way. This method is progressive in better defining unimodal responses than other methods such as multiple regression (McCune, 2006a). NPMR was applied using the software HyperNiche version 2.3 (McCune and Mefford, 2009). The response of Chl-a was estimated using a local mean multiplicative smoothing function with Gaussian weighting. NPMR models were produced by adding predictors stepwise with fit expressed as a cross-validated R2 (xR2) which can be interpreted in a similar way as a measure of fit like a traditional R2. The sensitivity, a measure of influence of each variable included in the NPMR model, was estimated by altering the range of predictors by ±0.05 (i.e., 5%) with resulting deviations scaled as a proportion of the observed range of the response variable. Sensitivity can be used to evaluate the relative importance of variables included in models because NPMR models are unlike linear regression and have no fixed coefficients or slopes. More details including the mathematical formula along with worked examples have been published (McCune, 2006a; McCune, 2006b; McCune and Mefford, 2009). These options selected together with the data used allow for repetition of the analysis.
3 Results
3.1 Time series analysis
3.1.1 Burned area
The BA product provided the amount of area burned for the three sub-basins and for each day (through the Day Of the Year) of the period of interest 2003–2020. Figure 2 shows the distribution of the annual area burned per land cover and the monthly amounts for the three sub-basins of the study area. To make results easier to present, we aggregated land cover class into six major categories: Cropland, Forest, Mixed Natural, Shrubs, Grass and Sparse vegetation. Overall, most of the fires occurred in forest with more than 70% of the total area burned for the 18-year period covered by this analysis; the other important classes for burning were Cropland (13%) and Grassland (8%). These trends confirm previous studies on global distribution of fires across biomes (Giglio et al., 2013). A north to south gradient of the land cover affected by fires can be observed with most of the burns in the Forest class for the Angara sub-basin and the largest proportion of burned surface in Forest and Mixed natural for Barguzin, and Forest and Cropland for Selenga (Figure 2).
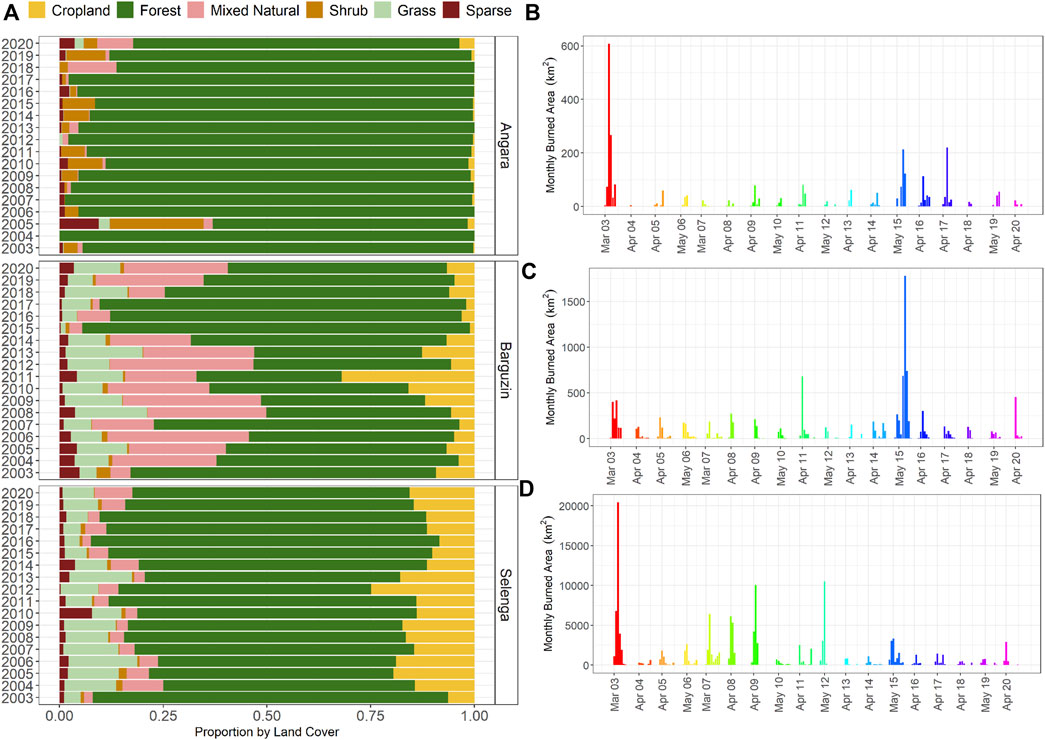
FIGURE 2. The annual area burned by Land Cover (A) and the seasonality of Firecci51 BA totals for the three sub-basins: Angara (B), Barguzin (C) and Selenga (D). Notice that the y-axis range change by sub-basin as a consequence of the different rate of area affected by fires and the three regions.
In Supplementary Table S1 the total burned area for each year and sub-basin investigated is reported in the Supplementary Materials section. Looking at the inter-annual variability of fires, 2003 was the most intense year for the Selenga and Angara sub-basins with total annual burned area respectively of 34,410 km2 (8% of the sub-basin surface) and 1,067 km2 (5% of the sub-basin surface); in Barguzin, the largest amount of area burned was 3,929 km2 in 2015 (18% of the sub-basin surface).
The seasonal distribution of burned areas is graphically presented in Supplementary Figure S1 (Supplementary Materials) where color scale from blue to red represents the proportion of area burned in each month with respect to total annual value. The figure clearly shows differences among the sub-basins primary as a function of the latitude: Angara is the basin with shortest fire season and lowest amount of area burned. Months with greatest area burned are April and May for the southernmost basins (Barguzin and Selenga) while fire season start later for the Angara basin (i.e., June in most of the years analysed) (Fig. S1).
3.1.2 Water quality variables
Figures 3, 4 show monthly boxplots of Chl-a and Turbidity, respectively, and trend lines from Ordinary Least Square (OLS) regression. Within the considered periods, p1 and p2, Chl-a and Turbidity concentrations (median) did not change significantly (p > 0.05), with exceptions of the decreasing Chl-a trend for the p1 period in Barguzin and Selenga (p < 0.05) (Figure 3) and of the Turbidity increasing trend in the Barguzin (p < 0.05) (Figure 4). In these cases, despite a significant trend, both significance level and R2 (<0.1) are low. Only considering the entire period (2003–2020), both Chl-a and Turbidity values have positive trends that are highly significant (p < 0.001, ***) but, given the 4-year gap, this result is not reliable.
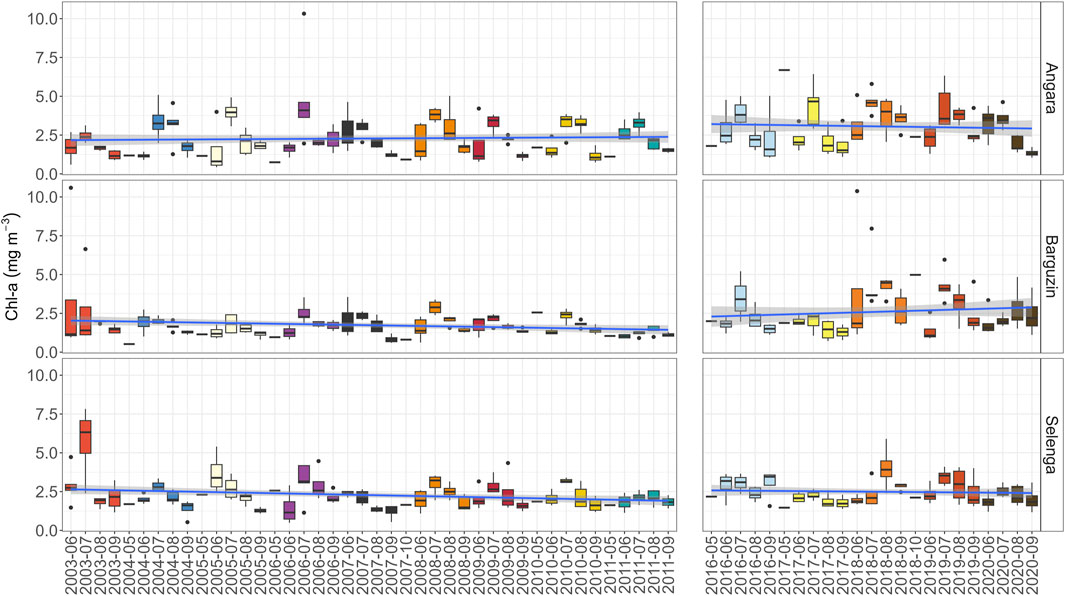
FIGURE 3. Boxplots of daily chlorophyll-a (Chl-a; mg m-3) values showing monthly statistics (median, quartiles, range and outliers) split for the two periods: 2003–2011 and 2016–2020 for the three basins.
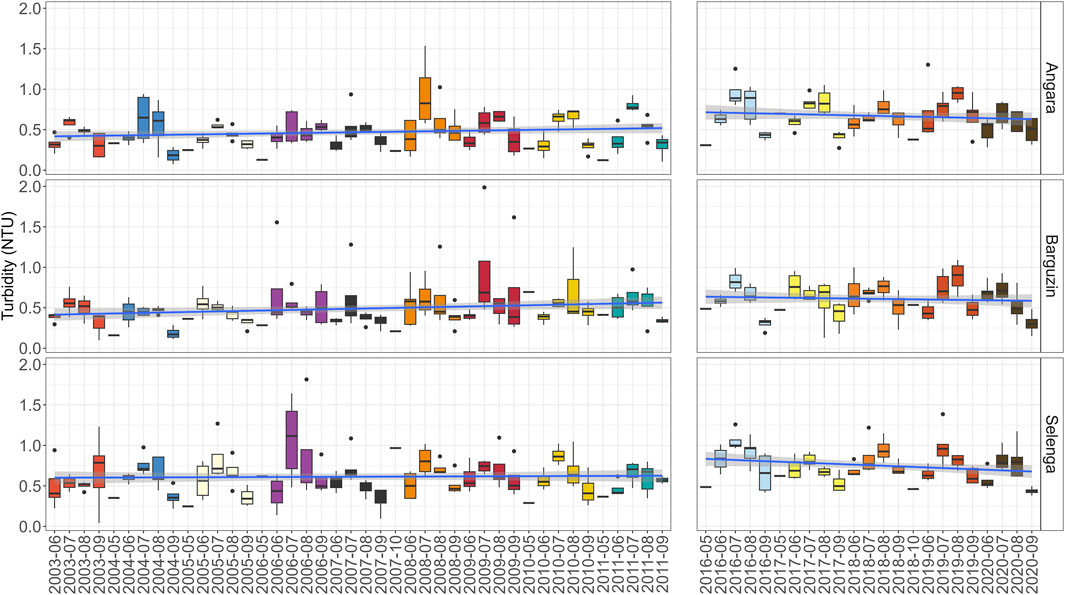
FIGURE 4. Boxplots of daily Turbidity (NTU) values showing monthly statistics (median, quartiles, range and outliers) split for the two periods: 2003–2011 and 2016–2020 for the three basins.
The Chl-a average (±standard deviation) concentration for the period June-September was 2.28 ± 1.24 in Angara, 1.73 ± 1.00 mg m-3 in Barguzin and 2.27 ± 1.03 mg m-3 in Selenga during p1, while 3.06 ± 1.36 mg m-3 in Angara, 2.61 ± 1.56 mg m-3 in Barguzin and 2.49 ± 0.84 mg m-3 in Selenga during p2. In Figure 3, we observe a slight increase in the median Chl-a values in July from 2004 to 2006 in the Angara sub-basin. Turbidity average was 0.47 ± 0.21 NTU in Angara, 0.49 ± 0.26 NTU in Barguzin and 0.61 ± 0.26 NTU in Selenga during p1, while 0.67 ± 0.21 NTU, 0.61 ± 0.21 NTU and 0.75 ± 0.21 NTU in the three sub-basins, respectively during p2. Detailed results of the OLS fitting analysis are reported in Supplementary Table S2.
In order to highlight anomalous weekly values of Chl-a concentration and Turbidity, possibly induced by fires, we aggregated daily values into weekly averages also to limit the impact of missing daily observation in monitoring the dynamic biological processes. Figure 5 shows weekly values of the standardized difference with respect to the long-term/multi-annual weekly average for Chl-a and Turbidity for the three sub-basins Angara, Barguzin and Selenga, respectively. Positive (weekly average above the multi-annual average) and negative (weekly average below multi-annual average) are represented as green and red, respectively, and the ratio between weekly burned area and basin area (burned area proportion) is also plotted as black bars. As in previous graphs, the two period p1 and p2 were treated independently. This figure could help in identifying temporal correlation with positive or negative weekly values significantly different from the average concentration of Chl-a and Turbidity in the period p1 and p2 likely due to fire activity. In all the sub-basins both positive and negative Z-score values were observed with a high variability for both variables.
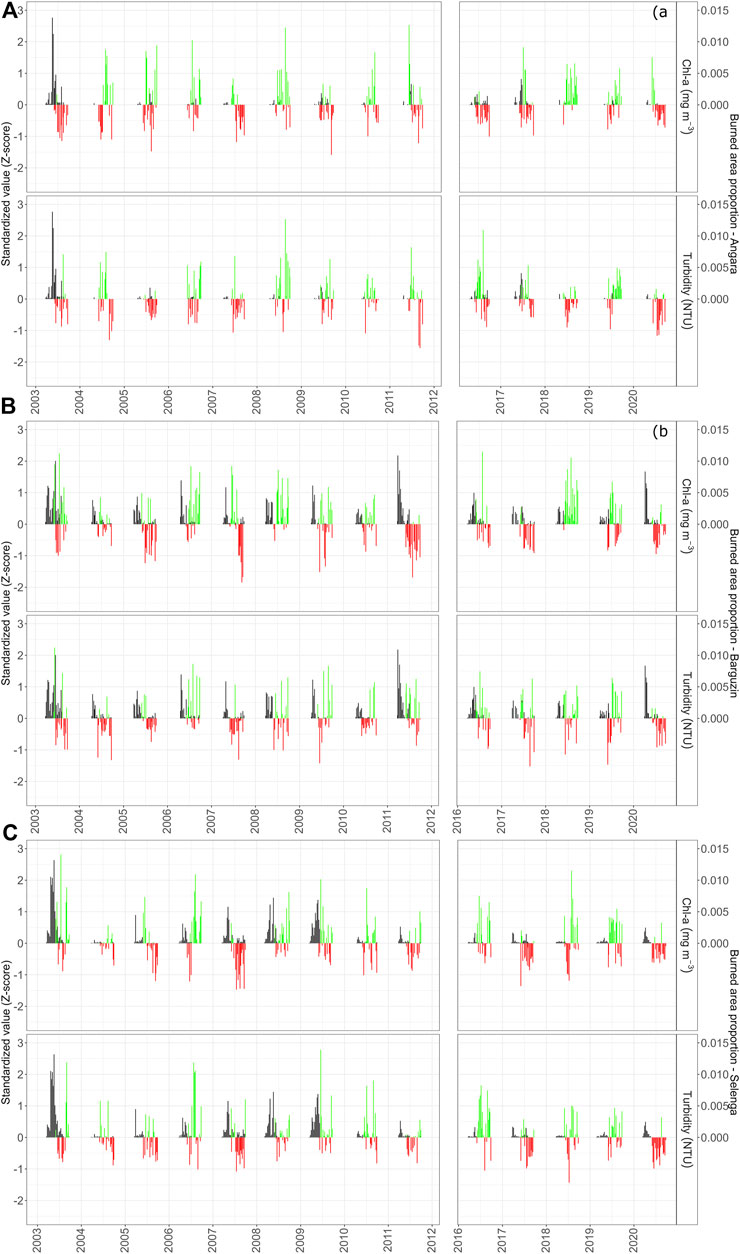
FIGURE 5. Z-score for chlorophyll-a (Chl-a) and Turbidity computed over the 2003–2011 (left column) and 2016–2020 (right column) for the Angara (A), Barguzin (B) and Selenga (C) sub-basins; positive and negative values are plotted as green and red and the ratio between weekly burned area and sub-basin area (burned area proportion) is plotted as black bars (value on the secondary y-axis).
3.1.3 Precipitation
The ERA5 dataset included the total precipitation which were extracted on a daily basis for the three sub-basins. In Supplementary Table S1 the total annual precipitation for Angara, Barguzin and Selenga is reported. It is clear a gradient of decreasing precipitation from north to south in all years of the period 2003–2020. Annual precipitation was on average 786 ± 87 mm in Angara, 611 ± 94 mm in Barguzin, and 429 ± 33 mm in Selenga. In Angara and Barguzin sub-basins the maximum precipitation was registered in 2008 (973 and 808 mm, respectively), while the minimum values were registered in 2016 (615 mm) and 2015 (409 mm), respectively. The range of precipitation in Selenga sub-basin in the period investigated is from 369 to 476 mm registered in 2014 and 2018, respectively. Supplementary Figure S2 shows the distribution of daily precipitation (grouped by months with the boxplot statistics) for the three sub-basins for the period 2003–2020: the range of daily precipitation varies from 0 to 40 mm with the most wet periods in July and August. The analysis carried out with Ordinary Least Square (OLS) regression for daily precipitation showed that there is no significant trend in rainfall for the three sub-basins (p > 0.05).
3.2 Non-parametric Multiplicative Regression (NPMR): Fire, meteo-climatic, and water quality data
Non-parametric Multiplicative Regression (NPMR) was used to estimate the response of average weekly Chl-a and Turbidity concentration to climate and the environmental parameters (Table 2). Analysis was carried out for each of the three catchments for both time periods (2003–2011 and 2016–2020). The results are presented in two steps (Table 2), the first being when only the time component was included as the predictor and the second step was when other variables were introduced step-wise to increase the xR2. Generally, the time component was most important, accounting for an average of 83% of the xR2 when comparing step 1 (just time component) with step 2 (all variables). Considering models with only the time, the weekly component was more important, having an average sensitivity value across models of 0.46 compared with 0.18 for year. Of the six regression models for Chl-a performed with environmental components (3 sub-basins × 2 time periods) fire was included as a variable in three of them with sensitivity ranging from 0.03 to 0.23. For turbidity, fire was included in three models with sensitivity ranging from 0.05 to 0.34. Fire was not consistently included in models for either parameters, time-period or sub-basin. Wind components were also included in three models for both Chl-a and Turbidity. For the two sub-basins situated to the east of Lake Baikal the u wind component was significant in the models with a sensitivity ranging from 0.04 to 0.18. In contrast, for the sub-basin to the south of Lake Baikal (Selenga) the v wind component was included in the models (sensitivity 0.01–0.16). Rain was included in three models of the two eastern sub-basins with sensitivity ranging from 0.2 to 0.5, but was not included in the southern Selenga sub-basin. Snow depth was included in two models but at low sensitivity (<0.1). Air temperature was included in five models with sensitivity ranging from 0.01 to 0.33.
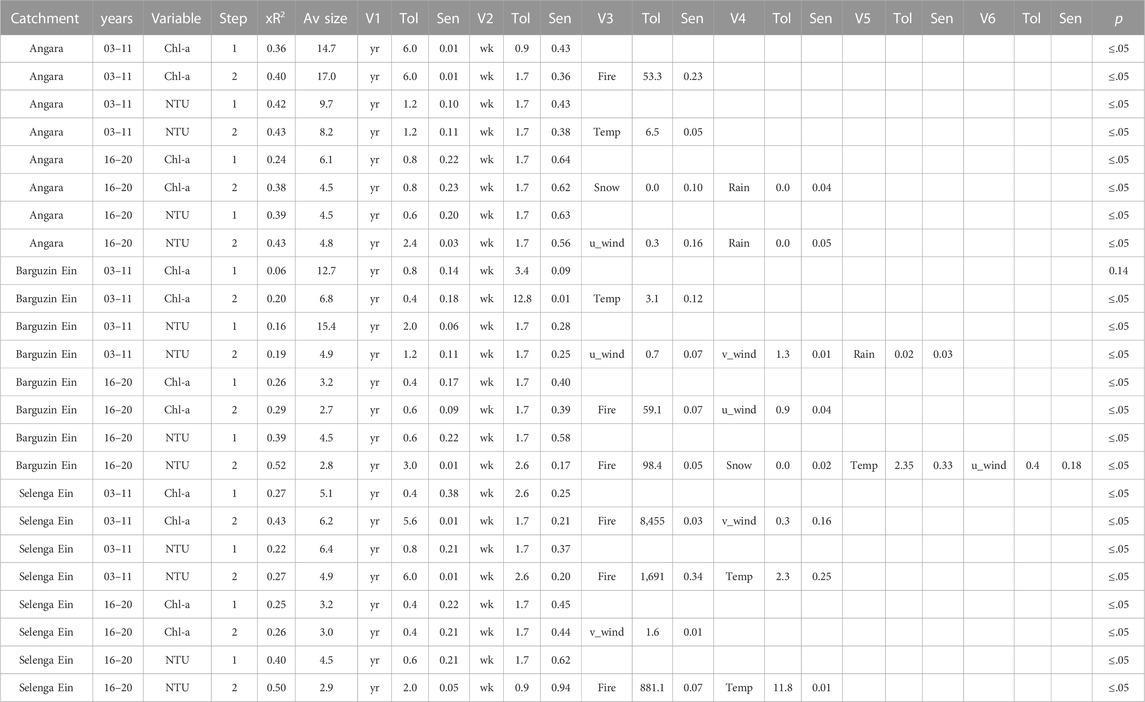
TABLE 2. Results of NPMR (Non-parametric Multiplicative Regression) models for chlorophyll-a (Chl-a) and Turbidity (NTU). Analysis was carried out by sub-basin, years (2003–2011 and 2016–2020), step 1 with only time variables, step 2 with all variables included xR2 = cross-validated R2; Av size = Average neighborhood size; V1 = variable 1, Tol. = Tolerance; Sen. = Sensitivity. Variables included in models: year (yr); week (wk); Fire (Cumulative burned area km2); Temperature (Temp); Snow (snow depth m); Rain (precipitation mm); v_wind (v wind component); u_wind (u wind component).
4 Discussion
In order to interpret the findings of our study, it is necessary to consider the main characteristics of Lake Baikal, which include large extension (length of >600 km) along the north-south axis, extreme depth (mean depth >700 m) and an overall condition of low nutrients concentrations and low biological productivity. Previous studies showed a large-scale north-south gradient in physical, chemical and biological parameters (Goldman et al., 1996). The lake catchment is vast (>550,000 km2) and covering a diverse range of land cover types, land morphology (in latitude and altitude), snow cover amount and ice formation and break up timing. All these gradients are reflected in the characteristics of three different sub-basins investigated, which are aligned along the north-south axis, and ultimately in their role in transferring fire-derived burned material and soil-released nutrients to the lake. According to our initial hypothesis, the runoff of burned materials and nutrients to the lake might affect its water quality inducing detectable changes in Chl-a and Turbidity.
4.1 Time series of fire, water quality variables, and precipitations
The first aim of this study was the analysis of time series of burned area, water quality variables derived from EO datasets and precipitation derived from model reanalysis dataset in three main sub-basins of the large oligotrophic Lake Baikal.
In this study, we found a gradient from north to south in terms of amount of burned areas and land cover types, with the norther Angara sub-basin characterized by the smallest burned areas and Forest types most affected, while the southern Selenga sub-basin with the largest burned areas affecting mainly Croplands beyond Forest. The middle sub-basin Barguzin was characterized by intermediate amount of burned areas affecting mostly Forest and Mixed natural land cover types. The fire occurrence was registered mainly during the spring months (April-May) for Barguzin and Selenga, while later in June for the norther Angara. The seasonality of precipitation could affect vegetation water content and consequently fire occurrence and propagation. In the Baikal boreal region, precipitation occurred mainly in the summer, which means commonly after fire occurrence during the studied period in the three sub-basins investigated.
Years most affected by fires, in terms of burned areas, were 2003 for Angara and Selenga and 2015 for Barguzin. Indeed, 2003 was one of the most devastating fire years in Eastern Siberia in the recent history due to very dry winter season and above normal high temperatures in summer months (Sitnov and Mokhov, 2018; Natole et al., 2021). Although the high number of fire events was observed in all boreal regions from Northern Eurasia to Northern America, some exceptions can be pointed out as in the case of the Barguzin basin where 2003 burned area was half the amount of area burned in the worst year 2015, which was an exceptional dry year for this sub-basin (year with minimum precipitation in the period 2003–2020).
In a recent work, Safronov (2020) analyzed EO-derived land cover products in the eastern portion of Lake Baikal catchment for an 8-year period (2010–2018) finding that the surface covered by forests was around 20%, and that this area decreased by about 2.4% with the highest deforestation occurring between 2013 and 2017. In addition, the combination of active fire and burned area products with precipitation data allowed the identification of the relationship between aridity and fire hotspot. The author stated also that these two factors alone could not explain the steep increase (20-fold) in the number of wildfires. In fact, this study concluded that natural ignitions sources such as dry thunderstorms and pyrocumulus lightning can be the sources of fire in remote regions, but fires located closer to settlements and human activities might be of anthropogenic nature.
In our study we detected a gradient of decreasing precipitations from north to south, in the three different sub basins, with the northern Angara being the wettest basin with the highest annual average precipitations; these conditions were observed every year during the whole time period from 2003 to 2020 (see Supplementary Table S1; Supplementary Figure S2). Time series analysis of precipitation (OLS regression) during the considered period shows no significant trend.
Chl-a and turbidity concentrations did not show a clear temporal pattern along the two periods investigated with few significant exceptions. In fact, in the period 2003–2011 Chl-a showed a decreasing trend in the southern basins (Barguzin and Selenga), while Turbidity showed an increasing trend in Barguzin These findings suggest that the different areas investigated of Lake Baikal are still oligotrophic with average Chl-a ranging from 1.7 to 3.0 mg m-3 (OECD, 1982; Poikane et al., 2011) with clear waters (average Turbidity below 1 NTU). Similarly low values are present in other lakes in this region, with Chl-a ranging from 0.2 to 3.2 mg m-3, attributed to large catchments with low vegetation and human activity (Mitamura et al., 2010). We can speculate that this deep lake shows resilience in the medium-long term to ongoing climate change effects and anthropogenic pressures.
Focusing on the fire events occurred in 2003, which have caused an extremely large burned area, it is observable from our results that during late spring/summer of the following years no significant increases of Chl-a and Turbidity concentration occurred. Smith et al. (2011) also observed that large storage reservoirs might reduce the magnitude and rate of change of suspended solids inputs from tributary streams thus resulting in lower peaks, which can be the case of the large and deep Baikal Lake. However, in the Angara sub-basin, which is characterized by later vegetation period, fire occurrence (May-June) and snowmelt timing, we observed an increase of the Chl-a median values in July in the 3 years following 2003, which can be explained by the release of dissolved constituents by upstream snowmelt favouring downstream phytoplankton growth. Murphy et al., 2015 observed that rain storm events post-wildfire are the main drivers of the high increase in suspended solids, DOC and nitrate concentrations in a river watershed in Colorado, US. They highlighted also that the inter-annual variations in run-off depends on snowmelt from headwater areas. Therefore, precipitation intensity in the burned area was most important for sediment load instead snowmelt runoff affects mainly DOC load. Moreover, Mast et al. (2016) reported for the Big Thompson River in Colorado a 2-year study after wildfire events that burned 15% of the catchment. They found that the dissolved constituents from the watershed increased 20%–52% in the first 2 years following the fire, in particular, the major ions concentration increased after the first postfire snowmelt period, and nitrate in the second one. In addition, they found that turbidity due to sediment transport was instead influenced mainly by summer storms.
4.2 Relationships between fire, meteo-climatic data, and water quality variables
The second aim of this study was the analysis of the spatio-temporal relationships between fire occurrence, meteo-climatic conditions and changes in Chl-a and Turbidity for the three sub basins of Lake Baikal. Our hypothesis was that the impact of vegetation removal due to wildfires on the erosion and surface transport processes of sediments and nutrients, triggered by rainfall events, could induce changes in Turbidity and/or concentration of Chl-a in Baikal.
In order to investigate short-term effects of precipitations as runoff carrier of burned material and nutrients to the lake, we performed a graphic analysis evaluating the succession of fire events, periods of precipitation and detectable changes in water quality variables. A general response was not observed, while inter-annual differences were found among sub-basins. In Selenga during 2003 concentration of Turbidity and Chl-a started to increase one and 3 weeks after the high-intensity fires (weeks 16–21), associated with precipitation events, with maximum Chl-a concentration (7.8 mg m-3) reached during the 30th week (Supplementary Figures S3A,B). In the same year, in Barguzin sub-basin a prolonged fire season correspond to a peak in Chl-a (10.6 mg m-3) during the 24th week (Supplementary Figure S4A). However, in Barguzin after another intense fire (2011) and following precipitation, there was no positive response in Chl-a concentration (Supplementary Figure S4B).
In order to estimate the response of Chl-a and Turbidity concentration to fires and to climate and environmental parameters, Non-parametric Multiplicative Regression (NPMR) was utilized. The results for all the regression models, combined in three sub-basins (Angara, Barguzin, and Selenga) by two time periods (p1 = 2003–2011 and p2 = 2016–2020), showed that temporal variability (seasonal and annual) was the main predictor of Chl-a and Turbidity concentrations. Additional parameters included in the models resulted significant for different sub-basins. In the northern Angara cumulative burned area and precipitation parameters, such as rain and snow, resulted important factors especially during p2. Angara has a longer ice and snow period and a shorter vegetation period (Goldman et al., 1996) and in our results, Angara is also the sub-basin with more total and annual average precipitation (Supplementary Table S1; Supplementary Figure S2) when compared to the other sub-basins and its catchment includes vast mountain areas (Figure 1). Angara in its upper course flows in a west-southwest direction across mountainous terrain, with rapids and waterfalls, thus it is likely to be considerably affected by runoff of burned material and released nutrients to the lake. In the southern Selenga sub-basin, fires and southerly winds (v wind component) were significant factors for both chlorophyll-a and turbidity concentrations. The importance of southerly winds in the model may indicate that burned material derived from fires occurring in the Selenga sub-basin have been transported by southerly winds to the lake surface, directly affecting lake water quality variables. However, this hypothesis will need further investigation to be tested by appropriate models including atmospheric variables. The lack of precipitation parameters in the Selenga models may indicate that, although dealing with a very vast sub-basin, burned materials and nutrients released in the soil may not be transported to the lake water within a short time-frame. Selenga is a major river in the region and, when approaching Lake Baikal, it crosses a broad alluvial plain where it slows down and deposits sediments (United States Geological Survey, 2010). The presence of extensive croplands and the activities of water extraction and canalization for agricultural uses from the Selenga (Mun et al., 2008) are possibly important factors interfering with the transport via river or terrestrial pathways of fire-derived burned material and associated nutrients to the recipient lake. For the Barguzin sub-basin, fires and easterly winds (u-wind component) were the most significant factors affecting Chl-a and turbidity, indicating a potential effect of burned material derived from fires, which are located in the eastern part of the lake, being transported directly by winds to the recipient lake.
In conclusion, in this study we have not observed a clear and consistent response of water quality variables to the occurrence of fires in Baikal catchment. Several reasons could explain this result. First of all, the enormous water volume of Lake Baikal, although being oligotrophic, may be unlikely to be affected in a short time scale by regional fire events, since burned material produced and nutrients released from the soil may be too small to result in quick and permanent changes in water quality. Second, burned material and nutrients may find it difficult to actually reach the lake water body because the lake catchment, and in particular the outflow areas of the three main tributaries, may function as filters for nutrient input to the lake. Fire-derived nutrients may be taken up by soils or vegetation before reaching the lake, as was documented in other studies, i.e., Alaska (Lewis et al., 2014). In Minnesota a large forest fire caused increased phosphorus levels in runoff waters, but much of this phosphorus was immobilized in the soil before reaching nearby lakes (McColl and Grigal, 1975). The important role of the rivers deltas in retaining fire-derived material and nutrients is supported by previous studies on the Selenga River, the main tributary of Lake Baikal (Khodzher et al., 2017; Roberts et al., 2020); the river loads its water to the lake, together with pollutants and micro- and macro-nutrients, which are the successfully filtered by the extensive delta (5,000 km2, Sorokovikova et al., 2017) before flowing to the lake. Another important reason for the lack of a clear response of water quality variables to fires could be related to some limitations of satellite observations (e.g., cloud cover) in the boreal region. While the burned area signal in satellite images is persistent (removal of vegetation cover can be detected later than the occurrence of the fire), anomalies in water quality estimates can be detected only from simultaneous observations.
Other studies on the effect of fires on boreal lake’s water quality (Lewis et al., 2014) found that nutrients (nitrogen and phosphorus) and chlorophyll concentrations were not affected by fires. Although we did not find clear general relationships between the occurrence of fires and changes in Lake Baikal water quality variables, our study has highlighted the importance of geographical and morphological features in lake sub-basins. Northern Angara was the sub-basin with more annual precipitation and a prolonged period of ice and snow cover, with river Angara flowing through steep mountain areas in which rain and snow meltwater may quickly drain and transport fire-derived nutrients to the lake. In the NPMR analysis, the water quality variables of Angara ROIs were the most influenced by precipitation parameters (snow and rain) and in the time series analysis it was the sub basin with an indication of Chl-a summer increase lasted for one–three years following the intense fire of 2003. Angara had more precipitations than the other sub basins, more fire-derived material and nutrients that are transported through atmospheric vectors may fall directly onto the lake surface, triggering slight increases in primary production and Chl-a.
Our findings fit into the body of literature supporting that the intrinsic complexity associated to lake responses to wildfire is dependent on a wide range of different ecological variables. For example, McCullough et al. (2019) in their review on the effects of fire on lakes in the United States suggested that lake responses may be weaker compared to river and stream, but prolonged in time depending on factor such as water residence time. These effects are commonly rapid depending on fire extent and severity and lasted for two-three years following fire. They also pointed out that there is a knowledge gap in quantifying how much fire effect in rivers propagate downstream to lakes and how these waterbodies are sensitive to river input, which depends on factors such as lake volume, water residence time and trophic status.
Data availability statement
The datasets analyzed for this study can be found in online repositories. Products from both Fire_cci and Lakes_cci projects were downloaded from the Centre for Environmental Data Analysis archive (CEDA, https://catalogue.ceda.ac.uk/) as part of the ESA CCI Open Data Portal Project.
Author contributions
MP, DS, RC, MB, and CG formulated the original concept for this study. LP, GT, DS, MP, RC, and GF, implemented the data processing and analysis. MP, RC, and DS drafted the manuscript. MB, CG, and CA revised the manuscript. All authors contributed to the final manuscript and gave final approval for publication.
Funding
This work is part of an ESA funded Climate Change Initiative projects (Lakes_cci Contract Nos. 40000125030/18/I-NB and Fire_cci Contract Nos. 4000126706/19/I-NB).
Acknowledgments
Satellite products were downloaded from the Centre for Environmental Data Analysis archive (CEDA, https://catalogue.ceda.ac.uk/) within the ESA CCI project framework. The ERA5 meteo-climatic data from ERA5, were provided by ECMWF. We would like to thanks Stefan Simis and Jean-François Crétaux for the useful discussion for the analysis developed in this study. We would like to acknowledge the reviewers for valuable comments and suggestions that helped improve this study.
Conflict of interest
The authors declare that the research was conducted in the absence of any commercial or financial relationships that could be construed as a potential conflict of interest.
Publisher’s note
All claims expressed in this article are solely those of the authors and do not necessarily represent those of their affiliated organizations, or those of the publisher, the editors and the reviewers. Any product that may be evaluated in this article, or claim that may be made by its manufacturer, is not guaranteed or endorsed by the publisher.
Supplementary material
The Supplementary Material for this article can be found online at: https://www.frontiersin.org/articles/10.3389/frsen.2023.1107275/full#supplementary-material
References
Adrian, R., O'Reilly, C. M., Zagarese, H., Baines, S. B., Hessen, D. O., Keller, W., et al. (2009). Lakes as sentinels of climate change. Limnol. Oceanogr. 54 (6), 2283–2297. doi:10.4319/lo.2009.54.6_part_2.2283
Brown, K. P., Gerber, A., Bedulina, D., and Timofeyev, M. A. (2021). Human impact and ecosystemic health at Lake Baikal. Wiley Interdiscip. Rev. Water 8 (4), e1528. doi:10.1002/wat2.1528
Cannon, S. H., Gartner, J. E., Wilson, R. C., Bowers, J. C., and Laber, J. L. (2008). Storm rainfall conditions for floods and debris flows from recently burned areas in southwestern Colorado and southern California. Geomorphology 96 (3-4), 250–269. doi:10.1016/j.geomorph.2007.03.019
Carrea, L., Crétaux, J. F., Liu, X., Wu, Y., Calmettes, B., Duguay, C. R., et al. (2023). Satellite-derived multivariate world-wide lake physical variable timeseries for climate studies. Scientific Data 10 (1), 30.
CEDA (2022a). CEDA archive. Available at: https://catalogue.ceda.ac.uk/uuid/01b00854797d44a59d57c8cce08821eb (Accessed July, 2022).
CEDA (2022b). CEDA archive. Available at: https://catalogue.ceda.ac.uk/uuid/a07deacaffb8453e93d57ee214676304 (Accessed July, 2022).
Chuvieco, E., Mouillot, F., van der Werf, G. R., San Miguel, J., Tanase, M., Koutsias, N., et al. (2019). Historical background and current developments for mapping burned area from satellite Earth observation. Remote Sens. Environ. 225, 45–64. doi:10.1016/j.rse.2019.02.013
Chuvieco, E., Roteta, E., Sali, M., Stroppiana, D., Boettcher, M., Kirches, G., et al. (2022). Building a small fire database for Sub-Saharan Africa from Sentinel-2 high-resolution images. Sci. total Environ. 845, 157139. doi:10.1016/j.scitotenv.2022.157139
Colman, S. M. (1998). Water-level changes in Lake Baikal, Siberia: Tectonism versus climate. Geology 26 (6), 531–534. doi:10.1130/0091-7613(1998)026<0531:WLCILB>2.3.CO;2
Free, G., Bresciani, M., Pinardi, M., Simis, S., Liu, X., Albergel, C., et al. (2022). Investigating lake chlorophyll-a responses to the 2019 European double heatwave using satellite remote sensing. Ecol. Indic. 142, 109217. doi:10.1016/j.ecolind.2022.109217
Free, G., Giardino, C., Pinardi, M., et al. (2021). Climate assessment Report. European Space Agency. Accessible at: https://climate.esa.int/media/documents/CCI-LAKES-0044-CAR_v3.0.pdf.
Giglio, L., Loboda, T., Roy, D. P., Quayle, B., and Justice, C. O. (2009). An active-fire based burned area mapping algorithm for the MODIS sensor. Remote Sens. Environ. 113 (2), 408–420. doi:10.1016/j.rse.2008.10.006
Giglio, L., Randerson, J., and van der Werf, G. (2013). Analysis of daily, monthly, and annual burned area using the Fourth-Generation Global Fire Emissions Database (GFED4). J. Geophys. Res. Biogeosciences 118 (1), 317–328. doi:10.1002/jgrg.20042
Goldman, R. C., Elser, J. J., Richards, R. C., Reuter, J. E., Priscu, J. C., and Levin, A. L. (1996). Thermal stratification, nutrient dynamics, and phytoplankton productivity during the onset of spring phytoplankton growth in Lake Baikal, Russia. Hydrobiologia 331, 9–24. doi:10.1007/BF00025403
Hersbach, H., Bell, B., Berrisford, P., Hirahara, S., Horányi, A., and Muñoz-Sabater, J. (2020). The ERA5 global reanalysis. Quarterly Journal of the Royal Meteorological Society 146(730), 1999–2049.
Hijmans, R. J., and van Etten, J. (2012). raster: Geographic analysis and modeling with raster data. Available at: http://CRAN.R-project.org/package=raster.
Khodzher, T. V., Domysheva, V. M., Sorokovikova, L. M., Sakirko, M. V., and Tomberg, I. V. (2017). Current chemical composition of Lake Baikal water. Inland Waters 7 (3), 250–258. doi:10.1080/20442041.2017.1329982
Lehner, B., and Grill, G. (2013). Global river hydrography and network routing: Baseline data and new approaches to study the world’s large river systems. Hydrol. Process. 27 (15), 2171–2186. doi:10.1002/hyp.9740
Lewis, T. L., Lindberg, M. S., Schmutz, J. A., and Bertram, M. R. (2014). Multi-trophic resilience of boreal lake ecosystems to forest fires. Ecology 95 (5), 1253–1263. doi:10.1890/13-1170.1
Liu, D., Zhou, C., Keesing, J. K., Serrano, O., Werner, A., Fang, Y., et al. (2022). Wildfires enhance phytoplankton production in tropical oceans. Nat. Commun. 13 (1), 1348–1349. doi:10.1038/s41467-022-29013-0
Liu, X., Steele, C., Simis, S., Warren, M., Tyler, A., Spyrakos, E., et al. (2021). Retrieval of Chlorophyll-a concentration and associated product uncertainty in optically diverse lakes and reservoirs. Remote Sens. Environ. 267, 112710. doi:10.1016/j.rse.2021.112710
Lizundia-Loiola, J., Otón, G., Ramo, R., and Chuvieco, E. (2020). A spatio-temporal active-fire clustering approach for global burned area mapping at 250 m from MODIS data. Remote Sens. Environ. 236, 111493. doi:10.1016/j.rse.2019.111493
Malmon, D. V., Reneau, S. L., Katzman, D., Lavine, A., and Lyman, J. (2007). Suspended sediment transport in an ephemeral stream following wildfire. J. Geophys. Res. Earth Surf. 112 (2), F02006. doi:10.1029/2005JF000459
Mast, M. A., Murphy, S. F., Clow, D. W., Penn, C. A., and Sexstone, G. A. (2016). Water-quality response to a high-elevation wildfire in the Colorado Front Range. Hydrol. Process. 30 (12), 1811–1823. doi:10.1002/hyp.10755
McColl, J. G., and Grigal, D. F. (1975). Forest fire: Effects on phosphorus movement to lakes. Science 188 (4193), 1109–1111. doi:10.1126/science.188.4193.1109
McCullough, I. M., Cheruvelil, K. S., Lapierre, J. F., Lottig, N. R., Moritz, M. A., Stachelek, J., et al. (2019). Do lakes feel the burn? Ecological consequences of increasing exposure of lakes to fire in the continental United States. Glob. Change Biol. 25 (9), 2841–2854. doi:10.1111/gcb.14732
McCune, B., and Mefford, M. J. (2009). HyperNiche. Nonparametric multiplicative habitat modeling. USA: MjM Software: Gleneden Beach, OR.
McCune, B. (2006b). Non-parametric habitat models with automatic interactions. J. Veg. Sci. 17, 819–830. doi:10.1111/j.1654-1103.2006.tb02505.x
McCune, B. (2006a). Nonparametric multiplicative regression for habitat modeling. Corvallis, OR, USA: Oregon State University.
Mitamura, O., Khadbaatar, D., and Noriko, I. (2010). Comparative investigation of chemical and biological characteristics in waters and trophic state of Mongolian lakes. Limnology 11, 17–30. doi:10.1007/s10201-009-0280-x
Moody, J. A., and Martin, D. A. (2009). Synthesis of sediment yields after wildland fire in different rainfall regimes in the Western United States. Int. J. Wildland Fire 18 (1), 96–115. doi:10.1071/WF07162
Moody, J. A., Shakesby, R. A., Robichaud, P. R., Cannon, S. H., and Martin, D. A. (2013). Current research issues related to post-wildfire runoff and erosion processes. Earth-Science Rev. 122, 10–37. doi:10.1016/j.earscirev.2013.03.004
Moore, M. V., Hampton, S. E., Izmest'Eva, L. R., Silow, E. A., Peshkova, E. V., and Pavlov, B. K. (2009). Climate change and the world's “sacred sea”—lake Baikal, Siberia. BioScience 59 (5), 405–417. doi:10.1525/bio.2009.59.5.8
Mueller, H., Hamilton, D. P., and Doole, G. J. (2016). Evaluating services and damage costs of degradation of a major lake ecosystem. Ecosyst. Serv. 22, 370–380. doi:10.1016/j.ecoser.2016.02.037
Mun, Yu., Ko, I. H., and Janchivdorj, L. (2008). Integrated water management model on the selenge river basin. Status Survey and investigation (phase I), seoul. Tae Joo Park: Korea Environment Institute. Available at: http://repository.kei.re.kr/handle/2017.oak/19384.
Murphy, J. D., Johnson, D. W., Miller, W. W., Walker, R. F., and Blank, R. R. (2006a). Prescribed fire effects on forest floor and soil nutrients in a Sierra Nevada forest. Soil Sci. 171 (3), 181–199. doi:10.1097/01.ss.0000193886.35336.d8
Murphy, J. D., Johnson, D. W., Miller, W. W., Walker, R. F., Carroll, E. F., and Blank, R. R. (2006b). Wildfire effects on soil nutrients and leaching in a Tahoe Basin watershed. J. Environ. Qual. 35 (2), 479–489. doi:10.2134/jeq2005.0144
Murphy, S. F., Writer, J. H., McCleskey, R. B., and Martin, D. A. (2015). The role of precipitation type, intensity, and spatial distribution in source water quality after wildfire. Environ. Res. Lett. 10 (8), 084007. doi:10.1088/1748-9326/10/8/084007
Natole, N., Ying, Y., Buyantuev, A., Stessin, M., Buyantuev, V., and Lapenis, A. (2021). Patterns of mega-forest fires in east Siberia will become less predictable with climate warming. Environ. Adv. 4, 100041. doi:10.1016/j.envadv.2021.100041
Naumenko, S. A., Logacheva, M. D., Popova, N. V., Klepikova, A. V., Penin, A. A., Bazykin, G. A., et al. (2017). Transcriptome-based phylogeny of endemic Lake Baikal amphipod species flock: Fast speciation accompanied by frequent episodes of positive selection. Mol. Ecol. 26 (2), 536–553. doi:10.1111/mec.13927
Pacheco, F. A., and Fernandes, L. F. S. (2021). Hydrology and stream water quality of fire-prone watersheds. Curr. Opin. Environ. Sci. Health 21, 100243. doi:10.1016/j.coesh.2021.100243
Pereira, J. M. C., Chuvieco, E., Beaudoin, A., and Desbois, N. (1997). “A review of remote sensing methods for the study of large wildland fires,” in Report of the megafires project ENV-CT96-0256. August 1997. Editor E. Chuvieco (Alcala de Henares: Universidad de Alcala).
Poikane, S., van den Berg, M., Hellsten, S., de Hoyos, C., Ortiz-Casas, J., Pall, K., et al. (2011). Lake ecological assessment systems and intercalibration for the European water framework directive: Aims, achievements and further challenges. Procedia Environ. Sci. 9, 153–168. doi:10.1016/j.proenv.2011.11.024
Popovicheva, O., Molozhnikova, E., Nasonov, S., Potemkin, V., Penner, I., Klemasheva, M., et al. (2021). Industrial and wildfire aerosol pollution over world heritage Lake Baikal. J. Environ. Sci. 107, 49–64. doi:10.1016/j.jes.2021.01.011
Prosser, I. P., and Williams, L. (1998). The effect of wildfire on runoff and erosion in native Eucalyptus forest. Hydrol. Process. 12 (2), 251–265. doi:10.1002/(SICI)1099-1085(199802)12:2<251::AID-HYP574>3.0.CO;2-4
QGIS Development Team (2020). QGIS geographic information system. Open source geospatial foundation project. Available at: http://qgis.osgeo.org.
R Core Team (2013). R: A language and environment for statistical computing. Vienna, Austria: R Foundation for Statistical Computing. Available at: http://www.R-project.org/.
Roberts, S., Adams, J. K., Mackay, A. W., Swann, G. E., McGowan, S., Rose, N. L., et al. (2020). Mercury loading within the Selenga River basin and Lake Baikal, Siberia. Environ. Pollut. 259, 113814. doi:10.1016/j.envpol.2019.113814
Robichaud, P. R., Elliot, W. J., Pierson, F. B., Hall, D. E., and Moffet, C. A. (2007). Predicting postfire erosion and mitigation effectiveness with a web-based probabilistic erosion model. Catena 71 (2), 229–241. doi:10.1016/j.catena.2007.03.003
Roy, D. P., Boschetti, L., Justice, C. O., and Ju, J. (2008). The collection 5 MODIS burned area product — global evaluation by comparison with the MODIS active fire product. Remote Sens. Environ. 112 (9), 3690–3707. doi:10.1016/j.rse.2008.05.013
Safronov, A. N. (2020). Effects of climatic warming and wildfires on recent vegetation changes in the Lake Baikal Basin. Climate 8 (4), 57. doi:10.3390/cli8040057
Shimaraev, M. N., Verbolov, V. I., Granin, N. G., and Sherstyankin, P. P. (1994). Physical limnology of Lake Baikal: A review. Okayama- Irkutsk: BICER Publishers, 81.
Simis, S., Selmes, N., Calmettes, B., Duguay, C., Merchant, C. J., Malnes, E., et al. (2020). ESA lakes climate change initiative (Lakes_cci): Product user guide. doi:10.5285/3C324BB4EE394D0D876FE2E1DB217378
Sitnov, S., and Mokhov, I. (2018). A comparative analysis of the characteristics of active fires in the boreal forests of eurasia and North America based on satellite data. Izv. Atmos. Ocean. Phys. 54 (9), 966–978. doi:10.1134/S0001433818090347
Smith, H. G., Sheridan, G. J., Lane, P. N., Nyman, P., and Haydon, S. (2011). Wildfire effects on water quality in forest catchments: A review with implications for water supply. J. Hydrology 396 (1-2), 170–192. doi:10.1016/j.jhydrol.2010.10.043
Solovyev, V. S., Kozlov, V. I., and Mullayarovy, V. A. (2009). Satellite Monitoring of Forest Fires and Thunderstorm Activity in Yakutia ed S I Solovyev (Yakutsk: Publishing House of Yakutian Scientific Center of Siberian Branch of the Russian Academy of Sciences).
Solovyev, V. S., and Budishchev, A. A. (2010). Disturbances of aerosol optical thickness of the atmosphere caused by forest fires in Yakutia. Atmospheric and Oceanic Optics 23 (6), 538–541.
Sorokovikova, L. M., Sinyukovich, V. N., Tomberg, I. V., Popovskaya, G. I., Chernyshev, M. S., Ivanov, V. G., et al. (2017). The status of the aquatic ecosystem of the Selenga river delta under long-duration low-water conditions. Geogr. Nat. Resour. 38 (1), 60–67. doi:10.1134/S1875372817010085
Subin, Z. M., Murphy, L. N., Li, F., Bonfils, C., and Riley, W. J. (2012). Boreal lakes moderate seasonal and diurnal temperature variation and perturb atmospheric circulation: Analyses in the community earth system model 1 (CESM1). Tellus A Dyn. Meteorology Oceanogr. 64 (1), 15639. doi:10.3402/tellusa.v64i0.15639
United States Geological Survey (2010). The Selenga River Delta, USGS Landsat image archive. Available at: https://eros.usgs.gov/media-gallery/image-of-the-week/the-selenge-river-delta.
Warren, M. A., Simis, S. G., and Selmes, N. (2021). Complementary water quality observations from high and medium resolution Sentinel sensors by aligning chlorophyll-a and turbidity algorithms. Remote Sens. Environ. 265, 112651. doi:10.1016/j.rse.2021.112651
Whitman, E., Parisien, M. A., Thompson, D. K., and Flannigan, M. D. (2019). Short-interval wildfire and drought overwhelm boreal forest resilience. Sci. Rep. 9 (1), 18796–18812. doi:10.1038/s41598-019-55036-7
Williamson, C. E., Dodds, W., Kratz, T. K., and Palmer, M. A. (2008). Lakes and streams as sentinels of environmental change in terrestrial and atmospheric processes. Front. Ecol. Environ. 6, 247–254. doi:10.1890/070140
Williamson, C. E., Saros, J. E., Vincent, W. F., and Smol, J. P. (2009). Lakes and reservoirs as sentinels, integrators, and regulators of climate change. Limnol. Oceanogr. 54 (6), 2273–2282. doi:10.4319/lo.2009.54.6_part_2.2273
Keywords: chlorophyll-a, turbidity, lakes, climate change, wildfires, timeseries
Citation: Pinardi M, Stroppiana D, Caroni R, Parigi L, Tellina G, Free G, Giardino C, Albergel C and Bresciani M (2023) Assessing the impact of wildfires on water quality using satellite remote sensing: the Lake Baikal case study. Front. Remote Sens. 4:1107275. doi: 10.3389/frsen.2023.1107275
Received: 24 November 2022; Accepted: 13 February 2023;
Published: 06 March 2023.
Edited by:
Deepak R. Mishra, University of Georgia, United StatesReviewed by:
Filiz Dadaser-Celik, Erciyes University, TürkiyeIleana Aracely Callejas, University of California, Los Angeles, United States
Copyright © 2023 Pinardi, Stroppiana, Caroni, Parigi, Tellina, Free, Giardino, Albergel and Bresciani. This is an open-access article distributed under the terms of the Creative Commons Attribution License (CC BY). The use, distribution or reproduction in other forums is permitted, provided the original author(s) and the copyright owner(s) are credited and that the original publication in this journal is cited, in accordance with accepted academic practice. No use, distribution or reproduction is permitted which does not comply with these terms.
*Correspondence: Mariano Bresciani, bresciani.m@irea.cnr.it