- 1Department of Water and Climate Risk, Institute for Environmental Studies, VU Amsterdam, Amsterdam, Netherlands
- 2Urban Technology, Amsterdam University of Applied Sciences, Amsterdam, Netherlands
- 3School of Environment, Water and Natural Resources Management, SEKU, Kwa Vonza, Kenya
- 4Department of Geography, University of California, Santa Barbara, Santa Barbara, CA, United States
In Eastern Africa, increasing climate variability and changing socioeconomic conditions are exacerbating the frequency and intensity of drought disasters. Droughts pose a severe threat to food security in this region, which is characterized by a large dependency on smallholder rain-fed agriculture and a low level of technological development in the food production systems. Future drought risk will be determined by the adaptation choices made by farmers, yet few drought risk models … incorporate adaptive behavior in the estimation of drought risk. Here, we present an innovative dynamic drought risk adaptation model, ADOPT, to evaluate the factors that influence adaptation decisions and the subsequent adoption of measures, and how this affects drought risk for agricultural production. ADOPT combines socio-hydrological and agent-based modeling approaches by coupling the FAO crop model AquacropOS with a behavioral model capable of simulating different adaptive behavioral theories. In this paper, we compare the protection motivation theory, which describes bounded rationality, with a business-as-usual and an economic rational adaptive behavior. The inclusion of these scenarios serves to evaluate and compare the effect of different assumptions about adaptive behavior on the evolution of drought risk over time. Applied to a semi-arid case in Kenya, ADOPT is parameterized using field data collected from 250 households in the Kitui region and discussions with local decision-makers. The results show that estimations of drought risk and the need for emergency food aid can be improved using an agent-based approach: we show that ignoring individual household characteristics leads to an underestimation of food-aid needs. Moreover, we show that the bounded rational scenario is better able to reflect historic food security, poverty levels, and crop yields. Thus, we demonstrate that the reality of complex human adaptation decisions can best be described assuming bounded rational adaptive behavior; furthermore, an agent-based approach and the choice of adaptation theory matter when quantifying risk and estimating emergency aid needs.
Introduction
Droughts regularly affect communities, leading to water and food shortages, reduced crop yields, loss of livelihood, and famine (Barron et al., 2003; Ifejika et al., 2008). The impacts are difficult to quantify because they are often delayed and may last several years (Wilhite, 2000; United Nations Development Programme, 2007). Moreover, the magnitude of these impacts not only depends on the severity of the drought event and the number of people exposed but also on how people adapt to periods of reduced water availability (Mude et al., 2007; Birkmann et al., 2013). Although several studies have dealt with uncertainties in estimating drought hazard, the interplay between adaptation and drought risk has often been neglected (Wens, 2019). Consequently, vulnerability has typically been included as a static factor, which assumes a “business-as-usual” level of future adaptation (Adger et al., 2018; De Pinto et al., 2019; Wens et al., 2019).
In reality, adaptive behavior is highly dynamic (Dobbie, 2013; De Koning, 2019). People implement drought adaptation measures based on past experiences and changes in their natural and socioeconomic environment (e.g., Wilhite, 2002; Stefanovi, 2015; González et al., 2016). Understanding the dynamic interplay between the physical water system and human adaptation has sparked the novel socio-hydrology scientific field (Sivapalan et al., 2012; Baldassarre et al., 2015). Recognizing this socio-hydrological feedback is found necessary for better understanding the fluctuations in drought risk over time, driven by a combination of physical drivers (e.g., climate variability), socioeconomic developments, and human adaptive behaviors (e.g., Van Loon et al., 2016; Hagenlocher et al., 2019).
Research has attempted to simulate the adaptive decisions of individuals facing the harmful effects of hazard events using recognized economic theories, such as the expected utility theory (EUT, Von Neumann and Morgenstern, 1945) for economic rational decision-making under uncertainty (e.g., Haer et al., 2019). However, human adaptive behavior under uncertain conditions is rarely rational (Eiser et al., 2012; Holden, 2015), and people tend to exhibit “bounded rational” logic when deciding on adaptation measures (Asgary and Levy, 2009; Van Duinen et al., 2016). For example, research in disaster risk management has shown people overestimate the probability of rare events, and adaptive behavior and risk perception are shaped by factors including worry, past experiences, and socioeconomic conditions (Tongruksawattana, 2014; Mwongera et al., 2017).
The existence of bounded rational behavior has been confirmed by studies on agricultural drought risk (e.g., Van Duinen et al., 2012; Gebrehiwot and van der Veen, 2015; Elagib et al., 2017), evidenced by low adoption of wells and irrigation measures among farmers, despite such measures being economically efficient (Ngigi et al., 2005b; Khisa et al., 2014b; Bouma et al., 2016; Wambua and Akuja, 2016). It has been suggested that farmers' adaptation decisions are influenced by a biased perception of risk and a lack of trust in their own control over drought risk (Murgor et al., 2013; Ochieng et al., 2016; Nkatha, 2017; Khisa, 2018; Van Valkengoed and Steg, 2019). Other factors that have been shown to influence the adoption of adaptation practices include limited access to financial, human, social, natural, and physical capital (Kalungu et al., 2013; Matere et al., 2016; Bunclark et al., 2018). Knowledge dissemination through social networks and the gaining of required skills through extension services are increasingly seen as essential for improving the agricultural drought practices of smallholder farmers (Kitinya et al., 2012; Van Duinen et al., 2016).
Although the importance of including such complex human adaptive behavior in risk assessments is increasingly recognized (Palmer and Smith, 2014; Groeneveld et al., 2017; Schlüter et al., 2017), identifying the key variables that steer adaptation decisions is difficult (e.g., Klabunde and Willekens, 2016; Aerts et al., 2018). Alternative theories for modeling adaptive behavior—adding psychological and sociological drivers in addition to economic ones—can be applied to overcome this challenge. Examples of such complex theories include the prospect theory (Kahneman and Tversky, 1979; Asgary and Levy, 2009; Holden and Quiggin, 2017), the theory of planned behavior (Wheeler et al., 2013; Sutton, 2014; Van Dijk et al., 2016), and the protection motivation theory (Maddux and Rogers, 1983; Grothmann and Patt, 2005). Among these, the protection motivation theory (PMT) is one that has been successfully applied to describe farmers' dynamic drought-adaptive behavior in multiple studies (Dang et al., 2014b; Gebrehiwot and van der Veen, 2015; Van Duinen et al., 2015a; Keshavarz and Karami, 2016; Zheng and Dallimer, 2016).
In the present research, we studied the factors that drive drought adaptation decisions of smallholder farmers by comparing business-as-usual and economic rational behavior—the latter modeled following the EUT–, with the more complex, empirically supported bounded rational behavior—modeled following the PMT. We developed an innovative dynamic drought risk adaptation model, ADOPT, which links the physical crop growth model Aquacrop (FAO, 2009; Vanuytrecht et al., 2014; Foster et al., 2017b) with a behavioral model capable of simulating each of the abovementioned scenarios. ADOPT thus simulates the adaptive actions and interactions of individual farm households in relation to experienced agricultural drought risk. It applies an agent-based approach, the primary tool for modeling individual adaptation decisions and complex interactions (Railsback and Grimm, 2012). In agent-based models (ABMs), agents (e.g., government, households) have the capacity to learn and adapt in response to changes in other agents and the environment (Matthews et al., 2007; Palmer and Smith, 2014). ABMs provide a bottom-up method for tracing behavior over time, and simulate human–human and human–environment interactions at the local level, which can lead to the emergence of patterns at the macro-level (Dobbie et al., 2018; Wens et al., 2019). Such models include probabilistic functions that describe the individual behavioral dynamics of heterogeneous decisions-makers with different socio-economic backgrounds, are actively applied to study farmers' behavior in several contexts, such as drought management and farm innovation (Barreteau et al., 2004; Gunkela and Külls, 2011; Schreinemachers and Berger, 2011; Van Oel and Van Der Veen, 2011; Van Duinen et al., 2012; Blair and Buytaert, 2016). In this paper, the ADOPT model framework is showcased for subsistence households in semi-arid rural Kenya over the period 1982–2013. Survey data on household behavior in Kitui, Kenya (Wens, 2019) were used to create a heuristic understanding of the co-evolution of drought risk and human adaptation decisions and to initialize the agents (farm households) in the model. The intent of this study is not to be predictive; but to demonstrate how an agent-based approach and the choice of behavioral theory affect the estimation of drought vulnerability and risk over time.
The remainder of this paper is organized as follows: section Case Study Description introduces the semi-arid study area in Kenya for which the model is calibrated. Section ADOPT Model Description contains the model description detailing both agricultural drought simulations using the FAO crop model Aquacrop, and human decision simulations following three scenarios: business-as-usual, economic rational (expected utility theory), and bounded rational (protection motivation theory). Section Results presents the results of drought risk simulations using ADOPT, and section Discussion provides the discussion and conclusions on how different assumptions on adaptive behaviors influence drought risk estimations.
Materials and Methods
Case Study Description
The case study is representative for the rural areas of three semi-arid counties, Kitui (30.430 km2, 1.136187 citizens), Machakos (6.043 km2, 1.421.932 citizens), and Makueni (8.008 km2, 987,653 citizens) in south-eastern Kenya (TEGEMEO, 2000; ILRI, 2006; Rapsomanikis, 2010; Wens, 2018, 2019) (Ilri, data Tegemeo Institute, 2000, 2004, 2007, 2010; Wens, 2019). They are characterized by a dry savanna/warm tropical climate (Njoka et al., 2016). Agriculture in this area is dominated by rain-fed subsistence production systems with households largely dependent on crop and livestock production for income (United Nations Development Programme, 2007; Wambua and Akuja, 2016). Maize remains the most important food crop, and drought-induced yield reductions of maize are largely synonymous with food insecurity and dependence on external aid (Brooks et al., 2005, 2009; Alessandro et al., 2015). High temperatures coupled with unreliable rainfall have caused significant shocks for rural communities in past decades, such as in 1999/2000, 2004/2005, 2010/2011, and 2017/2019 (Erenstein et al., 2011b; Kioko, 2013). Furthermore, extreme temperature and rainfall deficiency events have been occurring on an increasingly frequently basis (FEWSNET, 2010; Khisa et al., 2014a; Government of the Republic of Kenya, 2017; Khisa, 2017).
Kenyan households have a long history of adapting to droughts using traditional and emerging practices (Black et al., 2012; Recha et al., 2012; KEFRI et al., 2014; Shiferaw et al., 2014; Kalungu et al., 2015; Kimani et al., 2015; Gbegbelegbe et al., 2017). An example is the building of fanya juu terraces, which are a combination of trenches and sand bunds in sloping cropland to for increase the storage of runoff on horizontally created terraces (Biamah et al., 1993; Makurira et al., 2011; Hailu et al., 2012; Muriu et al., 2017; Wolka et al., 2018). Another method is residue mulching, which involves covering the soil surface with plant material to retain soil moisture through reduced evaporation and increased infiltration (Okeyo et al., 2014; Mo et al., 2016; Mfitumukiza et al., 2017; Mugambiwa, 2018). While the maintenance of these two in-soil water storage measures can be demanding in terms of labor, implementation knowledge is available and they do not require large initial investments (Lasage and Verburg, 2015). Irrigation—although highly efficient economically (Nakawuka et al., 2018)—is less popular among smallholder farmers because the implementation of irrigation techniques requires advanced and often costly infrastructure, technical knowledge, and institutional support (Sijali and Okumu, 2002; Ngigi et al., 2005a; Kulecho and Weatherhead, 2006; Ngigi, 2019). Moreover, the amount of surface runoff that many areas receive is too small to irrigate (Barron and Okwach, 2005; Rockström and Falkenmark, 2015). To provide extra water, cost-effective shallow wells can be installed, which can be linked to automated irrigation systems, such as a drip system (Ngigi, 2003; Venzi et al., 2015).
Recently, the authors administered a survey in the case study area to build an understanding on households' drought vulnerability dynamics and changing capacity to cope with droughts (Wens, 2018, 2019). The data collection method involved administration of a short questionnaire among employees of Kenyan national disaster coordination units (n = 10); semi-structured expert interviews (n = 10) with NGOs, governmental water authorities, and pioneer farmers in the Kitui district in Kenya; and an in-depth questionnaire among smallholder farmers in central Kitui (n = 250). While this questionnaire only provides data about a snapshot in time, questions were focused on the dynamics of vulnerability. Rather than asking only questions related to current practices, the survey was designed to also inquire aspirations, challenges and intentions to adopt new drought adaptation measures in the past and the future. Based on this survey, the following on-farm drought adaptation measures were considered in the present research: (i) the improvement of in-soil storage using mulch cover; (ii) the construction of fanya juu terraces; (iii) the digging of shallow wells on property; and (iv) the installation of drip irrigation infrastructure. Currently, mulch, fanya juu, well, and drip irrigation techniques are applied by 15, 45, 15, and 5% of households interviewed in the area, respectively. The survey was also applied to create economic household profiles (see Appendix A), estimate the investment and maintenance costs of the measures, and drive the utility functions and decision rules of the ADOPT model.
ADOPT Model Description
ADOPT (Figure 1) works on the resolution of a subsistence farm managed by one rural household, and consists of two dynamically linked subroutines: (i) the agricultural model AquacropOS (Foster et al., 2017a), which simulates maize yield based on crop characteristics, soil characteristics, daily weather conditions and farm water management (blue box in Figure 1; subsection Simulating Annual Maize Yield per Farm); and (ii) a behavioral model, which can simulate the adaptation decisions of households assuming either business-as-usual behavior, or through applying a behavioral theory: the expected utility theory (EUT, assuming economic rational behavior) or the protection motivation theory (PMT, assuming bounded rational behavior) (red box in Figure 1; subsection Simulating the Adaptive Behavior of Subsistence Farmers). This setup allows for the assessment of socio-hydrological feedbacks between farm decisions concerning the level of drought adaptation measures and the drought impacts they experienced (purple arrows; subsection Simulating Annual Drought Impact for Subsistence Farmers): in ADOPT, it is explicitly modeled how drought adaptation measures influence crop yield, which impacts the farm income thus household food security and financial assets, which ultimately alters farmers' risk perception and capacity to adopt new adaptation measures (as detailed impact assessments are highly location-specific and effective adaptation depends on the understanding of drought risk at scales close to which decisions are made, the spatial resolution of the model is at the field scale of the farm households (on average 0.6 ha). A complete model description including an overview, design concepts, details, and decision making, as well as a summary of the input data can be found in Appendix A, following the ODD + D protocol for ABMs (Müller et al., 2013).
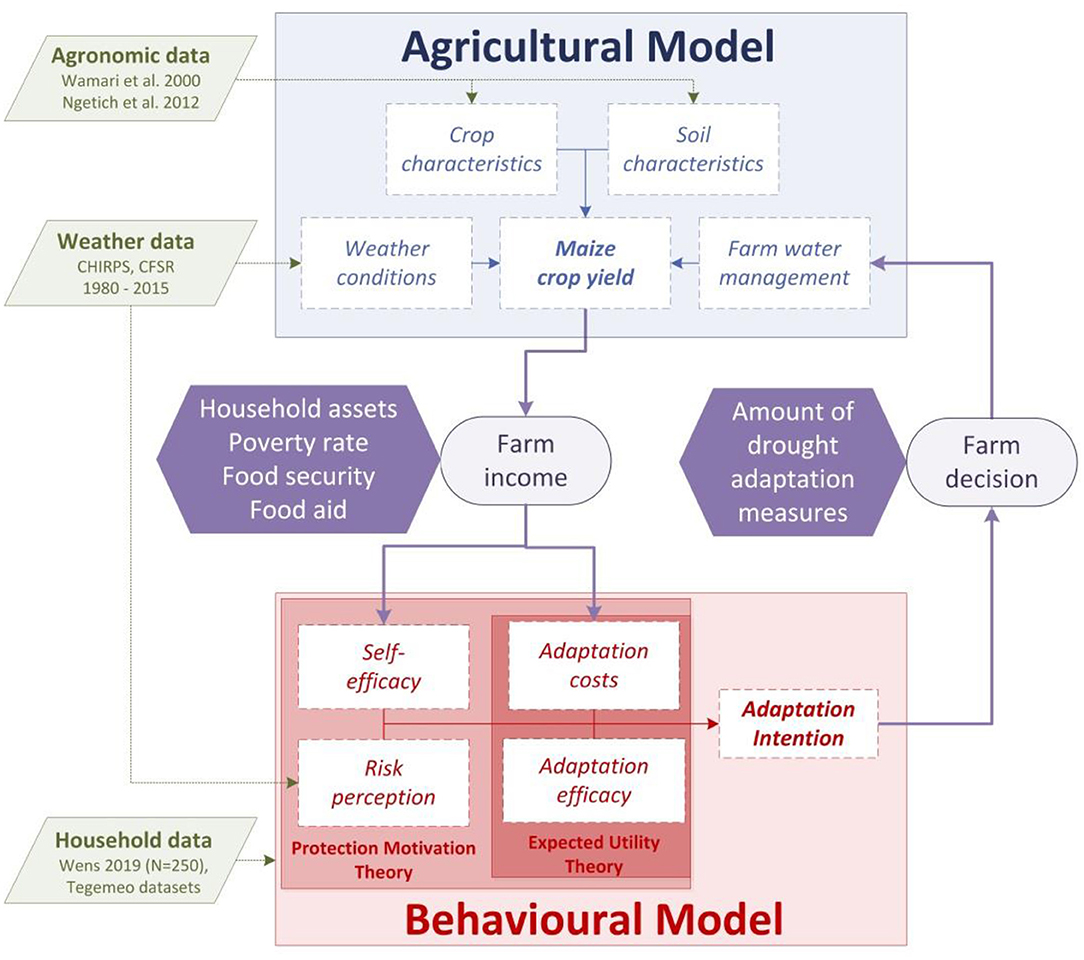
Figure 1. Modeling scheme of the agricultural drought risk adaptation model ADOPT. Seasonal maize crop production is simulated using weather and agronomic data in AquacropOS at the household scale (Blue). The resulting household maize yield is translated into farm income, which is fed into a behavioral model (Red). Adaptive behavior is modeled per farm household using one of three scenarios: No adaptive behavior, economic rational adaptive behavior, or bounded rational behavior. Following expected utility theory, the intention to adapt is modeled to be a function of Adaptation costs, adaptation efficacy, and household assets. Following protection motivation theory, the intention to adapt is a function of risk perception, self-efficacy, adaptation costs, and adaptation efficacy. The individual farm households' intention to adapt leads to the yearly decision whether or not to adopt a new adaptation measure. These adaptation decisions influence their future on-farm water management, thus establishing a feedback. Survey, weather, and agronomic data used as input to the model (Green), whereas yearly risk indicators (household assets, poverty, food insecurity, and food aid) and the adoption rate of drought adaptation measures are outputs of the model (purple).
Simulating Annual Drought Impact for Subsistence Farmers
To simulate individual crop yield and adaptation decisions over time, a heterogeneous sample of 1,000 farm households in an area of 100 km2, representative for the case of Kitui, Makueni, or Machakos, was chosen to be initialized (Tegemeo Institute, 2000, 2004, 2007, 2010; Wens, 2018, 2019). These households have several characteristics that influence their annual harvest, adaptation decisions, and drought vulnerability, including family and farm size, social network, access to extension services, possible adaptation measures, and off-farm income sources. All household characteristics were stochastically derived from the averages and standard deviations of different household characteristics in previous research and a questionnaire (n = 250) by Wens (2019) performed as part of this study (Appendix A). The average farm size is 0.6 ha, heterogeneously distributed among the households. Other spatial characteristics, such as proximity to a river of town were omitted, as they do not influence the model variables. To simulate seasonal market volatility in response to maize availability, the average maize price of Kitui Town market (US$0.35/kg) was weighted by comparing the percentage of total seasonal harvest with the average (30 years) harvest in the study area. From this, maize prices fluctuate between US$0.2/kg (favorable seasons) and US$0.5/kg (seasons with drought-induced crop losses; (Winter-nelson and Amegbeto, 1998; Nyoro et al., 2005; FEWSNET, 2018).
ADOPT runs as follows:
▪ For each season and household, maize production is simulated using AquacropOS (subsection 2.2.2) based on daily weather conditions as well as the drought adaptation measures applied by the households. This maize harvest is partly allocated to account for the households' food needs—estimated as 103 kg per year per adult (DTMA, 2015) and any additional harvest is sold (farm income, increasing the households' financial assets). Shortages are made up through purchasing (reducing the households' financial assets) at the maize price of the simulated season.
▪ Each year, all households spend money on non-food and farm input (expenditure), reducing their financial assets, and have a potential off-farm income source (e.g., casual labor, livestock breeding, private business, and brick making) that increases their financial assets. Moreover, based on demographic data, a household could increase or decrease in size over the simulation period, altering its food demand (demographic numbers are based on Wens, 2019).
▪ Each year, all households evaluate their intention to adopt a new drought adaptation measure. This intention is influenced by the household's financial assets and the behavioral rules of the scenario applied (subsection Simulating the Adaptive Behavior of Subsistence Farmers). The adoption of such measures influences the households' individual maize production in the following years.
To express the direct and indirect effects drought risk, it is chosen to track, in addition to agricultural production, the following metrics in ADOPT:
▪ Poverty (households) is calculated per household assuming a poverty line of US$ 1 per day.
▪ Food insecurity (households) occurs if households' food needs exceeds their maize production.
▪ Food aid (US$) is estimated as the food shortage of all households multiplied by the maize price.
The cumulative amount of food shortage (kg) is estimated following two procedures. The first excludes the agent-based approach, assuming all farm households have the same farm area, number of family members, and are equally wealthy (and rich enough to buy their food needs). In this procedure, food shortage is calculated by examining how many households the total regional food supply (sum of the harvest for all households) could feed. Food shortage is thus the difference between supply and needs. The second includes the agent-based approach and food shortage is calculated on an individual household level. Food shortage occurs if households are in food insecurity, and if they do not have the financial means to meet their needs or if the regional food supply does not allow them to buy the extra maize required to fulfill their food needs. The difference between the two procedures helps exemplifying the added value of an agent-based approach.
Simulating Annual Maize Yield per Farm
The open source version of the FAO crop-water model Aquacrop, AquacropOS (Steduto et al., 2009, 2012; Foster et al., 2017a; Foster and Brozović, 2018), was used to simulate biomass and harvestable yield responses of maize to water availability (Vanuytrecht et al., 2014). The model is designed for regions with water-limited agricultural production, such as semi-arid Kenya. By explicitly modeling the plant growth up to harvestable yield, AquacropOS enables the assessment of the effects of water and agricultural management on crop production (Heng et al., 2009). It has been used by numerous studies in Kenya (e.g., Ngetich et al., 2012; Wamari et al., 2012; Omoyo et al., 2015).
Historical weather data (1981–2013; Figure 2) for Kitui were used as input for AquacropOS, which includes the daily gridded CHIRPS rainfall dataset (Funk et al., 2015b), which combines 0.05° resolution satellite imagery with in-situ station data. Furthermore, daily minimum and maximum temperature (°C), relative humidity, wind speed (m/s), and solar radiation (s) from the Kitui area were obtained from the Climate Forecast System Reanalysis (CFSR) dataset from the National Center for Environmental Prediction of the United States National Oceanic and Atmospheric Administration (Dile and Srinivasan, 2014). These data were employed to calculate reference evapotranspiration using the Penman–Monteith equation (Allen, 2004; Ayugi et al., 2020).
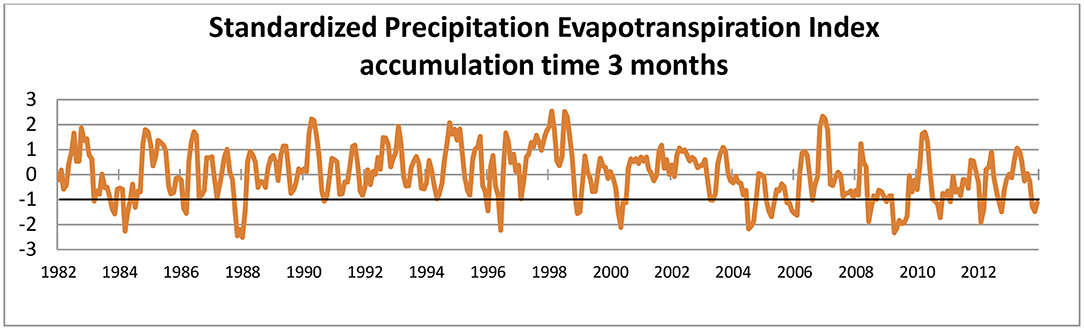
Figure 2. Standardized Precipitation Evaporation Index (SPEI) calculated using CHIRPS Precipitation data and CFSR Evaporation data for 1982–2013. An accumulation time of 3 months was used to show the seasonal climate variability. Values below 0 indicate conditions dryer than average. If such dryer than average conditions are prolonged and occur during the crop growing cycle, drought impacts on agricultural production can be expected.
Maize-specific parameters, such as the duration of flowering and number of plants per hectare were derived from Ngetich et al. (2014) and Wamari et al. (2012), who conducted an extensive study on the calibration and validation of Aquacrop for Katumani maize in Kenya. Remaining factors were calibrated to obtain realistic crop yield in the range (700–1,200 in good years) reported by Brooks et al. (2009). The period of cropping (growing period of 75–180 days) is limited to the rainy seasons (May–June and October–February) (Government of Kenya, 2007; Black et al., 2012; Mo et al., 2016). The hydraulic properties of the soil were adopted from the case study in semi-arid Kenya executed by Ngetich et al. (2014).
To simulate the effect on crop yield for the four drought adaptation measures discussed, AquacropOS was run for all possible combinations of these measures: It is possible for a household to have a well, perform manual or drip irrigation, and/or have fanya juu terraces, and/or apply mulch to fields simultaneously (see section Case Study Description). Here it was assumed that households who apply mulch to their fields were assumed to have a year round 50% coverage of mulch, which AquacropOS converted to a lower evaporation from the soil by 0.6 (Raes et al., 2012). Households with fanya juu terraces were assumed to have contour pits and bunds with a height of 60 cm (Wolka et al., 2018). Households with a shallow well are assumed to manually water their crops in times of deficit; the maximum irrigation depth was set at 12 mm, the wetted area was set at 30%, and the soil moisture target was set at 40% depletion (Filho and de Trinchera Gomez, 2018). Since no distributed hydrological model was included, wells are assumed to provide enough water for irrigation at any time, not influenced by the digging of other wells in their surroundings or by long-lasting droughts. In our AquacropOS setup, we assumed that manual watering was a soil moisture-based technique: households would water their field if it feels dry (below 50% of total plant available water). Furthermore, the application efficiency was assumed to be rather low: 45% for manual watering (Howell, 2003). Drip irrigation infrastructure allows for daily irrigation but can only be implemented if a shallow well is already installed. The application efficiency was set to 90% (Kenya Ministry of Environment, Water and Natural Resources and Kenyan Water Resources Management Authority, 2013). Results of the AquacropOS pre-runs showed that crop yields averaged to 0.5 (±0.25) t/ha under no adaptation measures; 0.6 (±0.25) t/ha under mulching or terraces; 0.8 t/ha using manual irrigation; and 1 t/ha using drip irrigation.
Simulating the Adaptive Behavior of Subsistence Farmers
In ADOPT, three different behavioral scenarios were explored: (1) business as usual (BAU); (2) economic rational behavior (following expected utility theory, EUT); and (3) bounded rational behavior [following the Protection Motivation theory, PMT (e.g., Grothmann and Patt, 2005; Dobbie, 2013; Dang et al., 2014a,b; Gebrehiwot and van der Veen, 2015; Stefanovi, 2015; Van Duinen et al., 2015a,b, 2016; Keshavarz and Karami, 2016; Zheng and Dallimer, 2016)] (see Table 1). the BAU and EUT scenarios reflected the common assumptions of no or full economic rational adaptive behavior in drought risk models, and helped to position the more complex, empirically observed bounded rational behavior (PMT).
Business as usual: no new adaptation
To represent the case of risk negligence, no additional adaptation decisions are made by households in the BAU scenario. This suggests that households do not perceive any change in risk or see no benefit in adaptation, and hence will act independently from it. Households have an initial level of drought adaptation (see Appendix A) which does not change over time. It was assumed that these farmers take loans to maintain the measures if needed. The use of this scenario helps position the dynamic-adaptation approach in drought risk assessments.
Economic rational behavior: expected utility theory
The EUT assumes that people seek to maximize their preferences for safety or risk, evaluating the value ascribed to the outcomes (“the utility”) of different adaptation actions and the probability that each will occur (Haer et al., 2016a; Schlüter et al., 2017). Applied to the Kenya case of drought management, rational households are fully self-interested, have full information about expected gains and losses, and always choose the adaptation option that gives the highest utility within their budget constraint. Households evaluate costs (e.g., possible yield loss and installation costs of drought adaptation measures) and benefits (e.g., reduction in possible yield loss) and their associated probabilities objectively, and attempt to maximize their expected utility given these costs and benefits (Shaw and Woodward, 2008). Social behavior, habits, and norms are ignored and suboptimal choices are not taken into account (Gigerenzer and Goldstein, 1996). It was also assumed that these farmers (as in BAU) cannot lose measures as they take loans to maintain them if required. The use of this scenario helps position the bounded rational adaptation approach in drought risk assessments.
Based on the AquacropOS pre-runs for all combinations of each of the four adaptation measures, the yield gains (B) calculated as the difference in losses between situations with- and without additional measures as well as the drought probability (p) were derived. Wealth (W) is an individual household variable tracked over time. Implementation and maintenance costs (C) of the adaptation options were obtained from experts in the fields (Appendix A). Assuming a slight risk adversity among the households, the general utility function applied in model is U (x) = ln x, which is a function with constant relative risk aversion. As is generally done in studies applying the EUT (e.g., Haer et al., 2016b), Every year, households adopt the adaptation measure with the highest expected utility (Equation 1), if its action utility proved higher than the utility of no action over a period of 10 years and if they can afford the initial implementation costs.
where
• p, the probability of a drought season—defined as a season with SPEI-3 value < −1.
• W, the wealth (total assets in USD) of the household.
• C, the cost of the adaptation measure in USD.
• B, the benefits (yield gain) in drought years (Bd) and non-drought years (Bn) in USD.
Bounded rational behavior: protection motivation theory
the EUT has been recognized as having limitations because of the assumptions of full information and the lack of social interactions (Van Duinen et al., 2012). Bounded rational behavior, influenced by social, economic, and psychological factors, can be included either by adding it to the utility maximization functions or by choosing alternative theories. the use of the PMT, which has been proven to be a valuable tool for understanding the adaptation decisions of individuals under drought risk, backed up by stakeholder surveys in lower-income countries (subsection Bounded rational behavior: protection motivation theory). This socio-cognitive model of bounded rational private adaptation integrates the effect of available resources and perceived climate risks into one framework for explaining the determinants of individual adaptation (Floyd et al., 2000; Grothmann and Patt, 2005). Indeed, the inclusion of socioeconomic and cognitive factors has been supported by a number of local case studies, which have found off-farm employment, group membership, labor availability, access to extension services, and farm experiences, to be the main drivers for the adoption of drought adaptation measures (e.g., Mutune et al., 2011; Jager and Janssen, 2012; Oremo, 2013; Mutua-Mutuku et al., 2017; Mutunga et al., 2017; Shikuku et al., 2017). Furthermore, a recent survey in Kitui confirmed that the factors included in PMT are indeed key determinants for the adaptive behavior in the face of agricultural drought risk (Wens, 2019).
PMT states that a person's intention to adapt is formed through the risk appraisal process, and coping appraisal process (Grothmann and Patt, 2005; Bubeck et al., 2012). While the PMT is a qualitative theory, in ADOPT we have formalized this theory, assigning a value between 0 and 1 to all factors of the theory, while allowing room for uncertainties in the form of varying weights for all the factors. In ADOPT, all the individual households form an intention to adapt (Equation 2), a certain adaptation measure (m), on an annual basis (t) as follows:
If a household has the financial capacity to pay for a considered measure (Stefanovi, 2015), the intention to adapt is translated into the likelihood the household will adopt this measure in the following years. Whether the household actually adopts the measure is stochastically determined for each household, each year, based on this likelihood. When households have adopted a measure, they will keep the measure. They are assumed to take a loan if they cannot pay then maintenance costs: not maintaining a measure is assumed to double the maintenance costs for the following year.
Although Stefanovi (2015), Van Duinen et al. (2015a), and Keshavarz and Karami (2016) have found positive relationships between the factors of PMT and observed protective behavior, a level of uncertainty exists related to the relative importance of risk appraisal and coping appraisal in the specific context of smallholder households' adaptation decisions in semi-arid Kenya. Therefore, the α and β parameters were introduced as weights for the two cognitive processes. To address the associated uncertainty, they were widely varied (α, β ϵ [0.334:0.666]) in a sensitivity analysis.
Risk appraisal (Equation 3), in our model-application a value between 0 (not aware of any risk) and 1 (frequently exposed to risk and lost all crop yield last year due to drought), is formed by combining the perceived risk probability and perceived risk severity, shaped by rational and emotional factors (Deressa et al., 2009, 2011; Van Duinen et al., 2015b). Whereas, risk perception is based in part on past experiences, several studies have suggested that households place greater emphasis on recent harmful events (Gbetibouo, 2009; Rao et al., 2011; Eiser et al., 2012). To include this cognitive bias, each household has a drought disaster memory, defined as follows (Viglione et al., 2014).
The drought occurrence in year t is a binary value with a value of 1 if the SPEI-3 value falls below −1. The disaster damage of a household is related to their harvest loss during the drought year, which is defined as the difference between their current and average harvest over the last 10 years.
Coping Appraisal (Equation 4, in our model-application a value between 0 (no appreciation of the adaptation options at all, no ability to pay for the measures) and 1 (full trust in own capacity, in the efficiency of the measures and easily able to pay for it) represents a households' subjective “ability to act to the costs of a drought adaptation measures, given the adaptation measures' efficiency in reducing risk” (Stefanovi, 2015; Van Duinen et al., 2015a). In ADOPT, coping appraisal is a combination of the households' perceived Self-Efficacy (financial, labor, and knowledge capacity of the farming households), adaptation efficacy (or response efficacy) of the measure, and its adaptation costs (or response cost):
Although Stefanovi (2015), Van Duinen et al. (2015b), and Keshavarz and Karami (2016) quantified the relationships between the factors driving the subjective coping appraisal of individuals, a level of uncertainty remains related to the relative importance of these drivers in the context of smallholder households' adaptation decisions in semi-arid Kenya. Therefore, weights (γ, δ, ε ϵ [0.25:0.50]) were introduced and varied in a sensitivity analysis using different ADOPT model runs.
The Adaptation Costs of the possible measures (see Appendix A) were expressed in terms of a percentage of the households' assets (value between 0 and 1, with a maximum of 1 as this reduces the intention to adapt to 0).
The Adaptation Efficacy (value between 0 and 1) of each measure was calculated as the percentage of yield gain, with a maximum of 100%. Because a lack of information is a significant barrier to the adoption of drought adaptation measures (Deressa et al., 2009; Ifejika, 2010; Below et al., 2012), this expected yield gain was estimated for two types of households: those that receive regular extension services (training in farm practices by the government or NGOs) and those that do not:
▪ For households that receive extension services (randomly assigned during model initialization), the expected yield gains were calculated as the change in annual average yield after the adoption of the drought adaptation measure, using estimates from pre-runs of AquacropOS. Therefore, this assumed prior, unbiased knowledge about the efficacy of adaptation measures.
▪ Households without access to extension services had to rely on their neighbors to obtain information on adaptation efficacy, and their expected yield gain was estimated as the difference between the yields of neighboring households that had already adopted a specific measure and the households' own current yield. These households thus have a biased adaptation efficacy.
A meta-analysis of factors motivating climate change adaptive behavior found that perceived self-efficacy was strongly associated with adaptation decisions (Van Valkengoed and Steg, 2019). In this research, we assumed that younger household heads, household heads with a higher education (human capacity), larger households (labor), and female household heads have a higher self-efficacy (value between 0 and 1) and thus are more likely to adopt the adaptation measures (Oremo, 2013; Charles et al., 2014; Tongruksawattana, 2014; Muriu et al., 2017).
Model Sensitivity
ADOPT was run 50 times per adaptive behavioral scenario in a Monte-Carlo simulation to average the effect of the initialization of household characteristics (household size, farm-size, age, education, off-farm income, and expenditures). To support generalizability and simplicity, these household characteristics were stochastically determined at the start of each run, assuming a normal distribution, with the averages and standard deviations of household characteristics reported in the survey datasets (Wens, 2019). Moreover, the bounded rational scenario in ADOPT was run 48 × 50 times, with 6 × 8 differentiating combinations of weights for the Risk Appraisal and Coping Appraisal factors (α, β ϵ [0.334:0.666], sum always equals 1) and the Self-Efficacy, Adaptation Efficacy, and Adaptation Costs factors (γ, δ, ε ϵ [0.25:0.50], sum always equals 1). Since it was not possible to calibrate the weights of all PMT factors (this would require more surveys over a long period of time), this sensitivity assessment where each factor was halved or doubled in importance, was conducted to explore the possibility space when accounting for bounded rational behavior.
Besides, it was also investigated what the effect of the share of households receiving extension services on the outcome would be. This is done because the survey results (60%) and the literature (often estimated around 30%, e.g., TEGEMEO datasets) strongly disagreed on the amount of households who have a correct idea about the costs and benefits, and do not rely on their neighbors to show how measures should be implemented).
Results
In this section, the results of the ADOPT model runs are presented to explore the difference in adoption rate of drought adaptation measures under BAU, economic rational behavior (EUT), and bounded rational (PMT) behavior (section Adoption of Drought Adaptation Measures). Moreover, we investigate the difference in maize harvest and financial assets of the households in the three scenarios (section Maize Harvest and Financial Assets), and the consequences of this on the evolution of drought risk over time in the form of poverty rates, household food insecurity, and food aid needs (section Drought Risk).
Adoption of Drought Adaptation Measures
Using the three behavioral theories, ADOPT simulated the intention to adopt and the resulting adoption rate of adaptation measures over time. Based on a pre-run in Aquacrop, it is clear that a combination of all four measures is the most effective way to reduce negative impacts of droughts on crop yield, while a combination of a well with irrigation is the most economic efficient solution. Figure 3 shows the adoption rate of the four different drought adaptation measures by the modeled households. Applying mulch to the fields was a cheap and economic efficient adaptation option, and therefore its adoption in the economic rational scenario reaches 81% after 5 years (Figure 3, upper left panel). In contrast, a gradual adoption was found in the bounded rational simulation, reaching 34% (11–49%) adoption by the end of the simulation period. The adoption in this scenario is influenced by the households' risk perception as can be seen in the steep increase in the application of mulch during and after the 1999–2000 drought. Fanya Juu terraces, an indigenous technique already applied by 25% of the farmers at the start of the simulation, were very popular (Figure 3, upper right panel). An immediate adoption of 85% was seen in the economic rational simulation, and a gradual adoption up to 43% (35–53%) after 30 years was seen in the bounded rational simulation. The installation of a shallow well is economically efficient but expensive, which led to less overall and more gradual adoption in the economic rational and bounded rational scenarios. Households prefer to install a well and then install drip irrigation (Figure 3, bottom panels): first they apply manual irrigation, and after saving money they are able to buy drip irrigation infrastructure. Overall, 44 and 10% (3–20%) of the economic rational and bounded rational households were able to install a well, which could drastically increase their crop yields. In general, the households with a larger farm were the ones able to adopt this technique. Furthermore, 43% of all economic rational households and 5% (1–11%) of the bounded rational households were able to then further reduce their drought vulnerability by adopting drip irrigation by 2010.
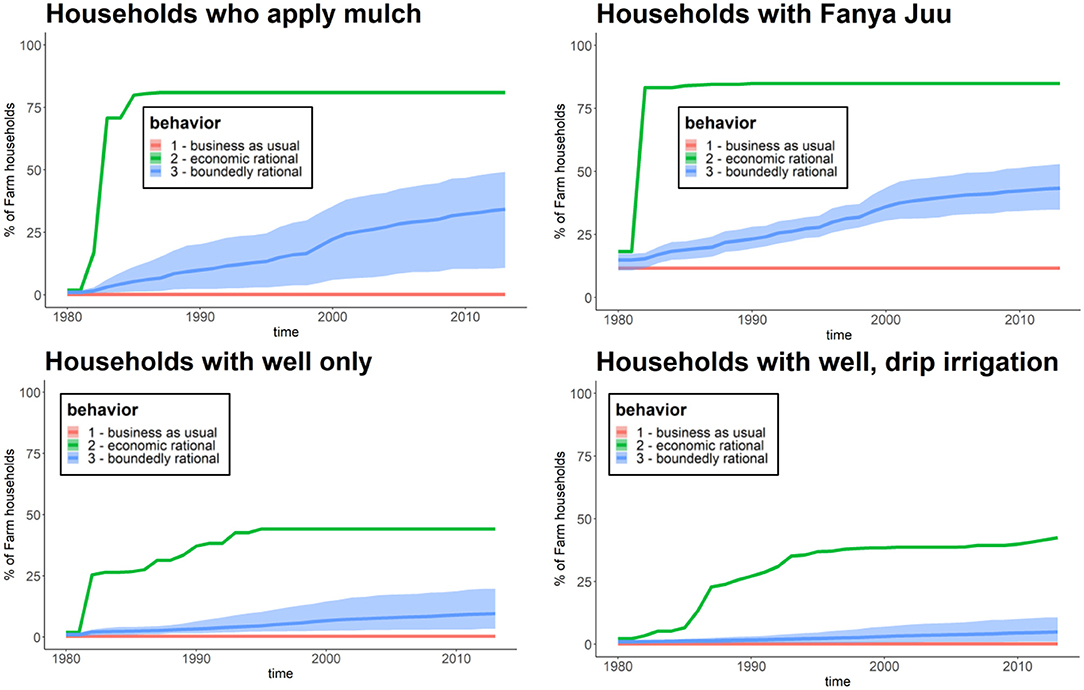
Figure 3. Adoption of different drought adaptation measures over time, for three behavioral scenarios—economic rational (EUT, green), bounded rational (PMT, blue), and business as usual/no adaptation (red)—and for four different drought adaptation measures (fanya juu terraces, mulching, manual irrigation, and drip irrigation). Shaded blue areas show the variance across 48 bounded rational model runs, a result of the variety in weights of the PMT factors.
In the bounded rational scenario, adoption occurred gradually since households that do not receive extension services cannot adopt measures they do not see in use in their neighbors' fields. Bounded rational farmers also adopted less economically efficient measures as their limited information reduced their ability to calculate the costs and benefits of all adaptation options. Given the observed adoption rates (Wens, 2019) of fanya juu terraces (45%), mulching (12%), well construction (16%), and drip irrigation techniques (6%), we find that the economic rational scenario largely overestimated the adaptation of all measures. Besides, in this scenario, we see that after 30 years, 42.5% of the households adopted one measure, 19.5% adopted two, 3% households adopted three and only 0.5% households adopted all four measures. From the survey, it is reported that 42.3% of the respondents adopted one measure, 12.3% adopted two, 1.5% adopted three and 0.7% adopted all four measures. The estimated adoption rates using bounded rational scenario thus best reflected the observations, except in the case of mulch application. The inclusion of PMT behavior is thus better able to capture some of the variability in adoption decisions, but is nevertheless still not a complete explanation of the observed adaptive behavior of households in semi-arid Kenya.
Maize Harvest and Financial Assets
Figure 4 presents the ADOPT average maize harvest results (in kg, based on two growing seasons) for the three behavioral scenarios. These maize harvests were affected by drought events (orange bars) and the adoption of drought adaptation measures over time. The results showed that the historical drought disasters registered in EMDAT (1984, 1991/1992, 1994, 1999/2000, and 2008/2009) were also apparent in our modeled harvest, boosting confidence in the capacity of ADOPT to simulate maize harvest variability. The highest harvest numbers were achieved by economic rational households, which proactively invest in the most economically efficient adaptation measures. Economic rational drought management leads to a more efficient adaptation strategy, and thus lower vulnerability to water shortage and higher average maize production compared with bounded rational drought management. When comparing the bounded rational and BAU scenarios, the bounded rational households yielded greater harvests over time. They adopted adaptation measures mostly after experiencing droughts, thereby gradually moving away from the BAU scenario.
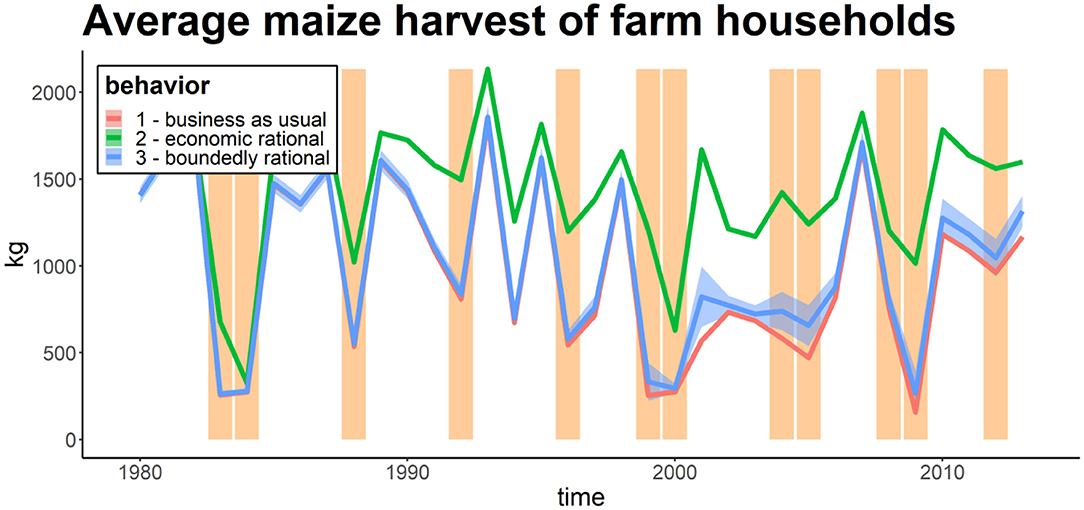
Figure 4. Average annual maize harvest of the modeled households in kg/year for three behavioral scenarios: no adaptation decisions (BAU), economic rational (EUT), and bounded rational (PMT). Drought years (SPEI3 value in crop season < −1) are visualized as vertical orange bars. The shaded blue area show the variance among 48 bounded rational model runs, a result of the variety in weights of the PMT factors.
The average harvest varied by ~1,467 kg under economic rational conditions, 992 kg (935–1,062 kg) under bounded rational conditions, and 919 kg under BAU conditions. The average crop yield was 614 kg/ha (591–644 kg/ha) under the bounded rational scenario, whereas it elevated to 805 kg/ha when assuming economic rational households and decreased to 583 kg/ha when assuming BAU households. The bounded rational simulation numbers were close to the observed values in Kitui of 680 kg/ha (605 kg/ha with outliers (biased answers of the survey) removed) (Wens, 2019).
The adoption of drought adaptation measures and resulting yield gains has a prominent effect on the household's wealth over time (Figure 5). Although adaptation initially required a large investment, thereby reducing financial assets, it is economically efficient in the long turn. This is most clear in the economic rational scenario, where a high initial adoption happens in 1981, and a return on investment after ~12 years, resulting in much wealthier households compared with the business-as-usual rational scenario. The more gradual adoption in the bounded rational scenario only results in an initial decrease, then a slight increase in average wealth, as compared to the BAU scenario. Besides, also droughts have a pronounced effect on a household's wealth over time, as illustrated by the sharp decline in wealth during and following the consecutive droughts of 1983–1984, 1999–2000, and 2004–2005. The increased frequency of severe droughts, starting with the millennium drought, had a distinct effect on the households in the bounded rational and BAU scenarios. The economic rational scenario households proved more resilient to these shocks, as majority of the farmers are able to adopt irrigation infrastructure resulting in an almost constant increase in wealth over time.
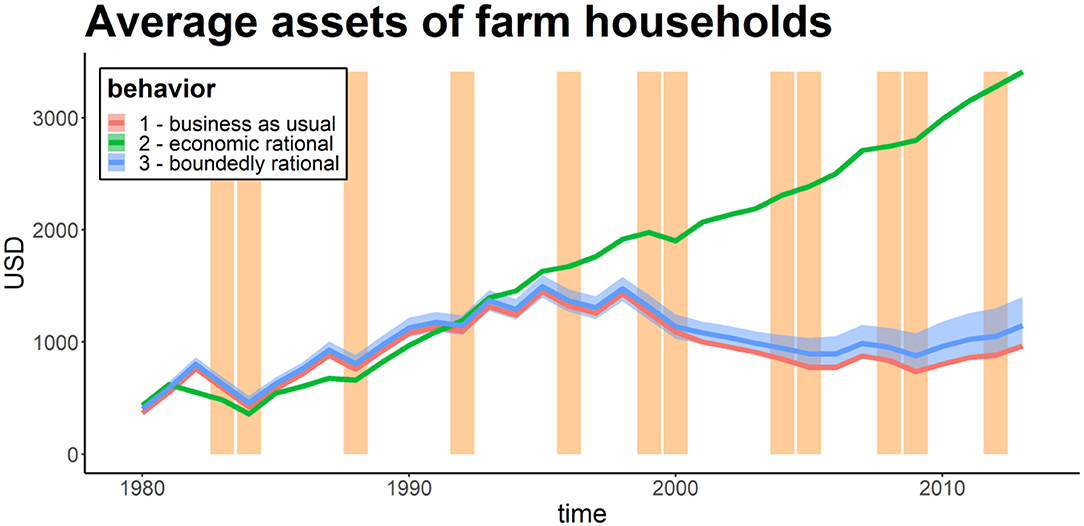
Figure 5. Households' financial assets (in US$/year) over time. Visualized is the median assets stock of all households modeled for three scenarios: no adaptation decisions (BAU), economic rational (EUT), and bounded rational (PMT) adaptive behavior. Drought years (SPEI value in crop season < −1) are visualized as vertical orange bars. The shaded blue area shows the variance among 48 bounded rational model runs, a result of the variety in weights of the PMT factors.
Drought Risk
As expected, droughts have a negative effect on household food security (Ifejika et al., 2008; Erenstein et al., 2011a), whereas drought adaptation measures have a neutralizing effect. Figure 6 shows that the peaks in food insecurity in the economic rational scenario were significantly lower than those of the BAU scenario, the food insecurity rate in the bounded rational scenario slightly reduced over time compared with BAU. This highlights that economic rational households are less food insecure compared with bounded rational households because of their relatively large uptake of adaptation measures before a drought event. However, the resilience to droughts of bounded rational households was higher compared with the BAU scenario; that is, they were less food insecure during and after drought years. On average, 44, 39 (36–43%), and 30% of the households lived in food insecurity in the BAU, bounded, and economic rational behavior scenarios, respectively. Food insecurity rates in the bounded rational scenario aligned with (Ulrich et al., 2012) who reported a food insecurity rate of 15% in normal years (on average 17% in the bounded rational scenario)—with a sharp outlier to 91% in the 1999–2000 drought and subsequent years (87% in the bounded rational scenario).
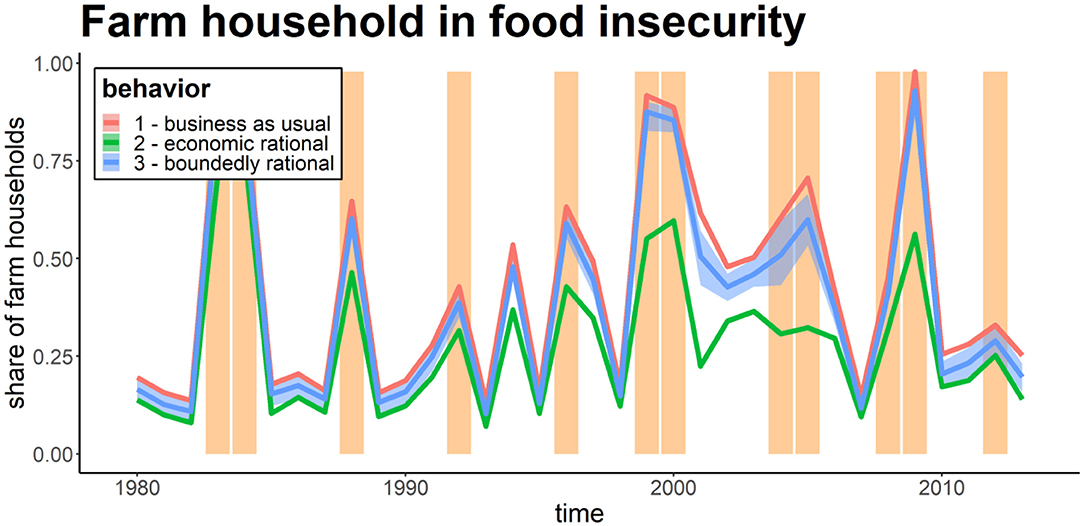
Figure 6. Share of households in food insecurity over time. Variability over time visualized for three behavioral scenarios: economic rational (EUT), bounded rational (PMT), and no adaptation decisions (BAU). Drought years (SPEI value in crop season < −1) are visualized as vertical orange bars. The shaded blue area shows the variance among 50 bounded rational model runs, a result of the variety in weights of the PMT factors.
The ADOPT results presented in Figure 7 show averages of 35, 45 (41–49%), and 49% of the households in poverty under the economic rational, bounded rational, and BAU scenarios, respectively. These estimates were similar to the 46% reported in rural areas by the (Kitui County, 2013). Drought shocks have a profound effect on the poverty level, as can be clearly seen during the recent drought of 2008–2009, when 80% of all households fell into poverty conditions after being hit. The effect of droughts on poverty was also observed by (Few et al., 2006; Mwongera et al., 2013). Notably, our results exhibited an overall increase in poverty while the average financial assets increased through time. The standard deviation of households' maize harvest and financial assets widened over time, which could be a sign that inequality in wealth increased.
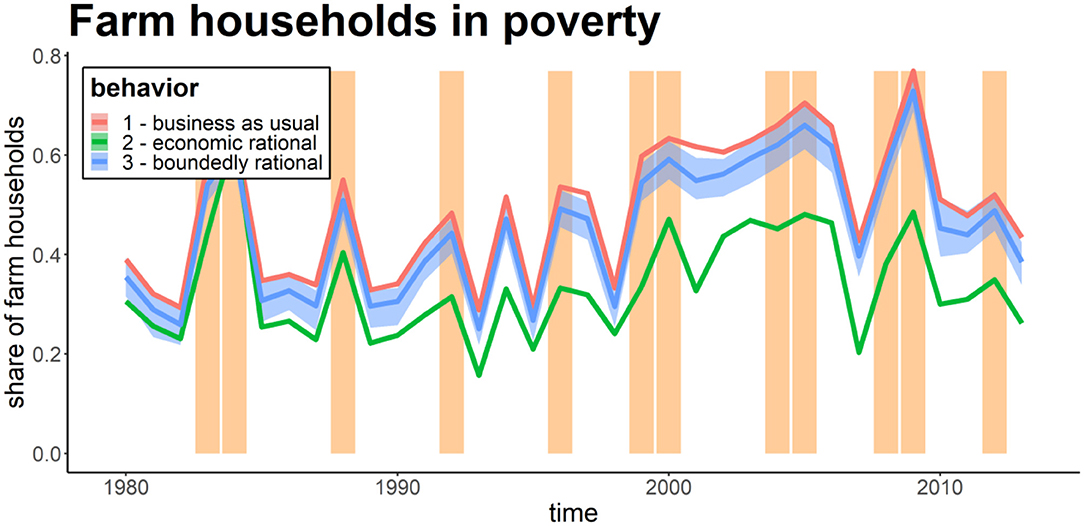
Figure 7. Share of households in poverty. Variability over time is visualized for three behavioral scenarios: economic rational (EUT), bounded rational (PMT), and no adaptation decisions (BAU). Drought years (SPEI value in crop season < −1) are visualized as vertical orange bars. The shaded blue area shows the variance among 50 bounded rational model runs, a result of the variety in weights of the PMT factors.
Tracking individual households over time, ADOPT showed that it was predominantly the rich households that were able to optimize their drought management, produce more, become richer over time, and thus be able to adopt even more drought adaptation measures. By contrast, less wealthy households did not succeed in adopting sufficient drought adaptation measures, suffered more from large drought impacts, and thus stayed poor. This is clear from Figure 7 where, although gradual adoption happens, poverty levels do not decrease over time: This can be attributed to the “poverty trap effect” (Muyanga, 2004; Mango et al., 2009) as identified by (Ifejika et al., 2008; Ulrich et al., 2012). This is also evident when evaluating the average assets of households with zero, one, two, three or four measures, equaling on average US$600, US$850, US$2600, US$4,800, and US$5,400 by the end of the running period, and while evaluating households' start income, start assets and end assets (Table 2). There appears to be a significant difference (one,-sided t-test, alpha = 0.05) between the start income of all groups and between the end assets of all groups of Table 1. This shows that existing inequalities between households are exacerbated over time as a result of a path-dependent Matthew effect. The ability to adopt measures at an early stage reduces the vulnerability to droughts and thereby increases financial capacity to further increase resilience. Conversely, a lack of capacity to adapt translates to a progressively diminishing lack of capacity, a poverty trap.
Figure 8 depicts the amount of food aid (US$) required to ensure food security per 1,000 households as modeled through ADOPT. Food aid was calculated as the sum of US$ required for all households to fulfill their food requirements, accounting for a variable food price. Thus, ADOPT can be used to calculate the average annual costs of droughts (i.e., the direct economic loss for a government assuming it provides full food aid to all households in need; see Table 3). In the BAU, bounded rational, and economic rational scenarios, the annual average losses caused by droughts (costs to governments) equaled US$ 71.1k, 48.0k, and 19.9k per 1,000 households, respectively, on an annual basis. These estimates are a result of food supply shortages in the study area caused by droughts as well as the limited possibilities for households to buy food because of extreme poverty. Differences were significant between the three scenarios, as the bounded rational scenario estimated needs 32.5% lower and the economic rational scenario estimated needs 72.0% lower than the business as usual scenario where no additional adaptation happened. There is a clear decrease in drought vulnerability in the economic rational scenario, with emergency needs reduced to almost zero. The high total production, due to the large amount of households which adopted irrigation techniques, results in ample food supply even in times of drought. This is not the case in the BAU and bounded rational scenario, where the regional food supply is insufficient during drought years.
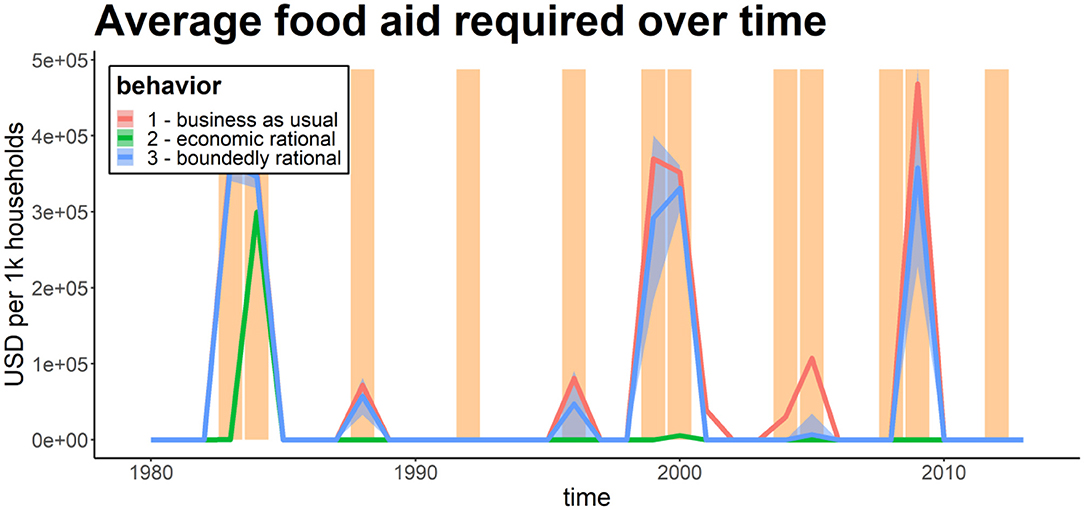
Figure 8. Food aid required per thousand households. Food aid was calculated as the cumulative amount of food shortage of all individual households multiplied by the maize price, accounting for a variable food price. Variability over time is visualized for three behavioral scenarios: economic rational (EUT), bounded rational (PMT), and no adaptation decisions (BAU). Drought years (SPEI value in crop season < −1) are visualized as vertical orange bars. The shaded blue area shows the variance among 50 bounded rational model runs, a result of the variety in weights of the PMT factors.
Furthermore, Table 3 shows the ADOPT estimation of the average annual cost of droughts for a government (US$ per thousand households) for providing all the food needs for households in food insecurity in case of a heterogeneous and homogeneous set of households. When the total harvest in the study area was assumed to be equally distributed among all households and all households could afford to buy their food need equivalents, average annual food aid needs over this period would be US$ 34.7k, 27.6k, and 7.7k USD for the BAU, bounded rational decision, and economic rational decision scenarios, respectively. This method of calculating aid needs, through excluding the agent-based approach, yielded underestimations of food aid needs by 51% as compared to the respective heterogeneous, multi-agent-based estimations. This showcases the added value of evaluating risks and the needs for food aid on an individual household basis.
Moreover, comparing food aid numbers for the severe droughts of 1984, 2000, and 2009, the influence of the different adaptive behaviors on the drought disaster impact can be observed. In the '83–'84 drought, a food aid peak of US$ 745k could be observed in the bounded rational scenario, whereas BAU assumptions resulted in an estimate of US$ 758k and the economic rational scenario yielded an estimate of only US$ 256k. This is 66% less than the BAU scenario, which means the sudden adoption of drought adaptation measures in the economic rational scenario is immediately effective in disaster risk reduction. During the 1999–2001 drought, the BAU, bounded rational, and economic rational scenarios exhibited emergency aid needs of US$ 820k, US$ 428k, and US$ 0, respectively, and the 2009 drought ends up with emergency aid needs of US$ 490k, US$ 205k, and US$ 0 in the BAU, bounded rational and economic rational scenarios, respectively. For the bounded rational scenario, this translates to a reduction in the disaster impact of 47.8% in 2000 and 58.1% in 2009, as compared to the BAU conditions. For the economic rational scenario, this translates to a full reduction in the disaster impact, resilience is achieved. These vast differences reveal the large influence of adaptation, and the assumptions about the dynamics of it, on drought risk assessments.
Discussion
In this contribution, we present a socio-hydrological, agent-based model, ADOPT, which integrates the crop model AquacropOS with a behavioral model to apply different decision theories. The ADOPT model framework is capable to be calibrated for any drought-prone area dominated by smallholder farmers. Here, ADOPT is deployed to model subsistence farmers in Kitui, Kenya. Our model highlights the importance of accounting for the behavior of individuals and households who, through adaptive decision-making, influence the drought risk they are subjected to. A failure to account for behavior in drought risk models leads to an underestimation of required food aid. While results should be interpreted with care given the assumptions and simplifications made, the use of various behavioral models show the range of possible behavioral effects as they emerge from an integrated social-hydrological feedback. The ADOPT model is thus useful as a first step in integrating adaptive behavior in drought risk modeling, while more work is required to parameterize the complex behavioral rules needed to accurately predict future drought risk scenarios. The following paragraphs discuss the differences between the applied model scenarios, model calibration, and validation and sensitivity analysis.
Model Scenarios
Insights into the co-evolution of human adaptation and drought risk are vital to the assessment of future drought risk and the development of any disaster risk reduction strategy. However, the assumptions about adaptive behavior, implemented through the use of different decision theories, highly influence the estimations of future risk (De Koning, 2019). Through comparing complex behavioral dynamics against a BAU and an economic rational scenario, we were able to show the relative influence of empirical adaptive behavior (and its uncertainty) on general food security and poverty indicators in a Kenya-based case study.
While economically rational farmers implement affordable adaptation measures at a fast rate, thereby increasing their maize yield, the adoption of drought adaptation measures occurs more gradually under bounded rational conditions. This slower uptake is influenced by the occurrence of droughts, which have a negative effect on the financial assets of households, thus reducing their coping appraisal. However, the frequency of droughts in the study area keeps their risk appraisal rather high. The intention to adopt for bounded rational farmers is therefore almost never zero, which is also confirmed by empirical evidence (Wens et al., 2019). The bounded rational scenario leads to more realistic estimations of the adoption of drought adaptation measures, except for the estimations on mulching. This labor-intensive technique, which also limits the feeding of unharvested crop residue to livestock, is potentially undervalued in the model.
Furthermore, the ADOPT results clearly shows the ability to simulate the dynamics of a poverty trap: smallholder farmers can be impoverished due to drought shocks, disabling them from adopting drought adaptation measures, and consecutive droughts can be a reason for remaining poor and increasing food insecurity. The adoption of drought adaptation measures by bounded rational households reduced drought-related economic loss by 30% compared with the BAU scenario; economic rational households exhibited a reduction of 78% compared with BAU. From this estimation, it is evident that the choice of adaptation theory matters when estimating risk.
Model Validation
The validation of a complex behavioral model is challenging because of unique feedbacks and a lack of empirical data (Claessens et al., 2012; Brown et al., 2017). Hence, this study must be seen as a sensitivity analysis for assessing the influence of behavioral dynamics on drought risk rather than an attempt to reproduce the correct absolute estimates on yield and risk. However, to derive realistic results, we followed some of the recommendations described by Cirillo and Gallegati (2012) to establish the model, and used observed data to initialize and calibrate ADOPT. For example, (1) we used reanalysis climate data from CHIRPS (Funk et al., 2015a); (2) AquacropOS is specifically designed for semi-arid areas, such as Eastern Africa (Vanuytrecht et al., 2014) and was calibrated to the specific geographical characteristics of Kenya (based on Ngetich et al., 2012); and (3) we undertook a survey in the region (Wens, 2019; n = 250) to obtain the empirical socioeconomic parameters to initialize ADOPT. While this data was complemented with existing household survey data from 2000, 2004, 2007, 2010 (Tegemeo Institute, 2000, 2004, 2007, 2010), it remains a limitation to represent 30 years of model dynamics by data from a few snapshots in time
The calibration of Aquacrop was done based on research done by other authors, who calibrated Aquacrop for this region. A dedicated experiment of crop yield under different weather, soil and management circumstances would have decreased this uncertainty, but this was beyond the scope of this research. However, A validation of ADOPT model results against historical average maize yields showed that simulated yields of 0.6 t/ha (±0.25) matched the peaks and averages for the Kitui region of 0.6 t/ha (±0.4) (e.g., Hansen and Indeje, 2004; Barron and Okwach, 2005; Aylward et al., 2015; Mumo et al., 2018; Ayugi et al., 2020). Furthermore, the simulated range of people in food insecurity and people in poverty fitted the observed ranges and variance over time: Ifejika et al. (2008) reported that 91% of rural households experienced food insecurity during the 1999–2000 drought, which is similar to the modeled peak using ADOPT. Peaks in poverty in 2000, around 2004, and 2009–2010 were also observed by (Nyariki and Wiggins, 1997; Johnson and Wambile, 2011; Oluoko-Odingo, 2011).
Sensitivity Analysis
A sensitivity analysis was performed to evaluate the effect of assumptions in PMT on the uptake of adaptive measures and related effects on yields. Sensitivities were visualized by shaded uncertainty ranges in the graphs found in section Results. Changing the initialization values of the households did not significantly affect the average maize harvest nor average aid needed over time. When varying the share of households receiving extension services between 10 and 90%, estimates on average household maize harvest varied between 971 and 1,000 kg per year, respectively. When varying the PMT weights α and β, changing the relative importance of risk appraisal and coping appraisal (subsection 2.2.3, Adaptation Intention Equation 2), between 66 and 33%, the resulting household maize harvest estimates ranged between 993 and 987 kg per year, respectively. Varying the weights in the coping appraisal equation, changing the relative importance of self-efficacy, adaptation-efficacy and adaptation costs (γ, δ, and ε, subsection Simulating the Adaptive Behavior of Subsistence Farmers, Equation 4) between 25 and 50%, household maize harvest estimates ranged between 982 kg (when adaptation costs have a weight of 50%) and 1,003 kg (when adaptation efficacy has a weight of 50%).
The estimated average food aid needs over the 30 years of simulations varied between US$ 61.544–53.584 when changing the share of households receiving extension services between 10 and 90%; between US$ 57.152 and 57.391 when changing the relative influence of risk perception from 66 to 33% (Equation 2); and between US$ 54.360 and 58.955 when altering the weights of self-efficacy, adaptation efficacy, and adaptation costs (Equation 4). It is clear that the weights used to estimate the intention to adopt did influence the final outcome: Increasing the influence risk perception or giving more importance to adaptation efficacy increased the average maize harvest, while it decreased the average aid needed. assumptions about an external factor—the extension services—are shown to have a larger impact on the results: raising the share of households receiving this form of training increased the average maize harvest and reduced the aid needed more than any of the changes in weight of the PMT factors. This indicates that increasing the frequency of extension services in addition to raising households' risk perception or thrust in the adaptive efficacy, can positively influence the adoption rate hence decrease drought risk. However, the variability introduced by the different behavioral scenarios, and thus the assumptions of BAU (static vulnerability) and economic rational behavior (full information), are highlighted as the largest influencing factors on the results, proving the importance of correctly including human adaptive behavior in drought risk models.
Scope for Further Research
Not all possible adaptive behaviors could be included in our modeling setup. For example, Kenyan households often cope with droughts by increasing livestock sales, serving as a buffer against absolute poverty in times of failed harvests (Few et al., 2006; Ifejika, 2010; Oluoko-Odingo, 2011). In ADOPT, livestock trade is not included, explaining the relatively high peaks in poverty during droughts. Furthermore, the potential application of different drought tolerant seeds and/or varieties can be included, which would require detailed information about the varieties agronomic sensitivity to water stress. Another way to reduce modeling uncertainty in future applications is including a changing planting date based on weather predictions, as is reported on the field (Wens, 2019) but currently impossible in AquacropOS. Future research could work to include these drought adaptation strategies to create a broader picture of smallholder adaptive behavior in the face of droughts in semi-arid Kenya. Besides, while now only precipitation was seen as a source of water, a follow up study could couple the ADOPT model to a spatially distributed hydrological model and investigate the influence of water abstractions on the water availability in rivers or groundwater. As such, proximity to the river and other geographic drivers for adaptation can be included.
Concluding Remarks
Smallholder farms in Africa are increasingly affected by droughts, which are expected to intensify with climate change and socioeconomic trends. However, while these farmers have a critical role regarding efficient use of water and the production of food (e.g., UN Water Action decade 2018–2028, UN Decade of Family Farming 2019–2029), their individual decision-making is often neglected in drought risk assessments (Moran et al., 2007). Disentangling the role of emergent adaptation decisions improves the understanding of current and future drought risk (Aerts et al., 2018; Wens et al., 2019). Aiming to address this modeling gap, we developed a socio-hydrological, agent-based drought risk model, ADOPT, which couples a physically based crop growth model (AquacropOS) with an adaptation decision model. Designed using socio-hydrological and agent-based modeling approaches, ADOPT simulates the two-way interaction between rain-fed agricultural production variability and the emergence of drought adaptation measures. Initialized with new survey data from households in central Kitui, the model showcased its ability to analyze historical yield losses caused by droughts, the impact of these losses on smallholder farmers, and the adaptive response of farmers to droughts. Three different behavioral scenarios were tested: one where households could not adopt new adaptation measures (business as usual), one where they behaved economically rationally (following the expected utility theory), and a more realistic approach where they followed a bounded rational logic (using the protection motivation theory).
Results highlight that current estimations of drought risk and the need for emergency food aid can be improved using an agent-based approach. Besides, we show that working with an “average household”—thus not accounting for the existing inequality—underestimates the number of aid needs. Furthermore, our study finds that assumptions about the adaptive behavior of households highly influence drought risk estimations. ADOPT simulations show how the dynamics of adaptive decision-making, which emerge from interactions between individual agents and their environments, influence yields and other risk indicators. Accounting for bounded rational decision-making, a significant difference in annual average yield loss could be seen in comparison to the more conventional BAU or economic rational scenarios. Moreover, the magnitudes estimated while assuming bounded rational households were found to be closest to observed data. By incorporating the effects of bounded rational adaptive behavior, we conclude that ADOPT is better able to better simulate drought risk over time than classic assumptions with respect to adaptation behavior.
Following recent research in socio-hydrology, the ADOPT model can be seen as an experimental setup for improving our understanding of the socioeconomic and environmental factors that influence drought risk and management over time. While now, it shows how different drivers steer household's adaptation decisions and how these affects their personal and community drought risk, it could also be used to assess future drought risk and to evaluate the effect(iveness) of specific NGO or governmental actions and policy. The ADOPT framework can be applied to study food insecurity in other case studies, or used to answer questions about other adaptation strategies, aiming at improved livelihoods and reduced drought risk. Besides, it can be employed to study the effect of certain governmental policies on households' drought risk behavior or, coupled to a spatially distributed hydrological model, it can enable us to study trade-offs between drought risk for different water users up- and downstream catchments. This work represents an important next step in drought risk modeling and toward enhanced agricultural water management.
Data Availability Statement
The household survey dataset analyzed for this study can be found online in the mission report by Wens et al. (2019).
Author Contributions
MW did the survey that provided input information for the model, developed the ADOPT, and took lead in writing the manuscript. MM and RL supported MW on the field in Kenya and provided knowledge of Kenyan drought adaptation context. JJ and TH assisted in the agent-based modeling procedure and translating adaptive behavioral theories into model coding language. TV and JA contributed to the creative process and setup of the research, and editing of the paper. All authors contributed to the article and approved the submitted version.
Conflict of Interest
The authors declare that the research was conducted in the absence of any commercial or financial relationships that could be construed as a potential conflict of interest.
Acknowledgments
We gratefully acknowledge funding from the Netherlands Organization for Scientific Research (NWO) VICI research project number 453-582 13-006. The authors are grateful to Mr. Mutinda from SASOL foundation for his insights on the drought adaptation measures in Kitui and to Dr. Snelders (Center for International Cooperation, VU Amsterdam) for sharing the contacts acquired during the long collaboration between the VU in the Netherlands and SEKU in Kenya. The authors also want to thank Nick, Stella, Bobsammy, Nicholas, Emmanuel for their contribution to the data collection. Lastly, the authors acknowledge the constructive feedback and interesting comments of the three reviewers, which helped improving this manuscript significantly.
Supplementary Material
The Supplementary Material for this article can be found online at: https://www.frontiersin.org/articles/10.3389/frwa.2020.00015/full#supplementary-material
References
Adger, W. N., Brown, I., and Surminsk, S. (2018). Advances in risk assessment for climate change adaptation policy subject areas : Phil. Trans. R. Soc. A 376:201:1–13. doi: 10.1098/rsta.2018.0106
Aerts, J. C. J. H., Botzen, W. J., Clarke, K. C., Cutter, S. L., Hall, J. W., Merz, B., et al. (2018). Integrating human behaviour dynamics into flood disaster risk assessment. Nat. Clim. Change 8, 193–199. doi: 10.1038/s41558-018-0085-1
Alessandro, S. D., Caballero, J., Simpkin, S., and Lichte, J. (2015). “Agriculture global practice technical assistance paper,” in Kenya Agricultural Risk Assessment (Washington, DC: World Bank Group).
Allen, R. (2004). “Penman-Monteith equation,” in Encyclopedia of Soils in the Environment, ed D. Hillel (Encyclopedia of Soils in the Environment, Elsevier), 180–188. doi: 10.1016/B0-12-348530-4/00399-4
Asgary, A., and Levy, J. (2009). A review of the implications of prospect theory for natural hazards and disaster planning. Int. J. Environ. Res. 3, 379–394.
Aylward, C., Biscaye, P., Harris, K. P., Lafayette, M., True, Z., Anderson, C. L., et al. (2015). Maize Yield Trends and Agricultural Policy in East Africa Macro-Level Literature Review for Six Countries. EPAR Technical Report No. 310. Washington, DC: Evans School Policy Analysis and Research (EPAR); University of Washington.
Ayugi, B., Tan, G., Niu, R., Dong, Z., Ojara, M., Mumo, L., et al. (2020). Evaluation of meteorological drought and flood scenarios over Kenya, East Africa. Atmosphere 11:307. doi: 10.20944/preprints201912.0343.v1
Baldassarre, G. D., Viglione, A., Carr, G., Kuil, L., Yan, K., Brandimarte, L., et al. (2015). Debates—perspectives on socio-hydrology: capturing feedbacks between physical and social processes. Water Resour. Res. 51, 4770–4781. doi: 10.1002/2014WR016416
Barreteau, O., Bousquet, F., Millier, C., and Weber, J. (2004). Suitability of multi-agent simulations to study irrigated system viability: application to case studies in the senegal river valley. Agric. Syst. 80, 255–275. doi: 10.1016/j.agsy.2003.07.005
Barron, J., and Okwach, G. (2005). Run-off water harvesting for dry spell mitigation in maize (zea mays l.): results from on-farm research in semi-arid Kenya Agric. Water Manage. 74, 1–21. doi: 10.1016/j.agwat.2004.11.002
Barron, J., Rockström, J., Gichuki, F., and Hatibu, N. (2003). Dry spell analysis and maize yields for two semi-arid locations in East Africa. Agric. For. Meteorol. 117, 23–37. doi: 10.1016/S0168-1923(03)00037-6
Below, T. B., Mutabazi, K. D., Kirschke, D., Franke, C., Sieber, S., Siebert, R., et al. (2012). Can farmers' adaptation to climate change be explained by socio-economic household-level variables?. Glob. Environ. Change 22, 223–235. doi: 10.1016/j.gloenvcha.2011.11.012
Biamah, E. K., Gichuki, F. N., and Kaumbutho, P. G. (1993). Tillage methods and soil and water conservation in eastern Africa. Soil Till. Res. 27, 105–123. doi: 10.1016/0167-1987(93)90064-V
Birkmann, J., Cardona, O. D., Carreño, M. L., Barbat, A. H., Pelling, M., Schneiderbauer, S., et al. (2013). Framing vulnerability, risk and societal responses: the move framework. Nat. Hazards 67, 193–211. doi: 10.1007/s11069-013-0558-5
Black, J., Oduor, A., Cheregony, K., and Nyabenge, M. (2012). Rainwater Harvesting Inventory of Kenya: An Overview of Techniques, Sustainability Factors and Stakeholders. Nairobi: ICRAF.
Blair, P., and Buytaert, W. (2016). A System Dynamics Model of Human-Water Interaction in Anthropogenic Droughts. Geophysical Research Abstract Vol 18.
Bouma, J. A., Hegde, S. S., and Lasage, R. (2016). Assessing the returns to water harvesting: a meta-analysis. Agric. Water Manage. 163, 100–109. doi: 10.1016/j.agwat.2015.08.012
Brooks, N., Adger, W. N., and Kelly, P. M. (2005). The determinants of vulnerability and adaptive capacity at the national level and the implications for adaptation. Glob. Environ. Change 15, 151–63. doi: 10.1016/j.gloenvcha.2004.12.006
Brooks, S., Thompson, J., Odame, H., Kibaara, B., Nderitu, S., Karin, F., et al. (2009). Environmental Change and Maize Innovation in Kenya: Exploring Pathways in and out of Maize. STEPS Working Paper. Brighton. Available online at: http://www.ids.ac.uk/files/dmfile/STEPSWorkingPaper36.pdf
Brown, C., Alexander, P., Holzhauer, S., and Rounsevell, M. D. A. (2017). Behavioral models of climate change adaptation and mitigation in land-based sectors. Wiley Interdiscipl. Rev. Clim. Change 8:e448. doi: 10.1002/wcc.448
Bubeck, P., Botzen, W. J. W., and Aerts, J. C. J. H. (2012). A review of risk perceptions and other factors that influence flood mitigation behavior. Risk Anal. 32, 1481–1495. doi: 10.1111/j.1539-6924.2011.01783.x
Bunclark, L., Gowing, J., Oughton, E., Ouattara, K., Ouoba, S., and Benao, D. (2018). Understanding farmers' decisions on adaptation to climate change: exploring adoption of water harvesting technologies in burkina faso. Glob. Environ. Change 48, 243–254. doi: 10.1016/j.gloenvcha.2017.12.004
Charles, N., Rashid, H., and James, C. (2014). Analysis of determinants of farm-level adaptation measures to climate change in Southern Africa. J. Dev. Agric. Econ. 6, 232–241. doi: 10.5897/JDAE12.0441
Cirillo, P., and Gallegati, M. (2012). The empirical validation of an agent-based model. East. Econ. J. 38, 525–547. doi: 10.1057/eej.2011.34
Claessens, L., Antle, J. M., Stoorvogel, J. J., Valdivia, R. O., Thornton, P. K., and Herrero, M. (2012). A method for evaluating climate change adaptation strategies for small-scale farmers using survey, experimental and modeled data. Agric. Syst. 111, 85–95. doi: 10.1016/j.agsy.2012.05.003
Dang, H. L., Li, E., Nuberg, I., and Bruwer, J. (2014a). Farmers' assessments of private adaptive measures to climate change and influential factors: a study in the mekong delta, Vietnam. Nat. Hazards 71, 385–401. doi: 10.1007/s11069-013-0931-4
Dang, H. L., Li, E., Nuberg, I., and Bruwer, J. (2014b). Understanding farmers' adaptation intention to climate change: a structural equation modelling study in the mekong delta, Vietnam. Environ. Sci. Policy 41, 11–22. doi: 10.1016/j.envsci.2014.04.002
De Koning, K. (2019). Modelling human decisions in coupled human and natural systems (Ph.D. thesis), Department of Governance and Technology for Sustainability, University of Twente, Enschede, Netherlands.
De Pinto, A., Smith, V. H., and Robertson, R. D (2019). The role of risk in the context of climate change, land use choices and crop production: evidence from zambia. Clim. Res. 79, 39–53. doi: 10.3354/cr01581
Deressa, T. T., Hassan, R. M., and Ringler, C. (2011). Perception of and adaptation to climate change by farmers in the nile basin of ethiopia. J. Agric. Sci. 149, 23–31. doi: 10.1017/S0021859610000687
Deressa, T. T., Hassan, R. M., Ringler, C., Alemu, T., and Yesuf, M. (2009). Determinants of farmers' choice of adaptation methods to climate change in the nile basin of ethiopia. Glob. Environ. Change 19, 248–255. doi: 10.1016/j.gloenvcha.2009.01.002
Dile, Y. T., and Srinivasan, R. (2014). Evaluation of CFSR climate data for hydrologic prediction in data-scarce watersheds: an application in the blue nile river basin. J. Am. Water Resour. Assoc. 50, 1226–1241. doi: 10.1111/jawr.12182
Dobbie, S. (2013). Simulating Household Decision Making in Rural Malawi; Empirical Characterisation of Agent Behaviour During Times of Drought Stress. Southampton: University of Southampton.
Dobbie, S., Schreckenberg, K., Dyke, J. G., Schaafsma, M., and Balbi, S. (2018). Agent-based modelling to assess community food security and sustainable livelihoods. J. Artif. Soc. Soc. Simul. 21:9. doi: 10.18564/jasss.3639
DTMA. (2015). Maize in Kenya: Chance for Getting Back to Former Glory? A Quarterly Bulletin of the Drought Tolerant Maize for Africa Project vol. 4. Nairobi: CIMMYT & IITA.
Eiser, J. R., Bostrom, A., Burton, I., Johnston, D. M., McClure, J., Paton, D., et al. (2012). Risk interpretation and action: a conceptual framework for responses to natural hazards. Int. J. Disas. Risk Reduc. 1, 5–16. doi: 10.1016/j.ijdrr.2012.05.002
Elagib, N. A., Musa, A. A., and Sulieman, H. M. (2017). “Socio-hydrological framework of farmer-drought feedback: darfur as a case study nadir,” in Water Resources in Arid Areas: The Way Forward, eds O. Abdalla, A. Kacimov, M. Chen, A. Al-Maktoumi, T. Al-Hosni, and I. Clark (Cham: Springer), 461–479. doi: 10.1007/978-3-319-51856-5_27
Erenstein, O., Kassie, G. T., Langyintuo, A., and Mwangi, W. (2011a). Characterization of Maize Producing Households in Drought Prone Regions of Eastern Africa. Socioeconomics Working Paper 1. (Mexico, DF: CIMMYT).
Erenstein, O., Kassie, G. T., and Mwangi, W. (2011b). “Comparative analysis of maize based livelihoods in drought prone regions of eastern Africa: adaptation lessons for climate change,” in Increasing Agricultural Productivity & Enhancing Food Security in Africa: New Challenges and Opportunities, 1–13. Available online at: http://addis2011.ifpri.info/files/2011/10/Paper_4C_Olaf-Ernestein.pdf.
Few, R., Osbahr, H., Bouwer, L. M., Viner, D., and Sperling, F. (2006). Linking Climate Change Adaptation and Disaster Risk Management for Sustainable Poverty Reduction. Synthesis Report, MWH 475000177.001–6 rev. 1. Geneva.
FEWSNET (2010). A Climate Trend Analysis of Kenya. Available online at: http://www.fews.net/docs/Publications/FEWSKenyaClimateTrend~Analysis.pdf
Filho, W. L., and de Trinchera Gomez, J. (Eds.). (2018). Rainwater-Smart Agriculture in Arid and Semi- Arid Areas. Cham: Springer.
Floyd, D. L., Prentice-Dunn, S., and Rogers, R. W. (2000). A meta-analysis of research on protection motivation theory. J. Appl. Soc. Psychol. 30, 407-429 doi: 10.1111/j.1559-1816.2000.tb02323.x
Foster, T., and Brozović, N. (2018). Simulating crop-water production functions using crop growth models to support water policy assessments. Ecol. Econ. 152, 9–21. doi: 10.1016/j.ecolecon.2018.05.019
Foster, T., Brozović, N., Butler, A. P., Neale, C. M. U., Raes, D., Steduto, P., et al. (2017a). AquaCrop-OS : an open source version of FAO's crop water productivity model. Agric. Water Manag. 181, 18–22. doi: 10.1016/j.agwat.2016.11.015
Foster, T., Brozović, N., Butler, A. P., Neale, C. M. U., Raes, D., Steduto, P., et al. (2017b). “AquaCrop-OS : a tool for resilient management of land and water resources in agriculture,” in 19th EGU General Assembly, EGU2017, Proceedings From the Conference Held 23–28 April, 2017 (Vienna), 2842.
Funk, C., Nicholson, S. E., Landsfeld, M., Klotter, D., Peterson, P., and Harrison, L. (2015a). The centennial trends greater horn of africa precipitation dataset. Sci. Data 2, 1–15. doi: 10.1038/sdata.2015.50
Funk, C., Peterson, P., Landsfeld, M., Pedreros, D., Verdin, J., Shukla, S., et al. (2015b). The climate hazards infrared precipitation with stations–a new environmental record for monitoring extremes. Sci. Data 2, 1–21. doi: 10.1038/sdata.2015.66
Gbegbelegbe, S., Serem, J., Stirling, C., Kyazze, F., Radeny, M., Misiko, M., et al. (2017). Smallholder farmers in eastern africa and climate change: a review of risks and adaptation options with implications for future adaptation programmes. Clim. Dev.10, 289–306. doi: 10.1080/17565529.2017.1374236
Gbetibouo, G. A. (2009). Understanding Farmers' Perceptions and Adaptations to Climate Change and Variability: The Case of the Limpopo Basin, South AfricaAfrica. IFPRI Discussion Paper 849. Washington, DC: IFPRI Discussion Paper 00849.
Gebrehiwot, T., and van der Veen, A. (2015). Farmers prone to drought risk: why some farmers undertake farm-level risk-reduction measures while others not? Environ. Manag. 55, 588–602. doi: 10.1007/s00267-014-0415-7
Gigerenzer, G., and Goldstein, D. G. (1996). Reasoning the fast and frugal way: models of bounded rationality. Psychol. Rev. 62, 650–669. doi: 10.1037/0033-295X.103.4.650
González, T. I., Urquijo, J., Blauhut, V., Villarroya, F., and De Stefano, L. (2016). Learning from experience: a systematic review of assessments of vulnerability to drought. Nat. Hazards 80, 951–973. doi: 10.1007/s11069-015-2006-1
“Mwingi and Kitui districts agricultural management guide Chapter 3,” in Farm Management Handbook of Kenya. Natural Conditions and Farm Information. 2nd Edn, Vol. 11/ C, eds R. Jaetzold, H. Schmidt, Z. B. Hornet, and C. A. Shisanya (Nairobi: Ministry of Agriculture), 467–571.
Government of the Republic of Kenya (2017). Kenya Climate Smart Agriculture Strategy-2017-2026 (Nairobi: Ministry of Agriculture Livestock and Fisheries), 102.
Groeneveld, J., Müller, B., Buchmann, C. M., Dressler, G., Guo, C., Hase, N., et al. (2017). Theoretical foundations of human decision-making in agent-based land use models e a review. Environ. Model. Softw. 87, 39–48. doi: 10.1016/j.envsoft.2016.10.008
Grothmann, T., and Patt, A. (2005). Adaptive capacity and human cognition: the process of individual adaptation to climate change Glob. Environ. Change 15, 199–213. doi: 10.1016/j.gloenvcha.2005.01.002
Gunkela, A., and Külls, C. J. (2011). Towards agent-based modelling of stakeholder behaviour–a pilot study on drought vulnerability of decentral water supply in NE Brazil. Hydrol. Earth Syst. Sci. 8, 9089–9112. doi: 10.5194/hessd-8-9089-2011
Haer, T., Wouter Botzen, W. J., de Moel, H., and Jeroen, C. J. H. A. (2016a). Integrating household risk mitigation behavior in flood risk analysis: an agent-based model approach. Risk Anal. 37, 1997–1992. doi: 10.1111/risa.12740
Haer, T., Wouter Botzen, W. J., and Jeroen C.J.H., Aerts. (2019). Advancing disaster policies by integrating dynamic adaptive behaviour in risk assessments using an agent-based modelling approach. Environ. Res. Lett. 14:ab0770. doi: 10.1088/1748-9326/ab0770
Haer, T., Wouter Botzen, W. J., and Jeroen, C. J. H. A. (2016b). The effectiveness of flood risk communication strategies and the influence of social networks-insights from an agent-based model. Environ. Sci. Policy 60, 44–52. doi: 10.1016/j.envsci.2016.03.006
Hagenlocher, M., Meza, I., Anderson, C. C., Min, A., Renaud, F. G., Walz, Y., et al. (2019). Drought vulnerability and risk assessments: state of the art, persistent gaps, and research agenda. Environ. Res. Lett. 14:083002. doi: 10.1088/1748-9326/ab225d
Hailu, W., Moges, A., and Yimer, F. (2012). The effects of ‘Fanya Juu’ soil conservation structure on selected soil physical & chemical properties: the case of goromti watershed, western ethiopia. Resour. Environ. 2, 132–140. doi: 10.5923/j.re.20120204.02
Hansen, J. W., and Indeje, M. (2004). Linking dynamic seasonal climate forecasts with crop simulation for maize yield prediction in semi-arid kenya. Agric. For. Meteorol. 125, 143–157. doi: 10.1016/j.agrformet.2004.02.006
Heng, L. K., Hsiao, T., Evett, S., Howell, T., and Steduto, P. (2009). Validating the FAO aquacrop model for irrigated and water deficient field maize. Agron. J. 101, 488–498. doi: 10.2134/agronj2008.0029xs
Holden, S. T. (2015). Risk Preferences, Shocks and Technology Adoption: Farmers' Responses to Drought Risk. Report number: CLTS No.3/2015 (Norway, AS: Norwegian University of Life Sciences).
Holden, S. T., and Quiggin, J. (2017). Climate risk and state-contingent technology adoption: shocks, drought tolerance and preferences. Eur. Rev. Agric. Econ. 44, 285–308. doi: 10.1093/erae/jbw016
Ifejika, C., Kiteme, B., and Wiesmann, U. (2008). Droughts and famines : the underlying factors and the causal links among agro-pastoral households in semi-arid makueni district, Kenya. Glob. Environ. Change 18, 220–33. doi: 10.1016/j.gloenvcha.2007.05.001
Ifejika, S. C. (2010). Drought coping and adaptation strategies: understanding adaptations to climate change in agro-pastoral livestock production in makueni district, Kenya. Eur. J. Dev. Res. 22, 623–642. doi: 10.1057/ejdr.2010.39
ILRI (2006). Mapping Climate Vulnerability and Poverty in Africa 1. Available online at: https://cgspace.cgiar.org/bitstream/handle/10568/3416/9291462578_content.pdf?sequence=1
Jager, W., and Janssen, M. (2012). “An updated conceptual framework for integrated modeling of human decision making: The Consumat II,” in Paper for Workshop Complexity in the Real World @ ECCA - From Policy Intelligence to Intelligent Policy (Brussels).
Johnson, N., and Wambile, A. (Eds.). (2011). The Impacts of the Arid Lands Resource Management Project (ALRMPII) on Livelihoods and Vulnerability in the Arid and Semi-Arid Lands of Kenya. ILRI Research Report 25, 95. Available online at: https://cgspace.cgiar.org/bitstream/handle/10568/3416/9291462578_content.pdf?sequence=1
Kahneman, D., and Tversky, A. (1979). Prospect theory: an analysis of decision under risk. Econometrica 47, 263–292. doi: 10.2307/1914185
Kalungu, J. W., Filho, W. L., and Harris, D. (2013). Smallholder farmers' perception of the impacts of climate change and variability on rain-fed agricultural practices in semi-arid and sub-humid regions of Kenya. J. Environ. Earth Sci. 3, 129–140.
Kalungu, J. W., Filho, W. L., Onyango, D., and Cheruiyot, H. K. (2015). “Assessing the impact of rainwater harvesting technology as adaptation strategy for rural communities in Makueni,” in Handbook of Climate Change Adaptation, ed W. Leal Filho (Berlin; Heidelberg: Springer-Verlag) 1615–1634. doi: 10.1007/978-3-642-38670-1_23
KEFRI TARDA, and CDA. (2014). Integrated Programme to Build Resilience to Climate Change & Adaptive Capacity of Vulnerable Communities in Kenya. Nairobi: KEFRI; TARDA; CDA.
Kenya Ministry of Environment, and Natural Resources and Kenyan Water Resources Management Authority. (2013). The Project on the Development of the National Water Master Plan 2030. Final Report Volume. Kenya Ministry of Environment, Water and Natural Resources and Kenyan Water Resources Management Authority.
Keshavarz, M., and Karami, E. (2016). Farmers' pro-environmental behavior under drought: application of protection motivation theory. J. Arid Environ. 127, 128–136. doi: 10.1016/j.jaridenv.2015.11.010
Khisa, G. V. (2018). People's perception on climate change and its effects on livelihood in kitui county. Int. J. Dev. Sustain. 7, 70–81.
Khisa, G. V., Oteng, S. B., and Mikalitsa, S. M. (2014a). Effect of climate change on small scale agricultural production and food security in kitui district, Kenya. J. Agric. Nat. Res. Sci. 1, 34–44.
Khisa, G. V., Oteng, S. B., and Mikalitsa, S. M. (2014b). Coping strategies against climate change in agricultural production in kitui district, Kenya. Agric. Nat. Res. 1, 71–86.
Khisa, G. V. (2017). Rainfall and temperature variability and its effect on food security in Kitui county, Kenya. Int. J. Dev. Sustain. 6, 924–939.
Kimani, M. W., Gitau, A. N., and Ndunge, D. (2015). Rainwater harvesting technologies in makueni county, Kenya. Res. Inventy Int. J. Eng. Sci. 5, 39–49. doi: 10.6007/IJARBSS/v5-i4/1577
Kioko, M. J. B. (2013). Who stoel the rain? The case of recent severe droughts in Kenya. Eur. Sci. J. 9 (5):29–40. doi: 10.19044/esj.2013.v9n5p%25p
Kitinya, K. T, Onwonga, R. N., Onyango, C., Mbuvi, J. P., and Kironchi, G. (2012). Climate change and variability: farmers' perception, experience and adaptation strategies in C in Makueni County, Kenya. Asian J. Agric. Rural Dev. 2, 411–421.
Kitui County. (2013). First County Integrated Development Plan 2013–2017: Planning for Sustainable Socio-Economic Growth and Development. Available online at: http://www.kituicountyassembly.org/userfiles/KITUICOUNTYINTEGRATEDDEVELOPMENTPLANJuly2014(1).pdf
Klabunde, A., and Willekens, F. (2016). Decision-making in agent-based models of migration: state of the art and challenges. Eur. J. Popul. 32, 73–97. doi: 10.1007/s10680-015-9362-0
Kulecho, I. K., and Weatherhead, E. K. (2006). Adoption and experience of low-cost drip irrigation in Kenya. Irrig. Drainage 55, 435–44. doi: 10.1002/ird.261
Lasage, R., and Verburg, P. H. (2015). Evaluation of small scale water harvesting techniques for semi-arid environments. J. Arid Environ. 118, 48–57. doi: 10.1016/j.jaridenv.2015.02.019
Maddux, J. E., and Rogers, R. W. (1983). Protection motivation and self-efficacy: a revised theory of fear appeals and attitude change. J. Exp. Soc. Psychol. 19, 469–79. doi: 10.1016/0022-1031(83)90023-9
Makurira, H., Savenije, H. H. G., Uhlenbrook, S., Rockström, J., and Senzanje, A. (2011). The effect of system innovations on water productivity in subsistence rainfed agricultural systems in semi-arid tanzania. Agric. Water Manag. 98, 1696–1703. doi: 10.1016/j.agwat.2011.05.003
Mango, N., Kristjanson, P., Krishna, A., Radeny, M., Omolo, A., and Arunga, M. (2009). Why Is It Some Households Fall Into Poverty at the Same Time Others Are Escaping Poverty? Evidence From Kenya. Available online at: http://books.google.com/books?hl=en&lr=&id=H7Ii0jS7CPoC&oi=fnd&pg=PR4&dq=%22Planning+and+National+Development.+First+and+foremost,+the+authors'+wishes+to%22+%22Development+in+the+research+areas+we+visited+in+making+contacts+and+the%22+%22community+and+hou
Matere, S. J., Mbatia, O. L. E., Nzuma, J. M., and Nyamwaro, S. O. (2016). Financial benefit-cost analysis of terraces in maize-pigeon pea intercrop in semi-arid areas of Kenya. J. Agric. Environ. Sci. 5, 140–148. doi: 10.15640/jaes.v5n1a14
Matthews, R. B., Gilbert, N. G., Roach, A., Polhill, J. G., and Gotts, N. M. (2007). Agent-based land-use models: a review of applications. Landsc. Ecol. 22, 1447–59. doi: 10.1007/s10980-007-9135-1
Mfitumukiza, D., Barasa, B., and Emmanuel, N. (2017). Ecosystem-based adaptation to drought among agro-pastoral farmers: opportunities and constraints in nakasongola district, central Uganda. Environ. Manag. Sustain. Dev. 6:31. doi: 10.5296/emsd.v6i2.11132
Mo, F., Wang, J., Xiong, Y., Nguluu, S. N., and Li, F. M. (2016). Ridge-furrow mulching system in semiarid Kenya: a promising solution to improve soil water availability and maize productivity. Eur. J. Agron. 80, 124–36. doi: 10.1016/j.eja.2016.07.005
Moran, E., Carpenter, S. R., Ostrom, E., Deadman, P., Lubchenco, J., Folke, C., et al. (2007). Complexity of coupled human and natural systems. Science 317, 1513–1516. doi: 10.1126/science.1144004
Mude, A., Ouma, R., van de Steeg, J., Kariuki, J., Opiyo, D., and Tipilda, A. (2007). Kenya Adaptation to Climate Change in the Arid Lands: Anticipating, Adapting to and Coping With Climate Risks in Kenya–Operational Recommendations for Kaccal. ILRI Research Report 18. Nairobi: ILRI (International Livestock Research Institute).
Mugambiwa, S. S. (2018). Adaptation measures to sustain indigenous practices and the use of indigenous knowledge systems to adapt to climate change in mutoko rural district of Zimbabwe. Jàmbá: J. Disas. Risk Stud. 10, 1–9. doi: 10.4102/jamba.v10i1.388
Müller, B., Bohn, F., Dreßler, G., Groeneveld, J., Klassert, C., Martin, R., et al. (2013). Describing human decisions in agent based models–ODD + D, an extension of the ODD protocol. Environ. Model. Softw. 48, 37–48. doi: 10.1016/j.envsoft.2013.06.003
Mumo, L., Yu, J., and Fang, K. (2018). Assessing impacts of seasonal climate variability on maize yield in kenya assessing impacts of seasonal climate variability on maize yield in Kenya. Int. J. Plant Prod. 12, 297–307. doi: 10.1007/s42106-018-0027-x
Murgor, F., Owino, J., Cheserek, G., and Saina, C. (2013). Factors influencing farmers' decisions to adapt rain water harvesting techniques in keiyo district, Kenya. J. Emerg. Trends Econ. Manag. Sci. 4:133. Available online at: https://hdl.handle.net/10520/EJC135869
Muriu, F. W., Mucheru-Muna, M., Waswa, F., and Mairura, F. S. (2017). Socio-economic factors influencing utilisation of rain water harvesting and saving technologies in tharaka south, eastern Kenya. Agric. Water Manag. 194, 150–159. doi: 10.1016/j.agwat.2017.09.005
Mutua-Mutuku, M., Nguluu, S. N., Akuja, T., Lutta, M., and Bernard, P. (2017). Factors that influence adoption of integrated soil fertility and water management practices by smallholder farmers in the semi-arid areas of eastern kenya. Trop. Subtrop. Agroecosyst. 20, 141–153. Available online at: https://www.redalyc.org/articulo.oa?id=93950595012
Mutune, J., Mburu, J., Nyikal, R., and Kironchi, G. (2011). Determinants of adoption of conservation tillage practices in maize-cowpea cropping systems : the case of makueni district, Kenya. J. Soil Sci. Environ. Manag. 2, 354–361.
Mutunga, E. J., Ndungu, C. K., and Muendo, P. (2017). Smallholder farmers perceptions and adaptations to climate change and variability in Kitui County, Kenya. J. Earth Sci. Clim. Change 8:389. doi: 10.4172/2157-7617.1000389
Muyanga, M. (2004). Insights to rural household food insecurity in Kenya. Uganda J. Agric. Sci. 9, 791–96.
Mwongera, C., Läderach, P., Twyman, J., Mashisia, K., Okolo, W., Eitzinge, A., et al. (2013). Increasing food security and farming system resilience in east africa through wide-scale adoption of climate-smart agricultural practices. Food Agric. Org. 2012, 1–68.
Mwongera, C., Shikuku, K. M., Twyman, J., Läderach, P., Ampaire, E., Van Asten, P., et al. (2017). Climate smart agriculture rapid appraisal (CSA-RA): a tool for prioritizing context-specific climate smart agriculture technologies. Agric. Syst. 151, 192–203. doi: 10.1016/j.agsy.2016.05.009
Nakawuka, Prossie, Langan, S., Schmitter, P., and Barron, J. (2018). A review of trends, constraints and opportunities of smallholder irrigation in east Africa. Glob. Food Sec. 17, 196–212. doi: 10.1016/j.gfs.2017.10.003
Ngetich, K. F., Diels, J., Shisanya, C. A., Mugwe, J. N., Mucheru-muna, M., and Mugendi, D. N. (2012). “Calibration and validation of aquacrop model for maize in sub-humid and semi-arid regions of central highlands of Kenya,” in Third RUFORUM Biennial Meeting, 24–28 September 2012 (Entebbe).
Ngetich, K. F., Diels, J., Shisanya, C. A., Mugwe, J. N., Mucheru-muna, M., and Mugendi, D. N. (2014). Effects of selected soil and water conservation techniques on runoff, sediment yield and maize productivity under sub-humid and semi-arid conditions in Kenya. Catena 121, 288–296. doi: 10.1016/j.catena.2014.05.026
Ngigi, S. (2019). Technical evaluation of low head drip irrigation technologies in Kenya sustainable smallholder land & water management systems identifying promising smallholder water. Irrig. Drainage 57, 450–462. doi: 10.1002/ird.360
Ngigi, S. N. (2003). What is the limit of up-scaling rainwater harvesting in a river basin? Phys. Chem. Earth 28, 943–956. doi: 10.1016/j.pce.2003.08.015
Ngigi, S. N., Savenije, H. H. G., Rockström, J., and Gachene, C. K. (2005b). Hydro-economic evaluation of rainwater harvesting and management technologies: farmers' investment options and risks in semi-arid laikipia district of Kenya. Phys. Chem. Earth 30, 772–782. doi: 10.1016/j.pce.2005.08.020
Ngigi, S. N., Savenije, H. H. G., Thome, J. N., Rockström, J., and De Vries, F. W. T. P. (2005a). Agro-hydrological evaluation of on-farm rainwater storage systems for supplemental irrigation in laikipia district, Kenya. Agric. Water Manag. 73, 21–41. doi: 10.1016/j.agwat.2004.09.021
Njoka, J. T., Yanda, P., Maganga, F., Liwenga, E., Kateka, A., Henku, A., et al. (2016). Kenya: Country Situation Assessment Working Paper–PRISE. Nairobi: PRISE (Pathways to Resilience in Semi-arid Economies) project, Center for Sustainable Dryland Ecosystems and Societies (CSDES).
Nkatha, K. D. (2017). Factors Influencing the Adoption of ‘Zai’ Pit Farming Technology To Enhance Food Security : The Case of Makueni County, Kenya. Nairobi: University of Nairobi.
Nyariki, D. M., and Wiggins, S. (1997). Household food insecurity in sub-saharan africa: lessons from Kenya. Br. Food J. 99, 249–262. doi: 10.1108/00070709710179363
Nyoro, J. K., Kirimi, L., and Jayne, T. S. (2005). Competitivenes of Kenyan and Ugandan Maize Production: Challenge For The Future. Tegemeo Working paper 10. Nairobi: Tegemeo Institute of Agricultural Policy and Development, p. 1–37.
Ochieng, J., Kirimi, L., and Mathenge, M. (2016). Effects of climate variability and change on agricultural production: the case of small scale farmers in Kenya. NJAS Wageningen J. Life Sci. 77, 71–78. doi: 10.1016/j.njas.2016.03.005
Okeyo, A. I., Mucheru-Muna, M., Mugwe, J., Ngetich, K. F., Mugendi, D. N., Diels, J., et al. (2014). Effects of selected soil and water conservation technologies on nutrient losses and maize yields in the central highlands of Kenya. Agric. Water Manag. 137, 52–58. doi: 10.1016/j.agwat.2014.01.014
Oluoko-Odingo, A. A. (2011). Vulnerability and adaptation to food insecurity and poverty in Kenya. Ann. Assoc. Am. Geogr. 101, 1–20. doi: 10.1080/00045608.2010.532739
Omoyo, N. N., Wakhungu, J., and Otengi, S. (2015). Effects of climate variability on maize yield in the arid and semi arid lands of lower eastern Kenya. Agric. Food Sec. 4:8. doi: 10.1186/s40066-015-0028-2
Oremo, F. O. (2013). Small-scale farmers' perceptions and adaptation measures to climate change in Kitui County, Kenya (Thesis). Available online at: http://erepository.uonbi.ac.ke/handle/11295/59852
Palmer, P. I., and Smith, M. J. (2014). Model human adaptation to climate change. Nature 512:365. doi: 10.1038/512365a
Raes, D., Steduto, P., Hsiao, T. C., and Fereres, E. (2012). Chapter 3: Calculation Procedures. Aquacrop Reference Manual Verion 4.0. FAO. Available online at: www.fao.org/publications
Railsback, S. F., and Grimm, V. (Eds.). (2012). Agent-Based and Individual-Based Modeling. A Practical Introduction. Princeton, NJ; Oxford: Princeton University Press.
Rao, K. P. C. C., Ndegwa, W. G., Kizito, K., and Oyoo, A. (2011). Climate variability and change: farmer perceptions and understanding of intra-seasonal variability in rainfall and associated risk in semi-arid Kenya. Exp. Agric. 47, 267–291. doi: 10.1017/S0014479710000918
Rapsomanikis, G. (2010). The economic lives of smallholder farmers. Food Agric. Org. 4, 1–4. doi: 10.13140/RG.2.1.3223.9440
Recha, J., Kinyangi, J., and Omondi, H. (2012). Climate Related Risks and Opportunities for Agricultural Adaptation in Semi-Arid Eastern Kenya. Wageningen: CCAFS, p. 1–40.
Rockström, J., and Falkenmark, M. (2015). Agriculture: increase water harvesting in Africa. Nature 519, 283–285. doi: 10.1038/519283a
Schlüter, M., Baeza, A., Dressler, G., Frank, K., Groeneveld, J., Jager, W., et al. (2017). A framework for mapping and comparing behavioural theories in models of social-ecological systems. Ecol. Econ. 131, 21–35. doi: 10.1016/j.ecolecon.2016.08.008
Schreinemachers, P., and Berger, T. (2011). An agent-based simulation model of human-environment interactions in agricultural systems. Environ. Model. Softw. 26, 845–859. doi: 10.1016/j.envsoft.2011.02.004
Shaw, W. D., and Woodward, R. T. (2008). Why environmental and resource economists should care about non-expected utility models. Resour. Energy Econ. 30, 66–89. doi: 10.1016/j.reseneeco.2007.05.001
Shiferaw, B., Tesfaye, K., Berresaw, M. K., Abate, T., Prasanna, B. M., and Menkir, A. (2014). Managing vulnerability to drought and enhancing livelihood resilience in sub-saharan africa: technological, institutional and policy options. Weather Clim Extremes 3, 67–79. doi: 10.1016/j.wace.2014.04.004
Shikuku, K. M., Winowiecki, L., Twyman, J., Eitzinger, A., Perez, J. G., Mwongera, C., et al. (2017). Smallholder farmers' attitudes and determinants of adaptation to climate risks in east Africa. Clim Risk Manag. 16, 234–245. doi: 10.1016/j.crm.2017.03.001
Sijali, I. V., and Okumu, R. A. (2002). New Irrigation Technologies. The Changing Face of Irrigation in Kenya: Opportunities for Anticipating Change in Eastern and Southern Africa. Available online at: https://publications.iwmi.org/pdf/H030833.pdf
Singh, P. K., and Chudasama, H. (2017). Pathways for drought resilient livelihoods based on people's perception. Clim. Change 140, 179–193. doi: 10.1007/s10584-016-1817-8
Sivapalan, M., Savenije, H. H. G., and Blöschl, G. (2012). Socio-hydrology: a new science of people and water. Hydrol. Proces. 26, 1270–1276. doi: 10.1002/hyp.8426
Steduto, P., Hsiao, T. C., Fereres, E., and Raes, D. (2012). Crop Yield Response to Water. Fao Irrigation and Drainage Paper. Available online at: www.fao.org
Steduto, P, Raes, D., Hsiao, T. C., and Fereres, E. (2009). AquaCrop: a new model for crop prediction under water deficit conditions,” in Drought Management: Scientific and Technological Innovations, ed A. López-Francos (Zaragoza: CIHEAM), 285–292.
Stefanovi, Julia. (2015). Smallholder Farming Systems in Kenya: Climate Change Perception, Adaptation and Determinants. Basel: Uneversity of Basel.
Sutton, S. (2014). “Theory of planned behaviour,” in Cambridge Handbook of Psychology, Health and Medicine, 2nd Edn, eds S. Ayers, A. Baum, C. McManus, S. Newman, K. Wallston, J. Weinman, et al. (Cambridge: Cambridge University Press), 223–228.
Tegemeo Institute (2000). Tegemeo Agricultural Monitoring and Policy Analysis Project (TAMPA 1) - Household Survey 2000 Data Documentation.. (Nairobi: Egerton University Tegemeo Institute of Agricultural Policy and Development; Michigan State University), 1–14.
Tegemeo Institute (2004). Tegemeo Agricultural Policy Research Analysis (TAPRA) Project–Household Survey 2004. Nairobi: Tegemeo Institute.
Tegemeo Institute (2007). Tegemeo Agricultural Policy Research Analysis (TAPRA) Project–Household Survey 2007. Nairobi: Tegemeo Institute.
Tegemeo Institute (2010). Tegemeo Agricultural Policy Research Analysis (TAPRA) Project–Household Survey 2010. Nairobi: Tegemeo Institute.
TEGEMEO. (2000). Tegemeo Institute of Agricultural Policy and Michigan State University Tegemeo Agricultural Monitoring and Policy. Policy Analysis, 1–14.
Tongruksawattana, S. (2014). Climate Shocks and Choice of Adaptation Strategy for Kenyan Maize-Legume Farmers: Insights from Poverty, Food Security and Gender Perspectives. Socioeconomics Program Working Paper. 11. Socioeconomics Program Working Paper. Mexico, DF: CIMMYT.
Ulrich, A., Speranza, C. I., Roden, P., Kiteme, B., Wiesmann, U., and Nüsser, M. (2012). Small-scale farming in semi-arid areas: livelihood dynamics between 1997 and 2010 in laikipia, Kenya. J. Rural Stud. 28, 241–251. doi: 10.1016/j.jrurstud.2012.02.003
United Nations Development Programme (2007). Kenya Natural Disaster Profile. Enhanced Security Unit, 1–42. Available online at: papers2://publication/uuid/E50886E6-A430-492A-965B-A81CFBAF51C5
Van Dijk, W. F.A., Lokhorst, A. M., Berendse, F., and de Snoo, G. R. (2016). Factors underlying farmers' intentions to perform unsubsidised agri-environmental measures. Land Use Policy 59, 207–216. doi: 10.1016/j.landusepol.2016.09.003
Van Duinen, R., Filatova, T., Geurts, P., and van der Veen, A. (2015a). Coping with drought risk: empirical analysis of farmers' drought adaptation in the south-west netherlands. Region. Environ. Change 15, 1081–1093. doi: 10.1007/s10113-014-0692-y
Van Duinen, R., Filatova, T., Geurts, P., and van der Veen, A. (2015b). Empirical analysis of farmers' drought risk perception: objective factors, personal circumstances, and social influence. Risk Anal. 35, 741–755. doi: 10.1111/risa.12299
Van Duinen, R., Filatova, T., Jager, W., and van der Veen, A. (2016). Going beyond perfect rationality: drought risk, economic choices and the influence of social networks. Ann. Region. Sci. 57, 335–369. doi: 10.1007/s00168-015-0699-4
Van Duinen, R., Filatova, T., and van der Veen, A. (2012). “The role of social interaction in farmers' climate adaptation choice,” in International Environmental Modelling and Software Society (IEMSs) 2012. International Congress on Environmental Modelling and Software Managing Resources of a Limited Planet. Available online at: http://doc.utwente.nl/81052/1/2012.12.07_RiannevanDuinenfinal.pdf
Van Loon, A. F., Stahl, K., Di Baldassarre, G., Clark, J., Rangecroft, S., Wanders, N., et al. (2016). Drought in a human-modified world: reframing drought definitions, understanding, and analysis approaches understanding, and analysis approaches. Hydrol. Earth Syst. Sci. 20, 3631–3650. doi: 10.5194/hess-20-3631-2016
Van Oel, P. R., and Van Der Veen, A. (2011). Using agent-based modeling to depict basin closure in the naivasha basin, kenya: a framework of analysis. Proc. Environ. Sci. 7, 32–37. doi: 10.1016/j.proenv.2011.07.007
Van Valkengoed, A. M., and Steg, L. (2019). Meta-analyses of factors motivating climate change adaptation behaviour. Nat. Clim. Change 9, 158–163. doi: 10.1038/s41558-018-0371-y
Vanuytrecht, E., Raes, D., Steduto, P., Hsiao, T. C., Fereres, E., Lee K., et al. (2014). AquaCrop : FAO'S crop water productivity and yield response model. Environ. Model. Softw. 62, 351–360. doi: 10.1016/j.envsoft.2014.08.005
Venzi, F. S., Mulwa, S. A., and Ndunge, K. D. (2015). Modern technology and sustainable irrigation of small scale dry-land farming in kitui county, Kenya. Afr. J. Project Plan. Manag. 1, 100–118. Available online at: http://hdl.handle.net/11295/86895
Viglione, A., Di Baldassarre, G., Brandimarte, L., Kuil, L., Carr, G., Salinas, J. L., et al. (2014). Insights from socio-hydrology modelling on dealing with flood risk–roles of collective memory, risk-taking attitude and trust. J. Hydrol. 518, 71–82. doi: 10.1016/j.jhydrol.2014.01.018
Von Neumann, J., and Morgenstern, O. (1945). Theory of Games and Economic Behavior. Princeton: John von Neumann.
Wamari, J. O., Sijali, V. I., Kheng, L., Mutwiri, J., and Obutiati, E. (2012). Use of Aquacrop Model to Predict Maize Yields Under Varying Rainfall and Temperature in a Semi-Arid Environment in Kenya. JMRS.
Wambua, R. M., and Akuja, T. E. (2016). Assessment of water and soil management approaches for small-scale dry land farming in Kitui. Int. J. of Agr. Res. and Rev. 4, 504–13.
Wens, M. (2018). Survey Report Kitui. Amsterdam. Available online at: https://research.vu.nl/en/publications/survey-report-kitui-kenya-results-of-a-questionaire-regardings-us
Wens, M. (2019). Survey Report Kitui. Amsterdam. Available online at: https://research.vu.nl/en/publications/survey-report-kitui-kenya-expert-evaluation-of-model-setup-and-pr
Wens, M., Johnson, M. J., Zagaria, C., and Veldkamp, T. I. E. (2019). Integrating human behavior dynamics into drought risk assessment—a sociohydrologic, agent-based approach. WIREs Water 6:e1345. doi: 10.1002/wat2.1345
Wheeler, S., Zuo, A., and Bjornlund, H. (2013). Farmers' climate change beliefs and adaptation strategies for a water scarce future in australia. Glob. Environ. Change 23, 537–47. doi: 10.1016/j.gloenvcha.2012.11.008
Wilhite, D. A. (2000). “Chapter 1 drought as a natural hazard: concepts and definitions,” in Drought: A Global Assessment, 1st Edn., Vol. I, ed D. A. Wilhite (London: Routledge), 3–18.
Wilhite, D. A. (2002). Combating drought through preparedness. Nat. Resour. Forum 26, 275–285. doi: 10.1111/1477-8947.00030
Winter-nelson, A., and Amegbeto, K. (1998). Option values to conservation and agriculural price policy: application to terrace construction in Kenya. Am. J. Agr. Econ. 80, 409–418. doi: 10.2307/1244512
Wolka, K., Mulder, J., and Biazin, B. (2018). Effects of soil and water conservation techniques on crop yield, runoff and soil loss in sub-saharan africa: a review. Agric. Water Manag. 207, 67–79. doi: 10.1016/j.agwat.2018.05.016
Keywords: agent-based model, drought adaptation, drought risk, adaptive behavior, adaptation decisions, socio-hydrology
Citation: Wens M, Veldkamp TIE, Mwangi M, Johnson JM, Lasage R, Haer T and Aerts JCJH (2020) Simulating Small-Scale Agricultural Adaptation Decisions in Response to Drought Risk: An Empirical Agent-Based Model for Semi-Arid Kenya. Front. Water 2:15. doi: 10.3389/frwa.2020.00015
Received: 28 March 2020; Accepted: 03 June 2020;
Published: 17 July 2020.
Edited by:
Saket Pande, Delft University of Technology, NetherlandsReviewed by:
Mohammad Ghoreishi, University of Saskatchewan, CanadaMohammad Faiz Alam, International Water Management Institute, Sri Lanka
Jimmy O'Keeffe, Imperial College London, United Kingdom
Copyright © 2020 Wens, Veldkamp, Mwangi, Johnson, Lasage, Haer and Aerts. This is an open-access article distributed under the terms of the Creative Commons Attribution License (CC BY). The use, distribution or reproduction in other forums is permitted, provided the original author(s) and the copyright owner(s) are credited and that the original publication in this journal is cited, in accordance with accepted academic practice. No use, distribution or reproduction is permitted which does not comply with these terms.
*Correspondence: Marthe Wens, marthe.wens@vu.nl