- 1Institute for Environment and Human Security, United Nations University, Bonn, Germany
- 2Working Group Eco-Hydrology and Water Resource Management, Department of Geography, University of Bonn, Bonn, Germany
- 3Working Group Hydro-Climatology and Water Resource Management, Research Laboratory on Spaces, Exchanges and Human Security, Department of Geography, University of Lomé, Lomé, Togo
- 4Graduate Research Program Climate Change and Water Resource, West Africa Science Service Centre on Climate Change and Adapted Land Use, University of Abomey-Calavi, Abomey-Calavi, Benin
River floods are a common environmental hazard, often causing severe damages, loss of lives and livelihood impacts around the globe. The transboundary Lower Mono River Basin of Togo and Benin is no exception in this regard, as it is frequently affected by river flooding. To enable adequate decision-making in the context of flood risk management, it is crucial to understand the drivers of risk, their interconnections and how they co-produce flood risks as well as associated uncertainties. However, methodological advances to better account for these necessities in risk assessments, in data-scarce environments, are needed. Addressing the above, we developed an impact chain via desk study and expert consultation to reveal key drivers of flood risk for agricultural livelihoods and their interlinkages in the Lower Mono River Basin of Benin. Particularly, the dynamic formation of vulnerability and its interaction with hazard and exposure is highlighted. To further explore these interactions, an alpha-level Bayesian Network was created based on the impact chain and applied to an exemplary what-if scenario to simulate changes in risk if certain risk drivers change. Based on the above, this article critically evaluates the benefits and limitations of integrating the two methodological approaches to understand and simulate risk dynamics in data-scarce environments. The study finds that impact chains are a useful model approach to conceptualize interactions of risk drivers. Particularly in combination with a Bayesian Network approach, the method enables an improved understanding of how different risk drivers interact within the system and allows for dynamic simulations of what-if scenarios, for example, to support adaptation planning.
Introduction
Floods are one of the most devastating hazards, regularly causing considerable damage to goods, infrastructure, human well-being, and livelihoods (Nguyen et al., 2020; UNDRR - UN Office for Disaster Risk Reduction, and CRED - Centre for Research on the Epidemiology of Disasters, 2020). Damages and losses due to flooding are predicted to further increase in the future (Jongman et al., 2012; Hirabayashi et al., 2013; Dottori et al., 2018; Intergovernmental Panel on Climate Change, 2021) calling for knowledge-based solutions to reduce current and prevent future flood risks. Understanding the root causes, drivers, patterns, and dynamics of flood risks for people, assets, sectors and systems, and associated uncertainties is important to inform adequate risk management (Adger et al., 2018; Hagenlocher et al., 2020). Yet, a limiting factor for effective decision-making is a lack of understanding of the highly dynamic processes and uncertainties within flood risk assessments (Di Baldassarre et al., 2014). Only a clear understanding of the system, its dynamic behavior, and specific risks (e.g., risk of loss of life, risk of impacts on livelihoods, risk of infrastructure damage, etc.) allows to e.g., identify potential unintended outcomes of intended adaptation measures. This implies also a sound understanding of interconnections as well as associated uncertainties. Therefore, to enhance decision-making for flood risk reduction and adaptation, improved methodologies for assessing flood risk dynamics are required.
One of the most frequently mentioned dimensions of risk dynamics refers to processes and variations of risk and risk drivers over time (Villa et al., 2014; Sinare and Gordon, 2015; Di Baldassarre et al., 2019; Michaelis et al., 2020). A further, frequently considered dimension of risk dynamics refers to a variation over space (Bagstad et al., 2013; Villa et al., 2014; Sinare and Gordon, 2015; Sinare et al., 2016; Di Baldassarre et al., 2019; Shinn and Hall-Reinhard, 2019). Furthermore, dynamic interactions within and among systems in the form of interactions of risk drivers or feedback loops are identified in the literature (Turner et al., 2003; Villa et al., 2014; Di Baldassarre et al., 2019; Michaelis et al., 2020). In addition to risk dynamics, the concept of uncertainty is commonly addressed in the scientific discourse of water and flood risk management (Höllermann and Evers, 2015, 2019; Di Baldassarre et al., 2016; Höllermann, 2018) as well as in the context of risk assessment and risk modeling (Döll and Romero-Lankao, 2017; De Brito et al., 2019; Di Baldassarre et al., 2019; Hagenlocher et al., 2020). Uncertainty in the context of participatory risk assessment and modeling results from multiple sources. Examples are ontological and epistemic uncertainty (Di Baldassarre et al., 2016; Döll and Romero-Lankao, 2017; Jurgilevich et al., 2017; Hagenlocher et al., 2020), uncertainty related to software and data (Walker et al., 2003; Hagenlocher et al., 2020), uncertainty regarding the conceptual representation of the system and methodological approach (Döll and Romero-Lankao, 2017; Jurgilevich et al., 2017; Hagenlocher et al., 2020), as well as bias, ambiguity and linguistic uncertainty in participatory processes (Döll and Romero-Lankao, 2017; Hagenlocher et al., 2020).
Based on the high level of uncertainty and dynamic processes regarding flood risks, a need for innovative assessment and modeling approaches has been identified (Terzi et al., 2019). In addition, Di Baldassarre et al. (2014) and Merz et al. (2014) criticize that flood risk assessments have historically been conducted from a rather narrow hazard perspective. Following the widely acknowledged understanding of risk as a function of hazard, exposure, and vulnerability (IPCC - Intergovernmental Panel on Climate Change, 2014; UNDRR - UN Office for Disaster Risk Reduction, 2019), Sivapalan et al. (2012), Merz et al. (2014) and Di Baldassarre et al. (2019) emphasize the need to better understand the interactions between society and floods. Over the past decades, a large array of approaches and methods for comprehensive climate, natural hazard and disaster risk assessments that consider hazard, exposure and vulnerability drivers have been developed and applied across sectors, systems, spatial and temporal scales (see e.g., Jurgilevich et al., 2017; Adger et al., 2018; Ward et al., 2020 for recent reviews of the literature). However, existing reviews focusing specifically on flood risk assessments (e.g., Apel et al., 2009; de Moel et al., 2015; Díez-Herrero and Garrote, 2020; Ward et al., 2020; Nguyen et al., 2021) reveal that despite these developments the focus is still largely centered around the hydrological part of flood risk or the use of damage functions to represent the vulnerability of specific assets to flooding. More comprehensive assessments that also take into account social, economic, political or governance-related drivers of flood risks, and their dynamic interaction, are still rather the exception.
Moreover, it is evident that the inclusion of participatory methods and transdisciplinary approaches in risk assessments is crucial for an adequate reflection of local needs and knowledge (Döll and Romero-Lankao, 2017; Cains and Henshel, 2019; Hagenlocher et al., 2020). To enhance the understanding of how different drivers of risk interact with a focus on specific possible impacts, impact chains have been introduced as a novel approach to assess vulnerabilities and risks associated with climate change and natural hazards in a participatory manner (Fritzsche et al., 2014; Zebisch et al., 2017; Hagenlocher et al., 2018). Despite their focus on inter-driver relations and specific risks, impact chains have mainly been used for static index-based vulnerability and risk assessments that, per definition, do not enable the analysis of how different drivers of risk influence each other. For example, the methodology has been applied to assess the vulnerability of smallholders pertaining to water supply in Bolivia (Zebisch et al., 2021), vulnerability to climate change and adaptation impacts in Pakistan (Zebisch et al., 2021), as well as to identify priority areas for adaptation to climatic impacts on agriculture in Burundi (Schneiderbauer et al., 2020). Nevertheless, approaches to using the impact chain methodology as a starting point for more dynamic risk assessments are still lacking.
To account for risk dynamics and uncertainty the use of sophisticated assessment tools such as Agent-based Models or Bayesian Networks (BN) is on the rise (Terzi et al., 2019). Frequently, BN modeling is referred to in the literature as a well-suited approach for the integration of multiple data sources, including qualitative information (Balbi et al., 2016; Terzi et al., 2019). Moreover, BNs are appropriate for reflecting uncertainty in a transparent way, which is especially beneficial in complex systems with a high level of dynamic processes and a low level of certainty (Chen and Pollino, 2012). While BNs have already been widely used in disciplines such as medical diagnostics (Lauritzen and Spiegelhalter, 1988), their popularity is increasingly growing also in other disciplines such as environmental and ecological management (Marcot et al., 2006; Harris et al., 2017; Graham et al., 2019) as well as in the context of coastal and riverine flood risk assessment and management (Balbi et al., 2016; Maskrey et al., 2016; Abebe et al., 2018; Jung et al., 2021). Though the idea for a structured integration of BN with participatory expert consultation for environmental management emerged at least two decades ago (Cain, 2001; Marcot et al., 2006; Maskrey et al., 2016), the integration of BN remains widely unexplored in the realm of flood risk science. For instance, a study by Wu et al. (2020) integrates BN modeling with geographic information system (GIS) methods to assess urban flood risk. Yet, the study refrains from stakeholder consultation. The integration of stakeholder-validated impact chains with BN modeling to account for flood risk dynamics and uncertainty as illustrated in this article remains a novel approach.
To apply this novel approach, the Lower Mono River Basin in Benin was selected as a case study area. The basin is regularly negatively affected by recurring fluvial floods, which pose a real threat to local livelihoods (Nicholson et al., 2021). Since agriculture is a crucial source of income for many rural households in Benin (ILO - International Labour Organization, 2019), this research focuses on the specific risk of loss of agricultural livelihoods due to flooding in the Lower Mono River Basin. Regarding the study area, several flood risk assessments have been carried out (Kissi et al., 2015; Ntajal et al., 2017). Kissi et al. (2015) provide a static, indicator-based flood vulnerability assessment for selected communities in the Lower Mono River Basin in Togo, while Ntajal et al. (2017) considered hazard, exposure, vulnerability, and capacities to describe flood risk. In contrast to Kissi et al. (2015) and Ntajal et al. (2017), Schudel et al. (forthcoming) take a more holistic approach by assessing flood risk including hazard, exposure, and vulnerability for the whole transboundary Lower Mono River Basin using an indicator-based approach. Yet, specific impacts of flooding (e.g., agricultural livelihoods), as well as the dynamic relation among the different drivers of risk, are not sufficiently acknowledged in existing assessments. The study area can be considered data-scarce regarding the specific flood risk for agricultural livelihoods. First, qualitative information on drivers of flood risk for agricultural livelihoods in the region is limited since no study, to our knowledge, has focused on this impact-specific risk. Second, while general socio-economic indicators are available for the region (INSAE - Institut National de la Statistique et de l'Analyse Economique, 2016b), the amount, scale, and scope of available quantitative data is not sufficient to adequately assess this highly context-dependent risk. Hence, the need for an improved understanding of the dynamic interaction of risk drivers to support the formulation of adequate strategies and policies to reduce the risk of severe impacts is pressing. Addressing the above-outlined research gaps the main objectives of this study are:
(i) to improve the understanding of how different risk drivers interact to co-produce flood risk for agricultural livelihoods in the research area; and,
(ii) to illustrate and critically discuss benefits and limitations of integrating impact chains with BN analysis as a tool for understanding and simulating risk dynamics in data-scarce environments.
To structure the article, first, the methodology (section Methodology) is introduced, containing information regarding the study area, the methodological approach for impact chain development and validation as well as the rationale for qualitative BN development. Second, the results are presented (section Results) including results obtained through the impact chain as well as results from an illustrative what-if scenario that was applied to the qualitative BN. Third, the benefits and limitations of the methodology, and their implications are critically discussed (section Discussion). Ultimately, the article concludes with final remarks (section Conclusion).
Methodology
To address the first objective, an impact chain was developed and validated applying a qualitative, participatory, multi-method approach. In a second step, a qualitative alpha-level BN was created based on the impact chain. Its utility for simulating risk dynamics was illustrated and evaluated with an exemplary what-if scenario (Figure 1).
Study Area
Benin forms part of a geographic region that is highly affected by extreme weather and climate change (Niang et al., 2014). The tropical regions of West Africa are estimated to be affected by climate trends 10 to 20 years earlier than the international average (Niang et al., 2014). In contrast to the clear warming trends, precipitation trends for the study area are subject to greater variability and uncertainty. Projected precipitation developments of different models present considerable inconsistencies regarding the amplitude and direction of change (Niang et al., 2014). The high uncertainty of precipitation development is further underlined by the diverging trends identified for the region (Lamboni et al., 2019). Even though precipitation development under climate change for West Africa remains scientifically contested, increasing trends in river floods since 1980 are observed for the region (Emmanuel et al., 2019; Tramblay et al., 2020). For the time period from 1961 to 2016, Obada et al. (2021) have identified a decrease in the amount of consecutive wet days, but an intensification in extreme precipitation in Benin. This development leads to an increase in flash floods in the region. Also, for southern Benin, including the Mono River Basin, trends of increasing flood frequency and extreme rainfall are observed (Sanchez et al., 2012; Baudoin, 2014).
The Lower Mono River, describing the Mono River south of the Nangbeto Dam, is a significant hydrological and political factor for Benin, demarcating the southern part of the border between Benin and Togo (Figure 2). The Lower Mono River Basin is characterized by its low elevation and flat to gentle slopes and valleys (INSAE - Institut National de la Statistique et de l'Analyse Economique, 2016b; Ntajal et al., 2017). Furthermore, land use/land cover is dominated by savanna vegetation and rainfed agricultural land (INSAE - Institut National de la Statistique et de l'Analyse Economique, 2016b; ESA - European Space Agency, 2020). Regarding socio-economic configurations of the study area, a first generic impression may be derived from the Human Development Index (HDI). Benin has an HDI value of 0.52, corresponding to the 163rd position in the international ranking (UNDP - United Nations Development Programme, 2019). Especially in the rural areas, agriculture remains the main economic sector in terms of employment rates, with 38.3% of the total workforce in Benin engaged in agriculture (The World Bank, 2021). Also, a lack of income diversity was identified for the rural population which is mostly dependent on subsistence farming (Kissi et al., 2015; Ntajal et al., 2017). On the Beninese side of the Lower Mono River Basin, more than 97% of the agricultural households focus on crop production, while livestock farming and fisheries are negligible. Cassava, maize and beans are the most cultivated products, followed by peanuts (Couffo district) and vegetables (Mono district) (INSAE - Institut National de la Statistique et de l'Analyse Economique, 2016a).
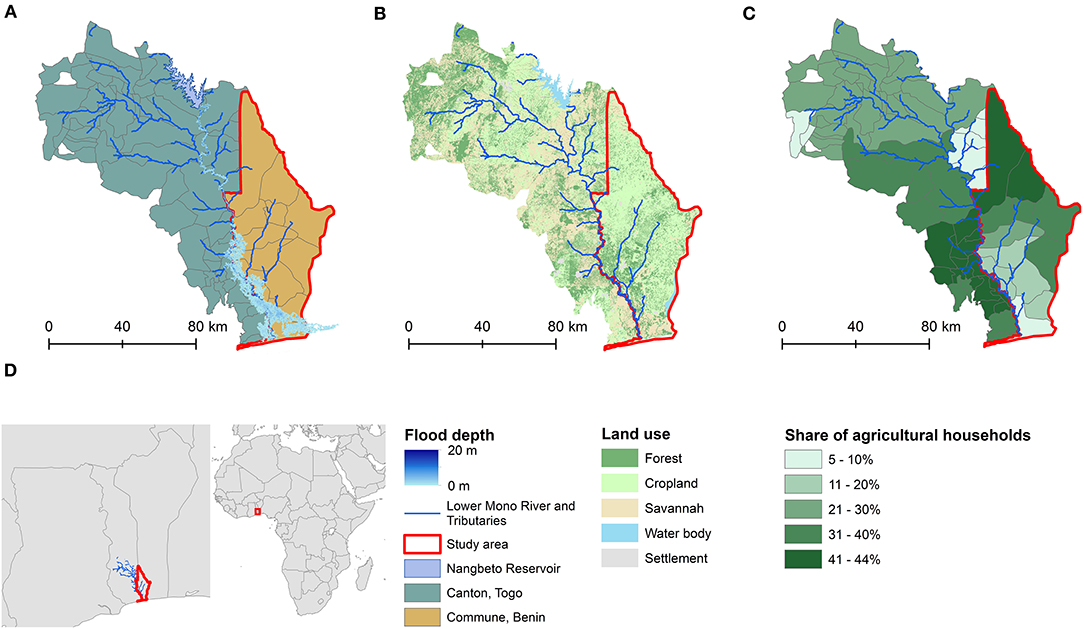
Figure 2. Characteristics of the Lower Mono River Basin. (A) Extent of a 10-year return period flood hazard in the Lower Mono River Basin (Almoradie and Houngue, 2021), (B) land use in the Lower Mono River Basin (CENATEL - Centre National de Télédétection et de Suivi Ecologique, 2015; MEDDPN - Ministry of Environment Forest Resources, 2015), (C) share of agricultural households per administrative area (INSAE - Institut National de la Statistique et de l'Analyse Economique, 2016b; NSEED - Institut National de la Statistique et des Etudes Economiques et Démographiques et AFRISTAT, 2019), (D) location of Lower Mono River Basin in West Africa.
One of the central issues for concern is the destructive impact of flooding on agricultural livelihood systems and the population directly depending on agriculture (Ntajal et al., 2017). Generally driven by heavy precipitation, flood events mostly occur during the rainy seasons which extend from May to June and September to October (Kissi et al., 2015). A crucial component of the hydrological system of the Mono River is the Nangbeto Dam, located in Togo. Due to its hydroelectric power station, Nangbeto Dam is also an important socio-economic factor in the region. However, its contribution to flood events in the Lower Mono River Basin is contested among the population (Ago et al., 2005). Nevertheless, the presence of the Nangbeto Dam has reduced the intra-annual discharge variability of the Mono River leading to the eradication of former seasonal dry outs in the lower basin (Ago et al., 2005). In conclusion, the frequent occurrence of river floods, combined with the socio-economic configurations of the region including the high dependency on subsistence farming suggests the potential for elevated flood risk in the study area.
Impact Chain Development and Participatory Impact Chain Validation
To apply the impact chain methodology, first the specific risk of interest (here: impacts on agricultural livelihoods) was selected. This was followed by the identification of risk drivers and their interactions, and further refinement and validation of the impact chain based on a multi-method approach. A draft impact chain was developed based on the flood risk drivers identified in a participatory approach by Schudel et al. (forthcoming) and based on the experiences of several of the co-authors from a field visit to the study area. To specify the impact chain for the risk of loss of agricultural livelihoods, a literature review (Supplementary Data Sheet 3) was conducted, followed by two rounds of expert consultations, which refined the impact chain. For the literature review, sources used by Schudel et al. (forthcoming) were screened with a particular focus on agricultural livelihoods. Subsequently, the draft impact chain was validated via stakeholder consultation.
Due to the global COVID-19 pandemic, in-situ workshops were not feasible, calling for alternative approaches to stakeholder engagement. In response to this, a series of online workshops (08/2020) were organized. The group of 17 participants consisted of representatives from academic, governmental, and non-governmental institutions (Supplementary Data Sheet 4). The group was homogeneous considering their local expertise from the Lower Mono River Basin and heterogeneous from a thematic perspective. The group's heterogeneity of thematic expertise reduces the potential for epistemic uncertainty and thematic bias but increases the potential for ambiguity and linguistic uncertainty (Döll and Romero-Lankao, 2017). Consequently, a common understanding of key terminology and concepts was established with two introductory videos, one explaining the concept of risk following the IPCC fifth assessment report (IPCC - Intergovernmental Panel on Climate Change, 2014), the other explaining the conceptual framework of impact chains in the French language. The first session aimed at validating general drivers of flood risk and associated indicators derived from literature and a field survey in October 2019 (Schudel et al., forthcoming). Specific questions pertaining to key drivers of flood risk for agricultural livelihoods were included in this session as well (Schudel et al., forthcoming). The second workshop did not focus on key drivers of risk, but on their dynamic interactions and interplay that co-produces the particular risk. From a methodological perspective, online surveys were followed by group discussions. The survey asked for the evaluation of the strength of each causal link contained in the impact chain. The potential answer options included “no influence,” “low importance,” “high importance” and “I don't know.” All links with at least one answer of “no influence” were selected for further discussion. Also, all links without a ≥75% tendency toward one answer option were selected for subsequent discussion (Supplementary Data Sheet 1).
Development of a Bayesian Network Based on Impact Chain
Based on the final impact chain which was validated by the stakeholders, a BN was developed to illustrate how these methodological approaches can be combined. Next, the BN was applied to assess dynamic interactions of risk drivers and their changes using an exemplary what-if scenario.
During the workshops, stakeholders adjusted and validated the content and structure of the impact chain and further highlighted factors that are considered particularly relevant for explaining flood risk for agricultural livelihoods in the region. The authors used this information to simplify the impact chain focusing on particularly relevant risk drivers and interactions (Supplementary Figure 1). This simplified impact chain was then translated into the graphical component of a BN model. The underlying probability values which are stored for each variable in their conditional probability tables (CPTs) have been qualitatively selected and refined based on the authors' judgment. This process was strongly informed by the authors' experiences made during the impact chain development and validation process. As the process of parameterization was strictly qualitative, the assigned probability values shall depict a general logic of interaction rather than exact quantitative calculations of likelihood (further information on the methodology is provided in Supplementary Data Sheet 2; full list of CPT parameters is provided in Supplementary Document 3).
Our approach to BN modeling is partially informed by the suggested methodology in Marcot et al. (2006) and consists of six consequent steps (Table 1). For BN modeling we used the GeNIe software (BayesFusion, 2020). The first three steps include adjustments to the graphical and logical structure of the final impact chain and subsequent modifications of the directed acyclic graph (DAG) which refers to the graphical component of the BN. Steps 4 and 5 account for the quantitative part of the BN contained in the CPTs of the network's variables and encompass a first parameterization, qualitative evaluation, and successive qualitative re-parameterization of the model, generating an operational alpha-level model in the sense of Marcot et al. (2006). Ultimately, step 6 comprises the development of an exemplary scenario based on the alpha-level model (Table 1; Supplementary Data Sheet 2).
Results
Impact Chain: Key Drivers of Risk and Their Interlinkages
Figure 3 shows the final, validated impact chain. It comprises drivers and their interlinkages related to hazard, exposure, and vulnerability as well as external drivers and how they all contribute to the impact-specific risk.
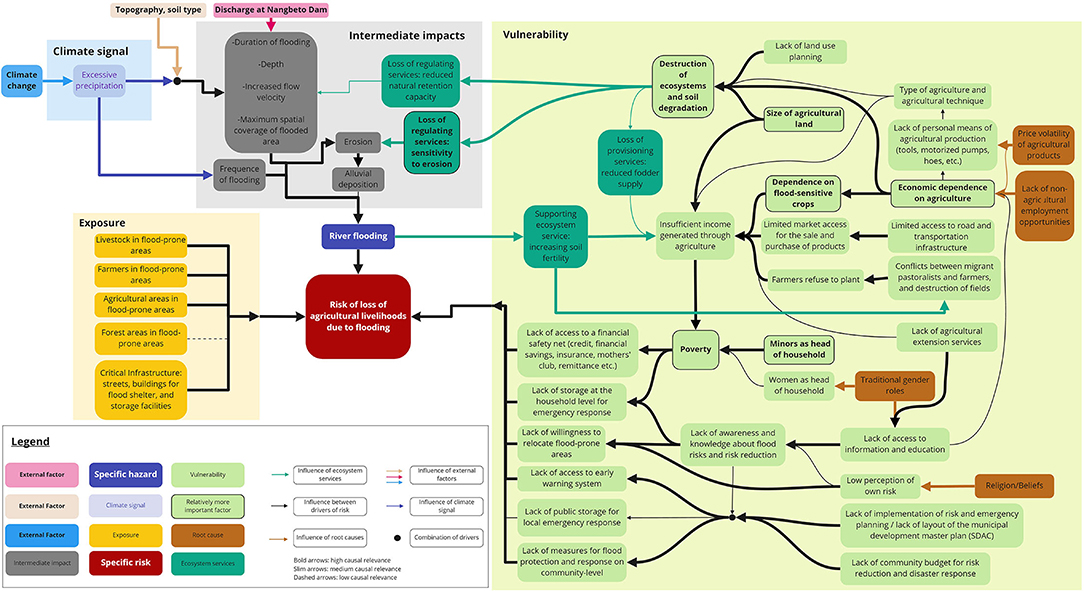
Figure 3. Validated impact chain. Bold arrows: high causal influence; thin arrows: medium causal influence; dashed arrows: low causal influence. For high resolution image refer to Supplementary Figure 2.
The hazard component is influenced by excessive precipitation (climate signal), which in turn is influenced by climate change (Niang et al., 2014). During expert consultation, participants mentioned that especially changing seasonality of extreme rainfall contributes to increased uncertainty regarding the temporal distribution of Mono River floods. The dynamic combination of excessive precipitation with the external drivers of soil type and topography are considered strong explanatory variables for determining the duration of flooding, flood depth, flow velocity and maximum spatial coverage of the flood by workshop participants. Workshop participants also perceived a high influence of discharge at Nangbeto Dam on flood duration, depth, velocity, and spatial extent. The influence of loss of natural retention capacity for explaining the related drivers of duration, flood depth, flow velocity and flood extent was considered less relevant by stakeholders. In contrast, participants identified flood duration, frequency, depth, velocity, and spatial extent as relevant characteristics of river flooding as well as drivers of erosion. Erosion was highlighted as a crucial factor explaining alluvial deposition (or sedimentation), but alluvial deposition was identified as a less relevant driver for the overall flood hazard. The ecosystem's sensitivity to erosion was considered particularly relevant in the context of intermediate impacts.
Due to the focus on agricultural livelihoods, livestock, agricultural areas, and agriculture-dependent populations located in potentially flood-prone areas were considered as exposed elements. Further, critical infrastructures were also identified as a relevant exposed element, including streets, buildings used as flood shelters as well as storage facilities (mainly for agricultural produce).
In the context of vulnerability, drivers which were considered particularly relevant by stakeholders are “destruction of ecosystems and soil degradation,” “size of agricultural land,” “dependence on flood sensitive crops,” “economic dependence on agriculture,” “poverty,” and “minors as head of household.” Economic dependence on agriculture (see section Study Area) was identified as a crucial driver of vulnerability which is influenced by the lack of non-agricultural employment opportunities. The economic dependence on agriculture is considered a cause for the dependence on flood-sensitive crops. Furthermore, stakeholders highlighted that most cultivated products (see section Impact Chain Development and Participatory Impact Chain Validation) are flood sensitive. Though rice paddies and young palm trees are considered less sensitive to flooding by stakeholders, workshop participants mentioned that flood intensity regularly exceeds even their tolerance level, frequently resulting in crop failure. This dependency on flood-sensitive crops leads to a reduction of agricultural income following flood events.
Additionally, strong causality between economic dependence on agriculture and the destruction of ecosystems and soil degradation was identified. The destruction of ecosystems and soil degradation is also driven by the type of agriculture and the agricultural techniques. Following stakeholder opinion, a large size of agricultural land has high causal relevance for ecosystem destruction and soil degradation since more land is converted from its original vegetation to farmland. The destruction of ecosystems and soil degradation is further driven by a lack of land-use planning that may lead to e.g., inappropriate agricultural practices. Moreover, stakeholders identified reduced fodder supply [loss of provisioning ecosystem services (ESS)] as an effect of the destruction of ecosystems and soil degradation, but also as a cause for insufficient income generated through agriculture. Furthermore, insufficient income generated through agriculture is influenced by the size of agricultural land available for farming households and by limited access to markets based on road and transport infrastructure.
Further, poverty was identified as a particularly relevant driver of vulnerability; strongly influenced by insufficient income generated through agriculture. Furthermore, poverty is driven by the gender and age of the head of household. It was highlighted that households headed by minors are especially susceptible to poverty. Moreover, poverty is evaluated by stakeholders as an important explanatory variable for the lack of access to financial safety nets. Poverty is considered a strong causal driver for the lack of storage for emergency response at the household level. This refers to the provision of food and other essential goods after a flood event. In addition, the lack of storage at the household level is strongly driven by a lack of awareness and knowledge about flood risks and risk reduction.
In this context, risk perception and religion, especially traditional beliefs, need to be accounted for. Experts and stakeholders mentioned that traditional beliefs are dominant in the study area and the Mono River is commonly considered divine. Hence, numerous places adjacent to the river are of high spiritual importance for the local population leading to a strong attachment to the ancestral land. Based on these traditional views, river floods and their impacts are frequently believed to be an act of gods rather than a socio-environmental process that can be governed. Regarding risk governance, various drivers are mentioned, such as the lack of access to early warning systems as well as the lack of measures for flood protection and response at the community level. However, stakeholders have not attributed higher relevance to drivers related to risk governance.
What-If Scenarios in Alpha-Level Bayesian Network
The exemplary BN scenario visualizes the dynamic interactions between the high dependence on flood-sensitive crops and other drivers of vulnerability as well as the resulting flood risk for agricultural livelihoods.
The first scenario illustrates an assumed baseline condition (due to the lack of quantitative data) regarding economic dependence on agriculture and dependence on flood-sensitive crops in the region. Based on the authors knowledge of the study areas the economic dependence on agriculture is considered high. This was also further confirmed in the stakeholder workshops.
Figure 4 visualizes the drivers influenced both directly and indirectly by high dependence of the population on flood-sensitive crops. The dependence on flood-sensitive crops directly affects the auxiliary node “combined effects: vulnerability based on farm size, dependence on flood-sensitive crops, and market access.” The auxiliary node indicates a change toward higher vulnerability. Given the assumption that the cause of change in the auxiliary node is known (high dependence on flood-sensitive crops) the other potential causes (market access and farm size) remain unaffected. This increase in the probability of the presence of adverse conditions is further passed through the network. Consequently, leading to a higher probability of insufficient income generated through agriculture. As a result, the likelihood of high poverty levels increases as well.
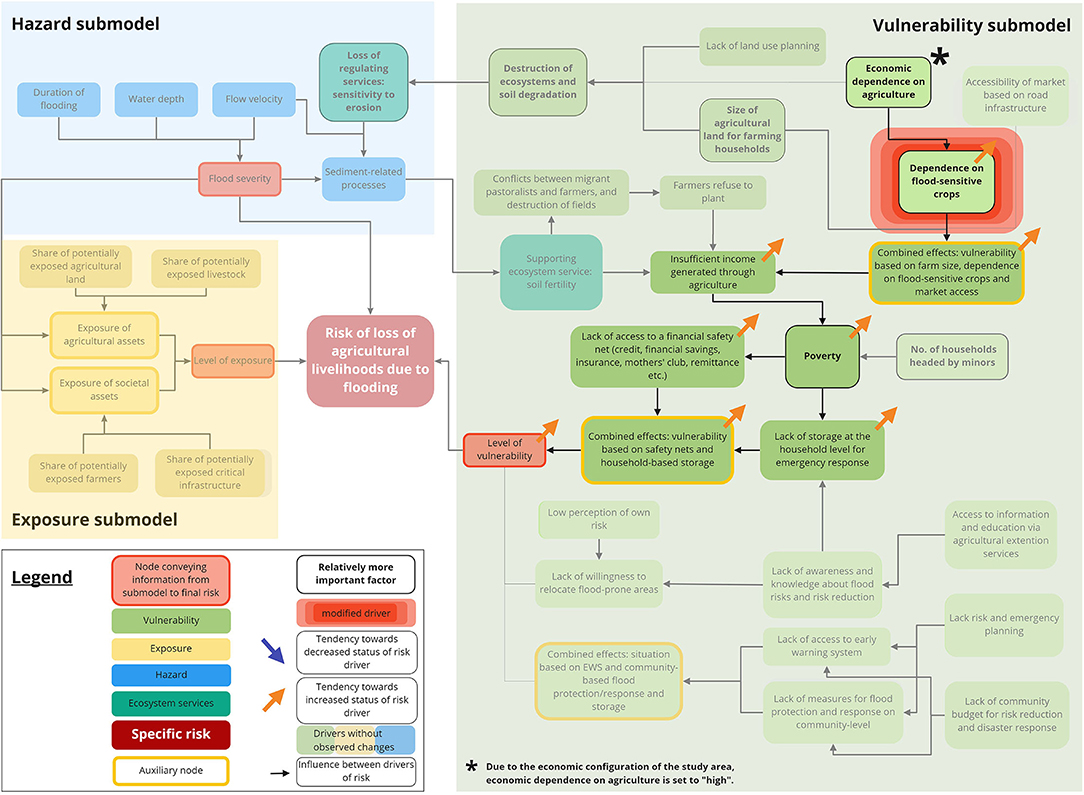
Figure 4. First illustrative scenario depicting the system behavior if dependence on flood-sensitive crops is observed to be high.
As a result of high poverty levels, a lack of access to financial safety nets as well as lack of storage at the household level for emergency response become more likely. Both, access to safety nets and household-based storage feed into the auxiliary node “combined effects: vulnerability based on safety nets and household-based storage.” Ultimately, the auxiliary node directly affects the overall level of vulnerability, suggesting an increased probability of observing higher vulnerability of agricultural livelihoods. While the overall level of vulnerability is altered through the high dependency on flood-sensitive crops, the change is not strong enough to increase overall risk (Figure 4).
The second scenario illustrates a simplified example of how the effects of adaptation options (here: reducing dependency on flood-sensitive crops while not changing high economic dependency on agriculture, e.g., through the introduction of new, more resilient crop types) can be simulated using a what-if scenario.
Figure 5 shows that the same drivers within the vulnerability submodel are affected, yet, in the opposite direction. Reduced dependency on flood sensitive crops (from high to low) leads to reduced loss of income in times of floods and therefore a reduced likelihood to encounter high levels of poverty. A decrease in the potential for high poverty increases the probability that financial safety nets are accessible and household-based storage for emergency response may be available. Ultimately, the change in dependence on flood-sensitive crops translates into a decreased likelihood of high overall vulnerability. In contrast to the first scenario, a change in the overall risk is observed for the second scenario. Knowing that the dependence on agriculture is high, certainty regarding the dependence on flood-sensitive crops is not sufficient to assume an increased overall risk, certainty regarding the independence of flood-sensitive crops, however, leads to a higher probability of lower overall risk.
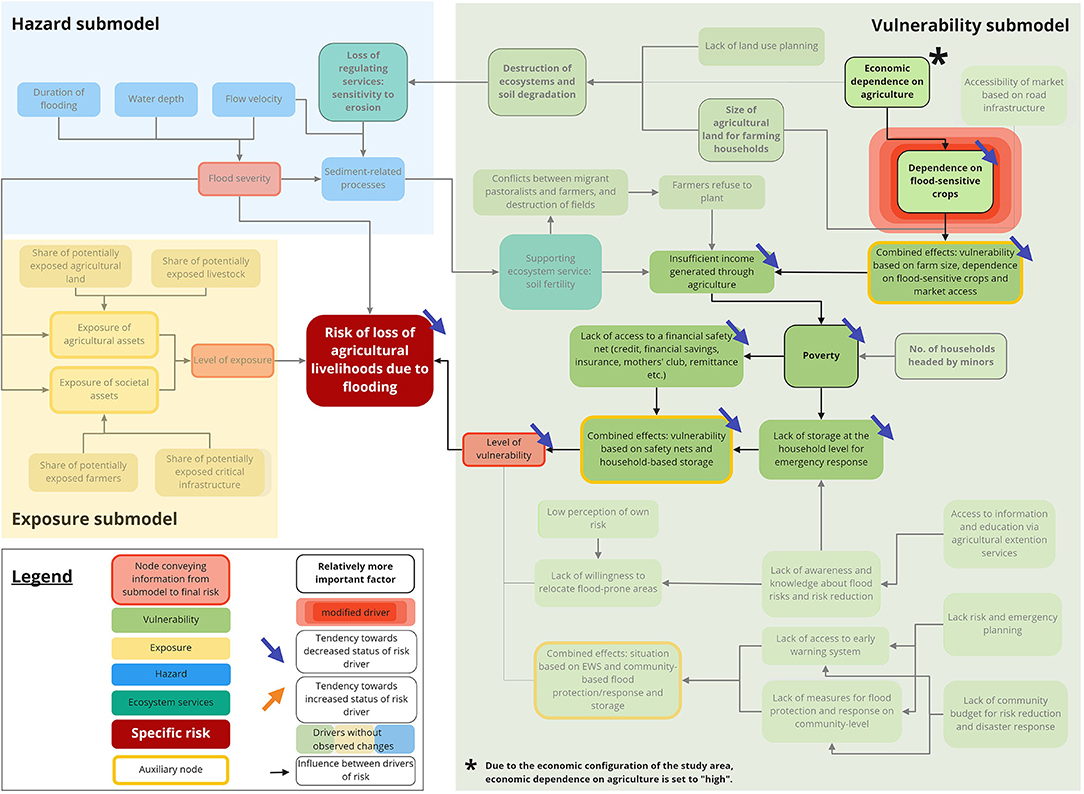
Figure 5. Second illustrative scenario depicting the system behavior if dependence on flood-sensitive crops is observed to be low.
Discussion
Strengths and Weaknesses of the Methodological Approach
The study shows that the chosen approach of combining impact chains and BN modeling enables identifying key drivers of flood risk as well as their interlinkages. The validated impact chain has proven to be a valuable model approach to represent the complexity of flood risk and associated relationships between risk drivers in the study area. The participatory integration of stakeholders is a widely acknowledged approach to create more robust results through the inclusion of multiple points of view and experiences (Döll and Romero-Lankao, 2017; Nyumba et al., 2018; Hagenlocher et al., 2020). Especially in environments where quantitative data is scarce, such as in Benin, stakeholder participation is a valuable source of information. In fact, during the stakeholder workshops, no explicit questions were asked regarding spatio-temporal dynamics associated with risk drivers. However, upon discussing inter-driver relationships stakeholders reflected on spatio-temporal dynamics, such as e.g., temporary migratory movements into the region. These movements have been associated by workshop participants to a temporal axis based on the occurrence of flood events and increased soil fertility as a result thereof as well as intra-annual seasonality. The impact chain reflects on these dynamics by indicating interlinkages but does not explicitly specify time-steps.
The application of an impact chain as a conceptual model approach provides multiple benefits. Impact chains can be developed (i) without quantitative data and (ii) in a participatory approach benefiting from local and expert knowledge. Additionally, (iii) inter-driver relationships as well as (iv) relationships among subcomponents of risk can be visualized which enables a process of (v) weighting and evaluation of differentiated relevance of drivers and their interlinkages. Furthermore, (vi) direct feedback loops as well as (vii) root causes of risk drivers can be incorporated into the impact chain. Particularly the latter is crucial for effective risk reduction and adaptation since the understanding of root causes enables addressing the underlying issue(s) instead of targeting superficial symptoms (Wisner et al., 2004; Ribot, 2011; Eriksen et al., 2021). However, impact chains are also subject to limitations. The cause-effect relationship may suggest (i) linearity and (ii) deterministic relationships among risk drivers which potentially neglects non-linear and stochastic interactions. Also, impact chains do not (iii) specify uncertainty, nor do they account explicitly for (iv) spatial and temporal configurations of the system which is a considerable limitation when supporting decision-making for risk reduction and adaptation.
To test if BN can further improve the understanding of interlinkages and dynamic interactions of risk drivers, a qualitative BN was developed based on the impact chain. The BN was applied to visualize how an exemplary what-if scenario may reveal hidden dependencies and interactions among risk drivers. Based on the assumption that a system is more likely to change its potential states than its underlying rules of cause-and-effect, the logical structure of the network is more decisive for model quality than the actual quantitative value of parameters (Pearl and Mackenzie, 2018). Thus, particularly in the absence of reliable quantitative data, a sound conceptual model of the underlying system is crucial (Marcot et al., 2006; Nyberg et al., 2006; Chen and Pollino, 2012). Here, the multi-method approach for impact chain development and participatory validation provided this sound conceptual model of the relevant system. The assessment has shown that a BN in combination with an impact chain provides multiple benefits for assessing risk dynamics in data-scarce environments. This study supports the argument that the ability of BNs to integrate qualitative data enables their application in data-scarce environments and the inclusion of stakeholder/expert knowledge (Table 2). The similar graphical structure of cause-and-effect of BNs and the impact chain further facilitates the integration of the two methods and enables easy understanding of the logical structure (Table 2).
Moreover, the exemplary scenario has shown that BNs are able to explore system behavior under varying conditions. This potential for scenario assessments and the opportunity to quickly update individual model components is particularly interesting for decision-making under uncertainty (Table 2). The use of probability values to reflect on system behavior enables BNs to account transparently and consistently for uncertainty in a system (Table 2). Especially, the reflection of uncertainty in participatory processes e.g., due to ambiguity among stakeholders regarding specific drivers and their interactions via probability values is a benefit (Table 2). The general potential of continuous updating of probabilities to adjust the level of uncertainty and the ability to evaluate decision-outcomes are core strengths of BNs. Even though not considered here due to the scope of this study, options to integrate time-steps in a BN or to integrate BNs into GIS software exist to improve spatial and temporal representation (Stritih et al., 2020; Norsys Software Corp., 2020. Also, model complexity can be reduced by integrating other models into the BN structure, as shown in this study with the structural adjustment of the hazard-submodel to the hydrodynamic model elaborated in the context of the CLIMAFRI project (Table 2).
Nevertheless, BNs are subject to a variety of limitations. The validated impact chain contains a high level of detail, e.g., including root causes of vulnerability and climate change. On the one hand, this degree of detail enables a comprehensive understanding of the system under scrutiny. On the other hand, a high level of complexity poses specific challenges for the transition from impact chain to BN. To deal with complexity, a structured approach for simplification was applied. Yet, the BN still consisted of a large number of individual parameters increasing the potential for wrong assumptions and decreasing the feasibility to manually assign parameter values (Table 3). Another critical factor is the depth of submodels and their asymmetry. The term “model depth” refers to the number of causal connections between input and end node. If the submodels differ in depth, the models are asymmetric regarding the length of their causal chains. In this case, a considerable level of asymmetry can be noted between the subcomponents of risk and vulnerability. This results in a higher sensitivity of the final risk to hazard components, restricting the potential to compare e.g., the influence of vulnerability and hazard drivers on the final risk outcome (Table 3). These limitations become evident in the context of root causes e.g., as depicted for vulnerability in the impact chain. Due to the causal distance of root causes, their impact on the final risk is very limited in this exemplary BN. Therefore, combined with the need to simplify the model to keep parameter elicitation feasible, root causes were discarded. In fact, this is a considerable limitation given that root causes (e.g., of vulnerability) are a key to sustainable adaptation and risk reduction.
Unlike dealing with uncertainty, accounting for dynamics is not at the core of BN capacities. Even though integrating indirect feedback between different subcomponents of risk is possible and beneficial for exploring dynamic interactions between e.g., vulnerability and hazard components, direct feedback loops cannot be incorporated into a BN. Due to the graphical structure of a DAG and the computational implications of Bayesian updating, dynamics associated with direct feedback loops cannot be processed by a BN which is a major limitation when dealing with bi-directional dynamics (Table 3). Additionally, there are limits to the amount of spatial and temporal dynamics that can be depicted in one BN. This is also due to the rapid growth of CPTs in complex models and the resulting large size of possible interactions restricting the ease of updating parameters (Table 3). Thus, the time-consuming manual evaluation and refinement of CPTs may potentially limit the usability of complex BNs for qualitative and participatory model building (Table 3).
Implications of Findings
The integration of impact chain and BN methods are a promising starting point for assessing risk dynamics in data-scarce environments. Even though reliable data in the research area regarding dynamics of flood risk for agricultural livelihoods is limited, key drivers and their interactions were identified. The qualitative BN and its application to an illustrative what-if scenario enabled a visual evaluation of related system components and their behavior if case-specific information becomes available. However, integrating an impact chain (even though simplified) into a BN without quantitative data has limitations. A high level of detail and asymmetric structure in the impact chain and BN may lead to a time-consuming process of manual parameter elicitation and distortion regarding the sensitivity of individual submodels. This adversely affects the feasibility of model creation and reliability of information concerning the final risk. The application of multiple smaller BN models could be a solution when dealing with large complex systems, or for assessing adaptation impacts e.g., on root causes. Still, without quantitative validation the robustness of assigned parameters is limited. For future work, we suggest a validation process similar to Marcot et al. (2006) consisting of an iterative process of testing and validating the model against quantitative data, if available. In addition, we recommend evaluating the impact chain again, after BN modeling, to identify potential inconsistencies in the system representation and logical interaction of risk drivers. However, first qualitative assessments regarding potential directions of change within the system are possible without explicitly quantifying the level of change. The ability to detect changes and hidden interdependencies within the system is a valuable advantage to identify further research and data priorities as well as potential entry points for adaptation planning.
Moreover, the methodology may be used in combination with more traditional data driven risk assessments. As this article uses a similar set of risk drivers as used for the indicator-based assessment provided by Schudel et al. (forthcoming), insights on dynamic interactions within the system can be translated to the risk index developed by Schudel et al. (forthcoming). Particularly, hidden interdependencies and directions of change become visible in the BN and can be integrated into index development. Traditionally, upon receiving information for a particular indicator, only the specific indicator would be adjusted for the index. Now, a whole set of directly or indirectly dependent indicators can be adjusted toward a certain direction, potentially enabling a more precise representation of the actual system's risk.
Even though this study has focused on flood risk for agricultural livelihoods, the approach can be transferred to other contexts as well. Hence, the integration of the two approaches should further be tested to assess risk dynamics and adaptation outcomes for different perils (e.g., droughts or hurricanes) and contexts, such as, for example, urban or coastal environments.
Conclusion
Risk assessment approaches that focus on understanding and displaying the dynamic nature of flood risk and its underlying drivers are limited. This article addresses this gap of specific flood risk drivers and their interactions related to agricultural livelihoods in the Lower Mono River Basin. Moreover, the study contributes to the broader scientific discourse by illustrating a structured approach of integrating an impact chain with BN modeling as well as discussing the potential benefits and limitations of the methodology. In summary, the impact chain provides valuable insights on the key drivers and their interlinkages that co-produce the specific risk in the study area. Additionally, the exemplary BN was able to illustrate the potential of assessing what-if scenarios related to flood risk dynamics. The results obtained through the exemplary scenario modeling may inform a first assessment of how drivers of risk are modified under changing conditions, including an overview of affected components of risk as well as the potential direction of change. Hence, the impact chain can provide a starting point for further assessments of relevant key drivers and their interlinkages e.g., for the transboundary Mono River Basin, including Togo. Moreover, the impact chain may also be a useful tool to identify and locate adaptation options to reduce flood risk for agricultural livelihoods within the system. The combination of the impact chain with the exemplary BN suggests a considerable potential to inform indicator-based risk assessments as well. As new information regarding the state of one driver becomes available, the BN allows identifying other potentially affected components of risk and their direction of change. This information may be applied to update a risk index, allowing to account for dynamics in an otherwise static representation of risk. Ultimately, the article suggests that the integration of the impact chain methodology with BN modeling is a promising approach toward an improved representation of flood risk dynamics, especially in the absence of reliable quantitative data. However, its particular potential for informing more static, e.g., indicator-based assessments needs further research, evidence and validation.
Data Availability Statement
The original contributions presented in the study are included in the article/Supplementary Material, further inquiries can be directed to the corresponding author.
Author Contributions
MW and MH designed the concept for the analysis. MH and YW drafted the initial impact chain following a field visit to the region before the pandemic. MW conducted the literature review and expert consultations, revised the impact chain with the support of AA, LS, and YW, developed the alpha-level BN with the support of AA under the supervision of MH, and drafted the manuscript. LS, YW, MW, KK, and JA organized and facilitated the stakeholder workshops. All authors have contributed to the interpretation of the results, made substantial contributions to the manuscript, and approved the final manuscript.
Funding
This research has received funding through the CLIMAFRI project (Grant No. FKZ: 01 LZ 1710 A-E) funded by the German Federal Ministry of Education and Research (BMBF).
Conflict of Interest
The authors declare that the research was conducted in the absence of any commercial or financial relationships that could be construed as a potential conflict of interest.
Publisher's Note
All claims expressed in this article are solely those of the authors and do not necessarily represent those of their affiliated organizations, or those of the publisher, the editors and the reviewers. Any product that may be evaluated in this article, or claim that may be made by its manufacturer, is not guaranteed or endorsed by the publisher.
Acknowledgments
The authors would like to thank the participants in the expert consultations and stakeholder workshops for the valuable inputs, contributions and feedback. Further, we would like to thank the three reviewers for the constructive comments and Caitlyn Eberle for proofreading.
Supplementary Material
The Supplementary Material for this article can be found online at: https://www.frontiersin.org/articles/10.3389/frwa.2022.837688/full#supplementary-material
References
Abdulkareem, S. A., Mustafa, Y. T., Augustijn, E.-W., and Filatova, T. (2019). Bayesian networks for spatial learning: a workflow on using limited survey data for intelligent learning in spatial agent-based models. GeoInformatica 23, 243–268. doi: 10.1007/s10707-019-00347-0
Abebe, Y., Kabir, G., and Tesfamariam, S. (2018). Assessing urban areas vulnerability to pluvial flooding using GIS applications and Bayesian Belief Network model. J. Cleaner Prod. 174, 1629–1641. doi: 10.1016/j.jclepro.2017.11.066
Adger, W. N., Brown, I., and Surminski, S. (2018). Advances in risk assessment for climate change adaptation policy. Philos. Trans. A Math. Phys. Eng. Sci. 376, 1–13. doi: 10.1098/rsta.2018.0106
Agboola, O. A., Downs, C. T., and O'Brien, G. (2020). Ecological Risk of water resource use to the wellbeing of macroinvertebrate communities in the rivers of KwaZulu-Natal, South Africa. Front. Water 2:584936. doi: 10.3389/frwa.2020.584936
Ago, E. E., Petit, F., and Ozer, P. (2005). Analyse des inondations en aval du barrage de Nangbeto sur le fleuve Mono (Togo et au Bénin). Geo-Eco-Trop 29, 1–14. Available online at: http://hdl.handle.net/2268/17768
Almoradie, A., and Houngue, R. (2021). Image of 10 Year Return Period Flood Depth in the Lower Mono River Basin. Available online at: http://ewa.uni-bonn.de/projects/climafri/BC_Depth_HQ10.png (accessed December 15, 2021).
Apel, H., Aronica, G. T., Kreibich, H., and Thieken, A. H. (2009). Flood risk analyses—how detailed do we need to be? Nat Hazards 49, 79–98. doi: 10.1007/s11069-008-9277-8
Bagstad, K. J., Johnson, G. W., Voigt, B., and Villa, F. (2013). Spatial dynamics of ecosystem service flows: a comprehensive approach to quantifying actual services. Ecosyst. Serv. 4, 117–125. doi: 10.1016/j.ecoser.2012.07.012
Balbi, S., Villa, F., Mojtahed, V., Hegetschweiler, K. T., and Giupponi, C. (2016). A spatial Bayesian network model to assess the benefits of early warning for urban flood risk to people. Nat. Hazards Earth Syst. Sci. 16, 1323–1337. doi: 10.5194/nhess-16-1323-2016
Baudoin, M.-A. (2014). Enhancing climate change adaptation in Africa assessing the role of local institutions in Southern Benin. Clim. Dev. 6, 122–131. doi: 10.1080/17565529.2013.844677
BayesFusion, L. (2020). GeNIe Modeler: Complete Modeling Freedom. Available online at: https://www.bayesfusion.com/genie/ (accessed August 12, 2020)
Cain, J. (2001). Planning Improvements in Natural Resources Management: Guidelines for Using Bayesian Networks to Support the Planning and Management of Development Programmes in the Water Sector and Beyond. Oxon: Centre for Ecology & Hydrology.
Cains, M. G., and Henshel, D. (2019). Community as an equal partner for region-based climate change vulnerability, risk, and resilience assessments. Curr. Opin. Environ. Sustain. 39, 24–30. doi: 10.1016/j.cosust.2019.06.005
CENATEL - Centre National de Télédétection et de Suivi Ecologique (2015). Land Use in Benin, Based on Sentinel 2 and High Resolution Aerials. Cotonou: CENATEL.
Chen, S. H., and Pollino, C. A. (2012). Good practice in Bayesian network modelling. Environ. Model. Softw. 37, 134–145. doi: 10.1016/j.envsoft.2012.03.012
De Brito, M., Almoradie, A., and Evers, M. (2019). Spatially-explicit sensitivity and uncertainty analysis in a MCDA-based flood vulnerability model. Int. J. Geogr. Inf. Sci. 33, 1788–1806. doi: 10.1080/13658816.2019.1599125
de Moel, H., Jongman, B., Kreibich, H., Merz, B., and Penning-Rowsell, Ward, P. (2015). Flood risk assessments at different spatial scales. Mitig. Adapt. Strateg. Glob. Change 20, 865–890. doi: 10.1007/s11027-015-9654-z
Di Baldassarre, G., Brandimarte, L., and Beven, K. (2016). The seventh facet of uncertainty: wrong assumptions, unknowns and surprises in the dynamics of human–water systems. Hydrol. Sci. J. 61, 1748–1758. doi: 10.1080/02626667.2015.1091460
Di Baldassarre, G., Kemerink, J. S., Kooy, M., and Brandimarte, L. (2014). Floods and societies: the spatial distribution of water-related disaster risk and its dynamics. WIREs Water 1, 133–139. doi: 10.1002/wat2.1015
Di Baldassarre, G., Sivapalan, M., Rusca, M., Cudennec, C., Garcia, M., Kreibich, H., et al. (2019). Sociohydrology: scientific challenges in addressing the sustainable development goals. Water Resour. Res. 55, 6327–6355. doi: 10.1029/2018WR023901
Díez-Herrero, A., and Garrote, J. (2020). Flood risk assessments: applications and uncertainties. Water 12, 2096. doi: 10.3390/w12082096
Döll, P., and Romero-Lankao, P. (2017). How to embrace uncertainty in participatory climate change risk management-A roadmap. Earth's Future 5, 18–36. doi: 10.1002/2016EF000411
Dottori, F., Szewczyk, W., Ciscar, J.-C., Zhao, F., Alfieri, L., Hirabayashi, Y., et al. (2018). Increased human and economic losses from river flooding with anthropogenic warming. Nat. Clim. Change 8, 781–786. doi: 10.1038/s41558-018-0257-z
Duggan, J. M., Eichelberger, B. A., Ma, S., Lawler, J. J., and Ziv, G. (2015). Informing management of rare species with an approach combining scenario modeling and spatially explicit risk assessment. Ecosyst. Health Sustain. 1, 1–18. doi: 10.1890/EHS14-0009.1
Emmanuel, A. L., Batablinlè, L., Célestin, M., and Hodabalo, K. (2019). Future extremes temperature: trends and changes assessment over the Mono River Basin, Togo (West Africa). JWARP 11, 82–98. doi: 10.4236/jwarp.2019.111006
Eriksen, S., Schipper, E. L. F., Scoville-Simonds, M., Vincent, K., Adam, H. A., Brooks, N., et al. (2021). Adaptation interventions and their effect on vulnerability in developing countries: Help, hindrance or irrelevance? World Dev. 141:105383. doi: 10.1016/j.worlddev.2020.105383
ESA - European Space Agency (n. d.). Cover Map 201. Available online at: http://maps.elie.ucl.ac.be/CCI/viewer/ (accessed January 09, 2021)..
Fritzsche, K., Schneiderbauer, S., Bubeck, P., Kienberger, S., Buth, M., Zebisch, M., et al. (2014). The Vulnerability Sourcebook: Concept and Guidelines for Standardised Vulnerability Assessments. Available online at: https://www.adaptationcommunity.net/download/va/vulnerability-guides-manuals-reports/vuln_source_2017_EN.pdf
Graham, S. E., Chariton, A. A., and Landis, W. G. (2019). Using Bayesian networks to predict risk to estuary water quality and patterns of benthic environmental DNA in Queensland. Integr. Environ. Assess. Manag. 15, 93–111. doi: 10.1002/ieam.4091
Hagenlocher, M., Schneiderbauer, S., Sebesvari, Z., Bertram, M., Renner, K., Renaud, F. G., et al. (2018). Climate Risk Assessment for Ecosystem-Based Adaptation: A Guidebook for Planners and Practitioners. Available online at: https://www.adaptationcommunity.net/wp-content/uploads/2018/06/giz-eurac-unu-2018-en-guidebook-climate-risk-asessment-eba.pdf
Hagenlocher, M., Thieken, A., Schneiderbauer, S., Aguirre Ayerbe, I., Dobes, P., Donovan, A., et al. (2020). “Risk Assessment,” in Science for Disaster Risk Management 2020: Acting Today, Protecting Tomorrow, eds A. Casajus Valles, M. Marin Ferrer, K. Poljanšek, and I. Clark (Luxembourg: Publications Office of the European Union), 54–65.
Harris, M. J., Stinson, J., and Landis, W. G. (2017). A Bayesian approach to integrated ecological and human health risk assessment for the South River, Virginia Mercury-Contaminated Site. Risk Anal. 37, 1341–1357. doi: 10.1111/risa.12691
Herring, C. E., Stinson, J., and Landis, W. G. (2015). Evaluating nonindigenous species management in a Bayesian networks derived relative risk framework for Padilla Bay, WA, USA. Integr. Environ. Assess. Manag. 11, 640–652. doi: 10.1002/ieam.1643
Hirabayashi, Y., Mahendran, R., Koirala, S., Konoshima, L., Yamazaki, D., Watanabe, S., et al. (2013). Global flood risk under climate change. Nat. Clim. Change 3, 816–821. doi: 10.1038/nclimate1911
Höllermann, B. (2018). Decision-making under uncertainty in model-based water management: The science-practice interface. (PhD thesis). University of Bonn, Bonn, Germany.
Höllermann, B., and Evers, M. (2015). Integration of uncertainties in water and flood risk management. Proc. IAHS 370, 193–199. doi: 10.5194/piahs-370-193-2015
Höllermann, B., and Evers, M. (2019). Coping with uncertainty in water management: Qualitative system analysis as a vehicle to visualize the plurality of practitioners' uncertainty handling routines. J. Environ. Manage 235, 213–223. doi: 10.1016/j.jenvman.2019.01.034
ILO - International Labour Organization (2019). Employment Distribution by Economic Activity (by Sex and Rural/Urban Areas). ILO Modelled Estimates. Available online at: https://ilostat.ilo.org/data/ (accessed August, 12, 2020).
INSAE - Institut National de la Statistique et de l'Analyse Economique (2016a). Cahier des villages et quartiers de ville du departement du Cuoffo et du Mono. RGPH-4, 2013. Cotonou: INSAE.
INSAE - Institut National de la Statistique et de l'Analyse Economique (2016b). Principaux Indicateurs Socio Demographiques et Economiques du Departement du Mono. RGPH-4, 2013. Cotonou: INSAE.
NSEED - Institut National de la Statistique et des Etudes Economiques et Démographiques et AFRISTAT (2019). Enqu Régionale Intégrée sur l'Emploi et le Secteur Informel, 2017 : Rapport final. Lomé, Togo et Bamako, Mali.
Intergovernmental Panel on Climate Change (2021). “Summary for policymakers,” in Climate Change 2021: The Physical Science Basis: Contribution of Working Group I to the Sixth Assessment Report of the Intergovernmental Panel on Climate Change, eds V. Masson-Delmotte, P. Zhai, A. Pirani, S. L. Connors, C. Péan, S. Berger, et al. (Cambridge: Cambridge University Press). (In Press) Preprint. 1–41.
IPCC - Intergovernmental Panel on Climate Change (2014). AR5 Climate Change 2014: Impacts, Adaptation, and Vulnerability. Part A: Global and Sectoral Aspects. Contribution of Working Group II to the Fifth Assessment Report of the Intergovernmental Panel on Climate Change. Cambridge; New York, NY: Cambridge University Press. doi: 10.1017/CBO9781107415416
Jakeman, A. J., Letcher, R. A., and Norton, J. P. (2006). Ten iterative steps in development and evaluation of environmental models. Environ. Model. Softw. 21, 602–614. doi: 10.1016/j.envsoft.2006.01.004
Johns, A. F., Graham, S. E., Harris, M. J., Markiewicz, A. J., Stinson, J. M., and Landis, W. G. (2017). Using the Bayesian network relative risk model risk assessment process to evaluate management alternatives for the South River and upper Shenandoah River, Virginia. Integr. Environ. Assess. Manag. 13, 100–114. doi: 10.1002/ieam.1765
Jongman, B., Ward, P. J., and Aerts, J. C. (2012). Global exposure to river and coastal flooding: long term trends and changes. Global Environ. Change 22, 823–835. doi: 10.1016/j.gloenvcha.2012.07.004
Jung, M.-K., Kim, J.-Y., Lee, B., and Kwon, H.-H. (2021). Exploring the combined risk of sea level rise and storm surges using a Bayesian Network Model: application to saemangeum seawall. J. Coast. Res. 114, 186–190. doi: 10.2112/JCR-SI114-038.1
Jurgilevich, A., Räsänen, A., Groundstroem, F., and Juhola, S. (2017). A systematic review of dynamics in climate risk and vulnerability assessments. Environ. Res. Lett. 12, 1–15. doi: 10.1088/1748-9326/aa5508
Kissi, A. E., Abbey, G. A., Agboka, K., and Egbendewe, A. (2015). Quantitative assessment of vulnerability to flood hazards in downstream area of Mono Basin, South-Eastern Togo: Yoto District. JGIS 07, 607–619. doi: 10.4236/jgis.2015.76049
Kragt, M. E. (2009). A Beginners Guide to Bayesian Network Modelling for Integrated Catchment Management. Technical Report No. 9. Hobart: Landscape Logic. Available online at: https://www.utas.edu.au/__data/assets/pdf_file/0011/588467/TR_9_BNs_for_Integrated_Catchment_Management.pdf
Lamboni, B., Emmanuel, L. A., Manirakiza, C., and Djibib, Z. M. (2019). Variability of Future Rainfall over the Mono River Basin of West-Africa. AJCC 08, 137–155. doi: 10.4236/ajcc.2019.81008
Lauritzen, S. L., and Spiegelhalter, D. J. (1988). Local computations with probabilities on graphical structures and their application to expert systems. J. R. Stat. Soc. B (Methodological) 50, 157–224. doi: 10.1111/j.2517-6161.1988.tb01721.x
Marcot, B. G., Steventon, J. D., Sutherland, G. D., and McCann, R. K. (2006). Guidelines for developing and updating Bayesian belief networks applied to ecological modeling and conservation. Can. J. For. Res. 36, 3063–3074. doi: 10.1139/x06-135
Maskrey, S. A., Mount, N. J., and Thorne, C. R. (2021). Doing flood risk modelling differently: evaluating the potential for participatory techniques to broaden flood risk management decision-making. J Flood Risk Manag. 15, e12757. doi: 10.1111/jfr3.12757
Maskrey, S. A., Mount, N. J., Thorne, C. R., and Dryden, I. (2016). Participatory modelling for stakeholder involvement in the development of flood risk management intervention options. Environ. Model. Softw. 82, 275–294. doi: 10.1016/j.envsoft,.2016.04.027
MEDDPN - Ministry of Environment and Forest Resources (2015). Land Use in Togo, Based on Rapid Eye. Lomé: MEDDPN.
Merz, B., Aerts, J., Arnbjerg-Nielsen, K., Baldi, M., Becker, A., Bichet, A., et al. (2014). Floods and climate: emerging perspectives for flood risk assessment and management. Nat. Hazards Earth Syst. Sci. 14, 1921–1942. doi: 10.5194/nhess-14-1921-2014
Michaelis, T., Brandimarte, L., and Mazzoleni, M. (2020). Capturing flood-risk dynamics with a coupled agent-based and hydraulic modelling framework. Hydrol. Sci. J. 65, 1458–1473. doi: 10.1080/02626667.2020.1750617
Neil, M., Fenton, N., Osman, M., and Lagnado, D. (2019). Causality, the critical but often ignored component guiding us through a world of uncertainties in risk assessment. J. Risk Res. 16, 1–5. doi: 10.1080/13669877.2019.1604564
Nguyen, M. T., Sebesvari, Z., Souvignet, M., Bachofer, F., Braun, A., Garschagen, M., et al. (2021). Understanding and assessing flood risk in Vietnam: current status, persisting gaps, and future di rections. J. Flood Risk Manag. 14:e12689. doi: 10.1111/jfr3.12689
Nguyen, V. D., Metin, A. D., Alfieri, L., Vorogushyn, S., and Merz, B. (2020). Biases in national and continental flood risk assessments by ignoring spatial dependence. Sci. Rep. 10, 1–8. doi: 10.1038/s41598-020-76523-2
Niang, I., Ruppel, O. C., Abdrabo, M. A., Essel, A., Lennard, C., Padgham, J., et al. (2014). “Africa,” in AR5 Climate Change 2014: Impacts, Adaptation, and Vulnerability. Part B: Regional Aspects. Contribution of Working Group II to the Fifth Assessment Report of the Intergovernmental Panel on Climate Change, eds V. R. Barros, C. B. Field, D. J. Dokken, M. D. Mastrandrea, K. J. Mach, T. E. Bilir, et al. (Cambridge; New York, NY: Cambridge University Press), 1199–1265.
Nicholson, S. E., Fink, A. H., Funk, C., Klotter, D. A., and Satheesh, A. R. (2021). Meteorological causes of the catastrophic rains of October/November 2019 in equatorial Africa. Global Planet. Change 208, 103687. doi: 10.1016/j.gloplacha.2021.103687
Netica Application. Available online at: https://www.norsys.com/netica.html (accessed August 12, 2020)..
Ntajal, J., Lamptey, B. L., Mahamadou, I. B., and Nyarko, B. K. (2017). Flood disaster risk mapping in the Lower Mono River Basin in Togo, West Africa. Int J Disaster Risk Reduct 23, 93–103. doi: 10.1016/j.ijdrr.2017.03.015
Nyberg, J. B., Marcot, B. G., and Sulyma, R. (2006). Using Bayesian belief networks in adaptive management (1). Can. J. For. Res. 36, 3104–3116. doi: 10.1139/x06-108
Nyumba, T. O., Wilson, K., Derrick, C. J., Mukherjee, N., and Geneletti, D. (2018). The use of focus group discussion methodology: Insights from two decades of application in conservation. Methods Ecol. Evol. 9, 20–32. doi: 10.1111/2041-210X.12860
Obada, E., Alamou, E. A., Biao, E. I., and Zandagba, E. B. J. (2021). Interannual variability and trends of extreme rainfall indices over Benin. Climate 9, 160. doi: 10.3390/cli9110160
Pearl, J. (1988). Probabilistic Reasoning in Intelligent Systems: Networks of Plausible Inference. San Francisco, CA: Morgan Kaufman Publishers, Inc.
Pearl, J., and Mackenzie, D. (2018). The Book of Why: The New Science of Cause and Effect. New York, NY: Basic Books.
Rachid, G., Alameddine, I., Najm, M. A., Qian, S., and El-Fadel, M. (2021). Dynamic Bayesian Networks to assess anthropogenic and climatic drivers of saltwater intrusion: a decision support tool toward improved management. Integr. Environ. Assess. Manag. 17, 202–220. doi: 10.1002/ieam.4355
Ribot (2011). Vulnerability before adaptation: toward transformative climate action. Global Environ. Change 21, 1160–1162. doi: 10.1016/j.gloenvcha.2011.07.008
Sahlin, U., Helle, I., and Perepolkin, D. (2021). “This is what we don't know”: treating epistemic uncertainty in Bayesian Networks for risk assessment. Integr. Environ. Assess. Manag. 17, 221–232. doi: 10.1002/ieam.4367
Sanchez, A. C., Fandohan, B., Assogbadjo, A. E., and Sinsin, B. (2012). A countrywide multi-ethnic assessment of local communities' perception of climate change in Benin (West Africa). Clim. Dev. 4, 114–128. doi: 10.1080/17565529.2012.728126
Schneiderbauer, S., Baunach, D., Pedoth, L., Renner, K., Fritzsche, K., Bollin, C., et al. (2020). Spatial-explicit climate change vulnerability assessments based on impact chains. Findings from a case study in Burundi. Sustainability 12, 6354. doi: 10.3390/su12166354
Shinn, J. E., and Hall-Reinhard, A. (2019). Emphasizing livelihoods in the study of social-ecological systems: insights from fishing practices in the Okavango Delta, Botswana. S. Afr. Geogr. J. 101, 121–139. doi: 10.1080/03736245.2018.1562365
Sinare, H., and Gordon, L. J. (2015). Ecosystem services from woody vegetation on agricultural lands in Sudano-Sahelian West Africa. Agric. Ecosyst. Environ. 200, 186–199. doi: 10.1016/j.agee.2014.11.009
Sinare, H., Gordon, L. J., and Enfors Kautsky, E. (2016). Assessment of ecosystem services and benefits in village landscapes – A case study from Burkina Faso. Ecosyst.Serv. 21, 141–152. doi: 10.1016/j.ecoser.2016.08.004
Sivapalan, M., Savenije, H. H. G., and Blöschl, G. (2012). Socio-hydrology: a new science of people and water. Hydrol. Process. 26, 1270–1276. doi: 10.1002/hyp.8426
Srikrishnan, V., and Keller, K. (2021). Small increases in agent-based model complexity can result in large increases in required calibration data. Environ. Model. Softw. 138, 1–9. doi: 10.1016/j.envsoft.2021.104978
Stritih, A., Rabe, S. -E., Robaina, O., Grêt-Regamey, A., and Celio, E. (2020). An online platform for spatial and iterative modelling with bayesian networks. Environ. Model Softw. 127, 1–18. doi: 10.1016/j.envsoft.2020.104658
Terzi, S., Torresan, S., Schneiderbauer, S., Critto, A., Zebisch, M., and Marcomini, A. (2019). Multi-risk assessment in mountain regions: a review of modelling approaches for climate change adaptation. J. Environ. Manag. 232, 759–771. doi: 10.1016/j.jenvman.2018.11.100
The World Bank (2021). Employment in agriculture (% of total employment) (modeled ILO estimate) - Benin. Available online at: https://data.worldbank.org/indicator/SL.AGR.EMPL.ZS?locations=BJ (accessed November 18, 2021)
Tramblay, Y., Villarini, G., and Zhang, W. (2020). Observed changes in flood hazard in Africa. Environ. Res. Lett. 15, 1040b105. doi: 10.1088/1748-9326/abb90b
Turner, B. L., Kasperson, R. E., Matson, P. A., McCarthy, J. J., Corell, R. W., Christensen, L., et al. (2003). A framework for vulnerability analysis in sustainability science. Proc. Natl. Acad. Sci. USA 100, 8074–8079. doi: 10.1073/pnas1231335100
UNDP - United Nations Development Programme (2019). Human Development Report 2019: Beyond Income, Beyond Averages, Beyond Today: Inequalities in Human Development in the 21st Century. New York, NY: UNDP.
UNDRR - UN Office for Disaster Risk Reduction (2019). Global Assessment Report on Disaster Risk Reduction 2019. Geneva.
UNDRR - UN Office for Disaster Risk Reduction CRED - Centre for Research on the Epidemiology of Disasters. (2020). Human Cost of Disasters: An Overview of the Last 20 Years 2000-2019. Available online at: https://reliefweb.int/sites/reliefweb.int/files/resources/Human%20Cost%20of%20Disasters%202000-2019%20Report%20-%20UN%20Office%20for%20Disaster%20Risk%20Reduction.pdf
Villa, F., Bagstad, K. J., Voigt, B., Johnson, G. W., Portela, R., Honzák, M., et al. (2014). A methodology for adaptable and robust ecosystem services assessment. PLoS ONE 9, e91001. doi: 10.1371/journal.pone.0091001
Walker, W. E., Harremoës, P., Rotmans, J., van der Sluijs, J. P., van Asselt, M., Janssen, P., et al. (2003). Defining uncertainty: a conceptual basis for uncertainty management in model-based decision support. Integr. Assess. 4, 5–17. doi: 10.1076/iaij.4.1.5.16466
Ward, P. J., Blauhut, V., Bloemendaal, N., Daniell, J. E., de Ruiter, M. C., Duncan, M. J., et al. (2020). Review article: natural hazard risk assessments at the global scale, Nat. Hazards Earth Syst. Sci. 20, 1069–1096. doi: 10.5194/nhess-20-1069-2020
Wisner, B., Blaikie, P., Cannon, T., and Davis, I. (2004). At Risk: Natural Hazards, People's Vulnerability and Disasters. London; New York, NY: Routledge.
Wu, Z., Shen, Y., Wang, H., and Wu, M. (2020). Urban flood disaster risk evaluation based on ontology and Bayesian Network. J. Hydrol. 583, 124596. doi: 10.1016/j.jhydrol.2020.124596
Zagorecki, A., and Druzdzel, M. J. (2004). “An empirical study of probability elicitation under Noisy-OR assumption,” in Proceedings of the Seventeenth International Florida Artificial Intelligence Research Society Conference: Miami Beach, Florida, May 17 - 19, 2004, eds V. Barr, and Z. Markov (Menlo Park, CA: AAAI Press), 880–885.
Zebisch, M., Schneiderbauer, S., Fritzsche, K., Bubeck, P., Kienberger, S., Kahlenborn, W., et al. (2021). The vulnerability sourcebook and climate impact chains – a standardised framework for a climate vulnerability and risk assessment. Int. J. Clim. Change Strat. Manage. 13, 35–59. doi: 10.1108/IJCCSM-07-2019-0042
Zebisch, M., Schneiderbauer, S., Renner, K., Below, T., Brossmann, M., Ederer, W., et al. (2017). Risk Supplement to the Vulnerability Sourcebook: Guidance on How to Apply the Vulnerability Sourcebook's Approach With the New IPCC AR5 Concept of Climate Risk. Available online at: https://www.adaptationcommunity.net/wp-content/uploads/2017/10/GIZ-2017_Risk-Supplement-to-the-Vulnerability-Sourcebook.pdf.
Keywords: drivers of risk, risk assessment, conceptual model, flood risk, Benin, vulnerability
Citation: Wetzel M, Schudel L, Almoradie A, Komi K, Adounkpè J, Walz Y and Hagenlocher M (2022) Assessing Flood Risk Dynamics in Data-Scarce Environments—Experiences From Combining Impact Chains With Bayesian Network Analysis in the Lower Mono River Basin, Benin. Front. Water 4:837688. doi: 10.3389/frwa.2022.837688
Received: 16 December 2021; Accepted: 07 February 2022;
Published: 11 March 2022.
Edited by:
Hossein Tabari, KU Leuven, BelgiumReviewed by:
Biljana Basarin, University of Novi Sad, SerbiaJosias Láng-Ritter, Aalto University, Finland
Hiroaki Ikeuchi, Ministry of Land, Infrastructure, Transport and Tourism, Japan
Copyright © 2022 Wetzel, Schudel, Almoradie, Komi, Adounkpè, Walz and Hagenlocher. This is an open-access article distributed under the terms of the Creative Commons Attribution License (CC BY). The use, distribution or reproduction in other forums is permitted, provided the original author(s) and the copyright owner(s) are credited and that the original publication in this journal is cited, in accordance with accepted academic practice. No use, distribution or reproduction is permitted which does not comply with these terms.
*Correspondence: Mario Wetzel, d2V0emVsLm1hcmlvQHBvc3Rlby5kZQ==