- 1Supercomputer Education and Research Centre, Indian Institute of Science, Bangalore, India
- 2Department of Biomedical Informatics, School of Medicine, Pittsburgh, PA, United States
- 3Intelligent Systems Program, School of Computing and Information, University of Pittsburgh, Pittsburgh, PA, United States
Accelerated efforts to identify intervention strategies for the COVID-19 pandemic caused by SARS-CoV-2 need to be supported by deeper investigations into host invasion and response mechanisms. We constructed the neighborhood interactome network of the 332 human proteins targeted by SARS-CoV-2 proteins, augmenting it with 1,941 novel human protein-protein interactions predicted using our High-precision Protein-Protein Interaction Prediction (HiPPIP) model. Novel interactors, and the interactome as a whole, showed significant enrichment for genes differentially expressed in SARS-CoV-2-infected A549 and Calu-3 cells, postmortem lung samples of COVID-19 patients and blood samples of COVID-19 patients with severe clinical outcomes. The PPIs connected host proteins to COVID-19 blood biomarkers, ACE2 (SARS-CoV-2 entry receptor), genes differentiating SARS-CoV-2 infection from other respiratory virus infections, and SARS-CoV-targeted host proteins. Novel PPIs facilitated identification of the cilium organization functional module; we deduced the potential antiviral role of an interaction between the virus-targeted NUP98 and the cilia-associated CHMP5. Functional enrichment analyses revealed promyelocytic leukaemia bodies, midbody, cell cycle checkpoints and tristetraprolin pathway as potential viral targets. Network proximity of diabetes and hypertension associated genes to host proteins indicated a mechanistic basis for these co-morbidities in critically ill/non-surviving patients. Twenty-four drugs were identified using comparative transcriptome analysis, which include those undergoing COVID-19 clinical trials, showing broad-spectrum antiviral properties or proven activity against SARS-CoV-2 or SARS-CoV/MERS-CoV in cell-based assays. The interactome is available on a webserver at http://severus.dbmi.pitt.edu/corona/.
1 Introduction
COVID-19 (Coronavirus Disease 2019) is an infectious virus outbreak which emerged as an epidemic in one city in December 2019, and within 3 months swept across 220 countries and territories, developing into a pandemic global health crisis with more than 180 million confirmed infected cases and more than 4 million deaths as of 1 July 2021 (WHO). The novel coronavirus (SARS-CoV-2/nCoV-19) has been identified as the causative agent of this disease (Rothan and Byrareddy, 2020). Coronaviruses are a large family of viruses that are pathogenic in mammals and birds. In humans, they cause respiratory infections ranging from the common cold to possibly fatal acute respiratory distress syndrome (ARDS) and acute lung injury (ALI), which are noted in COVID-19 as well as in its predecessors, namely, SARS (severe acute respiratory syndrome, 2002–2003) and MERS (middle east respiratory syndrome, 2012) (Gralinski and Baric, 2015). SARS-CoV-2 is airborne, and causes no symptoms in several infected people who may become silent carriers of the disease to the more vulnerable population. COVID-19 is spreading at an exponential rate globally, prompting scientists across the globe to investigate the mechanisms of its host invasion and host response to viral infection, in hopes of discovering treatment strategies to combat the outbreak.
The viral infection sets off a cascade of interactions among multiple genes and proteins in the host cell. This complex network has the potential to restrict viral replication in host cells, or conversely, to be taken over by the virus for its perpetuation. Several research groups have studied the effects of SARS-CoV-2 on the host from a systems-level perspective (Blanco-Melo et al., 2020a; Gordon et al., 2020a; Zhou et al., 2020a). 332 human proteins that bind to SARS-CoV-2 proteins were identified through affinity purification—mass spectrometry (AP-MS) by Gordon et al. (Gordon et al., 2020b). Melo et al. identified more than 6,000 genes differentially expressed in A549, Calu-3 and NHBE cell lines upon SARS-CoV-2 infection, and in COVID-19 patients (Blanco-Melo et al., 2020b). Bojkova et al. monitored SARS-CoV-2 infection in Caco2 cell line and generated temporal infection profiles of 2,687 genes in the host translatome and 6,258 proteins in the proteome (Denisa Bojkova and Koch, 2020). Data generated by these studies can be employed to conduct systematic, unbiased and data-driven investigations into COVD-19 from the perspective of the host, by constructing the relevant protein interactome (i.e., protein-protein interaction network).
Protein-protein interactions (PPIs) drive the cellular machinery and facilitate biological processes including signal transduction, formation of cellular structures and enzymatic complexes. When viral proteins bind to some proteins in the host cell, this effect may spread along the interactome through regulatory and biophysical interactions, affecting other proteins in the PPI network, posing deeper implications for viral infection, host immunity, and the effect of therapeutics (Barabási et al., 2011). Despite being critical to unravelling novel disease mechanisms and drugs, ∼75% of estimated PPIs are currently unknown and several disease-associated genes have no known PPIs. More than ∼600,000 PPIs are said to exist in the human interactome (Keskin et al., 2016) and only ∼150,000 PPIs are known from PPI repositories such as HPRD (Keshava Prasad et al., 2008) and BioGRID (Stark et al., 2006). Detecting the PPIs using experimental techniques such as co-immunoprecipitation (Co-IP) (Blasche and Koegl, 2013; Trepte et al., 2015) is prohibitively laborious and time-consuming at large scale. Tens of thousands of PPIs are being added into the interactome through systematic high throughput studies with yeast two hybrid (Y2H) system (Luck et al., 2020) and AP–MS (Huttlin et al., 2020). Despite this, a large part of the interactome remains unknown. Hence, computational algorithms have been developed to predict PPIs in human as well as model organisms (Deng et al., 2003; Raja et al., 2013; You et al., 2013; Emamjomeh et al., 2014; Hopf et al., 2014; Jia et al., 2015; Kotlyar et al., 2015; Garzón et al., 2016; Malavia et al., 2017a). We have previously developed a computational model called HiPPIP (High-Precision Protein-Protein Interaction Prediction) that was deemed highly accurate by computational evaluations and experimental validations (Zhu et al., 2014; Ganapathiraju et al., 2016a; Dunham and Ganapathiraju, 2022). HiPPIP computes features of protein pairs such as cellular localization, molecular function, biological process membership, genomic location of the gene, and gene expression in microarray experiments, and classifies the pairwise features as interacting or non-interacting based on a random forest model (Ganapathiraju et al., 2016a). Though each of the features by itself is not an indicator of an interaction, a machine learning model was able to use the combined features to make predictions with high precision. The threshold of HiPPIP to classify a protein-pair as “a PPI” was set high in such a way that it yields very high-precision predictions even if low recall. Seventeen of the predicted PPIs were tested experimentally and were shown to be true PPIs, namely, 8 PPIs validated by co-immunoprecipitation: DDX58-OASL (Zhu et al., 2014), HMGB1-FLT1 (Ganapathiraju et al., 2016a), HMGB1-KL (Ganapathiraju et al., 2016a), STT3A-RPS25 (Ganapathiraju et al., 2016a), STT3A-SYCP3 (Ganapathiraju et al., 2016a), STT3A-MCAM (Ganapathiraju et al., 2016a), PDCD1-<hidden> (unpublished validation), YWHAE1-<hidden> (unpublished validation), five PPIs validated by in vitro pull-down and mass spectrometry: ALB-KDR (Karunakaran et al., 2021), ALB-PDGFRA (Karunakaran et al., 2021), BAP1-PARP3 (Karunakaran et al., 2021), CLPS-CUTA (Karunakaran et al., 2021), HMGB1-CUTA (Karunakaran et al., 2021) and 4 PPIs validated by co-localization: STX3-LPXN (Ganapathiraju et al., 2016a), STX4-MAPK3 (Ganapathiraju et al., 2016a), IFT88-KL (unpublished validation) and WDR5-IGFBP3 (unpublished validation). Some of the predicted PPIs proved to have high translational impact. For example, we predicted that the human OASL protein (IFN-inducible oligoadenylate synthetases-like) interacts with RIG-I (retinoic acid-inducible gene I); it was validated to be a true PPI. Further investigations conclusively showed that this interaction is responsible for activating cellular innate immunity to virus infections: OASL enhances antiviral signalling mediated by the viral RNA sensor RIG-I by binding through its C-terminal ubiquitin-like domain (Zhu et al., 2014). Other high-impact results from interactome analysis include shared PPIs explaining inverse epidemiological relationship between schizophrenia and rheumatoid arthritis (Malavia et al., 2017b) and cilia-transduced cell signaling in congenital heart disease (Li et al., 2015; Liu et al., 2017), and more (Karunakaran et al., 2019a).
In this work, we present the human protein-protein interactome of the proteins targeted by SARS-CoV-2 (Gordon et al. (2020a)). A concept diagram of the analysis carried out here is shown in Figure 1. Key contributions of this work are about 2,000 previously unknown human PPIs that are computationally predicted with high-precision, and the results of analyzing the network of known and predicted interactions with functional annotations and with SARS-CoV-2-relevant transcriptomic and proteomic data. Importantly, we are making this interactome, with rich annotations, available on a webserver and in graph formats downloadable for further computational analyses.
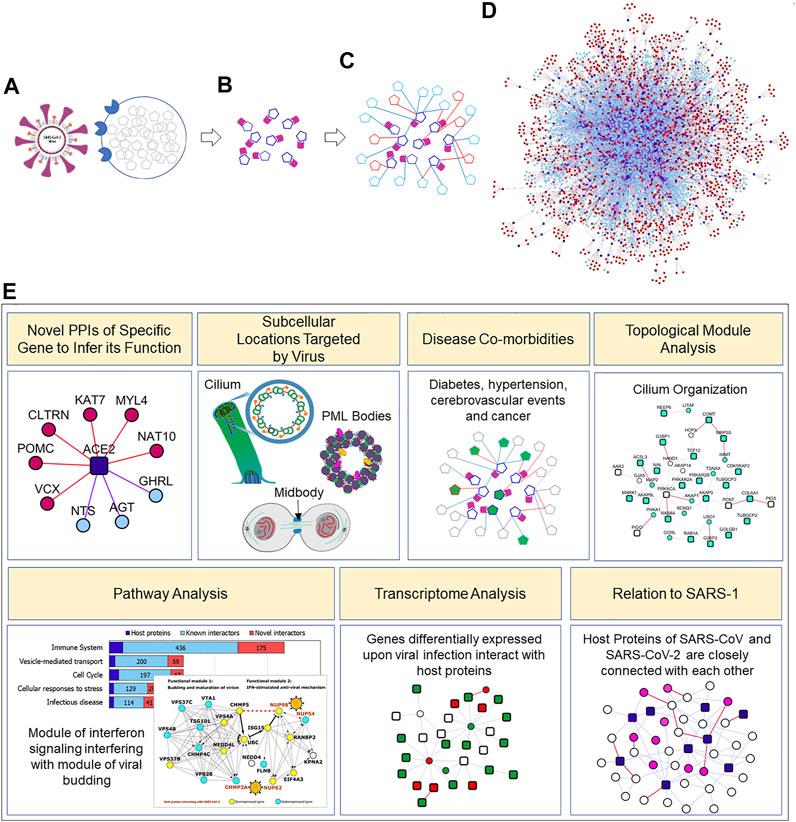
FIGURE 1. Concept diagram of the analysis presented in the paper: Clockwise from top-left: (A). SARS-CoV-2 enters the host cell with the help of an interaction between its surface-anchored “spike protein” and the ACE2 receptor on the host cell. Once the virus gains entry into the host cell, it hijacks the host cellular machinery to promote viral genome replication, and viral mRNA and protein synthesis (B). SARS-CoV-2 viral proteins (purple) produced in this manner interact with a specific set of host cell proteins (dark blue; identified by Gordon et al. (2020a). In this study, (C). we assembled the known (light blue) and computationally predicted/novel (red) interactors of the host proteins, and (D). systematically studied this expanded neighbourhood network of virus-targeted host proteins (E). Various types of analysis carried out in this work. Several more systems studies are possible with the interactome both because of new types of analysis or analysis with new data sources. Cilium, PML bodies and midbody figures are from (Morgan, 2007; Wilson, 2015; Hoischen et al., 2018) (with creative commons license on WikiMedia). Novel PPIs of the SARS-CoV-2 targeted host proteins predicted by HiPPIP can be found at http://severus.dbmi.pitt.edu/corona/.
2 Results
We collected 332 host proteins that were identified to interact with 27 SARS-CoV-2 viral proteins identified from the 2019-nCoV/USA-WA1/2020 strain by Gordon et al. (2020a). To assemble the interactome of these host proteins, we compiled known PPIs from HPRD (Keshava Prasad et al., 2008) (Human Protein Reference Database) and BioGRID (Stark et al., 2006) (Biological General Repository for Interaction Datasets), and predicted novel PPIs by applying the HiPPIP algorithm described in our earlier work (Ganapathiraju et al., 2016b) (Supplementary Table S1). Note that the interactome presented here is human protein interactome, and not a virus-host interactome; the relevance to COVID-19 is that the core proteins for which the interactome is assembled are those that the viral proteins bind to. Specifically, as shown in Figure 2, we assembled the known and novel interactors (round light blue and red colored nodes, respectively) of the host proteins (square-shaped dark blue colored nodes) targeted by SARS-CoV-2 viral proteins (diamond-shaped green colored nodes). HiPPIP predicted ∼2,600 PPIs of which ∼600 PPIs were previously known, leaving ∼2,000 PPIs to be considered as novel PPIs of the host proteins. There were an additional 3,500 PPIs that were known and not predicted by HiPPIP. This is as expected as the HiPPIP prediction threshold has been fixed to achieve high precision by compromising recall, which is required for adoption into biology; in other words, it is set to predict only a few PPIs out of the hundreds of thousands of unknown PPIs, but those will be highly accurate. It has to be noted that neither PPI prediction nor high throughput PPI screening can be performed with high-precision and high-recall. Co-IP based methods show high-precision and extremely-low recall (detecting only one PPI at a time), whereas multi-screen high-quality yeast 2-hybrid methods show high-precision with low recall (detecting a few tens of thousands of PPIs). Thus, HiPPIP is on par with other methods in terms of precision and the number of new PPIs detected. Recently, state-of-the-art algorithms that were developed after HiPPIP have been extensively evaluated, but none of them reached the superior performance achieved by HiPPIP (Dunham and Ganapathiraju, 2022). Seventeen novel PPIs predicted by HiPPIP in our other studies were tested, and all validated to be true; the experiments were carried out by diverse research labs. Overall, the host protein (HoP) interactome consisted of 4,408 proteins and 6,076 interactions. A partial network of host proteins and their novel interactors is shown in Figure 3A. Several COVID-centric network biology studies (Zhou et al., 2020b; Gysi et al., 2020; Kumar et al., 2020) presented analysis of the “known PPI neighborhood” of the host proteins targeted by SARS-CoV-2. Contrary to this, in our study, we augment this neighborhood with 1,941 computationally predicted PPIs of high precision (Figure 2), so as to 1) present a more complete version of the host protein interactome, 2) facilitate discovery of previously unknown disease mechanisms, and 3) allow characterization of under-studied host proteins through functional associations of their predicted interactors. Moreover, the network is made available on an interactive webserver to enable biologists to examine the novel interactions relevant to their specific protein or pathway of interest, and as downloadable files in various formats to facilitate its investigation in conjunction with transcriptomic/proteomic data by computational systems biologists.
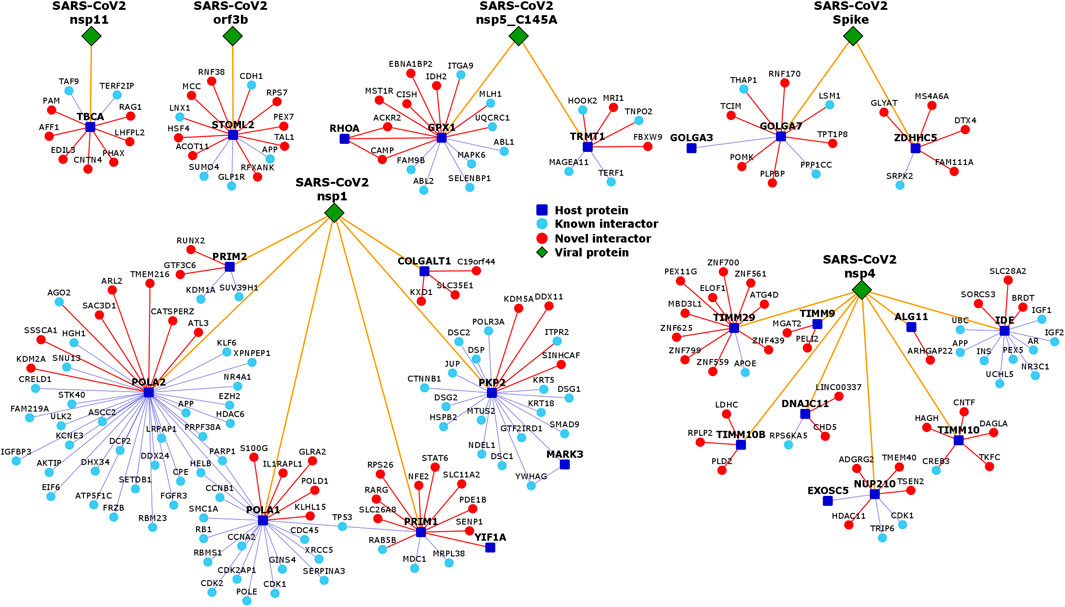
FIGURE 2. Neighborhood networks of SARS-CoV-2-targeted host proteins expanded by novel PPIs: Genes are shown as nodes and PPIs as edges. The network diagrams illustrate augmentation of the host protein interactome with computationally predicted novel interactors (shown as round red colored nodes). The data pertaining to the interaction (golden yellow edges) of SARS-CoV-2 viral proteins (shown as diamond shaped dark green colored nodes) with host proteins (square shaped dark blue colored nodes) was collected from Gordon et al. (Gordon et al., 2020a). The data pertaining to known PPIs (blue edges) of the host proteins with known interactors (round light blue colored nodes) was collected from BioGRID and HPRD. To present a more complete version of the interactome, we computationally predicted novel PPIs (red edges) of the host proteins with novel interactors (round red colored nodes) using the HiPPIP algorithm. Each of these novel PPIs not only serves as valuable candidates for further experimentation, but also facilitates systems-level analysis of the host protein interactome and generation of new testable hypotheses. Note that the figure only shows the neighbourhood networks of a few selected viral proteins. The complete list of interactions between viral proteins and host proteins, and between host proteins and its interactors can be found in http://severus.dbmi.pitt.edu/corona/.
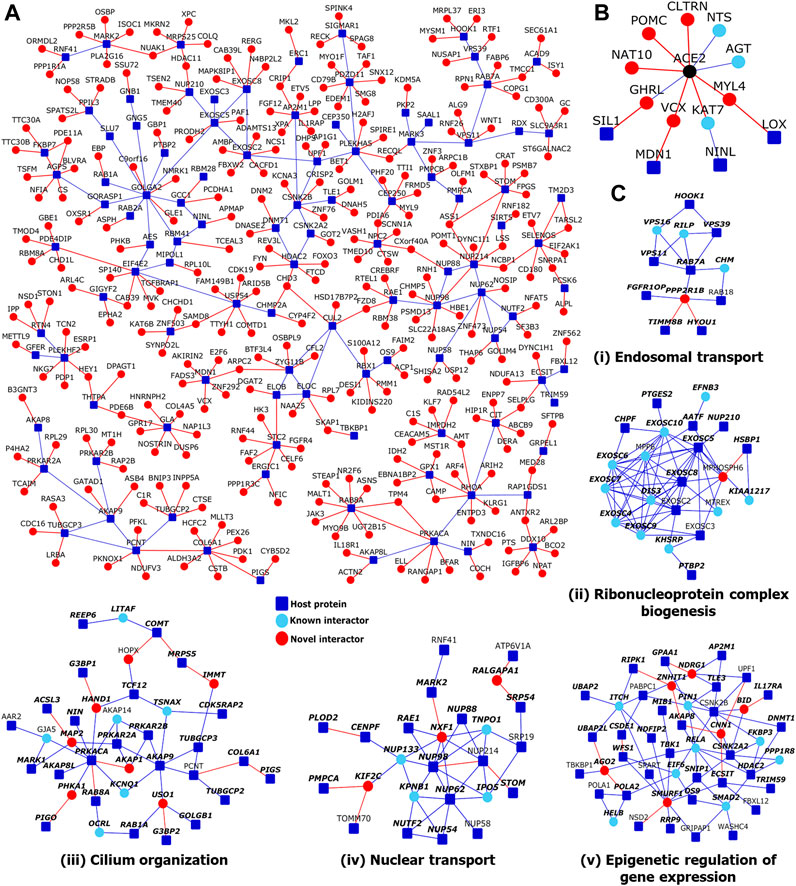
FIGURE 3. Network views of protein-protein interactions in the host protein interactome: (A) Partial view of the HoP interactome: Genes are shown as nodes and PPIs as edges. As the full network is very large, only a partial view showing a large connected component of novel interactors and their neighbors, all of which have transcriptomic/proteomic evidence related to SARS-CoV-2 (Supplementary Table S2), is shown. Legend: Dark blue square-shaped nodes: host proteins targeted by SARS-CoV-2; red nodes/edges: novel interactors/interactions; light blue nodes and blue edges: known interactors and interactions. (B) ACE2 interactome: PPIs of ACE2 protein, extended to show four host proteins that are two-edges away from it. Color legend is as in (A-C) Modules identified from network topology: Five out of seventeen total modules each with 3 or more nodes are shown. Each module was enriched in a specific GO biological process: (i) Endosomal transport, (ii) Ribonucleoprotein complex biogenesis, (iii) Cilium organization, (iv) Nuclear transport and (v) Epigenetic regulation of gene expression. Within each module shown in (i-v), nodes with bold italicized labels depict genes with at least one transcriptomic/proteomic evidence relevant to SARS-CoV-2.
We verified whether any of the 2,000 novel PPIs came up in recently released interactome maps such as HuRI (HI-Union) (Luck et al., 2020) and BioPlex (Huttlin et al., 2020). While there was no overlap with the HI-union dataset, there were 8 PPIs in the BioPlex map (ADAM9-ADAM32, P3H3-OS9, PVR-NECTIN2, SRRM2-SNIP1, PABPC4-LUC7L2, PRKACA-AKAP1, NDUFA13-ECSIT, and NPTX1-NPTX2). The small overlap is not surprising because even high-throughput biotechnological methods discover different parts of the interactome with only small overlaps with each other, thus demonstrating complementary strengths (Luck et al., 2020).
2.1 Wiki-CORONA: A Web Server of Novel Host PPIs
The HoP interactome is available on a website called Wiki-CORONA (http://severus.dbmi.pitt.edu/corona/). It has advanced search capabilities, and presents comprehensive annotations, namely Gene Ontology, diseases, drugs and pathways, of the two proteins of each PPI side-by-side. Here, a user can query for results such as “PPIs where one protein is anti-viral and the other is involved in immunity,” and then see the results with the functional details of the two proteins side-by-side. The PPIs and their annotations also get indexed in major search engines like Google and Bing. Querying by biomedical associations is a unique feature which we developed in Wiki-Pi that presented known interactions of human proteins (Orii and Ganapathiraju, 2012).
2.2 Interconnections of ACE2 With Host Proteins Targeted by SARS-CoV-2
SARS-CoV-2 engages the host receptor ACE2 (angiotensin-converting enzyme 2) for cell entry (Hoffmann et al., 2020). The interactions of SARS-CoV-2 viral proteins with host proteins were studied by Gordon et al. in human embryonic kidney cells (HEK-293T/17) (Gordon et al., 2020a), which show very low endogenous expression of ACE2 (Warner et al., 2005); even if HEK-293 cells were transfected with ACE2 to allow heterologous ACE2 expression, its protein product may undergo proteolytic cleavage mediated by ADAM17 (Lambert et al., 2005). Possibly due to this reason, ACE2 was not identified as a host protein in that study (Gordon et al., 2020b). Therefore, we assembled the known and novel PPIs of ACE2 separately, owing to its crucial role in SARS-CoV-2 infection. Then, we extracted the shortest paths in the interactome connecting ACE2 to any of the 332 host proteins using methods described in our prior work, LENS (Lens for Enrichment and Network Studies of human proteins) (Handen and Ganapathiraju, 2015). We found that ACE2 was connected to 4 host proteins targeted by SARS-CoV-2 (SIL1, LOX, MDN1 and NINL) through an intermediate interactor, i.e. separated by two edges, where one or both intermediary PPIs were novel predicted ones (see red edges in Figure 3B). Thus, we showed that novel PPIs connect ACE2 to multiple host proteins through intermediary proteins.
These connections revealed interesting insights: ACE2 is a key player of the renin-angiotensin hormone system that regulates blood pressure and electrolyte balance (Burrell et al., 2004). In line with this, we found that its interactors, AGT (angiotensin), GHRL, CLTRN and POMC, were associated with the Reactome Pathway peptide hormone metabolism (p-value = 2.9 × 10−5). ACE2 and its interactors were also enriched in the Gene Ontology (GO) Biological Process circulatory system process (ACE2, AGT, NTS, POMC, GHRL and the host protein MYL4; p-value = 1 × 10−3). Three host proteins were associated with numerous vascular and cardiac phenotypes: LOX with abnormality of blood volume homeostasis, aortic root aneurysm, ascending aortic dissection, carotid artery dilatation, coronary artery atherosclerosis, cystic medial necrosis of the aorta, descending thoracic aorta aneurysm, dilatation of the cerebral artery, left ventricular failure, peripheral arterial stenosis, MYL4 with paroxysmal atrial fibrillation and bradycardia, and SIL1 with abnormal aldolase level. The co-morbidity of hypertension, diabetes and cardiovascular diseases among the group of COVID-19 patients with high fatality rate (Fang et al., 2020a) warrants a closer look at ACE2 and the host proteins linked to cardiac and vascular phenotypes. We also examined the interconnections of the host proteins with other proteins that facilitate SARS-CoV-2 entry into host cells, namely, TMPRSS2, CTSB, CTSL, NRP1, AGTR2 and OR51E2 (Cantuti-Castelvetri et al., 2020; Cui et al., 2020; Hoffmann et al., 2020; Kerslake et al., 2020). Five out of these 6 proteins—TMPRSS2, CTSB, CTSL, NRP1, and AGTR2—were found to be connected to 33 host proteins via 52 intermediate interactors including 12 novel interactors (Supplementary Figure S1). Detailed investigations may be necessary to understand the relationships of these host cellular entry proteins to other host factors targeted by SARS-CoV-2.
2.3 Identification of Network Modules From the Host Protein Interactome
Viruses have been shown to target network modules of host proteins (Jäger et al., 2012; Hafirassou et al., 2017; Yang et al., 2019). These modules could either correspond to 1) protein complexes in which proteins interact within a specific location/time/condition to perform a function in a coordinated manner (e.g., RNA splicing machinery and transcription machinery), or 2) to form dynamic, yet functionally coherent units, in which the proteins interact with one another at different times/conditions to carry out a biological process (e.g., signaling pathways and cell cycle regulation) (Spirin and Mirny, 2003). We employed “Netbox” software implementation with data consisting of SARS-CoV-2 target proteins (core proteins) and all human PPIs (Cerami et al., 2010) to identify network modules. It expands the core proteins by adding nodes from the interactome whose number of links to core proteins are statistically significant compared to its degree in the human interactome. From this network, it identifies highly interconnected modules. It was able to connect 323 proteins (220 host proteins targeted by SARS-CoV-2 and 103 linker proteins) into 21 modules, of which 14 modules had 4 or more nodes each. For comparison, when novel PPIs were not included, it connected 199 proteins (138 host proteins and 61 linker proteins) into 18 modules of which 10 had 4 or more proteins each. Scaled modularity score (Z-score compared to random networks) was 17.0 with novel PPIs, and it was 14.5 without novel PPIs (Z-score compared to corresponding random networks). Five modules formed with novel interactors had statistically significant enrichment of GO biological process terms: epigenetic regulation of gene expression (p-value = 3.3 × 10−4, odds ratio = 10.4), nuclear transport (p-value = 2.4 × 10−12, odds ratio = 21.6), cilium organization (p-value = 1.28 × 10−3, odds ratio = 7.8), ribonucleoprotein complex biogenesis (p-value = 0, odds ratio = 22.4), and vesicle-mediated transport between endosomal compartments (p-value = 9.4 × 10−6, odds ratio = 123.4) (Figures 3Ci–v). When novel PPIs were excluded, some of these associations were missed (epigenetic regulation of gene expression and cilium organization) and the modules were smaller, but 3 additional functional modules were found: cell cycle G2/M phase transition (p-value = 1.9 × 10−3, odds ratio = 21.7), DNA replication (p-value = 4.9 × 10−3, odds ratio = 55.25) and cell-cell signaling by Wnt (p-value = 4.9 × 10−3, odds ratio = 9.3). Hence, although several biological processes detected by including the novel PPIs could also be detected using only the known PPIs, functional modules such as cilium organization were only uncovered on inclusion of the novel PPIs that we predicted for the host proteins (namely, COMT-HOPX, MRPS5-IMMT, G3BP1-HAND1, ACSL3-MAP2, PRKACA-AKAP1, PIGO-PHKA1 and G3BP2-USO1). In summary, the novel PPIs improved existing COVID-related knowledge by facilitating the identification of functional modules, which would have remained hidden if one had only used known PPIs for module identification.
2.4 Overlap of the Host Protein Interactome With Transcriptome and Proteome Data
We systematically analyzed the overlap of the HoP interactome with gene expression profiles induced by SARS-CoV and SARS-CoV-2. Statistically significant overlaps were found with the genes differentially expressed in A549 (human lung alveolar carcinoma) cell lines transfected with ACE2 and infected with a high load of SARS-CoV-2 (multiplicity of infection/MOI = 2.0) (p-value = 3.67 × 10−17, odds ratio = 1.26), Calu-3 (human lung epithelial carcinoma) cell line infected with a high SARS-CoV-2 load (MOI = 2.0) (p-value = 1.98 × 10−3, odds ratio = 1.12) and postmortem lung samples of COVID-19 positive patients (p-value = 8.3 × 10−17, odds ratio = 1.37) [GSE147507 (Blanco-Melo et al., 2020b)]. Significant enrichment of the novel interactors that were predicted to interact with the host proteins targeted by SARS-CoV-2 was noted in the A549 cell line (p-value = 1.6 × 10−3, odds ratio = 1.17) and COVID-19 patient (p-value = 1.18 × 10−2, odds ratio = 1.19) datasets. Many proteins in the interactome, including novel interactors, were differentially expressed in epithelial cells infected with SARS-CoV (GSE17400, Calu-3 cell, 48 h post-infection; p-value = 4.76 × 10−12). Several proteins also showed differential expression after infection by Urbani strain of SARS-CoV (GSE37827, Calu-3 cells, 72 h post-infection), in peripheral blood mononuclear cells of SARS patients (GSE1739 (Reghunathan et al., 2005)), in A549 cell line infected with a low SARS-CoV-2 load (MOI = 0.2) and in NHBE (normal human bronchial epithelial) cell line infected with a high SARS-CoV-2 load (MOI = 2.0), but their overlaps were not statistically significant. Most importantly, the interactome demonstrated statistically significant overlaps with the genes differentially expressed in the leukocytes of COVID-19 patients with ARDS admitted to the intensive care unit (ICU) versus those receiving non-intensive care (p-value = 4.63E-10, odds ratio = 1.13) (GSE157103 (Overmyer et al., 2021)) and whole blood of COVID-19 patients critical in ICU with ARDS versus non-critical patients on oxygen [p-value = 0.035, odds ratio = 1.04) (GSE172114 (Carapito et al., 2021)). This suggested that the HoP interactome can be used as a framework to contextualize the gene expression signatures differentiating the various clinical outcomes of COVID-19. Additionally, we showed the overlap of the interactome with genes differentially expressed in blood samples of COVID-19 patients admitted to the ICU with ARDS compared with non-critical patients on oxygen (p-value = 6.03 × 10−7, odds ratio = 1.11) (GSE172114 (Carapito et al., 2021)) and in peripheral blood mononuclear cells (PBMCs) of COVID-19 patients in ICU versus healthy subjects (p-value = 1.55 × 10−27, odds ratio = 1.25) and COVID-19 patients with moderate symptoms versus healthy subjects (p-value = 5.08 × 10−30, odds ratio = 1.35) (GSE152418 (Arunachalam et al., 2020)). Statistically significant enrichments for novel interactors were also found with PBMCs of ICU-admitted COVID-19 patients (p-value = 1.69 × 10−5, odds ratio = 1.18) and those with moderate symptoms (p-value = 8.01 × 10−4, odds ratio = 1.18). In summary, the overlaps of the transcriptional profiles induced by SARS-CoV/SARS-CoV-2 with the HoP interactome 1) ascertained the biological validity of the HoP interactome, 2) contextualized the differentially expressed genes within the mechanistic framework of the protein interactome and 3) highlighted novel interactors of the host proteins targeted by SARS-CoV-2 that may be prioritized for further study.
Melo et al. had identified 120 genes differentially expressed upon infection by SARS-CoV-2 in the A549 cell line compared with infection by respiratory syncytial virus and/or influenza A virus (GSE147507 (Blanco-Melo et al., 2020a)). Of these, only 2 differentially expressed genes (DEGs) were among the 332 host proteins targeted by SARS-CoV-2. Our study revealed several interesting links between host proteins and these DEGs (Figure 4A): 1) although only 2 DEGs were found among the host proteins themselves, 31 DEGs were direct interactors of 38 host proteins, with some DEGs interacting with multiple host proteins; 2) 13 novel PPIs existed between the two sets: AAR2-SAMHD1, TUBGCP2-C1R, IMPDH2-C1S, GOLGA7-TCIM, RAB8A-STEAP1, GDF15-EHF, REEP5-PDK4 FAM162A-PARP14, STOML2-CDH1, FGA-RAB14, FBXL12-C19orf66, ECSIT-C19orf66 and EIF4H-PTPN12; 3) 108 DEGs and 285 host proteins connected to each other via a common interactor (there were 808 such shared interactors between DEGs and host proteins; statistically significant overlap with odds ratio = 1.5, p-value = 7.12 × 10−54); 4) Pathway enrichment analysis of overlapping interactome (consisting of 808 shared interactors, and the DEGs and the host proteins that they interact with) revealed enrichment of several immune-related pathways (each with FDR-corrected p-value < 0.05).
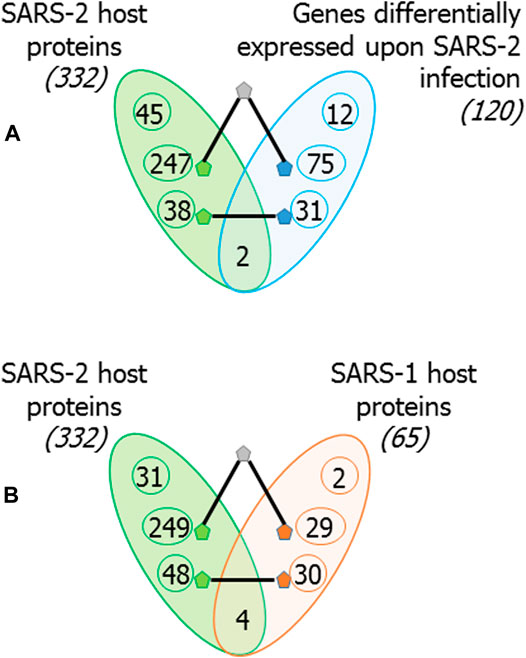
FIGURE 4. Overlap of the host proteins targeted by SARS-CoV-2 with genes differentially expressed upon SARS-CoV-2 infection and with host proteins targeted by SARS-CoV: (A) 120 genes were identified to be differentially expressed in A549 cell line upon SARS-CoV-2 infection compared with infection by respiratory syncytial virus and/or influenza virus. Of these, 2 are also host proteins targeted by SARS-CoV-2, 31 have direct PPIs with SARS-CoV-2 host proteins and 75 link to SARS-CoV-2 host proteins via an intermediate interactor. Thus, 108 out of 120 genes differentially expressed upon SARS-CoV-2 infections are closely connected to SARS-2 host proteins. Note that only closest connections are shown; i.e., a directly interacting DEG may also be connected via an intermediate interactor to host proteins, but it is counted among former. (B) Similar counts are shown for 65 host proteins targeted by SARS-CoV: 4 are common, 30 have direct PPIs, and 29 link to SARS-CoV-2 host proteins via an intermediate interactor. Thus, 63 out of 65 host proteins targeted by SARS-CoV are closely connected to host proteins targeted by SARS-CoV-2.
Messner et al. had identified 27 protein biomarkers whose expression varied according to the WHO severity grades for COVID-19 infection (i.e. no oxygen support, with oxygen support and critical) (Messner et al., 2020). Out of these, 11 biomarkers were identified in our study as interactors of the host proteins targeted by SARS-CoV-2. This included 8 proteins, ACTB, C1R, C1S, CD14, FGA, GSN, ITIH3 and SAA1, which were predicted as novel interactors of the host proteins, TCF12, RALA, TUBGCP2, IMPDH2, REEP5, RAB14, RHOA and GNG5.
Next, we considered the overlap between 65 host proteins that were identified to interact with SARS-CoV proteins by Pfefferle et al. (2011) and the host proteins targeted by SARS-CoV-2. Only 4 proteins were common to both (BZW2, MARK2, MARK3 and SMOC1) (Figure 4B). However, the interactome revealed that 50 host proteins targeted by SARS-CoV-2 had direct interactions with 32 host proteins targeted by SARS-CoV, and that 8 of these were novel PPIs (N4BP2L2-EXOSC8, NMB-MRPS5, MKRN2-MRPS25, HOXC6-BRD2, XPA-AP2M1, VKORC1- DCTPP1, RSRP1-CEP350 and TPSAB1-ADAMTS1). 29 host proteins targeted by SARS-CoV were connected to 249 host proteins targeted by SARS-CoV-2 via a common interactor.
GO biological process terms such as autophagic mechanism (odds ratio = 4.5, p-value = 2.2 × 10−5) regulation of mitochondrion organization (odds ratio = 7.5, p-value = 5.5 × 10−5) and protein localization to mitochondrion (odds ratio = 7.8, p-value = 3.74 × 10−4) were enriched in the overlapping interactome, suggesting that these processes may be commonly targeted by both these viruses. Mitochondria may be directly targeted by viral proteins, and may be affected by the cellular changes arising from viral infection. They may also play a crucial role in viral pathogenesis due to their function as immune signalling hubs (Khan et al., 2015). These organelles are constantly eliminated and recycled through a process called mitophagy. Viruses can modulate mitochondrial function and mitophagy to exacerbate infection (Khan et al., 2015).
In summary, 63 out of the 65 host proteins targeted by SARS-CoV, and 108 out of the 120 genes differentially expressed upon SARS-CoV-2 infection interacted directly or through an intermediate interactor with the host proteins targeted by SARS-CoV-2 (Figure 4).
3,787 (86%) proteins in the interactome are supported by the above mentioned transcriptomic and proteomic evidence, and are listed in Supplementary Table S2. In fact, the selected novel interactors shown in Figure 2A all have transcriptomic/proteomic evidence.
We studied tissue-specific expression of the proteins in the interactome using GTEx data (Lonsdale et al., 2013). Genes with an expression level greater than 1 TPM (transcripts per million) and relative expression at least 5-fold higher in a particular tissue (tissue-enriched) or a group of 2-7 tissues (group-enriched) were considered (Fagerberg et al., 2014). As expected, many genes showed specific expression in lung, which is the target tissue of the virus, and in spleen, which regulates the immune response of the host (Figure 5). Host proteins targeted by SARS-CoV-2 had novel PPIs with 37 lung-specific proteins and 49 spleen-specific proteins. Apart from these expected tissue associations, we noted that the host proteins also had novel PPIs with 61 brain-specific and 28 heart-specific proteins, which is of importance as cerebrovascular diseases and coronary heart diseases are co-morbidities among COVID-19 non-survivors (Figure 5) (Fang et al., 2020b).
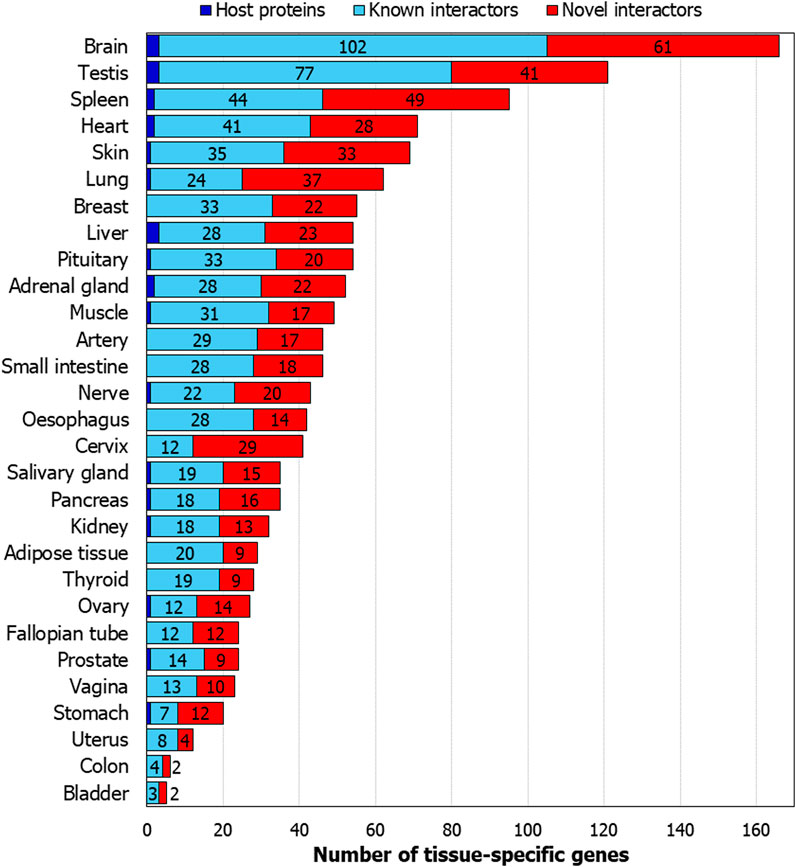
FIGURE 5. Tissue-specific genes in the host protein interactome: Number of genes from the interactome which show tissue specificity are shown. The genes show at least 5-fold higher expression in a tissue or a group of 2-7 tissues compared to all the other tissues.
2.5 Functional Enrichment Analysis of the Host Protein Interactome
We identified functional associations of the HoP interactome using the gene set analysis toolkit called WebGestalt (Liao et al., 2019). WebGestalt computes enrichment of specific functional groups (e.g., a Reactome Pathway) in an input list (e.g., genes in the HoP interactome). Statistical significance is computed using Fisher’s exact test, and corrected using the Benjamini–Hochberg method for multiple test adjustment. WebGestalt was chosen for its user-friendly interface, intuitive plots, large collection of functional categories from different types of functional databases and multiple enrichment analysis methods. This analysis yielded information from different biological levels that may potentially be influenced by SARS-CoV-2 infection:
(1) Co-morbidity relationships: proteins encoded by the genes associated with diabetes and hypertension showed network proximity to the host proteins targeted by SARS-CoV-2
(2) Subcellular locations: PML (promyelocytic leukaemia) bodies and the midbody may function as subcellular targets of SARS-CoV-2, since proteins localizing to these structures were found to be significantly enriched in the HoP interactome
(3) Cellular processes: enrichment of proteins involved in cell cycle phase transitions may allude to SARS-CoV-2 modulating critical junctures in the host cell cycle to facilitate viral infection
(4) Cellular pathways: the post-transcriptional tristetraproline-mediated regulatory pathway is significantly associated with the interactome and may be targeted by SARS-CoV-2 proteins to weaken host immune response
2.6 Co-Morbidity Relationships
We studied the association of interactome genes with any genetic disorders/traits in the OMIM database. 155 genes in the interactome, including 9 host protein-encoding genes, and 121 known interactors and 25 novel interactors of host proteins, were found to be associated with 35 disorders (overlap of each disease had p-value < 0.05). This included 13 types of cancers, 7 metabolic disorders, 4 neurological disorders, 3 developmental disorders, 2 eye-related disorders, 2 vascular diseases, 1 infectious disease, 1 inflammatory disorder, 1 respiratory disorder and 1 skin disease (Figure 6; Table 1). Some of these diseases enriched in the interactome are co-morbidities among non-survivors and critically ill COVID patients (e.g., diabetes, hypertension, cerebrovascular events and cancer) (Fang et al., 2020b; Sidaway, 2020). 13 genes in the interactome were associated with non-insulin dependent diabetes mellitus (odds ratio = 10.8, p-value = 4.38 × 10−10), 6 genes with essential hypertension (odds ratio = 12, p-value = 2.34 × 10−5), 3 genes with ischemic stroke (odds ratio = 14.4, p-value = 1.7 × 10−3) and 10 genes with lung cancer (odds ratio = 14.1, p-value = 2.36 × 10−9). Network proximity of the proteins associated with these co-morbid conditions to the SARS-CoV-2 host proteins may explain why patients with these conditions are increasingly affected by the viral infection. Further investigations are necessary to dissect these co-morbidities. Treatment strategies that prevent the deterioration of the underlying genetic conditions must be devised to combat COVID-19 in susceptible individuals. Additionally, neurological disorders such as Alzheimer’s disease (odds ratio = 15.3, p-value = 5.13 × 10−7) and schizophrenia (odds ratio = 12, p-value = 4.19 × 10−6) were also found to be enriched in the interactome, warranting further investigations into these potential co-morbidities.
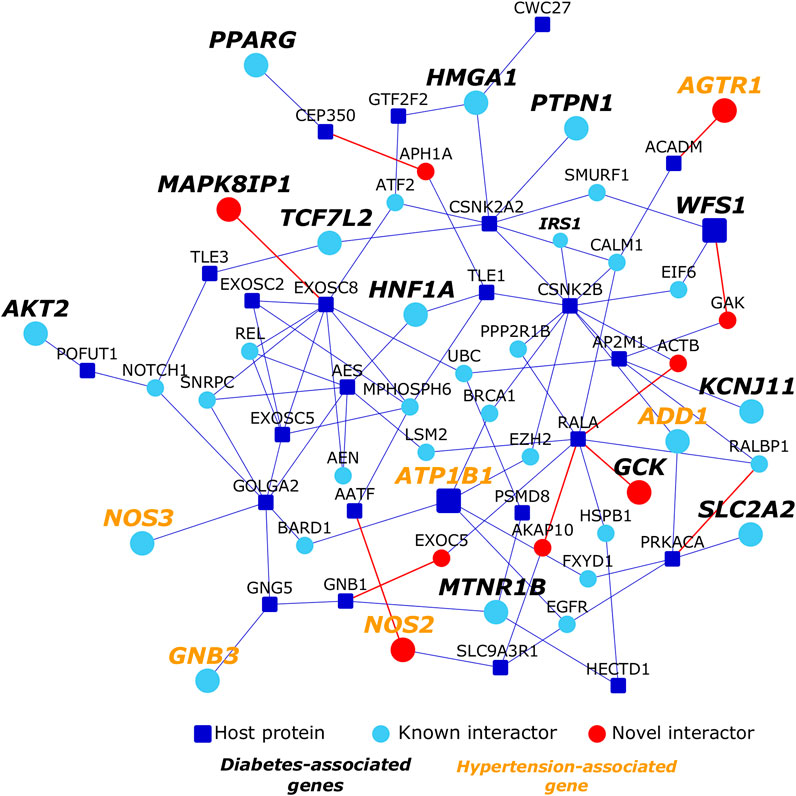
FIGURE 6. Network proximity of diabetes and hypertension to host proteins targeted by SARS-CoV-2: Dark blue nodes are host proteins targeted by SARS-CoV-2, light blue nodes are known interactors and red nodes are novel interactors. Nodes with bold black italicized labels are diabetes-associated genes, whereas nodes with bold orange colored italicized labels are hypertension-associated genes.
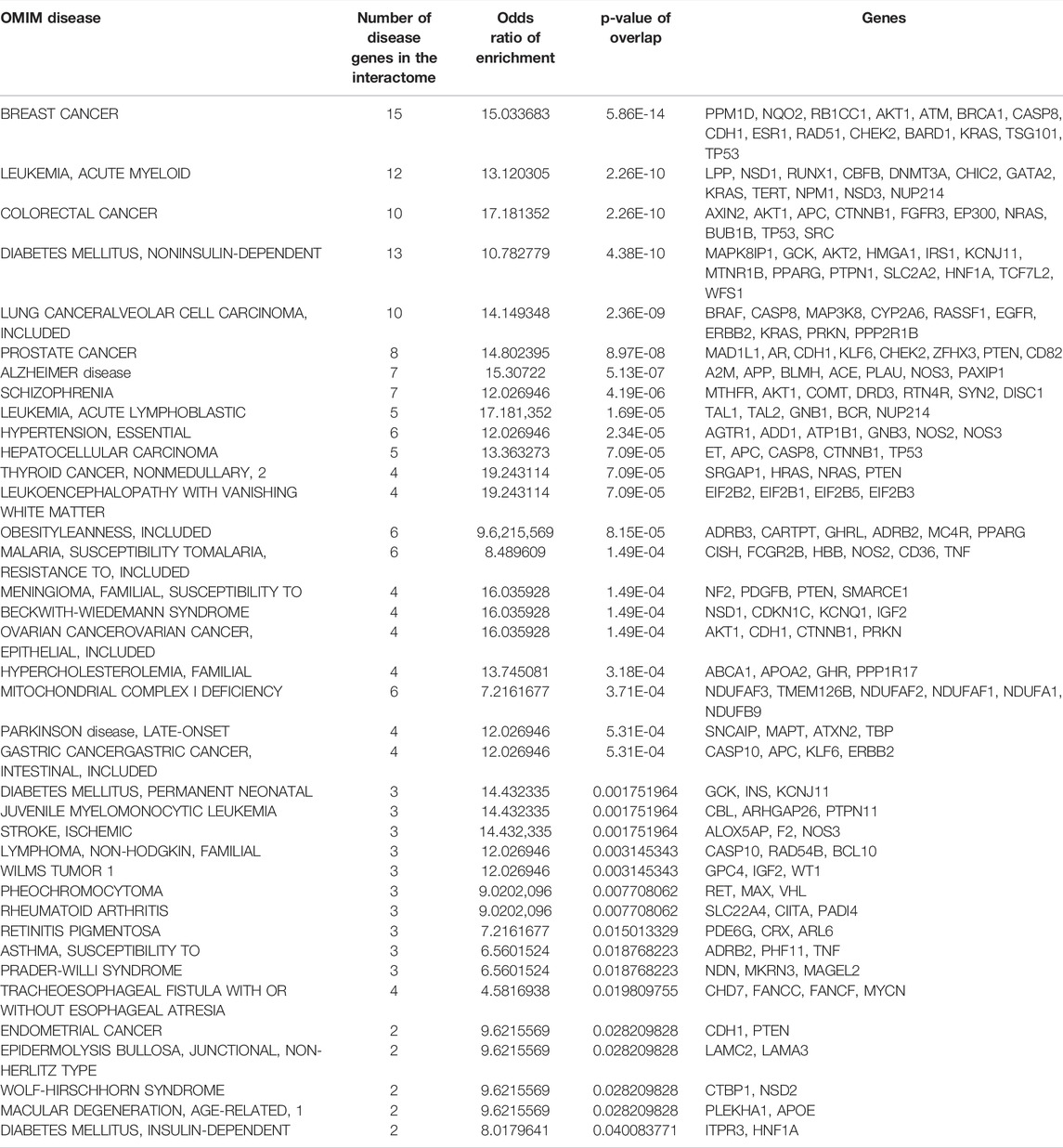
TABLE 1. List of OMIM diseases enriched in the interactome: Details of enrichment of the disease genes in the interactome including the number of disease-associated genes, odds ratio and statistical significance (p-value) of enrichment are shown.
2.7 Subcellular locations
Gene Ontology enrichment analysis of the interactome identified several subcellular locations that may be targeted by SARS-CoV-2. Cellular locations included points of virus entry such as the cell-substrate junction, nuclear periphery and specific sites from where viral proteins may potentiate viral replication, gene expression and modulate the immune response of the host such as the midbody, nuclear chromatin and PML (promyelocytic leukaemia) body (each term with p-value < 1 × 10−4). PML bodies are nuclear sub-compartments that repress viral replication through entrapment or epigenetic silencing of the viral genomes (Scherer and Stamminger, 2016). Components of PML bodies activate interferon-stimulated genes and cytokines, and may also be upregulated on induction of interferons (Scherer and Stamminger, 2016). Viruses have been known to target PML bodies to circumvent the anti-viral defences of the host cell (Scherer and Stamminger, 2016). 61 proteins in the HoP interactome are PML components. These include the host protein AKAP8L, which has been known to promote retroviral gene expression, and 55 known interactors and 5 novel interactors (RNF111, SP140, ELF4, NFE2, and CIART) of other host proteins targeted by SARS-CoV-2. Our model predicted an interaction of EIF4E2 with SP140, an interferon-inducible PML component; SARS-CoV-2 may target these proteins. The midbody is a microtubule-rich structure that connects the daughter cells and marks the site of abscission during cytokinesis. Viruses have been known to recruit certain protein complexes that also localize to the midbody during cytokinesis, to the host cell membrane to promote its scission and thereby the release of viruses (Morita et al., 2010). This co-opting of proteins may explain the enrichment of midbody proteins in the HoP interactome. 83 proteins in the HoP interactome, including 11 host proteins (RHOA, CENPF, CIT, RAB8A, NUP62, SCCPDH, SPART, RDX, ARF6, CNTRL and RALA), 63 known interactors and 9 novel interactors (KIF4A, BIRC5, INCENP, ALKHB4, DNM2, DDX11, ARL2BP, ABRAXAS2 and WIS) are known to localize to the midbody.
2.8 Cellular processes
Enriched biological processes in the interactome included G1/S and G2/M mitotic cell cycle phase transitions, regulation of vesicle-mediated transport, covalent chromatin modification and nuclear transport (p-value < 1 × 10−4). The response of the host cell to SARS-CoV-2 infection has been shown to be significantly delayed and devoid of several anti-viral mechanisms (Blanco-Melo et al., 2020a). During early stages of the infection, it is possible that the virus induces a G1/S phase transition to surreptitiously synergize the replication of the viral genome with that of the host genome (Fan et al., 2018). In the later stages, it may block the G2/M phase transition to maximise the levels of viral genome (Fan et al., 2018). We found novel interactions of host proteins with 34 proteins involved in cell cycle phase transition: ANAPC4, ANAPC7, ARPP19, CCNB3, CDC14B, CDC16, CDC7, CEP164, CETN2, CLSPN, CRLF3, DCTN1, DNM2, DYNC1H1, E2F6, ENSA, FBXL7, GFI1, GML, HYAL1, INHBA, JADE1, NEUROG1, NPAT, ORC2, PPM1D, RAD17, SPDYA, TAOK2, TICRR, TRIAP1, XPC, ZFP36L1 and ZNF655. Corroborating our hypothesis, a significantly large number of Vero E6 cells infected with SARS-CoV-2 were found to be in S and the G2/M phases, indicating that SARS-CoV-2 may induce cell cycle arrest between S and G2 phases to promote infection (Bouhaddou et al., 2020).
2.9 Cellular pathways
The HoP interactome showed a statistically significant enrichment of several pathways related to viral entry and infection such as infectious disease, HIV life cycle, vesicle-mediated transport and membrane trafficking (Figure 7). Several immunity-related pathways that mediate host response such as MyD88 dependent TLR4 signalling and ISG15 anti-viral mechanism were also identified.
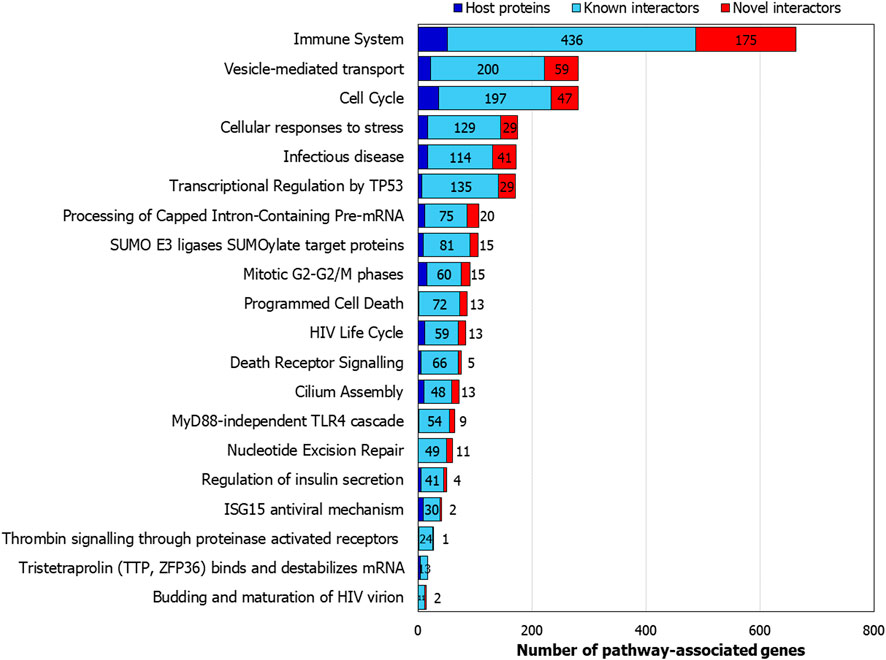
FIGURE 7. Pathways associated with the host protein interactome: Number of genes from the interactome associated with selected pathways are shown.
The transcriptional profile of the host cell after SARS-CoV-2 infection had revealed a remarkably limited anti-viral response compared to that elicited by seasonal influenza-A and respiratory syncytial viruses (Blanco-Melo et al., 2020a). This prompted us to inspect a post-transcriptional regulatory pathway that was enriched in the HoP interactome, namely, the Reactome pathway called tristetraprolin (ZFP36) binds and destabilizes mRNA (p-value < 1 × 10−4). ZFP36 is an RNA-binding protein that targets AU-rich sites in the mRNA transcripts coding for immune proteins and destabilizes them by promoting deadenylation of their poly (A) tails (Moore et al., 2018; Blackshear, 2002). YWHAB increases cytoplasmic localization of ZFP36, possibly preventing destabilization of these genes and attenuation of immune response (Brook et al., 2006). We extracted the direct PPIs of the 17 genes belonging to this pathway from the HoP interactome and isolated this sub-network for further inspection (Figure 8). Our predictions showed that the host protein DCAF7, which is known to function as a scaffold protein and a facilitator of PPIs, interacted with YWHAB (Figure 8). This raised the possibility that the virus protein Nsp9 (which interacts with DCAF7) may somehow perturb YWHAB-induced cytoplasmic localization of ZFP36 through its action on DCAF7. Nsp9 may activate or promote the sequestration of YWHAB with DCAF7, thereby reducing its capacity to form a complex with ZFP36. ZFP36-mediated destabilization of immune genes may then lead to a weakened immune response, creating an environment conducive for SARS-CoV-2 infection. We also identified 3 drugs targeting the proteins in this sub-network using Drug Bank (Wishart et al., 2008): resveratrol targeting KHSRP and APP, known interactors of the host protein EXOSC2, which is involved in the tristetraprolin (TTP) pathway, staurosporine targeting TTP-associated MAPKAPK2, which has been predicted to interact with PABPC1, and dacarbazine targeting the host protein POLA2 (Figure 8). Gene expression profiles induced by these drugs in various cell lines were found to have a negative correlation with SARS-associated gene expression profiles, namely, that of lung fibroblast MRC5 cells infected with SARS-CoV and peripheral blood mononuclear cells of SARS patients (analysis using NextBio; https://www.nextbio.com) (Kupershmidt et al., 2010; Chattopadhyay and Ganapathiraju, 2017). Resveratrol has been proposed as a therapeutic option for SARS-CoV-2 based on its antagonistic properties against MERS-CoV (Lin et al., 2017).
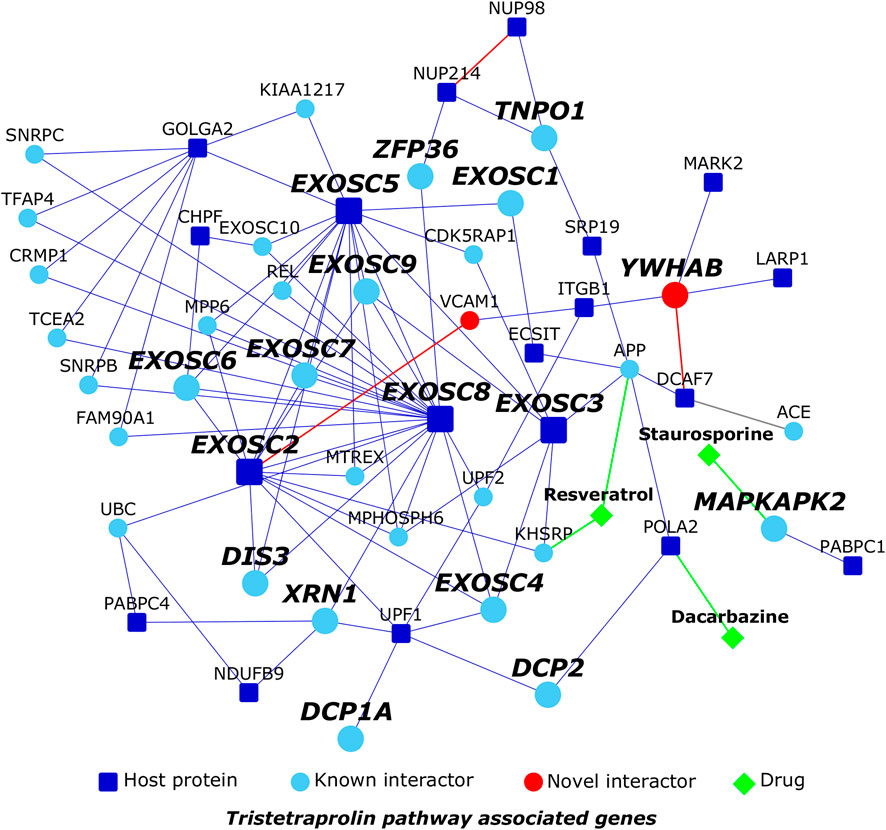
FIGURE 8. Tristetraprolin pathway: Dark blue nodes are host proteins targeted by SARS-CoV-2, light blue nodes are known interactors, and red nodes are novel interactors. Diamond-shaped green colored nodes depict drugs. Nodes with bold black italicized labels are proteins involved in the tristetraprolin pathway.
2.10 Interconnections of Ciliary Proteins With Host Proteins Targeted by SARS-CoV-2
SARS coronavirus, which emerged in 2002, has been known to induce necrosis in ciliated airway epithelium of humans in a species-specific manner (Sims et al., 2005). SARS-CoV-2’s host receptor ACE2 is highly expressed in ciliated respiratory cells (Sungnak et al., 2020). Cilia may serve as virus entry points and potential modulators of viral pathogenesis. This conjecture prompted us to investigate the ciliary association of the host proteins targeted by SARS-CoV-2 and their interactors in the HoP interactome. For this, we studied its overlap with an interactome of 165 ciliary proteins that we constructed in a similar manner (Karunakaran et al., 2020). The ciliary protein interactome contained 1,665 proteins. 617 of these proteins, and specifically 30 core ciliary proteins, were also found in SARS-CoV-2’s host protein interactome, and the overlap was found to be statistically significant (p-value = 2.24E-10, odds ratio = 1.22). 14 novel predicted interactions connected host proteins to ciliary proteins: NUP98-CHMP5, GG3BP1-DNAH1, SEPSECS-DNAH1, NEK9-IFT43, TLE1-DNAH5, ATP6AP1-CETN2, C1orf50-ZMYND12, RAB10-IFT172, TOR1AIP1-GPR161, DNAJC19-CETN3, NLRX1-IFT46, FKBP7-TTC30B, POLA2-TMEM216 and NDUFB9-DRC7.
Pathway analysis of the 617 common proteins (i.e., common to HoP and cilia interactomes) revealed two interesting pathways: budding and maturation of HIV virion (p-value = 1.29 × 10−6; odds ratio = 8.8) and anti-viral mechanism by IFN-stimulated genes (p-value = 1.3 × 10−2; odds ratio = 2.98). We predicted that the ciliary protein, CHMP5, involved in the former pathway, interacts with the host protein, NUP98, which is involved in the latter pathway. This prompted us to ask whether the predicted interaction connected the functional modules of viral budding to interferon (IFN) signaling.
2.10.1 Novel Interaction of NUP98 With CHMP5 May Activate an IFN-Stimulated Pathway That Interferes With Viral Budding
We extracted the PPIs of the 20 proteins belonging to viral budding and IFN pathways, and isolated this sub-network containing 171 proteins and 176 PPIs for further analysis. Firstly, we identified 343 functional interactions (i.e., activation, inhibition etc.) among 98 proteins in the network. Strikingly, distinct functional modules were identified for both the pathways; CHMP5 seemed to serve as a connector from the viral budding pathway to the IFN pathway through NUP98 (Figure 9). The gene UBC was shared between the clusters.
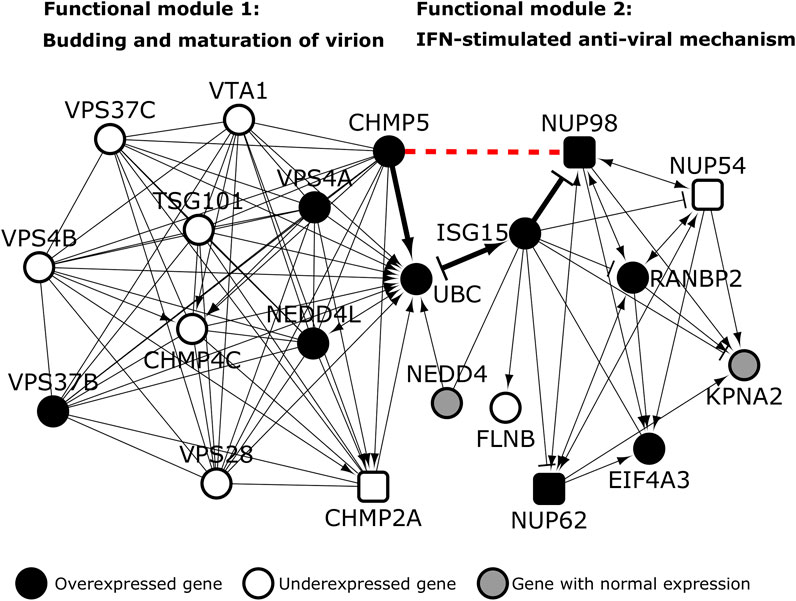
FIGURE 9. Functional modules of viral budding and interferon-mediated anti-viral pathway: Host proteins are depicted as square shaped nodes. The novel predicted interaction of the host protein NUP98 with CHMP5 (red dashed line) may set off an ISG15-mediated pathway that culminates in interference of viral budding. Black colored nodes and transparent nodes indicate overexpression and underexpression in SARS-CoV-infected Calu-3 lung cells respectively. Grey colored nodes depict genes with normal expression. → indicates “activation,” -| indicates “inhibition” and—indicates “part of the same complex/physical association”.
We then checked whether the genes in these modules were differentially expressed in Calu-3 lung cells infected with SARS CoV Urbani (for 72 h) versus mock infected cells. This was done to identify the functional interactions that may remain active during viral infection. It was assumed that differential expression of the genes would directly impact the proteins encoded by them and their interactions. 20 genes including NUP98 and CHMP5 were found to be differentially expressed (Figure 9). Viruses hijack the ESCRT/VPS4 (endosomal sorting complex required for transport) machinery of the host cell to release viral particles through membrane scission (Pincetic et al., 2010). This machinery is normally recruited during endocytic and membrane repair processes in the host cell. The process of membrane scission is catalyzed by various ESCRT-III proteins including CHMP5 (Pincetic et al., 2010). VPS4 is an ATPase that is found in the cytoplasm in its inactive form. Activation of the VPS4 and its ATPase activity is essential for membrane budding and the release of viral particles (Pincetic et al., 2010). VPS4 is activated on membranes in the presence of its co-activator VTA (also known as LIP5). VTA is delivered to the membranes by ESCRT-III proteins such as CHMP5 (Pincetic et al., 2010). Hence, the interaction of VPS4 and VTA is facilitated by CHMP5. However, when interferons are induced in the host cell following viral infection, ISGs (interferon stimulated genes) such as ISG15, a dimer homologue of ubiquitin, may be activated (Pincetic et al., 2010). ISG15 may then conjugate to CHMP5 and promote its accumulation in the membrane, effectively blocking the interaction of VTA with VPS4 and preventing viral budding (Pincetic et al., 2010). The novel interaction of CHMP5 with NUP98 may serve as the critical juncture at which the IFN-stimulated anti-viral mechanism interferes with viral budding. NUP98, a protein induced on viral expression, has been shown to promote anti-viral gene expression in drosophila (Panda et al., 2014). Both CHMP5 and NUP98 are overexpressed following SARS-CoV Urbani infection. This interaction may serve as a signal for the initiation of ISG15-mediated interference of viral budding. ISG15 may further regulate this mechanism through feedback inhibition of NUP98. Hence, potentiation of this anti-viral mechanism through administration of recombinant interferon alfa-2b and interferon alfacon-1 may be a feasible therapeutic option for SARS-CoV-2. Both these interferons induce gene expression profiles negatively correlated with SARS-associated profiles. The machinery of ESCRT-III and VPS4 is co-opted into two subcellular structures that are intricately linked to cilia function, namely, the centrosomes and the midbody (Morita et al., 2010). It is important to study these structures as potential modulators of viral infections.
2.11 Potentially Repurposable Drugs
We followed the established approach of comparing drug-induced versus disease-associated differential expression (Sirota et al., 2011) to identify drugs for SARS-CoV-2. For this, we used a software suite called BaseSpace Correlation Engine (previously called NextBio) (https://www.nextbio.com) (Kupershmidt et al., 2010; Chattopadhyay and Ganapathiraju, 2017). This data analysis platform was used because it allows users to study the effect of diseases and/or drugs on thousands of pre-processed publicly available gene expression datasets and has helped to identify drug candidates for diseases such as schizophrenia (Karunakaran et al., 2019b) (currently undergoing clinical trials (Vishwajit Nimgaonkar, 2022; Vishwajit Nimgaonkar, 2024)) and mesothelioma (Karunakaran et al., 2021) in the past. We compiled a list of 933 chemical compounds whose differential gene expression profiles (drug versus no drug) were negatively correlated with at least one of the four SARS differential gene expression datasets (infected versus non-infected); the 4 SARS datasets we studied were: Calu-3 epithelial cells infected for 48 h with SARS-CoV versus mock infected cells (GSE17400), Calu-3 lung cells infected for 72 h with SARS-CoV Urbani versus mock infected cells (GSE37827), lung fibroblast MRC5 cells 24 h post SARS-CoV infection (high MOI) versus mock infection (GSE56189) and PBMCs from SARS patients versus healthy subjects [GSE1739 (Reghunathan et al., 2005)]. We also compiled a list of 381 chemical compounds with gene expression profiles negatively correlated with the profile induced in human bronchial epithelial (NHBE) and lung cancer (A549) cells infected with the SARS-CoV-2 strain USA-WA1/2020 [GSE147507 (Blanco-Melo et al., 2020a)] Although in each case, there would be some genes that are differentially expressed in the same direction for both the drug and the disease (i.e., both cause some genes to overexpress, or both cause some genes to under express), the overall effect on the entire transcriptome would be an anti-correlation. A correlation score is generated by NextBio based on the strength of the overlap between the drug and disease datasets. Statistical criteria such as correction for multiple hypothesis testing are applied and the correlated datasets are then ranked by statistical significance. A numerical score of 100 is assigned to the most significant result, and the scores of the other results are normalized with respect to this top-ranked result.
Next, we identified 1,130 drugs that target at least one protein in the HoP interactome using WebGestalt (Liao et al., 2019). We used the “redundancy reduction” feature provided by WebGestalt to prioritize drugs with highly significant overlaps with the interactome, while also capturing all the unique target gene sets. This feature used an affinity propagation algorithm, which clusters sets of genes in the interactome targeted by specific drugs using Jaccard index as the similarity metric, and identifies a “representative” for each cluster (one drug and its targets), having the most significant p-value among all the gene sets in that cluster. This resulted in 209 drugs for further consideration. Given a class of drugs targeting the same set of proteins, this method ensures that only those individual drugs that target a statistically significant number of proteins in the interactome are prioritized for further analysis.
Fifty-six drugs were found in common to the above two analyses, i.e., these drugs not only targeted genes in the HoP interactome, but also induced gene expression profiles which are negatively correlated with that induced by SARS-CoV (Table 2) and SARS-CoV-2 (Table 3). 13 drugs showed negative correlation with both expression profiles. 24 of these have supporting evidence for biological relevance (see Appendix) through clinical trial data and published literature (Figure 10).
• 4 drugs showed activity against SARS-CoV-2 in vitro (cyclosporine, sorafenib, tamoxifen, anisomycin)
• 1 chemical compound (nitric oxide) found here is already being tested against SARS-CoV-2 in clinical trials
• 1 drug (ramipril) belongs to the class of receptors targeted by SARS-CoV-2
• 5 drugs display clinical activity against SARS or MERS (resveratrol, sirolimus, mycophenolic acid, interferon alpha-2b, interferon alfacon-1)
• 3 drugs (quercetin, verapamil, progesterone) are active against influenza viruses
• 2 drugs are active against DNA viruses (leflunomide, daunorubicin), and
• 8 drugs show activity against other RNA viruses (clotrimazole, didanosine, paclitaxel, fenofibrate, cerivastatin, thioridazine, pioglitazone, miglitol)
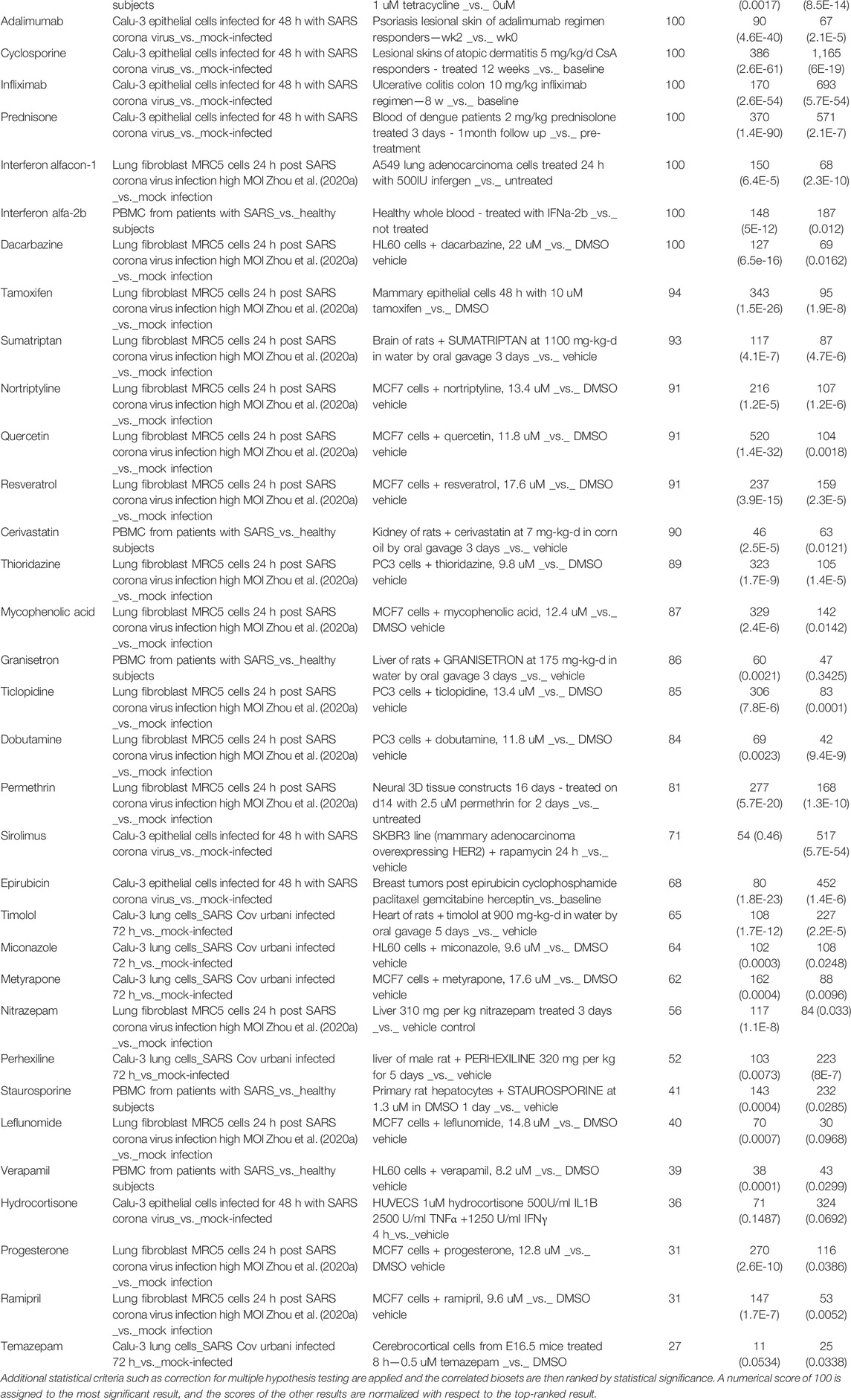
TABLE 2. 51 drugs with expression profiles negatively correlated with SARS-associated profiles: The correlation score is based on the strength of the overlap or enrichment between the two biosets.
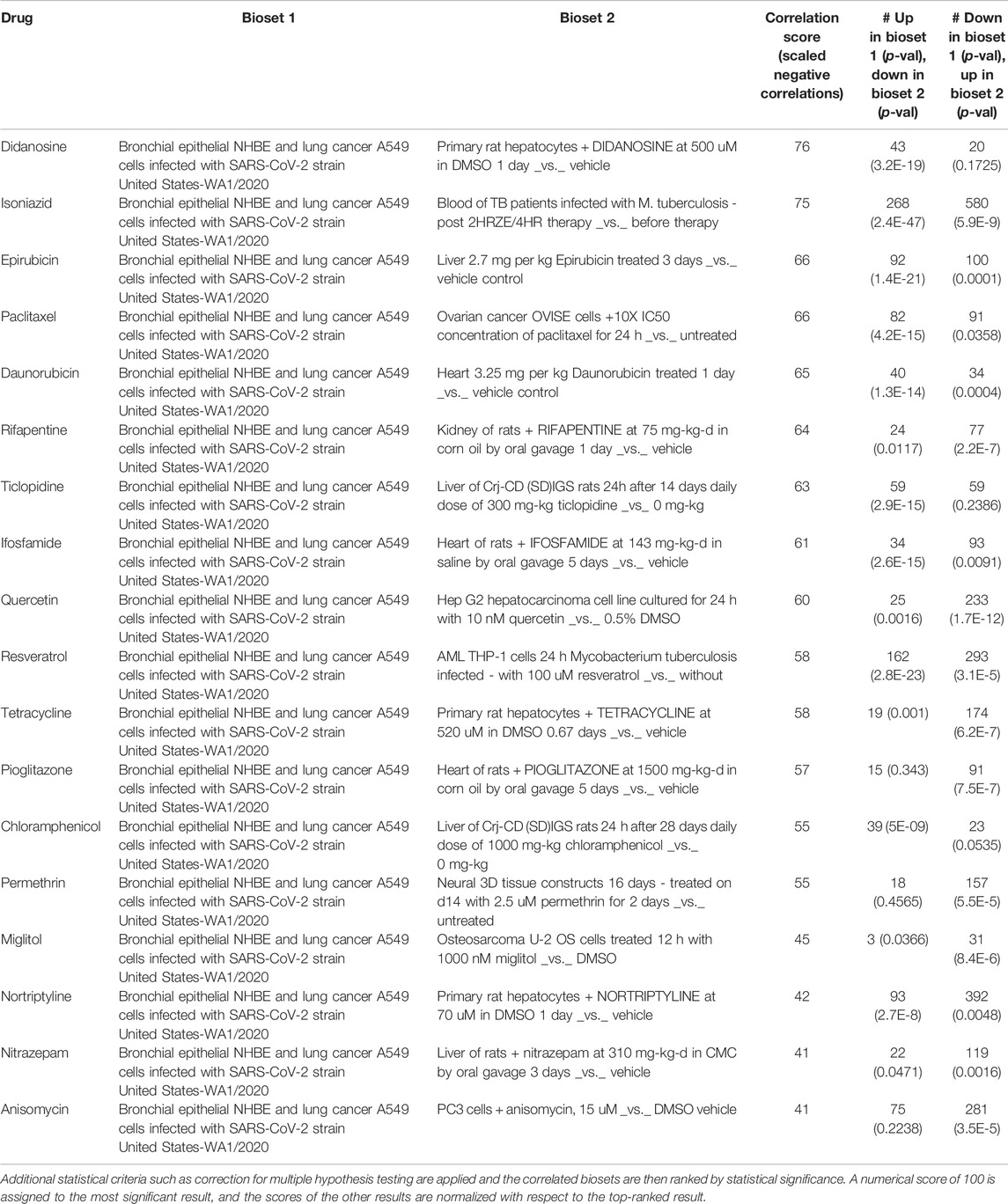
TABLE 3. 18 drugs with expression profiles negatively correlated with COVID-associated profile: The correlation score is based on the strength of the overlap or enrichment between the two biosets.
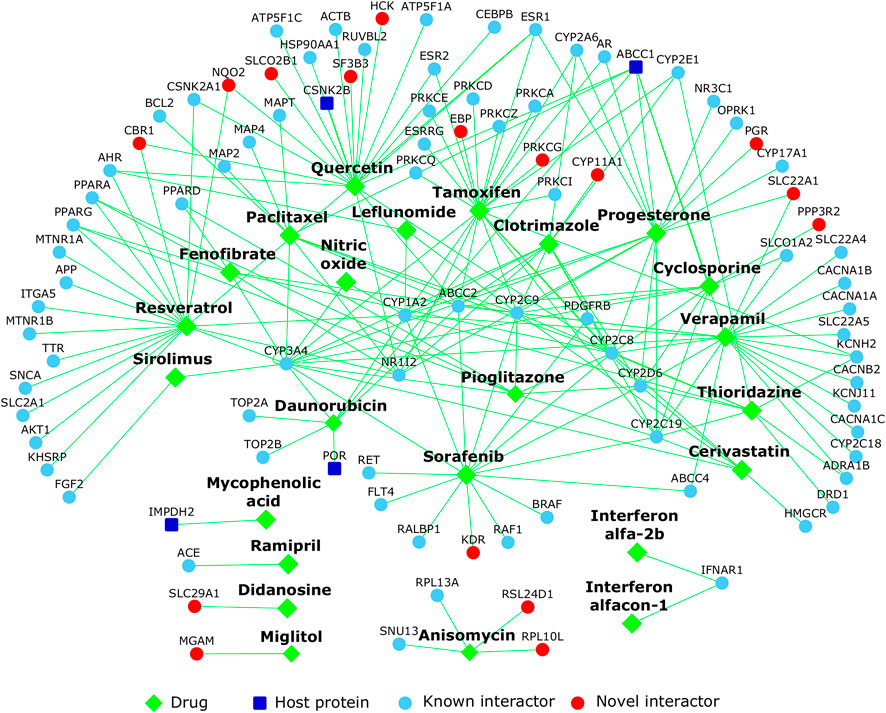
FIGURE 10. Repurposable drugs for COVID-19: The network shows the drugs (diamond shaped green colored nodes) that target the proteins in the host protein interactome. Host proteins targeted by SARS-CoV-2 are shown as dark blue nodes, their known interactors are light blue and novel interactors are red.
Eight drugs from our shortlist were independently identified or prioritized by other groups, namely: leflunomide [Chen et al. (Chen et al., 2021)], sirolimus [Zhou et al. (Zhou et al., 2020a)], leflunomide, quercetin and verapamil [Gysi et al. (Gysi et al., 2020)], interferon alfa-2b, resveratrol, cyclosporine and mycophenolic acid [Li et al. (Li and De Clercq, 2020)]. Additionally, 8 out of the 24 shortlisted drugs were also found among 127 broad-spectrum antiviral drugs active against 80 viruses (https://drugvirus.info/). These are cyclosporine, leflunomide, mycophenolic acid, sirolimus, sorafenib, tamoxifen, anisomycin and verapamil. Fourteen drugs were found to induce expression profiles negatively correlated with the profiles of ICU-admitted COVID-19 patients with ARDS versus non-critical patients on oxygen [GSE172114 (Carapito et al., 2021)], namely, cerivastatin, cyclosporine, didanosine, leflunomide, miglitol, mycophenolic acid, paclitaxel, quercetin, resveratrol, sirolimus, sorafenib, tamoxifen, thioridazine and verapamil. Three drugs—didanosine, miglitol and resveratrol—induced profiles negatively correlated with that of COVID-19 patients in ICU versus healthy subjects [GSE152418 (Arunachalam et al., 2020)]. Additionally 4 drugs (sorafenib, quercetin, verapamil and cerivastatin) induced profiles negatively correlated with the profiles of ICU-admitted COVID-19 patients with ARDS versus those receiving non-intensive care [GSE157103 (Overmyer et al., 2021)] and 2 drugs (resveratrol and didanosine) with profiles of COVID-19 patients critical in ICU with ARDS versus non-critical patients on oxygen [GSE172114 (Carapito et al., 2021)]. These drugs could be examined for their differential clinical activity in critical versus non-critical cases.
3 Discussion
In this study, to gain insight into the biological processes and pathways that may be involved in host response upon SARS-CoV-2 infection, we assembled the interactome of the host proteins targeted by the virus. The host protein (HoP) interactome has ∼4,000 previously known PPIs in addition to ∼2,000 PPIs that we computationally predicted. The interactome and its annotations are made available on the website that is freely accessible, Wiki-Corona. The HoP interactome was found to share large and statistically significant overlaps with gene expression profiles induced by SARS-CoV and SARS-CoV-2. Proteins with tissue-specific gene expression in lungs, spleen, brain and heart were also found in the interactome. Topologically connected modules in the network showed functional association to cilium organization, nuclear transport, ribonucleoprotein complex biogenesis, endosomal transport and epigenetic regulation of gene expression. The interactome was enriched for subcellular locations and host cellular processes that may be targeted by SARS-CoV-2. It also showed significant associations with several disorders including cancers, metabolic, neurological, developmental and vascular disorders. For example, the host proteins were found to directly interact with proteins associated with two co-morbidities, hypertension and diabetes, which are commonly found among COVID-19 non-survivors. Protein biomarkers showing varied expression across the different stages of COVID-19 were predicted as novel interactors of the host proteins targeted by SARS-CoV-2. The SARS-CoV-2 host proteins and ciliary proteins shared several common interactors. The role of cilia as viral entry points and modulators of viral infections should be investigated further on this premise. On further analysis of the shared interactome, we hypothesized that the novel interaction of NUP98 with CHMP5, a ciliary (and centrosome and midbody-localizing) protein, may activate an IFN-stimulated pathway with the potential to interfere with viral budding. We shortlisted drugs potentially repurposable for COVID-19 based on the negative correlation of drug-induced versus disease-associated gene expression profiles. These included drugs with proven in vitro activity against SARS-CoV-2, those that were already being tested for their clinical activity against SARS-CoV-2, those with proven activity against SARS-CoV/MERS-CoV, broad-spectrum antiviral drugs, and those identified/prioritized by other computational re-purposing studies.
Our computational approach has several limitations. Drug-associated expression profiles analyzed in this study were induced in several types of cell lines (including cancer cell lines) that may not be directly relevant to COVID-19 or SARS-CoV-2 infection. The effect of the proposed repurposable drugs should be studied in human bronchial epithelial cells and/or in human lung cancer cell lines, both of which were recently used to study host transcriptional response upon SARS-CoV-2 infection (Blanco-Melo et al., 2020a). The repurposable drugs discussed in this study can simply be identified through comparative transcriptomic analysis; i.e., by comparing drug-induced expression profiles with SARS-CoV/SARS-CoV-2-induced profiles. However, by considering the drugs targeting the proteins in the HoP interactome, we attempted to provide starting points for a mechanistic and experimentally testable basis for the negative correlation observed between the drugs and the viral infection at the transcriptomic level. These starting points are typically subnetworks (e.g., Figure 10) showing interconnections of the drug targets and the host proteins targeted by the virus in the human interactome. The sets of host proteins interacting with SARS-CoV and SARS-CoV-2, which were analyzed in our study to elucidate common pathways targeted by these viruses, were themselves identified using different protein interaction mapping techniques (AP–MS and Y2H) in different studies. These techniques differ from each other with respect to the nature of the PPIs that they detect: AP–MS identifies direct and indirect interactions among members of stable protein complexes, while Y2H may identify direct and more transient interactions between pairs of proteins. Therefore, each of these techniques may detect a different portion of the virus-host interactome. The marginal overlap observed between the sets of host proteins interacting with SARS-CoV and SARS-CoV-2 could be attributed to the differences in the interactome subspaces detected by Y2H and AP–MS, respectively. In this scenario, one may expect the neighborhood networks of these host proteins to also exhibit this discordance. However, we observed extensive interconnections between these sets of proteins, via direct and intermediate (known as well as novel) interactors. This shows that 1) it is the ability of the different techniques to detect different subspaces of the interactome in a complementary manner that makes them valuable, 2) machine learning methods may capture novel PPIs that other techniques fail to capture, and 3) computational methods may be employed to piece together an integrated view of the interactome, despite the limitations of the individual mapping techniques.
The novelty of this work stems from several aspects. 1) Despite an explosive increase in the generation of COVID-19 related data, knowledge on the mechanistic basis of the host cellular response to SARS-CoV-2 infection is limited. Therefore, we prioritized dataset mining and hypothesis generation over data generation by integrating and analyzing publicly available multi-omics data within the functional landscape of the protein interactome using bioinformatic tools. This approach directly contributes towards COVID-19 research prioritization, namely, selection of pathways and drugs for experimental dissection and clinical interventions. 2) Computationally predicted PPIs enhanced hypothesis generation by linking host genes across various high throughput studies in as-yet-undiscovered ways. 3) To facilitate analysis by both computational and biomedical scientists, all the results are being released in multiple data formats in open access and via an interactive webserver (see Data Availability). 4) The HoP interactome will facilitate several future systems biology studies derived from overlaying the interactome with data generated for research on coronaviruses, and specifically on COVID-19. In summary, the interactome will be useful for carrying out several studies in the future with rapidly emerging data to generate biologically insightful results that may be translated to biomedically actionable results.
4 Methods
4.1 Compilation of Host Proteins and Prediction of Novel Interactions
The list of 332 host proteins identified to interact with 27 SARS-CoV-2 proteins was compiled from data files in Gordon et al. (2020a). Novel PPIs of these proteins were predicted using the HiPPIP model that we developed (Ganapathiraju et al., 2016b). Each host protein (say N1) was paired with each of the other human protein say (M1, M2, … Mn), and each pair was evaluated with the HiPPIP model (Ganapathiraju et al., 2016b). The predicted interactions of each of the host proteins were extracted (namely, the pairs whose score is >0.5, a threshold which through computational evaluations and experimental validations was revealed to indicate interacting partners with high confidence). This resulted in 1941 newly discovered PPIs of the host proteins. The interactome figures were created using Cytoscape (Shannon et al., 2003).
The significance of the overlap of this interactome with two datasets, namely, with the ciliary protein interactome and the interactome of 120 genes differentially expressed in SARS-CoV-2-infected A549 cell line (Blanco-Melo et al., 2020a), was computed based on hypergeometric distribution.
4.2 Identification of Network Modules
Network modules among the host proteins targeted by SARS-CoV-2 and their interactors were identified using Netbox (Cerami et al., 2010). Netbox reports modularity and a scaled modularity score, as compared with the modularity observed in 1,000 random permutations of the subnetwork. Scaled modularity refers to the standard deviation difference between the observed subnetwork and the mean modularity of the random networks (Wang and Zhang, 2007).
4.3 Transcriptome and Proteome Analysis
Statistical significance of the overlaps between genes in the HoP interactome and SARS-CoV/SARS-CoV-2 induced/associated transcriptomic/proteomic datasets was computed based on hypergeometric distribution. In this method, p-value is computed from the probability of k successes in n draws (without replacement) from a finite population of size N containing exactly K objects with an interesting feature.
N = Total number of genes/proteins assayed.
K = Number of SARS-CoV/SARS-CoV-2-induced/associated genes/proteins.
n = Number of genes in the HoP interactome.
k = K ∩ n.
4.4 Tissue-Specificity Analysis
Tissue-specificity of the genes in the HoP interactome were checked using TissueEnrich (Jain and Tuteja, 2019). The analysis was based on genes from the GTEx database (Lonsdale et al., 2013). This included ‘“tissue-enriched genes” with at least 5-fold higher mRNA levels in a particular tissue compared to all the other tissues, “group-enriched genes” with at least 5-fold higher mRNA levels in a group of 2-7 tissues and “tissue-enhanced genes” with at least 5-fold higher mRNA levels in a particular tissue compared to average levels in all tissues.
4.5 Functional Enrichment Analysis
Gene Ontology, Pathway and genetic disorder enrichments were computed using WebGestalt (Liao et al., 2019). WebGestalt computes the distribution of genes belonging to a particular functional category in the input list (i.e., genes in the HoP interactome/ACE2 and its interactors) and compares it with the background distribution of genes belonging to this functional category among all the genes that belongs to any functional category in the database selected by the user. Statistical significance of functional category enrichment is computed using Fisher’s exact test, and corrected using the Benjamini–Hochberg method for multiple test adjustment. Annotations with FDR-corrected p-value < 0.05 were considered significant. ReactomeFIViz, a Cytoscape plugin, was used to extract known functional interactions among genes in the HoP interactome that were involved in viral budding and interferon signaling pathways (Wu et al., 2014).
4.6 Potentially Repurposable Drugs
The list of chemical compounds whose gene expression profiles correlated negatively with four SARS datatsets and one COVID-19 dataset were compiled using the BaseSpace correlation software (https://www.nextbio.com) (List 1). The datasets considered were human bronchial epithelial (NHBE) and lung cancer (A549) cells infected with the SARS-CoV-2 strain USA-WA1/2020 [GSE147507 (Blanco-Melo et al., 2020a)], Calu-3 epithelial cells infected for 48 h with SARS-CoV versus mock infected cells (GSE17400), Calu-3 lung cells infected for 72 h with SARS-CoV Urbani versus mock infected cells (GSE37827), lung fibroblast MRC5 cells 24 h post SARS-CoV infection (high MOI) versus mock infection (GSE56189) and peripheral blood mononuclear cells (PBMCs) from patients with SARS versus healthy subjects [GSE1739 (Reghunathan et al., 2005)]. Next, we identified drugs that targeted at least one gene in the HoP interactome using WebGestalt (Liao et al., 2019). After employing the “redundancy reduction” feature in WebGestalt to reduce the search space of drugs, we were left with a fewer number of drugs (List 2). In this feature, an affinity propagation algorithm clusters gene sets in the interactome targeted by specific drugs using Jaccard index as the similarity metric, and identifies a “representative” for each cluster (one drug and its targets), having the most significant p-value among all the gene sets in that cluster. We then compared list 1 and list 2 to identify the drugs that not only target proteins in the interactome but are also negatively correlated with SARS/COVID-19.
List of drugs validated to be effective against SARS-CoV-2 in cell-based assays were obtained from the COVID-19 Gene and Drug Set Library (https://amp.pharm.mssm.edu/covid19/) (Kuleshov et al., 2020).
The drug-protein interactome figure was created using Cytoscape (Shannon et al., 2003).
Data Availability Statement
The interactome, consisting of both known and novel computationally predicted protein-protein interactions, is being released via a webserver (http://severus.dbmi.pitt.edu/corona/) and as Supplementary Table S1. The membership of the proteins in the additional data sources referred to in this work can be found in Supplementary Table S2. Further inquiries can be directed to the corresponding author.
Author Contributions
MKG carried out interactome construction, web-based dissemination, and other algorithmic analyses. KBK carried out bioinformatic analysis over the interactome with multiple omics data, including its study design and interpretation, with supervision from NB and MKG. Manuscript has been drafted by KBK and edited and approved by all authors.
Funding
MKG's effort was supported by the Genomics Analysis Core facility and by the Department of Biomedical Informatics of University of Pittsburgh. A part of this work is based on the results from the project funded by the Biobehavioral Research Awards for Innovative New Scientists (BRAINS) grant R01MH094564 awarded to MKG by the National Institute of Mental Health of National Institutes of Health (NIMH/NIH) of United States. The content is solely the responsibility of the authors and does not necessarily represent the official views of NIMH.
Conflict of Interest
The authors declare that the research was conducted in the absence of any commercial or financial relationships that could be construed as a potential conflict of interest.
Publisher’s Note
All claims expressed in this article are solely those of the authors and do not necessarily represent those of their affiliated organizations, or those of the publisher, the editors and the reviewers. Any product that may be evaluated in this article, or claim that may be made by its manufacturer, is not guaranteed or endorsed by the publisher.
Acknowledgments
MKG acknowledges the contribution of past graduate and undergraduate students who have worked on website and database development and system administration, whose neat work has made it possible to post these results on this website. The content is solely the responsibility of the authors and does not necessarily represent the official views of the National Institute of Mental Health, the National Institutes of Health.
Supplementary Material
The Supplementary Material for this article can be found online at: https://www.frontiersin.org/articles/10.3389/fsysb.2022.815237/full#supplementary-material
Supplementary Figure S1 | Network proximity of cellular entry proteins and host proteins targeted by SARS-CoV-2: Dark blue nodes are host proteins targeted by SARS-CoV-2, light blue nodes are known interactors and red nodes are novel interactors. Brown-colored nodes with bold black italicized labels are the “cellular entry proteins” that facilitate the entry of SARS-CoV-2 into host cells.
Supplementary Table S1 | List of proteins and protein-protein interactions in the host protein interactome, and the integrated interactome with virus-host PPIs and PPIs in the neighborhood network of host proteins: Computationally predicted interactors and interactions are indicated as “novel interactors” and “novel PPIs” respectively, whereas previously known interactors and interactions are shown as “known interactors” and “known PPIs”.
Supplementary Table S2 | Complete list of SARS/COVID-related biological evidences of genes in the host protein interactome: A tick mark indicates the presence of a particular evidence for a given gene.
References
Amaral, L., Viveiros, M., and Molnar, J. (2004). Antimicrobial Activity of Phenothiazines. In Vivo 18 (6), 725–731.
Arunachalam, P. S., Wimmers, F., Mok, C. K. P., Perera, R. A. P. M., Scott, M., Hagan, T., et al. (2020). Systems Biological Assessment of Immunity to Mild versus Severe COVID-19 Infection in Humans. Science 369 (6508), 1210–1220. doi:10.1126/science.abc6261
Barabási, A.-L., Gulbahce, N., and Loscalzo, J. (2011). Network Medicine: a Network-Based Approach to Human Disease. Nat. Rev. Genet. 12 (1), 56–68. doi:10.1038/nrg2918
Blackshear, P. J. (2002). Tristetraprolin and Other CCCH Tandem Zinc-finger Proteins in the Regulation of mRNA Turnover. Biochem. Soc. Trans. 30 (Pt 6), 945–952. doi:10.1042/bst0300945
Blanco-Melo, D., Nilsson-Payant, B. E., Liu, W-C., Møller, R., Panis, M., Sachs, D., et al. (2020). SARS-CoV-2 Launches a Unique Transcriptional Signature from in Vitro, Ex Vivo, and in Vivo Systems. Cambridge, Massachusetts: Cell.
Blanco-Melo, D., Nilsson-Payant, B. E., Liu, W. C., Uhl, S., Hoagland, D., Møller, R., et al. (2020). Imbalanced Host Response to SARS-CoV-2 Drives Development of COVID-19. Cell 181, 1036–e9. doi:10.1016/j.cell.2020.04.026
Blasche, S., and Koegl, M. (2013). Analysis of Protein-Protein Interactions Using LUMIER Assays. Virus-Host Interactions: Methods Protoc. 1, 17–27. doi:10.1007/978-1-62703-601-6_2
Bojkova, D., Klann, K., Koch, B., Widera, M., Krause, D., and Ciesek, S. (2020). SARS-CoV-2 Infected Host Cell Proteomics Reveal Potential Therapy Targets. Nature 583 (7816), 469–472.
Bouhaddou, M., Memon, D., Meyer, B., White, K. M., Rezelj, V. V., Correa Marrero, M., et al. (2020). The Global Phosphorylation Landscape of SARS-CoV-2 Infection. Cell. 182 (3), 685–712. e19. doi:10.1016/j.cell.2020.06.034
Brook, M., Tchen, C. R., Santalucia, T., McIlrath, J., Arthur, J. S. C., Saklatvala, J., et al. (2006). Posttranslational Regulation of Tristetraprolin Subcellular Localization and Protein Stability by P38 Mitogen-Activated Protein Kinase and Extracellular Signal-Regulated Kinase Pathways. Mol. Cell Biol. 26 (6), 2408–2418. doi:10.1128/mcb.26.6.2408-2418.2006
Burrell, L. M., Johnston, C. I., Tikellis, C., and Cooper, M. E. (2004). ACE2, a New Regulator of the Renin-Angiotensin System. Trends Endocrinol. Metab. 15 (4), 166–169. doi:10.1016/j.tem.2004.03.001
Cantuti-Castelvetri, L., Ojha, R., Pedro, L. D., Djannatian, M., Franz, J., Kuivanen, S., et al. (2020). Neuropilin-1 Facilitates SARS-CoV-2 Cell Entry and Infectivity. Science 370 (6518), 856–860. doi:10.1126/science.abd2985
Carapito, R., Li, R., Helms, J., Carapito, C., Gujja, S., Rolli, V., et al. (2021). Identification of Driver Genes for Critical Forms of COVID-19 in a Deeply Phenotyped Young Patient Cohort. Sci. Translational Med. 1, eabj7521.
Cerami, E., Demir, E., Schultz, N., Taylor, B. S., and Sander, C. (2010). Automated Network Analysis Identifies Core Pathways in Glioblastoma. PloS one 5, e8918. doi:10.1371/journal.pone.0008918
Cham, L. B., Friedrich, S.-K., Adomati, T., Bhat, H., Schiller, M., Bergerhausen, M., et al. (2019). Tamoxifen Protects from Vesicular Stomatitis Virus Infection. Pharmaceuticals 12 (4), 142. doi:10.3390/ph12040142
Chang, J., Warren, T. K., Zhao, X., Gill, T., Guo, F., Wang, L., et al. (2013). Small Molecule Inhibitors of ER α-glucosidases Are Active against Multiple Hemorrhagic Fever Viruses. Antivir. Res. 98 (3), 432–440. doi:10.1016/j.antiviral.2013.03.023
Chattopadhyay, A., and Ganapathiraju, M. (2017). Demonstration Study: A Protocol to Combine Online Tools and Databases for Identifying Potentially Repurposable Drugs. Data 2 (2), 15. doi:10.3390/data2020015
Chen, F., Shi, Q., Pei, F., Vogt, A., Porritt, R. A., Garcia, G., et al. (2021). A Systems-Level Study Reveals Host-Targeted Repurposable Drugs against SARS-CoV-2 Infection. Mol. Syst. Biol. 17 (8), e10239. doi:10.15252/msb.202110239
Cheng, K.-W., Cheng, S.-C., Chen, W.-Y., Lin, M.-H., Chuang, S.-J., Cheng, I.-H., et al. (2015). Thiopurine Analogs and Mycophenolic Acid Synergistically Inhibit the Papain-like Protease of Middle East Respiratory Syndrome Coronavirus. Antivir. Res. 115, 9–16. doi:10.1016/j.antiviral.2014.12.011
Cho, H., Kim, J., Kim, S., Kyaw, Y., Win, A., and Cheong, J. (2015). Sorafenib Suppresses Hepatitis B Virus Gene Expression via Inhibiting JNK Pathway. Hepatoma Res. 1, 97.
Cui, C., Huang, C., Zhou, W., Ji, X., Zhang, F., Wang, L., et al. (2020). AGTR2, One Possible Novel Key Gene for the Entry of SARS-CoV-2 into Human Cells. IEEE/ACM transactions on computational biology and bioinformatics.
Deng, M., Zhang, K., Mehta, S., Chen, T., and Sun, F. (2003). Prediction of Protein Function Using Protein-Protein Interaction Data. J. Comput. Biol. 10 (6), 947–960. doi:10.1089/106652703322756168
Dunham, B., and Ganapathiraju, M. K. (2022). Benchmark Evaluation of Protein–Protein Interaction Prediction Algorithms. Molecules 27 (1), 41.
Ellinger, B., and Zaliani, A. (2020). Identification of Inhibitors of SARS-CoV-2 In-Vitro Cellular Toxicity in Human (Caco-2) Cells Using a Large Scale Drug Repurposing Collection. Res. Square 1, 10.
Emamjomeh, A., Goliaei, B., Torkamani, A., Ebrahimpour, R., Mohammadi, N., and Parsian, A. (2014). Protein-protein Interaction Prediction by Combined Analysis of Genomic and Conservation Information. Genes Genet. Syst. 89 (6), 259–272. doi:10.1266/ggs.89.259
Españo, E., Nam, J.-H., Song, E.-J., Song, D., Lee, C.-K., and Kim, J.-K. (2019). Lipophilic Statins Inhibit Zika Virus Production in Vero Cells. Sci. Rep. 9 (1), 11461. doi:10.1038/s41598-019-47956-1
Fagerberg, L., Hallström, B. M., Oksvold, P., Kampf, C., Djureinovic, D., Odeberg, J., et al. (2014). Analysis of the Human Tissue-specific Expression by Genome-wide Integration of Transcriptomics and Antibody-Based Proteomics. Mol. Cell Proteomics 13 (2), 397–406. doi:10.1074/mcp.m113.035600
Falzarano, D., De Wit, E., Rasmussen, A. L., Feldmann, F., Okumura, A., Scott, D. P., et al. (2013). Treatment with Interferon-Α2b and Ribavirin Improves Outcome in MERS-CoV-Infected Rhesus Macaques. Nat. Med. 19 (10), 1313–1317. doi:10.1038/nm.3362
Fan, Y., Sanyal, S., and Bruzzone, R. (2018). Breaking Bad: How Viruses Subvert the Cell Cycle. Front. Cel. Infect. Microbiol. 8, 396. doi:10.3389/fcimb.2018.00396
Fang, L., Karakiulakis, G., and Roth, M. (2020). Are Patients with Hypertension and Diabetes Mellitus at Increased Risk for COVID-19 Infection? Lancet Respir. Med. 8, e21. doi:10.1016/S2213-2600(20)30116-8
Fang, L., Karakiulakis, G., and Roth, M. (2020). Are Patients with Hypertension and Diabetes Mellitus at Increased Risk for COVID-19 Infection? Lancet Respir. Med. 8 (4), e21. doi:10.1016/s2213-2600(20)30116-8
Ganapathiraju, M. K., Karunakaran, K. B., and Correa-Menendez, J. (2016). Predicted Protein Interactions of IFITMs Which Inhibit Zika Virus Infection. F1000Res. 5, 1919. doi:10.12688/f1000research.9364.1
Ganapathiraju, M. K., Thahir, M., Handen, A., Sarkar, S. N., Sweet, R. A., Nimgaonkar, V. L., et al. (2016). Schizophrenia Interactome with 504 Novel Protein-Protein Interactions. npj Schizophr 2, 16012. doi:10.1038/npjschz.2016.12
Garzón, J. I., Deng, L., Murray, D., Shapira, S., Petrey, D., and Honig, B. (2016). A Computational Interactome and Functional Annotation for the Human Proteome. Elife 5, e18715. doi:10.7554/eLife.18715
Gordon, D. E., Jang, G. M., Bouhaddou, M., Xu, J., Obernier, K., O’Meara, M. J., et al. (2020). A SARS-CoV-2-Human Protein-Protein Interaction Map Reveals Drug Targets and Potential Drug-Repurposing. London, United kingdom: Nature.
Gordon, D. E., Jang, G. M., Bouhaddou, M., Xu, J., Obernier, K., White, K. M., et al. (2020). A SARS-CoV-2 Protein Interaction Map Reveals Targets for Drug Repurposing. Nature 583, 459–468. doi:10.1038/s41586-020-2286-9
Gralinski, L. E., and Baric, R. S. (2015). Molecular Pathology of Emerging Coronavirus Infections. J. Pathol. 235 (2), 185–195. doi:10.1002/path.4454
Gysi, D. M., Valle, Í. D., Zitnik, M., Ameli, A., Gan, X., Varol, O., et al. (2020). Network Medicine Framework for Identifying Drug Repurposing Opportunities for Covid-19. Proceedings of the National Academy of Sciences 118 (19).
Hafirassou, M. L., Meertens, L., Umaña-Diaz, C., Labeau, A., Dejarnac, O., Bonnet-Madin, L., et al. (2017). A Global Interactome Map of the Dengue Virus NS1 Identifies Virus Restriction and Dependency Host Factors. Cell Rep. 21 (13), 3900–3913. doi:10.1016/j.celrep.2017.11.094
Hall, O. J., Limjunyawong, N., Vermillion, M. S., Robinson, D. P., Wohlgemuth, N., Pekosz, A., et al. (2016). Progesterone-based Therapy Protects against Influenza by Promoting Lung Repair and Recovery in Females. Plos Pathog. 12, e1005840. doi:10.1371/journal.ppat.1005840
Handen, A., and Ganapathiraju, M. K. (2015). LENS: Web-Based Lens for Enrichment and Network Studies of Human Proteins. BMC Med. Genomics 8 (S4), S2. doi:10.1186/1755-8794-8-s4-s2
Hart, B. J., Dyall, J., Postnikova, E., Zhou, H., Kindrachuk, J., Johnson, R. F., et al. (2014). Interferon-β and Mycophenolic Acid Are Potent Inhibitors of Middle East Respiratory Syndrome Coronavirus in Cell-Based Assays. J. Gen. Virol. 95 (Pt 3), 571–577. doi:10.1099/vir.0.061911-0
Hoffmann, M., Kleine-Weber, H., Schroeder, S., Krüger, N., Herrler, T., Erichsen, S., et al. (2020). SARS-CoV-2 Cell Entry Depends on ACE2 and TMPRSS2 and Is Blocked by a Clinically Proven Protease Inhibitor. Cell 181, 271–e8. doi:10.1016/j.cell.2020.02.052
Hoischen, C., Monajembashi, S., Weisshart, K., and Hemmerich, P. (2018). Multimodal Light Microscopy Approaches to Reveal Structural and Functional Properties of Promyelocytic Leukemia Nuclear Bodies. Front. Oncol. 8, 125. doi:10.3389/fonc.2018.00125
Hopf, T. A., Schärfe, C. P., Rodrigues, J. P., Green, A. G., Kohlbacher, O., Sander, C., et al. (2014). Sequence Co-evolution Gives 3D Contacts and Structures of Protein Complexes. Elife 3, e03430. doi:10.7554/eLife.03430
Huttlin, E. L., Bruckner, R. J., Navarrete-Perea, J., Cannon, J. R., Baltier, K., Gebreab, F., et al. (2020). Dual Proteome-Scale Networks Reveal Cell-specific Remodeling of the Human Interactome. Cell 184 (11), 3022–40.e28.
Imai, H., Dansako, H., Ueda, Y., Satoh, S., and Kato, N. (2018). Daunorubicin, a Topoisomerase II Poison, Suppresses Viral Production of Hepatitis B Virus by Inducing cGAS-dependent Innate Immune Response. Biochem. biophysical Res. Commun. 504 (4), 672–678. doi:10.1016/j.bbrc.2018.08.195
Jäger, S., Cimermancic, P., Gulbahce, N., Johnson, J. R., McGovern, K. E., Clarke, S. C., et al. (2012). Global Landscape of HIV-Human Protein Complexes. Nature 481 (7381), 365–370. doi:10.1038/nature10719
Jain, A., and Tuteja, G. (2019). TissueEnrich: Tissue-specific Gene Enrichment Analysis. Bioinformatics 35 (11), 1966–1967. doi:10.1093/bioinformatics/bty890
Jeon, S., Ko, M., Lee, J., Choi, I., Byun, S. Y., Park, S., et al. (2020). Identification of Antiviral Drug Candidates against SARS-CoV-2 from FDA-Approved Drugs. Washington DC: Antimicrobial Agents and Chemotherapy.
Jia, J., Liu, Z., Xiao, X., Liu, B., and Chou, K.-C. (2015). iPPI-Esml: an Ensemble Classifier for Identifying the Interactions of Proteins by Incorporating Their Physicochemical Properties and Wavelet Transforms into PseAAC. J. Theor. Biol. 377, 47–56. doi:10.1016/j.jtbi.2015.04.011
Karunakaran, K. B., Chaparala, S., and Ganapathiraju, M. K. (2019). Potentially Repurposable Drugs for Schizophrenia Identified from its Interactome. Sci. Rep. 9 (1), 12682–12714. Available at: https://www.nature.com/articles/s41598-019-48307-w. doi:10.1038/s41598-019-48307-w
Karunakaran, K. B., Chaparala, S., Lo, C. W., and Ganapathiraju, M. K. (2020). Cilia Interactome with Predicted Protein-Protein Interactions Reveals Connections to Alzheimer's Disease, Aging and Other Neuropsychiatric Processes. Sci. Rep. 10 (1), 15629–15716. Available at: https://www.nature.com/articles/s41598-020-72024-4. doi:10.1038/s41598-020-72024-4
Karunakaran, K. B., Chaparala, S., and Ganapathiraju, M. K. (2019). Potentially Repurposable Drugs for Schizophrenia Identified from its Interactome. Sci. Rep. 9 (1), 12682. doi:10.1038/s41598-019-48307-w
Karunakaran, K. B., Yanamala, N., Boyce, G., Becich, M. J., and Ganapathiraju, M. K. (2021). Malignant Pleural Mesothelioma Interactome with 364 Novel Protein-Protein Interactions. Cancers 13 (7), 1660. doi:10.3390/cancers13071660
Kerslake, R., Hall, M., Randeva, H., Spandidos, D., Chatha, K., Kyrou, I., et al. (2020). Co-expression of P-eripheral O-lfactory R-eceptors with SARS-CoV-2 I-nfection M-ediators: Potential I-mplications beyond L-oss of S-mell as a COVID-19 S-ymptom. Int. J. Mol. Med. 46 (3), 949–956. doi:10.3892/ijmm.2020.4646
Keshava Prasad, T. S., Goel, R., Kandasamy, K., Keerthikumar, S., Kumar, S., Mathivanan, S., et al. (2008). Human Protein Reference Database-2009 Update. Nucleic Acids Res. 37 (Suppl. l_1), D767–D772. doi:10.1093/nar/gkn892
Keskin, O., Tuncbag, N., and Gursoy, A. (2016). Predicting Protein-Protein Interactions from the Molecular to the Proteome Level. Chem. Rev. 116 (8), 4884–4909. doi:10.1021/acs.chemrev.5b00683
Khan, M., Syed, G. H., Kim, S.-J., and Siddiqui, A. (2015). Mitochondrial Dynamics and Viral Infections: a Close Nexus. Biochim. Biophys. Acta (Bba) - Mol. Cell Res. 1853 (10), 2822–2833. doi:10.1016/j.bbamcr.2014.12.040
Kotlyar, M., Pastrello, C., Pivetta, F., Lo Sardo, A., Cumbaa, C., Li, H., et al. (2015). In Silico prediction of Physical Protein Interactions and Characterization of Interactome Orphans. Nat. Methods 12 (1), 79–84. doi:10.1038/nmeth.3178
Kuleshov, M. V., Stein, D. J., Clarke, D. J. B., Kropiwnicki, E., Jagodnik, K. M., Bartal, A., et al. (2020). The COVID-19 Drug and Gene Set Library. Patterns (N Y) 1, 100090. doi:10.1016/j.patter.2020.100090
Kumaki, Y., Day, C. W., Wandersee, M. K., Schow, B. P., Madsen, J. S., Grant, D., et al. (2008). Interferon Alfacon 1 Inhibits SARS-CoV Infection in Human Bronchial Epithelial Calu-3 Cells. Biochem. biophysical Res. Commun. 371 (1), 110–113. doi:10.1016/j.bbrc.2008.04.006
Kumar, N., Mishra, B., Mehmood, A., Mohammad Athar, M., and M Shahid Mukhtar, M. S. (2020). Integrative Network Biology Framework Elucidates Molecular Mechanisms of Sars-Cov-2 Pathogenesis. iScience 23 (9), 101526. doi:10.1016/j.isci.2020.101526
Kupershmidt, I., Su, Q. J., Grewal, A., Sundaresh, S., Halperin, I., Flynn, J., et al. (2010). Ontology-based Meta-Analysis of Global Collections of High-Throughput Public Data. PLoS One 5 (9), 1. doi:10.1371/journal.pone.0013066
Lambert, D. W., Yarski, M., Warner, F. J., Thornhill, P., Parkin, E. T., Smith, A. I., et al. (2005). Tumor Necrosis Factor-α Convertase (ADAM17) Mediates Regulated Ectodomain Shedding of the Severe-Acute Respiratory Syndrome-Coronavirus (SARS-CoV) Receptor, Angiotensin-Converting Enzyme-2 (ACE2). J. Biol. Chem. 280 (34), 30113–30119. doi:10.1074/jbc.m505111200
Li, G., and De Clercq, E. (2020). Therapeutic Options for the 2019 Novel Coronavirus (2019-nCoV). Nat. Rev. Drug Discov. 19, 149–150. doi:10.1038/d41573-020-00016-0
Li, H. S., Kuok, D. I. T., Cheung, M. C., Ng, M. M. T., Ng, K. C., Hui, K. P. Y., et al. (2018). Effect of Interferon Alpha and Cyclosporine Treatment Separately and in Combination on Middle East Respiratory Syndrome Coronavirus (Mers-cov) Replication in a Human In-Vitro and Ex-Vivo Culture Model. Antivir. Res. 155, 89–96. doi:10.1016/j.antiviral.2018.05.007
Li, Y., Klena, N. T., Gabriel, G. C., Liu, X., Kim, A. J., Lemke, K., et al. (2015). Global Genetic Analysis in Mice Unveils central Role for Cilia in Congenital Heart Disease. Nature 521 (7553), 520–524. doi:10.1038/nature14269
Liao, Y., Wang, J., Jaehnig, E. J., Shi, Z., and Zhang, B. (2019). WebGestalt 2019: Gene Set Analysis Toolkit with Revamped UIs and APIs. Nucleic Acids Res. 9, 1. doi:10.1093/nar/gkz401
Lin, S.-C., Ho, C.-T., Chuo, W.-H., Li, S., Wang, T. T., and Lin, C.-C. (2017). Effective Inhibition of MERS-CoV Infection by Resveratrol. BMC Infect. Dis. 17 (1), 144. doi:10.1186/s12879-017-2253-8
Liu, X., Yagi, H., Saeed, S., Bais, A. S., Gabriel, G. C., Chen, Z., et al. (2017). The Complex Genetics of Hypoplastic Left Heart Syndrome. Nat. Genet. 49 (7), 1152–1159. doi:10.1038/ng.3870
Lonsdale, J., Thomas, J., Salvatore, M., Phillips, R., Lo, E., Shad, S., et al. (2013). The Genotype-Tissue Expression (GTEx) Project. Nat. Genet. 45 (6), 580–585.
Luck, K., Kim, D. K., Lambourne, L., Spirohn, K., Begg, B. E., Bian, W., et al. (2020). A Reference Map of the Human Binary Protein Interactome. Nature 580, 402–408. doi:10.1038/s41586-020-2188-x
Malavia, T., Chaparala, S., Wood, J., Chowdari, K., Prasad, K., McClain, L., et al. (2017). Generating Testable Hypotheses for Schizophrenia and Rheumatoid Arthritis Pathogenesis by Integrating Epidemiological, Genomic and Protein Interaction Data Npj Schizophrenia. London, United Kingdom: Nature.
Malavia, T. A., Chaparala, S., Wood, J., Chowdari, K., Prasad, K. M., McClain, L., et al. (2017). Generating Testable Hypotheses for Schizophrenia and Rheumatoid Arthritis Pathogenesis by Integrating Epidemiological, Genomic, and Protein Interaction Data. npj Schizophr 3 (1), 11. doi:10.1038/s41537-017-0010-z
Mehta, D. R., Ashkar, A. A., and Mossman, K. L. (2012). The Nitric Oxide Pathway Provides Innate Antiviral protection in Conjunction with the Type I Interferon Pathway in Fibroblasts. PLoS One 7, e31688. doi:10.1371/journal.pone.0031688
Messner, C. B., Demichev, V., Wendisch, D., Michalick, L., White, M., Freiwald, A., et al. (2020). Ultra-high-throughput Clinical Proteomics Reveals Classifiers of COVID-19 Infection. Cell Syst. 11 (1), 11–24. e4. doi:10.1016/j.cels.2020.05.012
Moore, M. J., Blachere, N. E., Fak, J. J., Park, C. Y., Sawicka, K., Parveen, S., et al. (2018). ZFP36 RNA-Binding Proteins Restrain T Cell Activation and Anti-viral Immunity. Elife 7, 7. doi:10.7554/eLife.33057
Morgan, D. O. (2007). Figure 5-2 Stages of Late M Phase in a Vertebrate Cell. London, United Kingdom: New Science Press, 1.
Morita, E., Colf, L. A., Karren, M. A., Sandrin, V., Rodesch, C. K., and Sundquist, W. I. (2010). Human ESCRT-III and VPS4 Proteins Are Required for Centrosome and Spindle Maintenance. Proc. Natl. Acad. Sci. 107 (29), 12889–12894. doi:10.1073/pnas.1005938107
Nugent, K. M., and Shanley, J. D. (1984). Verapamil Inhibits Influenza A Virus Replication. Arch. Virol. 81 (1-2), 163–170. doi:10.1007/BF01309305
Omeragic, A., Kara-Yacoubian, N., Kelschenbach, J., Sahin, C., Cummins, C. L., Volsky, D. J., et al. (2019). Peroxisome Proliferator-Activated Receptor-Gamma Agonists Exhibit Anti-inflammatory and Antiviral Effects in an EcoHIV Mouse Model. Sci. Rep. 9 (1). Available at: https://www.nature.com/articles/s41598-019-45878-6. doi:10.1038/s41598-019-45878-6
Orii, N., and Ganapathiraju, M. K. (2012). Wiki-pi: a Web-Server of Annotated Human Protein-Protein Interactions to Aid in Discovery of Protein Function. PLoS One 7 (11), e49029. doi:10.1371/journal.pone.0049029
Overmyer, K. A., Shishkova, E., Miller, I. J., Balnis, J., Bernstein, M. N., Peters-Clarke, T. M., et al. (2021). Large-scale Multi-Omic Analysis of COVID-19 Severity. Cell Syst. 12 (1), 23–40. e7. doi:10.1016/j.cels.2020.10.003
Panda, D., Pascual-Garcia, P., Dunagin, M., Tudor, M., Hopkins, K. C., Xu, J., et al. (2014). Nup98 Promotes Antiviral Gene Expression to Restrict RNA Viral Infection inDrosophila. Proc. Natl. Acad. Sci. USA 111 (37), E3890–E3899. doi:10.1073/pnas.1410087111
Perry, C. M., and Noble, S. (1999). Didanosine. Drugs 58 (6), 1099–1135. doi:10.2165/00003495-199958060-00009
Pfefferle, S., Schöpf, J., Kögl, M., Friedel, C. C., Müller, M. A., Carbajo-Lozoya, J., et al. (2011). The SARS-Coronavirus-Host Interactome: Identification of Cyclophilins as Target for Pan-Coronavirus Inhibitors. Plos Pathog. 7 (10), e1002331. doi:10.1371/journal.ppat.1002331
Pincetic, A., Kuang, Z., Seo, E. J., and Leis, J. (2010). The Interferon-Induced Gene ISG15 Blocks Retrovirus Release from Cells Late in the Budding Process. J. Virol. 84 (9), 4725–4736. doi:10.1128/jvi.02478-09
Quintana, V. M., Selisko, B., Brunetti, J. E., Eydoux, C., Guillemot, J. C., Canard, B., et al. (2020). Antiviral Activity of the Natural Alkaloid Anisomycin against Dengue and Zika Viruses. Antivir. Res. 176, 104749. doi:10.1016/j.antiviral.2020.104749
Raja, K., Subramani, S., and Natarajan, J. (2013). PPInterFinder-a Mining Tool for Extracting Causal Relations on Human Proteins from Literature. Database (Oxford) 2013, bas052. doi:10.1093/database/bas052
Reghunathan, R., Jayapal, M., Hsu, L.-Y., Chng, H.-H., Tai, D., Leung, B. P., et al. (2005). Expression Profile of Immune Response Genes in Patients with Severe Acute Respiratory Syndrome. BMC Immunol. 6 (1), 2. doi:10.1186/1471-2172-6-2
Rothan, H. A., and Byrareddy, S. N. (2020). The Epidemiology and Pathogenesis of Coronavirus Disease (COVID-19) Outbreak. J. Autoimmun. 109, 102433. doi:10.1016/j.jaut.2020.102433
Ryang, J., Yan, Y., Song, Y., Liu, F., and Ng, T. B. (2019). Anti-HIV, Antitumor and Immunomodulatory Activities of Paclitaxel from Fermentation Broth Using Molecular Imprinting Technique. AMB Expr. 9 (1), 194. doi:10.1186/s13568-019-0915-1
Scherer, M., and Stamminger, T. (2016). Emerging Role of PML Nuclear Bodies in Innate Immune Signaling. J. Virol. 90 (13), 5850–5854. doi:10.1128/jvi.01979-15
Sehgal, N., Kumawat, K. L., Basu, A., and Ravindranath, V. (2012). Fenofibrate Reduces Mortality and Precludes Neurological Deficits in Survivors in Murine Model of Japanese Encephalitis Viral Infection. PloS one 7 (4), e35427. doi:10.1371/journal.pone.0035427
Shannon, P., Markiel, A., Ozier, O., Baliga, N. S., Wang, J. T., Ramage, D., et al. (2003). Cytoscape: a Software Environment for Integrated Models of Biomolecular Interaction Networks. Genome Res. 13 (11), 2498–2504. doi:10.1101/gr.1239303
Shendi, A., Hung, R. Y., Caplin, B., Griffiths, P., and Harber, M. (2019). The Use of Sirolimus in Patients with Recurrent Cytomegalovirus Infection after Kidney Transplantation: A Retrospective Case Series Analysis. Saudi J. Kidney Dis. Transpl. 30 (3), 606–614. doi:10.4103/1319-2442.261333
Sidaway, P. (2020). COVID-19 and Cancer: what We Know So Far. Nat. Rev. Clin. Oncol. 17 (6), 336. doi:10.1038/s41571-020-0366-2
Sims, A. C., Baric, R. S., Yount, B., Burkett, S. E., Collins, P. L., and Pickles, R. J. (2005). Severe Acute Respiratory Syndrome Coronavirus Infection of Human Ciliated Airway Epithelia: Role of Ciliated Cells in Viral Spread in the Conducting Airways of the Lungs. J. Virol. 79 (24), 15511–15524. doi:10.1128/jvi.79.24.15511-15524.2005
Sirota, M., Dudley, J. T., Kim, J., Chiang, A. P., Morgan, A. A., Sweet-Cordero, A., et al. (2011). Discovery and Preclinical Validation of Drug Indications Using Compendia of Public Gene Expression Data. Sci. Transl Med. 3 (96), 96ra77. doi:10.1126/scitranslmed.3001318
Spirin, V., and Mirny, L. A. (2003). Protein Complexes and Functional Modules in Molecular Networks. Proc. Natl. Acad. Sci. 100 (21), 12123–12128. doi:10.1073/pnas.2032324100
Stark, C., Breitkreutz, B. J., Reguly, T., Boucher, L., Breitkreutz, A., and Tyers, M. (2006). BioGRID: a General Repository for Interaction Datasets. Nucleic Acids Res. 34 (Suppl. l_1), D535–D539. doi:10.1093/nar/gkj109
Sudarsanam, T. D., Sahni, R. D., and John, G. T. (2006). Leflunomide: a Possible Alternative for Gangciclovir Sensitive and Resistant Cytomegalovirus Infections. Postgrad. Med. J. 82 (967), 313–314. doi:10.1136/pgmj.2005.038521
Sungnak, W., Huang, N., Bécavin, C., Berg, M., and Network, H. C. A. (2020). SARS-CoV-2 Entry Genes Are Most Highly Expressed in Nasal Goblet and Ciliated Cells within Human Airways. ArXiv: Cornell University.
Torriani, G., Trofimenko, E., Mayor, J., Fedeli, C., Moreno, H., Michel, S., et al. (2019). Identification of Clotrimazole Derivatives as Specific Inhibitors of Arenavirus Fusion. J. Virol. 93 (6), e01744–18. doi:10.1128/JVI.01744-18
Touret, F., Gilles, M., Barral, K., Nougairède, A., Decroly, E., de Lamballerie, X., et al. (2020). In Vitro Screening of a FDA Approved Chemical Library Reveals Potential Inhibitors of SARS-CoV-2 Replication. BioRxiv: Cold Spring Harbor Laboratory.
Trepte, P., Buntru, A., Klockmeier, K., Willmore, L., Arumughan, A., Secker, C., et al. (2015). DULIP: a Dual Luminescence-Based Co-immunoprecipitation Assay for Interactome Mapping in Mammalian Cells. J. Mol. Biol. 427 (21), 3375–3388. doi:10.1016/j.jmb.2015.08.003
Vishwajit Nimgaonkar, M. D. P. (2024). Stanley Medical Research I, University of P. Acetazolamide for Treatment Resistant Schizophrenia. Stanley Medical Research Institute. Available at: https://clinicaltrials.gov/ct2/show/NCT04887792
Vishwajit Nimgaonkar, M. D. P. (2022). Stanley Medical Research I, University of P. Cromoglycate Adjunctive Therapy for Outpatients with Schizophrenia. Stanley Medical Research Institute.. Available at: https://clinicaltrials.gov/ct2/show/results/NCT03794076
Wang, Z., and Zhang, J. (2007). In Search of the Biological Significance of Modular Structures in Protein Networks. Plos Comput. Biol. 3 (6), e107. doi:10.1371/journal.pcbi.0030107
Warner, F. J., Lew, R. A., Smith, A. I., Lambert, D. W., Hooper, N. M., and Turner, A. J. (2005). Angiotensin-converting Enzyme 2 (ACE2), but Not ACE, Is Preferentially Localized to the Apical Surface of Polarized Kidney Cells. J. Biol. Chem. 280 (47), 39353–39362. doi:10.1074/jbc.m508914200
Weston, S., Coleman, C. M., Haupt, R., Logue, J., Matthews, K., Li, Y., et al. (2020). Broad Anti-coronaviral Activity of FDA Approved Drugs against SARS-CoV-2 in Vitro and SARS-CoV in Vivo. Journal of virology 94 (21), e01218–e01220.
Wilson, K. S. (2015). Figure 1.4 – Diagram of Flagellum Origin and Cross-Section. Probing Mech. Forces Flagella by Manipulation Media Viscosity Axonemal Struct. 9, 1.
Wishart, D. S., Knox, C., Guo, A. C., Cheng, D., Shrivastava, S., Tzur, D., et al. (2008). DrugBank: a Knowledgebase for Drugs, Drug Actions and Drug Targets. Nucleic Acids Res. 36 (Database issue), D901–D906. doi:10.1093/nar/gkm958
Wu, G., Dawson, E., Duong, A., Haw, R., and Stein, L. (2014). ReactomeFIViz: a Cytoscape App for Pathway and Network-Based Data Analysis. F1000Res 3, 146. doi:10.12688/f1000research.4431.2
Wu, W., Li, R., Li, X., He, J., Jiang, S., Liu, S., et al. (2016). Quercetin as an Antiviral Agent Inhibits Influenza A Virus (IAV) Entry. Viruses 8 (1), 6. doi:10.3390/v8010006
Yang, S., Fu, C., Lian, X., Dong, X., and Zhang, Z. (2019). Understanding Human-Virus Protein-Protein Interactions Using a Human Protein Complex-Based Analysis Framework. MSystems 4, 1. doi:10.1128/mSystems.00303-18
You, Z. H., Lei, Y. K., Zhu, L., Xia, J., and Wang, B. (2013). Prediction of Protein-Protein Interactions from Amino Acid Sequences with Ensemble Extreme Learning Machines and Principal Component Analysis. BMC bioinformatics 14 (8), S10. doi:10.1186/1471-2105-14-S8-S10
Zhao, Y., Ren, J., Fry, E. E., Xiao, J., Townsend, A. R., and Stuart, D. I. (2018). Structures of Ebola Virus Glycoprotein Complexes with Tricyclic Antidepressant and Antipsychotic Drugs. J. Med. Chem. 61 (11), 4938–4945. doi:10.1021/acs.jmedchem.8b00350
Zhou, Y., Hou, Y., Shen, J., Mehra, R., Kallianpur, A., Culver, D. A., et al. (2020). A Network Medicine Approach to Investigation and Population-Based Validation of Disease Manifestations and Drug Repurposing for COVID-19. PLoS Biology 18 (11), e3000970.
Zhou, Y., Hou, Y., Shen, J., Huang, Y., Martin, W., and Cheng, F. (2020). Network-based Drug Repurposing for Novel Coronavirus 2019-nCoV/SARS-CoV-2. Cell Discov 6 (1), 14. doi:10.1038/s41421-020-0153-3
Keywords: interactome analysis, protein-protein interactions, computational prediction, COVID-19, SARS-CoV-2, drugs, drug repurposing
Citation: Karunakaran KB, Balakrishnan N and Ganapathiraju MK (2022) Interactome of SARS-CoV-2 Modulated Host Proteins With Computationally Predicted PPIs: Insights From Translational Systems Biology Studies. Front. Syst. Biol. 2:815237. doi: 10.3389/fsysb.2022.815237
Received: 24 November 2021; Accepted: 11 February 2022;
Published: 29 April 2022.
Edited by:
Alyssa E. Barry, Deakin University, AustraliaReviewed by:
Gokhan Ertaylan, Flemish Institute for Technological Research (VITO), BelgiumRanjith Kumavath, Central University of Kerala, India
Copyright © 2022 Karunakaran, Balakrishnan and Ganapathiraju. This is an open-access article distributed under the terms of the Creative Commons Attribution License (CC BY). The use, distribution or reproduction in other forums is permitted, provided the original author(s) and the copyright owner(s) are credited and that the original publication in this journal is cited, in accordance with accepted academic practice. No use, distribution or reproduction is permitted which does not comply with these terms.
*Correspondence: Madhavi K. Ganapathiraju, bWFkaGF2aUBwaXR0LmVkdQ==