- College of Health and Life Sciences, Hamad Bin Khalifa University, Doha, Qatar
Recent research efforts provided compelling evidence of genome-wide DNA methylation alterations in aging and age-related disease. It is currently well established that DNA methylation biomarkers can determine biological age of any tissue across the entire human lifespan, even during development. There is growing evidence suggesting epigenetic age acceleration to be strongly linked to common diseases or occurring in response to various environmental factors. DNA methylation based clocks are proposed as biomarkers of early disease risk as well as predictors of life expectancy and mortality. In this review, we will summarize key advances in epigenetic clocks and their potential application in precision health. We will also provide an overview of progresses in epigenetic biomarker discovery in Alzheimer’s, type 2 diabetes, and cardiovascular disease. Furthermore, we will highlight the importance of prospective study designs to identify and confirm epigenetic biomarkers of disease.
Introduction
Aging is a complex and time-dependent deterioration of physiological process occurring in the majority of living organisms (Galloway, 1993). In humans, life expectancy has increased rapidly in the last few centuries due to a significant improvement in medical care and public health awareness (Crimmins, 2015). Consequently, increased life expectancy caused higher morbidity rates since advanced age is a predominant risk factor for several diseases including cancer, dementia, diabetes, and cardiovascular disease (CVD) (Jaul and Barron, 2017; Franceschi et al., 2018). Currently, there is an urgent need to improve health and longevity to increase not just the life span but also the health span of the elderly population. In recent years, several molecular and cellular processes have been reported to be linked to aging and contribute to its phenotype. Scientists proposed nine hallmarks of aging that can be classified into three categories: primary, antagonistic, or integrative (López-Otín et al., 2013). The primary hallmarks are defined as key factors causing cellular damage including genomic instability, telomere attrition, loss of proteostasis, and epigenetic alterations (López-Otín et al., 2013). During aging, there is a continuous accumulation of epigenetic changes, which might give rise to multiple age-related pathologies. A number of epidemiological studies revealed that monozygotic twins exhibit an increased rate of phenotypic discordance particularly for age-related diseases among older siblings (Frederiksen et al., 2002; Reynolds et al., 2005; Zwijnenburg et al., 2010; Greenwood et al., 2011; Castillo-Fernandez et al., 2014). This may be due to a gradual decrease in methylation conservation rates with successive cell divisions, a phenomenon referred to as “Epigenetic Drift” (Poulsen et al., 2007; Issa, 2014). This notion proposes an increased rate of stochastic methylation errors across the entire genome during aging. Indeed, several reports provided compelling evidence that older monozygotic twins exhibit global differences in DNA methylation (DNAm) patterns when compared to their younger counterparts (Fraga et al., 2005; Lévesque et al., 2014; Tan et al., 2016; Wang et al., 2018). Similarly, a centenarian’s methylome displays reduced DNA methylation levels as well as a decreased pair-wise correlation in the methylation status of neighboring CpG sites relative to the methylome of a newborn (Heyn et al., 2012).
In 1973, Vanyushin et al. (1973) were the first to describe global 5-methylcytosine (5mC) variations during aging in rats. Now, vastliterature have revealed genome-wide DNA methylation changes that occur in response to aging across multiple species. These age-related epigenetic alterations either arise systemically or are restricted to a specific tissue/cell type. Age-related DNA methylation changes also take place in germ cells and might be possibly transmitted to the offspring (Atsem et al., 2016; Potabattula et al., 2018). Since the sequencing of the human genome the scientific community has been trying to elucidate how the genetic code controls the spatial and temporal expression of genes. The essence of DNA lies within the dynamic interaction between the genetic sequence (i.e. genome) and the epigenome. In many ways, environmental influences alter gene expression through various mechanisms such as DNA methylation, hydroxymethylation, histone modifications, alternative splicing, etc. (Edwards and Myers, 2007). Recent advances in “omics” technologies availed new avenues toward implementing precision medicine based on the genetic, environmental, and lifestyle factors of each individual. Similarly, treatments of complex diseases is demanding better diagnostic and screening tools for early detection particularly in the initial phase of the disease. DNA methylation (5-methylcytosine) is a covalent epigenetic modification to the DNA by addition of a methyl group to the C-5 position of the cytosine ring by DNA methyltransferases (Dnmts). Whereas, DNA hydroxymethylation (5-hydroxymethylcytosine) is a more recently discovered modification involving the addition of a hydroxymethyl group to the 5′ position of cytosine. DNA hydroxymethylation has been reported to be enriched in the brain especially in the proximity of synaptic genes (Kriaucionis and Heintz, 2009; Khare et al., 2012). The role 5-hmC plays in various biological processes remains elusive, nevertheless scientists are starting to appreciate its importance in gene expression regulation. Methylation and demethylation processes are not only important for transcription regulation but also play a crucial role during development and cell differentiation (Moore et al., 2013). Recently, DNA methylation measurements were shown to be valuable age prediction tools, even surpassing in accuracy the age prediction models based on telomere length (Horvath et al., 2016a). DNA methylation-based age prediction models are not only accurate in predicting chronological age but can also estimate biological aging rates (Chen et al., 2016; Christiansen et al., 2016).
Epigenetic-Based Aging Clocks
It is only 6 years since Steve Horvath inaugurated a new era in epigenetics and aging research. In a landmark study, he developed a multivariate age predictor based on DNA methylation values of 353 individual CpG sites (Horvath, 2013). One of the main advantages of the Horvath clock is its ability to predict age systemically in all human cell types and tissues, excluding sperm. This is in contrast to other clocks that can be only applied to a single tissue (Hannum et al., 2013; Figure 1). Interestingly, the clock starts ticking early during development where fetal tissues as well as embryonic and induced pluripotent stem cells reveal a DNA methylation age (DNAm age) between −1 and 0 years (Horvath, 2013; Spiers et al., 2015). Till now, the biological mechanisms underlying changes measured by the epigenetic age clock have not been clearly identified. Therefore, recognizing genes that influence the rate of epigenetic aging might help determine such biological processes. Recent genome-wide association studies revealed tissue-specific association of variants in metabolism, immune system, aging, and autophagy -related genes with epigenetic age acceleration (Kananen et al., 2016; Lu et al., 2016, 2017, 2018). Epigenetic clocks have been also proposed to measure molecular processes involved in development and tissue homeostasis particularly those affecting stem cell differentiation as well as replenishment of committed cells (Horvath and Raj, 2018).
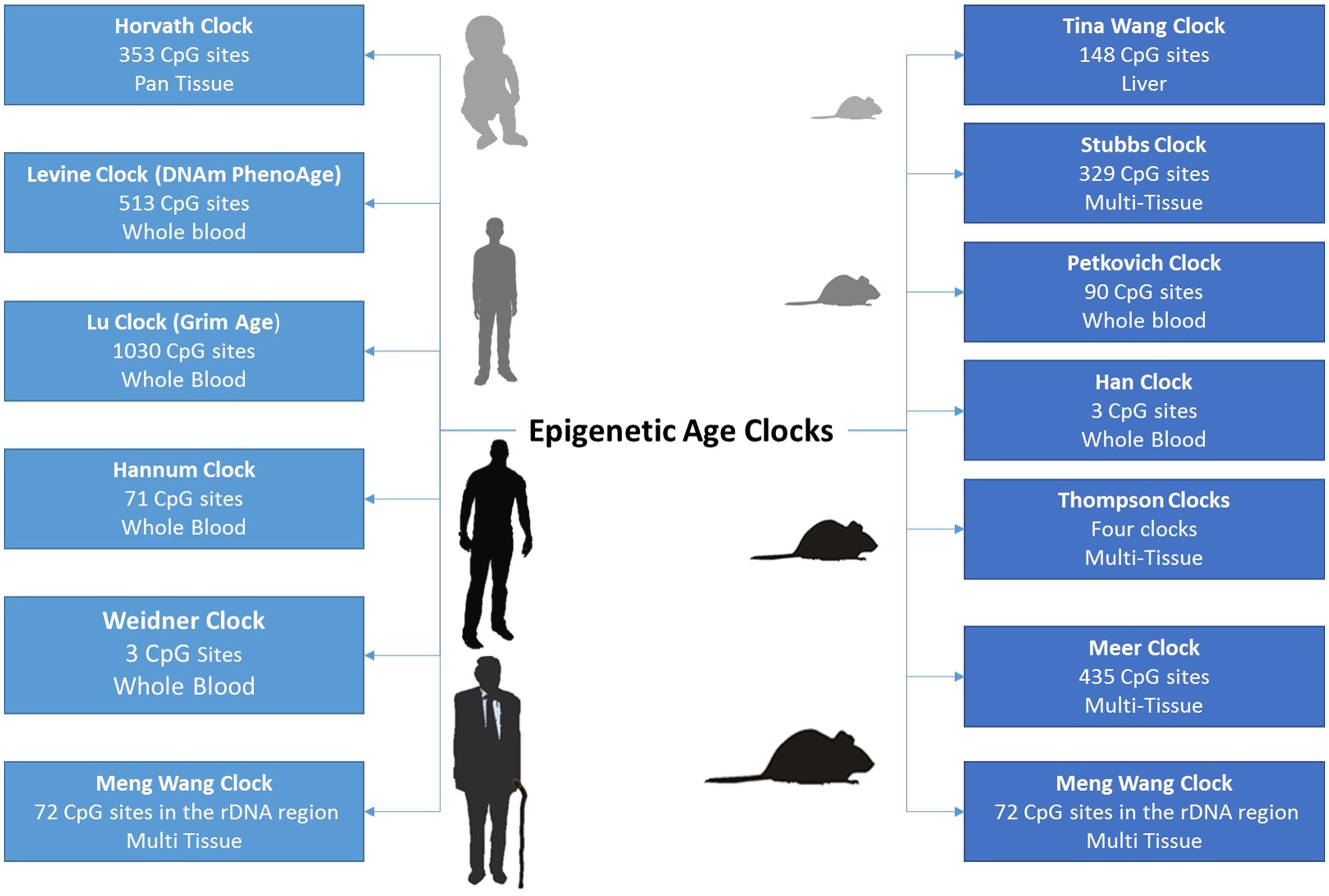
Figure 1. The growing number of epigenetic age clocks developed for both humans and mice, including the number of CpG sites comprising the age-prediction model, as well as the tissues in which age can be estimated.
By regressing DNAm age on chronological age, epigenetic clocks can determine whether biological age acceleration occurs in certain diseases or in response to environmental factors (Horvath and Raj, 2018). Using this approach, age acceleration measurements in blood were associated with body mass index (BMI), obesity, physical fitness, Huntington’s disease, Parkinson’s disease, sleep, and smoking (Horvath et al., 2014; Horvath and Ritz, 2015; Horvath et al., 2016b; Carroll et al., 2017; Quach et al., 2017; Levine et al., 2018). Epigenetic clocks are highly valuable age prediction tools nevertheless their true value as diagnostic biomarkers requires further confirmation (Figure 2). Such biomarkers are epigenetic modifications/marks used as a risk assessment and diagnostic tool to uncover sequence of events preceding the manifestation of disease. Biomarkers can be measured within tissue or body fluid, in the context of disease vs health state, for the purpose of disease detection, disease prognosis, response to therapy, and therapy monitoring (García-Giménez et al., 2016).
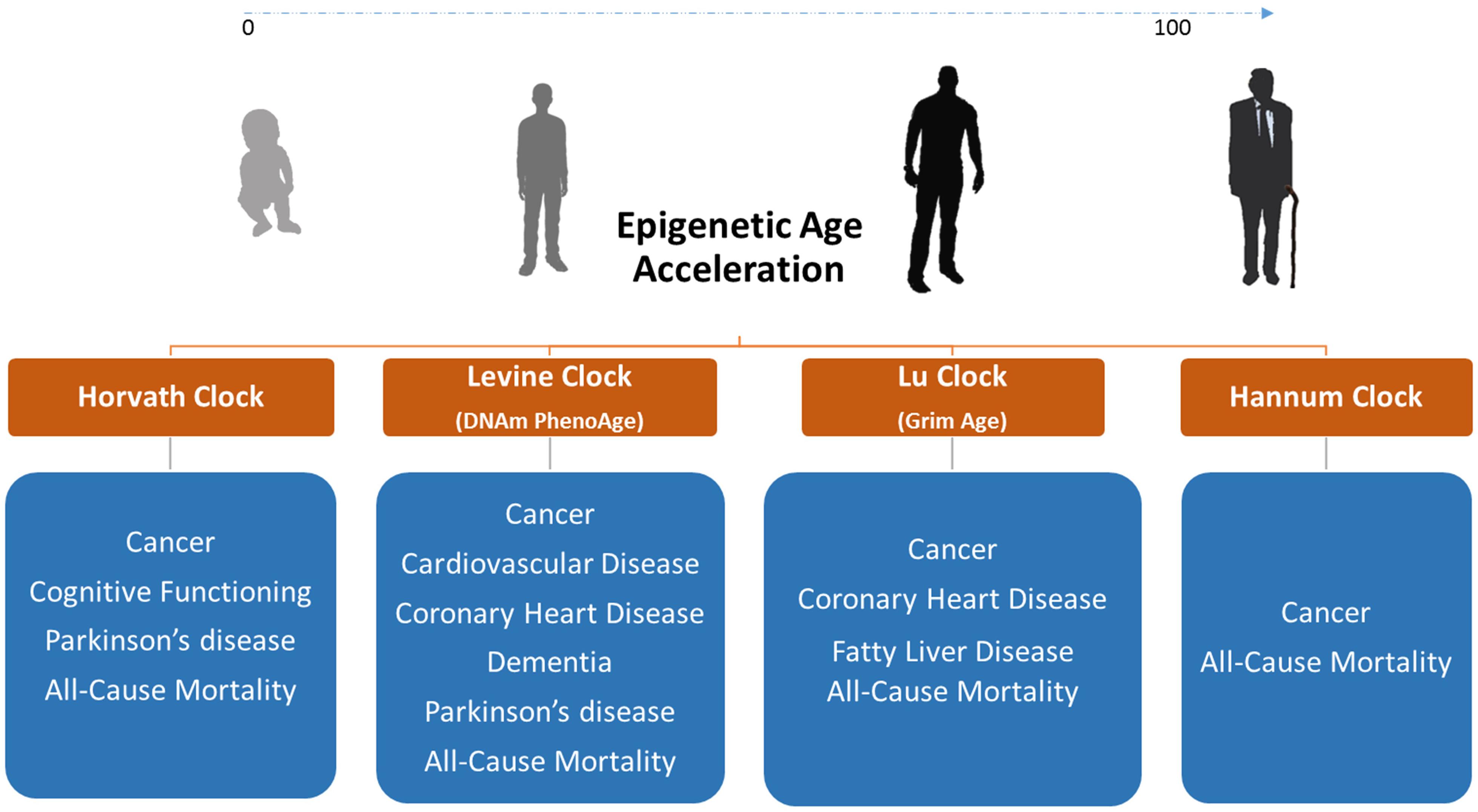
Figure 2. Diseases and conditions associated with DNAm age acceleration in blood DNA where epigenetic clocks can be used as biomarkers of disease. We only display diseases/conditions where epigenetic age acceleration is observed in blood or other non-invasive tissues. We do not show correlations with glucose, insulin, HDL, and triglyceride levels as well as with blood pressure since these factors are biomarkers on their own.
Evidently, epigenetic clocks were employed to study epigenetic age acceleration in age-related disorders. For e.g. several reports showed DNAm age acceleration associated with incidence, future onset, and mortality across several types of cancer (Levine et al., 2015a; Zheng et al., 2016; Ambatipudi et al., 2017). Similarly, DNAm age was reported to be a useful biomarker for predicting physical and mental fitness in elderly individuals (Marioni et al., 2015) and was shown to be associated with cholesterol (High Density Lipoprotein: HDL), insulin, glucose, and triglycerides levels (Quach et al., 2017; Levine et al., 2018). The adult progeroid disease, Werner syndrome, which mimics aging at a faster rate, also revealed DNAm age acceleration of >6 years (Maierhofer et al., 2017). Recently, the Horvath lab developed the DNAm PhenoAge clock by training their predictor on phenotypic age rather than chronological age (Levine et al., 2018). The DNAm PhenoAge is a powerful biomarker for measuring health- and life- span that relies on measurements from 513 CpG sites (Levine et al., 2018). This clock could conclusively predict CVD incidence using whole blood DNA methylation values. In 2019, the DNAm GrimAge clock was released where it was reported to predict mortality, cancer, and coronary heart disease (CHD) to a high level of accuracy (Lu et al., 2019). Epigenetic clocks that can estimate gestational age of neonates are also available (Knight et al., 2016). Using these clocks, we have demonstrated that DNAm age of children born via intracytoplasmic sperm injection (ICSI) lags half a week behind their naturally conceived counterparts (El Hajj et al., 2017).
In mice, epigenetic aging clocks were recently developed by relying on reduced representation bisulfite sequencing (RRBS) or whole genome bisulfite sequencing (WGBS) data (BI Ageing Clock Team et al., 2017; Petkovich et al., 2017; Wang et al., 2017; Meer et al., 2018; Thompson et al., 2018). These clocks provide useful biomarkers for measuring whether experimental interventions are able to slow the aging process in mice. Current research is focused on identifying evolutionary conserved pan-mammalian clocks that can calculate age across multiple species with varying lifespans. In addition, efforts are being invested in identifying clocks based on a handful of CpG sites since methylation arrays, RRBS, or WGBS remain relatively expensive compared to bisulfite pyrosequencing. In this aspect, Wolfgang Wagner’s group has shown that measurements from just three CpG sites can accurately readout lifespan in both humans and mice (Weidner et al., 2014; Han et al., 2018). More recently, an epigenetic clock based on ribosomal DNA methylation was reported to be evolutionary conserved across several species (Wang and Lemos, 2019).
Epigenetic Dysregulation in Type 2 Diabetes, Alzheimer’s Disease, and Cardiovascular Disease
The dynamic change between methylation and demethylation states introduces flexibility to the rigidly stable DNA code, allowing controlled changes in gene expression in response to external and internal environmental cues. These moldable, yet generally stable processes are becoming valuable tools for distinguishing healthy versus diseased states. In cancer, despite the genome-wide hypomethylation, CpG islands are hypermethylated and can serve as a biomarker for early cancer detection (Anglim et al., 2008). Recent studies have shown that changes in global content of 5mC and 5hmC are not only useful as early detection tools but also a valuable source for understanding the underlying mechanisms of cancer development and patient prognosis (Liu et al., 2019). There are several published reviews discussing epigenetic biomarkers in cancer, however, this review’s main focus will be on DNA methylation biomarkers in type 2 diabetes (T2D), Alzheimer’s disease (AD), and cardiovascular disease (CVD) (Supplementary Table S1). Here, it is important to mention that these biomarkers are independent of the epigenetic clock described in the previous section.
Type 2 Diabetes
According to the World Health Organization (WHO), >420 million adults suffer from diabetes where 1.6 million deaths per year are directly attributed to the disease (Chan, 2014). The increased lifespan in humans is one of the main contributors to the rising prevalence of diabetes in the older population. Currently, more than third the United States population above the age of 65 are diabetics with numbers projected to increase in the next decade. Type 2 Diabetes (T2D) is a metabolic disorder characterized by abnormally elevated blood glucose levels due to β-cells dysfunction and insulin resistance (Chatterjee et al., 2017). T2D is a complex multifactorial disease where a variety of genetic, epigenetic, and environmental factors contribute to its etiology (McCarthy, 2010). Common complications of diabetes include cardiovascular problems, neuropathy, nephropathy, and retinopathy due to high blood glucose levels (Jacobs et al., 2017). Therefore, prevention or early treatment are very important to prevent damage to several of the body’s systems. Despite the availability of well-established measures for diagnosing diabetes such as hemoglobin A1c (HbA1c) and fasting glucose, additional DNA-methylation based biomarkers can help complement current tests for screening and diagnosis. Identifying an individual during the pre-diabetic stage is very important for the management of the disease since ∼70% of persons with intermediate hyperglycaemia tend to develop T2D later in life.
Recently, efforts have focused on defining epigenetic risk factors associated with T2D as well as its major risk factors. Published reports have identified DNAm alterations in various tissues of T2D patients including blood, liver, pancreas, skeletal muscle, and adipose tissue (Ling and Rönn, 2019). These studies employed different approaches to quantify methylation changes including candidate gene analysis, global 5mC measurements, DNA methylation arrays, as well as WGBS (Volkov et al., 2017; Ling and Rönn, 2019). Evidently, the first reports describing epigenetic dyrsegulation in skeletal muscle and pancreatic islets of T2D patients applied a candidate gene approach. These studies identified increased DNA methylation and reduced gene expression in T2D-related genes such as INS, PDX1, PPARGC1A, and GLP1R (Ling et al., 2008; Barrès et al., 2009; Yang et al., 2012; Hall et al., 2013). Similarly, bisulfite pyrosequencing and methylation-specific PCR were employed to study methylation of key T2D genes in blood DNA. Investigated genes included KCNJ11, PPARgamma, PDK4, KCNQ1, PDX1, FTO, PEG3, TCF7L2, GCK, PRKCZ, BCL11A,GIPR, SLC30A8, IGFBP-7, PTPPN1, CAMK1D, CRY2, CALM2, TLR2, TLR4, and FFAR3 [reviewed in Willmer et al. (2018)]. Most of those studies suffered from low sample size apart of a report by Seman et al. (2015), which quantified methylation in the solute carrier family 30 member 8 (SLC30A8). Here, the authors detected hypermethylation at several CpG sites in SLC30A8 in 516 T2D subjects vs 476 individuals with normal glucose tolerance (Seman et al., 2015). Global changes in DNAm levels were also investigated using bisulfite pyrosequencing of ALU and LINE-1 elements, liquid chromatography mass spectrometry, Imprint Methylated DNA Quantification kit (Sigma-Aldrich), and High Performance Liquid Chromatography (HPLC). Conflicting results were reported which might be inherently related to low sample size and lack of replication in independent cohorts [reviewed in Willmer et al. (2018)].
The development of Infinium Methylation arrays and NGS-based methylation sequencing allowed simultaneous quantification of methylation at thousands of CpG sites. Several case-control array studies compared DNA methylation abnormalities in pancreatic islets, liver, and subcutaneous adipose tissue of T2D patients. The focus of this review is on methylation-based biomarkers therefore we will mainly describe changes reported in blood or other accessible tissues. One impressive example of such alterations is the occurrence of dynamic DNA methylation changes in Peripheral Blood Mononuclear Cells (PBMCs) ∼80–90 days prior to elevated glucose levels. This was observed by Chen et al. (2018) after longitudinally following a healthy individual over the course of 3 years while measuring DNA methylation levels using WGBS at 28 selected time-points. Another study by Toperoff et al. (2012) used a pooling-based methylation screen followed by individual-level replication in a prospective cohort to identify CpGs that can predict future T2D risk. The authors reported a single CpG site in the first intron of the fat mass and obesity-associated (FTO) gene to be hypomethylated prior to the appearance of T2D (Toperoff et al., 2012). DNA methylation alterations were also measured in concordant and discordant monozygotic twins for T2D using genome-wide methylated DNA immunoprecipitation sequencing (MeDIP-seq). This elegantly designed study uncovered differentially methylated regions (DMRs) located in the promoters of MALT1 and GPR61 (Yuan et al., 2014).
In addition to age, BMI is a major risk factor contributing to T2D and has been the focus of multiple epigenome-wide association studies (EWAS) studies. A large study on >10,000 samples identified DNA methylation changes across 187 loci correlating with high BMI levels. Out of the 187 “sentinel obesity biomarkers,” 62 loci were associated with T2D incidence including a probe in ABCG1 with the strongest significance. A methylation risk score based on the sum of these markers exhibited a higher predictive power of future T2D onset when compared to traditional risk factors such as obesity, fasting glucose, and hyperinsulinemia (Wahl et al., 2017). Similarly, a longitudinal follow-up study on Indian Asians and Europeans discovered five T2D methylation markers in whole blood DNA collected at baseline prior to diabetes onset. These markers located in ABCG1, PHOSPHO1, SOCS3, SREBF1, and TXNIP were associated with metabolic measures of insulin resistance including glucose concentration, BMI, waist-to-hip ratio, and homeostatic model assessment for insulin resistance (HOMA-IR) (Chambers et al., 2015). A conceptually related study tried to replicate the association between T2D and the five previously mentioned genes in subjects from the Botnia prospective cohort. Nonetheless, they could only confirm ABCG1 and PHOSPHO1 methylation as predictors of future T2D risk (Dayeh et al., 2016). This association was also observed in healthy individuals where ABCG1 methylation was reported to correlate with fasting insulin and HOMA-IR (Hidalgo et al., 2014).
Further EWAS studies could confirm methylation aberrations in some of the previously mentioned genes. A large EWAS analysis in Mexican-American individuals unraveled five CpG sites linked to T2D-related traits out of which 3 were located in TXNIP (cg19693031), ABCG1, and SAMD12 (Kulkarni et al., 2015). Two separate studies from Spain and Germany confirmed the association between decreasing methylation levels at TXNIP (cg19693031) and T2D, as well as with fasting glucose and HbA1c concentrations (Florath et al., 2016; Soriano-Tárraga et al., 2016). To end with EWAS, it is important to mention a meta-analysis by Walaszczyk et al. (2018) that took the initiative to confirm potential glycemic trait and T2D biomarkers. In this replication analyses, the authors concluded that a significant association between T2D and methylation sites in ABCG1, TXNIP, and SREBF1 exists, which makes them promising biomarkers for early T2D detection. As a final point, we have to emphasize the significance of non-genetic elements including blood sugar levels, patient age, BMI, and gender in predicting future diabetes risk. Thus, such factors should be integrated into a T2D predictive model that includes genetic and epigenetic biomarkers to improve early T2D detection and allow better disease prognosis.
Alzheimer’s Disease
Accumulation of errors in the epigenetic machinery during aging progression increases the risk for onset of age-related pathologies, such of those involving brain deterioration and neurodegeneration. The most common brain disorders affecting elderly individuals are those causing dementia through loss of synaptic plasticity, leading to memory impairment and defective learning capabilities. Alzheimer’s disease (AD) affects 45–60% of the population with dementia and its burden is expected to double by the year 2060 (Finder, 2010; Duong et al., 2017). AD is a polygenic, complex and age-related neurodegenerative disease clinically characterized by progressive memory loss and cognitive impairment. Its pathological features include accumulation of β-amyloid (Aβ) in senile plaques, the formation of neurofibrillary tangles (NFTs) composed of hyperphosphorylated protein tau, and massive neuronal loss mainly in the hippocampus as well as associated regions of the neocortex (Hardy, 2006). Several clinical and epidemiological aspects of AD indicate a role for epigenetic factors in its etiology. This is evident in monozygotic twins discordant for the disease where prognosis and age-of onset could vary by >10 years. Indeed, a broad spectrum of epigenetic pathways such as DNA methylation, histone modification, and non-coding RNAs (ncRNAs) appear to be aberrant. For e.g. Wang et al. (2008) reported that Alzheimer’s susceptibility loci have an age-specific epigenetic drift in brain and blood of individuals with late-onset AD. Several studies were conducted to identify epigenetic aberrations, as well as to differentiate specific methylation changes occurring in AD vs non-AD dementias [reviewed in: Lardenoije et al. (2015)]. Using southern blot analysis, West et al. (1995) first showed loss of methylation at a single site in the amyloid precursor protein (APP) gene in postmortem human brain of a single individual with AD. This was confirmed by Tohgi et al. (1999) who reported that hypomethylation of cytosine residues within the APP promoter with age results in Aβ deposition in the cerebral cortex of human autopsy brain samples. Nevertheless, new studies using bisulfite sequencing failed to replicate these findings (Brohede et al., 2010). Recently, neuronal fractions from postmortem brains of Alzheimer’s patients were reported to display significantly up-regulated expression of BRCA1, consistent with hypomethylation of a CpG island (CGI) in its promoter region. BRCA1 protein levels were also increased in response to Aβ deposition and became mislocalized to the cytoplasm, in both in vitro cellular and in vivo mouse models (Mano et al., 2017).
After the introduction of methylation arrays, a large study on >700 autopsied brain samples revealed methylation and expression changes in ANK1, CDH23, DIP2A, RHBDF2, RPL13, SERPINF1, and SERPINF2 (De Jager et al., 2014). Similarly, Lunnon and collaborators performed a large EWAS analysis on four brain regions where they reported a significant hypermethylation of ANK1 in the entorhinal cortex, superior temporal gyrus, and prefrontal cortex of AD individuals. The authors went on to measure methylation in pre-mortem blood DNA where they identified distinct differentially methylated probes (DMPs) to those in AD brains (Lunnon et al., 2014). The top ranked AD-associated blood DMPs were located in DAPK1, GAS1, and NDUFS5. Furthermore, epigenetic age acceleration was shown to be associated with AD neuropathological markers such as neuritic plaques, diffuse plaques, and amyloid load in the dorsolateral prefrontal cortex (Levine et al., 2015b). Down’s syndrome patients, predisposed to early onset AD, also display DNAm age acceleration in blood and brain tissue starting early during in utero development (Horvath et al., 2015; El Hajj et al., 2016) in addition to epigenetic dysregulation at the clustered protocadherin locus (Almenar-Queralt et al., 2019).
Presently, a definitive AD diagnosis is only possible through neuropathological examination of brain tissue after death. Therefore, it is important to identify clinical biomarkers that can help in early disease detection. In addition, the effectiveness of available FDA-approved treatments for AD increases when administered during early stages of the disease. Currently, ongoing research efforts are mainly focused on delineating AD-related epigenetic changes that occur in various brain regions. So far, only a limited number of studies have assessed DNA methylation changes in blood cells. These articles will be the subject of the next section, where we will first summarize findings observed using a candidate gene approach. In one of these studies, blood DNA methylation of the Brain-derived neurotrophic factor gene (BDNF) promoter and a tag SNP (rs6265) were shown to have a significant role in the progression of the amnestic mild cognitive impairment (aMCI) to AD. Here, the interaction between DNA methylation of CpG5 and AA genotype of rs6265 had a role in the progression of aMCI to AD (p = 0.003, OR = 1.399, 95% CI: 1.198–1.477) (Xie et al., 2017a). A 5-year longitudinal study also revealed BDNF promoter methylation as a significant independent predictor of aMCI to AD transformation (Xie et al., 2017b). Similarly, Nagata et al. (2015) reported higher DNA methylation affecting a single CpG site in the BDNF promoter of patients with AD. Nevertheless, it is important to note that Carboni et al. (2015) could not confirm methylation alterations in the BDNF promoter in peripheral blood of Alzheimer’s disease patients. Therefore, doubts remain as to whether BDNF promoter methylation changes occur in AD patients. Besides, DNA methylation levels were demonstrated to be significantly elevated in Coenzyme A Synthase (COASY) and Serine Peptidase Inhibitor (SPINT1) gene promoter regions in AD and aMCI (Kobayashi et al., 2016). DNA methylation at the NCAPH2/LMF2 promoter region was also found to be a useful biomarker for the diagnosis of AD and aMCI where it was shown to be associated with hippocampal atrophy through apoptosis (Shinagawa et al., 2016). Furthermore, Ozaki et al. (2017) could show that a decline in DNA methylation in intron 1 of Triggering receptor expressed on myeloid cells 2 gene (TREM2) causes higher mRNA expression in the leukocytes of AD subjects versus controls. Phosphatidylinositol Binding Clathrin Assembly Protein (PICALM) was another candidate gene whose methylation associated with cognitive decline in blood cells of AD patients (Mercorio et al., 2018). Higher global DNA methylation levels were also observed in the peripheral blood mononuclear cells of late onset Alzheimer disease (LOAD) patients. This hypermethylation was associated with APOEε4 allele (p = 0.0043) and APOEε3 carriers (p = 0.05) (Di Francesco et al., 2015). In the same way, Bollati et al. (2011) observed a hypermethylation of LINE-1 elements in AD patients after measuring DNA methylation at ALU, LINE-1, and alpha satellite repetitive elements.
In AD, epigenome-wide association studies (EWAS) on prospective cohorts are still lacking. To address this limitation, the German Study on Aging, Cognition and Dementia in Primary Care Patients (AgeCoDe) recruited >3300 healthy individuals at baseline to investigate markers for early detection of dementia and cognitive impairment. From this cohort, Lardenoije et al. (2019) identified 55 converters healthy at baseline that developed AD dementia at follow-up. Using DNA methylation arrays, several differentially methylated regions were spotted in blood of AD converters at baseline. By focusing on those regions, we could discern epigenetic dysregulation at six DMPs in blood DNA of Down’s syndrome patients who are at high risk of developing early onset AD. One of the DMPs mapped to ADAM10, a major alpha-secretase, responsible for APP cleavage in neurons (Haertle et al., 2019). It is still challenging to find a non-invasive biomarker that reflects AD pathogenesis in the brain. Nonetheless, the previously described epigenetic alterations might be considered potential biomarkers that require further research to assess their efficacy.
Cardiovascular Disease
Cardiovascular disease (CVD) is an umbrella term for a range of conditions that affect the heart or blood vessels. The main determinants of a person’s cardiovascular health is age, as well as several risk factors including diabetes, smoking, obesity, and high blood pressure. Epigenetic aging biomarkers based on “The Horvath Clock,” “DNAm PhenoAge,” and “DNAm GrimAge” were recently reported to be associated with CVD risk (Levine et al., 2018; Lind et al., 2018; Lu et al., 2019). Even though not much research is published on the epigenetics of CVD, however, the impact of epigenetics has been extensively studied in the aforementioned risk factors. The complex interplay of genetics, epigenetics, and environment have an important role in the pathogenesis and progress of these conditions. For e.g. a trans-ancestry genome wide association study (GWAS) identified 12 genetic variants associated with methylation levels, which influences susceptibility for hypertension (Kato et al., 2015). Similarly, elevated global DNA methylation levels were reported to be positively associated with CVD and its predisposing risk factors (Sharma et al., 2008; Kim et al., 2010). Another example by Infante et al. (2019) investigated DNA methylation and expression changes in coronary heart disease patients undergoing Cardiac Computed Tomography (CCT). They could show that genes involved in cholesterol bioactivity such as LDLR promoter have higher methylation in PBMNCs of CHD patients compared to healthy controls. LDLR promoter methylation was also associated with calcified plaque volume and total plague burden measured via CCT. A case-control study using Human CpG 12K Array (HCGI12K) revealed 72 DMRs hyper-methylated in patients with coronary artery disease (CAD) (Sharma et al., 2014). More recently, an EWAS analyses using the HumanMethylation450 BeadChips reported 211 CpG sites located on 196 genes to be differentially methylated in patients with a history of myocardial infarction (MI) (Rask-Andersen et al., 2016). A similar EWAS study on acute coronary syndrome revealed associations with blood methylation levels of 47 CpG sites located in genes involved in atherogenic signaling and immune response (Li et al., 2017). Nakatochi et al. (2017) also performed an EWAS analyses on blood DNA of patients suffering from MI which revealed three differentially methylated CpG sites in SGK1, SMARC4, and ZFHX3. A large EWAS study on the Women’s Health Initiative (discovery set) and Framingham Heart Study (FHS) – (replication set) identified three DMRs in SLC9A1, SLC1A5, and TNRC6C linked to CVD incidence (Westerman et al., 2018). The authors also performed a module based epigenetic analysis, which revealed three modules associated with CVD and its risk factors out of which two had strong concordance in both cohorts (Westerman et al., 2018).
A growing number of studies reported a possible role for DNA methylation in atherosclerosis pathogenesis (Newman, 1999; Napoli et al., 2012; Aavik et al., 2015; Liu et al., 2018). Atherosclerotic lesions are known to harbor differentially methylated CpGs in genes involved in endothelial and smooth muscle functions (Zaina et al., 2014). Circulating concentrations of tumor necrosis factor α, a pro-inflammatory cytokine linked to atherosclerosis, were recently shown to be associated with methylation changes in the immune response-related genes DTX3L-PARP9 and NLRC5. DNA methylation levels of those genes were also shown to negatively correlate with CHD incidence (Aslibekyan et al., 2018). Similarly, a large EWAS meta-analysis on serum C-reactive protein (CRP), an inflammation biomarker predicting heart failure, identified 58 CpG sites related to CRP levels. Several of those CpGs (51 sites) were associated with cardio-metabolic traits including CHD prevalence and incidence (Ligthart et al., 2016). More recently, focus shifted toward understanding the role of 5-Hydroxymethylcytosine in CVD, where reports have shown that global DNA hydroxymethylation levels could be better predictors of MI and CHD when compared to 5-mC. In elderly individuals, the incidence and degree of coronary atherosclerosis (CA) were linked to increased DNA hydroxymethylation levels in PBMCs (Jiang et al., 2019a). This lead the authors to propose a novel CA biomarker based on integrating carotid plaques scores, as well as DNA methylation and hydroxymethylation data (Jiang et al., 2019b).
From a precision health perspective, a machine learning based framework focused on the FHS cohort could detect CHD presence and foresee its incidence by implementing genetic, epigenetic and phenotypic data (Dogan et al., 2018a, b). Similarly, DNA methylation levels in the TRAF3 gene were reported to predict recurrence of ischemic events in patients treated with Clopidogrel (Gallego-Fabrega et al., 2016b). A conceptually related study from the same group identified PPM1A methylation to be associated with vascular recurrence after stroke in aspirin treated patients (Gallego-Fabrega et al., 2016a). Nonetheless, there must be a more concerted effort to establish whether the reported epigenetic alterations can be reliable CVD biomarkers.
Conclusion and Future Perspectives
Despite the extensive plethora of epigenetic modifications, measuring DNA methylation of specific CpG sites remains the most promising epigenetic biomarker. DNA methylation modifications are highly stable compared to RNA- or protein-based biomarkers, relatively easy to measure using non-invasive biospecimen, and are quantifiable marks on the DNA that can track the influences of various environmental and lifestyle factors (Berdasco and Esteller, 2019). Nevertheless, epigenetic biomarkers are still in the nascent stage and more research is warranted to move toward applications in healthcare. Still, efforts invested in developing biomarkers based on the epigenetic clocks has accelerated discoveries in the field. Furthermore, GRAIL a multi-billion dollar investment has chosen DNA methylation as its preferred approach for a non-invasive test for early cancer detection.
A key factor in the development of epigenetic clocks was the advent of Infinium Methylation arrays that enabled simultaneous quantification of DNA methylation starting from ∼27,000 individual CpG sites (Infinium HumanMethylation27 BeadChip) up to 850,000 sites via EPIC arrays. These methylation arrays provide a cost-effective approach for large-scale epigenetic epidemiology studies. Nevertheless, the human genome is comprised of 28 million CpG sites out of which 3% are measured using Epic Arrays. Even though, a few reports have mentioned that whole genome bisulfite sequencing (WGBS) is potentially inefficient due to non-dynamic methylation across a large fraction of CpG cites as well as the majority of WGBS reads being non-informative (Ziller et al., 2013). Nevertheless, sequencing costs are decreasing dramatically and more comprehensive DNA methylation datasets would become publicly available once whole-genome bisulfite and oxidative bisulfite sequencing becomes mainstream. Development of more accurate epigenetic biomarkers by relying on whole genome sequencing data will be a hot topic in the next years. Future work based on these data should be even more exciting and would have important implications for human health.
Author Contributions
All authors were involved in literature review, writing the manuscript, and figure preparation.
Conflict of Interest
The authors declare that the research was conducted in the absence of any commercial or financial relationships that could be construed as a potential conflict of interest.
Acknowledgments
The authors would like to thank Aya Abdelaal for helpful remarks to improve the manuscript.
Supplementary Material
The Supplementary Material for this article can be found online at: https://www.frontiersin.org/articles/10.3389/fgene.2020.00171/full#supplementary-material
TABLE S1 | Summary of the potential blood-based epigenetic biomarkers for Alzheimer’s disease, cardiovascular disease, and Type 2 diabetes.
References
Aavik, E., Lumivuori, H., Leppanen, O., Wirth, T., Hakkinen, S.-K., Brasen, J.-H., et al. (2015). Global DNA methylation analysis of human atherosclerotic plaques reveals extensive genomic hypomethylation and reactivation at imprinted locus 14q32 involving induction of a miRNA cluster. Eur. Heart J. 36, 993–1000. doi: 10.1093/eurheartj/ehu437
Almenar-Queralt, A., Merkurjev, D., Kim, H. S., Navarro, M., Ma, Q., Chaves, R. S., et al. (2019). Chromatin establishes an immature version of neuronal protocadherin selection during the naive-to-primed conversion of pluripotent stem cells. Nat. Genet. 51, 1691–1701. doi: 10.1038/s41588-019-0526-4
Ambatipudi, S., Horvath, S., Perrier, F., Cuenin, C., Hernandez-Vargas, H., Le Calvez-Kelm, F., et al. (2017). DNA methylome analysis identifies accelerated epigenetic ageing associated with postmenopausal breast cancer susceptibility. Eur. J. Cancer 75, 299–307. doi: 10.1016/j.ejca.2017.01.014
Anglim, P. P., Alonzo, T. A., and Laird-Offringa, I. A. (2008). DNA methylation-based biomarkers for early detection of non-small cell lung cancer: an update. Mol. Cancer 7:81. doi: 10.1186/1476-4598-7-81
Aslibekyan, S., Agha, G., Colicino, E., Do, A. N., Lahti, J., Ligthart, S., et al. (2018). Association of methylation signals with incident coronary heart disease in an epigenome-wide assessment of circulating tumor necrosis factor α. JAMA Cardiol. 3, 463–472. doi: 10.1001/jamacardio.2018.0510
Atsem, S., Reichenbach, J., Potabattula, R., Dittrich, M., Nava, C., Depienne, C., et al. (2016). Paternal age effects on sperm FOXK1 and KCNA7 methylation and transmission into the next generation. Hum. Mol. Genet. 25, 4996–5005. doi: 10.1093/hmg/ddw328
Barrès, R., Osler, M. E., Yan, J., Rune, A., Fritz, T., Caidahl, K., et al. (2009). Non-CpG methylation of the PGC-1α promoter through DNMT3B controls mitochondrial density. Cell Metab. 10, 189–198. doi: 10.1016/j.cmet.2009.07.011
Berdasco, M., and Esteller, M. (2019). Clinical epigenetics: seizing opportunities for translation. Nat. Rev. Genet. 20, 109–127. doi: 10.1038/s41576-018-0074-2
BI Ageing Clock Team, Stubbs, T. M., Bonder, M. J., Stark, A.-K., Krueger, F., von Meyenn, F., et al. (2017). Multi-tissue DNA methylation age predictor in mouse. Genome Biol. 18:68. doi: 10.1186/s13059-017-1203-5
Bollati, V., Galimberti, D., Pergoli, L., Dalla Valle, E., Barretta, F., Cortini, F., et al. (2011). DNA methylation in repetitive elements and Alzheimer disease. Brain Behav. Immun. 25, 1078–1083. doi: 10.1016/j.bbi.2011.01.017
Brohede, J., Rinde, M., Winblad, B., and Graff, C. (2010). A DNA methylation study of the amyloid precursor protein gene in several brain regions from patients with familial Alzheimer disease. J. Neurogenet. 24, 179–181. doi: 10.3109/01677063.2010.503978
Carboni, L., Lattanzio, F., Candeletti, S., Porcellini, E., Raschi, E., Licastro, F., et al. (2015). Peripheral leukocyte expression of the potential biomarker proteins Bdnf, Sirt1, and Psen1 is not regulated by promoter methylation in Alzheimer’s disease patients. Neurosci. Lett. 605, 44–48. doi: 10.1016/j.neulet.2015.08.012
Carroll, J. E., Irwin, M. R., Levine, M., Seeman, T. E., Absher, D., Assimes, T., et al. (2017). Epigenetic aging and immune senescence in women with insomnia symptoms: findings from the women’s health initiative study. Biol. Psychiatry 81, 136–144. doi: 10.1016/j.biopsych.2016.07.008
Castillo-Fernandez, J. E., Spector, T. D., and Bell, J. T. (2014). Epigenetics of discordant monozygotic twins: implications for disease. Genome Med. 6:60. doi: 10.1186/s13073-014-0060-z
Chambers, J. C., Loh, M., Lehne, B., Drong, A., Kriebel, J., Motta, V., et al. (2015). Epigenome-wide association of DNA methylation markers in peripheral blood from Indian Asians and Europeans with incident type 2 diabetes: a nested case-control study. Lancet Diabetes Endocrinol. 3, 526–534. doi: 10.1016/S2213-8587(15)00127-8
Chatterjee, S., Khunti, K., and Davies, M. J. (2017). Type 2 diabetes. Lancet 389, 2239–2251. doi: 10.1016/S0140-6736(17)30058-2
Chen, B. H., Marioni, R. E., Colicino, E., Peters, M. J., Ward-Caviness, C. K., Tsai, P.-C., et al. (2016). DNA methylation-based measures of biological age: meta-analysis predicting time to death. Aging (Albany NY) 8, 1844–1865. doi: 10.18632/aging.101020
Chen, R., Xia, L., Tu, K., Duan, M., Kukurba, K., Li-Pook-Than, J., et al. (2018). Longitudinal personal DNA methylome dynamics in a human with a chronic condition. Nat. Med. 24, 1930–1939. doi: 10.1038/s41591-018-0237-x
Christiansen, L., Lenart, A., and Tan, Q., Vaupel, J. W., Aviv, A., McGue, M., et al. (2016). DNA methylation age is associated with mortality in a longitudinal Danish twin study. Aging Cell 15, 149–154. doi: 10.1111/acel.12421
Crimmins, E. M. (2015). Lifespan and healthspan: past, present, and promise. Gerontologist 55, 901–911. doi: 10.1093/geront/gnv130
Dayeh, T., Tuomi, T., Almgren, P., Perfilyev, A., Jansson, P.-A., de Mello, V. D., et al. (2016). DNA methylation of loci within ABCG1 and PHOSPHO1 in blood DNA is associated with future type 2 diabetes risk. Epigenetics 11, 482–488. doi: 10.1080/15592294.2016.1178418
De Jager, P. L., Srivastava, G., Lunnon, K., Burgess, J., Schalkwyk, L. C., Yu, L., et al. (2014). Alzheimer’s disease: early alterations in brain DNA methylation at ANK1, BIN1, RHBDF2 and other loci. Nat. Neurosci. 17, 1156–1163. doi: 10.1038/nn.3786
Di Francesco, A., Arosio, B., Falconi, A., Micioni Di Bonaventura, M. V., Karimi, M., Mari, D., et al. (2015). Global changes in DNA methylation in Alzheimer’s disease peripheral blood mononuclear cells. Brain Behav. Immun. 45, 139–144. doi: 10.1016/j.bbi.2014.11.002
Dogan, M. V., Beach, S., Simons, R., Lendasse, A., Penaluna, B., and Philibert, R. (2018a). Blood-based biomarkers for predicting the risk for five-year incident coronary heart disease in the Framingham Heart Study via machine learning. Genes 9:641. doi: 10.3390/genes9120641
Dogan, M. V., Grumbach, I. M., Michaelson, J. J., and Philibert, R. A. (2018b). Integrated genetic and epigenetic prediction of coronary heart disease in the Framingham Heart Study. PLoS One 13:e0190549. doi: 10.1371/journal.pone.0190549
Duong, S., Patel, T., and Chang, F. (2017). Dementia: what pharmacists need to know. Can. Pharm. J. (Ott) 150, 118–129. doi: 10.1177/1715163517690745
Edwards, T. M., and Myers, J. P. (2007). Environmental exposures and gene regulation in disease etiology. Environ. Health Perspect 115, 1264–1270. doi: 10.1289/ehp.9951
El Hajj, N., Dittrich, M., Böck, J., Kraus, T. F. J., Nanda, I., Müller, T., et al. (2016). Epigenetic dysregulation in the developing Down syndrome cortex. Epigenetics 11, 563–578. doi: 10.1080/15592294.2016.1192736
El Hajj, N., Haertle, L., Dittrich, M., Denk, S., Lehnen, H., Hahn, T., et al. (2017). DNA methylation signatures in cord blood of ICSI children. Hum. Reprod. 32, 1761–1769. doi: 10.1093/humrep/dex209
Finder, V. H. (2010). Alzheimer’s disease: a general introduction and pathomechanism. J. Alzheimers Dis. 22, S5–S19. doi: 10.3233/JAD-2010-100975
Florath, I., Butterbach, K., Heiss, J., Bewerunge-Hudler, M., Zhang, Y., Schöttker, B., et al. (2016). Type 2 diabetes and leucocyte DNA methylation: an epigenome-wide association study in over 1,500 older adults. Diabetologia 59, 130–138. doi: 10.1007/s00125-015-3773-7
Fraga, M. F., Ballestar, E., Paz, M. F., Ropero, S., Setien, F., Ballestar, M. L., et al. (2005). Epigenetic differences arise during the lifetime of monozygotic twins. Proc. Natl. Acad. Sci. U.S.A. 102, 10604–10609. doi: 10.1073/pnas.0500398102
Franceschi, C., Garagnani, P., Morsiani, C., Conte, M., Santoro, A., Grignolio, A., et al. (2018). The continuum of aging and age-related diseases: common mechanisms but different rates. Front. Med. (Lausanne) 5:61. doi: 10.3389/fmed.2018.00061
Frederiksen, H., Gaist, D., Christian Petersen, H., Hjelmborg, J., McGue, M., Vaupel, J. W., et al. (2002). Hand grip strength: a phenotype suitable for identifying genetic variants affecting mid- and late-life physical functioning. Genet. Epidemiol. 23, 110–122. doi: 10.1002/gepi.1127
Gallego-Fabrega, C., Carrera, C., Reny, J.-L., Fontana, P., Slowik, A., Pera, J., et al. (2016a). PPM1A methylation is associated with vascular recurrence in aspirin-treated patients. Stroke 47, 1926–1929. doi: 10.1161/STROKEAHA.116.013340
Gallego-Fabrega, C., Carrera, C., Reny, J.-L., Fontana, P., Slowik, A., Pera, J., et al. (2016b). TRAF3 epigenetic regulation is associated with vascular recurrence in patients with ischemic stroke. Stroke 47, 1180–1186. doi: 10.1161/STROKEAHA.115.012237
Galloway, A. (1993). The evolutionary biology of aging. By Michael R. Rose, New York: Oxford University Press. 1991. ix + 221 pp. ISBN 0-19-506133-0. $35.00 (cloth). Am. J. Phys. Anthropol. 91, 260–262. doi: 10.1002/ajpa.1330910217
García-Giménez, J. L., Ushijima, T., and Tollefsbol, T. O. (2016). “Epigenetic biomarkers: new findings, perspectives, and future directions in diagnostics,” in Epigenetic Biomarkers and Diagnostics, ed. J. L. García-Giménez (Amsterdam: Elsevier), 1–18. doi: 10.1016/B978-0-12-801899-6.00001-2
Greenwood, T. A., Beeri, M. S., Schmeidler, J., Valerio, D., Raventós, H., Mora-Villalobos, L., et al. (2011). Heritability of cognitive functions in families of successful cognitive aging probands from the Central Valley of Costa Rica. J. Alzheimers Dis. 27, 897–907. doi: 10.3233/JAD-2011-110782
Haertle, L., Müller, T., Lardenoije, R., Maierhofer, A., Dittrich, M., Riemens, R. J. M., et al. (2019). Methylomic profiling in trisomy 21 identifies cognition- and Alzheimer’s disease-related dysregulation. Clin. Epigenet. 11:195. doi: 10.1186/s13148-019-0787-x
Hall, E., Dayeh, T., Kirkpatrick, C. L., Wollheim, C. B., Dekker Nitert, M., and Ling, C. (2013). DNA methylation of the glucagon-like peptide 1 receptor (GLP1R) in human pancreatic islets. BMC Med. Genet. 14:76. doi: 10.1186/1471-2350-14-76
Han, Y., Eipel, M., Franzen, J., Sakk, V., Dethmers-Ausema, B., Yndriago, L., et al. (2018). Epigenetic age-predictor for mice based on three CpG sites. eLife 7:e37462. doi: 10.7554/eLife.37462
Hannum, G., Guinney, J., Zhao, L., Zhang, L., Hughes, G., Sadda, S., et al. (2013). Genome-wide methylation profiles reveal quantitative views of human aging rates. Mol. Cell 49, 359–367. doi: 10.1016/j.molcel.2012.10.016
Hardy, J. (2006). A hundred years of Alzheimer’s disease research. Neuron 52, 3–13. doi: 10.1016/j.neuron.2006.09.016
Heyn, H., Li, N., Ferreira, H. J., Moran, S., Pisano, D. G., Gomez, A., et al. (2012). Distinct DNA methylomes of newborns and centenarians. Proc. Natl. Acad. Sci.U.S.A. 109, 10522–10527. doi: 10.1073/pnas.1120658109
Hidalgo, B., Irvin, M. R., Sha, J., Zhi, D., Aslibekyan, S., Absher, D., et al. (2014). Epigenome-wide association study of fasting measures of glucose, insulin, and HOMA-IR in the genetics of lipid lowering drugs and diet network study. Diabetes 63, 801–807. doi: 10.2337/db13-1100
Horvath, S. (2013). DNA methylation age of human tissues and cell types. Genome Biol. 14:R115. doi: 10.1186/gb-2013-14-10-r115
Horvath, S., Erhart, W., Brosch, M., Ammerpohl, O., von Schonfels, W., Ahrens, M., et al. (2014). Obesity accelerates epigenetic aging of human liver. Proc. Natl. Acad. Sci. U.S.A. 111, 15538–15543. doi: 10.1073/pnas.1412759111
Horvath, S., Garagnani, P., Bacalini, M. G., Pirazzini, C., Salvioli, S., Gentilini, D., et al. (2015). Accelerated epigenetic aging in Down syndrome. Aging Cell 14, 491–495. doi: 10.1111/acel.12325
Horvath, S., Gurven, M., Levine, M. E., Trumble, B. C., Kaplan, H., Allayee, H., et al. (2016a). An epigenetic clock analysis of race/ethnicity, sex, and coronary heart disease. Genome Biol. 17:171. doi: 10.1186/s13059-016-1030-0
Horvath, S., Langfelder, P., Kwak, S., Aaronson, J., Rosinski, J., Vogt, T. F., et al. (2016b). Huntington’s disease accelerates epigenetic aging of human brain and disrupts DNA methylation levels. Aging 8, 1485–1512. doi: 10.18632/aging.101005
Horvath, S., and Raj, K. (2018). DNA methylation-based biomarkers and the epigenetic clock theory of ageing. Nat. Rev. Genet. 19, 371–384. doi: 10.1038/s41576-018-0004-3
Horvath, S., and Ritz, B. R. (2015). Increased epigenetic age and granulocyte counts in the blood of Parkinson’s disease patients. Aging 7, 1130–1142. doi: 10.18632/aging.100859
Infante, T., Forte, E., Schiano, C., Punzo, B., Cademartiri, F., Cavaliere, C., et al. (2019). Evidence of association of circulating epigenetic-sensitive biomarkers with suspected coronary heart disease evaluated by Cardiac Computed Tomography. PLoS One 14:e0210909. doi: 10.1371/journal.pone.0210909
Issa, J.-P. (2014). Aging and epigenetic drift: a vicious cycle. J. Clin. Invest. 124, 24–29. doi: 10.1172/JCI69735
Jacobs, E., Hoyer, A., Brinks, R., Kuss, O., and Rathmann, W. (2017). Burden of mortality attributable to diagnosed diabetes: a nationwide analysis based on claims data from 65 million people in Germany. Diabetes Care 40, 1703–1709. doi: 10.2337/dc17-0954
Jaul, E., and Barron, J. (2017). Age-related diseases and clinical and public health implications for the 85 years old and over population. Front. Public Health 5:335. doi: 10.3389/fpubh.2017.00335
Jiang, D., Sun, M., You, L., Lu, K., Gao, L., Hu, C., et al. (2019a). DNA methylation and hydroxymethylation are associated with the degree of coronary atherosclerosis in elderly patients with coronary heart disease. Life Sci. 224, 241–248. doi: 10.1016/j.lfs.2019.03.021
Jiang, D., Wang, Y., Chang, G., Duan, Q., You, L., Sun, M., et al. (2019b). DNA hydroxymethylation combined with carotid plaques as a novel biomarker for coronary atherosclerosis. Aging 11, 3170–3181. doi: 10.18632/aging.101972
Kananen, L., Marttila, S., Nevalainen, T., Kummola, L., Junttila, I., Mononen, N., et al. (2016). The trajectory of the blood DNA methylome ageing rate is largely set before adulthood: evidence from two longitudinal studies. Age 38:65. doi: 10.1007/s11357-016-9927-9
Kato, N., Loh, M., Takeuchi, F., Verweij, N., Wang, X., Zhang, W., et al. (2015). Trans-ancestry genome-wide association study identifies 12 genetic loci influencing blood pressure and implicates a role for DNA methylation. Nat. Genet. 47, 1282–1293. doi: 10.1038/ng.3405
Khare, T., Pai, S., Koncevicius, K., Pal, M., Kriukiene, E., Liutkeviciute, Z., et al. (2012). 5-hmC in the brain is abundant in synaptic genes and shows differences at the exon-intron boundary. Nat. Struct. Mol. Biol. 19, 1037–1043. doi: 10.1038/nsmb.2372
Kim, M., Long, T. I., Arakawa, K., Wang, R., Yu, M. C., and Laird, P. W. (2010). DNA methylation as a biomarker for cardiovascular disease risk. PLoS One 5:e9692. doi: 10.1371/journal.pone.0009692
Knight, A. K., Craig, J. M., Theda, C., Bækvad-Hansen, M., Bybjerg-Grauholm, J., Hansen, C. S., et al. (2016). An epigenetic clock for gestational age at birth based on blood methylation data. Genome Biol. 17:206. doi: 10.1186/s13059-016-1068-z
Kobayashi, N., Shinagawa, S., Nagata, T., Shimada, K., Shibata, N., Ohnuma, T., et al. (2016). Usefulness of DNA methylation levels in COASY and SPINT1 gene promoter regions as biomarkers in diagnosis of Alzheimer’s disease and amnestic mild cognitive impairment. PLoS One 11:e0168816. doi: 10.1371/journal.pone.0168816
Kriaucionis, S., and Heintz, N. (2009). The nuclear DNA Base 5-hydroxymethylcytosine is present in purkinje neurons and the brain. Science 324, 929–930. doi: 10.1126/science.1169786
Kulkarni, H., Kos, M. Z., Neary, J., Dyer, T. D., Kent, J. W., Göring, H. H. H., et al. (2015). Novel epigenetic determinants of type 2 diabetes in Mexican-American families. Hum. Mol. Genet. 24, 5330–5344. doi: 10.1093/hmg/ddv232
Lardenoije, R., Iatrou, A., Kenis, G., Kompotis, K., Steinbusch, H. W. M., Mastroeni, D., et al. (2015). The epigenetics of aging and neurodegeneration. Prog. Neurobiol. 131, 21–64. doi: 10.1016/j.pneurobio.2015.05.002
Lardenoije, R., Roubroeks, J. A. Y., Pishva, E., Leber, M., Wagner, H., Iatrou, A., et al. (2019). Alzheimer’s disease-associated (hydroxy)methylomic changes in the brain and blood. Clin. Epigenet. 11:164. doi: 10.1186/s13148-019-0755-5
Lévesque, M. L., Casey, K. F., Szyf, M., Ismaylova, E., Ly, V., Verner, M.-P., et al. (2014). Genome-wide DNA methylation variability in adolescent monozygotic twins followed since birth. Epigenetics 9, 1410–1421. doi: 10.4161/15592294.2014.970060
Levine, M. E., Hosgood, H. D., Chen, B., Absher, D., Assimes, T., and Horvath, S. (2015a). DNA methylation age of blood predicts future onset of lung cancer in the women’s health initiative. Aging 7, 690–700. doi: 10.18632/aging.100809
Levine, M. E., Lu, A. T., Bennett, D. A., and Horvath, S. (2015b). Epigenetic age of the pre-frontal cortex is associated with neuritic plaques, amyloid load, and Alzheimer’s disease related cognitive functioning. Aging 7, 1198–1211. doi: 10.18632/aging.100864
Levine, M. E., Lu, A. T., Quach, A., Chen, B. H., Assimes, T. L., Bandinelli, S., et al. (2018). An epigenetic biomarker of aging for lifespan and healthspan. Aging 10, 573–591. doi: 10.18632/aging.101414
Li, J., Zhu, X., Yu, K., Jiang, H., Zhang, Y., Deng, S., et al. (2017). Genome-wide analysis of DNA methylation and acute coronary syndrome. Cir. Res. 120, 1754–1767. doi: 10.1161/CIRCRESAHA.116.310324
Ligthart, S., Marzi, C., Aslibekyan, S., Mendelson, M. M., Conneely, K. N., Tanaka, T., et al. (2016). DNA methylation signatures of chronic low-grade inflammation are associated with complex diseases. Genome Biol. 17:255. doi: 10.1186/s13059-016-1119-5
Lind, L., Ingelsson, E., Sundström, J., Siegbahn, A., and Lampa, E. (2018). Methylation-based estimated biological age and cardiovascular disease. Eur. J. Clin. Invest. 48:e12872. doi: 10.1111/eci.12872
Ling, C., Del Guerra, S., Lupi, R., Rönn, T., Granhall, C., Luthman, H., et al. (2008). Epigenetic regulation of PPARGC1A in human type 2 diabetic islets and effect on insulin secretion. Diabetologia 51, 615–622. doi: 10.1007/s00125-007-0916-5
Ling, C., and Rönn, T. (2019). Epigenetics in human obesity and type 2 diabetes. Cell Metab. 29, 1028–1044. doi: 10.1016/j.cmet.2019.03.009
Liu, J., Jiang, J., Mo, J., Liu, D., Cao, D., Wang, H., et al. (2019). Global DNA 5-hydroxymethylcytosine and 5-formylcytosine contents are decreased in the early stage of hepatocellular carcinoma. Hepatology 69, 196–208. doi: 10.1002/hep.30146
Liu, Y., Peng, W., Qu, K., Lin, X., Zeng, Z., Chen, J., et al. (2018). TET2: a novel epigenetic regulator and potential intervention target for atherosclerosis. DNA Cell Biol. 37, 517–523. doi: 10.1089/dna.2017.4118
López-Otín, C., Blasco, M. A., Partridge, L., Serrano, M., and Kroemer, G. (2013). The hallmarks of aging. Cell 153, 1194–1217. doi: 10.1016/j.cell.2013.05.039
Lu, A. T., Hannon, E., Levine, M. E., Crimmins, E. M., Lunnon, K., Mill, J., et al. (2017). Genetic architecture of epigenetic and neuronal ageing rates in human brain regions. Nat. Commun. 8:15353. doi: 10.1038/ncomms15353
Lu, A. T., Hannon, E., Levine, M. E., Hao, K., Crimmins, E. M., Lunnon, K., et al. (2016). Genetic variants near MLST8 and DHX57 affect the epigenetic age of the cerebellum. Nat. Commun. 7:10561. doi: 10.1038/ncomms10561
Lu, A. T., Quach, A., Wilson, J. G., Reiner, A. P., Aviv, A., Raj, K., et al. (2019). DNA methylation GrimAge strongly predicts lifespan and healthspan. Aging 11, 303–327. doi: 10.18632/aging.101684
Lu, A. T., Xue, L., Salfati, E. L., Chen, B. H., Ferrucci, L., Levy, D., et al. (2018). GWAS of epigenetic aging rates in blood reveals a critical role for TERT. Nat. Commun. 9:387. doi: 10.1038/s41467-017-02697-5
Lunnon, K., Smith, R., Hannon, E., De Jager, P. L., Srivastava, G., Volta, M., et al. (2014). Methylomic profiling implicates cortical deregulation of ANK1 in Alzheimer’s disease. Nat. Neurosci. 17, 1164–1170. doi: 10.1038/nn.3782
Maierhofer, A., Flunkert, J., Oshima, J., Martin, G. M., Haaf, T., and Horvath, S. (2017). Accelerated epigenetic aging in Werner syndrome. Aging 9, 1143–1152. doi: 10.18632/aging.101217
Mano, T., Nagata, K., Nonaka, T., Tarutani, A., Imamura, T., Hashimoto, T., et al. (2017). Neuron-specific methylome analysis reveals epigenetic regulation and tau-related dysfunction of BRCA1 in Alzheimer’s disease. Proc. Natl. Acad. Sci. 114, E9645–E9654. doi: 10.1073/pnas.1707151114
Marioni, R. E., Shah, S., McRae, A. F., Chen, B. H., Colicino, E., Harris, S. E., et al. (2015). DNA methylation age of blood predicts all-cause mortality in later life. Genome Biol. 16:25. doi: 10.1186/s13059-015-0584-6
McCarthy, M. I. (2010). Genomics, type 2 diabetes, and obesity. N. Engl. J. Med. 363, 2339–2350. doi: 10.1056/NEJMra0906948
Meer, M. V., Podolskiy, D. I, Tyshkovskiy, A., and Gladyshev, V. N. (2018). A whole lifespan mouse multi-tissue DNA methylation clock. eLife 7:e40675. doi: 10.7554/eLife.40675
Mercorio, R., Pergoli, L., Galimberti, D., Favero, C., Carugno, M., Dalla Valle, E., et al. (2018). PICALM gene methylation in blood of Alzheimer’s disease patients is associated with cognitive decline. J. Alzheimers Dis. 65, 283–292. doi: 10.3233/JAD-180242
Moore, L. D., Le, T., and Fan, G. (2013). DNA methylation and its basic function. Neuropsychopharmacology 38, 23–38. doi: 10.1038/npp.2012.112
Nagata, T., Kobayashi, N., Ishii, J., Shinagawa, S., Nakayama, R., Shibata, N., et al. (2015). Association between DNA methylation of the BDNF promoter region and clinical presentation in Alzheimer’s disease. Dement. Geriatr. Cogn. Dis. Extra 5, 64–73. doi: 10.1159/000375367
Nakatochi, M., Ichihara, S., Yamamoto, K., Naruse, K., Yokota, S., Asano, H., et al. (2017). Epigenome-wide association of myocardial infarction with DNA methylation sites at loci related to cardiovascular disease. Clin. Epigenetics 9:54. doi: 10.1186/s13148-017-0353-3
Napoli, C., Crudele, V., Soricelli, A., Al-Omran, M., Vitale, N., Infante, T., et al. (2012). Primary prevention of atherosclerosis: a clinical challenge for the reversal of epigenetic mechanisms? Circulation 125, 2363–2373. doi: 10.1161/CIRCULATIONAHA.111.085787
Newman, P. E. (1999). Can reduced folic acid and vitamin B12 levels cause deficient DNA methylation producing mutations which initiate atherosclerosis? Med. Hypotheses 53, 421–424. doi: 10.1054/mehy.1998.0794
Ozaki, Y., Yoshino, Y., Yamazaki, K., Sao, T., Mori, Y., Ochi, S., et al. (2017). DNA methylation changes at TREM2 intron 1 and TREM2 mRNA expression in patients with Alzheimer’s disease. J. Psychiatr. Res. 92, 74–80. doi: 10.1016/j.jpsychires.2017.04.003
Petkovich, D. A., Podolskiy, D. I, Lobanov, A. V., Lee, S.-G., Miller, R. A., and Gladyshev, V. N. (2017). Using DNA methylation profiling to evaluate biological age and longevity interventions. Cell Metab. 25, 954–960.e6. doi: 10.1016/j.cmet.2017.03.016
Potabattula, R., Dittrich, M., Böck, J., Haertle, L., Müller, T., Hahn, T., et al. (2018). Allele-specific methylation of imprinted genes in fetal cord blood is influenced by cis-acting genetic variants and parental factors. Epigenomics 10, 1315–1326. doi: 10.2217/epi-2018-0059
Poulsen, P., Esteller, M., Vaag, A., and Fraga, M. F. (2007). The epigenetic basis of twin discordance in age-related diseases. Pediatr. Res. 61, 38R–42R. doi: 10.1203/pdr.0b013e31803c7b98
Quach, A., Levine, M. E., Tanaka, T., Lu, A. T., Chen, B. H., Ferrucci, L., et al. (2017). Epigenetic clock analysis of diet, exercise, education, and lifestyle factors. Aging 9, 419–446. doi: 10.18632/aging.101168
Rask-Andersen, M., Martinsson, D., Ahsan, M., Enroth, S., Ek, W. E., Gyllensten, U., et al. (2016). Epigenome-wide association study reveals differential DNA methylation in individuals with a history of myocardial infarction. Hum. Mol. Genet. 25, 4739–4748. doi: 10.1093/hmg/ddw302
Reynolds, C. A., Finkel, D., McArdle, J. J., Gatz, M., Berg, S., and Pedersen, N. L. (2005). Quantitative genetic analysis of latent growth curve models of cognitive abilities in adulthood. Dev. Psychol. 41, 3–16. doi: 10.1037/0012-1649.41.1.3
Seman, N., Mohamud, W., Östenson, C.-G., Brismar, K., and Gu, H. F. (2015). Increased DNA methylation of the SLC30A8 gene promoter is associated with type 2 diabetes in a Malay population. Clin. Epigenetics 7:30. doi: 10.1186/s13148-015-0049-5
Sharma, P., Garg, G., Kumar, A., Mohammad, F., Kumar, S. R., Tanwar, V. S., et al. (2014). Genome wide DNA methylation profiling for epigenetic alteration in coronary artery disease patients. Gene 541, 31–40. doi: 10.1016/j.gene.2014.02.034
Sharma, P., Kumar, J., Garg, G., Kumar, A., Patowary, A., Karthikeyan, G., et al. (2008). Detection of altered global dna methylation in coronary artery disease patients. DNA Cell Biol. 27, 357–365. doi: 10.1089/dna.2007.0694
Shinagawa, S., Kobayashi, N., Nagata, T., Kusaka, A., Yamada, H., Kondo, K., et al. (2016). DNA methylation in the NCAPH2/LMF2 promoter region is associated with hippocampal atrophy in Alzheimer’s disease and amnesic mild cognitive impairment patients. Neurosci. Lett. 629, 33–37. doi: 10.1016/j.neulet.2016.06.055
Soriano-Tárraga, C., Jiménez-Conde, J., Giralt-Steinhauer, E., Mola-Caminal, M., Vivanco-Hidalgo, R. M., Ois, A., et al. (2016). Epigenome-wide association study identifies TXNIP gene associated with type 2 diabetes mellitus and sustained hyperglycemia. Hum. Mol. Genet. 25, 609–619. doi: 10.1093/hmg/ddv493
Spiers, H., Hannon, E., Schalkwyk, L. C., Smith, R., Wong, C. C. Y., O’Donovan, M. C., et al. (2015). Methylomic trajectories across human fetal brain development. Genome Res. 25, 338–352. doi: 10.1101/gr.180273.114
Tan, Q., Heijmans, B. T., Hjelmborg, J. V. B., Soerensen, M., Christensen, K., and Christiansen, L. (2016). Epigenetic drift in the aging genome: a ten-year follow-up in an elderly twin cohort. Int. J. Epidemiol. 45, 1146–1158. doi: 10.1093/ije/dyw132
Thompson, M. J., Chwiałkowska, K., Rubbi, L., Lusis, A. J., Davis, R. C., Srivastava, A., et al. (2018). A multi-tissue full lifespan epigenetic clock for mice. Aging 10, 2832–2854. doi: 10.18632/aging.101590
Tohgi, H., Utsugisawa, K., Nagane, Y., Yoshimura, M., Genda, Y., and Ukitsu, M. (1999). Reduction with age in methylcytosine in the promoter region -224 approximately -101 of the amyloid precursor protein gene in autopsy human cortex. Brain Res. Mol. Brain Res. 70, 288–292. doi: 10.1016/s0169-328x(99)00163-1
Toperoff, G., Aran, D., Kark, J. D., Rosenberg, M., Dubnikov, T., Nissan, B., et al. (2012). Genome-wide survey reveals predisposing diabetes type 2-related DNA methylation variations in human peripheral blood. Hum. Mol. Genet. 21, 371–383. doi: 10.1093/hmg/ddr472
Vanyushin, B. F., Nemirovsky, L. E., Klimenko, V. V., Vasiliev, V. K., and Belozersky, A. N. (1973). The 5-methylcytosine in DNA of rats. Tissue and age specificity and the changes induced by hydrocortisone and other agents. Gerontologia 19, 138–152.
Volkov, P., Bacos, K., Ofori, J. K., Esguerra, J. L. S., Eliasson, L., Rönn, T., et al. (2017). Whole-genome bisulfite sequencing of human pancreatic islets reveals novel differentially methylated regions in type 2 diabetes pathogenesis. Diabetes 66, 1074–1085. doi: 10.2337/db16-0996
Wahl, S., Drong, A., Lehne, B., Loh, M., Scott, W. R., Kunze, S., et al. (2017). Epigenome-wide association study of body mass index, and the adverse outcomes of adiposity. Nature 541, 81–86. doi: 10.1038/nature20784
Walaszczyk, E., Luijten, M., Spijkerman, A. M. W., Bonder, M. J., Lutgers, H. L., Snieder, H., et al. (2018). DNA methylation markers associated with type 2 diabetes, fasting glucose and HbA1c levels: a systematic review and replication in a case–control sample of the Lifelines study. Diabetologia 61, 354–368. doi: 10.1007/s00125-017-4497-7
Wang, M., and Lemos, B. (2019). Ribosomal DNA harbors an evolutionarily conserved clock of biological aging. Genome Res. 29, 325–333. doi: 10.1101/gr.241745.118
Wang, S.-C., Oelze, B., and Schumacher, A. (2008). Age-specific epigenetic drift in late-onset Alzheimer’s disease. PLoS One 3:e2698. doi: 10.1371/journal.pone.0002698
Wang, T., Tsui, B., Kreisberg, J. F., Robertson, N. A., Gross, A. M., Yu, M. K., et al. (2017). Epigenetic aging signatures in mice livers are slowed by dwarfism, calorie restriction and rapamycin treatment. Genome Biol. 18:57. doi: 10.1186/s13059-017-1186-2
Wang, Y., Karlsson, R., Lampa, E., Zhang, Q., Hedman, ÅK., Almgren, M., et al. (2018). Epigenetic influences on aging: a longitudinal genome-wide methylation study in old Swedish twins. Epigenetics 13, 975–987. doi: 10.1080/15592294.2018.1526028
Weidner, C., Lin, Q., Koch, C., Eisele, L., Beier, F., Ziegler, P., et al. (2014). Aging of blood can be tracked by DNA methylation changes at just three CpG sites. Genome Biol. 15:R24. doi: 10.1186/gb-2014-15-2-r24
West, R. L., Lee, J. M., and Maroun, L. E. (1995). Hypomethylation of the amyloid precursor protein gene in the brain of an Alzheimer’s disease patient. J. Mol. Neurosci. 6, 141–146. doi: 10.1007/BF02736773
Westerman, K., Sebastiani, P., Jacques, P., Liu, S., DeMeo, D., and Ordovás, J. M. (2018). DNA methylation modules associate with incident cardiovascular disease and cumulative risk factor exposure. bioRxiv [Preprint]. doi: 10.1101/471722
Willmer, T., Johnson, R., Louw, J., and Pheiffer, C. (2018). Blood-based DNA methylation biomarkers for type 2 diabetes: potential for clinical applications. Front. Endocrinol. 9:744. doi: 10.3389/fendo.2018.00744
Xie, B., Liu, Z., Liu, W., Jiang, L., Zhang, R., Cui, D., et al. (2017a). DNA methylation and tag SNPs of the BDNF gene in conversion of amnestic mild cognitive impairment into Alzheimer’s disease: a cross-sectional cohort study. J. Alzheimers Dis. 58, 263–274. doi: 10.3233/JAD-170007
Xie, B., Xu, Y., Liu, Z., Liu, W., Jiang, L., Zhang, R., et al. (2017b). Elevation of peripheral BDNF promoter methylation predicts conversion from amnestic mild cognitive impairment to Alzheimer’s disease: a 5-year longitudinal study. J. Alzheimers Dis. 56, 391–401. doi: 10.3233/JAD-160954
Yang, B. T., Dayeh, T. A., Volkov, P. A., Kirkpatrick, C. L., Malmgren, S., Jing, X., et al. (2012). Increased DNA methylation and decreased expression of PDX-1 in pancreatic islets from patients with type 2 diabetes. Mol. Endocrinol. 26, 1203–1212. doi: 10.1210/me.2012-1004
Yuan, W., Xia, Y., Bell, C. G., Yet, I., Ferreira, T., Ward, K. J., et al. (2014). An integrated epigenomic analysis for type 2 diabetes susceptibility loci in monozygotic twins. Nat. Commun. 5:5719. doi: 10.1038/ncomms6719
Zaina, S., Heyn, H., Carmona, F. J., Varol, N., Sayols, S., Condom, E., et al. (2014). DNA methylation map of human atherosclerosis. Circ. Cardiovasc. Genet. 7, 692–700. doi: 10.1161/CIRCGENETICS.113.000441
Zheng, Y., Joyce, B. T., Colicino, E., Liu, L., Zhang, W., Dai, Q., et al. (2016). Blood epigenetic age may predict cancer incidence and mortality. EBioMedicine 5, 68–73. doi: 10.1016/j.ebiom.2016.02.008
Ziller, M. J., Gu, H., Müller, F., Donaghey, J., Tsai, L. T.-Y., Kohlbacher, O., et al. (2013). Charting a dynamic DNA methylation landscape of the human genome. Nature 500, 477–481. doi: 10.1038/nature12433
Keywords: aging, DNA methylation, epigenetic clocks, biomarkers, Alzheimer’s disease, diabetes, cardiovascular diseases
Citation: Salameh Y, Bejaoui Y and El Hajj N (2020) DNA Methylation Biomarkers in Aging and Age-Related Diseases. Front. Genet. 11:171. doi: 10.3389/fgene.2020.00171
Received: 25 June 2019; Accepted: 13 February 2020;
Published: 10 March 2020.
Edited by:
Trygve Tollefsbol, University of Alabama at Birmingham, United StatesReviewed by:
Francesco Marabita, Karolinska Institutet (KI), SwedenSimonetta Friso, University of Verona, Italy
Copyright © 2020 Salameh, Bejaoui and El Hajj. This is an open-access article distributed under the terms of the Creative Commons Attribution License (CC BY). The use, distribution or reproduction in other forums is permitted, provided the original author(s) and the copyright owner(s) are credited and that the original publication in this journal is cited, in accordance with accepted academic practice. No use, distribution or reproduction is permitted which does not comply with these terms.
*Correspondence: Nady El Hajj, bmVsaGFqakBoYmt1LmVkdS5xYQ==
†These authors have contributed equally to this work