- 1Department of Radiology, Shaoxing People’s Hospital, Shaoxing, China
- 2Department of Radiology, The Second Affiliated Hospital, Zhejiang University School of Medicine, Hangzhou, China
- 3Department of Radiology, Shaoxing Maternity and Child Health Care Hospital, Shaoxing, Zhejiang, China
Objectives: To investigate whether cerebrospinal fluid (CSF) circulation markers alter in patients with probable cerebral amyloid angiopathy (pCAA) and whether they are associated with brain degeneration and cognitive impairment.
Methods: We screened pCAA patients from the ADNI3 database according to the Boston 2.0 Criteria. Fifty-two patients with cognitive impairment (26 pCAA; 26 age-sex-matched non-pCAA) and 26 age-sex-matched cognitively normal control (NC) were included in this study. All participants underwent neurological MRI and cognitive assessments. Choroid plexus (ChP) was segmented using a deep learning-based method and its volume was extracted. Diffusion tensor imaging analysis along the perivascular space (DTI-ALPS) was used to assess perivenous fluid mobility. AD pathological markers (Aβ and tau) were assessed using positron emission tomography. Brain parenchymal damage markers included white matter hyperintensities (WMH) volume and brain atrophy ratio. All markers were compared among the three groups. Correlations among the ChP volume, DTI-ALPS index, parenchymal damage markers, and cognitive scales were analyzed in the pCAA group.
Results: The three groups exhibited significant differences in cognitive scores, AD biomarkers, and imaging markers. Post hoc analyses showed that patients with pCAA had significantly higher WMH volume, higher Aβ and tau deposition, and lower DTI-ALPS compared to NC. However, no difference in ChPs volume was found among the groups. Controlling for age, sex, and vascular risk factors, partial correlation analyses showed a significant negative correlation between the DTI-ALPS and WMH volume fraction (r = −0.606, p = 0.002). ChP volume was significantly associated with the Montreal cognitive assessment score (r = −0.492, p = 0.028).
Conclusion: CSF circulation markers were associated with elevated WMH burden and cognitive impairments in probable CAA.
Introduction
Cerebral amyloid angiopathy (CAA) is a specific type of small vessel disease defined by β-amyloid (Aβ) deposition in cortical and leptomeningeal vessels (Charidimou et al., 2022; Beaman et al., 2022; Koemans et al., 2023; Greenberg and van Veluw, 2024). Aβ deposition may causes various detrimental effects, including vessel wall thickening and stiffening, loss of vascular smooth muscle cells, blood–brain barrier disruption, etc. (Beaudin et al., 2022; Deng et al., 2022; Chen et al., 2023; Fotiadis et al., 2016, 2021; Greenberg et al., 2020). These pathological factors can further lead to hemorrhagic or ischemic events and brain degeneration (Charidimou et al., 2019; Beaman et al., 2022; Wang et al., 2024; Rabin et al., 2022).
In recent years, the development of the brain waste clearance theory has provided new insights into the mechanisms of CAA-related brain degeneration (Nedergaard and Goldman, 2020). The choroid plexus (ChP) is the primary site of cerebrospinal fluid (CSF) secretion (Wichmann et al., 2021). CSF flows through the ventricles and subarachnoid spaces, further entering the perivascular spaces and brain parenchyma, where it flushes out metabolic waste (Jessen et al., 2015). Previous pathological and animal studies have found that Aβ may deposit in the ChP (Sasaki et al., 1997), inducing cellular toxicity and apoptosis (Vargas et al., 2010). Additionally, since vessel pulsatility is considered a major driving factor of CSF flow (Xie et al., 2024), Aβ deposition in cortical and leptomeningeal vessels may disrupt vessel wall movement and reduce CSF mobility. This can lead to stagnation of interstitial fluid (ISF), accumulation of toxic substances in the parenchyma, and subsequent neurodegeneration (Durrani et al., 2023; Chen et al., 2022; Kim et al., 2020; van Veluw et al., 2024b). This theory offers a new perspective for understanding the progression of brain degeneration in CAA (van Veluw et al., 2024a). However, as most previous studies were conducted in rodent models, there is a critical need for clinical translational research.
Magnetic resonance imaging (MRI) provides a clear visualization of ChP structure. Previous studies have found that ChP volume increases with aging and in various neurodegenerative diseases (Čarna et al., 2023; Jeong et al., 2023), potentially indicating pathological hypertrophy and decreased CSF production. However, no in vivo investigations have been conducted to examine ChP changes in CAA. Diffusion tensor imaging analysis along the perivascular space (DTI-ALPS) (Taoka et al., 2017) is a method used to evaluate fluid motion in the perivenous spaces. Research has demonstrated a strong correlation between DTI-ALPS and various neurological disorders (Hong et al., 2024; Jiang et al., 2023). Furthermore, DTI-ALPS can predict long-term outcomes in patients with Alzheimer’s disease (AD) (Huang et al., 2024), Parkinson’s disease (Zhou et al., 2024), and other conditions. Utilizing these CSF circulation markers may help elucidate the association between waste clearance and brain degeneration in CAA.
The definite diagnosis of CAA relies on histopathological analysis from brain autopsy or biopsy samples. Nonetheless, probable diagnosis could be inferred according to the Boston criteria, based on clinical and MRI information. In 2022, the Boston criteria were updated to version 2.0 (Charidimou et al., 2022), which demonstrated superior accuracy compared to the older version. To be specific, the Boston criteria 2.0 classify CAA into different diagnostic certainty levels: definite CAA, probable CAA with supporting pathology, probable CAA (pCAA), and possible CAA. The first two categories require full postmortem brain examination or biopsy confirmation. The pCAA is diagnosed based on combined clinical and imaging criteria. Clinical manifestations include spontaneous intracerebral hemorrhage, transient focal neurological episodes, or cognitive impairment/dementia (Perini et al., 2023). Imaging criteria require either: (1) at least two strictly lobar hemorrhagic lesions on T2*-weighted MRI, or (2) one lobar hemorrhagic lesion plus one white matter feature (either severe centrum semiovale perivascular spaces or multifocal white matter hyperintensities). Although the diagnosis of pCAA is not definitive, it provides a valuable framework for clinical research on this important disease.
In this study, we aimed to: (1) determine whether the two CSF circulation markers were altered in patients with pCAA as defined by the new Boston 2.0 criteria, and (2) examine the associations between CSF circulation markers, brain degeneration, and cognitive impairments. We hypothesized that patients with probable CAA exhibit altered CSF circulation markers, which may contribute to brain degeneration.
Methods and materials
Participants
The data used in this article were from the Alzheimer’s Disease Neuroimaging Initiative (ADNI) database.1 The database was launched by the National Institute on Aging (NIA), the Food and Drug Administration (FDA), and the National Institute of Biomedical Imaging and Bioengineering (NIBIB), aiming to explore whether serial MRI, positron emission tomography (PET), biological markers, and other neuropsychological assessment can be used for early detecting and tracking AD. Participant inclusion and exclusion criteria are available at www.adni-info.org. All procedures performed in studies involving human participants were under the ethical standards of the Institutional and National Research Committee and with the 1964 Helsinki Declaration and its later amendments or comparable ethical standards. Written informed consent was from all participants and authorized representatives before any protocol-specific procedures were carried out in the ADNI study. More details can be found at http://www.adni-info.org.
The diagnosis of pCAA was based on the Boston Criteria version 2.0 (Figure 1). A radiologist with over 10 years of diagnostic experience evaluated the entire ADNI3 baseline dataset, identifying lobar hemorrhagic lesions on T2* images. According to the Boston Criteria, subjects with cognitive impairments and either more than two lobar hemorrhagic lesions or one lobar hemorrhagic lesion plus one white matter feature (e.g., severe perivascular spaces in the centrum semiovale or white matter hyperintensities in a multispot pattern) were classified as pCAA. Since the diagnosis of probable CAA also requires clinical impairment, we included only those with cognitive impairment. To control for the potential influence of cognitive status, which has been associated with CSF circulation abnormalities, we also included a group with MCI but without CAA (MCI-nonCAA). Finally, 26 pCAA cases, 26 age- and sex-matched MCI-nonCAA, along with 26 age- and sex-matched normal controls (NC) were included in this study. The groups were matched using the propensity score matching tool in SPSS Statistics Version 27.0 (IBM).
Demographic and clinical data
Demographic and clinical data were obtained from the ADNI database,2 including age, sex, smoking status, and vascular risk factors such as hypertension, diabetes, hyperlipidemia, stroke, myocardial infarction, and atrial fibrillation. A summary vascular risk factor score (VRFs) was calculated by summing the presence of each factor.
Neuropsychological assessment
The neuropsychological assessment included the mini-mental state examination (MMSE), Montreal cognitive assessment (MoCA), and clinical dementia rating scale sum of boxes (CDR-SOB).
PET acquisition and preprocessing
The amyloid PET scans were performed using two tracers (florbetapir, or FBP; florbetaben, or FBB). For this study, we analyzed amyloid PET data processed by UC Berkeley and Lawrence Berkeley National Laboratory. The summary standardized uptake value ratio (SUVR) was calculated by averaging the uptake values across four primary cortical regions (frontal, anterior/posterior cingulate, lateral parietal, and lateral temporal) and normalizing by the whole cerebellum. To promote the harmonization of Aβ PET imaging, we utilized data from FBB and FBP to derive standardized Centiloid (CL) conversions, as previously described (Royse et al., 2021).
The tau PET scans were performed using one tracer (flortaucipir, or FTP). We also analyzed tau PET data processed by UC Berkeley and Lawrence Berkeley National Laboratory. The SUVR for the temporal meta-ROI was determined as a volume-weighted average of the SUVRs from the entorhinal cortex, amygdala, parahippocampal gyrus, fusiform gyrus, inferior temporal cortex, and middle temporal cortex. The detailed acquisition protocols are described in the ADNI PET Technical Procedures Manual.3
Magnetic resonance imaging acquisition
The imaging data from the ADNI were acquired from multiple centers utilizing standardized protocols. We include 3D T1-weighted (T1w), 3D T2 FLAIR, axial T2*-weighted, and axial diffusion tensor imaging (DTI) sequence. All imaging data were obtained using 3T scanners manufactured by three leading vendors (GE, Siemens, and Philips). Detailed information on the parameters can be found at https://adni.loni.usc.edu/methods/documents/mri-protocols/.
Brain segmentation
We performed brain segmentation in each subject using the “recon-all” processing pipeline in FreeSurfer (version 6.0).4 It segmented different tissue types and estimated the total intracranial volume (TIV). We calculated the brain parenchymal-to-TIV ratio to reflect brain atrophy.
WMH assessment and segmentation
T2 FLAIR images were used for WMH assessment. Visual assessment was performed using the Fazekas grading (Fazekas et al., 1993). For volume quantification, an automatic segmentation tool (Lesion Segmentation Tool) based on the lesion prediction algorithm of the Statistical Parametric Mapping software (SPM12)5 was used. The automatically created WMH labeled images were then manually corrected for misclassified tissues. WMH volume was extracted and normalized using the subject’s TIV.
Choroid plexus segmentation
For each subject, the 3D FLAIR image was registered to the 3D T1w image using linear registration. The ChP was then segmented based on both 3D T1w and 3D FLAIR images using an in-house-trained deep learning model. This model was trained using 44 subjects scanned with various scanners and tested on 15 subjects. Compared with manual labels, the model demonstrated excellent performance on the testing dataset, achieving a mean Dice coefficient of 0.878, a mean precision of 0.872, and a mean recall of 0.888. Visual inspection was performed to ensure segmentation quality. The ChP volume was then calculated and normalized using the subject’s total intracranial volume (TIV).
DTI-ALPS evaluation
The processing of DTI data was conducted using FSL 6.06 and MRtrix3.7 Preprocessing steps included Gibbs ringing removal, eddy-current and head motion correction, and bias field correction. Then, the b0 and b1000 images were used to fit an ellipsoid model using the DTIFIT command in FSL. All the generated results including the fractional anisotropy (FA) map and the mean diffusivity (MD) map were normalized to the MNI space using linear registration. Regions of interest (ROI) containing 5 voxels (40mm3) were placed at the projection and association fiber regions (Zhang Y. et al., 2021) based on the anisotropy color maps and then the diffusivities at the x, y, and z orientations were extracted. The DTI-ALPS index was calculated using the following formula: DTI-ALPS index, mean (Dxproj, Dxassoc) / mean (Dyproj, Dzassoc). The DTI-ALPS indices in the bilateral hemispheres were calculated, respectively, and the mean of them was used in further analyses. To reduce site effects, we conducted multicenter data harmonization on DTI-ALPS using the COMBAT method (Fortin et al., 2017).
Statistical analysis
All statistical analyses were performed using SPSS Statistics Version 27.0 (IBM) and R (Version 4.2.1). Mean ± SD and median (interquartile range) were reported for normally and non-normally distributed variables, respectively. Frequencies (%) were presented for categorical variables. A p-value <0.05 was considered statistically significant.
We first analyzed whether demographic, clinical, imaging, and disease markers differed among the three groups. Continuous variables were analyzed using ANOVA, categorical variables using Chi-square tests, and ordinal variables using Kruskal-Wallis tests. Post-hoc analyses were conducted to identify sources of differences.
Second, we performed Spearman’s correlation analyses to examine the associations between CSF circulation markers and brain parenchymal damage, AD pathological markers, and cognitive impairments in the pCAA group. Age and sex were included as covariates. Considering the possible influence of vascular risk factors on CSF circulation and brain degeneration, we also performed additional correlation analyses while controlling the VRFs.
Results
Table 1 shows the demographics of the included subjects. Figure 2 briefly illustrates the imaging findings of normal controls and pCAA patients. There were no significant differences in age, sex, diabetes, hyperlipidemia, smoking, stroke, MI, AF, brain parenchymal-to-TIV ratio among the three groups (all p > 0.05).
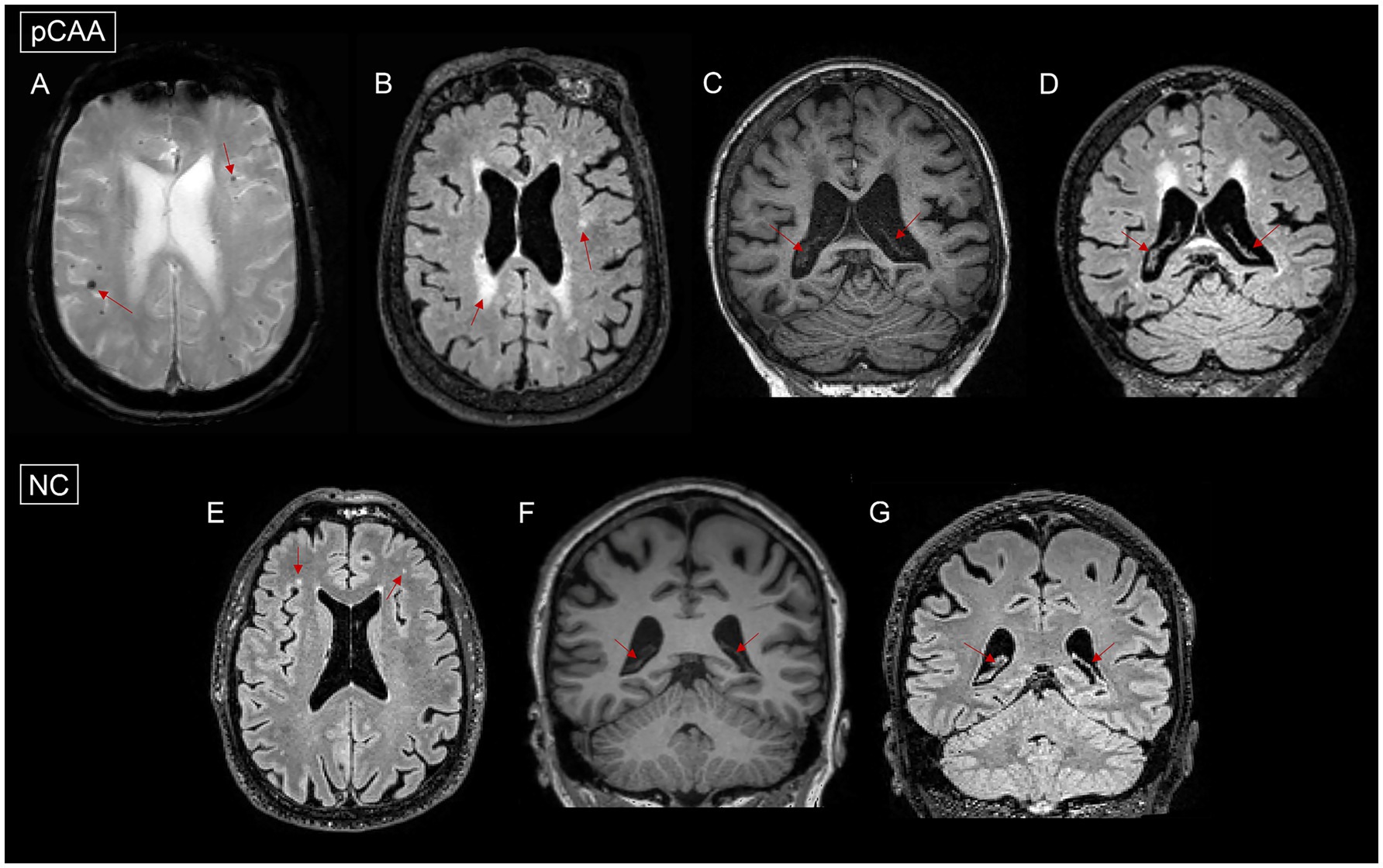
Figure 2. Images of microbleeds, white matter hyperintensities (WMH), and choroid plexus (ChP) in a normal control (NC) and a patient with probable cerebral amyloid angiopathy (pCAA). (A): pCAA demonstrates microbleeds on T2*-weighted sequences. (B): pCAA demonstrates white matter hyperintensities on T2 FLAIR sequences. (C): pCAA demonstrates ChP on T1-weighted sequences. (D): pCAA demonstrates ChP on T2 FLAIR sequences. (E): NC demonstrates white matter hyperintensities on T2 FLAIR sequences. (F): NC demonstrates ChP on T1-weighted sequences. (G): NC demonstrates ChP on T2 FLAIR sequences.
The NC, MCI-nonCAA, and pCAA groups exhibited significant differences in hypertension and cognitive assessments. For imaging markers, deep and periventricular WMH scores, WMH volume, and DTI-ALPS showed significant differences among the three groups. Post hoc analyses showed that patients with pCAA had significantly higher prevalence of hypertension, lower MMSE, lower MoCA, higher CDR-SOB, higher WMH burden, higher Aβ and tau deposition, and lower DTI-ALPS than NC. Compared to MCI-nonCAA, patients with pCAA showed significantly higher prevalence of hypertension, lower MoCA, higher WMH burden, and higher Aβ deposition. Although the pCAA group showed a trend of increased ChP volume, the difference was only marginally significant among the three groups.
In correlation analyses, we found that CSF circulation disorders were associated with downstream adverse events in the pCAA group. Specifically, we found a negative association (r = −0.478, p = 0.018) between DTI-ALPS and WMH volume fraction (Table 2). We did not observe that CSF circulation markers were associated with AD pathological markers. However, we found a negative association (r = −0.491, p = 0.024) between ChP volume fraction and MoCA (Table 2). After controlling for the VRFs, the findings remain consistent in additional correlation analyses (Table 3).
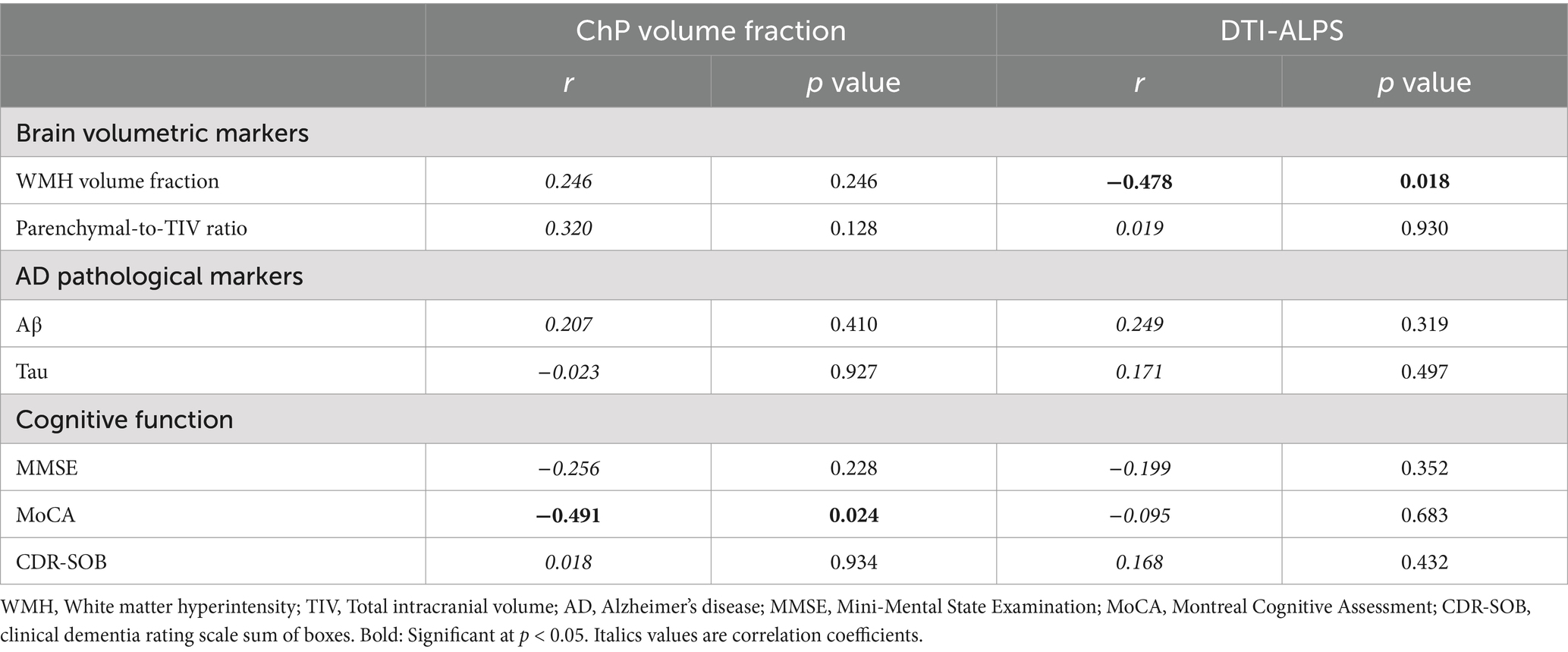
Table 2. Association between CSF circulation markers, brain degeneration and cognitive impairments in the pCAA group, controlling for age and sex.
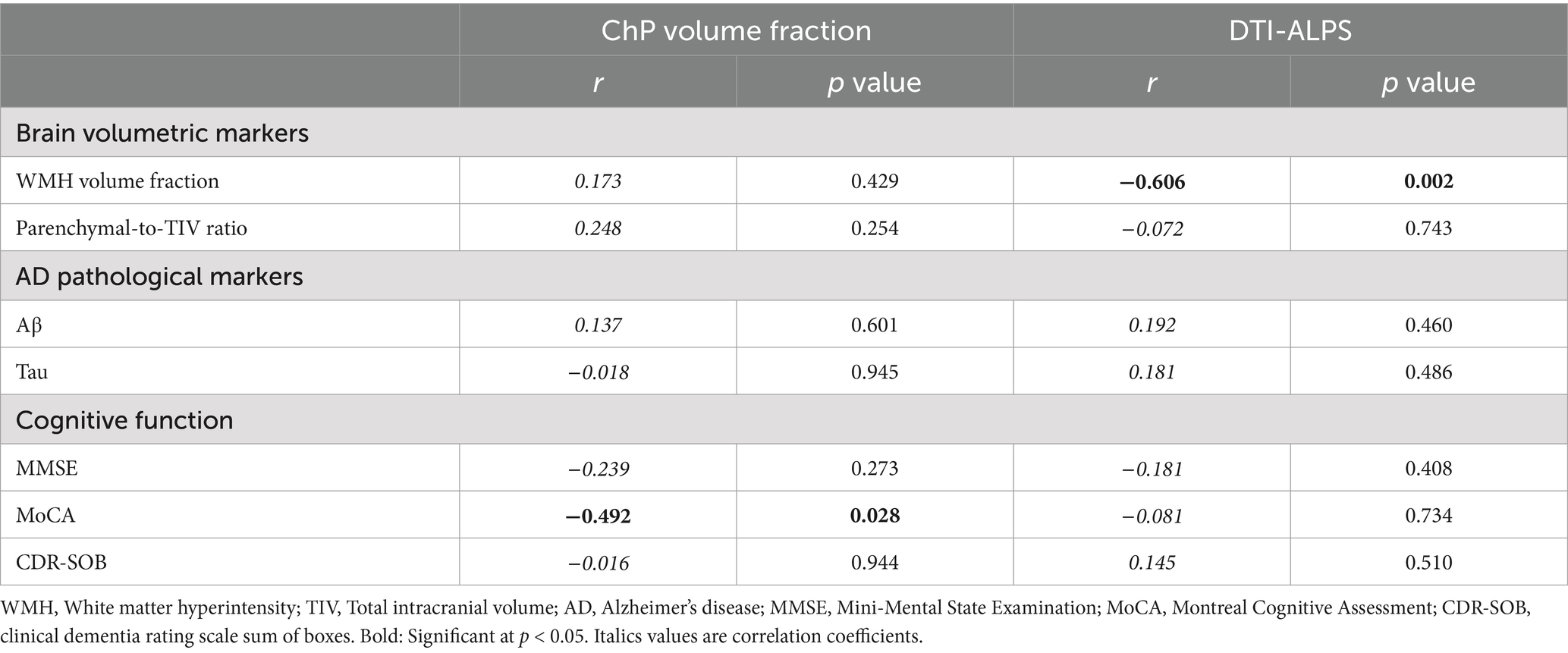
Table 3. Association between CSF circulation markers, brain degeneration and cognitive impairments in the pCAA group, controlling for age, sex, and VRFs.
Discussion
In this study, we examined two CSF circulation markers in NC, MCI-nonCAA, and pCAA groups. Our findings revealed that patients with pCAA had significantly lower DTI-ALPS values, higher WMH burden, more Aβ and tau deposition, and worse cognitive performance compared to NC. Within the pCAA group, a lower DTI-ALPS value was associated with a higher WMH burden, while a larger ChP volume correlated with a lower MoCA score. These results provide evidence of CSF circulation abnormalities in CAA, which may contribute to a better understanding of the disease’s etiology.
We screened over a thousand subjects in the ADNI3 database using the Boston 2.0 criteria for probable CAA and identified 26 cases. Unlike the high prevalence of CAA reported in pathological studies, imaging studies typically report a much lower prevalence (Jäkel et al., 2022). Additionally, variations in MRI scanners and protocols may affect the visualization of lesion features used to define CAA. The ADNI3 project acquired T2* images but did not include susceptibility-weighted imaging (SWI), further lowering the estimated prevalence (Buch et al., 2017). To disentangle the influence of cognitive status and CAA, we established two control groups. We applied a deep learning-based ChP segmentation method that utilizes two imaging modalities for segmentation. This method has a high segmentation accuracy compared to traditional tools.
We found significantly decreased DTI-ALPS and a trend of increased ChP volume for the pCAA group. As discussed before, the decreased DTI-ALPS indicated decreased fluid mobility in the perivenous space, while the increased ChP volume suggested pathological hypertrophy. This was in line with previous pathological and animal studies suggesting that CAA pathologies had a negative influence on CSF production and circulation. Nonetheless, when comparing to the MCI-nonCAA group, all results were non-significant, although the trends still existed. This is probably due to the small sample size and effect size.
The pCAA group had significantly higher WMH volume fraction and Aβ deposition compared to the MCI-nonCAA and NC groups. Regarding cognitive functions, the pCAA group showed lower cognitive scores in MoCA, but not MMSE and CDR, compared to the MCI-nonCAA group. These findings were consistent with the pathological theories of CAA, confirming that the Boston 2.0 diagnostic criteria of pCAA could correctly identify these patients.
In correlation analyses, we discovered that a lower DTI-ALPS was associated with a higher WMH burden in the pCAA group. Our previous studies demonstrated that venous disruption could cause stagnation of ISF and an increase in WMH volume (Zhang R. et al., 2021). The lower DTI-ALPS index, reflecting impaired perivenous fluid movement, may have similar effects. ISF stagnation can lead to the accumulation of metabolic waste products and pathological proteins, triggering inflammatory responses that further contribute to cellular damage, demyelination, and lesion formation (Abdul Hamid et al., 2024). Notably, increased ISF content itself may promote the development of WMH, as the FLAIR sequence is highly sensitive to changes in tissue water content. Additionally, we found that the ChP volume fraction was associated with MoCA score. It is possibly due to that ChP alterations disrupt brain homeostasis and accelerated brain degeneration (Godrich et al., 2022; Choi et al., 2023; Gokcal et al., 2021; Jo et al., 2021).
This research has several limitations. First, this is a cross-sectional study. The causal relationships between CSF circulation markers, parenchymal damage markers and cognitive decline over time still need to be explored in longitudinal studies. Second, we included pCAA without pathological confirmation. Some non-CAA participants might have been erroneously included. Furthermore, CAA can present with diverse clinical manifestations, including hemorrhagic stroke, transient focal neurological episodes, and cognitive impairment. Since our study exclusively enrolled patients with cognitive impairment, the generalizability of our findings to other CAA populations may be limited (Perini et al., 2023). Third, although we adjusted for age, sex, and vascular risk factors (VRFs), unmeasured confounding factors may still have influenced the findings. Fourth, T2*-GRE imaging was used to quantify microbleeds instead of the more sensitive SWI sequence, potentially contributing to a lower estimated prevalence. Fifth, the small sample size may have limited our ability to detect associations with smaller effect sizes.
Conclusion
In conclusion, our study suggested that CSF circulation markers were associated with elevated WMH burden and cognitive impairments in probable CAA. Further validations in cohorts with large sample sizes are needed.
Data availability statement
The datasets presented in this study can be found in online repositories. The names of the repository/repositories and accession number(s) can be found at: https://adni.loni.usc.edu/.
Ethics statement
The studies involving humans were approved by all procedures performed in studies involving human participants were under the ethical standards of the Institutional and National Research Committee and with the 1964 Helsinki declaration and its later amendments or comparable ethical standards. Written informed consent was from all participants and authorized representatives, and the study partners before any protocol specific procedures were carried out in the ADNI study. More details can be found at http://www.adni-info.org. The studies were conducted in accordance with the local legislation and institutional requirements. The participants provided their written informed consent to participate in this study.
Author contributions
LZ: Conceptualization, Data curation, Methodology, Writing – original draft, Writing – review & editing. LL: Conceptualization, Data curation, Methodology, Writing – original draft, Writing – review & editing. ML: Conceptualization, Data curation, Methodology, Writing – review & editing. LX: Writing – review & editing, Methodology. HH: Methodology, Writing – review & editing. QZ: Data curation, Writing – review & editing. SW: Data curation, Writing – review & editing. RZ: Writing – review & editing. ZZ: Conceptualization, Methodology, Writing – review & editing. PH: Conceptualization, Methodology, Writing – review & editing.
ADNI consortia information
Data used in the preparation of this article were obtained from the Alzheimer’s disease Neuroimaging Initiative (ADNI) database (http://www.adni.loni.usc.edu). As such, the investigators within the ADNI contributed to the design and implementation of ADNI and provided data but did not participate in analysis or writing of this report. A complete listing of ADNI investigators can be found at https://adni.loni.usc.edu/wp-content/uploads/ADNI%20Acknowledgement%20List_Feb2025_clean.pdf. Full list of consortium members and affiliations for ADNI The full list of members and affiliations could be found in https://adni.loni.usc.edu/wp-content/themes/freshnews-dev-v2/documents/policy/ADNI%20Groups%20Acknowledgements%20Journal%20Format.pdf.
Funding
The author(s) declare that financial support was received for the research and/or publication of this article. This work was supported by the National Natural Science Foundation of China (Grant Nos: 82371907; 82101987; 82402209) and the Natural Science Foundation of Zhejiang Province (Grant Nos: LZ24H180002; LQ20H180015; LQ24H180002).
Conflict of interest
The authors declare that the research was conducted in the absence of any commercial or financial relationships that could be construed as a potential conflict of interest.
Generative AI statement
The authors declare that no Gen AI was used in the creation of this manuscript.
Publisher’s note
All claims expressed in this article are solely those of the authors and do not necessarily represent those of their affiliated organizations, or those of the publisher, the editors and the reviewers. Any product that may be evaluated in this article, or claim that may be made by its manufacturer, is not guaranteed or endorsed by the publisher.
Abbreviations
DTI-ALPS, diffusion tensor imaging analysis of perivascular space; AD, Alzheimer’s disease; MCI, Mild cognitive impairment; MMSE, Mini-Mental State Examination; MoCA, Montreal Cognitive Assessment; NC, Normal control; Aβ, β-amyloid; CAA, cerebral amyloid angiopathy; FLAIR, fluid-attenuated inversion recovery; SVD, small vessel disease; VFT, Verbal Fluency Test; WM, white matter; PSMD, peak width of skeletonized mean diffusivity; SWI, susceptibility weighted imaging.
Footnotes
1. ^http://www.loni.ucla.edu/ADNI
3. ^http://adni.loni.usc.edu/wp-content/uploads/2010/05/ADNI2_PET_Tech_Manual_0142011.pdf
4. ^https://surfer.nmr.mgh.harvard.edu/
5. ^http://www.fil.ion.ucl.ac.uk/spm
References
Abdul Hamid, H., Hambali, A., Okon, U., Che Mohd Nassir, C. M. N., Mehat, M. Z., Norazit, A., et al. (2024). Is cerebral small vessel disease a central nervous system interstitial fluidopathy? IBRO Neurosci. Rep. 16, 98–105. doi: 10.1016/j.ibneur.2023.12.004
Beaman, C., Kozii, K., Hilal, S., Liu, M., Spagnolo-Allende, A. J., Polanco-Serra, G., et al. (2022). Cerebral microbleeds, cerebral amyloid angiopathy, and their relationships to quantitative markers of neurodegeneration. Neurology 98, e1605–e1616. doi: 10.1212/WNL.0000000000200142
Beaudin, A. E., McCreary, C. R., Mazerolle, E. L., Gee, M., Sharma, B., Subotic, A., et al. (2022). Cerebrovascular reactivity across the entire brain in cerebral amyloid angiopathy. Neurology 98, e1716–e1728. doi: 10.1212/WNL.0000000000200136
Buch, S., Cheng, Y.-C. N., Hu, J., Liu, S., Beaver, J., Rajagovindan, R., et al. (2017). Determination of detection sensitivity for cerebral microbleeds using susceptibility-weighted imaging. NMR Biomed. 30:e3551. doi: 10.1002/nbm.3551
Čarna, M., Onyango, I. G., Katina, S., Holub, D., Novotny, J. S., Nezvedova, M., et al. (2023). Pathogenesis of Alzheimer’s disease: involvement of the choroid plexus. Alzheimers Dement. 19, 3537–3554. doi: 10.1002/alz.12970
Charidimou, A., Boulouis, G., Frosch, M. P., Baron, J.-C., Pasi, M., Albucher, J. F., et al. (2022). The Boston criteria version 2.0 for cerebral amyloid angiopathy: a multicentre, retrospective, MRI-neuropathology diagnostic accuracy study. Lancet Neurol. 21, 714–725. doi: 10.1016/S1474-4422(22)00208-3
Charidimou, A., Boulouis, G., Greenberg, S. M., and Viswanathan, A. (2019). Cortical superficial siderosis and bleeding risk in cerebral amyloid angiopathy: a meta-analysis. Neurology 93, e2192–e2202. doi: 10.1212/WNL.0000000000008590
Chen, C.-H., Khnaijer, M. K., Beaudin, A. E., McCreary, C. R., Gee, M., Saad, F., et al. (2023). Subcortical volumes in cerebral amyloid angiopathy compared with Alzheimer’s disease and controls. Front. Neurosci. 17:1139196. doi: 10.3389/fnins.2023.1139196
Chen, X., Liu, X., Koundal, S., Elkin, R., Zhu, X., Monte, B., et al. (2022). Cerebral amyloid angiopathy is associated with glymphatic transport reduction and time-delayed solute drainage along the neck arteries. Nat. Aging 2, 214–223. doi: 10.1038/s43587-022-00181-4
Choi, S.-H., Letica, L., Brinson, L. L., Park, J. E., and Silva, A. C. (2023). Accelerated cerebral amyloid angiopathy and vascular alterations in a mixed mouse model of Alzheimer’s disease and cerebral small vessel disease. Alzheimers Dement. 19:e079185. doi: 10.1002/alz.079185
Deng, W., Guo, S., van Veluw, S. J., Yu, Z., Chan, S. J., Takase, H., et al. (2022). Effects of cerebral amyloid angiopathy on the brain vasculome. Aging Cell 21:e13503. doi: 10.1111/acel.13503
Durrani, R., Wang, M., Cox, E., Irving, E., Saad, F., McCreary, C. R., et al. (2023). Mediators of cognitive impairment in cerebral amyloid angiopathy. Int. J. Stroke 18, 78–84. doi: 10.1177/17474930221099352
Fazekas, F., Kleinert, R., Offenbacher, H., Schmidt, R., Kleinert, G., Payer, F., et al. (1993). Pathologic correlates of incidental MRI white matter signal hyperintensities. Neurology 43, 1683–1689. doi: 10.1212/wnl.43.9.1683
Fortin, J.-P., Parker, D., Tunç, B., Watanabe, T., Elliott, M. A., Ruparel, K., et al. (2017). Harmonization of multi-site diffusion tensor imaging data. Neuroimage 161, 149–170. doi: 10.1016/j.neuroimage.2017.08.047
Fotiadis, P., Pasi, M., Charidimou, A., Warren, A. D., and Schwab, K. M.Alzheimer’s Disease Neuroimaging Initiative, et al. (2021). Decreased basal ganglia volume in cerebral amyloid angiopathy. J. Stroke 23, 223–233. doi: 10.5853/jos.2020.04280
Fotiadis, P., van Rooden, S., van der Grond, J., Schultz, A., Martinez-Ramirez, S., Auriel, E., et al. (2016). Cortical atrophy in patients with cerebral amyloid angiopathy: a case-control study. Lancet Neurol. 15, 811–819. doi: 10.1016/S1474-4422(16)30030-8
Godrich, D., Pasteris, J., Martin, E. R., Schellenberg, G. D., Pericak-Vance, M. A., Cuccaro, M. L., et al. (2022). Alzheimer Disease candidate variants are associated with cerebral amyloid angiopathy. Alzheimers Dement. 18:e067799. doi: 10.1002/alz.067799
Gokcal, E., Becker, A. A., Horn, M. J., Das, A. S., Schwab, K., Biffi, A., et al. (2021). Abstract P442: the association of amyloid and tau burden with centrum semiovale perivascular spaces in cerebral amyloid angiopathy. Stroke 52:AP442. doi: 10.1161/str.52.suppl_1.P442
Greenberg, S. M., Bacskai, B. J., Hernandez-Guillamon, M., Pruzin, J., Sperling, R., and Van Veluw, S. J. (2020). Cerebral amyloid angiopathy and Alzheimer disease — one peptide, two pathways. Nat. Rev. Neurol. 16, 30–42. doi: 10.1038/s41582-019-0281-2
Greenberg, S. M., and van Veluw, S. J. (2024). Cerebral amyloid Angiopathy. Stroke 55, 1409–1411. doi: 10.1161/STROKEAHA.124.044293
Hong, H., Hong, L., Luo, X., Zeng, Q., Li, K., Wang, S., et al. (2024). The relationship between amyloid pathology, cerebral small vessel disease, glymphatic dysfunction, and cognition: a study based on Alzheimer’s disease continuum participants. Alz. Res. Therapy 16:43. doi: 10.1186/s13195-024-01407-w
Huang, S., Zhang, Y., Guo, Y., Du, J., Ren, P., Wu, B., et al. (2024). Glymphatic system dysfunction predicts amyloid deposition, neurodegeneration, and clinical progression in Alzheimer’s disease. Alzheimer’s Dementia 20, 3251–3269. doi: 10.1002/alz.13789
Jäkel, L., De Kort, A. M., Klijn, C. J. M., Schreuder, F. H. B. M., and Verbeek, M. M. (2022). Prevalence of cerebral amyloid angiopathy: a systematic review and meta-analysis. Alzheimers Dement. 18, 10–28. doi: 10.1002/alz.12366
Jeong, S. H., Park, C. J., Jeong, H.-J., Sunwoo, M. K., Ahn, S. S., Lee, S.-K., et al. (2023). Association of choroid plexus volume with motor symptoms and dopaminergic degeneration in Parkinson’s disease. J. Neurol. Neurosurg. Psychiatry 94, 1047–1055. doi: 10.1136/jnnp-2023-331170
Jessen, N. A., Munk, A. S. F., Lundgaard, I., and Nedergaard, M. (2015). The glymphatic system: a beginner’s guide. Neurochem. Res. 40, 2583–2599. doi: 10.1007/s11064-015-1581-6
Jiang, D., Liu, L., Kong, Y., Chen, Z., Rosa-Neto, P., Chen, K., et al. (2023). Regional glymphatic abnormality in behavioral variant frontotemporal dementia. Ann. Neurol. 94, 442–456. doi: 10.1002/ana.26710
Jo, S., Kim, H.-J., Lee, S. J., and Lee, J.-H. (2021). Role of white matter alteration in the relationship between regional microbleeds and cognitive impairment in cerebral amyloid angiopathy. Alzheimers Dement. 17:e052717. doi: 10.1002/alz.052717
Kim, S. H., Ahn, J. H., Yang, H., Lee, P., Koh, G. Y., and Jeong, Y. (2020). Cerebral amyloid angiopathy aggravates perivascular clearance impairment in an Alzheimer’s disease mouse model. Acta Neuropathol. Commun. 8:181. doi: 10.1186/s40478-020-01042-0
Koemans, E. A., Chhatwal, J. P., van Veluw, S. J., van Etten, E. S., van Osch, M. J. P., van Walderveen, M. A. A., et al. (2023). Progression of cerebral amyloid angiopathy: a pathophysiological framework. Lancet Neurol. 22, 632–642. doi: 10.1016/S1474-4422(23)00114-X
Nedergaard, M., and Goldman, S. A. (2020). Glymphatic failure as a final common pathway to dementia. Science 370, 50–56. doi: 10.1126/science.abb8739
Perini, G., Ramusino, M. C., Farina, L. M., Fabbro, B. D., Canavero, I., Picascia, M., et al. (2023). Cognitive versus hemorrhagic onset in cerebral amyloid angiopathy: neuroimaging features. Curr. Alzheimer Res. 20, 267–276. doi: 10.2174/1567205020666230713151211
Rabin, J. S., Nichols, E., La Joie, R., Casaletto, K. B., Palta, P., Dams-O’Connor, K., et al. (2022). Cerebral amyloid angiopathy interacts with parenchymal beta-amyloid to promote tau and cognitive decline. Alzheimers Dement. 18:e066555. doi: 10.1002/alz.066555
Royse, S. K., Minhas, D. S., Lopresti, B. J., Murphy, A., Ward, T., Koeppe, R. A., et al. (2021). Validation of amyloid PET positivity thresholds in centiloids: a multisite PET study approach. Alzheimers Res. Ther. 13:99. doi: 10.1186/s13195-021-00836-1
Sasaki, A., Iijima, M., Yokoo, H., Shoji, M., and Nakazato, Y. (1997). Human choroid plexus is an uniquely involved area of the brain in amyloidosis: a histochemical, immunohistochemical and ultrastructural study. Brain Res. 755, 193–201. doi: 10.1016/s0006-8993(97)00097-8
Taoka, T., Masutani, Y., Kawai, H., Nakane, T., Matsuoka, K., Yasuno, F., et al. (2017). Evaluation of glymphatic system activity with the diffusion MR technique: diffusion tensor image analysis along the perivascular space (DTI-ALPS) in Alzheimer’s disease cases. Jpn. J. Radiol. 35, 172–178. doi: 10.1007/s11604-017-0617-z
van Veluw, S. J., Benveniste, H., Bakker, E. N. T. P., Carare, R. O., Greenberg, S. M., Iliff, J. J., et al. (2024a). Is CAA a perivascular brain clearance disease? A discussion of the evidence to date and outlook for future studies. Cell. Mol. Life Sci. 81:239. doi: 10.1007/s00018-024-05277-1
van Veluw, S. J., Benveniste, H., and van Osch, M. J. P.Leducq Foundation Transatlantic Network of Excellence on Brain Clearance (2024b). A translational approach towards understanding brain waste clearance in cerebral amyloid angiopathy. Eur. Heart J. 45, 1500–1502. doi: 10.1093/eurheartj/ehae011
Vargas, T., Ugalde, C., Spuch, C., Antequera, D., Morán, M. J., Martín, M. A., et al. (2010). Abeta accumulation in choroid plexus is associated with mitochondrial-induced apoptosis. Neurobiol. Aging 31, 1569–1581. doi: 10.1016/j.neurobiolaging.2008.08.017
Wang, L., Liu, Q., Yue, D., Liu, J., and Fu, Y. (2024). Cerebral amyloid angiopathy: an undeniable small vessel disease. J Stroke 26, 1–12. doi: 10.5853/jos.2023.01942
Wichmann, T. O., Damkier, H. H., and Pedersen, M. (2021). A brief overview of the cerebrospinal fluid system and its implications for brain and spinal cord diseases. Front. Hum. Neurosci. 15:737217. doi: 10.3389/fnhum.2021.737217
Xie, L., Zhang, Y., Hong, H., Xu, S., Cui, L., Wang, S., et al. (2024). Higher intracranial arterial pulsatility is associated with presumed imaging markers of the glymphatic system: an explorative study. NeuroImage 288:120524. doi: 10.1016/j.neuroimage.2024.120524
Zhang, R., Huang, P., Jiaerken, Y., Wang, S., Hong, H., Luo, X., et al. (2021). Venous disruption affects white matter integrity through increased interstitial fluid in cerebral small vessel disease. J. Cereb. Blood Flow Metab. 41, 157–165. doi: 10.1177/0271678X20904840
Zhang, Y., Zhang, R., Ye, Y., Wang, S., Jiaerken, Y., Hong, H., et al. (2021). The influence of demographics and vascular risk factors on Glymphatic function measured by diffusion along perivascular space. Front. Aging Neurosci. 13:693787. doi: 10.3389/fnagi.2021.693787
Keywords: cerebral amyloid angiopathy, diffusion tensor imaging, perivascular space, Alzheimer’s disease, white matter hyperintensities, cognition
Citation: Zhao L, Liu L, Lin M, Xie L, Hong H, Zeng Q, Wang S, Zhang R, Zhao Z and Huang P (2025) Relationship between cerebrospinal fluid circulation markers, brain degeneration, and cognitive impairment in cerebral amyloid angiopathy. Front. Aging Neurosci. 17:1549072. doi: 10.3389/fnagi.2025.1549072
Edited by:
Stephen D. Ginsberg, Nathan S. Kline Institute for Psychiatric Research, United StatesReviewed by:
Tracy Fischer, Tulane University, United StatesMatteo Cotta Ramusino, Neurological Institute Foundation Casimiro Mondino (IRCCS), Italy
Copyright © 2025 Zhao, Liu, Lin, Xie, Hong, Zeng, Wang, Zhang, Zhao and Huang. This is an open-access article distributed under the terms of the Creative Commons Attribution License (CC BY). The use, distribution or reproduction in other forums is permitted, provided the original author(s) and the copyright owner(s) are credited and that the original publication in this journal is cited, in accordance with accepted academic practice. No use, distribution or reproduction is permitted which does not comply with these terms.
*Correspondence: Peiyu Huang, aHVhbmdweUB6anUuZWR1LmNu; Zhenhua Zhao, emhhbzIwNzVAMTYzLmNvbQ==
†These authors have contributed equally to this work