- 1Department of Agronomy, Iowa State University, Ames, IA, United States
- 2Department of Economics, Iowa State University, Ames, IA, United States
- 3Food Systems Research Center, University of Vermont, Burlington, VT, United States
- 4Iowa Soybean Association, Ankeny, IA, United States
- 5Department of Mechanical Engineering, Iowa State University, Ames, IA, United States
- 6Department of Computer Science, Missouri University of Science and Technology, Rolla, MI, United States
- 7Division of Applied Social Sciences – Agricultural and Applied Economics, University of Missouri, Columbia, MI, United States
- 8Department of Computer Science, University of Kentucky, Lexington, KY, United States
- 9Department of Applied Economics and Statistics, University of Delaware, Newark, DE, United States
To meet the grand challenges of agricultural production including climate change impacts on crop production, a tight integration of social science, technology and agriculture experts including farmers are needed. Rapid advances in information and communication technology, precision agriculture and data analytics, are creating a perfect opportunity for the creation of smart connected farms (SCFs) and networked farmers. A network and coordinated farmer network provides unique advantages to farmers to enhance farm production and profitability, while tackling adverse climate events. The aim of this article is to provide a comprehensive overview of the state of the art in SCF including the advances in engineering, computer sciences, data sciences, social sciences and economics including data privacy, sharing and technology adoption. More specifically, we provide a comprehensive review of key components of SCFs and crucial elements necessary for its success. It includes, high-speed connections, sensors for data collection, and edge, fog and cloud computing along with innovative wireless technologies to enable cyber agricultural system. We also cover the topic of adoption of these technologies that involves important considerations around data analysis, privacy, and the sharing of data on platforms. From a social science and economics perspective, we examine the net-benefits and potential barriers to data-sharing within agricultural communities, and the behavioral factors influencing the adoption of SCF technologies. The focus of this review is to cover the state-of-the-art in smart connected farms with sufficient technological infrastructure; however, the information included herein can be utilized in geographies and farming systems that are witnessing digital technologies and want to develop SCF. Overall, taking a holistic view that spans technical, social and economic dimensions is key to understanding the impacts and future trajectory of Smart and Connected Farms.
1 Introduction
Agriculture is one of the most important industries that directly or indirectly supports a large section of society. With continual challenges in crop production due to climatic variability, there is a need to consider integrating technical, economic, and social dimensions of research to meet the needs of agriculture. Among the most pressing constraints and challenges of modern agriculture, climate change-related crop yield decrease is among the most important (Kummu et al., 2021). For example, Climate change is projected to reduce agricultural productivity by 3 to 16% by 2080, with developing countries experiencing more significant reductions ranging from 10 to 25%; furthermore, crop diseases and other plant stresses may become more prevalent as temperatures increase and rainfall patterns become more variable (Mahato, 2014). This is ushering in a new paradigm of climate-smart agriculture that requires advances in information and communication technology (ICT) in crop production and agricultural research. This review establishes the concept of smart and connected farms and builds on various technologies and concepts, e.g., the internet of things (IoT), cyber-physical systems (CPS), smart and connected communities, and socio-economic factors, all in the context of empowering farmers.
2 Information and communication technology in crop production
Some of the technologies that make ICT include IoT (Holler et al., 2014; Perkel, 2017), robotics, big data, artificial intelligence, and cyber-agricultural systems. With the additional support of cloud services, IoT enables the analysis of large historical data, including soil properties, fertilizer distribution, insect arrival rate, temperature and humidity trend, and so on. While facilitating data collection at every stage of crop production, IoT also paves a path to data-driven services for intelligent farming. IoT mainly refers to the interconnection of sensor-embedded devices/equipment and is envisioned to improve the quality and experience of human living, including in smart agriculture. Potential IoT and wireless sensor networks (WSNs) applications have been reported (Satyanarayana and Mazaruddin, 2013; Sakthipriya, 2014; Patil and Kale, 2016; Xue and Huang, 2020; Bhat and Huang, 2021; Chen et al., 2021; Roy et al., 2021).
Farmers operate at varying farm size scales, which necessitates context-specific management. To compare scales, traditional farming manages farm operations at the field level, while smart farming allows decision-making at a much smaller scale, i.e., per square meter or plants per unit area. This shift is possible due to Cyber-Agricultural Systems (CAS) that include individualized sensing, modeling, and actuation using machine learning (ML) and coordinated teams of drones/robots that are enabled by autonomy (Gao et al., 2018; Sarkar et al., 2023). Automation and control systems provide a higher level of sophistication and precision that can improve the profitability and sustainability of modern production systems (Lowenberg-DeBoer et al., 2020). These technologies support software to help reduce inputs by more accurately targeting within-field areas with variable rate application of fertilizer and pesticides (Babcock and Pautsch, 1998). Consequently, by targeting these field inputs more accurately, farmers can reduce their chemical footprint on the field. The reduction in inputs saves costs by creating a profit potential for farmers. For example, the use of precision agriculture technologies allows farmers to operate in a more sustainable manner and increase their profitability (Bongiovanni and Lowenberg-DeBoer, 2004). While these examples are focused on the field level, similar approaches and technologies apply to livestock management (Astill et al., 2020) and controlled environment farms (Guo and Zhong, 2015).
Agricultural equipment manufacturers and service providers are developing technologies that implement precision farming using GPS location, application rate monitoring, satellite image analysis, and predictive models for weather and crop health. While individual farmers with robust economic condition and ability to move to new technologies are increasingly adopting advanced sensing and intelligent equipment (e.g., smart tractors, seed, and chemical sprayers, aerial surveillance), there is still a lack of efficient communications and computing technology that are effectively connecting farmers within a community, thereby preventing them from sharing data, analyses, and practices optimally. Overcoming these challenges will enable better decision-making at farms and increased crop production while collectively improving the overall well-being and quality of farmers’ life. Although farmers and their technology providers collect farm-level spatial data on planting and harvest, community-wide data collection and analysis will be helpful to formulate management responses to production threats that transcend farm boundaries, for example, weed seed dispersal, pesticide dispersion in air, insect-pest migration from farm to farm, disease spore movement through air and water, and soybean cyst nematode (Boyd and White, 2009; Zivan et al., 2017). With rapid networking, farm-level information and production threats can be communicated quickly across a wider agricultural community. Farmer communities can often formulate more effective responses to changing climate events with information sharing and decision-making, whereas isolated farmers or those who do not trust their sources of information often delay taking action against production threats (Obunyali et al., 2019). This necessitates a technology-driven, community-enabled tool for row crop farming that provides community-wide mitigation efforts against such threats.
3 Farmer networks, and smart and connected farms
To improve community-wide data sharing between farmers, novel socio-technical platforms are needed to create an SCF network, which also provides the benefit of an early warning system for damaging pests and other crop stressors. In Africa and parts of western Asia, a large network of farmers uses various sensors and networking tools to help predict and map the spread of locusts that cause major damage to affected countries (Cressman, 2013). This network of technology tools and farmer observations aims to predict when plague-level locust populations may arise and to take preemptive action to stop the locusts before they can cause damage that could cause problems with food insecurity (Cressman, 2013). Networked farms, data aggregation, and sharing data across farms and fields are suitable for limiting the effect of harmful pests in networked farms across the world.
Although community-based SCF are not yet common, farmers have conglomerated together for on-farm testing networks where experiments are done on the lands of participating farmers for the mutual benefit of all farmers. The data from these tests are more relevant and with a higher confidence level than can be tested by farmers individually. These community-based data collection and downstream decision-making have been done through on-farm networks but without IoT, smart sensors, ML, and other communication technologies. Collecting field experiment data from a network of interconnected farms can be more beneficial than collecting high-precision sensor data from a single farm. On-farm experiments allow for establishing cause-and-effect relationships regarding important crop traits and developing a generalized decision-making framework that can be applied to wider geographical areas and varying growing conditions in subsequent years. However, it is important to ensure that the technological tools and solutions generated are practical for decision-making rather than simply forming a dataset. This is particularly important for drone-based imagery, which might be of little value unless it enables farmers to act on the insights derived from image-based phenotyping.
Efforts have begun to create SCF, for example, Smart Integrated Farm Network for Rural Agricultural Communities (SIRAC), that aims to facilitate more effective data sharing, knowledge exchange, and coordinated responses to production threats. Figure 1 provides an overview of the SIRAC vision of connecting farms and gathering and sharing information across regions and sensing capabilities.
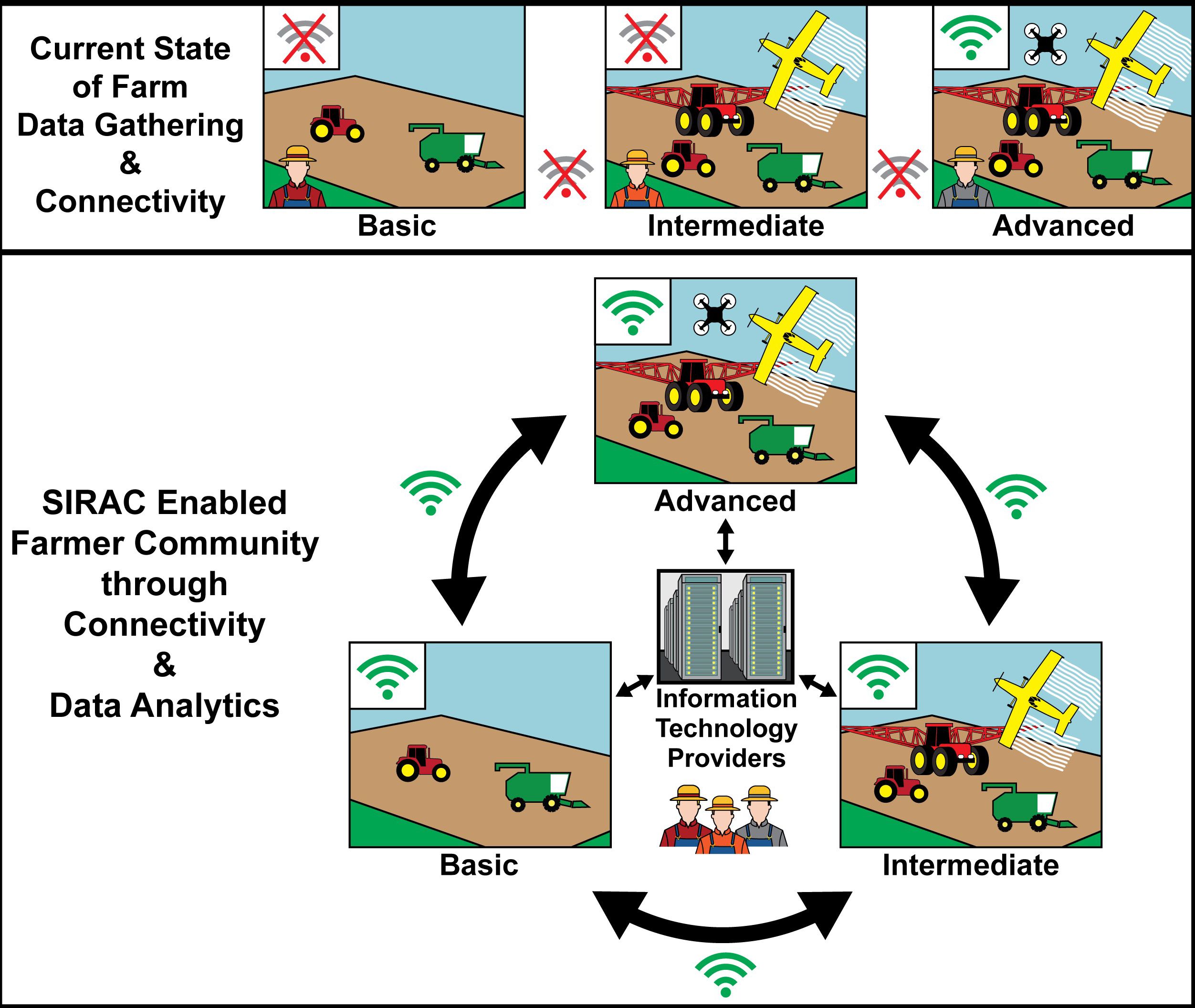
Figure 1 Example of a smart and connected farm network: Smart Integrated Farm Network for Rural Agricultural Communities (SIRAC) that is designed to tackle complex challenges to agricultural production including climate change. Such farms have advanced sensors and platform connectivity and data analytics utilizing Internet-of-Things and Cyber-Agricultural Systems.
Such SCFs will enable real-time monitoring of threats to farm production at a landscape level, which is critical for dealing with pests, diseases, weather, and management issues. SCFs will build on currently successful on-farm research trials that ensure the research and its outcomes are adapted to local farming environments and cultural practices (Kyveryga, 2019). Technologies designed to create a network of farms and share information among farmers offer potentially numerous solutions to productivity and sustainability in a changing world (Weiss et al., 2020). Furthermore, having a network of smart farms that can share data with one another and utilize remote sensing technologies provides the potential for discovering the extent of climate change’s effect on a farmer’s crop and the viability of growing that crop in areas that have historically supported those crops, potentially meaning a change in land use (Weiss et al., 2020). Another networked solution farms offer the balance they can provide between profitability, productivity, and sustainability with aggregated data to better use crop inputs.
3.1 High-speed connections for SCFs
While different regions in the world with IoT have varying circumstances and infrastructure, we will focus on the U.S. farms to illustrate the role of high-speed connections in the context of SCF. Only 26% of rural households have broadband connectivity vs. 93.5% overall Americans have high-speed internet (LoPiccalo, 2021). Available options for rural households are often limited or more expensive than available in urban areas when considering Dollars/Megabits per second, although newer satellite-based internet options are now available. Agricultural fields have lower coverage because fixed wireless is unavailable, and mobile wireless coverage is available only near highways or populated areas.
Several programs administered by the Federal Communications Commission (FCC) target rural areas to increase the availability of fixed and mobile broadband services for healthcare, schools, and farming. Four such programs to increase the availability of voice, fixed, and mobile broadband services in under-served and rural areas include: Connect America Fund (CAF) for rural areas; Lifeline for low-income consumers; E-rate for schools and libraries, and; the Rural Health Care Program. The FCC initiatives have identified high-speed connections with at least 25 Mbps downlink and 3 Mbps uplink. According to the FCC, connections with high-speed throughput are considered adequate to upload and download data used in precision farming and field inspection/monitoring tools using Unoccupied Aerial Vehicles (UAVs) or drones and remote sensors (Herr et al., 2023). The downlink-to-uplink ratio of 8 to 1 assumes a standard Internet browser information exchange model.
The programs for increasing connectivity with high-speed connections target family homes, offices, schools, and health centers but not agricultural fields, so other approaches are needed to extend coverage to these remote areas. As of December 2020, the FCC has awarded $9.2 billion to Internet Service Providers (ISPs) to deploy high-speed internet to unserved homes and businesses in rural areas, while $6+ billion is still to be awarded for reaching rates of 100Mbps downlink and 20 Mbps uplink (Shepardson, 2020). Extending the high-speed connection to all the fields owned or rented by the operation requires additional networking. Providing an internet access point connection to each field fragment provides a considerable networking challenge. Many farmers manage fields that are variable in size and are dispersed over large areas. Most farming operations include personally owned fields as well as leased fields. Providing ubiquitous coverage of each field is yet another challenge once an internet access point can be provided to each geographically disconnected field.
Solutions are available for connecting fields but require investment to install new internet services or expand existing ones. Figure 2 shows various methods that can be used to obtain an ISP connection. These include wired alternatives like a Digital Subscriber Line or Fiber to the Home. Wireless alternatives include mobile wireless provider service over LTE or 5G, Private fixed wireless provider using unlicensed RF bands, or Satellite service. The figure also illustrates connecting field sensors using WiFi technology to create a Local Area Network with internet access that allows sensor connectivity and supports Mobile phone and PC internet service. One common model for accessing precision farming information from agricultural equipment for fields with no internet requires the user to first offload the data from the equipment onto a tablet or PC, and then when they can reach a location with broadband connectivity, the PC connects to a cloud service and uploads the information and then processes and provides analysis and feedback. This model works very well for each equipment manufacturer but has drawbacks for the farmers. Different companies’ software does not always integrate well with each other, requiring farmers to use the same manufacturer for all operations or lose the benefits of the data collected with each system and complete farm data integration.
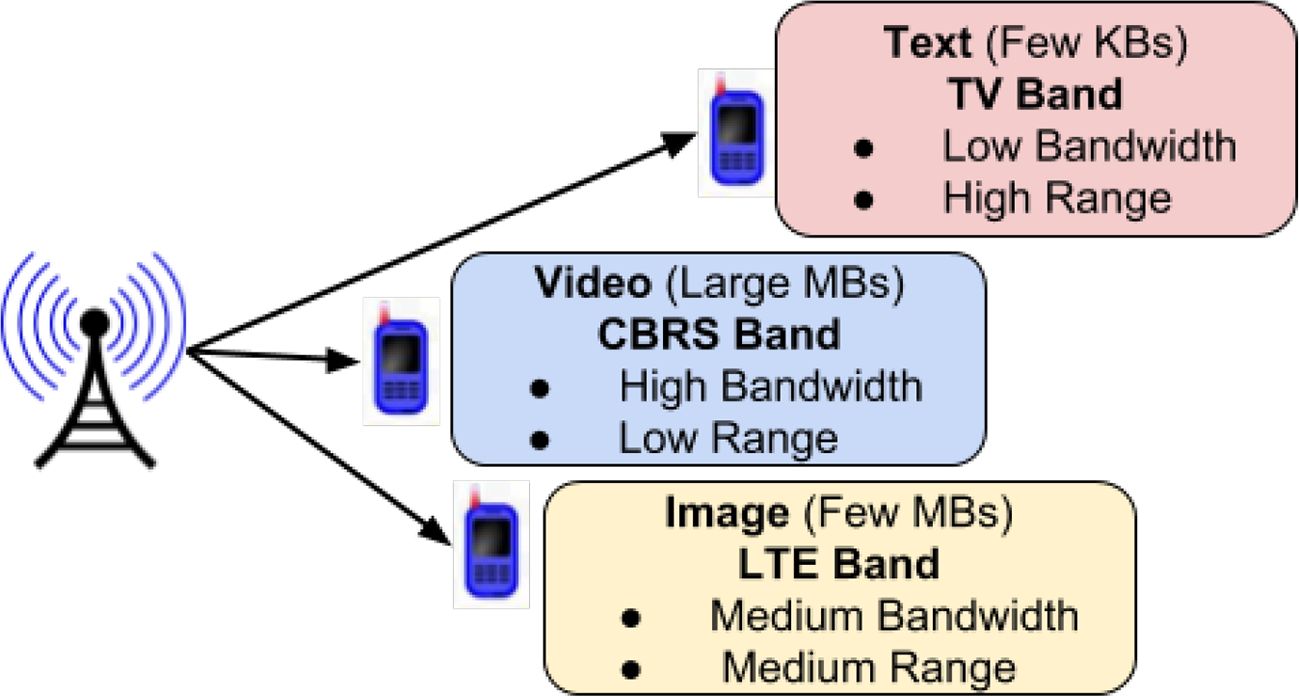
Figure 2 A motivating example of d-DSA paradigm: different bands are preferable depending on the traffic characteristics.
3.2 Sensors for data collection in SCFs
SCFs depend on timely and precise sensing (using sensors) of the crop and environment to make informed decisions, for example, when and where to irrigate or spray pesticides. Broadly, the sensors fall into two categories: passive and active. Passive sensors acquire data through light, radiation, heat, or vibrations generated by the physical objects (e.g., crops), while active sensors sense the objects by emitting a signal from their own radiation source and measuring the strength of the reflected or refracted signal (Barmeier and Schmidhalter, 2017).
These sensors can be deployed on a wide variety of scales, but are most commonly used on the ground, typically, this would be done by a handheld device at the plant level or field level using a UAV. Further, at the farm and county level, a satellite imaging system is commonly employed to monitor the crops. Depending on farmers’ interest in the crop production problem, the resolution, and type of sensor are decided, and appropriate phenotyping agents (robots, drones, satellites) are chosen to allow an increase in throughput as well as area covered (Guo et al., 2021). The most commonly used remote sensing modality is a red, green, blue (RGB) digital camera. This is the most easily interpretable data as it senses what the human eye can see. It is also the least expensive sensor when it is being mounted on a UAV. The ease of use both for data collection and downstream processing has made this type of sensor widely used in plant science, and its uses have ranged from biotic (Tetila et al., 2017; Rairdin et al., 2022) and abiotic (Naik et al., 2017; Zhang et al., 2017; Dobbels and Lorenz, 2019) stress detection, weed detection (Lottes et al., 2017), maturity estimation (Trevisan et al., 2020), and stand counts (Barreto et al., 2021).
The other commonly used sensor is a multispectral camera, which typically has anywhere from 3–10 bands and usually has bands in the RGB spectrum as well as the red-edge and near-infrared bands. These additional bands can provide additional information about crop health and development and have been used for many use cases listed above (Lottes et al., 2017) as well as yield prediction (Xu et al., 2021). The increased bands do typically increase the cost of these sensors, but they are still moderately priced and are easily integrated into a UAV. The additional bands can add complexity to downstream analysis and increase the data set size, but many farmers would be accustomed to planting health maps, which are easily generated from this data that can also be acquired via satellites.
Hyperspectral camera is more sophisticated and typically has 100 or more bands that range from the RGB spectrum to the near-infrared spectrum, but the difference is that there is a much higher spectral resolution with each band typically only being a few nanometers wide. These sensors have been used on UAVs but have had less use than multispectral cameras due to the high cost as well as the complexity of analyzing the data sets. Hyperspectral images have been used in conjunction with machine learning techniques to detect plant stress early in soybeans (Nagasubramanian et al., 2018), as well as for vegetation monitoring in barley (Aasen et al., 2015).
Finally, thermal imaging is another type of passive sensing that uses wavelengths in the far infrared spectrum, typically in the 7,500–14,000 nm wavelengths. Thermal imaging has been used on UAVs, and these sensors account for a moderate cost to purchase but are highly affected by environmental variables. Thermal cameras have been used for disease detection in soybean (Hatton et al., 2018), and have been shown to have correlations with biomass and seed yield in drybean (Sankaran et al., 2019), and also shown for irrigation scheduling in almonds (García-Tejero et al., 2018).
Light Detection and Ranging(LiDAR), an example of the active sensor, emits laser pulses that it then uses to detect its distance from an object. These pulses generate three-dimensional point clouds that have been used for terrain mapping from satellites that are utilized to map soil erosion (Gelder et al., 2018), and plant biomass estimation from UAVs (Shendryk et al., 2020). Processing LiDAR data requires different analysis pipelines than the other sensors because it is no longer working in a 2-dimensional space.
Different types of sensors will have different time sensitivity. Sensor types with very high time sensitivity, such as minutes or hours, may need a persistent connection to reach their full potential. Sensors with a low time sensitivity, measured in days, may do just fine with periodic connections. Some sensors, such as moisture sensors in the soil, may seem to have a fairly high time sensitivity. However, if the data can be processed locally, instead of in the cloud, this time sensitivity can be mitigated, with the decisions being automated and made in the field.
3.3 Edge, fog and cloud computing in SCFs
Despite the advantages of IoT, it is hard to communicate a large volume of sensory (time series or image) data from the agriculture field to the operators or back-end servers (on the cloud). Such communications not only consume considerable energy but also incur significant communication delays and generate substantial network traffic. A viable solution is edge computing, where the local processing and storage are available close to the end devices or users (Sirojan et al., 2018; Li, 2019; Esfandiari et al., 2021a). Due to the local processing of the tasks near the devices/users, edge computing reduces communication delay and energy consumption for transmitting the collected data. Recent advances in compression-decompression techniques using ML and deep learning (DL) models in Edge Computing can also help reduce the size of the data at the edge devices (Jiang et al., 2017; Zhang et al., 2017; Wang et al., 2020; Esfandiari et al., 2021b). The edge devices (e.g., sensors deployed in the farmland) have limited resources and cannot efficiently execute DL on them. Recently, for large-scale mobile edge computing, an efficient online computation offloading approach via deep reinforcement learning is proposed in (Hu et al., 2022).
To address the computing limitation of edge devices, an edge-fog architecture is introduced to process IoT data in an efficient way while avoiding the communication delay that occurs in cloud computing (Saha et al., 2020). Interested readers may refer (Bellavista et al., 2019) for a state-of-the-art survey on fog computing for IoT. Fog devices (e.g., laptops or low-end computing machines) are usually equipped with more resources than edge devices and are mostly located at a lesser communication distance than the cloud. It means that DL with light configuration (few layers and neurons) may be executed efficiently in fog. However, it is not a replacement for the cloud and thus cloud services are still preferable to perform heavy computational tasks in IoT-based farming, e.g., big data (collected from a large number of sensors deployed in the agricultural field) analysis and execution of resource-intensive DL models (e.g., ResNet (He et al., 2016)) for real-time crop monitoring. Therefore, harnessing the computing capabilities of edge, fog, and cloud through a combined architecture, as depicted in Figure 3, would be the mindful solution to SCFs and smart agriculture.
Case example 1: Let us consider a scenario with this architecture where several sensors are deployed across many acres of farmland to gather real-time data on soil moisture, crop infection severity, temperature, humidity, etc. Each sensor is attached to a controller (e.g., Arduino or Raspberry Pi) to process the data and it acts as an edge device that transmits this data to a nearby station (e.g., low-end machine) acting as a fog device collecting data from multiple edge devices over the wireless medium. Since the fog devices are capable enough to run light weighted ML and DL models, the farmers may be notified immediately to take necessary actions if any alerting situation is occurred or is predicted. However, for better prediction, historical and genetic information needs to be exploited by the models (de Azevedo Peixoto et al., 2017; Shook et al., 2021a, Shook et al., 2021b), which are usually stored in the cloud. The fog devices also offload the data to the cloud in order to receive highly accurate and precise results on insect population, yield prediction, the need for pesticide spray, and so on. The three layers (edge, fog, and cloud) architecture in smart agriculture reduces energy consumption, network traffic, and communication delay (Alharbi and Aldossary, 2021).
Case example 2: Another way to efficiently collect data from agriculture fields is via participatory sensing or mobile crowdsensing in which the farmers act as human sensors and supply useful information with the help of smartphones. See Luo et al. (2019); Roy et al. (2021) for an energy-aware fog-based framework for data forwarding in mobile crowdsensing; and how to improve IoT data quality in mobile crowdsensing, respectively. Researchers have proposed an energy-efficient data forwarding scheme in fog-based systems with deadline constraints (Saraswat et al., 2020). Deep neural networks and compression techniques to the edge devices for plant disease detection have been applied (De Vita et al., 2020, 2021). A drone-based approach to efficiently scouting bugs in orchards using multi-functional nets has been proposed (Betti Sorbelli et al., 2022a, Betti Sorbelli et al., 2022b).
3.4 Innovative wireless technologies for SCFs
A few recent studies aim to deliver limited Internet connectivity to rural areas. These include DakNet (Pentland et al., 2004) and JaldiMAC (Ben-David et al., 2010; Heimerl and Brewer, 2010; Mehendale et al., 2011; Saha et al., 2015). These solutions utilize either short-range technologies (e.g., WiFi, Bluetooth, ZigBee, and 6LowPAN) or long-range solutions (e.g., WiMax, GSM, 3G/4G, LTE/LTE-A, wireless mesh) or a combination thereof. Short-range technologies are generally not adequate for reliable rural connectivity covering wide geographical areas. Conversely, although cellular technologies provide larger transmission coverage and offer promising solutions in the rural context, limited business cases prohibit significant industry investments despite government subsidies (Hasan et al., 2014).
The recently proposed Long Range Wide Area Network (LoRaWAN) with its scalable star of stars network architecture and simple medium access mechanism, fulfills some of the requirements of providing connectivity in agricultural settings, i.e., long-range communication with low energy consumption (Shanmuga Sundaram et al., 2020). The LoRaWAN architecture consists of LoRa Nodes (LNs), LoRa Gateway (LG), and Network Server (NS). Each LG can be connected with a limited number of LNs on a given Spreading Factor (SF) through unlicensed channels. The SFs consume unequal energy and support uneven data transmission rate, and communication range (Kumari et al., 2022). Thus, the selection of appropriate SF for communicating the time series data from LN to LG helps reduce energy consumption and delay in smart agriculture applications. Although LoRaWAN provides promising applications in the agricultural domain, its very limited data rates make it unfit for some agricultural applications that require large data volumes. As an example, transferring large hyperspectral images collected by a drone would be infeasible with a low data rate technology such as LoRaWAN (Shah et al., 2017, 2018).
To overcome the limitations of existing wireless technologies and better utilize the under-utilized licensed spectrum resources, Dynamic Spectrum Access (DSA) (Akyildiz et al., 2006; Song et al., 2012) has emerged as an enabling technology. DSA networking is allowed (or in memoranda) by the United States Federal Communications Commission (FCC) in licensed channels such as TV band (Bahl et al., 2009; Khalil et al., 2017), GSM band (Hasan et al., 2014), LTE band (Tehrani et al., 2016), and CBRS Band. Similar policies are being adopted in other countries, such as Canada (Anonymous, 2024b) and South Africa (Anonymous, 2024a). DSA technologies have been investigated for rural connectivity, and most are based on TV whitespaces (TVWS) (Liang et al., 2008; Bahl et al., 2009; Kumar et al., 2016; Khalil et al., 2017), GSM whitespaces (Hasan et al., 2014) and LTE whitespaces (Surampudi and Mohanty, 2011). Although existing DSA architectures allow secondary devices to opportunistically access an unoccupied channel, they are restricted to an individual primary band only. Recent studies have shown that this is neither efficient nor effective under heterogeneous traffic demands and suffer from under- or over-provisioning of spectrum (Shah et al., 2017, 2018).
Consider an example in Figure 2. If the objective is to provide low end-to-end delay, for transmitting a certain text (a few Kilobytes) at large distances (several KMs), a TV band is possibly optimal because it offers high transmission coverage (several KMs) and adequate bandwidth (6 MHz). Whereas, for transmitting a large-sized video at very short distances (a few hundred meters), it may be more efficient to communicate over the CBRS band, which offers very high channel bandwidth (40 MHz) and low (yet sufficiently large) transmission coverage. Similarly, owing to a decent coverage and channel bandwidth, an LTE band is more promising for transmitting a medium-sized image at relatively small distances (a few KMs).
To address the heterogeneous traffic needs, we consider applying DSA on both unlicensed channels used in LoraWAN and licensed primary channels commonly used in the industry as shown in Figure 4. This approach can improve the efficiency and flexibility of channel selection according to generated data volume, transmission time requirements, and available bands. If the volume of generated data by LNs is small, offloading data to the network server can be performed with unlicensed channels through LoraWAN. Instead, large volume data such as images and videos will be transferred through the free licensed channels to the network server directly.
3.5 Data analysis and privacy
Data analytics is critical in precision agriculture, allowing farmers to adopt data-driven solutions. With numerous benefits, including better yield, reduced waste, and a greater yet precise understanding of environmental factors, data analytics reveals limitless future opportunities in agriculture. Profitable decisions in agriculture could be made when appropriate data are collected and analyzed timely, key stresses are identified, and prescriptive management actions are efficiently executed (Naik et al., 2017, 2017; Akintayo et al., 2018; Ghosal et al., 2018; Nagasubramanian et al., 2019; Chiozza et al., 2021; Chiranjeevi et al., 2022; Kar et al., 2021; Singh et al., 2021; Krause et al., 2022; Rairdin et al., 2022). These days data has become one of the key elements of smart farms and helps farmers in decisionmaking and maximizing productivity. Farms can create a lot of data streams, creating a “big data” situation. Big data has 10 dimensions (V’s): volume, velocity, variety, veracity, value, variability, vagueness, validity, venue, and vocabulary (Manyika et al., 2011; Kunisch, 2016; Kamilaris et al., 2017; Bhat and Huang, 2021). Wolfert et al. (2017) and Bhat and Huang (2021) in their studies comprehensively reviewed the application of big data in agriculture. Public and private companies are building solutions on this platform intending to solve crop production challenges faster, more accurately, and at a larger scale (Ghosal et al., 2019; Parmley et al., 2019a, Parmley et al., 2019b). Recent advances in data analysis methods such as computer vision, ML, and DL have empowered both researchers and farmers (Singh et al., 2016, 2018; Mahmud et al., 2021; Riera et al., 2021). There are continual improvements in ML methods and their applications, for example, self-supervised learning has shown improvement in the classification of agriculturally important insect pests in plants (Kar et al., 2023). For yield prediction, genotypic-topological graph neural network framework built on GraphSAGE has shown promise (Gupta and Singh, 2023). We have two broad categories of SCF-generated data: Raw sensor data and processed/refined data. The first pass of data analysis will turn the raw data into processed data and will be unique for each type of sensor. This is most likely to use some kind of ML approach. If the raw data is preserved, alternative data analysis methods can be used later, and results can be compared. For the refined data, data analysis will vary by the type of data and could be a simple statistical analysis that could use more complex ML methods for predictions or even a combination of the two.
Data privacy is an important consideration, particularly in the context of SCF, which heavily depends on analyzing and extracting higher-value information from users’ raw data. It will be very important to research all local legal obligations about managing users’ private data and maintain a privacy agreement with all users to clearly show how all data is used and/or shared. However, the main focus is to invest in techniques that entirely eliminate sharing raw data containing the exact value of data, statistical features, membership, and certain properties (Liu et al., 2021). Recently, distributed Machine Learning (DML), in particular, has had paradigm-shifting impacts on preserving data privacy. In DML, different parties have their private raw data and train a global model by transferring and aggregating the metadata (model parameters or gradients). Therefore, DML can be considered a privacy preserving technique when only metadata is shareable (Antwi-Boasiako et al., 2021). Two general categories are defined for DML based on the architecture of ML systems; centralized distributed learning and decentralized distributed learning. Transferring the higher-value information (metadata) in Federated Learning (FL) (Konečnỳ et al., 2016; Bonawitz et al., 2019; Nguyen et al., 2021), the most famous method in centralized distributed learning, happens between the parties and a parameter server (cloud). Then, the server aggregates the metadata to update the global model (Li et al., 2021). There are two different settings for federated learning; cross-device, which involves a large number of IoT devices, and cross-silo containing a small number of reliable clients (Kairouz et al., 2021). Due to the inherent feature of agriculture data such as weather data, soil data, and crop management data, which are scattered and siloed in different servers, Manoj et al. (2022) applied a federated averaging technique to train a ML model for soybean yield prediction. Similarly, using the federated learning-based method, researchers could detect the intrusion securely in smart agriculture (Friha et al., 2022).
On the other hand, in decentralized distributed learning, parties have peer-to-peer communication to exchange metadata; each party aggregates the received model parameters and updates the model. Decentralized learning architectures recently attracted attention and addressed the challenges in conventional centralized techniques, such as server latency, single point on failure, and traceability (Jiang et al., 2017; Nadiradze et al., 2021; Esfandiari et al., 2021b). To visually present how DML can greatly reduce the privacy risk, Figure 5A shows an instance of applying decentralized ML in agriculture in which each party has its private data collected from a specific IoT device and trains the model through collaborative communication of metadata based on the defined network topology (in this case a fully connected network). Esfandiari et al. (2021a) used decentralized distributed learning to train an autoencoder to find anomalies in maize data. This study showed finding anomalies in private DML settings helps detect plants with irregular growth and realize probable issues during the data collection (e.g., tilted camera, rain on the lens).
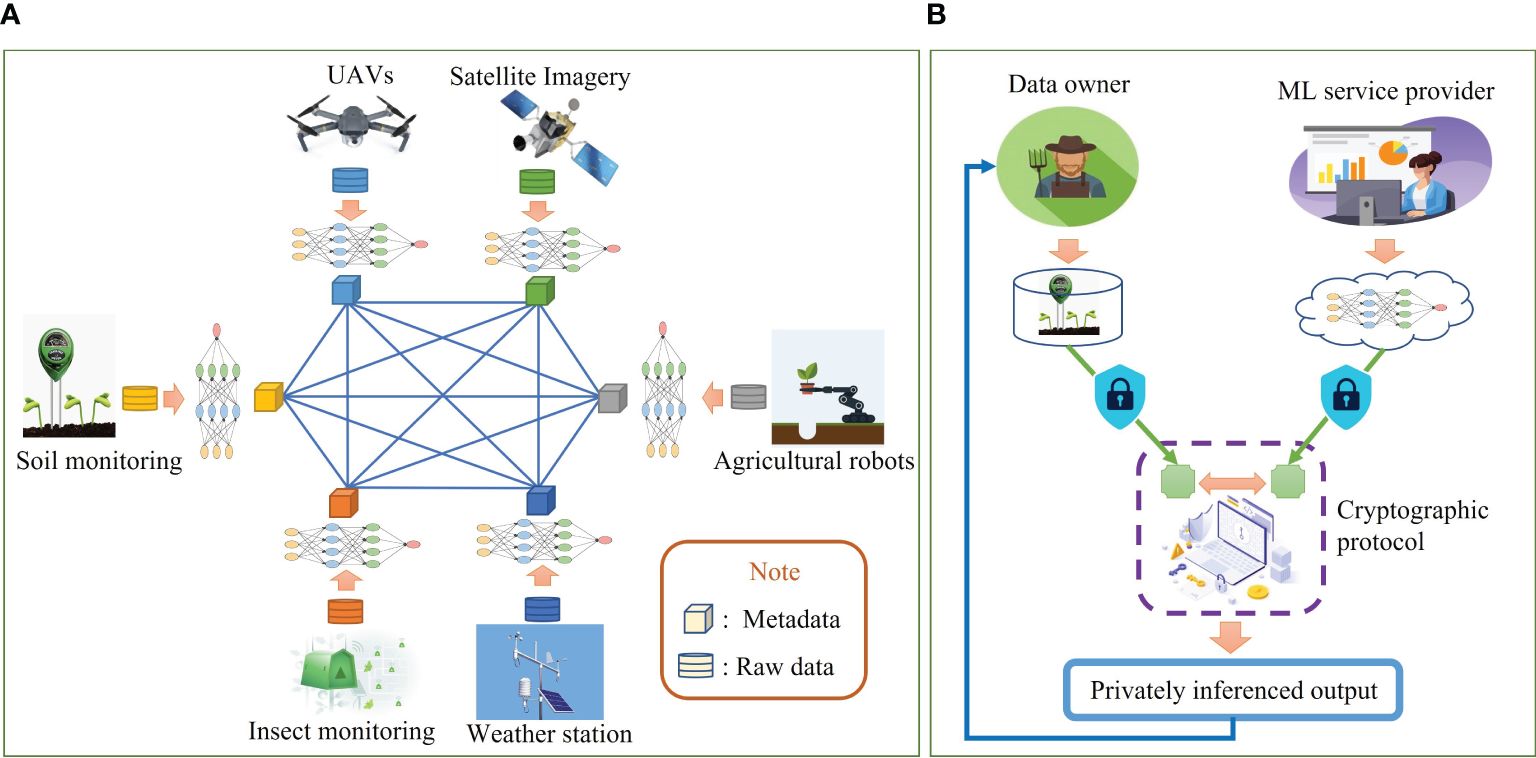
Figure 5 Privacy preserving techniques while using machine learning: (A) preserving data privacy by considering peer-to-peer communication of metadata (instead of raw data) to train a machine learning model, (B) private inference by back-and-forth communicating encrypted information between data owner and ML service provider.
Besides DML, which naturally gives a certain level of data privacy, encryption and obfuscation are also well-known ML schemes for privacy preservation. Encryption (cryptography-based method) and obfuscation can be applied to training data and/or ML model (Gai et al., 2016; Juvekar et al., 2018; Mishra et al., 2020) (in some cases, neither the data owner nor the ML model provider does not wish to share their private parameters). In the literature, the cryptographic and obfuscation protocols include homomorphic encryption, additive secret sharing, differential privacy, and garbled circuits (Dwork, 2008; Phong et al., 2017; Hussain et al., 2020; Duan et al., 2022). However, all of these methods are computationally heavy, making the training/inference process several orders of magnitude slower. In agriculture, Cho et al. (2021b) applied encryption by proposing novel deep neural network architectures for plant stress phenotyping that were geared towards preserving the privacy of both the user and service provider. In this method, some neural network architectures were redesigned by minimizing the number of nonlinear operations (ReLu function) to increase the inference time of encrypting model (Cho et al., 2021a). Their approach also addresses data trustworthiness, empowering farmers for SCFs. Figure 5B represents the encryption scheme where privacy preserves for both the user and service provider.
Moreover, DML and encryption techniques together were widely studied for privacy-preserving purposes (McMahan et al., 2017; Hao et al., 2019; Mandal and Gong, 2019; Zhao et al., 2019; Zhu et al., 2021). In this regard, homomorphic encryption and/or differential privacy are commonly combined with FL. In the agriculture setting, Durrant et al. (2022) show that the privacy concern in the agri-food sector can be overcome by using federated and model-sharing machine learning as well as applying differential privacy methods. The intrusion could also be detected through an FL-based gated recurrent unit neural network algorithm (FedGRU) using the encoded data (Kumar et al., 2021). Overall, combining DML and encryption methods would be a very interesting future topic, especially in SCFs.
4 The human dimensions (social sciences, economics)
During the last two decades, on-farm research became popular due to growing and intersecting interests among farmers, agronomists, and the research community. Numerous farmer networks were organized across the country for community-based on-farm evaluations of new and existing agronomic practices (Chapman et al., 2016). Organized groups of local farmers who use GPS-enabled equipment and treatment protocols developed by researchers to conduct on-farm trials/experiments on their farms, apply treatments within their fields, and collect data (Kyveryga et al., 2018). New analytical approaches were also developed to enhance analyses and interpretations of on-farm research data and develop dynamic decision aid tools (Kyveryga, 2019; Laurent et al., 2019). In addition, new community-based engagement and evaluation methods were adopted by public university extension personnel and private industry agronomists (Thompson et al., 2019).
4.1 Net-benefits of data-sharing platforms
Increased profitability and risk reduction are some of the benefits of digital agricultural innovations to farmers. However, there are also costs associated with adopting such new technologies. These may include the cost of investing in the required technological infrastructure; the cost associated with data collection and management; the cost of analyzing the data; and the cost of sharing the data output (Wysel et al., 2021). These are non-trivial considerations for small scale and less technologically developed farming enterprises, as the variations in risk-benefit are large. It is important to assess the overall cost of participating in a socio-technical platform and compare it with the potential economic gains.
4.2 Social barriers to data-sharing
The adoption of technologies is also impacted by various social and ethical barriers. Despite the promise of digital agricultural technologies and data-sharing platforms, farmers are often reluctant to engage with these technologies due to issues surrounding data ownership, control, and usage (Wiseman et al., 2019). Among other concerns, some farmers may be worried that technology providers could make profits off their data or share their data with third parties; and in some cases, there may be a lack of trust in the innovation itself in the context of change, such as climate change (Jakku et al., 2019; Wiseman et al., 2019). Also, there is a general lack of trust in data operators due to unequal power. Potential causes for farmers’ uncertainty about their rights to data ownership and usage is the lack of clarity in the terms and conditions of the agreements with service providers—not to mention the risk of potential data breaches (i.e., the risk that confidential, protected or sensitive information could be stolen or shared with third parties). Hence, there is a need for agricultural innovations that are trusted, salient and actionable for farmers to be willing to adopt them (Valdivia et al., 2018).
4.3 Agricultural community and the practice of farming
The concept of the practice of farming encompasses clearly defined values, institutions, and policies (Valdivia et al., 2012). This concept has been used to understand farmers’ behavior and actions in farming during times of policy changes to learn about how farmers transition to a new context (Shucksmith, 1993; Shucksmith and Herrmann, 2002). It has also informed the introduction of new practices, like the case of agroforestry in the context of traditional commodity farming (Raedeke et al., 2003; Valdivia et al., 2012), the development of genetically modified crops (Oreszczyn et al., 2010; Valdivia et al., 2014), and the creation of new technologies such as remote sensing with drones for agriculture (Valdivia et al., 2018).
Values and motivations differ in the practice of farming according to the types of crops, the nature of the market farmers engage in, and the institutions in place that support the practice (Valdivia et al., 2012). In commodities like corn and soybeans, the field of farming has an established network of organizations and institutions that support the practice. Within it, “habitus” consists of the shared values in the practice (Raedeke et al., 2003; Glover, 2010; Valdivia et al., 2021). The development of new tools can benefit from the understanding of these values, the role of existing institutions that are part of the practice, and the adaptive capacity of the decision-makers. It requires an understanding of the level at which these happen and what we need to learn about the characteristics of the decision makers (Ostrom, 2009) to inform how new tools can provide information in changing contexts that can be trusted and acted upon (Duarte Alonso et al., 2018; Valdivia et al., 2018).
4.4 Translational research and communities of practice
According to Woolf (2008), a translational research process is one that applies to advances in science toward the development of new technologies and processes, as well as guarantees that the products of research reach their intended population. By engaging the various stakeholders in participatory activities and sessions, the translational research process promotes learning, responds to challenges, and identifies opportunities. There are advantages to engaging the decision-makers from the onset of the innovation. This is critical in contexts of change. For example, simulations of pests in the context of climate change have shown that trusted and timely decisions increase the probability of success in reducing the impact of climate change. In the case of farming, the scale of the farm and that of the community and landscape as the scale of analysis, are the framework for the study of networks (Garrett et al., 2011, 2013). Participatory processes are means to facilitate learning and the development of trust (Patt et al., 2005; Gilles and Valdivia, 2009; Valdivia et al., 2009), as well as the co-production of knowledge (Yager et al., 2019).
It is argued that learning occurs as a result of social processes, and not merely through internalized cognitive processes; i.e., learning is the product of interactions between people, which lead to sharing of experiences and the creation of new knowledge (Oreszczyn et al., 2010). Therefore, communities of practice (CoPs), defined as groups of people who share a common activity, pursuit, or concern, are important in driving processes of change (Oreszczyn et al., 2010; Valdivia et al., 2014). The literature suggests that farmers’ own experience with innovation, as well as the experience of their neighbors, help in decreasing the unfamiliarity with that innovation—which often acts as a significant barrier to adoption (Foster and Rosenzweig, 1995).
In essence, the gains of learning by doing and knowledge spillovers are harnessed to a greater extent in an environment of information sharing. A CoP can help maximize farmers’ understanding of the benefits, their trust in the information source, and their eventual acceptance of the technology. Furthermore, a CoP encompasses the innovation pathway engaging all stakeholders—including feedback processes between scientists and farmers, and to other stakeholders in the practice of farming from both the public and private sectors (Garrett et al., 2013; Valdivia et al., 2014, 2018).
Moreover, there is a need to understand the types of incentives that farmers will respond to. There is evidence that farmers who state that privacy is important to them could still be incentivized to participate in data-sharing platforms (Turland and Slade, 2020). As a result, it is important to investigate various factors that could potentially boost participation rates in socio-technical platforms, as well as better understand the issues that influence the adoption and overall utilization of digital agricultural technologies.
4.5 Behavioral factors influencing agricultural technology adoption
Traditionally, agricultural adoption research has sought to explain adoption behavior in relation to extrinsic factors such as economic, institutional, and household-specific factors (Mwangi and Kariuki, 2015). A more recent strand of literature has included social networks, learning, and other behavioral forces (e.g., beliefs, risk, and trust) as determinants of adoption (Marra et al., 2003; Chavas and Nauges, 2020). For example, farmers belonging to a social group or organization are more likely to share information and engage in social learning about the technology (Katung and Akankwasa, 2008), hence increasing their likelihood to adopt the technology. However, social learning may also impact technology adoption negatively by increasing the likelihood of free-riding behavior among farming neighbors (Bandiera and Rasul, 2006).
In the context of agricultural technology adoption, different types of learning can occur simultaneously as the technology is being developed and deployed (Rosenberg and Nathan, 1982). Figure 6 shows the technology adoption loop. First, the developers can learn how to improve their technology through feedback from potential end-users (i.e., farmers). Second, the end-users (farmers) can improve their mastery of the adopted technology over time through personal user experience and through information acquired from various sources, including established social networks, extension specialists, and other farmers with previous experience using the technology. This creates a feedback loop process encompassing all actors in the agriculture ecosystem, from scientists and developers to potential end-users.
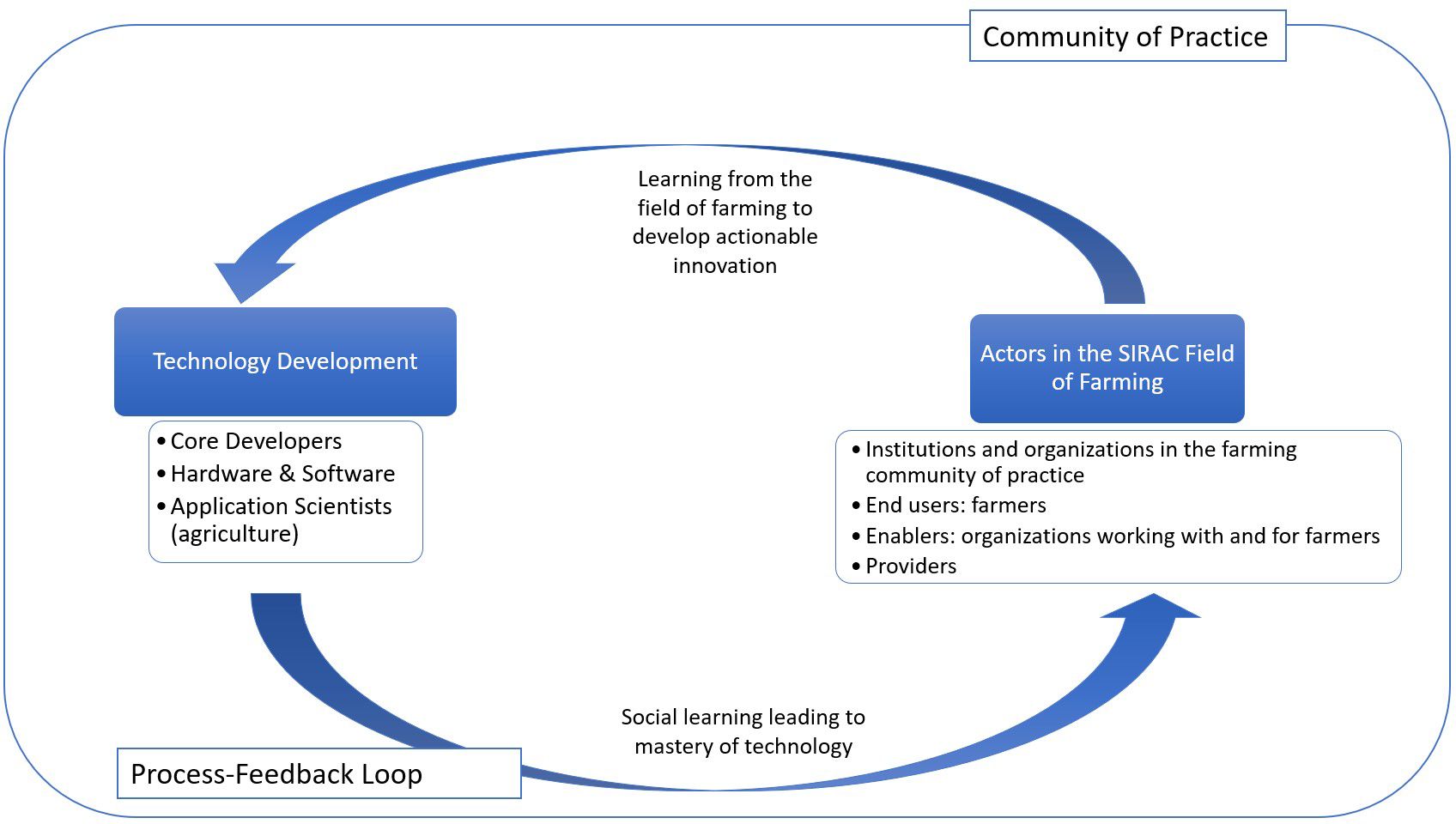
Figure 6 Translational research process within the farmer network, such as SIRAC community of practice. FIgure adapted from Bekee et al. (2024).
While social learning has received some attention in the agricultural adoption literature, the social preferences discussed extensively in other behavioral fields, including behavioral economics, have received little attention. In this regard, behavioral evidence demonstrates that other-regarding preferences, such as altruism and social norms about fairness, impact decision-making regarding technology adoption (Chouinard et al., 2008; Sheeder and Lynne, 2011). Modeling the influence of social networks on agricultural adoption decisions, beyond simple information diffusion, holds significant potential to improve the effectiveness of the deployment and adoption of agricultural innovations (Streletskaya et al., 2020). For instance, it can help technology developers understand to what extent farmers would conform to social norms as they see other farmers adopt new technology, and which are characteristics of the social networks they are more likely to join.
Cooperative behavior is another factor that impacts technology adoption, especially in settings that require collective action. In this regard, the behavioral literature has studied how beliefs, trust, and risk preferences shape cooperative behavior. The motivation to study the relationship between cooperation and trust is that, for a conditional cooperator, the decision to contribute to the formation of a public good (a technological platform in our context) requires some trust in the cooperativeness of others (Anderson et al., 2004; Gächter et al., 2004; Leonard et al., 2010). Such trust is obviously related to the individual belief held about others’ cooperativeness. In the context of agricultural technology adoption, for a farmer who is not a free rider, contributing to the creation of the technological platform (by sharing farm data) without knowing how many other farmers are going to contribute can be viewed as a decision under risk. Thus, each farmer’s expectations about the behavior of others is a crucial determinant of contribution outcomes (Cárdenas et al., 2017). Farmers who are more risk averse may choose to contribute less to compensate for the risk of others, not contributing (Charness and Villeval, 2009; Teyssier, 2012; Dannenberg et al., 2015). For example, Liu (2013) elicits risk preferences from Chinese cotton farmers and finds that risk-averse and loss-averse farmers tend to be late adopters of Bt-cotton. Modeling cooperative behavior in the context of technology adoption is important as farmers are heterogeneous in terms of their risk aversion and trustworthiness and are more likely to join trust-supporting social networks (Attanasio et al., 2012). This is more so when the resolution of the product depends on how many contribute to the platform.
4.6 The economics of learning with SCFs
The foundation for the economics of SCFs are models of learning (Lucas, 1988; Foster and Rosenzweig, 1995; Acemoglu, 2009; Conley and Udry, 2010). Farmers face risks and uncertainty attached to new farming practices or technologies because the suitability of the new farming methods depends on the farmer’s experience, knowledge, skill, and area-specific climate and agronomic conditions. The lack of reliable and persuasive sources of information about new technologies, their expected benefit, and how to apply them efficiently impedes changes in farming behavior (Moore, 2008). As a result, farmers’ adoption decision requires learning, and unfortunately, learning can be costly. Acquiring, validating, analyzing, and applying new information requires time and expertise not always available to farmers. SCFs can accelerate technological adoption by reducing learning costs (Foster and Rosenzweig, 1995; Conley and Udry, 2010; Carvalho and Voigtländer, 2014; Krishnan and Patnam, 2014). For example, in a recent prominent social learning analysis, economists show that network-based technology diffusion is cheaper than extension programs (BenYishay and Mobarak, 2019). SCFs can outperform traditional agricultural extension programs if the information disseminating nodes are incentivized to transfer information about the new technology. When leading farmers were given a small incentive to distribute information to others through the network, farmer-to-farmer learning programs outperformed the government extension program in increasing farmer knowledge of new technologies. The advantage of SCFs can be significantly large if the traditional extension program requires the agents to regularly visit rural areas, especially when the extension position is remote.
Figure 7 illustrates the basic structure of a learning model for assessing the benefits of SCFs for adopting novel pest management technologies. We compare two settings with different learning mechanisms. On the left side, Figure 7A shows the setting for the traditional pest management technique where there is no farmer network. Pest management involves uncertainty about the amount of pesticide and the timing of the application, and farmers manage uncertainty based on their prior beliefs and their limited knowledge about the new technology. This lack of expertise leads to high uncertainty and high variability in yields and profits. The different sizes of circular shapes in the figure represent this heterogeneity in agricultural productivity.
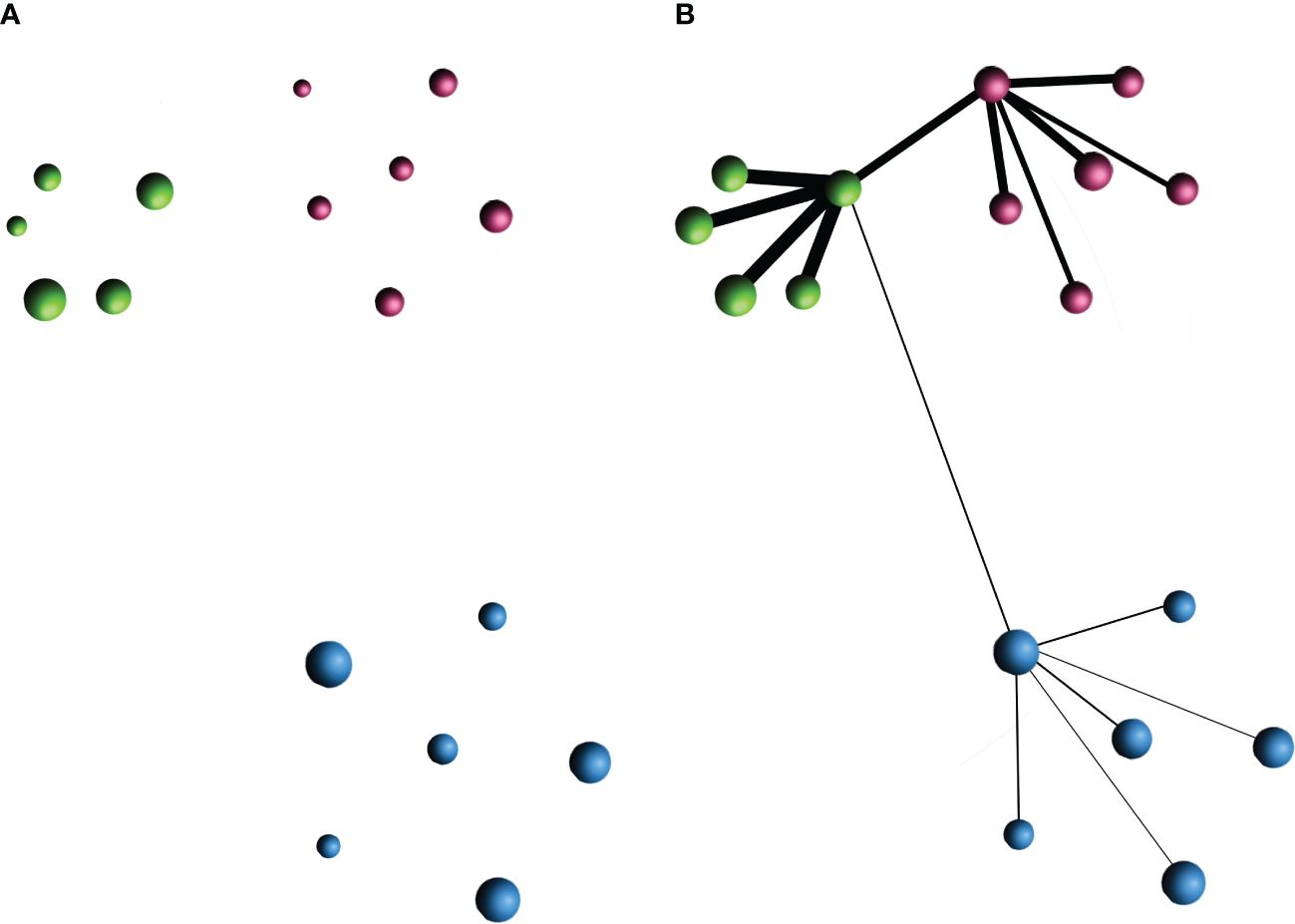
Figure 7 Size of the circular shapes indicates heterogeneity in agricultural production. The different colors stand for similar farms located in the same geographic cluster. In panel (B), the lines indicate the connections between farmers. The lines’ thickness indicates the precision of the signals. (A) Traditional Scouting; No network; (B) New pest management technology; with network.
The second setting illustrated in Figure 7B has a network of farmers with three clusters represented by different colors. In this setting, farmers use a new pest detection technology, digital cameras that continuously monitor selected farms for the degree of pest infestation. These digital cameras replace traditional scouting. Farmers can then learn from their peers through communication signals sent through the network. The critical parameters in this model are the precision or learning value of the signals, represented in panel b by the thickness of the connecting lines. For example, thicker lines, such as in the green cluster, mean that the signals among this homogeneous group of neighboring farmers are very informative. Using the distance between farmers to measure homogeneity and signal precision is common. For example, a receiving farmer may not trust the informational signal received from a distant farmer. The value of the SCF in the learning model illustrated in Figure 7 is measured by the reduction in uncertainty and the increase in farm profitability resulting from the higher frequency of precise network signals.
Finally, learning models can also capture the flow of unreliable information. For example, in the absence of network-based learning and expert advice, farmers may obtain information on the farming practices through word of mouth, generic broadcast programming, or agricultural input dealers, who may be poorly informed or have incentives to provide misleading information on the product, time of application, and efficiency. External agents such as pesticide retailers and commercial companies can shape the farmers’ pest management decisions (Moore, 2008). Moore (2008) shows that the flow of information from the retail network often plays the dominant role in pest management decisions and may negatively impact the farmers’ awareness of and willingness to adjust the input use or to adopt the new farming technology. Digital monitoring and SCF networking technology have the potential to enhance the accuracy of the information.
5 Conclusion - transferability, scalability, and adoptability of SCFS
In this review, we provided a comprehensive listing of technology tools and networking and communication infrastructure that enables the creation of SCFs. Currently, these conditions are not universally present in all countries. However, a SCF network has a high potential for transferability and scalability to regions where farmers have access to sensors, platforms for data collection, and internet connectivity at a minimum. In more industrialized economies, SCFs can be more easily created. SCFs can be established amongst farmers who are geographically neighbors. However, the network can have the flexibility to expand to dissimilar cropping systems, management practices, soil and climate conditions, and community members. Communities can become involved through local cooperatives and/or farmer organizations within and across states. This will lead to data sharing across farms, improving their ability to engage in farm management strategies to improve productivity and broader adoption. Scalability can be facilitated with better cellular connectivity or rural broadband access to cover more significant, not necessarily contiguous, areas. The sustained usage of the SCF network will depend on buy-in from its participants and centralized community resources to support such networks’ implementation and extended life cycle. There will be a need for continued education and awareness programs to ensure that the entire community is well-versed in the network’s capabilities and appreciates such a network’s benefits. SCFs offer varying benefits to farmers, for e.g., in early and effective pest management and mitigation of crop loss disasters. Networks are particularly helpful for vulnerable farmers with limited resources and experience. SCFs provide valuable expertise to farmers with limited access to other sources. Network design should consider farmer characteristics to optimize information sharing using ML and simulations, ensuring benefits for all farmers. SCFs have significant policy implications, such as complementing agricultural insurance policies and reducing losses and premiums. Since network participation benefits vary among farmers, differentiated pricing strategies and subsidies may be necessary. Policymakers can use network simulations to optimize participation costs and extend network benefits to a wide range of farmers.
There is a need for research in the area of new/improved technologies for rural connectivity and community decision-making, integrated with translational social research to address issues of adaptability, trust, and risk preferences, and economics research to justify the benefits to farmers. The research focusing on assessing social and economic incentives for farmers and other stakeholders will facilitate participation in the network, possibly through new partnerships with cooperatives, while ensuring farmer data privacy and the development of data use agreements. These SCF networks enabling rural communication technologies, including privacy-preserving distributed data analytics and machine learning tools, can apply to a broad range of cyber-physical systems applications, such as IoTs, transportation networks, and smart grids. In alignment with these technologies, the integration of Cyber-agricultural systems (CAS) offers a vision of ultra-precision agriculture, integrating improved sustainability, profitability, and technology by employing efficient sensing, AI, and robotics to address crop issues at the individual plant level (Sarkar et al., 2023). These research topics will require a trans-disciplinary team of researchers from multiple domains, including agronomy, computer science, plant pathology, behavioral science, economics, sensing and machine learning, and precision agriculture, along with integral participation of farmers to define and conduct these research, so the benefits of SCF network is immediate and useful. The usefulness of citizen science data sets (iNaturalist, 2023) for deep learning-powered real-time identification of insects is an example of bridging the gap in the development of SCF (Chiranjeevi et al., 2023; Saadati et al., 2023).
Author contributions
AS: Conceptualization, Funding acquisition, Supervision, Writing – original draft, Writing – review & editing, Project administration. BJB: Writing – original draft, Writing – review & editing. BB: Writing – original draft, Writing – review & editing. SB: Writing – original draft, Writing – review & editing. SF: Writing – original draft, Writing – review & editing. FF: Writing – original draft, Writing – review & editing. AG: Writing – original draft, Writing – review & editing. AJ: Writing – original draft, Writing – review & editing. JM-P: Writing – original draft, Writing – review & editing. KM: Writing – original draft, Writing – review & editing. AP: Writing – original draft, Writing – review & editing. VT: Writing – original draft, Writing – review & editing. XT: Writing – original draft, Writing – review & editing. AV: Writing – original draft, Writing – review & editing. MC: Writing – original draft, Writing – review & editing, Supervision. SD: Writing – original draft, Writing – review & editing, Conceptualization, Funding acquisition, Supervision. GD: Writing – original draft, Writing – review & editing, Conceptualization, Funding acquisition, Supervision. PK: Writing – original draft, Writing – review & editing, Conceptualization, Funding acquisition, Supervision. SSa: Writing – original draft, Writing – review & editing, Conceptualization, Funding acquisition, Supervision. MS: Writing – original draft, Writing – review & editing, Conceptualization, Funding acquisition, Supervision. SSi: Writing – original draft, Writing – review & editing, Conceptualization, Funding acquisition, Supervision. CV: Conceptualization, Funding acquisition, Supervision, Writing – original draft, Writing – review & editing.
Funding
The author(s) declare financial support was received for the research, authorship, and/or publication of this article. This work was supported by Smart Integrated Farm Network for Rural Agricultural Communities (SIRAC) (NSF S&CC 1952045), AI Institute for Resilient Agriculture (USDA-NIFA 2021–67021-35329), COALESCE: COntext Aware LEarning for Sustainable CybEr-Agricultural Systems (NSF CPS Frontier 1954556), FACT: A Scalable Cyber Ecosystem for Acquisition, Curation, and Analysis of Multispectral UAV Image Data (USDA-NIFA 2019–67021-29938), and USDA CRIS Project IOW04714.
Acknowledgments
We thank farmer partners in the community of practice, awareness community, as well as the Iowa Soybean Association.
Conflict of interest
The authors declare that the research was conducted in the absence of any commercial or financial relationships that could be construed as a potential conflict of interest.
Publisher’s note
All claims expressed in this article are solely those of the authors and do not necessarily represent those of their affiliated organizations, or those of the publisher, the editors and the reviewers. Any product that may be evaluated in this article, or claim that may be made by its manufacturer, is not guaranteed or endorsed by the publisher.
References
Aasen H., Burkart A., Bolten A., Bareth G. (2015). Generating 3d hyperspectral information with lightweight uav snapshot cameras for vegetation monitoring: From camera calibration to quality assurance. ISPRS J. Photogrammetry Remote Sens. 108, 245–259. doi: 10.1016/j.isprsjprs.2015.08.002
Akintayo A., Tylka G. L., Singh A. K., Ganapathysubramanian B., Singh A., Sarkar S. (2018). A deep learning framework to discern and count microscopic nematode eggs. Sci. Rep. 8, 1–11. doi: 10.1038/s41598-018-27272-w
Akyildiz I. F., Lee W.-Y., Vuran M. C., Mohanty S. (2006). Next generation/dynamic spectrum access/cognitive radio wireless networks: A survey. Comput. Networks 50, 2127–2159. doi: 10.1016/j.comnet.2006.05.001
Alharbi H. A., Aldossary M. (2021). Energy-efficient edge-fog-cloud architecture for iot-based smart agriculture environment. IEEE Access 9, 110480–110492. doi: 10.1109/ACCESS.2021.3101397
Anderson L. R., Mellor J. M., Milyo J. (2004). Social capital and contributions in a public-goods experiment. Am. Economic Rev. 94, 373–376. doi: 10.1257/0002828041302082
Antwi-Boasiako E., Zhou S., Liao Y., Liu Q., Wang Y., Owusu-Agyemang K. (2021). Privacy preservation in distributed deep learning: A survey on distributed deep learning, privacy preservation techniques used and interesting research directions. J. Inf. Secur. Appl. 61, 102949. doi: 10.1016/j.jisa.2021.102949
Astill J., Dara R. A., Fraser E. D., Roberts B., Sharif S. (2020). Smart poultry management: Smart sensors, big data, and the internet of things. Comput. Electron. Agric. 170, 105291. doi: 10.1016/j.compag.2020.105291
Attanasio O., Barr A., Cardenas J. C., Genicot G., Meghir C. (2012). Risk pooling, risk preferences, and social networks. Am. Economic Journal: Appl. Economics 4, 134–167. doi: 10.1257/app.4.2.134
Babcock B. A., Pautsch G. R. (1998). Moving from uniform to variable fertilizer rates on iowa corn: Effects on rates and returns. J. Agric. Resource Economics 23, 385–400.
Bahl P., Chandra R., Moscibroda T., Murty R., Welsh M. (2009). White space networking with wi-fi like connectivity. ACM SIGCOMM Comput. Communication Rev. 39, 27–38. doi: 10.1145/1592568.1592573
Bandiera O., Rasul I. (2006). Social networks and technology adoption in northern Mozambique. Economic J. 116, 869–902. doi: 10.1111/j.1468-0297.2006.01115.x
Barmeier G., Schmidhalter U. (2017). High-throughput field phenotyping of leaves, leaf sheaths, culms and ears of spring barley cultivars at anthesis and dough ripeness. Front. Plant Sci. 8, 1920. doi: 10.3389/fpls.2017.01920
Barreto A., Lottes P., Yamati F. R. I., Baumgarten S., Wolf N. A., Stachniss C., et al. (2021). Automatic uav-based counting of seedlings in sugar-beet field and extension to maize and strawberry. Comput. Electron. Agric. 191, 106493. doi: 10.1016/j.compag.2021.106493
Bekee B., Segovia M. S., Valdivia C. (2024). Adoption of smart farm networks: a translational process to inform digital agricultural technologies. Agric. Hum. Values. doi: 10.1007/s10460-024-10566-3
Bellavista P., Zanni A., Corradi A., Berrocal J., Fischini L., Das S. K. (2019). A survey on fog computing for the internet of things. Pervasive Mobile Computing 52, 71–99. doi: 10.1016/j.pmcj.2018.12.007
Ben-David Y., Vallentin M., Fowler S., Brewer E. (2010). “Jaldimac: taking the distance further,” in Proceedings of the 4th ACM workshop on networked systems for developing regions, vol. 2. (New York City, New York: ACM).
BenYishay A., Mobarak A. M. (2019). Social learning and incentives for experimentation and communication. Rev. Economic Stud. 86, 976–1009. doi: 10.1093/restud/rdy039
Betti Sorbelli F., Coro F., Das S. K., Di Bella E., Maistrello L., Palazzetti L., et al. (2022a). “A drone-based application for scouting halyomorpha halys bugs in orchards with multifunctional nets,” in 2022 IEEE International Conference on Pervasive Computing and Communications Workshops and other Affiliated Events (PerCom Workshops). Pisa, Italy: IEEE. doi: 10.1109/PerComWorkshops53856.2022.9767309
Betti Sorbelli F., Coro F., Das S. K., Maistrello L., Palazzetti L., Pinotti C. M. (2022b). “Drone-based optimal and heuristic orienteering algorithms towards bug detection in orchards,” in 18th International Conference on Distributed Computing in Sensor Systems (DCOSS), Marina del Rey, Los Angeles, CA, USA: IEEE. doi: 10.1109/DCOSS54816.2022.00032
Bhat S. A., Huang N.-F. (2021). Big data and ai revolution in precision agriculture: Survey and challenges. IEEE Access 9, 110209–110222. doi: 10.1109/ACCESS.2021.3102227
Bonawitz K., Eichner H., Grieskamp W., Huba D., Ingerman A., Ivanov V., et al. (2019). Towards federated learning at scale: System design. Proc. Mach. Learn. Syst. 1, 374–388.
Bongiovanni R., Lowenberg-DeBoer J. (2004). Precision agriculture and sustainability. Precis. Agric. 5, 359–387. doi: 10.1023/B:PRAG.0000040806.39604.aa
Boyd N. S., White S. (2009). Impact of wild blueberry harvesters on weed seed dispersal within and between fields. Weed Sci. 57, 541–546. doi: 10.1614/WS-08-156.1
Cárdenas J.-C., Janssen M. A., Ale M., Bastakoti R., Bernal A., Chalermphol J., et al. (2017). Fragility of the provision of local public goods to private and collective risks. Proc. Natl. Acad. Sci. 114, 921–925. doi: 10.1073/pnas.1614892114
Carvalho V. M., Voigtländer N. (2014). Input diffusion and the evolution of production networks (Cambridge, Massachusetts: National Bureau of Economic Research). Tech. rep.
Chapman K., Kyveryga P., Morris T., Menke T. (2016). Farmer network design manual. Environmental Defense Fund.
Charness G., Villeval M.-C. (2009). Cooperation and competition in intergenerational experiments in the field and the laboratory. Am. Economic Rev. 99, 956–978. doi: 10.1257/aer.99.3.956
Chavas J.-P., Nauges C. (2020). Uncertainty, learning, and technology adoption in agriculture. Appl. Economic Perspect. Policy 42, 42–53. doi: 10.1002/aepp.13003
Chen M., Liang W., Das S. K. (2021). “The utility maximization for data collection in UAV-enabled sensor networks via judiciously determining hovering locations,” in 19th IEEE International Conference on Pervasive Computing and Communications (PerCom), Vol. 1–10.
Chiozza M. V., Parmley K. A., Higgins R. H., Singh A. K., Miguez F. E. (2021). Comparative prediction accuracy of hyperspectral bands for different soybean crop variables: From leaf area to seed composition. Field Crops Res. 271, 108260. doi: 10.1016/j.fcr.2021.108260
Chiranjeevi S., Sadaati M., Deng Z. K., Koushik J., Jubery T. Z., Mueller D., et al. (2023). Deep learning powered real-time identification of insects using citizen science data. arXiv preprint arXiv:2306.02507. doi: 10.48550/arXiv.2306.02507
Chiranjeevi S., Young T., Jubery T. Z., Nagasubramanian K., Sarkar S., Singh A. K., et al. (2022). “Exploring the use of 3d point cloud data for improved plant stress rating,” in 2022 AAAI workshop on AI for agriculture and food systems. openreview.net, Amherst, Massachusetts.
Cho M., Ghodsi Z., Reagen B., Garg S., Hegde C. (2021a). Sphynx: Relu-efficient network design for private inference. arXiv preprint arXiv:2106.11755. doi: 10.1109/MSEC.2022.3165475
Cho M., Nagasubramanian K., Singh A. K., Singh A., Ganapathysubramanian B., Sarkar S., et al. (2021b). “Privacy-preserving deep models for plant stress phenotyping,” in AI for agriculture and food systems. In 2022 AAAI workshop on AI for agriculture and food systems. openreview.net, Amherst, Massachusetts.
Chouinard H. H., Paterson T., Wandschneider P. R., Ohler A. M. (2008). Will farmers trade profits for stewardship? heterogeneous motivations for farm practice selection. Land Economics 84, 66–82. doi: 10.3368/le.84.1.66
Conley T. G., Udry C. R. (2010). Learning about a new technology: Pineapple in Ghana. Am. economic Rev. 100, 35–69. doi: 10.1257/aer.100.1.35
Cressman K. (2013). Role of remote sensing in desert locust early warning. J. Appl. Remote Sens. 7, 075098. doi: 10.1117/1.JRS.7.075098
Dannenberg A., Löschel A., Paolacci G., Reif C., Tavoni A. (2015). On the provision of public goods with probabilistic and ambiguous thresholds. Environ. Resource economics 61, 365–383. doi: 10.1007/s10640-014-9796-6
de Azevedo Peixoto L., Moellers T. C., Zhang J., Lorenz A. J., Bhering L. L., Beavis W. D., et al. (2017). Leveraging genomic prediction to scan germplasm collection for crop improvement. PloS One 12, e0179191. doi: 10.1371/journal.pone.0179191
De Vita F., Nocera G., Bruneo D., Tomaselli V., Giacalone D., Das S. K. (2020). “Quantitative analysis of deep leaf: a plant disease detector on smart edge,” in 2020 IEEE International Conference on Smart Computing (SMARTCOMP). Bologna, Italy: IEEE. doi: 10.1109/SMARTCOMP50058.2020
De Vita F., Nocera G., Bruneo D., Tomaselli V., Giacalone D., Das S. K. (2021). Porting deep neural networks on the edge via dynamic k-means compression: A case study of plant disease detection. Pervasive Mobile Computing 75, 101437. doi: 10.101j.pmcj.2021.101437
Dobbels A. A., Lorenz A. J. (2019). Soybean iron deficiency chlorosis high-throughput phenotyping using an unmanned aircraft system. Plant Methods 15, 1–9. doi: 10.1186/s13007-019-0478-9
Duan J., Zhou J., Li Y., Huang C. (2022). Privacy-preserving and verifiable deep learning inference based on secret sharing. Neurocomputing 483, 221–234. doi: 10.1016/j.neucom.2022.01.061
Duarte Alonso A., Kok S., O’Shea M. (2018). Family businesses and adaptation: A dynamic capabilities approach. J. Family economic Issues 39, 683–698. doi: 10.1007/s10834-018-9586-3
Durrant A., Markovic M., Matthews D., May D., Enright J., Leontidis G. (2022). The role of cross-silo federated learning in facilitating data sharing in the agri-food sector. Comput. Electron. Agric. 193, 106648. doi: 10.1016/j.compag.2021.106648
Dwork C. (2008). “Differential privacy: A survey of results,” in International conference on theory and applications of models of computation (New York City, New York, United States: Springer), 1–19.
Esfandiari Y., Nagasubramanian K., Fotouhi F., Schnable P. S., Ganapathysubramanian B., Sarkar S. (2021a). “Distributed deep learning for persistent monitoring of agricultural fields,” in NeurIPS 2021 AI for science workshop. Virtual: Neural Information Processing Systems Foundation).
Esfandiari Y., Tan S. Y., Jiang Z., Balu A., Herron E., Hegde C., et al. (2021b). “Cross-gradient aggregation for decentralized learning from Non-IID data,” in International Conference on Machine Learning. 3036–3046, PMLR.
Foster A. D., Rosenzweig M. R. (1995). Learning by doing and learning from others: Human capital and technical change in agriculture. J. political Economy 103, 1176–1209. doi: 10.1086/601447
Friha O., Ferrag M. A., Shu L., Maglaras L., Choo K.-K. R., Nafaa M. (2022). Felids: Federated learning-based intrusion detection system for agricultural internet of things. J. Parallel Distributed Computing 165, 17–31. doi: 10.1016/j.jpdc.2022.03.003
Gächter S., Herrmann B., Thöni C. (2004). Trust, voluntary cooperation, and socio-economic background: survey and experimental evidence. J. Economic Behav. Organ. 55, 505–531. doi: 10.1016/j.jebo.2003.11.006
Gai K., Qiu M., Zhao H., Xiong J. (2016). “Privacy-aware adaptive data encryption strategy of big data in cloud computing,” in 2016 IEEE 3rd international conference on cyber security and cloud computing (CSCloud) (Beijing, China: IEEE), 273–278. doi: 10.1109/CSCloud.2016.52
Gao T., Emadi H., Saha H., Zhang J., Lofquist A., Singh A., et al. (2018). A novel multirobot system for plant phenotyping. Robotics 7, 61. doi: 10.3390/robotics7040061
García-Tejero I., Gutiérrez-Gordillo S., Ortega-Arévalo C., Iglesias-Contreras M., Moreno J., SouzaFerreira L., et al. (2018). Thermal imaging to monitor the crop-water status in almonds by using the non-water stress baselines. Scientia Hortic. 238, 91–97. doi: 10.1016/j.scienta.2018.04.045
Garrett K. A., Dobson A., Kroschel J., Natarajan B., Orlandini S., Tonnang H. E., et al. (2013). The effects of climate variability and the color of weather time series on agricultural diseases and pests, and on decisions for their management. Agric. For. Meteorology 170, 216–227. doi: 10.1016/j.agrformet.2012.04.018
Garrett K. A., Forbes G., Savary S., Skelsey P., Sparks A. H., Valdivia C., et al. (2011). Complexity in climate-change impacts: an analytical framework for effects mediated by plant disease. Plant Pathol. 60, 15–30. doi: 10.1111/j.1365-3059.2010.02409.x
Gelder B., Sklenar T., James D., Herzmann D., Cruse R., Gesch K., et al. (2018). The daily erosion project–daily estimates of water runoff, soil detachment, and erosion. Earth Surface Processes Landforms 43, 1105–1117. doi: 10.1002/esp.4286
Ghosal S., Blystone D., Singh A. K., Ganapathysubramanian B., Singh A., Sarkar S. (2018). An explainable deep machine vision framework for plant stress phenotyping. Proc. Natl. Acad. Sci. 115, 4613–4618. doi: 10.1073/pnas.1716999115
Ghosal S., Zheng B., Chapman S. C., Potgieter A. B., Jordan D. R., Wang X., et al. (2019). A weakly supervised deep learning framework for sorghum head detection and counting. Plant Phenomics 2019, 1525874. doi: 10.34133/2019/1525874
Gilles J. L., Valdivia C. (2009). Local forecast communication in the Altiplano. Bull. Am. Meteorological Soc. 90, 85–92. doi: 10.1175/2008BAMS2183.1
Glover J. L. (2010). Capital usage in adverse situations: Applying bourdieu’s theory of capital to family farm businesses. J. Family Economic Issues 31, 485–497. doi: 10.1007/s10834-010-9225-0
Guo W., Carroll M. E., Singh A., Swetnam T. L., Merchant N., Sarkar S., et al. (2021). Uas-based plant phenotyping for research and breeding applications. Plant Phenomics 2021. doi: 10.34133/2021/9840192
Guo T., Zhong W. (2015). “Design and implementation of the span greenhouse agriculture internet of things system,” in 2015 international conference on fluid power and mechatronics (FPM) (Harbin, China: IEEE), 398–401. doi: 10.1109/FPM.2015.7337148
Gupta A., Singh A. (2023). Agri-gnn: A novel genotypic-topological graph neural network framework built on graphsage for optimized yield prediction. arXiv preprint arXiv:2310.13037. doi: 10.48550/arXiv.2310.13037
Hao M., Li H., Xu G., Liu S., Yang H. (2019). “Towards efficient and privacy-preserving federated deep learning,” in ICC 2019–2019 IEEE international conference on communications (ICC) (New York City, New York: IEEE), 1–6. doi: 10.1109/ICC.2019.8761267
Hasan S., Heimerl K., Harrison K., Ali K., Roberts S., Sahai A., et al. (2014). “Gsm whitespaces: An opportunity for rural cellular service,” in Dynamic Spectrum Access Networks (DYSPAN), 2014 IEEE International Symposium on. Virginia, USA: IEEE. 271–282.
Hatton N., Sharda A., Schapaugh W., van der Merwe D. (2018). “Remote thermal infrared imaging for rapid screening of sudden death syndrome in soybean,” in 2018 is in American Society of Agricultural and Biological Engineers, Vol. 1. Michigan: ASABE.
He K., Zhang X., Ren S., Sun J. (2016). “Deep residual learning for image recognition,” in Proceedings of the IEEE conference on computer vision and pattern recognition. (New York: IEEE), 770–778.
Heimerl K., Brewer E. (2010). “The village base station,” in Proceedings of the 4th ACM workshop on networked systems for developing regions, vol. 14. (New York City, New York: ACM).
Herr A. W., Adak A., Carroll M. E., Elango D., Kar S., Li C., et al. (2023). Unoccupied aerial systems imagery for phenotyping in cotton, maize, soybean, and wheat breeding. Crop Sci. 63, 1722–1749. doi: 10.1002/csc2.21028
Holler J., Tsiatsis V., Mulligan C., Karnouskos S., Avesand S., Boyle D. (2014). Internet of things (New York City, New York: Academic Press).
Hu Z., Niu J., Ren T., Dai B., Li Q., Xu M., et al. (2022). “An efficient on-line computation offloading approach for large-scale mobile edge computing via deep reinforcement learning,” in IEEE Transactions on Services Computing (Special Issue on Edge AI As-a-Service). (New York: IEEE). doi: 10.1109/TSC.2021.3116280
Hussain S., Li B., Koushanfar F., Cammarota R. (2020). “Tinygarble2: Smart, efficient, and scalable yao’s garble circuit,” in Proceedings of the 2020 Workshop on Privacy-Preserving Machine Learning in Practice. New York, NY: Association for Computing Machinery, 65–67.
Jakku E., Taylor B., Fleming A., Mason C., Fielke S., Sounness C., et al. (2019). if they don’t tell us what they do with it, why would we trust them?“ trust, transparency and benefit-sharing in smart farming. NJAS-Wageningen J. Life Sci. 90, 100285. doi: 10.1016/j.njas.2018.11.002
Jiang Z., Balu A., Hegde C., Sarkar S. (2017). Collaborative deep learning in fixed topology networks. Adv. Neural Inf. Process. Syst. 30. doi: 10.48550/arXiv.1706.07880
Juvekar C., Vaikuntanathan V., Chandrakasan A. (2018). “{GAZELLE}: A low latency framework for secure neural network inference,” in 27th USENIX Security Symposium (USENIX Security 18). 1651–1669.
Kairouz P., McMahan H. B., Avent B., Bellet A., Bennis M., Bhagoji A. N., et al. (2021). Advances and open problems in federated learning. Foundations Trends® Mach. Learn. 14, 1–210. doi: 10.1561/2200000083
Kamilaris A., Kartakoullis A., Prenafeta-Boldú F. X. (2017). A review on the practice of big data analysis in agriculture. Comput. Electron. Agric. 143, 23–37. doi: 10.1016/j.compag.2017.09.037
Kar S., Nagasubramanian K., Elango D., Carroll M. E., Abel C. A., Nair A., et al. (2023). Selfsupervised learning improves classification of agriculturally important insect pests in plants. Plant Phenome J. 6, e20079. doi: 10.1002/ppj2.20079
Kar S., Nagasubramanian K., Elango D., Nair A., Mueller D. S., O’Neal M. E., et al. (2021). “Self-supervised learning improves agricultural pest classification,” in AI for agriculture and food systems. In 2022 AAAI workshop on AI for agriculture and food systems. openreview.net, Amherst, Massachusetts. doi: 10.1002/essoar.10508841.1
Katung E., Akankwasa K. (2008). Community-based organizations and their effect on the adoption of agricultural technologies in Uganda: A study of Banana (Musa spp.) Pest management technology. Acta Horticulturae (879), 719–726. doi: 10.17660/ActaHortic.2010.879.77
Khalil M., Qadir J., Onireti O., Imran M. A., Younis S. (2017). “Feasibility, architecture and cost considerations of using tvws for rural internet access in 5g,” in Innovations in Clouds, Internet and Networks (ICIN), 2017 20th Conference on. 23–30. (New York: IEEE), 23–30.
Konečnỳ J., McMahan H. B., Yu F. X., Richtárik P., Suresh A. T., Bacon D. (2016). Federated learning: Strategies for improving communication efficiency. arXiv preprint arXiv:1610.05492. doi: 10.48550/arXiv.1610.05492
Krause M. D., das Gracas Dias K. O., Singh A. K., Beavis W. D. (2022). Using large soybean historical data to study genotype by environment variation and identify mega-environments with the integration of genetic and non-genetic factors. bioRxiv. doi: 10.1101/2022.04.11.487885
Krishnan P., Patnam M. (2014). Neighbors and extension agents in Ethiopia: Who matters more for technology adoption? Am. J. Agric. Economics 96, 308–327. doi: 10.1093/ajae/aat017
Kumar P., Gupta G. P., Tripathi R. (2021). PEFL: Deep privacy-encoding-based federated learning framework for smart agriculture. IEEE Micro 42, 33–40. doi: 10.1109/MM.2021.3112476
Kumar A., Karandikar A., Naik G., Khaturia M., Saha S., Arora M., et al. (2016). Toward enabling broadband for a billion plus population with TV white spaces. IEEE Commun. Magazine 54, 28–34. doi: 10.1109/MCOM.2016.7509375
Kumari P., Mishra R., Gupta H. P., Dutta T., Das S. K. (2022). An energy efficient smart metering system using edge computing in LoRa network. IEEE Trans. Sustain. Computing (Special Issue Energy-Efficient Edge Computing). 7, 786–798. doi: 10.1109/TSUSC.2021.3049705
Kummu M., Heino M., Taka M., Varis O., Viviroli D. (2021). Climate change risks pushing one-third of global food production outside the safe climatic space. One Earth 4, 720–729. doi: 10.1016/j.oneear.2021.04.017
Kunisch M. (2016). Big data in agriculture–perspectives for a service organization. Landtechnik 71, 1–3. doi: 10.15150/lt.2016.3117
Kyveryga P. M. (2019). On-farm research: Experimental approaches, analytical frameworks, case studies, and impact. Agron. J. 111, 2633–2635. doi: 10.2134/agronj2019.11.0001
Kyveryga P. M., Mueller T. A., Mueller D. S. (2018). On-farm replicated strip trials Vol. 13 (Hoboken, New Jersey: John Wiley & Sons, Ltd), 189–207. doi: 10.2134/precisionagbasics.2016.0096
Laurent A., Kyveryga P., Makowski D., Miguez F. (2019). A framework for visualization and analysis of agronomic field trials from on-farm research networks. Agron. J. 111, 2712–2723. doi: 10.2134/agronj2019.02.0135
Leonard T., Croson R. T., de Oliveira A. C. (2010). Social capital and public goods. J. Socio-Economics 39, 474–481. doi: 10.1016/j.socec.2010.02.009
Li K. (2019). Computation offloading strategy optimization with multiple heterogeneous servers in mobile edge computing. IEEE Trans. Sustain. Computing, 1–1. doi: 10.1109/TSUSC.2019.2904680
Li Q., Wen Z., Wu Z., Hu S., Wang N., Li Y., et al. (2021). A survey on federated learning systems: vision, hype and reality for data privacy and protection. IEEE Trans. Knowledge Data Eng. 35, 3347–3366. doi: 10.1109/TSUSC.2019.2904680
Liang Y.-C., Hoang A. T., Chen H.-H. (2008). Cognitive radio on tv bands: a new approach to provide wireless connectivity for rural areas. IEEE Wireless Commun. 15, 16–22. doi: 10.1109/MWC.2008.4547518
Liu E. M. (2013). Time to change what to sow: Risk preferences and technology adoption decisions of cotton farmers in China. Rev. Economics Stat 95, 1386–1403. doi: 10.1162/REST_a_00295
Liu B., Ding M., Shaham S., Rahayu W., Farokhi F., Lin Z. (2021). When machine learning meets privacy: A survey and outlook. ACM Computing Surveys (CSUR) 54, 1–36. doi: 10.1145/3436755
LoPiccalo K. (2021). Impact of broadband penetration on U.S. farm productivity. OEA Working Paper 50 Office Economics Anal. FCC. doi: 10.2139/ssrn.3790850
Lottes P., Khanna R., Pfeifer J., Siegwart R., Stachniss C. (2017). “Uav-based crop and weed classification for smart farming,” in 2017 IEEE international conference on robotics and automation (ICRA) (Amsterdam: IEEE), 3024–3031. doi: 10.1109/ICRA.2017.7989347
Lowenberg-DeBoer J., Huang I. Y., Grigoriadis V., Blackmore S. (2020). Economics of robots and automation in field crop production. Precis. Agric. 21, 278–299. doi: 10.1007/s11119-019-09667-5
Lucas J. R.E. (1988). On the mechanics of economic development. J. monetary economics 22, 3–42. doi: 10.1016/0304-3932(88)90168-7
Luo T. T., Huang J., Kanhere S. S., Zhang J., Das S. K. (2019). Improving iot data quality in mobile crowdsensing: A cross validation approach. IEEE Internet Things J. 6, 5651–5664. doi: 10.1109/JIoT.6488907
Mahato A. (2014). Climate change and its impact on agriculture. Int. J. Sci. Res. Publications 4, 1–6.
Mahmud M. S., Zahid A., Das A. K., Muzammil M., Khan M. U. (2021). A systematic literature review on deep learning applications for precision cattle farming. Comput. Electron. Agric. 187, 106313. doi: 10.1016/j.compag.2021.106313
Mandal K., Gong G. (2019). “PrivFL: Practical privacy-preserving federated regressions on highdimensional data over mobile networks,” in Proceedings of the 2019 ACM SIGSAC Conference on Cloud Computing Security Workshop. (New York, NY: Association for Computing Machinery), 57–68.
Manoj T., Makkithaya K., Narendra V. (2022). “A federated learning-based crop yield prediction for agricultural production risk management,” in 2022 IEEE delhi section conference (DELCON) (New Delhi, India: IEEE), 1–7.
Manyika J., Chui M., Brown B., Bughin J., Dobbs R., Roxburgh C., et al. (2011). Big data: The next frontier for innovation, competition, and productivity (New York City, New York: McKinsey Global Institute).
Marra M., Pannell D. J., Ghadim A. A. (2003). The economics of risk, uncertainty and learning in the adoption of new agricultural technologies: where are we on the learning curve? Agric. Syst. 75, 215–234. doi: 10.1016/S0308-521X(02)00066-5
McMahan B., Moore E., Ramage D., Hampson S., Arcas B. A. y. (2017). “Communication-efficient learning of deep networks from decentralized data,” in Proceedings of the 20th International Conference on Artificial Intelligence and Statistics, (AISTATS) 2017 (Fort Lauderdale, Florida, USA: PMLR) 54, 1273–1282.
Mehendale H., Paranjpe A., Vempala S. (2011). Lifenet: a flexible ad hoc networking solution for transient environments Vol. 41 (New York City, New York: ACM). doi: 10.1145/2018436
Mishra P., Lehmkuhl R., Srinivasan A., Zheng W., Popa R. A. (2020). “Delphi: A cryptographic inference service for neural networks,” in 29th USENIX Security Symposium (USENIX Security 20). 2505–2522.
Moore K. M. (2008). Network framing of pest management knowledge and practice. Rural Sociology 73, 414–439. doi: 10.1526/003601108785766552
Mwangi M., Kariuki S. (2015). Factors determining adoption of new agricultural technology by smallholder farmers in developing countries. J. Economics Sustain. Dev. 6 (5), 208–217.
Nadiradze G., Sabour A., Davies P., Li S., Alistarh D. (2021). Asynchronous decentralized sgd with quantized and local updates. Adv. Neural Inf. Process. Syst. 34, 6829–6842.
Nagasubramanian K., Jones S., Sarkar S., Singh A. K., Singh A., Ganapathysubramanian B. (2018). Hyperspectral band selection using genetic algorithm and support vector machines for early identification of charcoal rot disease in soybean stems. Plant Methods 14, 1–13. doi: 10.1186/s13007-018-0349-9
Nagasubramanian K., Jones S., Singh A. K., Sarkar S., Singh A., Ganapathysubramanian B. (2019). Plant disease identification using explainable 3d deep learning on hyperspectral images. Plant Methods 15, 98. doi: 10.1186/s13007-019-0479-8
Naik H. S., Zhang J., Lofquist A., Assefa T., Sarkar S., Ackerman D., et al. (2017). A real-time phenotyping framework using machine learning for plant stress severity rating in soybean. Plant Methods 13, 23. doi: 10.1186/s13007-017-0173-7
Nguyen D. C., Ding M., Pathirana P. N., Seneviratne A., Li J., Poor H. V. (2021). Federated learning for internet of things: A comprehensive survey. IEEE Commun. Surveys Tutorials 23, 1622–1658. doi: 10.1109/COMST.2021.3075439
Obunyali C. O., Karanja J., Oikeh S. O., Omanya G., Mugo S., Beyene Y., et al. (2019). On-farm performance and farmers’ perceptions of droughttego-climate-smart maize hybrids in Kenya. Agron. J. 111 (6), 2754–2768. doi: 10.2134/agronj2019.08.0600
Oreszczyn S., Lane A., Carr S. (2010). The role of networks of practice and webs of influencers on farmers’ engagement with and learning about agricultural innovations. J. Rural Stud. 26, 404–417. doi: 10.1016/j.jrurstud.2010.03.003
Ostrom E. (2009). A general framework for analyzing sustainability of social-ecological systems. Science 325, 419–422. doi: 10.1126/science.1172133
Parmley K. A., Higgins R. H., Ganapathysubramanian B., Sarkar S., Singh A. K. (2019b). Machine learning approach for prescriptive plant breeding. Sci. Rep. 9, 1–12. doi: 10.1038/s41598-019-53451-4
Parmley K., Nagasubramanian K., Sarkar S., Ganapathysubramanian B., Singh A. K. (2019a). Development of optimized phenomic predictors for efficient plant breeding decisions using phenomicassisted selection in soybean. Plant Phenomics 2019, 5809404. doi: 10.34133/2019/5809404
Patil K., Kale N. (2016). “A model for smart agriculture using iot,” in 2016 international conference on global trends in signal processing, information computing and communication (ICGTSPICC). (New York: IEEE), 543–545.
Patt A., Suarez P., Gwata C. (2005). Effects of seasonal climate forecasts and participatory workshops among subsistence farmers in Zimbabwe. Proc. Natl. Acad. Sci. 102, 12623–12628. doi: 10.1073/pnas.0506125102
Pentland A., Fletcher R., Hasson A. (2004). Daknet: Rethinking connectivity in developing nations. Computer 37, 78–83. doi: 10.1109/MC.2004.1260729
Perkel J. M. (2017). The internet of things comes to the lab. Nature 542, 125–126. doi: 10.1038/542125a
Phong L. T., Aono Y., Hayashi T., Wang L., Moriai S. (2017). Privacy-preserving deep learning via additively homomorphic encryption. IEEE Trans. Inf. Forensics Secur. 13, 1333–1345. doi: 10.1109/TIFS.2017.2787987
Raedeke A. H., Green J. J., Hodge S. S., Valdivia C. (2003). Farmers, the practice of farming and the future of agroforestry: an application of bourdieu’s concepts of field and habitus. Rural Sociology 68, 64–86. doi: 10.1111/j.1549-0831.2003.tb00129.x
Rairdin A., Fotouhi F., Zhang J., Mueller D. S., Ganapathysubramanian B., Singh A. K., et al. (2022). Deep learning-based phenotyping for genome wide association studies of sudden death syndrome in soybean. Front. Plant Sci. 13. doi: 10.3389/fpls.2022.966244
Riera L. G., Carroll M. E., Zhang Z., Shook J. M., Ghosal S., Gao T., et al. (2021). Deep multiview image fusion for soybean yield estimation in breeding applications. Plant Phenomics 2021. doi: 10.34133/2021/9846470
Rosenberg N., Nathan R. (1982). Inside the black box: technology and economics (Cambridge, United Kingdom: Cambridge University Press). doi: 10.1017/CBO9780511611940
Roy S., Ghosh N., Ghosh P., Das S. K. (2021). bioMCS 2.0: A distributed, energy-aware fog-based framework for data forwarding in mobile crowdsensing. Pervasive Mobile Computing 73, 101381. doi: 10.1145/3369740.3369788
Saadati M., Balu A., Chiranjeevi S., Jubery T. Z., Singh A. K., Sarkar S., et al. (2023). Out-ofdistribution detection algorithms for robust insect classification. arXiv preprint arXiv:2305.01823. doi: 10.48550/arXiv.2305.01823
Saha R., Chakraborty A., Misra S., Das S. K., Chatterjee C. (2020). DLSense: Distributed learning-based smart virtual sensing for precision agriculture. IEEE Sensors J. 21, 17556–17563. doi: 10.1109/JSEN.2020.3048593
Saha S., Nandi S., Paul P. S., Shah V. K., Roy A., Das S. K. (2015). Designing delay constrained hybrid ad hoc network infrastructure for post-disaster communication. Ad Hoc Networks 25, 406–429. doi: 10.1016/j.adhoc.2014.08.009
Sakthipriya N. (2014). An effective method for crop monitoring using wireless sensor network. MiddleEast J. Sci. Res. 20, 1127–1132. doi: 10.5829/idosi.mejsr.2014.20.09.114152
Sankaran S., Quirós J. J., Miklas P. N. (2019). Unmanned aerial system and satellite-based high resolution imagery for high-throughput phenotyping in dry bean. Comput. Electron. Agric. 165, 104965. doi: 10.1016/j.compag.2019.104965
Saraswat S., Gupta H. P., Dutta T., Das S. K. (2020). Energy efficient data forwarding scheme in fog based ubiquitous system with deadline constraints. IEEE Trans. Network Service Manage. 17, 213–226. doi: 10.1109/TNSM.4275028
Sarkar S., Ganapathysubramanian B., Singh A., Fotouhi F., Kar S., Nagasubramanian K., et al. (2023). Cyber-agricultural systems for crop breeding and sustainable production. Trends Plant Sci. 29, 130–149. doi: 10.1016/j.tplants.2023.08.001
Satyanarayana G., Mazaruddin S. (2013). “Wireless sensor based remote monitoring system for agriculture using ZigBee and GPS,” in Conference on advances in communication and control systems, Vol. 3. (Paris: ATLANTIC Press), 237–241.
Shah V. K., Bhattacharjee S., Silvestri S., Das S. K. (2017). “Designing sustainable smart connected communities using dynamic spectrum access via band selection,” in Proceedings of the 4th ACM International Conference on Systems for Energy-Efficient Built Environments, Vol. 12, (New York: ACM).
Shah V. K., Bhattacharjee S., Silvestri S., Das S. K. (2018). Designing green communication systems for smart and connected communities via dynamic spectrum access. ACM Trans. Sensor Networks (TOSN) 14, 31. doi: 10.1145/3274284
Shanmuga Sundaram J. P., Du W., Zhao Z. (2020). A survey on LoRa networking: research problems, current solutions, and open issues. IEEE Commun. Surveys Tutorials 22, 371–388. doi: 10.1109/COMST.9739
Sheeder R. J., Lynne G. D. (2011). Empathy-conditioned conservation:“walking in the shoes of others“. as Conserv. farmer. Land Economics 87, 433–452. doi: 10.3368/le.87.3.433
Shendryk Y., Sofonia J., Garrard R., Rist Y., Skocaj D., Thorburn P. (2020). Fine-scale prediction of biomass and leaf nitrogen content in sugarcane using uav lidar and multispectral imaging. Int. J. Appl. Earth Observation Geoinformation 92, 102177. doi: 10.1016/j.jag.2020.102177
Shepardson D. (2020). enFCC awards $9.2 billion to deploy broadband to 5.2 million U.S. homes, businesses. Reuters.
Shook J., Gangopadhyay T., Wu L., Ganapathysubramanian B., Sarkar S., Singh A. K. (2021a). Crop yield prediction integrating genotype and weather variables using deep learning. PloS One 16, e0252402. doi: 10.1371/journal.pone.0252402
Shook J. M., Lourenco D., Singh A. K. (2021b). Patriot: A pipeline for tracing identity-by-descent for chromosome segments to improve genomic prediction in self-pollinating crop species. Front. Plant Sci. 12, 2095. doi: 10.3389/fpls.2021.676269
Shucksmith M. (1993). Farm household behaviour and the transition to post-productivism. J. Agric. economics 44, 466–478. doi: 10.1111/j.1477-9552.1993.tb00288.x
Shucksmith M., Herrmann V. (2002). Future changes in British agriculture: projecting divergent farm household behaviour. J. Agric. Economics 53, 37–50. doi: 10.1111/j.1477-9552.2002.tb00004.x
Singh A. K., Ganapathysubramanian B., Sarkar S., Singh A. (2018). Deep learning for plant stress phenotyping: trends and future perspectives. Trends Plant Sci. 23, 883–898. doi: 10.1016/j.tplants.2018.07.004
Singh A., Ganapathysubramanian B., Singh A. K., Sarkar S. (2016). Machine learning for high-throughput stress phenotyping in plants. Trends Plant Sci. 21, 110–124. doi: 10.1016/j.tplants.2015.10.015
Singh A., Jones S., Ganapathysubramanian B., Sarkar S., Mueller D., Sandhu K., et al. (2021). Challenges and opportunities in machine-augmented plant stress phenotyping. Trends Plant Sci. 26, 53–69. doi: 10.1016/j.tplants.2020.07.010
Sirojan T., Lu S., Phung B. T., Zhang D., Ambikairajah E. (2018). Sustainable deep learning at grid edge for real-time high impedance fault detection. IEEE Trans. Sustain. Computing 7, 1–1. doi: 10.1109/TSUSC.2018.2879960
Song M., Xin C., Zhao Y., Cheng X. (2012). Dynamic spectrum access: from cognitive radio to network radio. IEEE Wireless Commun. 19, 23–29. doi: 10.1109/MWC.2012.6155873
Streletskaya N. A., Bell S. D., Kecinski M., Li T., Banerjee S., Palm-Forster L. H., et al. (2020). Agricultural adoption and behavioral economics: Bridging the gap. Appl. Economic Perspect. Policy 42, 54–66. doi: 10.1002/aepp.13006
Surampudi P., Mohanty S. (2011). LTE-advanced in white space: A complementary technology. Radisys White Paper.
Tehrani R. H., Vahid S., Triantafyllopoulou D., Lee H., Moessner K. (2016). Licensed spectrum sharing schemes for mobile operators: A survey and outlook. IEEE Commun. Surveys Tutorials 18, 2591–2623. doi: 10.1109/COMST.2016.2583499
Tetila E. C., MaChado B. B., Belete N. A., Guimarães D. A., Pistori H. (2017). Identification of soybean foliar diseases using unmanned aerial vehicle images. IEEE Geosci. Remote Sens. Lett. 14, 2190–2194. doi: 10.1109/LGRS.2017.2743715
Teyssier S. (2012). Inequity and risk aversion in sequential public good games. Public Choice 151, 91–119. doi: 10.1007/s11127-010-9735-1
Thompson L. J., Glewen K. L., Elmore R. W., Rees J., Pokal S., Hitt B. D. (2019). Farmers as researchers: In-depth interviews to discern participant motivation and impact. Agron. J. 111, 2670–2680. doi: 10.2134/agronj2018.09.0626
Trevisan R., Pérez O., Schmitz N., Diers B., Martin N. (2020). High-throughput phenotyping of soybean maturity using time series UAV imagery and convolutional neural networks. Remote Sens. 12, 3617. doi: 10.3390/rs12213617
Turland M., Slade P. (2020). Farmers’ willingness to participate in a big data platform. Agribusiness 36, 20–36. doi: 10.1002/agr.21627
Valdivia C., Barbieri C., Gold M. A. (2012). Between forestry and farming: Policy and environmental implications of the barriers to agroforestry adoption. Can. J. Agric. Economics/Revue Can. d’agroeconomie 60, 155–175. doi: 10.1111/j.1744-7976.2012.01248.x
Valdivia C., Danda M. K., Sheikh D., James H. S., Gathaara V., Mbure G., et al. (2014). Using translational research to enhance farmers’ voice: a case study of the potential introduction of GM cassava in Kenya's coast. Agric. Hum. Values 31, 673–681. doi: 10.1007/s10460-014-9536-0
Valdivia C. B., Gold M. A., Barbieri C., Gao J., Flora C. B. (2021). Human and institutional dimensions of agroforestry. Pp 489-519, in Garrett H. E., Jose S., Gold M. A. (eds.) North American Agroforestry. 3rd Edition. (Madison, WI, USA: American Society of Agronomy-Crop Science Society of America-Soil Science Society of America (ASA-CSSA-SSSA)). doi: 10.1002/9780891183785.ch17
Valdivia C., Gold M., Zabek L., Arbuckle J., Flora C. (2009). Human and institutional dimensions of agroforestry. In: Garrett H. E. eds. North American Agroforestry: An Integrated Science and Practice. 2nd Edition. (Madison, WI: America Society of Agronomy Inc), 339–367. doi: 10.2134/2009.northamericanagroforestry.2ed.c13
Valdivia C., James H. S. Jr., Quiroz R. (2018). New technology, ethical tensions and the mediating role of translational research. In Ethical tensions from new technology: the case of agricultural biotechnology (pp. 162–172). (Wallingford UK: CAB International).
Wang X., Han Y., Leung V. C. M., Niyato D., Yan X., Chen X. (2020). Convergence of edge computing and deep learning: A comprehensive survey. IEEE Commun. Surveys Tutorials 22, 1–1. doi: 10.1109/COMST.9739
Weiss M., Jacob F., Duveiller G. (2020). Remote sensing for agricultural applications: A meta-review. Remote Sens. Environ. 236, 111402. doi: 10.1016/j.rse.2019.111402
Wiseman L., Sanderson J., Zhang A., Jakku E. (2019). Farmers and their data: An examination of farmers’ reluctance to share their data through the lens of the laws impacting smart farming. NJASWageningen J. Life Sci. 90, 100301. doi: 10.1016/j.njas.2019.04.007
Wolfert S., Ge L., Verdouw C., Bogaardt M.-J. (2017). Big data in smart farming–a review. Agric. Syst. 153, 69–80. doi: 10.1016/j.agsy.2017.01.023
Woolf S. H. (2008). The meaning of translational research and why it matters. Jama 299, 211–213. doi: 10.1001/jama.2007.26
Wysel M., Baker D., Billingsley W. (2021). Data sharing platforms: How value is created from agricultural data. Agric. Syst. 193, 103241. doi: 10.1016/j.agsy.2021.103241
Xu W., Chen P., Zhan Y., Chen S., Zhang L., Lan Y. (2021). Cotton yield estimation model based on machine learning using time series UAV remote sensing data. Int. J. Appl. Earth Observation Geoinformation 104, 102511. doi: 10.1016/j.jag.2021.102511
Xue D., Huang W. (2020). Smart agriculture wireless sensor routing protocol and node location algorithm based on internet of things technology. IEEE Sensors J. 21, 24967–24973. doi: 10.1109/JSEN.2020.3035651
Yager K., Valdivia C., Slayback D., Jimenez E., Meneses R. I., Palabral A., et al. (2019). Socioecological dimensions of Andean pastoral landscape change: bridging traditional ecological knowledge and satellite image analysis in Sajama national park, Bolivia. Regional Environ. Change 19, 1353–1369. doi: 10.1007/s10113-019-01466-y
Zhang J., Naik H. S., Assefa T., Sarkar S., Reddy R., Singh A., et al. (2017). Computer vision and machine learning for robust phenotyping in genome-wide studies. (London: Nature Portfolio) 7, 1–11. doi: 10.1038/srep44048
Zhao L., Wang Q., Zou Q., Zhang Y., Chen Y. (2019). Privacy-preserving collaborative deep learning with unreliable participants. IEEE Trans. Inf. Forensics Secur. 15, 1486–1500. doi: 10.1109/TIFS.10206
Zhu H., Wang R., Jin Y., Liang K., Ning J. (2021). Distributed additive encryption and quantization for privacy preserving federated deep learning. Neurocomputing 463, 309–327. doi: 10.1016/j.neucom.2021.08.062
Keywords: cyber-agricultural systems, IoT, data analytics, precision agriculture, edge computing, sensors, farmer networks, technology adoption
Citation: Singh AK, Balabaygloo BJ, Bekee B, Blair SW, Fey S, Fotouhi F, Gupta A, Jha A, Martinez-Palomares JC, Menke K, Prestholt A, Tanwar VK, Tao X, Vangala A, Carroll ME, Das SK, DePaula G, Kyveryga P, Sarkar S, Segovia M, Silvestri S and Valdivia C (2024) Smart connected farms and networked farmers to improve crop production, sustainability and profitability. Front. Agron. 6:1410829. doi: 10.3389/fagro.2024.1410829
Received: 01 April 2024; Accepted: 07 June 2024;
Published: 08 August 2024.
Edited by:
Francesco Morari, University of Padua, ItalyReviewed by:
Rajan Bhatt, Punjab Agricultural University, IndiaEvans Asenso, University of Ghana, Ghana
Copyright © 2024 Singh, Balabaygloo, Bekee, Blair, Fey, Fotouhi, Gupta, Jha, Martinez-Palomares, Menke, Prestholt, Tanwar, Tao, Vangala, Carroll, Das, DePaula, Kyveryga, Sarkar, Segovia, Silvestri and Valdivia. This is an open-access article distributed under the terms of the Creative Commons Attribution License (CC BY). The use, distribution or reproduction in other forums is permitted, provided the original author(s) and the copyright owner(s) are credited and that the original publication in this journal is cited, in accordance with accepted academic practice. No use, distribution or reproduction is permitted which does not comply with these terms.
*Correspondence: Asheesh K. Singh, c2luZ2hha0BpYXN0YXRlLmVkdQ==