- 1Agronomy Department, Universidade Estadual de Londrina (UEL), Londrina, Brazil
- 2Plant Breeding, Instituto de Desenvolvimento Rural do Paraná (IDR-Paraná), Londrina, Brazil
- 3GDM Seeds, Cambé, Brazil
- 4Department of Plant Science, Section of Crop and Ecosystem Sciences, University of California, Davis, Davis, CA, United States
Beans are highly important for food and nutritional security in several South America, Asia, and Africa countries. Exploiting this crop’s genetic diversity is essential for breeding programs’ success. The objective of this study was to identify genomic regions associated with total phenolic content (TPC), total flavonoid content (FLA), and antioxidant activity (DPPH) in common bean grains in a Mesoamerican bean diversity panel. Phenotypic data were collected from two locations (Research Stations of the Paraná Rural Development Institute IAPAR-EMATER – IDR-Paraná) in the municipalities of Londrina and Guarapuava, Paraná, Brazil, and were subjected to variance analysis, heritability estimation, as well as histogram, density distribution, and boxplot analyses to assess genetic progress for black and carioca cultivars. For the genotypic data, four multi-locus genome-wide association studies (GWAS) were conducted to detect significant QTNs for the target traits. All analyses were performed using the R software. Analysis of variance showed a significant effect of genotype and genotype × environment interaction (p<0.01) for all traits, while no significant effect was observed for the environment. Heritability ranged from 93.1% to 99.8%, indicating low influence of environmental variation. Significant correlations (p<0.01) were observed among the traits evaluated. After filtering the SNPs obtained via GBS, 25,011 high-quality SNPs were identified for the GWAS study. After removing SNPs with high linkage disequilibrium, 707 SNPs were retained for population structure analysis. In total, 147 unique QTNs were significant for the three biochemical traits studied (TPC, FLA, and DPPH), of which 41 were detected at least twice by one of the seven multi-locus methods used or in different environments analyzed (Londrina, Guarapuava, and LSmeans). Candidate genes linked to the biosynthesis of phenolic compounds and antioxidant activity were also identified, highlighting the genetic complexity of these traits.
1 Introduction
The common bean (Phaseolus vulgaris L.) is a cornerstone of human nutrition, serving as the most significant legume for direct human consumption globally. Rich in carbohydrates and proteins, common bean offer a balanced nutritional profile and a diverse array of amino acids that surpass that of cereals (Sá et al., 2020; Huertas et al., 2023). With an energy value of 340 calories per 100 grams, common beans can fulfill up to 35% of daily protein requirements while providing essential vitamins and dietary fiber. Furthermore, beans are a critical source of micronutrients, boosting iron (Fe) concentrations 4-10 times greater and zinc (Zn) concentrations 2-3 times higher than staple cereals like maize, wheat, and rice (Blair, 2013). Currently, bean cultivation spans approximately 36.79 million hectares globally, producing an estimated 28.34 million tons, cementing its role as a dietary staple, particularly across Latin America and Eastern and Southern Africa (FAOSTAT, 2024; Myers and Kmiecik, 2017).
Brazil stands out as one of the world’s leading producers and consumers of common beans. In 2023, the country produced approximately 3.04 million tons (CONAB: Companhia Nacional de Abastecimento, 2024), with an average consumption of 12.2 kilograms per person. The carioca commercial group is the preferred type, accounting for approximately 60% of the Brazilian consumer market, characterized by its cream-colored seed coat with brown stripes, followed by the black group, which occupies around 20% of the market (EMBRAPA, 2024).
Beyond their status as an essential source of proteins and micronutrients, common beans are also rich in bioactive phenolic compounds, which have been linked to a reduced risk of chronic diseases, including cardiovascular and neurodegenerative disorders, diabetes, and cancer (Rodríguez Madrera et al., 2020). The phenolic profile of common beans has been extensively characterized, with phenolic acids, such as p-coumaric, ferulic, and cinnamic acids, predominating in the cotyledons, while a diversity of flavonoids is concentrated in the seed coat (Rodríguez Madrera et al., 2021; Kajiwara et al., 2022; Campa et al., 2023).
Extensive genetic variability in the phenolic composition of common bean has been documented, highlighting opportunities for selecting promising parental lines in breeding programs (de Lima et al., 2014; Myers and Kmiecik, 2017; Rodríguez Madrera et al., 2020; Nogueira et al., 2021; Campa et al., 2023). Myers et al. (2019) assessed total phenolic content (TPC) in 149 snap bean accessions, revealing considerable variability among genotypes and identifying 11 quantitative trait nucleotides (QTNs) associated with TPC. Among these, seven candidate genes were linked to these QTNs, with five regulatory genes implicated in elevated phenolic content. Similarly, Campa et al. (2023) investigated the genetic basis of ten flavonoid compounds in a panel of 308 bean accessions, uncovering 31 significant QTNs. This analysis led to the identification of 58 candidate genes, including 31 previously characterized in the phenylpropanoid pathway and 27 newly proposed based on association studies and homology with anthocyanin-related genes from Arabidopsis thaliana.
Enhancing the concentration of phenolic compounds in commercial common bean cultivars is a key strategy for biofortification aimed at addressing malnutrition in developing nations where beans are a dietary staple, such as Brazil (Delfini et al., 2021b; Nogueira et al., 2021). In this study, we identified genomic regions associated with the accumulation of total phenols, flavonoids, and antioxidant activity in common bean grains using a Brazilian diversity panel enriched with Mesoamerican accessions adapted to tropical environments. Our findings provide valuable genetic insights to inform breeding programs that develop nutritionally enhanced beans with increased health benefits.
2 Materials and methods
2.1 Genetic material
The Mesoamerican common bean diversity panel (BDP) used in this study was developed to gather accessions adapted to tropical conditions, reflecting the diversity of Brazil’s domesticated Mesoamerican gene pool (Delfini et al., 2021a). This panel includes cultivars, landraces, and breeding lines, all conserved in the gene bank of the Instituto de Desenvolvimento Rural do Paraná IAPAR-EMATER (IDR-Paraná). This study analyzed a subset of the Brazilian diversity panel of common beans (BDP), including 178 Mesoamerican common bean accessions.
The experiments were conducted during the 2018 rainy season at the research stations of the IDR-Paraná in the municipalities of Londrina (23°22’8”S; 51°9’48” W) and Guarapuava (25°23’51”S; 51°32’36” W), located in the Paraná state, Brazil. The experimental design adopted was a randomized block design with four replications. Each plot consisted of four rows, 2.00 m in length, with 0.5 m spacing between rows and a density of 12 plants per linear meter. Pest, disease, and weed control was carried out according to crop recommendations. After physiological maturity, each experimental plot was harvested, and a sample of 100 g of grains without physical or insect damage and free of disease was taken. These samples were stored in a cold chamber at a temperature of 5.6°C and 33% humidity until laboratory analyses were performed.
2.2 Phenotyping for total phenolics, flavonoids, and DPPH
Samples of 50 g of grains from each experimental plot were ground in a mill (Perten 3100) to obtain a homogeneous powder using a 60-mesh sieve. The bean flour samples were packaged and stored at -18°C until analysis. The moisture content of the ground grains was determined by drying at 105 ± 3°C until constant weight, using triplicate repetitions of approximately 2 grams each, with the results expressed as a percentage (Brasil, 2009).
For the determination of total phenolic content (TPC), total flavonoid content (FLA), and antioxidant activity (DPPH), a metabolic extract was obtained following the methodology of González and Vázquez (2008), with minor modifications. The extract was prepared by extracting 1.0 g of fresh samples with 10 mL of 70% ethanol (v/v), homogenized in a blender (500 W) for 20 seconds, followed by centrifugation at 1013 x g (Excelsa 2 Fanem model 205N) for five minutes.
To determine TPC, 1.0 mL of the metabolic extract was mixed with 1.0 mL of methanol, 1.0 mL of 0.2 N Folin-Ciocalteu reagent, and 1.0 mL of 10% sodium carbonate (w/v). The mixture was allowed to rest for 30 minutes in the dark at room temperature (25°C). Subsequently, absorbance was measured at a wavelength of 765 nm using a Micronal spectrophotometer (AJX1600). Gallic acid was used as a standard at 10.0–100.0 mg L-1 concentrations, and results were expressed as mg of gallic acid equivalents per 100 g of sample (mg GAE 100 g-1) (Swain and Hillis, 1959).
FLA was determined by mixing 1.0 mL of the metabolic extract with 1.0 mL of 5.0% aluminum chloride (w/v) and 2.0 mL of methanol, allowing the mixture to rest for 30 minutes in the dark. Absorbance was then measured using a spectrophotometer (Micronal, AJX-1600) at 425 nm. Quercetin was used as a standard at concentrations of 50.0–500.0 mg L-1, and results were expressed as mg of quercetin equivalents (QE) per 100 g of sample (mg QE 100 g-1) (Lee et al., 1995).
For determining antioxidant capacity (DPPH) by scavenging the 2,2-diphenyl-1-picrylhydrazyl (DPPH•) radical, 50.0 μL of the metabolic extract was mixed with 1.0 mL of 100 mM acetate buffer (pH 5.5), 1.0 mL of methanol, and 0.5 mL of ethanolic DPPH solution (250.0 μM). The tubes were kept at room temperature in the dark for 15 minutes. The absorbance of the remaining DPPH• radical was measured using a spectrophotometer (Thermo-Genesys) at a wavelength of 517 nm. The calibration curve was prepared using Trolox (6-hydroxy-2,5,7,8-tetramethylchroman-2-carboxylic acid) as a standard, and results were expressed as μmol of Trolox equivalent antioxidant capacity (TEAC) per 100 g of fresh sample (Brand-Williams et al., 1995).
Phenotypic data were subjected to variance analysis, and heritability was subsequently determined. Histogram, density distribution, and boxplot graphs were generated for the groups of bean accessions evaluated, categorized by grain color (carioca, black, and colored) and genetic material type (cultivars, lines, and landraces). T-test was used to evaluate the difference between the bean groups. All analyses were performed using R software (R Core Team, 2024) (https://www.r-project.org), using the packages ggplot2 (Wickham and Wickham, 2016), FactoMineR (Lê et al., 2008), and cluster (Maechler, 2019) packages.
2.3 Genotyping and genome wide association study
The genotyping-by-sequencing (GBS) technique was used to obtain the SNPs. The methodology used, as well as the results of the population structure and linkage disequilibrium (LD) analyses, are detailed in a previous work (Delfini et al., 2021a). In summary, GBS was conducted using the restriction enzyme CviAII (Ariani et al., 2016) and the data were imputed using Beagle software version 5 (Browning et al., 2018), and only SNPs anchored to chromosomes in the common bean reference genome (Schmutz et al., 2014) were used.
A total of six multi-locus genome-wide association studies (GWAS) were implemented in the MLM.GUI 4.0 software (Wen et al., 2018) was used to detect significant QTNs for the target traits: mrMLM, FASTmrMLM, FASTmrEMMA, pLARmEB, pKWmEB, and ISIS-EM-BLASSO. Population structure (Q) and kinship matrix (K) were included in the model to reduce false positives and enhance analytical power. STRUCTURE results (K = 2) were used for Q, and a kinship matrix was calculated using the mrMLM.GUI 4.0 software. For all methods, default parameters were used, and a logarithmic odds score (LOD) ≥ 3 was used as the critical value for significant associations. The phenotypic data used for GWAS were the adjusted means from each of the two environments. To obtain more reliable results, only QTNs that showed repeatability (i.e., detected at least twice using different methods or in different environments) were considered significant and were used in searches for favorable alleles and candidate genes.
2.4 Identification of favorable alleles
For each QTN, all accessions in the panel were initially divided into two groups based on the QTN genotype, and alleles associated with a positive effect on the phenotype (i.e., those associated with an increase in the analyzed trait) were identified. Subsequently, the number of favorable alleles for each accession was determined, and the association between the accumulation of these superior alleles in the same accession and trait was verified. Boxplots were generated using R software for better visualization of the results.
2.5 Candidate gene search
The search for potential candidate genes focused on QTNs detected using multiple methods or environments. The search radius (physical distance) was limited according to the decline of half the LD corrected for population structure and relatedness (r2vs). Genes within the association region were identified based on the reference genome annotation of common bean Phaseolus vulgaris v.2, published on the Phytozome v10.3 website (http://phytozome.net). Subsequently, genes with putative functions related to the traits of interest were selected as candidate genes, as indicated by Gene Ontology (GO) annotation (http://www.geneontology.org/).
3 Results
3.1 Nutritional characterization
The analysis of variance showed significant effects for genotypes and genotype × environment interactions (p<0.01) for all traits evaluated, while no significant effect was observed for the environment (Table 1). The experimental coefficient of variation ranged from 3.67 to 6.40%, and heritability ranged from 93.1 to 99.8%, indicating a low influence of environmental variation. Significant correlations (p<0.01) were observed among traits, with values of 0.74 (TPC × FLA), 0.48 (TPC × DPPH), and 0.50 (FLA × DPPH).
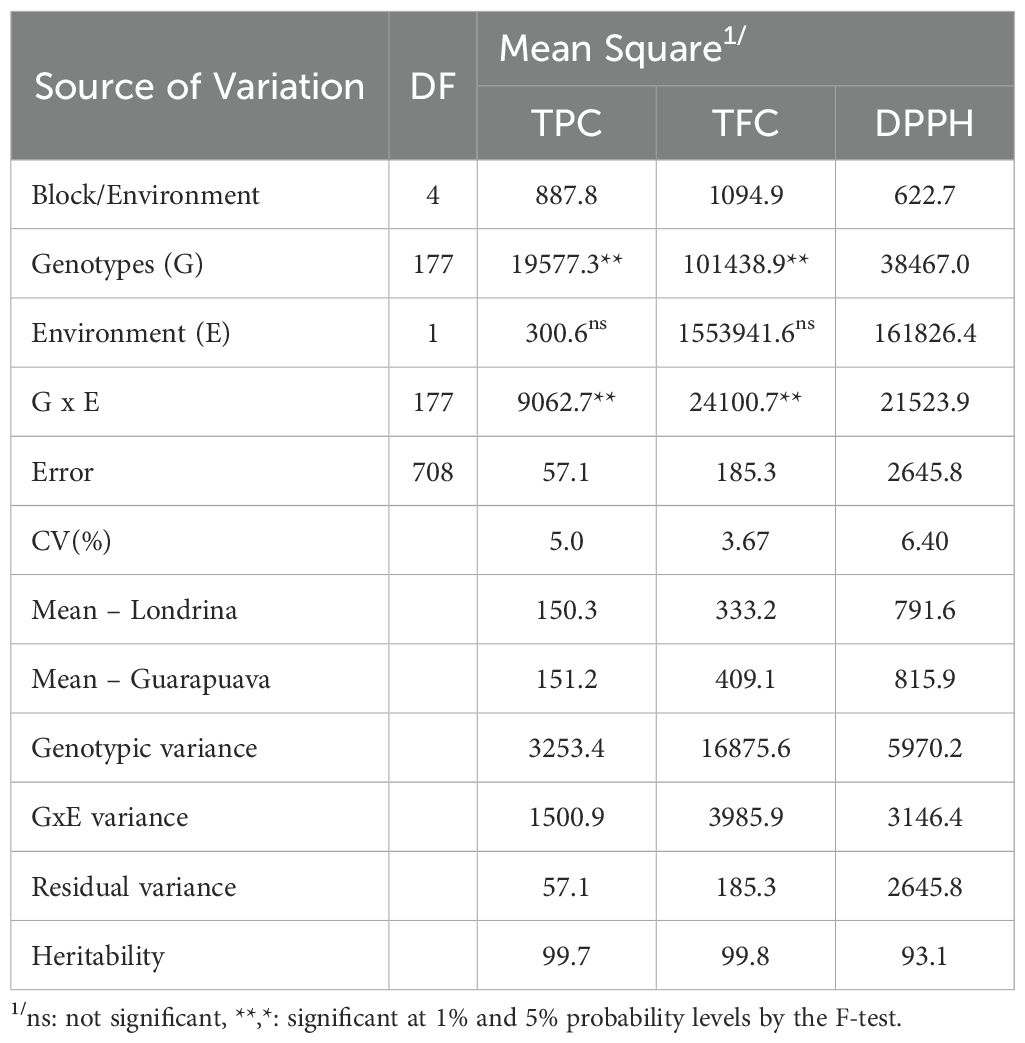
Table 1. Analysis of variance and genetic parameters for total phenols (TPC), flavonoids (TFC), and antioxidant activity (DPPH) detected in accessions from the Brazilian Diversity Panel (BDP) of Mesoamerican beans evaluated in two environments.
No differences were observed among the cultivar groups (cultivars, lines, and landraces) for TPC (Figure 1). Regarding the color groups (Carioca, black, and colored), the Carioca group had the highest average value (165.70 mg GAE 100 g-¹), followed by the colored and black types, with 154.31 and 132.67 mg GAE 100 g-¹, respectively. For the carioca group, the highest TPC values were found in the genotypes FEB200, FEB178, TAA Gol, Carioca1070, and LP07 (332.42, 290.17, 277.83, 249.90, and 249.36 mg GAE 100 g-¹, respectively), while in the black group, the highest values were found in BAT76, DOR445, ARC2, Awauna, and BlackHawk (354.36, 309.73, 272.58, 228.78, and 210.16 mg GAE 100 g-¹, respectively).
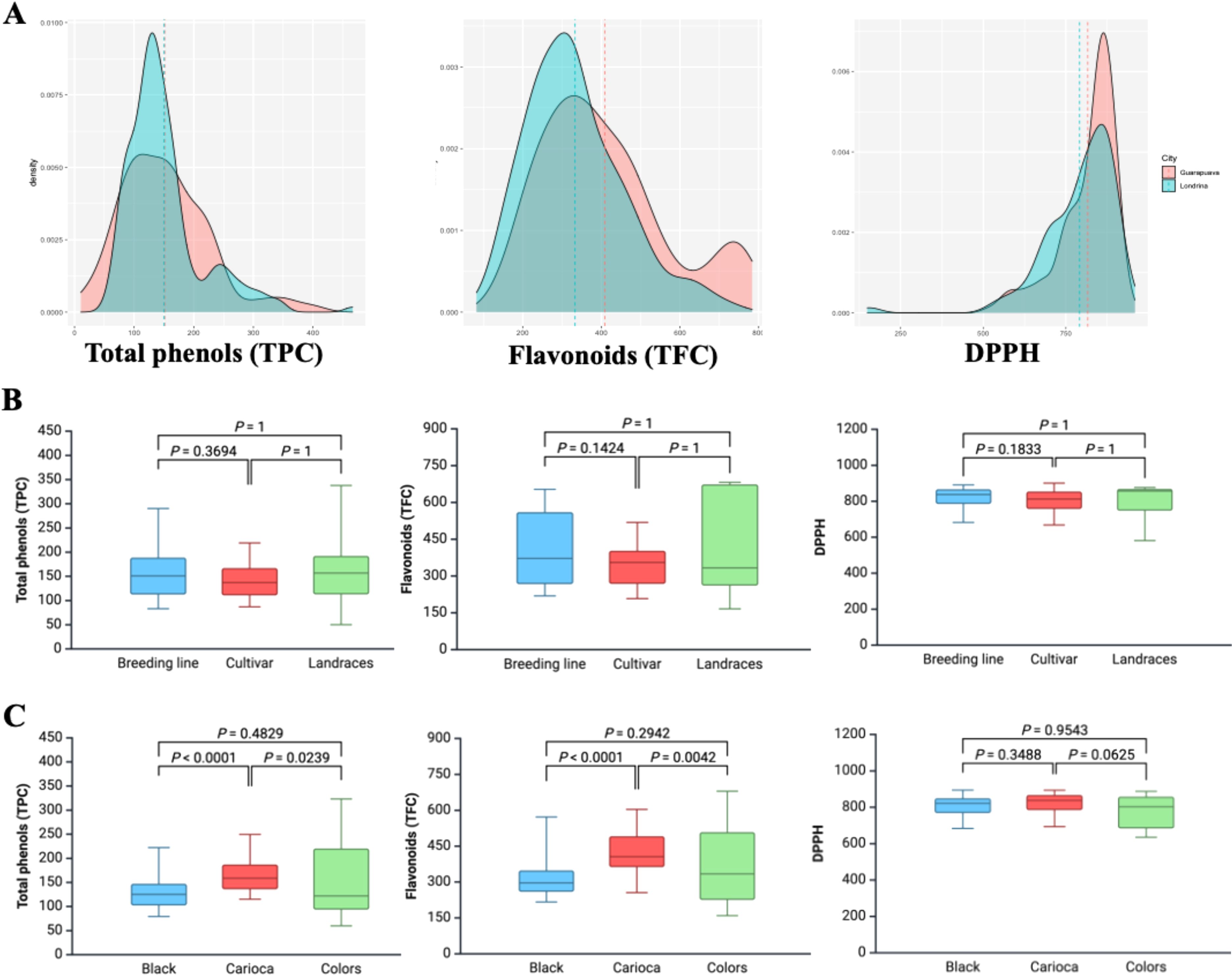
Figure 1. (A) density distribution, and boxplot for (B) genetic material and (C) seed colors for total phenols (TPC), flavonoids (TFC), and antioxidant activity (DPPH) detected in accessions from the Brazilian Diversity Panel (BDP) of Mesoamerican beans evaluated in two environments.
Total flavonoid content (FLA) ranged from 108.55 to 712.73 mg QE 100 g-¹, with no differences observed among the cultivar groups. The carioca group (422.18 mg QE 100 g-¹) had higher average FLA values than colored and black beans (371.47 and 315.57 mg QE 100 g-¹, respectively). In the carioca group, the highest FLA values were observed in the genotypes Carioca1070, LP15, FEB200, LP14, and LP13 (653.34, 626.26, 615.80, 606.14, and 603.07 mg QE 100 g-¹, respectively), while in the black group, they were DOR445, BAT76, BlackHawk, ARC2, and LP09 (687.66, 671.44, 602.26, 574.89, and 567.31 mg QE 100 g-¹, respectively).
The average value for antioxidant activity (DPPH) was 803.80 μmol TEAC 100 g-¹ (range: 444.91–944.34 μmol TEAC 100 g-¹). The average values for landraces, breeding lines, and cultivars were 788.11, 816.53, and 797.92 μmol TEAC 100 g-¹, respectively, and for the carioca, black, and colored grain groups, they were 813.75, 807.36, and 776.65 μmol TEAC 100 g-¹, respectively. The highest antioxidant activity values were observed in the carioca group genotypes BRS Ametista, Aporé, IAPAR 72, LP31, and FEB178 (936.41, 931.53, 893.61, 893.32, and 893.28 μmol TEAC 100 g-¹, respectively), and in the black group genotypes Diamante Negro, BRS Campeiro, BRS Esplendor, ICAQuetzal, and LP29 (944.34, 923.41, 903.60, 894.75, and 892.72 μmol TEAC 100 g-¹, respectively).
Linear regression analysis showed no significant effects (p>0.05) for all traits in both the carioca and black groups, indicating a lack of genetic progress (Figure 2). The coefficient of determination (R²) was 0.00 for most traits.
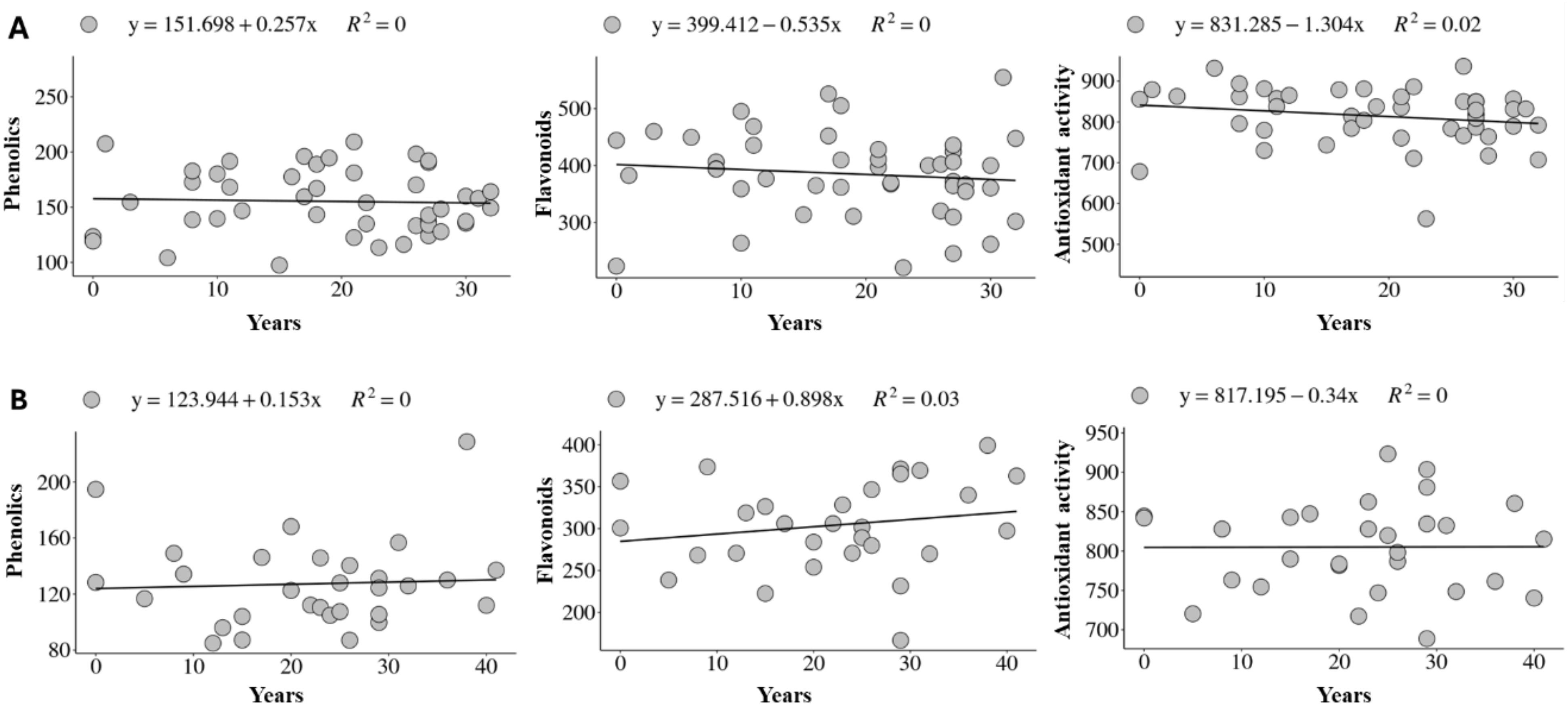
Figure 2. Genetic progress for total phenols, flavonoids, and antioxidant activity detected in accessions from the Brazilian Diversity Panel (BDP) of Mesoamerican beans evaluated in two environments for the carioca (A) and black (B) groups.
3.2 Genome-wide association study
In total, 147 unique QTNs were identified as significant for the three biochemical traits studied (TPC, FLA, and DPPH), with 41 of these QTNs being detected at least twice by one of the seven multi-locus methods used or in different environments analyzed (LDA, GUA, and LSmeans), as shown in Table 2 and Supplementary Figure S1. Table 2 presents the QTNs that demonstrated repeatability. The analyses revealed variation in the number of QTNs associated with each trait: 11 QTNs for TPC, 17 for FLA, and 13 for DPPH. One pleiotropic QTN was identified, showing a significant association at least twice for more than one trait, specifically shared between TPC and FLA. In addition to this pleiotropic QTN, seven overlapping QTNs were found within a genomic region defined by linkage disequilibrium (LD) of ± 296 kb. Two QTNs associated with FLA and DPPH were located on chromosome Pv2, where an overlap occurred. Other overlapping QTNs, all associated with DPPH, were identified on chromosome Pv06. Additionally, on chromosome Pv11, overlapping QTNs were observed for all three traits: TPC, FLA, and DPPH.
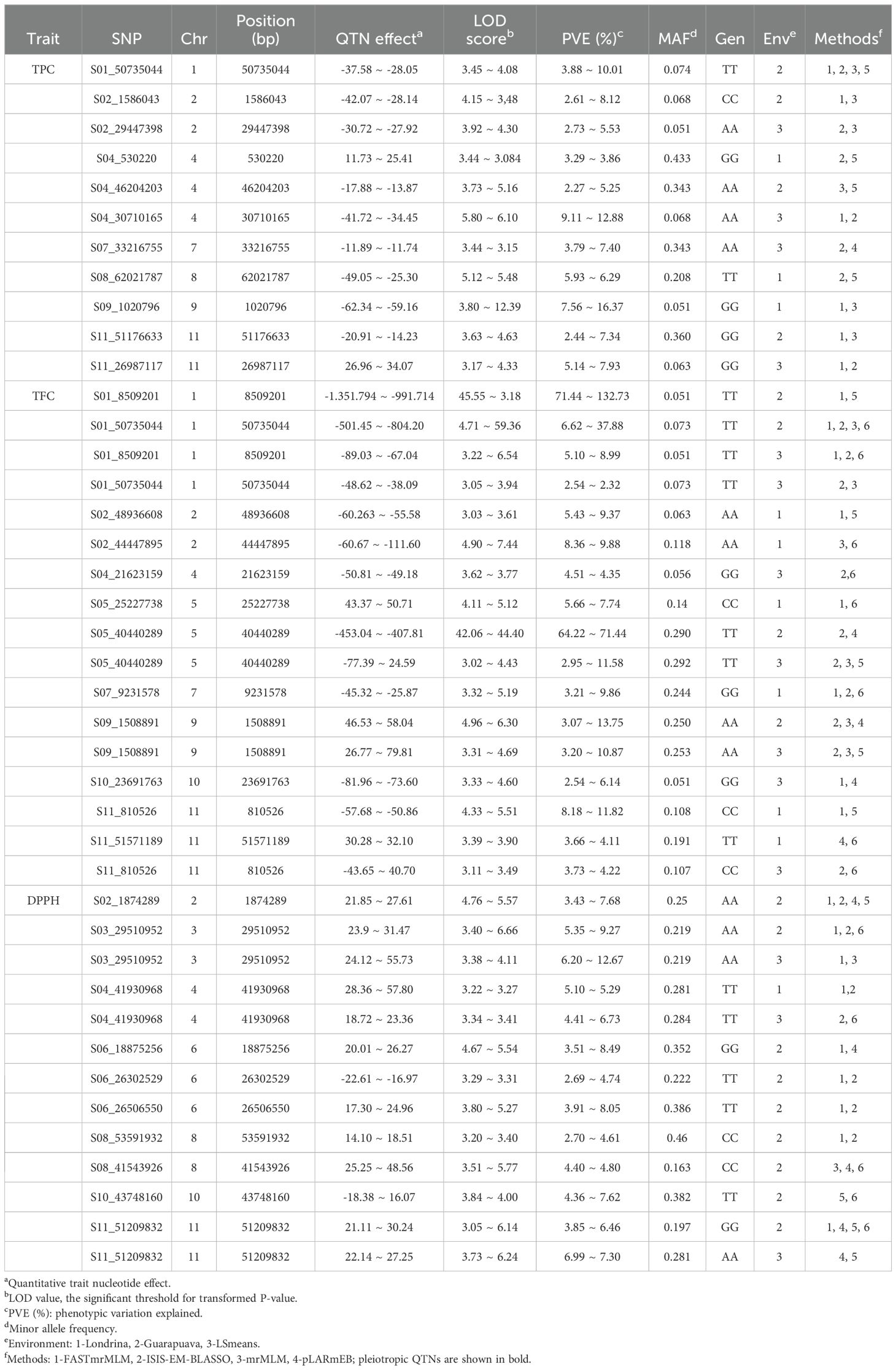
Table 2. QTNs associated with biochemical traits detected at least twice by different methods and in different environments in bean accessions from the Brazilian Diversity Panel (BDP).
QTNs were identified on all 11 common bean chromosomes, with chromosomes Pv02 and Pv11 having the highest number of QTNs, with five each. The phenotypic variation explained (PVE) by these QTNs varied significantly, ranging from very low values, close to 2%, to 16.37%. Although some QTNs showed negligible effects, the observed effects varied from -135.18 to 79.81.
Different PVE and QTN effect results were obtained for the same QTN using different methods, with more significant results for PVE. For instance, the FASTmrMLM method identified some QTNs with effects and PVE close to zero. ISIS EM-BLASSO identified the highest number of significant SNPs among the six methods used, followed by pLARmEB, FASTmrMLM, mrMLM, pKWmEB, and FASTmrEMMA. However, when focusing only on QTNs that demonstrated repeatability, the mrMLM and FASTmrMLM methods stood out, followed by FASTmrEMMA, ISIS EM-BLASSO, pKWmEB, and pLARmEB. The FASTmrMLM method achieved 99% efficiency, indicating that only 1% of the SNPs detected by this method did not show repeatability. In contrast, the mrMLM, ISIS-EM-BLASSO, and pLARmEB methods achieved efficiencies of 70, 52, and 10%, respectively. Although the ISIS EM-BLASSO method initially identified the highest number of SNPs, many of these SNPs were discarded in subsequent stages of the study.
The Guarapuava environment identified the highest initial number of significant SNPs, with 53, followed by Londrina and LSmeans, with 49 and 45, respectively. However, when considering the more stable SNPs, Guarapuava had the highest number, followed by LSmeans, while the Londrina environment had the lowest number of significant SNPs.
3.3 Favorable alleles
Favorable alleles that demonstrated repeatability were identified in each QTNs, meaning alleles were associated with increased biochemical content. Subsequently, an analysis was conducted to investigate whether the accumulation of these alleles corresponded to an increase in TPC, FLA, and DPPH content (Figure 3). The results indicated a progressive increase in TPC, FLA, and DPPH levels in bean grains, proportional to the number of favorable alleles in the genotype.
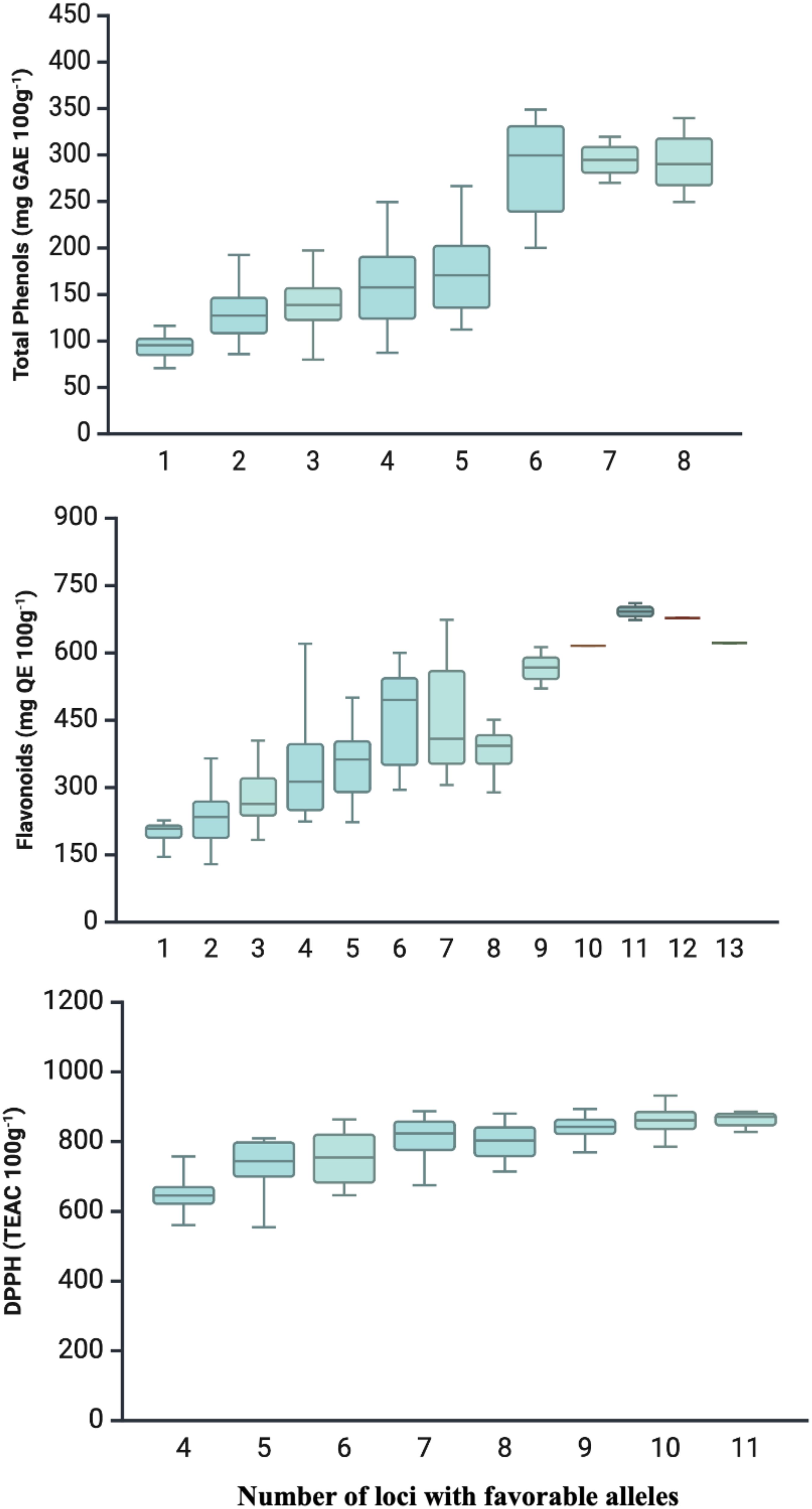
Figure 3. Accumulation of favorable alleles in relation to adjusted global means (LSmeans) for total phenols (mg GAE 100g-1), flavonoids (mg QE 100g-1), and antioxidant activity (DPPH) (80 mmol TEAC 100g-1) traits present in common bean accessions from the Brazilian Diversity Panel (BDP).
3.4 Candidate genes
Initially, genes in the genomic regions identified around the QTNs were searched. Some QTNs were located within the candidate genes, while others were at varying distances, ranging from 6.6 to 287 kbp (kilobase pairs) from the QTNs. This highlights the intricate and multifaceted nature of genetic regulation, emphasizing the need for further research and exploration to fully understand genetic associations.
The identified gene functions were associated with various processes, including redox reactions, protein phosphorylation, transcriptional regulation, metabolic processes, transmembrane transport, and metal ion transport, reflecting the diversity of biological functions of genes linked to QTNs. All the biochemical compounds studied had at least one candidate gene whose function was related to their composition, as indicated in Table 3. The highest number of candidate genes was found for DPPH, followed by FLA, with 8 and 2 candidate genes, respectively, while only one gene was associated with TPC.
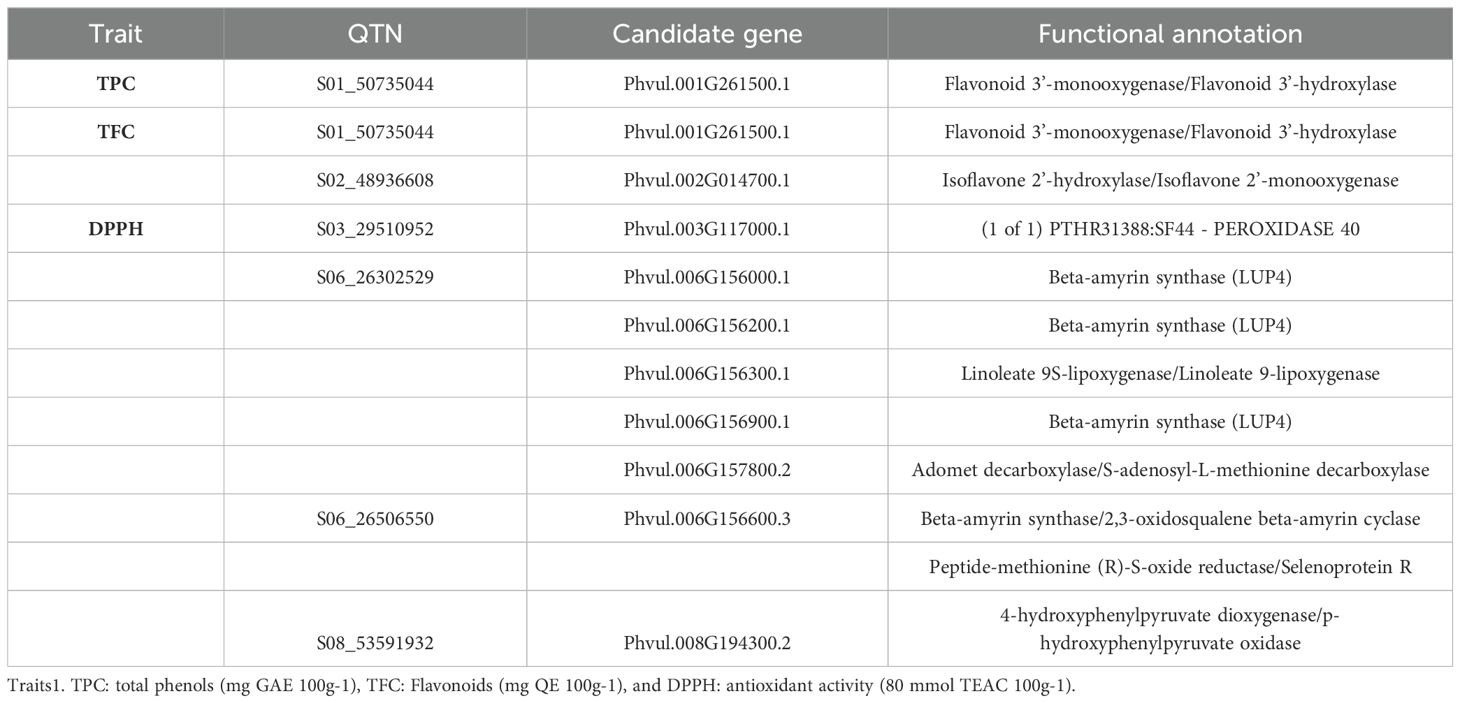
Table 3. List of potential candidate genes located in genomic regions underlying quantitative trait nucleotides (QTNs) associated with variation in nutritional content in common bean.
4 Discussion
Phenolics, defined by at least one phenol unit with one or more hydroxyl substituents, are essential secondary metabolites in plants, impacting plant physiology and human health. In plants, phenolics fulfill diverse functions, such as shielding against UV radiation, activating defense mechanisms against biotic and abiotic stresses, attracting pollinators, modulating hormones like auxins, and contributing to tissue pigmentation (Kołton et al., 2022). These compounds act as potent antioxidants for human health, reducing oxidative stress linked to degenerative diseases (Gan et al., 2017; Zeb, 2020). Common beans are notably rich in phenolic compounds, particularly in the seed coat, and their levels are influenced by both genetic (e.g., cultivar) and environmental (e.g., growing location) factors (Rocha-Guzmán et al., 2007; Rodríguez Madrera et al., 2020; Campa et al., 2023). Identifying genes or genomic regions involved in the biosynthesis of phenolic and antioxidant compounds in bean grains could significantly bolster breeding programs to develop cultivars with enhanced nutraceutical properties. In this study, we utilized a Brazilian diversity panel, including Mesoamerican varieties adapted to tropical conditions, to gain genetic insights that could facilitate the development of superior bean cultivars.
The broad variability in TPC, FLA and DPPH observed within the Brazilian common bean diversity panel underscores the potential for selecting genotypes enriched in bioactive compounds. This genetic diversity offers a resource for breeding improvement and an opportunity to identify genomic regions associated with these traits. The variability observed is consistent with findings from other studies involving diverse bean germplasms (Gan et al., 2017; Rodríguez Madrera et al., 2020, 2021; Márquez et al., 2024). Notably, the high heritability values for TPC, FLA, and DPPH suggest that these traits are primarily under genetic control, thus enhancing the likelihood of successful selection for bean lines with elevated concentrations of bioactive compounds. Ribeiro and Mezzomo (2020) similarly reported high heritability estimate for total phenolics in recombinant lines of Andean beans, further reinforcing the genetic determination of these traits.
Despite the high heritability values observed for bioactive compounds, there was a lack of genetic progress for these traits in the carioca and black bean groups. This can be partly attributed to the fact that these characteristics have not been the focus of breeding programs, coupled with a relatively narrow genetic base of Brazilian Mesoamerican beans. This potential trade-off between yield and nutraceutical properties underscores the need to incorporate specific evaluations of bioactive compounds into breeding programs to promote improvements in the nutritional quality of cultivars. In their study, Ribeiro and Maziero (2024) evaluated the genetic progress of Brazilian Mesoamerican bean cultivars for both macro- and micronutrients. They reported annual increases of 0.59, 5.39 and 3.39% for potassium, calcium, and magnesium, respectively, while gains for micronutrients were negative. These results underscore the need to integrate comprehensive nutrient evaluations into the bean breeding pipeline, thereby facilitating effective biofortification of cultivars.
The high correlation between TPC and FLA underscores the substantial contribution of flavonoids to the overall polyphenol composition in common beans. Furthermore, the positive correlations of both TPC and FLA with DPPH emphasize the role of these bioactive compounds in antioxidant defense mechanisms. These results align with findings from previous studies (Gan et al., 2017; Rodríguez Madrera et al., 2020; Nogueira et al., 2021). Interestingly, carioca group accessions showed higher TPC and FLA levels than the black group. However, Rodríguez Madrera et al. (2020), in their evaluation of the phenolic profile—including 12 hydroxycinnamic acids and derivatives, 13 anthocyanins, and 15 flavonols—across a panel of 220 common bean lines, found no clear relationship between phenolic composition and seed phenotype, indicating that phenolic diversity is not strictly linked to seed color traits.
In the study in question, among the ML-GWAS methods employed, ISIS EM-BLASSO stood out for identifying the highest number of significant SNPs compared to the other methods, which include pLARmEB, FASTmrMLM, mrMLM, pKWmEB, and FASTmrEMMA, in descending order of effectiveness. However, focusing on QTNs that demonstrated repeatability, the mrMLM and FASTmrMLM methods excelled. They were followed by FASTmrEMMA, ISIS EM-BLASSO, pKWmEB, and pLARmEB, respectively. These results align with other studies in the literature (Ma et al., 2018; Zhang et al., 2018; Fang et al., 2020; Delfini et al., 2021b). FASTmrMLM, an evolution of mrMLM, stands out for being faster, more reliable, having higher statistical power, greater estimation accuracy, and a low false-positive rate (Tamba and Zhang, 2018). This reliability of FASTmrMLM can instill confidence in its use. While ML-GWAS methods share similar approaches, the differential identification of QTNs reflects the distinct screening and estimation models of each (Lee et al., 2023). Based on the results, FASTmrMLM is considered the most reliable method due to its low rate of false-positive associations.
Several QTNs were detected at least twice, using different methods or across various environments, and are considered reliable. The recurrent detection of these QTNs confirms the robustness and complementarity of employing diverse methods and contexts. It also demonstrates that certain QTNs exhibit consistent behavior in distinct environments. Additionally, the combined application of multiple statistical methods offers the advantage of more easily identifying QTNs with minor effects, which are essential for traits with complex genetic bases, such as TPC and FLA (Sharma et al., 2020; Campa et al., 2023; Lee et al., 2023).
In addition to being important for gene identification, GWAS analyses can also facilitate the discovery of genomic regions associated with traits of interest. The information obtained through markers can be utilized in breeding programs to effectively integrate specific loci into elite germplasms (Ibrahim et al., 2020). The QTNs identified in this study may play a significant role in this process, as the accumulation of favorable alleles is linked to a progressive increase in the content of bioactive compounds and antioxidant activity.
The availability of genomic sequences and gene annotations for common beans has enabled the identification of genes located in genomic regions near the QTNs, potentially associated with bioactive compound content and antioxidant activity. For TPC and FLA, genes that play important roles in the biosynthesis of flavonoids and the modification of isoflavones, a subclass of flavonoids, were identified.
For antioxidant activity, the gene PTHR31388 gene plays a significant role in plant antioxidant and defense responses. The beta-amyrin synthase gene (LUP4) is involved in the biosynthesis of specific compounds called saponins. Although it does not directly act on antioxidant activity, the saponins derived from it may contribute to the plant’s antioxidant properties (Elekofehinti, 2015). The beta-amyrin synthase gene catalyzes the conversion of 2,3-oxidosqualene into beta-amyrin, a critical step in producing several secondary compounds, including saponins. The gene encoding linoleate 9S-lipoxygenase is a key enzyme in the plant oxylipin pathway. It catalyzes the oxidation of linoleic acid and other polyunsaturated fatty acids to form fatty acid hydroperoxides, which are intermediates in the biosynthesis of various bioactive compounds, including jasmonates. The adenosylmethionine decarboxylase gene plays a central role in the metabolism of polyamines, known for their antioxidant properties (Chen et al., 2019). Finally, the gene encoding the enzyme 4-hydroxyphenylpyruvate dioxygenase is critical in a key step of the catabolism of the amino acid tyrosine, leading to the formation of homogentisate. This pathway is crucial for tyrosine degradation and the biosynthesis of important compounds such as plastoquinone and tocopherol (vitamin E), both of which have significant antioxidant activity.
Nevertheless, while these candidate genes provide insights into the genetic control of bioactive compounds, functional validation remains to be performed. Future studies employing CRISPR gene editing or overexpression experiments, alongside integrated transcriptomic and proteomic analyses are essential to confirm these gene-trait association.
5 Conclusion
This study revealed genetic diversity in the Mesoamerican common bean diversity panel, providing opportunities for nutritional biofortification. High heritability values for TPC, FLA and DPPH suggest a strong genetic basis, beneficial for breeding programs targeting enhanced bioactive compounds. GWAS identified 147 unique QTNs, with 41 showing repeatability, making them reliable targets for selection. Candidate genes linked to the biosynthesis of phenolic compounds and antioxidant activity were also identified, highlighting the genetic complexity of these traits.
Data availability statement
The raw data supporting the conclusions of this article will be made available by the authors, without undue reservation.
Author contributions
AFN: Conceptualization, Formal Analysis, Investigation, Methodology, Validation, Writing – original draft. VM-C: Funding acquisition, Resources, Supervision, Writing – review & editing. JdSN: Investigation, Methodology, Resources, Writing – review & editing. JD: Investigation, Methodology, Writing – review & editing. DFVF: Investigation, Methodology, Writing – review & editing. PG: Writing – review & editing. LSAG: Conceptualization, Project administration, Supervision, Writing – original draft, Writing – review & editing.
Funding
The author(s) declare that financial support was received for the research and/or publication of this article.The authors thank CAPES (Coordination for the Improvement of Higher Education Personnel -financial code 01) for granting the master scholarship to the first author.
Acknowledgments
The authors thank CAPES (Coordination for the Improvement of Higher Education Personnel – financial code 01) for granting the master scholarship to the first author.
Conflict of interest
The authors declare that the research was conducted in theabsence of any commercial or financial relationships that could beconstrued as a potential conflict of interest.
Generative AI statement
The author(s) declare that no Generative AI was used in the creation of this manuscript.
Publisher’s note
All claims expressed in this article are solely those of the authors and do not necessarily represent those of their affiliated organizations, or those of the publisher, the editors and the reviewers. Any product that may be evaluated in this article, or claim that may be made by its manufacturer, is not guaranteed or endorsed by the publisher.
Supplementary material
The Supplementary Material for this article can be found online at: https://www.frontiersin.org/articles/10.3389/fagro.2025.1537871/full#supplementary-material
References
Ariani A., Berny Mier y Teran J. C., Gepts P. (2016). Genome-wide identification of SNPs and copy number variation in common bean (Phaseolus vulgaris L.) using genotyping-by-sequencing (GBS). Mol. Breed. 36, 87. doi: 10.1007/s11032-016-0512-9
Blair M. W. (2013). Mineral biofortification strategies for food staples: the example of common bean. J. Agric. Food Chem. 61, 8287–8294. doi: 10.1021/jf400774y
Brand-Williams W., Cuvelier M. E., Berset C. (1995). Use of a free radical method to evaluate antioxidant activity. LWT - Food Sci. Technol. 28, 25–30. doi: 10.1016/S0023-6438(95)80008-5
Browning B. L., Zhou Y., Browning S. R. (2018). A one-penny imputed genome from next-generation reference panels. Am. J. Hum. Genet. 103, 338–348. doi: 10.1016/j.ajhg.2018.07.015
Campa A., Rodríguez Madrera R., Jurado M., García-Fernández C., Suárez Valles B., Ferreira J. J. (2023). Genome-wide association study for the extractable phenolic profile and coat color of common bean seeds (Phaseolus vulgaris L.). BMC Plant Biol. 23, 158. doi: 10.1186/s12870-023-04177-z
Chen D., Shao Q., Yin L., Younis A., Zheng B. (2019). Polyamine function in plants: metabolism, regulation on development, and roles in abiotic stress responses. Front. Plant Sci. 9. doi: 10.3389/fpls.2018.01945
CONAB: Companhia Nacional de Abastecimento (2024). Acompanhamento da safra brasileira (CONAB), 9. Available at: https://www.conab.gov.br/info-agro/safras/graos (Accessed January 23, 2025).
Delfini J., Moda-Cirino V., dos Santos Neto J., Ruas P. M., Sant’Ana G. C., Gepts P., et al. (2021a). Population structure, genetic diversity and genomic selection signatures among a Brazilian common bean germplasm. Sci. Rep. 11, 2964. doi: 10.1038/s41598-021-82437-4
Delfini J., Moda-Cirino V., dos Santos Neto J., Zeffa D. M., Nogueira A. F., Ribeiro L. A. B., et al. (2021b). Genome-wide association study for grain mineral content in a Brazilian common bean diversity panel. Theor. Appl. Genet. 134, 2795–2811. doi: 10.1007/s00122-021-03859-2
de Lima P. F., Colombo C. A., Chiorato A. F., Yamaguchi L. F., Kato M. J., Carbonell S. A. M. (2014). Occurrence of isoflavonoids in Brazilian common bean germplasm (Phaseolus vulgaris L.). J. Agric. Food Chem. 62, 9699–9704. doi: 10.1021/jf5033312
Elekofehinti O. O. (2015). Saponins: Anti-diabetic principles from medicinal plants–A review. Pathophysiology 22, 95–103. doi: 10.1016/j.pathophys.2015.02.001
EMBRAPA (2024). Available online at: https://www.embrapa.br/arroz-e-feijao (Accessed January 23, 2025).
Fang Y., Liu S., Dong Q., Zhang K., Tian Z., Li X., et al. (2020). Linkage analysis and multi-locus genome-wide association studies identify QTNs controlling soybean plant height. Front. Plant Sci. 11. doi: 10.3389/fpls.2020.00009
FAOSTAT Production. (2024). Crop and livestock products. . Available online at: https://www.fao.org/faostat/en/#data/QCL (Accessed February 21, 2024).
Gan R., Wang M., Lui W., Wu K., Dai S., Sui Z., et al. (2017). Diversity in antioxidant capacity, phenolic contents, and flavonoid contents of 42 edible beans from China. Cereal Chem. 94, 291–297. doi: 10.1094/CCHEM-03-16-0061-R
González Á.A.L., Vázquez J. A. A. (2008). Un método para anticiparse al estrés laboral: el modelo de Siegrist (I). Gestión práctica riesgos laborales: Integración y desarrollo la gestión la prevención 46), 52–59.
Huertas R., Karpinska B., Ngala S., Mkandawire B., Maling’a J., Wajenkeche E., et al. (2023). Biofortification of common bean (Phaseolus vulgaris L.) with iron and zinc: Achievements and challenges. Food Energy Secur 12, e406. doi: 10.1002/fes3.406
Ibrahim A. K., Zhang L., Niyitanga S., Afzal M. Z., Xu Y., Zhang L., et al. (2020). Principles and approaches of association mapping in plant breeding. Trop. Plant Biol. 13, 212–224. doi: 10.1007/s12042-020-09261-4
Kajiwara V., Moda-Cirino V., dos Santos Scholz M. B. (2022). Studies on nutritional and functional properties of various genotypes of Andean beans. J. Food Sci. Technol. 59, 1468–1477. doi: 10.1007/s13197-021-05157-7
Kołton A., Długosz-Grochowska O., Wojciechowska R., Czaja M. (2022). Biosynthesis regulation of folates and phenols in plants. Sci. Hortic. 291, 110561. doi: 10.1016/j.scienta.2021.110561
Lê S., Josse J., Husson F. (2008). FactoMineR: an R package for multivariate analysis. J. Stat. software 25, 1–18. doi: 10.18637/jss.v025.i01
Lee Y., Howard L. R., Villalón B. (1995). Flavonoids and antioxidant activity of fresh pepper (Capsicum annuum) cultivars. J. Food Sci. 60, 473–476. doi: 10.1111/j.1365-2621.1995.tb09806.x
Lee Y.-J., Yang B., Kim W. J., Kim J., Kwon S.-J., Kim J. H., et al. (2023). Genome-wide association study (GWAS) of the agronomic traits and phenolic content in sorghum (Sorghum bicolor L.) genotypes. Agronomy 13, 1449. doi: 10.3390/agronomy13061449
Ma L., Liu M., Yan Y., Qing C., Zhang X., Zhang Y., et al. (2018). Genetic dissection of maize embryonic callus regenerative capacity using multi-locus genome-wide association studies. Front. Plant Sci. 9. doi: 10.3389/fpls.2018.00561
Maechler M. (2019). Finding groups in data: Cluster analysis extended Rousseeuw et al. R package version, Vol. 2. 242–248.
Márquez K., Arriagada O., Pérez-Díaz R., Cabeza R. A., Plaza A., Arévalo B., et al. (2024). Nutritional characterization of Chilean landraces of common bean. Plants 13, 817. doi: 10.3390/plants13060817
Myers J. R., Kmiecik K. (2017). “Common Bean: Economic Importance and Relevance to Biological Science Research,” in The Common Bean Genome, Eds. de la VegaMarta M., Santalla M., Marsolais F. (Cham: Springer), 1–20. doi: 10.1007/978-3-319-63526-2_1
Myers J. R., Wallace L. T., Mafi Moghaddam S., Kleintop A. E., Echeverria D., Thompson H. J., et al. (2019). Improving the health benefits of snap bean: genome-wide association studies of total phenolic content. Nutrients 11, 2509. doi: 10.3390/nu11102509
Nogueira A. F., Moda-Cirino V., Delfini J., Brandão L. A., Mian S., Constantino L. V., et al. (2021). Morpho-agronomic, biochemical and molecular analysis of genetic diversity in the Mesoamerican common bean panel. PloS One 16, e0249858. doi: 10.1371/journal.pone.0249858
R Core Team. (2024). R: A Language and Environment for Statistical Computing [Computer software manual]. Vienna, Austria. Available online at: http://www.R-project.org/ (Version 4.5.0).
Ribeiro N. D., Maziero S. M. (2024). Genetic gain for mineral concentration in common bean cultivars released by the Southern Brazilian Common-Bean Network. Euphytica 220, 29. doi: 10.1007/s10681-023-03287-x
Ribeiro N. D., Mezzomo H. C. (2020). Phenotypic parameters of macromineral and phenolic compound concentrations and selection of Andean bean lines with nutritional and functional properties. Ciec. e Agrotecnologia 44, e000320. doi: 10.1590/1413-7054202044000320
Rocha-Guzmán N. E., Herzog A., González-Laredo R. F., Ibarra-Pérez F. J., Zambrano-Galván G., Gallegos-Infante J. A. (2007). Antioxidant and antimutagenic activity of phenolic compounds in three different colour groups of common bean cultivars (Phaseolus vulgaris). Food Chem. 103, 521–527. doi: 10.1016/j.foodchem.2006.08.021
Rodríguez Madrera R., Campa Negrillo A., Suárez Valles B., Ferreira Fernández J. J. (2020). Characterization of extractable phenolic profile of common bean seeds (Phaseolus vulgaris L.) in a Spanish diversity panel. Food Res. Int. 138, 109713. doi: 10.1016/j.foodres.2020.109713
Rodríguez Madrera R., Campa Negrillo A., Suárez Valles B., Ferreira Fernández J. J. (2021). Phenolic content and antioxidant activity in seeds of common bean (Phaseolus vulgaris L.). Foods 10, 864. doi: 10.3390/foods10040864
Sá A. G. A., Moreno Y. M. F., Carciofi B. A. M. (2020). Plant proteins as high-quality nutritional source for human diet. Trends Food Sci. Technol. 97, 170–184. doi: 10.1016/j.tifs.2020.01.011
Schmutz J., McClean P. E., Mamidi S., Wu G. A., Cannon S. B., Grimwood J., et al. (2014). A reference genome for common bean and genome-wide analysis of dual domestications. Nat. Genet. 46, 707–713. doi: 10.1038/ng.3008
Sharma M., Rahim M. S., Kumar P., Mishra A., Sharma H., Roy J. (2020). Large-scale identification and characterization of phenolic compounds and their marker–trait association in wheat. Euphytica 216, 127. doi: 10.1007/s10681-020-02659-x
Swain T., Hillis W. E. (1959). The phenolic constituents of Prunus domestica. I.—The quantitative analysis of phenolic constituents. J. Sci. Food Agric. 10, 63–68. doi: 10.1002/jsfa.2740100110
Tamba C. L., Zhang Y.-M. (2018). A fast mrMLM algorithm for multi-locus genome-wide association studies. bioRxiv. doi: 10.1101/341784
Wen Y.-J., Zhang H., Ni Y.-L., Huang B., Zhang J., Feng J.-Y., et al. (2018). Methodological implementation of mixed linear models in multi-locus genome-wide association studies. Brief Bioinform. 19, 700–712. doi: 10.1093/bib/bbw145
Wickham H., Wickham H. (2016). “Programming with ggplot2,” in Ggplot2: elegant graphics for data analysis, 241–253.
Zeb A. (2020). Concept, mechanism, and applications of phenolic antioxidants in foods. J. Food Biochem. 44, e13394. doi: 10.1111/jfbc.13394
Keywords: gene bank, genetic breeding, Phaseolur vulgaris, genotyping by sequencing (GBS), biofortification
Citation: Nogueira AF, Moda-Cirino V, dos Santos Neto J, Delfini J, Fagundes DFV, Gepts P and Gonçalves LSA (2025) Association mapping for total phenols, total flavonoids and antioxidant activity in a Mesoamerican bean diversity panel. Front. Agron. 7:1537871. doi: 10.3389/fagro.2025.1537871
Received: 01 December 2024; Accepted: 21 March 2025;
Published: 28 April 2025.
Edited by:
Ahmed M. Abdelghany, Damanhour University, EgyptReviewed by:
Sohail Abbas, Henan University, ChinaAbdulwahab Saliu Shaibu, Bayero University Kano, Nigeria
Sobhi F. Lamlom, Alexandria University, Egypt
Copyright © 2025 Nogueira, Moda-Cirino, dos Santos Neto, Delfini, Fagundes, Gepts and Gonçalves. This is an open-access article distributed under the terms of the Creative Commons Attribution License (CC BY). The use, distribution or reproduction in other forums is permitted, provided the original author(s) and the copyright owner(s) are credited and that the original publication in this journal is cited, in accordance with accepted academic practice. No use, distribution or reproduction is permitted which does not comply with these terms.
*Correspondence: Leandro Simões Azeredo Gonçalves, bGVhbmRyb3NhZ0B1ZWwuYnI=