- Department of Neurology, The Affiliated Huai'an Hospital of Xuzhou Medical University and The Second People's Hospital of Huai'an, Huai'an, China
Background: Post-stroke cognitive impairment (PSCI) is one of the main complications after stroke. The association between the hemoglobin-to-red cell distribution width ratio (HRR) and PSCI remains inadequately explored. Consequently, we performed a prospective study to assess whether HRR levels are associated with changes in cognitive function after acute ischemic stroke (AIS).
Methods: A total of 296 AIS patients were recruited. HRR was measured within 24 h of admission, and cognitive function was assessed using the Mini-Mental State Examination (MMSE) one month post-onset. Logistic regression analysis was performed to identify independent risk and protective factors for the occurrence of PSCI. Restricted cubic splines (RCS) were used to explore the dose-response relationship between HRR and PSCI.
Results: 129 of 296 participants (43.6%) developed cognitive impairment at 1 month. HRR in PSCI group was significantly lower than that in non-cognitive impairment group (P < 0.001). When HRR was taken as the categorical variable and with Q4 as the reference, the risk of PSCI in Q1 was the highest after adjusting multiple potential confounding factors (odds ratio [OR] = 2.702, 95% confidence interval [CI]= 1.222–5.977, P = 0.014). In addition, RCS curve exhibited that the relationship between HRR and PSCI was linear (P for nonlinear = 0.972, P for overall = 0.012). Subgroup analysis verified the stability of the results.
Conclusion: Reduced HRR levels were linked to an increased risk of cognitive impairment, indicating that HRR may serve as a predictive factor for PSCI.
1 Introduction
Stroke continues to be the primary cause of disability (Ma et al., 2021). Post-stroke cognitive impairment (PSCI) is a prevalent post-stroke complication, impacting between 25% and 81% of patients (Sun et al., 2014). Previous studies have indicated that the presence of cognitive impairment is associated with poorer functional outcomes and a higher risk of recurrent stroke (Lee et al., 2014; Synhaeve et al., 2015), and that PSCI significantly affects patients' neurological recovery and survival time (Rost et al., 2022). Furthermore, stroke survivors will face heightened medical expenses due to the aftermath. Consequently, new predictive biomarkers are being explored to improve the identification and early diagnosis of PSCI, thereby potentially offering fresh perspectives on treatment strategies for PSCI.
Red blood cells are the most important medium for transporting oxygen in blood and are closely related to the occurrence and development of ischemic stroke (IS). Both anemia and low hemoglobin levels are believed to elevate the risk of stroke. Additionally, abnormal hemoglobin levels (anemia and high hemoglobin) are linked to increased risks of ischemic cerebrovascular disease, including all-cause mortality, poor functional outcomes, stroke recurrence, and complex vascular events (Zhang et al., 2021).
Red cell distribution width (RDW) is an important parameter that indicates variability in red blood cell volume and is accessible through routine blood tests. Clinically, RDW is commonly used in combination with other hematological indicators to diagnose blood disorders. Recent studies have shown that variations in RDW levels are also associated with the neuroendocrine system, chronic inflammation, oxidative stress, and other factors. RDW is associated with various diseases and serves as an independent and strong risk factor for mortality from these conditions (Wang et al., 2020; Ding et al., 2022; Liang et al., 2022). A cohort study of healthy individuals found that RDW levels showed a positive correlation with IS incidence and common carotid intima-media thickness. This relationship may be attributed to systemic inflammation, nutritional deficiencies, and increased renin-angiotensin system activation (Söderholm et al., 2015). Studies have shown that increased RDW can predict stroke severity and unfavorable functional outcomes in patients with IS (Kara et al., 2015; Xue et al., 2022). Similarly, elevated RDW levels were linked to a higher mortality risk following acute ischemic stroke (AIS) (Shen and Shen, 2024).
The hemoglobin-to-red cell distribution width ratio (HRR) serves as a novel biomarker for inflammation, calculated using hemoglobin and RDW. HRR levels exhibit a negative association with stroke incidence; each unit increase in HRR decreases the likelihood of a stroke by 58% (Xiong et al., 2024). The lower the level of HRR, the worse the functional prognosis at 3 months and the higher the mortality in IS patients, and this relationship is negative nonlinear (Qin et al., 2022; Xie et al., 2024). RDW may be associated with cognitive function and has been found to be elevated in individuals with Alzheimer's disease (AD) and vascular dementia (VaD) (Öztürk et al., 2013; Jiang et al., 2021). Likewise, the association between anemia and cognitive impairment is well-documented (Winchester et al., 2018). High level of hemoglobin at baseline may decrease the incidence of PSCI and serve as a protective factor for cognitive function (Jia et al., 2022).
However, studies exploring the relationship between HRR and PSCI remain insufficient. We hypothesize that HRR might influence cognitive dysfunction risk in AIS patients. AIS patients were recruited to have their HRR levels measured on admission and then cognitive assessment was performed one month after stroke to investigate the association between HRR and PSCI.
2 Materials and methods
2.1 Study design and population
A prospective cohort study was conducted in which patients with AIS were admitted to the Department of Neurology of the affiliated Huai'an Hospital of Xuzhou Medical University between January and October 2023. Patients who met the criteria were included, and cognitive assessment was performed using the Mini-Mental State Examination (MMSE) 1 month after stroke. Venous blood samples were taken within 24 h of admission to assess HRR and other indicators. Clinical data was also recorded during hospitalization for analysis.
The inclusion criteria were as follows: (1) Patients met the diagnostic criteria of AIS and were confirmed by computed tomography or magnetic resonance imaging at admission; (2) Time from onset to admission ≤ 7 days; (3) Age between 18 and 80 years old; (4) Have the ability and willingness to complete regular follow-up, voluntarily signed informed consent.
The exclusion criteria were as follows: (1) Combined with other serious central nervous system diseases, such as Parkinson's disease or hydrocephalus; (2) Significant cognitive dysfunction before stroke; (3) Patients with severe speech, hearing, and visual impairment, intolerance, or cooperation with relevant examinations of this study; (4) Patients with a history of depression, anxiety, schizophrenia or long-term use of psychotropic drugs; (5) Severe liver, kidney, lung dysfunction, acute and chronic inflammatory diseases and other acute vascular ischemic diseases including acute myocardial infarction; (6) Previous autoimmune diseases, malignant tumors, and hematological diseases.
2.2 Data collection
Demographic characteristics, vascular risk factors, clinical data, and laboratory data were collected after admission. Including gender, age, education, body mass index (BMI), hypertension, diabetes, coronary heart disease, atrial fibrillation, previous stroke, smoking, drinking, and anemia (defined as hemoglobin <130 g/L for males and <120 g/L for females). The National Institutes of Health Stroke Scale (NIHSS) was used to assess the degree of neurological deficits at admission. The causes of stroke were collected (TOAST mechanism), and white blood cell count (WBC), hemoglobin, RDW, platelet count, mean corpuscular volume (MCV), and glycated hemoglobin (HbA1c) were recorded. HbA1c levels were reported in the National Glycohemoglobin Standardization Program (NGSP) units (A1c). According to the formula developed by Hoelzel et al. (2004), we convert the A1c value to the International Federation of Clinical Chemistry and Laboratory Medicine (IFCC) units (iA1c). Finally, iA1c was put into the formula developed by Koga et al. (2023) to calculate the estimated mean erythrocyte age (Mrbc) (which is approximately half of the red blood cells lifespan). The calculation formula involved is as follows: HRR = hemoglobin (g/L)/RDW (%); iA1c (mmol/mol) = 10.93 × HbA1c_NGSP (%) – 23.53; Mrbc (days) = 1.45 × iA1c (mmol/mol).
2.3 Evaluation of cognitive function
The patients were followed up at 1 month after stroke, two professionally trained neurologists used the MMSE Beijing version to evaluate the patients in a quiet environment. MMSE score range from 0 to 30, with higher scores indicating better cognitive function. According to the MMSE score, participants were divided into PSCI group and post-stroke non-cognitive impairment (PSNCI) group. In this study, patients with an MMSE score ≤ 17 points (illiterate), ≤ 20 points (years of education ≤ 6) or ≤ 24 points (years of education > 6) were considered to have cognitive impairment (Liu et al., 2017). The cognitive assessment was conducted by two trained physicians and consistency testing was performed prior to the start of the study to ensure the reliability of the assessment (Simple KAPPA Coefficient: 0.898).
2.4 Statistical analysis
Participants were divided into quartiles according to their HRR levels upon admission: Quartile1 (≤9.70), Quartile2 (9.71–10.73), Quartile3 (10.74–11.71), and Quartile4 (≥11.72). Continuous variables were expressed as means with standard deviation (SD) or median with interquartile range (IQR). Categorical variables were described using frequencies and percentages. Differences in baseline characteristics in the patients with and without were compared using an independent sample t-test, Pearson Chi-square test, Fisher's exact test, one-way analysis of variance (Bonferroni post-hoc test), and a Kruskal–Wallis H-test. Binary logistic regression analysis was used to detect the relationship between HRR and PSCI, and the adjusted covariates were variables with a P < 0.05 in univariate analysis. We established three logistic regression models, model 1: unadjusted; model 2: adjusted for gender, age, and education; model 3: adjusted for covariates from model 2 and further adjusted for atrial fibrillation, previous stroke, NIHSS, white blood cell count, and platelet count. Spline regression models were used to explore the shape of the association between HRR and PSCI, fitting a restricted cubic spline function with 3 knots (at the 5th, 50th, and 95th percentiles). In addition, patients were divided into subgroups based on gender, age (<65; ≥65), hypertension, diabetes, coronary heart disease, atrial fibrillation, smoking, drinking, anemia, and previous stroke. Subgroup analysis was used to verify the stability of the results. Correlation analyses were performed using Pearson correlation or Spearman rank-order correlation.
All the research data were analyzed by the SPSS (version 25.0), and R software (version 4.3.2). Two-tailed P < 0.05 was considered statistically significant.
3 Results
3.1 Study recruitment profile
A total of 511 AIS patients were enrolled at baseline and 339 met the inclusion criteria. During the 1-month follow-up, 1 patient died, and 42 patients were lost to follow-up due to missing data. Finally, 296 patients completed the follow-up and were included in the statistical analysis, and 129 patients were considered to have cognitive impairment. The incidence of PSCI in this study was 43.6% (Figure 1).
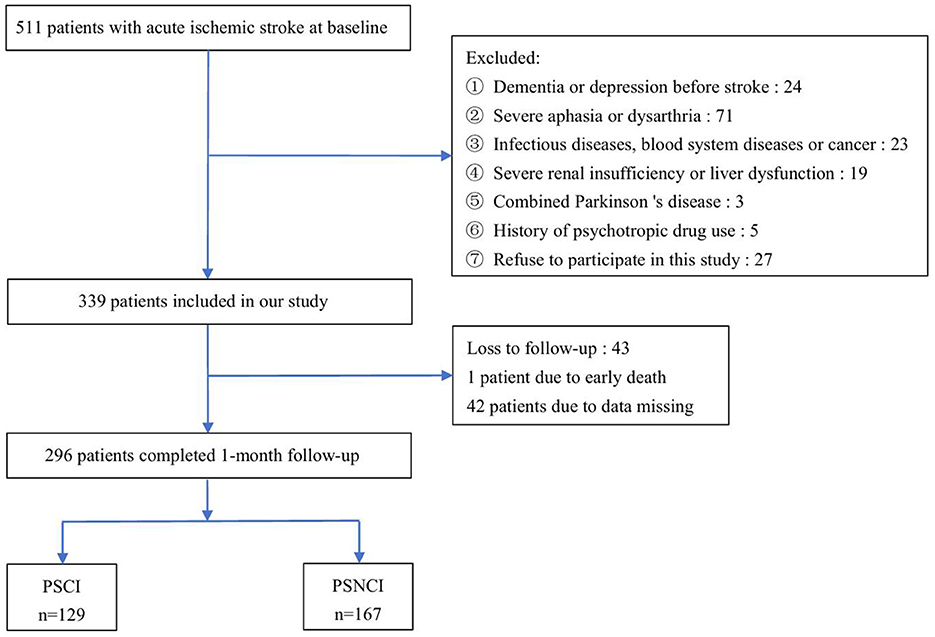
Figure 1. Study recruitment profile. PSCI, post-stroke cognitive impairment; PSNCI, post-stroke non-cognitive impairment.
3.2 Baseline characteristics of patients with PSCI and PSNCI
Table 1 shows the comparison of general baseline data between the PSCI group and the PSNCI group, and the HRR of patients in the PSCI group was significantly lower than that in the PSNCI group (P < 0.001). Additionally, gender, age, education, atrial fibrillation, previous stroke, NIHSS, white blood cell count, and platelet count showed statistically significant differences (all P < 0.05) (Table 1). To further evaluate the relationship between HRR stratification and PSCI, patients were categorized into four subgroups according to HRR levels: Q1(≤9.70), Q2 (9.71-10.73), Q3 (10.74-11.71), and Q4 (≥11.72). Significant differences in PSCI incidence were observed among the four groups (P < 0.001). Furthermore, gender, age, BMI, drinking, previous stroke, anemia, TOAST mechanism, NIHSS, hemoglobin, RDW, and MMSE score differed significantly across all groups (all P < 0.05) (Table 2).
3.3 Association between HRR and PSCI
As shown in Table 3, with the highest quartile of HRR as reference, patients in the first quartile of HRR had a significantly increased risk of PSCI (Model 1: OR = 4.436, 95%CI = 2.212-8.894, P < 0.001; Model 2: OR = 3.189, 95%CI = 1.495-6.804, P = 0.003; Model 3: OR =2.702, 95%CI = 1.222–5.977, P = 0.014). When used as a continuous variable, HRR level at admission was still correlated with PSCI, and confounding factors were controlled (Model 1: OR = 0.722, 95%CI = 0.625–0.834, P < 0.001; Model 2: OR = 0.760, 95%CI = 0.654–0.884, P < 0.001; Model 3: OR = 0.788, 95%CI = 0.673–0.921, P = 0.003). The trend analysis indicated that HRR was negatively correlated with the occurrence of PSCI (Table 3). In addition, the RCS curve revealed a linear relationship between HRR and PSCI with the adjustment of Model 3 (P for nonlinear = 0.972, P for overall = 0.012) (Figure 2). Further subgroup analysis showed no significant interactions between HRR levels and potential effect modifiers after adjusting for covariates in model 3 (all P for interaction > 0.05) (Figure 3).
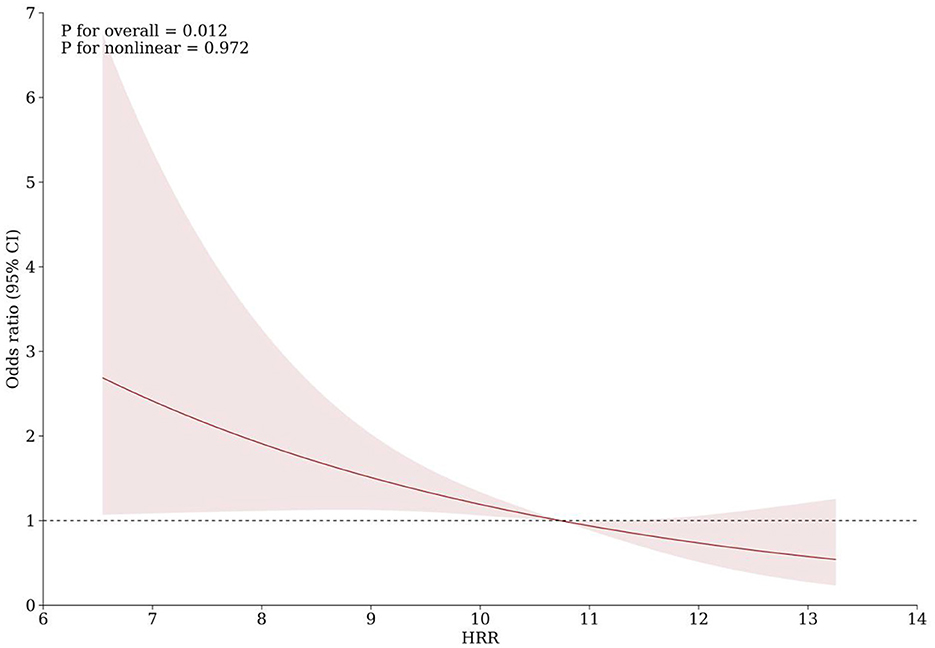
Figure 2. Association of HRR with risk of cognitive impairment after acute ischemic stroke. Odds ratios and 95% confidence intervals derived from restricted cubic spline regression, with knots placed at the 5th, 50th, and 95th percentiles of the distribution of HRR. The reference point is the median of HRR (10.73). Odds ratios were adjusted for the same covariates as model 3 in Table 3.
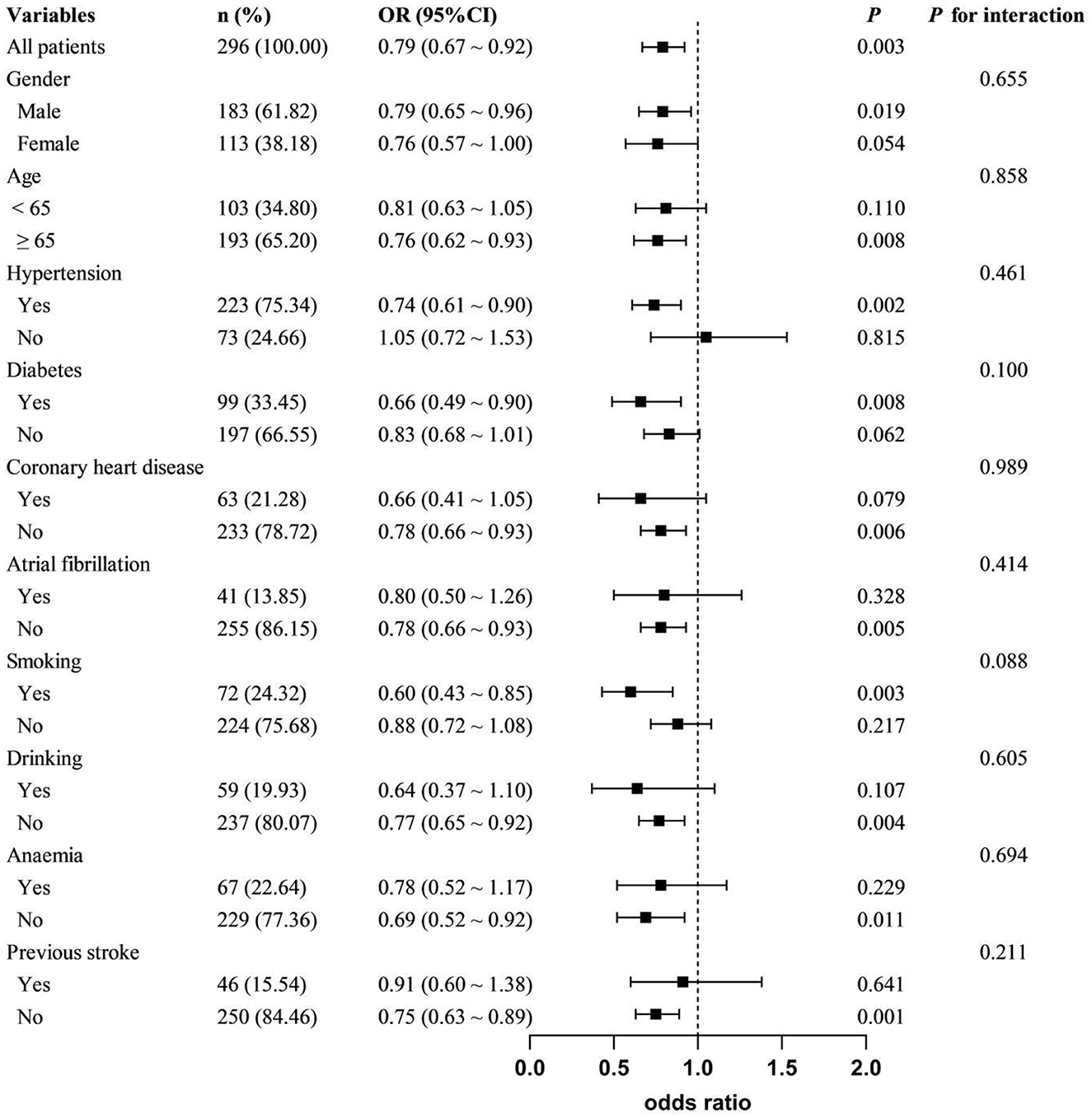
Figure 3. Subgroup analyses of the association between HRR and PSCI. Adjusted covariates included gender, age, education, atrial fibrillation, previous stroke, NIHSS, white blood cell count, and platelet count, except for the stratified variable.
4 Discussion
The primary finding of this study is that low HRR is an independent risk factor for cognitive impairment one month after stroke. As HRR levels increase, the risk of PSCI decreases, suggesting a protective role of HRR against cognitive impairment. The RSC curve indicates a linear relationship between HRR and PSCI, and additional subgroup analyses confirm the stability of these results. Thus, this study demonstrates that HRR is a novel and independent predictor of PSCI risk stratification.
Anemia is recognized as a significant, modifiable risk factor contributing to cognitive decline. Reduced hemoglobin levels were correlated with cognitive decline and VaD in elderly individuals (Andro et al., 2013; Tan et al., 2018). Jia et al. (2022) found that baseline hemoglobin levels were an independent protective factor for PSCI. However, the association between hemoglobin and PSCI within specific subgroups, such as gender, remains uncertain due to the absence of subgroup analysis. Based on the moderate negative correlation between RDW and MMSE score, RDW was considered to be positively correlated with the degree of cognitive impairment in AD patients (Öztürk et al., 2013). The MIND-China Study (Jiang et al., 2021) identified a similar J-shaped relationship between RDW and the odds ratios for AD and VaD, particularly in individuals without anemia. Weuve et al. (2014) found that for each 1 unit (%) increase in RDW, there is a 6% increase in the risk of dementia among the elderly. While anemia, RDW, and cognitive impairment have been extensively explored, HHR and PSCI remain under-studied. Our findings indicate that patients with reduced HHR levels have an increased risk of cognitive impairment. Moreover, the trend analysis and the RCS model reveal a negative linear relationship between the two. Given the physiological differences, female patients were prone to lower hemoglobin levels and anemia. Therefore, we conducted a gender-based subgroup analysis and discovered a stronger association in male (OR = 0.79; 95% CI: 0.65–0.96), albeit the difference was not statistically significant (P for interaction = 0.655). Previous studies have shown that RDW levels appear to be more susceptible to anemia, and thus manifest differences in cognitive impairment outcomes. However, our study did not detect a statistically significant interaction between anemic and non-anemic groups (P for interaction = 0.694). A previous stroke significantly influences the incidence of PSCI, with a notably higher proportion of stroke history observed in the PSCI group. We found significant differences in stroke history among groups with varying HRR levels (P = 0.007). Therefore, it is necessary to clarify whether stroke history affects the relationship between HRR and PSCI. Subgroup analysis showed a stronger association between HRR and PSCI in first-time stroke patients (OR = 0.75, 95% CI: 0.63–0.89, P = 0.001). However, no significant interaction was detected (P for interaction = 0.211). This suggests that HRR may be more broadly applicable for predicting PSCI in various populations. The sample size in this study is relatively small, especially in terms of the number of individuals with anemia. Therefore, further studies with larger sample sizes are needed to confirm our findings.
HRR may be involved in the occurrence and development of PSCI through diverse mechanisms. Decreased hemoglobin levels were associated with structural changes in the brain. Anemia and severe white matter impairment have been found to be associated with executive dysfunction in patients with mild cognitive impairment, suggesting that anemia is involved in the development of white matter lesions (Son et al., 2012). Low hemoglobin levels were also linked to lobular microbleeds and atrophy of the occipital cortex (Tan et al., 2018). Low hemoglobin and lack of hematopoietic raw materials can result in nervous system degeneration, altered iron metabolism, and elevated oxidative stress within brain tissue (Dröge and Schipper, 2007). Anemia induces chronic cerebral hypoxia, potentially contributing to cognitive decline by accelerating the accumulation of Abeta, enhancing tau hyperphosphorylation, disrupting the blood-brain barrier, and facilitating neuronal degeneration (Zhang and Le, 2010). Additionally, anemia can be accompanied by a deficiency of hematopoietic raw materials, inflammation, and malnutrition. These factors have also been validated in studies of PSCI (Lee et al., 2021; Shang et al., 2022; Xu et al., 2023). RDW represents the uniformity of the red blood cell volume size in the blood. An elevated RDW level suggests disruption in red blood cell homeostasis, potentially due to various metabolic abnormalities including malnutrition, cellular inflammation, telomere alterations, and oxidative stress (Salvagno et al., 2015). A series of studies have established RDW as an indicator of inflammation and oxidative stress in the body. Förhécz et al. (2009) observed that RDW correlated with various neuroinflammatory markers, including interleukin-6, C-reactive protein, and soluble tumor necrosis factor receptor I. The possible explanation is that elevated inflammatory factors may shorten the half-life of red blood cells, disrupt their membrane deformability, and impair their maturation, leading to the release of immature red blood cells into peripheral circulation (Lippi et al., 2009; Bazick et al., 2011). RDW is closely related to the lifespan of red blood cells. Prior research indicates that HbA1c or the ratio of HbA1c to glycated albumin might serve as indicators for assessing mean erythrocyte age (Koga et al., 2023). Therefore, we collected Mrbc calculated based on HbA1c to assess the effect of red blood cell mean lifespan on cognition. Since diabetes significantly impacts HbA1c levels, the Mrbc formula is more applicable to non-diabetic patients. Nevertheless, no significant differences in Mrbc were observed between the PSCI and PSNCI groups, even within the non-diabetic population (see Supplementary Figure 1). Possible reasons include that there are many influencing factors of PSCI and the influence of indicators related to red blood cell lifespan is limited. Additionally, stroke patients often display various complications, and some individuals in the non-diabetic population exhibit insulin resistance, which can influence HbA1c levels. Finally, the small sample size may limit the interpretation of results. Additional analyses revealed a weak positive correlation between HRR and Mrbc in non-diabetic participants (r = 0.176, P = 0.013) (see Supplementary Figure 2A). However, no significant correlations were found between Mrbc and hemoglobin (r = 0.137, P = 0.055) or Mrbc and RDW (r = −0.111, P = 0.120) (see Supplementary Figures 2B, C). This suggests that HRR is more strongly associated with red blood cell lifespan than a single indicator. Future research should confirm the utility of HRR in evaluating the lifespan of red blood cells in non-stroke individuals. Serum selenium, an essential component of the antioxidant defense system, exhibits a negative correlation with RDW (Semba et al., 2010). Elevated RDW levels may indicate severe oxidative stress and are potentially linked to neuronal damage in cerebral ischemia and reperfusion injury. In addition, inflammation and oxidative stress jointly contribute to the progression of atherosclerosis (Marchio et al., 2019), representing a crucial pathological mechanism underlying cognitive decline (Liu et al., 2017). These interpretations can be applied to the observed relationship between HRR and PSCI.
There are several limitations to this study. First, we only collected HRR data at admission and lacked relevant data during follow-up, so we were unable to analyze the impact of dynamic changes in HRR on PSCI. Second, study participants had low baseline NIHSS score, suggesting that the findings may only apply to patients with mild to moderate stroke. Third, the exclusion of patients with severe aphasia or dysarthria may introduce selection bias. Fourth, the lack of laboratory data on iron levels and other hematopoietic raw materials made it impossible to analyze the effect of specific anemia types and hematopoietic raw materials on the results. Future studies should incorporate these indicators into analyses to better guide clinical complementary therapy. Finally, we only assessed cognitive function through MMSE and future studies need more detailed neuropsychological assessments to assess cognitive function.
5 Conclusion
In summary, our findings suggest that low baseline HRR is associated with cognitive impairment one month after stroke. Compared to other markers, HRR is cost-effective and easy to access. Early clinical attention to this metric can effectively identify patients at high risk for PSCI.
Data availability statement
The raw data supporting the conclusions of this article will be made available by the authors, without undue reservation.
Ethics statement
The studies involving humans were approved by Ethics Committee of Affiliated Huai'an Hospital of Xuzhou Medical University. The studies were conducted in accordance with the local legislation and institutional requirements. The participants provided their written informed consent to participate in this study. Written informed consent was obtained from the individual(s) for the publication of any potentially identifiable images or data included in this article.
Author contributions
YL: Conceptualization, Investigation, Software, Validation, Visualization, Writing – original draft, Writing – review & editing. ZX: Conceptualization, Data curation, Investigation, Methodology, Software, Writing – original draft. PW: Data curation, Formal analysis, Methodology, Project administration, Visualization, Writing – original draft. FL: Data curation, Formal analysis, Writing – original draft. LZ: Methodology, Supervision, Writing – original draft. CC: Funding acquisition, Project administration, Resources, Writing – original draft. QG: Data curation, Project administration, Writing – original draft. MW: Data curation, Formal analysis, Software, Validation, Writing – original draft. ZZ: Methodology, Supervision, Validation, Visualization, Writing – review & editing, Writing – original draft.
Funding
The author(s) declare that financial support was received for the research and/or publication of this article. This work was supported by Huai'an municipal Science and Technology Program Project (HAB202322).
Acknowledgments
The authors thank the study participants and their relatives as well as the clinical staff for their support and contributions to this study.
Conflict of interest
The authors declare that the research was conducted in the absence of any commercial or financial relationships that could be construed as a potential conflict of interest.
Generative AI statement
The author(s) declare that no Gen AI was used in the creation of this manuscript.
Publisher's note
All claims expressed in this article are solely those of the authors and do not necessarily represent those of their affiliated organizations, or those of the publisher, the editors and the reviewers. Any product that may be evaluated in this article, or claim that may be made by its manufacturer, is not guaranteed or endorsed by the publisher.
Supplementary material
The Supplementary Material for this article can be found online at: https://www.frontiersin.org/articles/10.3389/fnagi.2025.1552956/full#supplementary-material
References
Andro, M., Le Squere, P., Estivin, S., and Gentric, A. (2013). Anaemia and cognitive performances in the elderly: a systematic review. Eur. J. Neurol. 20, 1234–1240. doi: 10.1111/ene.12175
Bazick, H. S., Chang, D., Mahadevappa, K., Gibbons, F. K., and Christopher, K. B. (2011). Red cell distribution width and all-cause mortality in critically ill patients. Crit. Care Med. 39, 1913–1921. doi: 10.1097/CCM.0b013e31821b85c6
Ding, Q., Su, Y., Li, C., and Ding, N. (2022). Red cell distribution width and in-hospital mortality in septic shock: a public database research. Int. J. Lab. Hematol. 44, 861–867. doi: 10.1111/ijlh.13925
Dröge, W., and Schipper, H. M. (2007). Oxidative stress and aberrant signaling in aging and cognitive decline. Aging Cell. 6, 361–370. doi: 10.1111/j.1474-9726.2007.00294.x
Förhécz, Z., Gombos, T., Borgulya, G., Pozsonyi, Z., Prohászka, Z., and Jánoskuti, L. (2009). Red cell distribution width in heart failure: prediction of clinical events and relationship with markers of ineffective erythropoiesis, inflammation, renal function, and nutritional state. Am. Heart J. 158, 659–666. doi: 10.1016/j.ahj.2009.07.024
Hoelzel, W., Weykamp, C., Jeppsson, J. O., Miedema, K., Barr, J. R., Goodall, I., et al. (2004). IFCC reference system for measurement of hemoglobin A1c in human blood and the national standardization schemes in the United States, Japan, and Sweden: a method-comparison study. Clin. Chem. 50, 166–174. doi: 10.1373/clinchem.2003.024802
Jia, W., Jia, Q., Pan, Y., Xiang, X., Liao, X., Zhao, X., et al. (2022). Relationship between baseline haemoglobin content and poststroke cognitive impairment. J. Clin. Neurosci. 101, 212–216. doi: 10.1016/j.jocn.2022.05.008
Jiang, Z., Han, X., Wang, Y., Hou, T., Cong, L., Tang, S., et al. (2021). Red cell distribution width and dementia among rural-dwelling older adults: the MIND-China study. J. Alzheimers. Dis. 83, 1187–1198. doi: 10.3233/JAD-210517
Kara, H., Degirmenci, S., Bayir, A., Ak, A., Akinci, M., Dogru, A., et al. (2015). Red cell distribution width and neurological scoring systems in acute stroke patients. Neuropsychiatr. Dis. Treat. 11, 733–739. doi: 10.2147/NDT.S81525
Koga, M., Kameyama, M., and Okumiya, T. (2023). Estimation of mean erythrocyte age using HbA1c or HbA1c/glycated albumin for evaluation of anemia severity. J. Clin. Lab. Anal. 37:e24947. doi: 10.1002/jcla.24947
Lee, M., Lim, J. S., Kim, Y., Lee, J. H., Kim, C. H., Lee, S. H., et al. (2021). Association between geriatric nutritional risk index and post-stroke cognitive outcomes. Nutrients 13:1776. doi: 10.3390/nu13061776
Lee, M., Saver, J. L., Hong, K. S., Wu, Y. L., Liu, H. C., Rao, N. M., et al. (2014). Cognitive impairment and risk of future stroke: a systematic review and meta-analysis. CMAJ. 186, E536–E546. doi: 10.1503/cmaj.140147
Liang, L., Huang, L., Zhao, X., Zhao, L., Tian, P., Huang, B., et al. (2022). Prognostic value of RDW alone and in combination with NT-proBNP in patients with heart failure. Clin. Cardiol. 45, 802–813. doi: 10.1002/clc.23850
Lippi, G., Targher, G., Montagnana, M., Salvagno, G. L., Zoppini, G., and Guidi, G. C. (2009). Relation between red blood cell distribution width and inflammatory biomarkers in a large cohort of unselected outpatients. Arch. Pathol. Lab. Med. 133, 628–632. doi: 10.5858/133.4.628
Liu, Z., Liu, Y., Tu, X., Shen, H., Qiu, H., Chen, H., et al. (2017). High serum levels of malondialdehyde and 8-OHdG are both associated with early cognitive impairment in patients with acute ischaemic stroke. Sci. Rep. 7:9493. doi: 10.1038/s41598-017-09988-3
Ma, Q., Li, R., Wang, L., Yin, P., Wang, Y., Yan, C., et al. (2021). Temporal trend and attributable risk factors of stroke burden in China, 1990-2019: an analysis for the Global Burden of Disease Study 2019. Lancet Public Health. 6, e897–e906. doi: 10.1016/S2468-2667(21)00228-0
Marchio, P., Guerra-Ojeda, S., Vila, J. M., Aldasoro, M., Victor, V. M., and Mauricio, M. D. (2019). Targeting early atherosclerosis: a focus on oxidative stress and inflammation. Oxid. Med. Cell. Longev. 2019:8563845. doi: 10.1155/2019/8563845
Öztürk, Z. A., Ünal, A., Yigiter, R., Yesil, Y., Kuyumcu, M. E., Neyal, M., et al. (2013). Is increased red cell distribution width (RDW) indicating the inflammation in Alzheimer's disease (AD). Arch. Gerontol. Geriatr. 56, 50–54. doi: 10.1016/j.archger.2012.10.002
Qin, Z., Liao, N., Lu, X., Duan, X., Zhou, Q., and Ge, L. (2022). Relationship between the hemoglobin-to-red cell distribution width ratio and all-cause mortality in ischemic stroke patients with atrial fibrillation: an analysis from the MIMIC-IV database. Neuropsychiatr. Dis. Treat. 18, 341–354. doi: 10.2147/NDT.S350588
Rost, N. S., Brodtmann, A., Pase, M. P., van Veluw, S. J., Biffi, A., Duering, M., et al. (2022). Post-stroke cognitive impairment and dementia. Circ. Res. 130, 1252–1271. doi: 10.1161/CIRCRESAHA.122.319951
Salvagno, G. L., Sanchis-Gomar, F., Picanza, A., and Lippi, G. (2015). Red blood cell distribution width: a simple parameter with multiple clinical applications. Crit. Rev. Clin. Lab. Sci. 52, 86–105. doi: 10.3109/10408363.2014.992064
Semba, R. D., Patel, K. V., Ferrucci, L., Sun, K., Roy, C. N., Guralnik, J. M., et al. (2010). Serum antioxidants and inflammation predict red cell distribution width in older women: the Women's Health and Aging Study I. Clin. Nutr. 29, 600–604. doi: 10.1016/j.clnu.2010.03.001
Shang, T., Ma, B., Shen, Y., Wei, C., Wang, Z., Zhai, W., et al. (2022). High neutrophil percentage and neutrophil-lymphocyte ratio in acute phase of ischemic stroke predict cognitive impairment: a single-center retrospective study in China. Front. Neurol. 13:907486. doi: 10.3389/fneur.2022.907486
Shen, H., and Shen, L. (2024). Red blood cell distribution width as a predictor of mortality and poor functional outcome after acute ischemic stroke: a meta-analysis and meta-regression. BMC Neurol. 24:122. doi: 10.1186/s12883-024-03610-6
Söderholm, M., Borné, Y., Hedblad, B., Persson, M., and Engström, G. (2015). Red cell distribution width in relation to incidence of stroke and carotid atherosclerosis: a population-based cohort study. PLoS ONE. 10:e0124957. doi: 10.1371/journal.pone.0124957
Son, S. J., Lee, K. S., Na, D. L., Seo, S. W., Kim, C. H., Kim, J. H., et al. (2012). The effect of anemia and white matter hyperintensities (WMH) on cognitive impairment in patients with amnestic mild cognitive impairment (MCI). Arch. Gerontol. Geriatr. 55, 251–256. doi: 10.1016/j.archger.2011.10.015
Sun, J. H., Tan, L., and Yu, J. T. (2014). Post-stroke cognitive impairment: epidemiology, mechanisms and management. Ann Transl Med. 2:80.
Synhaeve, N. E., Schaapsmeerders, P., Arntz, R. M., Maaijwee, N. A., Rutten-Jacobs, L. C., Schoonderwaldt, H. C., et al. (2015). Cognitive performance and poor long-term functional outcome after young stroke. Neurology. 85, 776–782. doi: 10.1212/WNL.0000000000001882
Tan, B., Venketasubramanian, N., Vrooman, H., Cheng, C. Y., Wong, T. Y., Chen, C., et al. (2018). Haemoglobin, magnetic resonance imaging markers and cognition: a subsample of population-based study. Alzheimers. Res. Ther. 10:114. doi: 10.1186/s13195-018-0440-5
Wang, R. R., He, M., Ou, X. F., Xie, X. Q., and Kang, Y. (2020). The predictive value of RDW in AKI and mortality in patients with traumatic brain injury. J. Clin. Lab. Anal. 34, e23373. doi: 10.1002/jcla.23373
Weuve, J., Mendes, D., Leon, C. F., Bennett, D. A., Dong, X., and Evans, D. A. (2014). The red cell distribution width and anemia in association with prevalent dementia. Alzheimer Dis. Assoc. Disord. 28, 99–105. doi: 10.1097/WAD.0b013e318299673c
Winchester, L. M., Powell, J., Lovestone, S., and Nevado-Holgado, A. J. (2018). Red blood cell indices and anaemia as causative factors for cognitive function deficits and for Alzheimer's disease. Genome Med. 10:51. doi: 10.1186/s13073-018-0556-z
Xie, X., He, K., Zhang, Y., and Wu, J. (2024). Association of hemoglobin-to-red cell distribution width ratio with the three-month outcomes in patients with acute ischemic stroke. Front. Neurol. 15, 1425633. doi: 10.3389/fneur.2024.1425633
Xiong, Y., Xie, S., Yao, Y., Chen, Y., Ding, J., Zhou, R., et al. (2024). Hemoglobin-to-red blood cell distribution width ratio is negatively associated with stroke: a cross-sectional study from NHANES. Sci. Rep. 14:28098. doi: 10.1038/s41598-024-79520-x
Xu, M., Hu, Y., Wu, J., Wu, Z., Yang, S., He, J., et al. (2023). Association between the serum iron and acute cognitive impairment after stroke: a cross-sectional study. J. Geriatr. Psychiatry Neurol. 36, 121–128. doi: 10.1177/08919887221095874
Xue, J., Zhang, D., Zhang, X. G., Zhu, X. Q., Xu, X. S., and Yue, Y. H. (2022). Red cell distribution width is associated with stroke severity and unfavorable functional outcomes in ischemic stroke. Front. Neurol. 13:938515. doi: 10.3389/fneur.2022.938515
Zhang, R., Xu, Q., Wang, A., Jiang, Y., Meng, X., Zhou, M., et al. (2021). Hemoglobin concentration and clinical outcomes after acute ischemic stroke or transient ischemic attack. J. Am. Heart Assoc. 10:e022547. doi: 10.1161/JAHA.121.022547
Keywords: stroke, cognitive impairment, hemoglobin, red cell distribution width, HRR
Citation: Liu Y, Xie Z, Wang P, Liu F, Zhao L, Chen C, Ge Q, Wang M and Zhi Z (2025) Relationship between the hemoglobin-to-red cell distribution width ratio and post-stroke cognitive impairment: a prospective study. Front. Aging Neurosci. 17:1552956. doi: 10.3389/fnagi.2025.1552956
Received: 29 December 2024; Accepted: 10 April 2025;
Published: 30 April 2025.
Edited by:
Hipólito Nzwalo, University of Algarve, PortugalReviewed by:
Masashi Kameyama, Tokyo Metropolitan Institute for Geriatrics and Gerontology, JapanFulvio Tartara, University Hospital of Parma, Italy
Copyright © 2025 Liu, Xie, Wang, Liu, Zhao, Chen, Ge, Wang and Zhi. This is an open-access article distributed under the terms of the Creative Commons Attribution License (CC BY). The use, distribution or reproduction in other forums is permitted, provided the original author(s) and the copyright owner(s) are credited and that the original publication in this journal is cited, in accordance with accepted academic practice. No use, distribution or reproduction is permitted which does not comply with these terms.
*Correspondence: Zhongwen Zhi, MTUxNTIzMzgwODlAMTYzLmNvbQ==; Mengchao Wang, Y213cXcxODI4QDE2My5jb20=
†These authors have contributed equally to this work and share first authorship