- Production and Population Health, Royal Veterinary College, Hertfordshire, United Kingdom
Animal welfare assessments that measure welfare outcomes, including behavior and health, can be highly valid. However, the time and skill required are major barriers to their use. We explored whether feasibility of welfare outcome assessment for dairy herds may be improved by rationalizing the number of measures included. We compared two approaches: analyzing whether strong pairwise associations between measures existed, enabling the subsequent exclusion of associated measures; and identifying possible summary measures—“iceberg indicators”—of dairy herd welfare that could predict herd welfare status. A cross-sectional study of dairy herd welfare was undertaken by a single assessor on 51 English farms, in which 96 welfare outcome measures were assessed. All measures showed at least one pairwise association; percentage of lame cows showed the most (33 correlations). However, most correlations were weak–moderate, suggesting limited scope for excluding measures from protocols based on pairwise relationships. A composite measure of the largest portion of herd welfare status was then identified via Principal Component Analysis (Principal Component 1, accounting for 16.9% of variance), and linear regression revealed that 22 measures correlated with this. Of these 22, agreement statistics indicated that percentage of lame cows and qualitative descriptors of “calmness” and “happiness” best predicted Principal Component 1. However, even these correctly classified only ~50% of farms according to which quartile of the Principal Component 1 they occupied. Further research is recommended, but results suggest that welfare assessments incorporating many diverse measures remain necessary to provide sufficient detail about dairy herd welfare.
Introduction
Welfare outcome (animal-based) measures are arguably the most valid indicators of animal welfare (Rushen and Passillé, 1992; Knierim and Winckler, 2009), so their inclusion in welfare assessment protocols is widely recommended (Capdeville and Veissier, 2001; Waiblinger et al., 2001; Webster et al., 2004; FAWC, 2005). However, welfare outcome assessment is often extremely time-consuming (Rushen and Passillé, 1992). This is partly because it generally needs to be undertaken across multiple animals (Ito et al., 2009; Mullan et al., 2009a; Endres et al., 2014) and/or multiple time points (Ito et al., 2009; Vasseur et al., 2012) to ensure sufficient reliability. For example, although it only takes ~1 min to assess avoidance distance in an individual dairy cow using the Welfare Quality® protocol, it can take up to 70 min (depending on herd size) to assess this at the herd level (Welfare Quality, 2009). Also, as animal welfare is multi-dimensional (Fraser et al., 1997; Botreau et al., 2007a) and there is no perfect welfare indicator (Mason and Mendl, 1993), welfare assessments need to be based on multiple measures (Blokhuis et al., 2010; Nicol et al., 2011). Although it is sometimes possible to assess multiple welfare outcome measures simultaneously [e.g., aspects of lying behavior, social behavior, and coughing can all be recorded within a single observation period (Welfare Quality, 2009)], the inclusion of multiple measures generally greatly increases overall assessment time. Full welfare outcome assessments can therefore take many hours to complete for a single herd (Welfare Quality, 2009).
The substantial implementation time of welfare outcome assessments is a major barrier to their widespread use on-farm (Knierim and Winckler, 2009; Sandgren et al., 2009; Blokhuis et al., 2010; Nyman et al., 2011; de Vries et al., 2013b). However, it is important they are implemented because of their enhanced validity compared with quicker, resource-based, assessments. Therefore, it is vital we work to develop solutions to improve their feasibility for legal inspections, welfare assurance schemes, and other assessments by farmers and veterinarians.
There are three main routes by which the feasibility of welfare outcome assessments could be improved, without compromising the overall reliability or validity of the assessments:
1) automating assessment activities (e.g., Rushen et al., 2012; Berckmans, 2014);
2) optimizing sampling strategies within farms, such as focal animal sample sizes and/or the length/frequency of observation periods to achieve an optimal balance between assessment feasibility and reliability (e.g., Ito et al., 2009; Main et al., 2010; Heath et al., 2015; Van Os et al., 2018);
3) optimizing the number of measures included in assessment protocols through the use of “summary measures” that can predict other or wider aspects of welfare, to achieve an optimal balance between assessment feasibility and validity (e.g., Mülleder et al., 2007; Nicol et al., 2011; Nyman et al., 2011).
In this paper, we focus on the latter route.
There is a small but growing body of research into the optimisation of the number of measures included in assessment protocols. Some studies have investigated relationships between individual welfare outcome measures to highlight areas of potential overlap within assessment protocols, identifying apparently redundant welfare outcome measures for exclusion (e.g., Mülleder et al., 2007; Nicol et al., 2011). Other studies have investigated the existence of putative iceberg indicators which can be used, on their own, to describe the wider welfare status of farms (e.g., Sandgren et al., 2009; Nyman et al., 2011). Iceberg indicators are termed as such because they “provide an overall assessment of welfare, just as the tip of an iceberg signals its submerged bulk beneath the water's surface” (FAWC, 2009).
Associations between different welfare outcomes should exist to some extent, because of causal relationships between them, such as an injury (one measure) causing cows to become lame (a second measure); because of shared underlying risk factors, such as poor housing resulting in dirtiness, injuries and lameness; or because different measures are supposed to be measuring the same thing (animal welfare). Indeed, associations between welfare outcome measures are commonly noted (e.g., Roche et al., 2009; Weary et al., 2009; de Vries et al., 2011). For example, reduced time lying down (Chapinal et al., 2009; Proudfoot et al., 2010) and poor body condition (Green et al., 2014; Randall et al., 2015) are risk factors for lameness across individual cows.
The potential of this approach for improving welfare outcome assessment feasibility has been considered in several species including cattle (Mülleder et al., 2007; de Vries et al., 2013a,b, 2014), pigs (Mullan et al., 2009b), and chickens (Nicol et al., 2011). These studies often did find significant pairwise associations between the measures investigated, but associations were generally weak (i.e., correlation coefficients of <0.4), leading authors to conclude there was little scope for using one measure to substitute for another (Mülleder et al., 2007; Mullan et al., 2009b; Nicol et al., 2011; de Vries et al., 2013a). However, a more encouraging result was reported by de Vries et al. (2014), who investigated the extent to which records-based welfare measures could predict directly observed welfare outcome measures; although the predictive ability of individual records-based measures was again poor, predictive performance substantially increased when these measures were combined into small subsets. Also, in broiler chickens, one study found considerable scope for using individual slaughter plant assessments of hockburn and footpad dermatitis to replace certain on-farm measures, which they strongly predicted, potentially reducing welfare assessment time by up to 3 h (de Jong et al., 2015).
The possible existence of iceberg indicators of welfare has received relatively little theoretical consideration to date. FAWC (2009) postulated that some welfare outcomes may be particularly effective at summarizing overall husbandry quality and animal welfare. The idea that single measures can provide a broad assessment of animal welfare is debated, however, because of the supposed multi-dimensional nature of animal welfare. For example, an effective iceberg indicator might need to capture the extent of pain, fear, hunger, disease, contentment, and more (Dawkins, 2006; Botreau et al., 2007a).
A few studies have attempted to improve welfare assessment feasibility by identifying potential iceberg indicators of welfare, with mixed success. Some of these studies investigated whether any easily available welfare input (de Vries et al., 2016) or welfare outcome (Sandgren et al., 2009; Nyman et al., 2011; Brouwer et al., 2015; Krug et al., 2015) measures held within national dairy databases could be used as screening tools to predict the welfare status of herds, as determined by on-farm welfare assessment. Most found that small subsets of records-based welfare outcome measures—related primarily to herd mortality, fertility and somatic cell counts—predicted herd welfare status with a moderate-high degree of accuracy, sensitivity and specificity. Thus, the authors concluded that there appeared to be scope for using records-based welfare outcome measures as a highly feasible means of estimating dairy herd welfare. Similarly, a study in pigs concluded there was good potential for using abattoir data as a feasible means of indicating wider welfare on pig farms (van Staaveren et al., 2017).
Other studies investigated whether particular aspects of the Welfare Quality® assessment protocol for dairy cows could predict the assessment's overall classification result, i.e., whether a farm was categorized as, for example, “acceptable” or “enhanced” (Andreasen et al., 2013; Heath et al., 2014b). Specifically, Andreasen et al. (2013) investigated whether the Qualitative Behavior Assessment (QBA) component of the Welfare Quality® protocol could predict the assessment's overall classification result, because it is intended to capture the animals' expressions of their own subjective experiences (e.g., Wemelsfelder et al., 2001; Wemelsfelder, 2007). However, they found no significant correlation between QBA and the overall classification result. In contrast, Heath et al. (2014a) found that—when analyzed within a diagnostic agreement framework—the QBA component of the Welfare Quality® protocol was reasonably good at predicting the overall classification result (67% predictive accuracy). That study investigated the extent to which many different components of the Welfare Quality® protocol, including welfare inputs, could predict the overall classification result. Unexpectedly, the best performing component was the “absence of thirst” criterion, which comprises several welfare input measures related to water provision. The authors argue that, rather than water provision being highly informative/integrative per se, this result likely reflects previously identified problems with the Welfare Quality® multi-criteria aggregation method used to generate the overall classification result (Heath et al., 2014a). This is because the “absence of thirst” criterion was unintentionally weighted more highly within the overall aggregation process compared with many of the other criteria within the assessment protocol (de Vries et al., 2013a), creating an especially strong relationship between this criterion and the overall classification (Heath et al., 2014a). A similar issue was described when using the Welfare Quality® protocol for broiler chickens, where the final classification was heavily influenced only by “drinker space” and “stocking density,” and the classification was extremely insensitive to changes in other constituent welfare outcome measures (Buijs et al., 2016). It is thus difficult to draw firm conclusions about the possible existence of iceberg indicators of welfare based on these latter studies, but clearly it will be important to develop a welfare classification system that does not give undue weight to constituent measures that are unlikely to be key determinants or signals of overall animal welfare.
In this study we aimed to evaluate whether the feasibility of welfare outcome assessment for dairy herds could be improved by rationalizing the number of measures used. The objectives were to identify pairwise correlations between measures of welfare and to identify putative iceberg indicators of welfare, via a cross-sectional study incorporating a comprehensive welfare outcome assessment.
Our hypotheses were that, if some welfare indicators are highly predictive of overall welfare, then firstly pairwise associations will exist between different individual welfare outcome measures for UK dairy herds; and secondly measures of the overall welfare statuses of UK dairy herds can be predicted by a few specific individual welfare outcome measures (iceberg indicators).
The two hypotheses are complementary, but it was necessary to test both because, whilst use of iceberg indicators would be the more efficient approach, it was uncertain that iceberg indicators would even exist. If none existed, then pairwise analyses could at least indicate if any individual measures were redundant (and thus could be excluded) because of a very strong correlation with another measure.
Materials and Methods
Selection and Recruitment of Farms
Farms were recruited from a database of 468 British dairy farms that had participated in the AHDB Dairy (formally DairyCo) Milkbench+ Profitability Benchmarking Scheme in 2012 [described in DairyCo (2014)]. We used a purposive stratified sampling approach to select farms of differing system types and potential welfare statuses into the study. To do this, relevant Milkbench+ variables (e.g., “amount of non-forage feed fed/cow/year” and “% cows culled”) were submitted to an exploratory principal component analysis (PCA), to help identify composite measures to give an approximation relevant to system type and welfare status. This revealed two distinct principal components, which did not map entirely onto system type or welfare status, but that appeared to describe the overall “production intensity” (e.g., average milk yield/cow/year, amount of non-forage feed fed/cow/year) and general “mortality/morbidity status” (e.g., % cows culled, average milk SCC/year) of farms, respectively. We used the “production intensity” and “mortality/morbidity” principal component scale quartile values to stratify the 468 Milkbench+ farms into a total of 16 farm system type/herd welfare status categories. The stratification process resulted in categories spanning (a) lower input/output farms with poorer welfare (higher mortality/morbidity), (b) lower input/output farms with better welfare through to (c) higher input/output farms with poorer welfare and (d) higher input/output farms with better welfare. A similar sampling approach has been used in a number of previous studies to actively recruit farms of a range of different system types (Haskell et al., 2006), herd sizes (Nyman et al., 2011), and herd welfare statuses (de Vries et al., 2013a).
For logistical reasons it was only possible to visit farms in the South/Midlands of England, which comprised 242 of the original 468 farms. All of the 16 farm system type/herd welfare status categories were still well-represented across the 242 farms. Farms were then selected for telephone recruitment at random from within each of the 16 categories in a sequential fashion, to ensure that approximately equal numbers of farms were recruited from within the 16 categories. Farms that accepted the invitation to participate during the recruitment telephone call were recruited providing they met the following criteria: (i) intention to participate in the Milkbench+ Profitability Benchmarking Scheme in 2013 (ensuring farm profitability data for 2013 for a related study); (ii) participation in milk recording at least every 6 weeks (ensuring availability of detailed herd milk production, milk quality, and fertility data); and (iii) use of separate housing for milking cows and dry cows/pre-calving heifers (the on-farm welfare assessment protocol focused on milking cows only and this ensured that non-milking animals were not accidentally scored).
Incentives to encourage participation in the study comprised on-farm feedback of mobility scoring results, farm performance benchmarking with respect to a number of key welfare outcome measures, and an overall summary report of the project findings. Also, participants were assured that the farm visit would not impact on the daily routine of the farm.
Data Collection
Overview
All farm visits were conducted by the same assessor (SC) between mid-September 2013 and mid-April 2014. Where possible, visits coincided with the farms' winter housing period, and each visit was conducted over 2 consecutive days. The visits comprised two main phases: an on-farm welfare outcome assessment of the milking herd, and a farmer interview. The on-farm welfare assessment took ~12–14 h to complete across the 2 days, depending on herd size. The interview was then undertaken on Day 2, with the member of farm staff responsible for herd health management (the “farmer”), taking ~60 min. The farmer was asked about the farm's record-keeping, and the assessor took photographic or electronic copies of relevant and available herd health/welfare records for subsequent review. The farmer was also asked to read the study information sheet and sign the associated study consent form, as well as additional consent forms enabling subsequent access to the farm's milking recording data and British Cattle Movement Service records. The methods were approved by the Royal Veterinary College (RVC)'s ethics committee and data were held securely in line with the RVC's guidelines on data confidentiality and protection.
Developing the Welfare Outcome Assessment Protocol
Welfare outcome measures were selected and considered for inclusion using four sources. Firstly, we conducted a review of existing assessment protocols for dairy cows developed either by animal welfare scientists and/or industry (e.g., Capdeville and Veissier, 2001; Waiblinger et al., 2001; Whay et al., 2003a; Welfare Quality, 2009; AssureWel, 2015). Secondly, to develop and supplement the list of measures we conducted a consultation of expert opinion involving members of both the AHDB Dairy “Health, Welfare & Nutrition” Research Partnership Work Package on welfare assessment, and the RVC Farm Animal Health and Production Group, to gather opinions on key welfare outcome measures to include in the protocol. Thirdly, we conducted UK dairy farmer and cattle vet focus groups and questionnaire survey (Collins, 2016a) to identify the participants' preferences for different welfare outcome measures and their opinions on potential iceberg indicators of dairy cow welfare; and lastly, we conducted on-farm assessment trial sessions at the RVC farm, and a formal pilot study on four dairy farms (selected as a convenience sample) in March 2013.
The individual welfare outcome measures included in the protocol needed to be valid, reliable and feasible (Winckler et al., 2003), although this is yet to be established for many commonly used welfare measures (Knierim and Winckler, 2009). Priority was given to already standardized welfare outcome measures [e.g., those included in Welfare Quality (2009)] for which these criteria had already been evaluated (e.g., Forkman and Keeling, 2009; Knierim and Winckler, 2009). When selecting measures for which multiple standardized versions were available, preference was given to UK dairy industry recommended measures [e.g., the AHDB Dairy Mobility Score and AssureWel cleanliness, abrasions and swellings scores were selected over equivalent Welfare Quality® measures (AHDB Dairy, 2015b; AssureWel, 2015)]. This was so that the assessment results could be meaningfully compared with existing UK studies, and the data generated could be easily interpreted by participating farmers.
Welfare Outcome Measures
The final welfare outcome assessment protocol featured measures related to different aspects of dairy cow production, health, physical condition and behavior. Welfare outcome measures were assessed at the cow-, cow group- or herd-level using lactating cow groups, and then summarized at the herd-level. Supplementary Table 1 provides an overview of the structure and content of the protocol. Full details of the structure and content of the protocol—including exact assessment procedures, descriptions of case definitions (e.g., “lame,” “dirty,” “aggressive head-butt,” etc.) and detailed procedures for summarizing the data collected at the herd-level—are provided in Supplementary Table 2.
Unfortunately, the prevalence and/or incidence of the “health event” welfare outcomes referred to in Supplementary Tables 1, 2 (i.e., mastitis, lameness, dystocia, milk fever, retained fetal membranes, metritis/endometritis, and displaced abomasums) could not ultimately be calculated. This was because most farm records were found to be of insufficient quality or quantity to provide suitably robust data for analysis, and so these measures could not be included in our final welfare outcome dataset.
Intra-Observer Reliability of Welfare Outcome Scoring
To help to ensure a good level of intra-observer reliability, the assessor underwent official training to measure the welfare outcomes included, where this was available (e.g., for the Welfare Quality® measures and the AHDB Dairy Mobility Score). Additionally, the assessor practiced data collection during the pilot studies, and the intra-observer reliability of the assessor's scoring was then formally assessed.
To develop suitable intra-observer reliability tests, relevant photographs and/or video footage of cows were collected during the assessment trial sessions, pilot study, and first few farm visits. Tests were successfully developed for individual qualitative descriptors (QDs), time taken to lie down, collisions during lying down, the continuous behavior sampling measures, mobility, body condition, cleanliness, abrasions, swellings, ocular discharge, nasal discharge, vulval discharge, diarrhea, injured tails, and cows lying incorrectly. Hampered respiration, chase-ups of lying cows, fighting bouts, or chasing bouts could not be included because they were too infrequent to capture on film. The response to assessor was also excluded because it was difficult to replicate using photographs/video footage. The developed agreement tests were undertaken at the beginning, middle, and end of the farm visit period and the results obtained at the three different time points were statistically compared.
Statistical Analysis
Investigating the Pairwise Relationships Between the Different Welfare Outcome Measures
All statistical analyses were completed using IBM SPSS Statistics v.22 and a type I error rate of 0.05 was used in all statistical tests. Pairwise analysis was important for identifying the degrees to which outcomes were correlated in an initial exploratory analysis, related to finding outcomes that were highly predictive of other outcomes. Pairwise relationships between continuous welfare outcome measures were investigated using correlations. Pearson's correlation tests were used when both measures were normally distributed. Where data were not normally distributed, natural logarithm or square root transformations were applied in an attempt to achieve normal distribution. Negatively skewed data were reversed prior to this. Variables with excessive zeros could not be transformed to achieve normal distribution.
Spearman's rank correlations were used when one or both of the measures could not be transformed to achieve normal distribution. The relationship between the various continuous welfare outcome measures and response to assessor (the only categorical welfare outcome measure in the protocol) was investigated using logistic regression. No correction was made to the p values to adjust for multiple testing, due to the exploratory nature of these various pairwise analyses (Bender and Lange, 2001).
Determining Herd Overall Welfare Status
To investigate whether any individual welfare outcome measures could predict the overall welfare status of herds, it was first necessary to develop a method for determining as closely as possible the herds' overall welfare status. Instead of condensing measures using specific “aggregation rules” informed mainly by expert opinion as in previous studies (e.g., Bracke et al., 2002; Botreau et al., 2008, 2009; Calamari and Bertoni, 2009), we attempted to aggregate measures into a composite overall welfare scale on the basis of their observed inter-relationships using PCA.
As PCA cannot be undertaken on variables with a lot of missing data or with low variance, such measures were excluded. These were QD distressed, frustrated and bored, mean number of chase ups, chasing bouts and fighting bouts per cow/hour, mean time to lie down, % collisions during lying down, all of the automatically recorded lying behavior measures, % cows dull and depressed, all of the substantial swelling measures except % cows with substantial swelling on the hind leg, % cows with lesions on the udder, % cows with diarrhea, % cows with hampered respiration, all of the milk recording measures, and all of the mortality measures. Also, due to multi-collinearity, in any identified ≥0.9 pairwise correlation the variable with the smallest number of correlations with other measures was excluded. This was particularly important for outcomes comprising several similar welfare measures, such as several alternative measures of cleanliness. Thus, all closely related alternative measures were removed before conducting the PCA (Field, 2013).
Principal components with eigenvalues of ≥1 were reviewed and interpreted on the basis of the various measures' factor loadings. Factor loadings of ≥0.4 were used as a threshold to indicate a meaningful association. In line with similar existing studies (e.g., Veissier et al., 2004; Van Reenen et al., 2005) the first principal component, which accounts for the most variance within a given dataset, was taken forward as our measure of the composite welfare scale (being the largest single aggregate measure of the originally submitted variables).
To avoid the circularity of investigating relationships between each individual measure and a composite scale within which it was nested (Heath et al., 2014a), multiple composite welfare scales were generated using the PCA method described, each time excluding the welfare outcome measure to be tested against it. In instances where there were multiple versions of the same measure (e.g., dirty and very dirty, or lame and very lame) all versions were excluded. This allowed us to test the extent to which each individual measure could predict the composite welfare scale as summarized by all other variables (e.g., “does the percentage of lame cows correlate with the composite welfare scale when the percentage of lame cows has been excluded from that composite scale?”).
Intra-class correlation coefficients were used to test the level of agreement between the various newly generated composite welfare scales and the original composite welfare scale to investigate any likely reduction in validity resulting from the systematic exclusion approach. Correlation between these scales was found to be statistically significant and very high (correlation coefficient >0.9) in every case (p < 0.001). Therefore, we proceeded to test individual variables against their own complementary composite PCA scales as proxy measures of herd overall welfare status.
Identifying Iceberg Indicators of Dairy Herd Welfare
Linear regression analysis was used to investigate whether any of the individual welfare outcome measures could predict herd overall welfare status (i.e., the measures' respective composite welfare scale). Measures excluded from the original PCA (e.g., due to missing data or multicollinearity) were compared with the original composite welfare scale. In addition, a separate PCA was undertaken on the 20 QD terms included in the protocol which produced (three) summary measures of the QBA (labeled, on the basis of factor loadings, herd “contentedness,” “agitation,” and “sociability”). Relationships between each of these three QBA principal components and their respective complementary composite welfare scales were also investigated using separate linear regressions. This additional PCA was conducted because QDs are not advised to be used independently of each other (e.g., Welfare Quality, 2009), whereas we also needed to test the descriptors independently in this exploratory study, because of our aim being to investigate whether any of the measures, including any QDs, could be removed.
Finally, all individual measures that were significantly associated with their complementary composite welfare scale were taken forward to a second stage of analysis of herd welfare status categories. This was necessary because, in applied contexts such as welfare assurance labeling schemes for consumers, welfare is summarized as categories [e.g., poor, acceptable, enhanced, or excellent (Welfare Quality, 2009)], rather than on a continuum (Webster et al., 2004; Honey, 2013). This second stage therefore investigated the ability of the individual welfare outcome measures to predict herd welfare categories that were created from the composite welfare scales. To do this, farms were categorized into quartiles on the composite welfare scales, creating four potential categories of overall “welfare status.” Mirroring this, farms were also categorized into quartiles for each significant individual welfare outcome measure. Agreement between the quartile allocations of each individual measure versus quartiles of the complementary welfare scale was assessed using predictive accuracy (% farms correctly classified), Cohen's Kappa statistic and Kendall's coefficient of concordance. This allowed us to test the extent to which farm categories that were created using each individual measure would match the farm categories that were created using the complementary welfare scales. Perfect agreement would indicate that the allocation of farms into quartiles according to an individual measure exactly matched the quartile allocation for the complementary welfare scale. Once again, due to the exploratory nature of the regression and agreement analyses used to explore the iceberg indicator question, no correction was made to the p values to adjust for multiplicity (Bender and Lange, 2001).
Results
Description of Farm Sample
In total 52 farms (each with a single herd) were recruited into the study. This was the number of farms practically possible to visit within the study period. One farm withdrew its participation before its visit and, therefore, the cross-sectional study was undertaken on a total of 51 farms. The median milking herd size of the farms was 180 cows (IQR = 84; min. = 57; max. = 1,545). Median days in milk ranged between 25 and 252 across the different herds (median = 179; IQR = 60), and the mean 305 day milk yield/cow was 8,290.9 L (SD = 1,622.7; min. = 4,742.3; max. = 11,608.1). Median milking cow parity was 2 (IQR = 1–3). Descriptive statistics for key categorical farm management variables for the final 51 farms assessed are displayed in Table 1. Most farms had Holstein Friesian or Holstein cows. Most farms had all-year-round calving, and cubicle housing systems, and most milked twice daily using a non-robotic system. The median number of days at grass during 2013 was 193.5 days (IQR = 82.3; min. = 0.0; max. = 294.0).
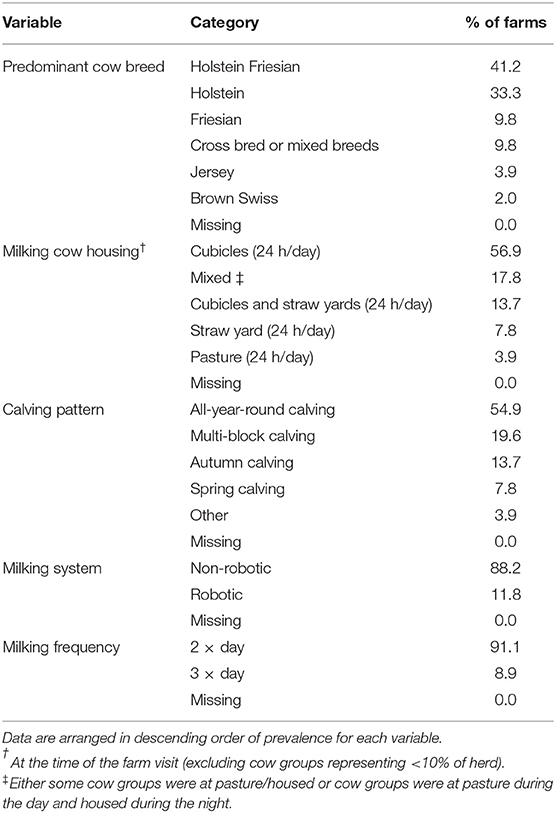
Table 1. Descriptive statistics for the categorical farm management variables for the 51 cross-sectional study farms.
Welfare Outcome Measure Descriptive Statistics
Descriptive statistics for the final 96 behavior-based, health and physical condition-based and records-based welfare outcome measures are displayed in Supplementary Table 3. The QDs receiving the highest visual analog scale (VAS) scores across farms were “relaxed,” “calm,” “positively occupied,” and “content.” The median herd mean for percentage cows feeding was 33.0%, and of ruminating was 27.4%. The median herd mean of cows lying down was 41.9%, and they lay down for a median herd mean of 10.5 h/day; only 0.1% lay incorrectly, but 23.9% of those observed lying down collided with housing equipment. A median of 1.2 agonistic episodes were seen per cow/h, whilst 0.2 equivalent episodes of social licking were seen. A median of 22.2% of cows per herd were lame, and 5.7% very lame. A median of 26.5% of cows/herd had nasal discharge, and a median of 18.6% had high somatic cell counts on their most recent test day. The median mortality was 5.3–6% cows/herd dying on farm, depending on the year. During the response to assessor test, 72.3% of herds were assessed as “calm/relaxed,” and 27.7% were assessed as “nervous/wary.”
The variation observed in welfare performance across the 51 farms depends on the individual welfare outcome measure in question. For example, the % of cows with swellings on their hind legs varied by 53.1% (0 – 53.1%) across the different herds, whereas the cows with swellings on their udders varied by just 4.2% (0 – 4.2%). As noted previously some welfare outcomes were rare, with many zeros. For example, at least 75% of the farms received scores of zero for 17 of the 96 welfare outcome measures (QD fearful, frustrated, bored and distressed; mean no. of fighting and chasing bouts per cow/h; % cows with diarrhea, hampered respiration or swelling on their udder; % cows with lesions on their udder, head/neck/shoulders and foreleg; and % cows with substantial swelling on the five body areas investigated).
Intra-observer Reliability
Intra-observer reliability was very good for all of the categorical welfare outcome measures tested (Supplementary Tables 4, 5). Cohen's Kappa values of >0.60 [indicating “substantial” agreement (Landis and Koch, 1977)], and Kendall's coefficient of concordance values of >0.70 [indicating “strong” agreement (Schmidt, 1997)] were consistently achieved across all three timepoints, for all measures. Intra-observer reliability was also good for the continuous welfare outcome measures tested. Intra class correlation coefficients of >0.40 (indicating “fair” reliability; Cicchetti, 1994) were consistently observed for all measures, with the exception of “QD happy” (which was weaker: coefficient = 0.17–0.44). Furthermore, for most comparisons, coefficients of >0.75 (indicating a “good” level of reliability) were achieved. It must be noted, however, that agreement was not always statistically significant for a number of the QD measures; the lack of significance could be because these agreement tests were based on only five observations due to little suitable video footage, whereas the tests for the other measures were based on between 18 and 60 observations.
Pairwise Associations Between Individual Welfare Outcome Measures
Each of the 95 continuous welfare outcome measures was significantly correlated with at least one other measure. Most significant correlations were at best only “moderate” in strength i.e., 0.4 to 0.7 (Martin and Bateson, 2007). Only 12 correlations were “high” strength (≥0.7 to <0.9) and only five “very high” strength (≥0.9), and these generally comprised pairs of measures that captured aspects of the same welfare outcome (e.g., “dirty” and “very dirty” hindquarters; Table 2).
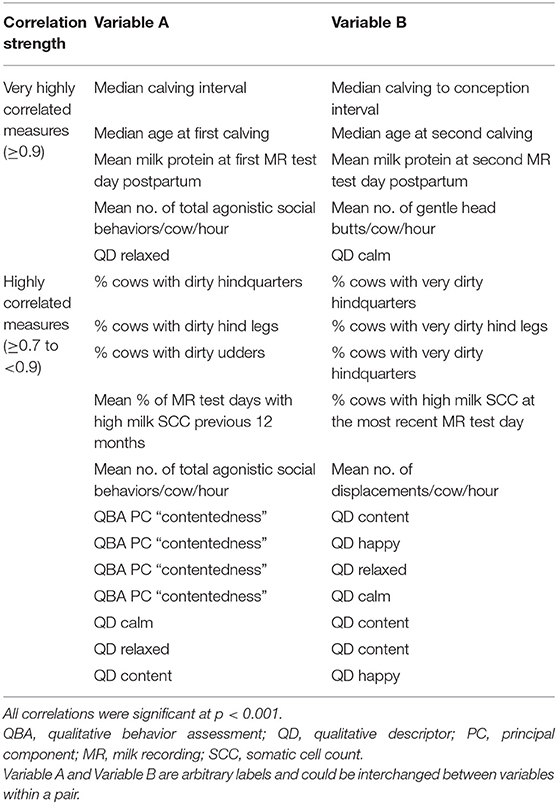
Table 2. Very high and high pairwise correlations detected between the 95 continuous welfare outcome measures.
The percentage of lame cows significantly correlated with the largest number of other measures (33), whereas both “mean number of chases/cow/hour” and “percentage heifer calves died on-farm 2012” significantly correlated with the fewest measures (one each). Supplementary Table 6 displays the welfare outcome measures that were at least moderately correlated with 10 or more other measures for information.
Finally, there were also significant pairwise relationships between four of the 95 continuous welfare outcome measures and the herds' response to assessor, which was recorded as the proportion of cows “calm/relaxed” vs. “nervous/wary” (0 vs. 1, respectively). These were “SD no. of lying bouts/day” (Coeff+/−S.E = 1.2+/−0.4; p = 0.016), “Percentage cows with low protein on first/second MR test day postpartum” (16.3+/−6.6; p = 0.019), age at first calving (60.7+/−20.2; p = 0.005), and age at second calving log10 (0.03+/−0.01; p = 0.004).
Determining Herd Overall Welfare Status
The PCA to create the overall welfare outcome scale reduced the 56 welfare outcome measures that could be included into 17 principal components, which together explained 85.3% of the variance in the dataset. Some missing data were tolerated within the dataset, but this meant that principal component scores could only be generated for 41 of the 51 farms. The first principal component (PC 1) explained 16.9% of the variance. Table 3 summarizes the 23 welfare outcome measures which had factor loadings of >0.4 for PC 1. On the basis of these factor loadings, it can be interpreted that farms with higher positive scores for PC 1 had a poorer welfare status. For example, they received lower QD happy and QD content scores and higher scores for QD apathetic and QD uneasy, and had higher percentages of dirty and lame cows. Overall, given both the breadth and strong welfare relevance of the 23 individual welfare outcomes measures with factor loadings of >0.4 for PC 1, this principal component was deemed a suitable proxy measure of herd welfare status for the purposes of the iceberg indicator analyses. Beyond PC 1, the other principal components were more difficult to interpret and less obviously relevant to welfare (Collins, 2016b). The decrease in their explanatory value upon examining the scree plot was fairly gradual rather than there being a clear step change, meaning there was no obvious “top” set of principal components to consider as the most important. Thus, despite the fairly low percentage of variance that PC 1 explained on its own, it was taken forward as the relevant composite welfare scale against which potential iceberg indicator measures could be tested.
Identifying Iceberg Indicators of Dairy Herd Welfare
Linear regressions revealed that 22 of the 96 welfare outcome measures were significantly associated with their respective composite welfare scales (Table 4). Most correlated in the expected direction; that is, most measures of poor welfare (e.g., dirty udders and coughs) correlated positively with the composite welfare scale, and most measures of good welfare (e.g., QD happy and QD content) correlated negatively. Although percentage cows ruminating, QD relaxed and QD calm appear to be exceptions, this was an artifact resulting from these variables being reversed during statistical transformation to correct for skewness.
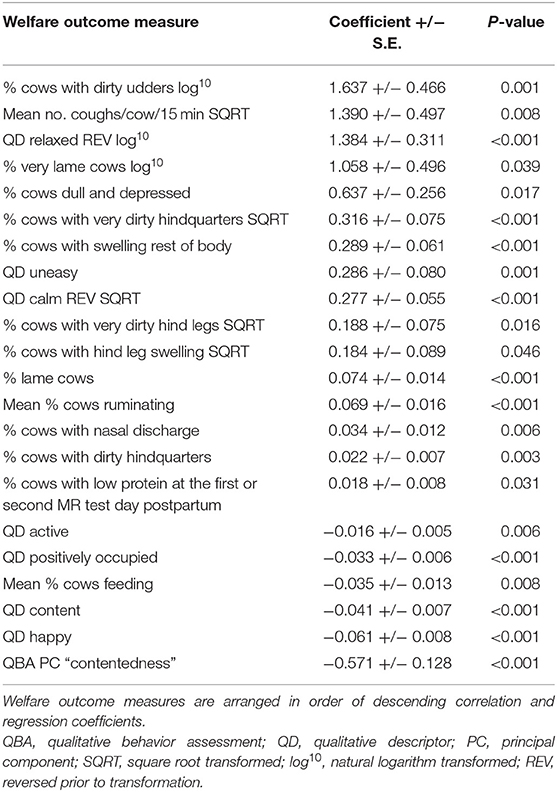
Table 4. Simple linear regression model results of the significant relationships between the individual welfare outcome measures and their respective composite welfare scales.
Table 4 shows that most correlations were with measures that had high loading onto PC 1, but a further five measures that could not be included in the PCA were also among those correlating with the composite welfare scale (these were: percentage cows dull and depressed, QD calm, percentage cows with hind leg swelling, percentage cows with low protein at the first or second MR test day postpartum, and the QBA “contentedness” principal component). Conversely, seven measures that had high loading on the composite welfare scale, did not show significant correlations with their respective composite welfare scales (these were: percentage cows with very dirty udders, percentage cows with dirty and very dirty hindlegs, QD apathetic, QD indifferent, QD lively, and QD friendly).
When the above 22 welfare outcomes were tested for their ability to predict composite welfare categories (i.e., each herd's quartile allocation), absolute agreement was at best only reasonable (Table 5). Most measures correctly classified <50% of the farms. Kappa statistics were often <0.2 [which indicates only “slight” agreement (Landis and Koch, 1977)]. Agreement on the basis of Kendall's coefficient of concordance, which accounts for the magnitude of any misclassifications, was often >0.7 indicating “strong” agreement (Schmidt, 1997). Overall, QD calm, QD happy and percentage of lame cows achieved the greatest level of agreement with their respective composite welfare categories. These all had Kendall's coefficients of concordance >0.7, and were the only measures to correctly classify (just) over 50% of farms and to obtain Cohen's Kappa statistics approaching >0.4 (the threshold indicating at least “moderate” agreement).
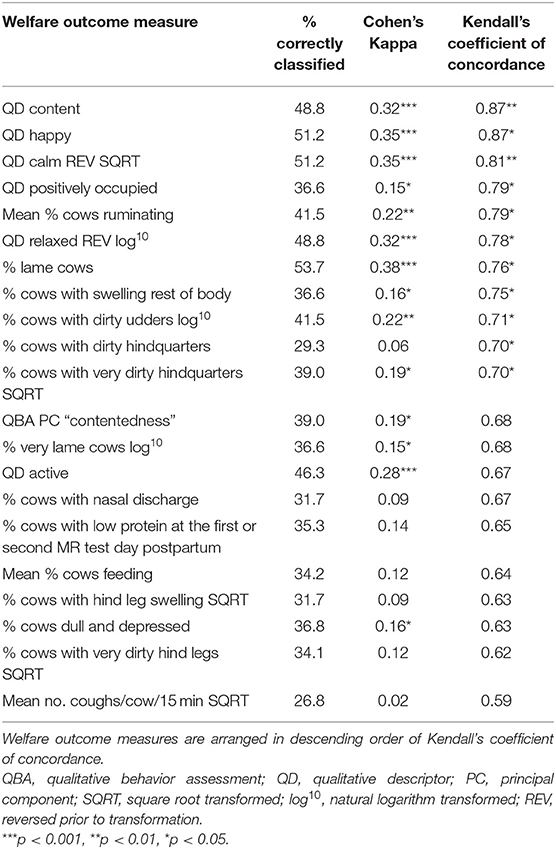
Table 5. The relative performance of welfare outcome measures in predicting their respective composite welfare categories.
Discussion
In this study, two different and complementary methods have been explored in an attempt to increase the feasibility of a comprehensive animal welfare outcome assessment used on UK dairy farms. The welfare outcome assessment protocol showed “good” to “very good” intra-observer reliability for almost all the measures that could be tested, and the large numbers of apparently biologically meaningful correlations between measures suggests the protocol had good internal validity. However, the findings suggest limited capacity for effectively reducing the numbers of welfare outcomes included within this two-day assessment, for reasons that will be discussed separately for the two approaches below.
The study sample can be considered suitably representative of the wider UK dairy farm population with respect to farm management. In line with the wider population, most farms in the study had Holstein-Friesian or Holstein cows as their predominant breed, housed their cows in cubicles, and calved all-year-round. Median herd size (180 cows) and mean 305 day milk yield/cow/year (8,290.9 L) were broadly similar to, but slightly higher than, the UK averages at the time [herd size: 126; milk yield: 7,535 L (AHDB Dairy, 2015a)]. With respect to welfare performance, both the median and range of percentage lame cows are broadly in line with recent UK prevalence estimates (Griffiths et al., 2018; Randall et al., 2019).
Pairwise Associations Between Individual Welfare Outcome Measures
As with previous literature (Mülleder et al., 2007; Mullan et al., 2009b; de Vries et al., 2013a), the results of the pairwise correlations reveal relatively little scope for reducing the number of welfare outcome measures included in assessment protocols for UK dairy herds. This is because, although many significant associations existed between measures, these associations were generally relatively weak. Just 17 high or very high associations were found between measures of the same “type” (e.g., QD relaxed vs. QD calm, median calving interval vs. median calving to conception interval and % cows with dirty udders vs. % cows with very dirty hindquarters), similar to previous work (de Vries et al., 2013a). In these cases, replacing one of these measures with the other would be possible, but would generally have little or no impact on assessment implementation time. For example, the Welfare Quality® observation time for QBA is 20 min regardless of how many QD terms are used. From our results, there is no obvious welfare outcome measure that could be excluded on the basis of high pairwise associations to enable meaningful time-saving and increased efficiency.
It is perhaps not surprising to primarily find pairwise associations of only relatively limited strength. Firstly, relationships between measures are often not causal in nature. Instead welfare compromises, such as lameness, poor body condition, or abnormal behavior, generally have very complex multifactorial etiologies whereby they are influenced over time by multiple different (interacting) risk factors (e.g., Espejo and Endres, 2007; Dippel et al., 2009). Secondly, and fundamentally, welfare outcome measures are indirect manifestations of the subjective, multidimensional welfare experience of animals (e.g., Mason and Mendl, 1993; Duncan, 2005). They may differ in terms of their validity and the extent to which they represent particular dimensions of welfare (Rushen and Passillé, 1992; Botreau et al., 2007a). However, it is reassuring that the correlations were generally biologically plausible and meaningful in terms of their direction of correlation being consistent with either good or poor welfare (Supplementary Table 6), suggesting validity of the welfare assessment protocol.
Identifying Iceberg Indicators of Dairy Herd Welfare
Our results show that significant associations did exist between 22 individual welfare outcome measures and composite welfare scales that we used to approximate herd overall welfare status (Table 4). On the basis of the methods used, the measures QD happy, QD calm, and percentage of lame cows were arguably the best performing predictors. It is encouraging that a combination of these three measures captured positive and negative dimensions of welfare. However, on their own each individual measure only correctly classified around 50% of farms.
It is unclear how much this level of performance is a consequence of the genuine predictive ability of the measures or the methods used to determine predictive ability. In the absence of a universal gold standard for welfare scale, there appears to be an unavoidable element of circularity within the identification process. That is, to determine whether individual welfare outcome measures can predict herd overall welfare status, we first need a gold standard measure of overall welfare status, and the most valid way to create this is by using welfare outcome measures themselves (Rushen and Passillé, 1992; Knierim and Winckler, 2009). Ideally, the measures used to determine the overall welfare status would be derived independently of the measures being tested, and yet they would still need to describe the same animals within the same situation (so that the underlying subjective welfare state would be consistent). We attempted to avoid the problem highlighted by Heath et al. (2014a) by testing the ability of each measure to predict an composite welfare summary measure that excluded itself. However, by definition, a measure with any predictive ability must be highly associated with variables comprising the summary scale. We removed predetermined cases of such circularity (e.g., when testing percentage lame cows, we removed not only that measure but also percentage of very lame cows from the summary PCA welfare component). Nevertheless, the pairwise associations show that many less obvious measures also correlated with certain individual measures (Supplementary Table 6). However, if we had removed all of these correlated variables from the complementary welfare scales, then, inevitably, the individual measure would no longer have shown much predictive ability. It is difficult to develop an approach to determine herd overall welfare status that does not rely on the measures that are also being tested as candidate iceberg indicators, because multiple measures are needed for a comprehensive assessment of multi-dimensional welfare (Botreau et al., 2007a,b).
If we accept the results provided by the method used in this study, it is noteworthy that several QD measures performed well in the analyses, whilst the QBA components did less well. Other studies to date have seemingly not explored QDs independently, because they are not intended for use alone, but the predictive value of QBA has been investigated. Heath et al. (2014a) found that the QBA component of the Welfare Quality protocol was reasonably good at predicting the assessment's overall classification result. Furthermore, de Vries et al. (2013a) found that non-QBA aspects of the Welfare Quality® protocol were moderately good at predicting farm performance with respect to QBA—in fact this was the best predicted aspect of the protocol. QBA has been described as a potentially highly “integrative” welfare assessment tool (Wemelsfelder et al., 2001), because it is intended to summarize all observed aspects of animal behavior and physical condition into terms describing the animals' subjective experience (Wemelsfelder, 2007). It also provides measures of positive welfare that are difficult to capture by other methods. However, Andreasen et al. (2013) found no relationship between QBA and herd overall welfare status (using the Welfare Quality® overall classification result), and other studies investigating relationships between QBA and other aspects of welfare are mixed (Heath et al., 2014a). Furthermore, questions exist around both the validity and reliability of QBA because it is so subjective (Wemelsfelder et al., 2000, 2001; Bokkers et al., 2012). In our study, QD happy was the only measure not to attain “fair” (or above) intra-observer reliability at all three timepoints, despite having amongst the best predictive ability. We cannot assume that the predictive value of the QD or QBA assessments would have been similar with another assessor, and perhaps the conflicting results in the aforementioned studies are due to inter-assessor variation. Inter-observer agreement regarding QDs and QBA is clearly an area for continued research, because QBA reliability has previously been found to be poor in some studies (Bokkers et al., 2012; Winckler, 2014). In order for any potential iceberg indicators to be of use in an applied setting, such as for legal or assurance purposes, they will need to display an appropriate level of intra- and inter-observer reliability. We would therefore recommend similar studies are attempted with different and a larger number of assessors, to investigate whether the present findings for QD calm and happy—as well as the other measures featured in our assessment protocol—can be replicated when other assessors are used.
It should also be noted that, in the present study, we treated the individual QD terms (such as QD calm and QD happy) as individual welfare outcome measures in their own right, alongside our PCA generated summary measures of QBA (“contentedness,” “sociability,” and “agitation”), in case any were found to be redundant. Existing work on the validation and reliability of the QBA approach has generally focused on the resulting PCA summary measures, rather than the individual descriptor terms themselves. Interestingly, our results do appear to suggest that the individual QD terms are able to capture welfare relevant information and, for the most part, provide fairly good levels of (intra) observer agreement. However, as suggested above, it will be important to ensure these findings can be replicated beyond the present study.
Lameness prevalence also performed well in our analyses. Lameness indicates pain (Whay et al., 2005), and is thus highly welfare relevant. It can be prevalent on UK farms (Barker et al., 2010) and, consequently, it is frequently cited as among the most important welfare measures for dairy cattle (Whay et al., 2003b). Consistent with our findings, de Vries et al. (2013a) found that non-lameness aspects of the Welfare Quality® protocol were moderately good at predicting the prevalence of (severely) lame cows—this was the second best predicted aspect of the protocol after QBA. Its predictive ability may be due to lameness having a multifactorial etiology, reflecting the general quality of farm management, environment and stockmanship (Dippel et al., 2009; Rutherford et al., 2009; Barker et al., 2010), and reflecting that pain thresholds are affected by mood (in humans and rodent models at least: Wiech and Tracey, 2009).
It is interesting to note that the findings of Sandgren et al. (2009) and Nyman et al. (2011), which described good predictive ability of welfare outcome measures related to mortality and fertility, were not replicated in our study. None of the mortality and fertility measures investigated here were significantly associated with their respective composite welfare scales (although they did show significant pairwise associations with certain other measures, e.g., Supplementary Table 6). Reasons for the discrepancy between studies are difficult to discern, as there were many differences in methods and sampling.
Other measures of welfare that are usually considered important and might have served as good iceberg indicators, including rumination, lying behavior, body condition, and vulval discharge (e.g., FAWC, 2009), did not perform especially well in this study. Body condition and vulval discharge varies considerably with lactation stage, but the farms in the present study exhibited a range of calving patterns and, therefore, herd stage of lactation was inconsistent across farms. Farms visited when cows were most “eligible” to have poor body condition/vulval discharge are likely to have more reliable estimates for these welfare outcomes than farms visited at a different time. In future, ideally measures of body condition/vulval discharge that take account of cow stage of lactation would be used, or, if this is not possible, the impact of stage of lactation could be investigated and possibly accounted for in the statistical analyses. Percentage of cows ruminating showed good predictive ability, but in an unexpected direction: higher percentages of cows ruminating significantly predicted measures of poorer welfare, whereas rumination is normally reduced with poor welfare conditions [e.g., metabolic disorders (Stangaferro et al., 2016a), severe metritis (Stangaferro et al., 2016c), and mastitis caused by E. coli (Stangaferro et al., 2016b), but seemingly not with lameness (Walker et al., 2008; Thorup et al., 2016)]. This unexpected finding might be an artifact of how we measured rumination, because the scan sampling section of the protocol started directly after morning feed delivery or return from milking. This means that cows were likely to be feeding at that time, rather than ruminating, and feeding and rumination were mutually exclusive behaviors (Schirmann et al., 2012). This is supported by the fact that % time spent ruminating correlated negatively with % time spent feeding (Supplementary Table 6), and greater % time spent feeding (in the hours following feed delivery) was significantly associated with better welfare on the composite welfare scale (Table 4). Farms are increasingly adopting rumination and activity monitoring, so continuous measures for both these will probably greatly assist further research in this area (Stangaferro et al., 2016a).
Method for Determining Herd Overall Welfare Status
The validity of the developed composite welfare scale(s) as a proxy for the herds' genuine overall welfare status was central to our attempts to identify iceberg indicators of dairy herd welfare. The use of PCA to aggregate measures based on their existing inter-relationships is a potentially more valid approach than, for example, the use of predetermined aggregation rules. There is currently very little scientific evidence on which to base such rules (e.g., relative weightings), so their use can lead to unexpected/unintentional aggregation results (de Vries et al., 2013a; Heath et al., 2014a; Buijs et al., 2016). Furthermore, the composite welfare scale provided a relatively comprehensive “overall” assessment of welfare because it incorporated a relatively large number of different measures (e.g., QBA of herd behavior, lameness, cleanliness, swellings, nasal discharge, coughing, rumination, and feeding behavior). However, some of the measures included in the PCA (e.g., abrasions and social behavior) were not well-represented by the composite welfare scale (PC 1). This does not necessarily mean that they did not help measure welfare and are therefore unimportant. On the contrary, they may be particularly important to retain within welfare assessment protocols precisely because they captured different aspects of welfare from the measures that loaded onto PC 1 (which after all did only explain 17% of the total variation). Aggregations via PCA are purely correlational and may not all be biologically meaningful, so whether using data derived weightings, theory driven weightings, or no weightings at all, any approach could have unintended consequences if it led to the wrong measures being retained or excluded. A third consideration about the validity of the PCA method for summarizing welfare is that some measures could not be included in the aggregation process, either because their data type was unsuitable for PCA (e.g., the milk recording data generated measures) or because they could not be collected in the first place (e.g., prevalence/incidence of mastitis, dystocia etc.). It is possible, therefore, that the composite welfare scale describes particular aspects of herd welfare as opposed to the herds' genuine overall welfare status. Ultimately, however, if welfare assessment protocols can be improved such that the individual measures are more suitable for inclusion within PCA, the developed composite welfare scale offers an alternative to predetermined aggregation methods, and is a promising proxy measure of herd overall welfare status.
Within this study we opted to use PC1 to create a measure of the overall herd welfare status, because it explained the most variation (albeit only 17%), and its loadings were consistent with an animal welfare interpretation. However, it is possible that other approaches could have been used to summarize the most important loadings on more than one principal component, although information might be lost through this selective method. Also, the precise method for unifying these loadings into a single value per farm could introduce the aforementioned difficulty of how variables from different components would need to be weighted.
We recommend that the validity of aggregation via PCA is further reviewed by investigating whether similar PCA results are achieved if the welfare outcome assessment protocol is repeated, for example, on different farms and/or if more welfare outcome measures are included in the analysis. Also, some of the measurement protocols should be reviewed to improve the likelihood of variables being suitable for inclusion within PCAs in future. For example, measurement protocols for variables that generated excessive zeros in this study, could be adjusted to lower the threshold for noting presence of the criterion being measured, or the timing of observations could be improved to better capture that measure.
Method for Identifying Iceberg Indicators of Dairy Herd Welfare
There are two potential limitations to converting farm welfare performance from a continuous scale into categories, approximating an applied rating (e.g., Welfare Quality, 2009). Firstly, the conversion will inevitably have resulted in a certain amount of information loss. That is, the use of four “welfare performance categories” provides less detail than the true variation in welfare performance observed across farms. Secondly, the (quartile value) thresholds used to determine farm category membership are arbitrary and specific to the farms sampled, rather than providing absolute standards. Some studies have since also used quartiles, although the authors did not distinguish all four quartiles, instead denoting the worst quartile as that indicating “poor” animal welfare on the farms falling within it, whilst the remaining three quartiles denoted “acceptable” animal welfare (e.g., de Vries et al., 2016; van Staaveren et al., 2017). If we had created only two categories, the kappa agreement ratings would almost certainly have been higher than they were (because there is less scope for error with fewer options). The use of four categories does serve to describe the farms' relative welfare performance more appropriately overall (farms in the first category did perform differently from farms in the fourth category), but the precise thresholds may not have been meaningful in terms of distinguishing “poor,” “acceptable,” “good,” or “excellent” animal welfare. Choice of threshold does influence how well different measures perform (Sandgren et al., 2009), so the relative predictive ability of the different measures could change if different thresholds were used. A challenge, however, will be identifying the most appropriate thresholds for benchmarking (Mendl, 1991; Botreau et al., 2007a).
In this study, we used agreement statistics to test the predictive ability of each individual outcome measure with regards to the farm welfare categories, but other approaches could be used. For example, discriminant analysis could have been used to identify important outcomes loading onto the quartiles identified by PCA (Presi and Reist, 2011). The results would still have been affected by where the category thresholds were defined, but discriminant analysis could be an efficient approach for future studies.
Conclusion
Overall, we found a large number of associations between the different welfare outcome measures included in our assessment protocol. However, most pairwise associations were weak to moderate, and existed between highly related measures, so there appears to be relatively little scope for excluding individual measures from assessment protocols based on their pairwise relationships. Linear regression analysis revealed that 22 measures were significantly associated with their respective composite welfare scale. Subsequent analysis of their ability to predict the quartile classification of herds revealed that, of these, QD calm, QD happy and percentage of lame cows were the best performing measures, although their predictive ability was only moderately good. These measures may therefore be regarded as potential iceberg indicators capturing both positive and negative aspects of dairy herd welfare. Further research using the same methodological approach with a new sample of farms, multiple assessors to investigate inter-observer reliability, and improvement of certain individual welfare outcome measures is needed to test the external validity of the statistical methods used, and to confirm or refute our findings.
Until valid and reliable approaches that reduce the time required to perform effective welfare assessments are developed, it remains necessary to complete full welfare assessments, ensuring that animal welfare issues are not missed and appropriate standards are recognized.
Data Availability Statement
The original contributions presented in the study are included in the article/Supplementary Material, further inquiries can be directed to the corresponding author.
Ethics Statement
The animal study was reviewed and approved by the Royal Veterinary College Ethics and Welfare Committee (Approval number: 2013 1236). Written informed consent was obtained from the owners for the participation of their animals in this study.
Author Contributions
SC, CB, CW, JC, and NB: contributed to conception and design of the study. SC: collected data, organized the database, performed the statistical analysis, and wrote the first draft of the manuscript. CB, CW, JC, and NB: supervised the project. Y-MC: gave statistical advice. CB: wrote sections of the manuscript. All authors contributed to manuscript revision, read, and approved the submitted version.
Funding
This project was funded via a BBSRC/RVC CASE studentship (VMG42) with AHDB Dairy (then named Dairy Co.).
Conflict of Interest
The authors declare that the research was conducted in the absence of any commercial or financial relationships that could be construed as a potential conflict of interest.
Publisher's Note
All claims expressed in this article are solely those of the authors and do not necessarily represent those of their affiliated organizations, or those of the publisher, the editors and the reviewers. Any product that may be evaluated in this article, or claim that may be made by its manufacturer, is not guaranteed or endorsed by the publisher.
Acknowledgments
We would like to thank Jenny Gibbons and the AHDB Dairy team for guidance and advice throughout the project. We are extremely grateful to staff at the 51 farms that participated in the cross-sectional study. Special thanks also go to all the following people and teams. Natalie Chancellor provided technical assistance with the development of the cross-sectional study intra-observer reliability tests, and the farm records review process. The AHDB Dairy Health, Welfare and Nutrition Research Partnership (particularly Cheryl Heath, David Main, Siobhan Mullan, and Marie Haskell), and the RVC Farm Animal Health and Production group (particularly Richard Booth), gave feedback on the development of the welfare outcome assessment protocol and the iceberg indicator aspects of the project. Paul Christian, Charlie Verity, and Graeme Webster at the RVC Farm, gave feedback on various aspects of the project and enabled pilot testing of the welfare outcome assessment protocol. Jo Speed provided AHDB Dairy Mobility Score training, and members of the Welfare Quality® consortium (particularly Christoph Winckler and Marlene Kirchner) provided Welfare Quality® protocol training. The Milkbench+ team (particularly Karolina Klaskova) assisted with the Milkbench+ profitability benchmarking scheme data. The British Cattle Movement Service provided movement data for the cross-sectional study farms.
Supplementary Material
The Supplementary Material for this article can be found online at: https://www.frontiersin.org/articles/10.3389/fanim.2021.703380/full#supplementary-material
References
AHDB Dairy (2015b). Mobility Scoring for Dairy Cows. Available online at: http://dairy.ahdb.org.uk/resources-library/technical-information/health-welfare/mobility-score-instructions/#.VhdFareFPcs (accessed October 07, 2015).
Andreasen, S. N., Wemelsfelder, F., Sandøe, P., and Forkman, B. (2013). The correlation of Qualitative Behavior Assessments with Welfare Quality® protocol outcomes in on-farm welfare assessment of dairy cattle. Appl. Anim. Behav. Sci. 143, 9–17. doi: 10.1016/j.applanim.2012.11.013
AssureWel (2015). Dairy Cows. Available online at: http://www.assurewel.org/dairycows (accessed October 07, 2015).
Barker, Z. E., Leach, K. A., Whay, H. R., Bell, N. J., and Main, D. C. J. (2010). Assessment of lameness prevalence and associated risk factors in dairy herds in England and Wales. J. Dairy Sci. 93, 932–941. doi: 10.3168/jds.2009-2309
Bender, R., and Lange, S. (2001). Adjusting for multiple testing - when and how? J. Clin. Epidemiol. 54, 343–349. doi: 10.1016/S0895-4356(00)00314-0
Berckmans, D. (2014). Precision livestock farming technologies for welfare management in intensive livestock systems. Revue Scientifique et Technique 33, 189–198. doi: 10.20506/rst.33.1.2273
Blokhuis, H. J., Veissier, I., Miele, M., and Jones, B. (2010). The Welfare Quality® project and beyond: Safeguarding farm animal well-being. Acta Agri. Scand. A Anim. Sci. 60, 129–140. doi: 10.1080/09064702.2010.523480
Bokkers, E. A. M., de Vries, M., Antonissen, I., and de Boer, I. J. M. (2012). Inter- and intra-observer reliability of experienced and inexperienced observers for the Qualitative Behaviour Assessment in dairy cattle. Anim. Welfare 21, 307–318. doi: 10.7120/09627286.21.3.307
Botreau, R., Bonde, M., Butterworth, A., Perny, P., Bracke, M. B. M., Capdeville, J., et al. (2007a). Aggregation of measures to produce an overall assessment of animal welfare. Part 1: a review of existing methods. Animal 1, 1179–1187. doi: 10.1017/S1751731107000535
Botreau, R., Bracke, M. B. M., Perny, P., Butterworth, A., Capdeville, J., Van Reenen, C. G., et al. (2007b). Aggregation of measures to produce an overall assessment of animal welfare. Part 2: analysis of constraints. Animal 1, 1188–1197. doi: 10.1017/S1751731107000547
Botreau, R., Capdeville, J., Perny, P., and Veissier, I. (2008). Multicriteria evaluation of animal welfare at farm level: an application of MCDA methodologies foundations of computing and decision. Sciences 31, 287–316.
Botreau, R., Veissier, I., and Perny, P. (2009). Overall assessment of animal welfare: strategy adopted in Welfare Quality®. Anim. Welfare 18, 363–370.
Bracke, M. B. M., Metz, J. H. M., Spruijt, B. M., and Schouten, W. G. P. (2002). Decision support system for overall welfare assessment in pregnant sows B: validation by expert opinion. J. Anim. Sci. 80, 1835–1845. doi: 10.2527/2002.8071835x
Brouwer, H., Stegeman, J. A., Straatsma, J. W., Hooijer, G. A., and Schaik, G.v. (2015). The validity of a monitoring system based on routinely collected dairy cattle health data relative to a standardized herd check. Prev. Vet. Med. 122, 76–82. doi: 10.1016/j.prevetmed.2015.09.009
Buijs, S., Ampe, B., and Tuyttens, F. A. M. (2016). Sensitivity of the Welfare Quality® broiler chicken protocol to differences between intensively reared indoor flocks: which factors explain overall classification? Animal 11, 244–253. doi: 10.1017/S1751731116001476
Calamari, L., and Bertoni, G. (2009). Model to evaluate welfare in dairy cow farms. Italian J. Anim Sci. 2009:23. doi: 10.4081/ijas.2009.s1.301
Capdeville, J., and Veissier, I. (2001). A method of assessing welfare in loose housed dairy cows at farm level, focusing on animal observations. Acta Agri. Scandi. A Anim. Sci. 51, 62–68. doi: 10.1080/090647001316923081
Chapinal, N., de Passillé, A. M., Weary, D. M., von Keyserlingk, M. A. G., and Rushen, J. (2009). Using gait score, walking speed, and lying behavior to detect hoof lesions in dairy cows. J. Dairy Sci. 92, 4365–4374. doi: 10.3168/jds.2009-2115
Cicchetti, D. V. (1994). Guidelines, criteria, and rules of thumb for evaluating normed and standardized assessment instruments in psychology. Psychol Asses. 6:284–290. doi: 10.1037/1040-3590.6.4.284
Collins, S. (2016a). “Chapter 3: investigating UK dairy farmer and cattle vet definitions of animal welfare and preferences for using different welfare outcomes,” in An Investigation of Whether and How Welfare Outcome Assessment Could Be Better Used by UK Dairy Farmers. (PhD Thesis), University of London, London, United Kingdom, 49–82.
Collins, S. (2016b). “Chapter 4: exploring the possibility of improving welfare outcome assessment feasibility by optimising the number of measures included in assessment protocols for dairy herds,” in An Investigation of Whether and How Welfare Outcome Assessment Could Be Better Used by UK Dairy Farmers. (PhD Thesis), University of London, London, United Kingdom, 83–134.
DairyCo (2014). Evidence Report: Analysis of the Milkbench+ and International Dairy Benchmarking Data for 2012/13, Kenilworth.
Dawkins, M. S. (2006). A user's guide to animal welfare science. Trends Ecol. Evol. 21, 77–82. doi: 10.1016/j.tree.2005.10.017
de Jong, I. C., Hindle, V. A., Butterworth, A., Engel, B., Ferrari, P., Gunnink, H., et al. (2015). Simplifying the Welfare Quality® assessment protocol for broiler chicken welfare. Animal 10, 117–127. doi: 10.1017/S1751731115001706
de Vries, M., Bokkers, E. A. M., Dijkstra, T., van Schaik, G., and de Boer, I. J. M. (2011). Invited review: associations between variables of routine herd data and dairy cattle welfare indicators. J. Dairy Sci. 94, 3213–3228. doi: 10.3168/jds.2011-4169
de Vries, M., Bokkers, E. A. M., van Schaik, G., Botreau, R., Engel, B., Dijkstra, T., et al. (2013a). Evaluating results of the Welfare Quality multi-criteria evaluation model for classification of dairy cattle welfare at the herd level. J. Dairy Sci. 96, 6264–6273. doi: 10.3168/jds.2012-6129
de Vries, M., Bokkers, E. A. M., van Schaik, G., Engel, B., Dijkstra, T., and de Boer, I. J. M. (2014). Exploring the value of routinely collected herd data for estimating dairy cattle welfare. J. Dairy Sci. 97, 715–730. doi: 10.3168/jds.2013-6585
de Vries, M., Bokkers, E. A. M., van Schaik, G., Engel, B., Dijkstra, T., and de Boer, I. J. M. (2016). Improving the time efficiency of identifying dairy herds with poorer welfare in a population. J. Dairy Sci. 99, 8282–8296. doi: 10.3168/jds.2015-9979
de Vries, M., Engel, B., den Uijl, I., van Schaik, G., Dijkstra, T., de Boer, I. J. M., et al. (2013b). Assessment time of the Welfare Quality® protocol for dairy cattle. Anim. Welfare 22, 85–93. doi: 10.7120/09627286.22.1.085
Dippel, S., Dolezal, M., Brenninkmeyer, C., Brinkmann, J., March, S., Knierim, U., et al. (2009). Risk factors for lameness in freestall-housed dairy cows across two breeds, farming systems, and countries. J. Dairy Sci. 92, 5476–5486. doi: 10.3168/jds.2009-2288
Duncan, I. J. H. (2005). Science-based assessment of animal welfare: farm animals. Revue scientifique et technique Office international des epizooties 24, 483–492. doi: 10.20506/rst.24.2.1587
Endres, M. I., Lobeck-Luchterhand, K. M., Espejo, L. A., and Tucker, C. B. (2014). Evaluation of the sample needed to accurately estimate outcome-based measurements of dairy welfare on farm. J. Dairy Sci. 97, 3523–3530. doi: 10.3168/jds.2013-7464
Espejo, L. A., and Endres, M. I. (2007). Herd-level risk factors for lameness in high-producing holstein cows housed in freestall barns. J. Dairy Sci. 90, 306–314. doi: 10.3168/jds.S0022-0302(07)72631-0
FAWC (2005). Report on the Welfare Implications of Farm Assurance Schemes. London: Farm Animal Welfare Committee.
FAWC (2009). Farm Animal Welfare in Great Britain: Past, Present and Future. London: Farm Animal Welfare Committee.
Forkman, B., and Keeling, L. J. (2009). “Assessment of animal welfare measures for dairy cattle, beef bulls and veal calves,” in Welfare Quality Reports No. 11, eds M. Miele and J. Roex (Uppsala).
Fraser, D., Weary, D. M., Pajor, E. A., and Milligan, B. N. (1997). A scientific conception of animal welfare that reflects ethical concerns. Anim. Welfare 6, 187–205.
Green, L. E., Huxley, J. N., Banks, C., and Green, M. J. (2014). Temporal associations between low body condition, lameness and milk yield in a UK dairy herd. Prev. Vet. Med. 113, 63–71. doi: 10.1016/j.prevetmed.2013.10.009
Griffiths, B. E., Grove White, D., and Oikonomou, G. (2018). A cross-sectional study into the prevalence of dairy cattle lameness and associated herd-level risk factors in England and Wales. Front. Vet. Sci. 5:65. doi: 10.3389/fvets.2018.00065
Haskell, M. J., Rennie, L. J., Bowell, V. A., Bell, M. J., and Lawrence, A. B. (2006). Housing system, milk production, and zero-grazing effects on lameness and leg injury in dairy cows. J. Dairy Sci. 89, 4259–4266. doi: 10.3168/jds.S0022-0302(06)72472-9
Heath, C. A. E., Browne, W. J., Mullan, S., and Main, D. C. J. (2014a). Navigating the iceberg: reducing the number of parameters within the Welfare Quality® assessment protocol for dairy cows. Animal 8, 1978–1986. doi: 10.1017/S1751731114002018
Heath, C. A. E., Lin, Y., Mullan, S., Browne, W. J., and Main, D. C. J. (2014b). Implementing Welfare Quality® in UK assurance schemes: evaluating the challenges. Anim. Welfare 23, 95–107. doi: 10.7120/09627286.23.1.095
Heath, C. A. E., Main, D. C. J., Mullan, S., Haskell, M. J., and Browne, W. J. (2015). Sequential sampling: a novel method in farm animal welfare assessment. Animal. 10, 349–356. doi: 10.1017/S1751731115001536
Honey, L. (2013). Assuring the welfare of food animals. Vet. Rec. 173, 568–569. doi: 10.1136/vr.f7319
Ito, K., Weary, D. M., and von Keyserlingk, M. A. G. (2009). Lying behavior: assessing within- and between-herd variation in free-stall-housed dairy cows. J. Dairy Sci. 92, 4412–4420. doi: 10.3168/jds.2009-2235
Knierim, U., and Winckler, C. (2009). On-farm welfare assessment in cattle: validity, reliability and feasibility issues and future perspectives with special regard to the Welfare Quality® approach. Anim. Welfare 18, 451–458.
Krug, C., Haskell, M. J., Nunes, T., and Stilwell, G. (2015). Creating a model to detect dairy cattle farms with poor welfare using a national database. Prev. Vet. Med. 122, 280–286. doi: 10.1016/j.prevetmed.2015.10.014
Landis, J. T., and Koch, G. G. (1977). The measurement of observer agreement for categorical data. Biometrics 33, 159–174. doi: 10.2307/2529310
Main, D. C. J., Barker, Z. E., Leach, K. A., Bell, N. J., Whay, H. R., and Browne, W. J. (2010). Sampling strategies for monitoring lameness in dairy cattle. J. Dairy Sci. 93, 1970–1978. doi: 10.3168/jds.2009-2500
Martin, P., and Bateson, P. (2007). Measuring Behaviour: An Introductory Guide. Cambridge: Cambridge University Press. doi: 10.1017/CBO9780511810893
Mason, G., and Mendl, M. (1993). Why is there no simple way of measuring animal welfare? Anim. Welfare 2, 301–319.
Mendl, M. (1991). Some problems with the concept of a cut-off point for determining when an animal's welfare is at risk. Appl. Anim. Behav. Sci. 31, 139–146. doi: 10.1016/0168-1591(91)90161-P
Mullan, S., Browne, W. J., Edwards, S. A., Butterworth, A., Whay, H. R., and Main, D. C. J. (2009a). The effect of sampling strategy on the estimated prevalence of welfare outcome measures on finishing pig farms. Appl. Anim. Behav. Sci. 119, 39–48. doi: 10.1016/j.applanim.2009.03.008
Mullan, S., Edwards, S. A., Butterworth, A., Whay, H. R., and Main, D. C. J. (2009b). Interdependence of welfare outcome measures and potential confounding factors on finishing pig farms. Appl. Anim. Behav. Sci. 121, 25–31. doi: 10.1016/j.applanim.2009.07.002
Mülleder, C., Troxler, J., Laaha, G., and Waiblinger, S. (2007). Can environmental variables replace some animal-based parameters in welfare assessment of dairy cows? Anim. Welfare 16, 153–156.
Nicol, C., Caplen, G., Edgar, J., Richards, G., and Browne, W. (2011). Relationships between multiple welfare indicators measured in individual chickens across different time periods and environments. Anim. Welfare 20, 133–143.
Nyman, A.-K., Lindberg, A., and Sandgren, C. H. (2011). Can pre-collected register data be used to identify dairy herds with good cattle welfare? Acta Vet. Scand. 53, S8–S8. doi: 10.1186/1751-0147-53-S1-S8
Presi, P., and Reist, M. (2011). Review of methodologies applicable to the validation of animal based indicators of welfare. EFSA Support. Publi. 8:171E. doi: 10.2903/sp.efsa.2011.EN-171
Proudfoot, K. L., Weary, D. M., and von Keyserlingk, M. A. G. (2010). Behavior during transition differs for cows diagnosed with claw horn lesions in mid lactation. J. Dairy Sci. 93, 3970–3978. doi: 10.3168/jds.2009-2767
Randall, L. V., Green, M. J., Chagunda, M. G. G., Mason, C., Archer, S. C., Green, L. E., et al. (2015). Low body condition predisposes cattle to lameness: an 8-year study of one dairy herd. J. Dairy Sci. 98, 3766–3777. doi: 10.3168/jds.2014-8863
Randall, L. V., Thomas, H. J., Remnant, J. G., Bollard, N. J., and Huxley, J. N. (2019). Lameness prevalence in a random sample of UK dairy herds. Vet. Rec. 184, 350–350. doi: 10.1136/vr.105047
Roche, J. R., Friggens, N. C., Kay, J. K., Fisher, M. W., Stafford, K. J., and Berry, D. P. (2009). Invited review: body condition score and its association with dairy cow productivity, health, and welfare. J. Dairy Sci. 92, 5769–5801. doi: 10.3168/jds.2009-2431
Rushen, J., Chapinal, N., and de Passillé, A. M. (2012). Automated monitoring of behavioural-based animal welfare indicators. Anim. Welfare 21, 339–350. doi: 10.7120/09627286.21.3.339
Rushen, J., and Passillé, A. M. B.d. (1992). The scientific assessment of the impact of housing on animal welfare: a critical review. Can. J. Anim. Sci. 72, 721–743. doi: 10.4141/cjas92-085
Rutherford, K. M. D., Langford, F. M., Jack, M. C., Sherwood, L., Lawrence, A. B., and Haskell, M. J. (2009). Lameness prevalence and risk factors in organic and non-organic dairy herds in the United Kingdom. Vet. J. 180, 95–105. doi: 10.1016/j.tvjl.2008.03.015
Sandgren, C. H., Lindberg, A., and Keeling, L. J. (2009). Using a national dairy database to identify herds with poor welfare. Anim. Welfare 18, 523–532.
Schirmann, K., Chapinal, N., Weary, D. M., Heuwieser, W., and von Keyserlingk, M. A. G. (2012). Rumination and its relationship to feeding and lying behavior in Holstein dairy cows. J. Dairy Sci. 95, 3212–3217. doi: 10.3168/jds.2011-4741
Schmidt, R. C. (1997). Managing delphi surveys using nonparametric statistical techniques. Decision Sci. 28, 763–774. doi: 10.1111/j.1540-5915.1997.tb01330.x
Stangaferro, M. L., Wijma, R., Caixeta, L. S., Al-Abri, M. A., and Giordano, J. O. (2016a). Use of rumination and activity monitoring for the identification of dairy cows with health disorders: part I. Metabolic and digestive disorders. J. Dairy Sci. 99, 7395–7410. doi: 10.3168/jds.2016-10907
Stangaferro, M. L., Wijma, R., Caixeta, L. S., Al-Abri, M. A., and Giordano, J. O. (2016b). Use of rumination and activity monitoring for the identification of dairy cows with health disorders: part II. Mastitis. J. Dairy Sci. 99, 7411–7421. doi: 10.3168/jds.2016-10908
Stangaferro, M. L., Wijma, R., Caixeta, L. S., Al-Abri, M. A., and Giordano, J. O. (2016c). Use of rumination and activity monitoring for the identification of dairy cows with health disorders: part III. Metritis. J. Dairy Sci. 99, 7422–7433. doi: 10.3168/jds.2016-11352
Thorup, V. M., Nielsen, B. L., Robert, P.-E., Giger-Reverdin, S., Konka, J., Michie, C., et al. (2016). Lameness affects cow feeding but not rumination behavior as characterized from sensor data. Front. Vet. Sci. 3:37. doi: 10.3389/fvets.2016.00037
Van Os, J. M. C., Winckler, C., Trieb, J., Matarazzo, S. V., Lehenbauer, T. W., Champagne, J. D., et al. (2018). Reliability of sampling strategies for measuring dairy cattle welfare on commercial farms. J. Dairy Sci. 101, 1495–1504. doi: 10.3168/jds.2017-13611
Van Reenen, C. G., O'Connell, N. E., Van der Werf, J. T. N., Korte, S. M., Hopster, H., Jones, R. B., et al. (2005). Responses of calves to acute stress: Individual consistency and relations between behavioral and physiological measures. Physiol. Behav. 85, 557–570. doi: 10.1016/j.physbeh.2005.06.015
van Staaveren, N., Doyle, B., Manzanilla, E. G., Calderón Díaz, J. A., Hanlon, A., and Boyle, L. A. (2017). Validation of carcass lesions as indicators for on-farm health and welfare of pigs. J. Anim. Sci. 95, 1528–1536. doi: 10.2527/jas2016.1180
Vasseur, E., Rushen, J., Haley, D. B., and de Passillé, A. M. (2012). Sampling cows to assess lying time for on-farm animal welfare assessment. J. Dairy Sci. 95, 4968–4977. doi: 10.3168/jds.2011-5176
Veissier, I., Capdeville, J., and Delval, E. (2004). Cubicle housing systems for cattle: comfort of dairy cows depends on cubicle adjustment. J. Anim. Sci. 82, 3321–3337. doi: 10.2527/2004.82113321x
Waiblinger, S., Knierim, U., and Winckler, C. (2001). The development of an epidemiologically based on-farm welfare assessment system for use with dairy cows. Acta Agri. Scand. A Anim. Sci. 51, 73–77. doi: 10.1080/090647001316923108
Walker, S. L., Smith, R. F., Routly, J. E., Jones, D. N., Morris, M. J., and Dobson, H. (2008). Lameness, activity time-budgets, and estrus expression in dairy cattle. J. Dairy Sci. 91, 4552–4559. doi: 10.3168/jds.2008-1048
Weary, D. M., Huzzey, J. M., and von Keyserlingk, M. A. G. (2009). Board-Invited Review: using behavior to predict and identify ill health in animals. J. Anim. Sci. 87, 770–777. doi: 10.2527/jas.2008-1297
Webster, A. J. F., Main, D. C. J., and Whay, H. R. (2004). Welfare assessment: indices from clinical observation. Anim. Welfare 13, 93–98.
Wemelsfelder, F. (2007). How animals communicate quality of life: the qualitative assessment of behaviour. Anim. Welfare 16, 25–31.
Wemelsfelder, F., Hunter, E. A., Mendl, M. T., and Lawrence, A. B. (2000). The spontaneous qualitative assessment of behavioural expressions in pigs: first explorations of a novel methodology for integrative animal welfare measurement. Appl. Anim. Behav. Sci. 67, 193–215. doi: 10.1016/S0168-1591(99)00093-3
Wemelsfelder, F., Hunter, T. E. A., Mendl, M. T., and Lawrence, A. B. (2001). Assessing the ‘whole animal’: a free choice profiling approach. Anim. Behav. 62, 209–220. doi: 10.1006/anbe.2001.1741
Whay, H. R., Main, D. C. J., Green, L. E., and Webster, A. J. F. (2003a). Animal-based measures for the assessment of welfare state of dairy cattle, pigs and laying hens: consensus of expert opinion. Anim. Welfare 12, 205–217.
Whay, H. R., Main, D. C. J., Green, L. E., and Webster, A. J. F. (2003b). Assessment of the welfare of dairy cattle using animal-based measurements: direct observations and investigation of farm records. Vet. Rec. 153, 197–202. doi: 10.1136/vr.153.7.197
Whay, H. R., Webster, A. J. F., and Waterman-Pearson, A. E. (2005). Role of ketoprofen in the modulation of hyperalgesia associated with lameness in dairy cattle. Vet. Rec. 157, 729–733. doi: 10.1136/vr.157.23.729
Wiech, K., and Tracey, I. (2009). The influence of negative emotions on pain: behavioral effects and neural mechanisms. NeuroImage 47, 987–994. doi: 10.1016/j.neuroimage.2009.05.059
Winckler, C. (2014). “Inter-observer agreement for qualitative behaviour assessment in dairy cattle in three different countries,” in WAFL 2014 : Proceedings of the 6th International Conference on the Assessment of Animal Welfare at Farm and Group Level, eds L. Mounier, and I. Veissier. (Wageningen: Wageningen Academic Publishers), 181.
Keywords: animal welfare, farm animals, on-farm welfare assessment, dairy cattle, lameness, qualitative behaviour assessment, iceberg indicators, classification methods
Citation: Collins S, Burn CC, Wathes CM, Cardwell JM, Chang Y-M and Bell NJ (2021) Time-Consuming, but Necessary: A Wide Range of Measures Should Be Included in Welfare Assessments for Dairy Herds. Front. Anim. Sci. 2:703380. doi: 10.3389/fanim.2021.703380
Received: 30 April 2021; Accepted: 11 October 2021;
Published: 17 November 2021.
Edited by:
Harry J. Blokhuis, Swedish University of Agricultural Sciences, SwedenReviewed by:
Sandra Edwards, Newcastle University, United KingdomChristoph Winckler, University of Natural Resources and Life Sciences Vienna, Austria
Copyright © 2021 Collins, Burn, Wathes, Cardwell, Chang and Bell. This is an open-access article distributed under the terms of the Creative Commons Attribution License (CC BY). The use, distribution or reproduction in other forums is permitted, provided the original author(s) and the copyright owner(s) are credited and that the original publication in this journal is cited, in accordance with accepted academic practice. No use, distribution or reproduction is permitted which does not comply with these terms.
*Correspondence: Charlotte C. Burn, Y2J1cm5AcnZjLmFjLnVr