- 1Division of Animal Science and Nutritional Sciences, West Virginia University, Morgantown, WV, United States
- 2Division of Biological and Health Sciences, University of Pittsburgh at Bradford, Bradford, PA, United States
We investigated the changes in the PBMC transcriptome profile of beef steers with divergent residual feed intake (RFI) following in vitro LPS stimulation. Negative-RFI beef steers (n =8, RFI= -2.00) and positive-RFI beef steers (n = 8, RFI = +1.59) were identified from a group of 40 crossbred beef steers (average BW = 360 ± 7.3 kg) after a 56-d RFI testing period. Whole blood samples were collected for PBMC extraction and were stimulated for 2 hours with LPS, followed by total RNA extraction and sequencing. The gene expression profiles of LPS-stimulated PBMCs and the LPS-unstimulated control group from negative- or positive- RFI beef steers were compared and analyzed. Differentially expressed genes were determined using FDR ≤ 0.05. In negative-RFI beef steers, there were 37 differentially expressed genes; the expression of 28 genes such as CD14, TREM1, THBS1, S100A12, S100A8, S100A9, CXCL5, IL1RN, and CCL20 were downregulated, whereas expression of 9 genes including CCL22, CD83, TRAF1, NFKBIZ, RSG16, CD60, and IL17A were upregulated in LPS-stimulated PBMC. In positive-RFI beef steers, we found 9 differentially expressed genes (CCL22, CD83, NFKBIZ, E1BK63, TRAF1, BCL2A1, IFNLR1, RSG16, and CD40), all of which were all upregulated. Gene ontology analysis of the differentially expressed genes revealed the enrichment of biological pathways related to defense and innate immune response, cell migration, and cellular response to lipopolysaccharide in negative-RFI beef steers, characteristic of a prompt and efficient immune reaction. In positive-RFI beef steers, biological processes associated with T cell activation and differentiation, positive regulation of adaptive immune response, and immune cell surface receptors were differentially enriched. Taken together, these findings suggest that negative-RFI beef steers may possess a more competent and energy-conserving immune response, marked by a quicker resolution of inflammation and a balanced pro- and anti-inflammatory response. These results enhance the understanding of the molecular mechanisms underlying feed efficiency, highlighting the potential role of immunocompetence in improving livestock productivity.
Introduction
Increased feed efficiency in ruminants has been of interest within the past decades due to its capability of reducing environmental impacts and increasing producer profitability. Feed efficiency is heavily influenced by factors like environment, diet, and management practices (Terry et al., 2021; Arthur et al., 2001). One of the most notable measures of feed efficiency in beef cattle is residual feed intake (RFI), which is calculated as the difference between actual feed intake and the predicted intake while considering the animal’s size and growth rate (Koch et al., 1963). A negative or low RFI indicates a decrease in feed intake while still achieving the desired average daily gain (ADG), making the animals efficient (Herd and Bishop, 2000). Several factors make up the physiological basis of RFI, some being feed intake amount, metabolism, and activity. Any slight variation in the efficiency of these factors may impact the overall RFI status of animals (Herd and Arthur, 2009; Cantalapiedra-Hijar et al., 2018).
Feed efficiency is a complex trait influenced by multiple physiological and metabolic factors, including immunocompetence and overall health. Understanding the interactions between these factors is essential for improving efficiency in livestock production. Lipopolysaccharide (LPS), a component of the outer membrane of Gram-negative bacteria, is commonly used as an inflammatory immunostimulant in vertebrates due to its ability to elicit an immune response. Exposure to LPS induces significant physiological changes, affecting gene expression, cytokine production, metabolism, and dry matter intake (Nordgreen et al., 2018; Raetz, 1990). Previous studies in pigs have demonstrated that the systemic inflammatory response following LPS stimulation, as well as the expression of genes involved in immune defense and cytokine production, is attenuated in animals with negative residual feed intake (RFI) compared to their positive-RFI counterparts (Liu et al., 2019; Onteru et al., 2013). Additionally, research on beef steers with negative RFI has identified upregulation of molecular mechanisms associated with enhanced energy conversion from feed, suggesting a metabolic advantage in these animals (Taiwo et al., 2022a). These findings highlight the potential link between feed efficiency and immune function, underscoring the need to investigate immune responses in RFI-divergent cattle.
The analysis of the transcriptome profile of peripheral blood mononuclear cells (PBMCs) during an immune response is a well-established approach for evaluating changes in gene expression. This method facilitates the identification of signaling and metabolic pathways influenced by immune activation, thereby enhancing our understanding of disease resistance and host defense mechanisms (Adler et al., 2013). Transcriptomic analyses of PBMCs are particularly valuable as they provide a comprehensive overview of gene expression directly from immune cells, which play a central role in pathogen defense, while also offering insight into the genetic regulation of economically relevant traits such as RFI (Kadarmideen, 2014). Previous studies have investigated the effects of LPS challenge on immune function, metabolism, and gene expression in steers (Lippolis et al., 2017; Kent-Dennis et al., 2020; Ferronato et al., 2024). However, research specifically examining the transcriptome response of PBMCs to LPS in beef cattle with divergent RFI is lacking. Investigating differences in gene expression profiles between RFI-divergent cattle may provide insight into the metabolic efficiency of immune responses, potentially linking immune function with growth performance and productivity. Therefore, we hypothesized that beef steers with divergent RFI would exhibit distinct PBMC transcriptome profiles in response to LPS challenge. The objective of this study was to evaluate the effects of LPS challenge on PBMC transcriptome profile of beef steers with divergent RFI.
Materials and methods
Animals and blood collection
All procedures and research protocols were reviewed and approved by West Virginia University Animal Care and Use Committee (Protocol No. 2204052569). Sixteen crossbred beef steers (8 negative-RFI and 8 positive-RFI) were used to evaluate the effects of in vitro LPS challenge on PBMC transcriptome profile. A total of 40 beef steers (average BW = 360 ± 7.3 kg) were selected for a 56-d RFI testing period. Individual feed intake was measured using the GrowSafe intake nodes (GrowSafe Systems Ltd., 125 Airdrie, Alberta, Canada), and daily body weight (BW) was regressed on time using simple linear regression to calculate initial BW, mid-test BW, and ADG. The difference between the predicted regression value and actual measured value was used to calculate RFI based on the equation reported by Durunna et al. (2011) and Taiwo et al. (2022b). More details of the feeding trial including diet and RFI calculation have been reported in these previous studies. After RFI determination, a total of 16 beef steers were identified with negative (n = 8; RFI = -2.00) and positive (n = 8; RFI = +1.59) RFI, which were then used to evaluate the effects of LPS challenge on PBMC transcriptome profile. After identification, blood samples from the 16 beef steers were collected by jugular venipuncture into 10-mL collection tubes containing EDTA.
Peripheral blood mononuclear cell separation
After collection, blood samples were centrifuged at 700 × g for 30 minutes at RT with a brake of 4 to separate white blood cells (WBCs) as described by Jacobs et al. (2020). Buffy coat from samples was removed using a pipette and mixed with 1 mL (1x) PBS (Fisher Scientific, Hampton, NH). Any remaining red blood cells (RBCs) were removed by adding 3 mL ACK Lysis Buffer (Quality Biological, Gaithersburg, MD), vortexing, and incubating for 5 minutes at RT and resuspended with PBS. To pellet WBCs, tubes were centrifuged at 112 × g for 5 minutes at RT. Cells were suspended with 3 mL PBS and layered over 5 mL of lymphocyte separation media (Corning, Fisher Scientific, Hampton, NH) and were centrifuged at 400 × g for 20 minutes with a brake of 1 so PBMCs could separate. The cells found in the middle layer were removed by pipette and resuspended in 10 mL PBS. Viability of cells was verified to have >80% PBMC, and total cell counts greater than 2 ×106 by using a cell counter (Countess II FL Automated Cell Counter, Thermo Fisher Scientific, Waltham, MA).
In vitro cell stimulation
Peripheral blood mononuclear cells were diluted to 1 ×106 in complete media consisting of RPMI 1640 (Cytiva Hyclone, Fisher Scientific, Hampton, NH) with 0.1% FBS (Corning, Fisher Scientific, Hampton, NH) and penicillin/streptomycin/neomycin antibiotic mixture (Fisher Scientific, Hampton, NH). The cells (500µL) were added to a 24-well round bottom cell culture plate (CellStar, Greiner Bio-one, Monroe, NC) at a concentration of 500,000 cells/well. Four wells were used for each animal, two for treatments and two for controls. We added 500µL LPS from E. coli O111:B4 (20 µg/mL, SKU L4391-1MG; Sigma-Aldrich, St. Louis, MO) diluted in complete media to each treatment well to begin stimulation, and we then added 500µL of complete media to each control well. The cells were incubated for two hours at 37°C and 5% CO2, a 2-hour stimulation window allows for the preliminary analysis of the early immune response triggered by LPS (Kaveh et al., 2012). The cells were removed after incubation, the wells were pooled together for each animal and were immediately processed for RNA isolation.
RNA extraction, sequencing, and statistical analysis
Total RNA was extracted from PBMC samples using the RNeasy Micro Kit (Catalog No. 74004; Qiagen, Germantown, MD), following the manufacturer’s instructions. RNA concentration was measured using a NanoDrop 2000 spectrophotometer (Thermo Fisher Scientific, Waltham, MA), with an A260/A280 ratio between 1.8 and 2.1. The quality of RNA samples was assessed using an Agilent 2100 Bioanalyzer (Agilent Technologies, Santa Clara, CA), ensuring RNA integrity numbers (RIN) above 8.
For library construction, messenger RNA was purified from the total RNA using poly-T oligo-attached magnetic beads. Fragmentation was followed by the synthesis of the first cDNA strand using random hexamer primers, then the second cDNA strand. The library was prepared through end repair, A-tailing, adapter ligation, size selection, amplification, and purification. The quality of the library was checked using Qubit and real-time PCR for quantification, and a bioanalyzer for size distribution detection. The qualified libraries were then sequenced on an Illumina NovaSeq 6000 platform, generating 150 bp paired-end reads per sample, according to the effective concentration and required data output.
Trimming and quality control of raw sequencing data were performed using CLC Genomics Workbench 25.0 (Qiagen, CLC BIO, Aarhus, Denmark) following the procedures described by Cánovas et al. (2014). Briefly, low-quality reads (Q-score ≤ 5), adapter-containing sequences, and reads with more than 10% ambiguous (N) base calls were removed. Quality control was assessed using the NGS quality control tool in CLC Genomics Workbench. High-quality reads were then mapped to the Bos taurus ARS-RCD1.3 reference genome using the RNA-seq analysis algorithm in CLC Genomics Workbench 25.0. Gene expression was quantified using the RNA-seq Analysis tool to evaluate differential gene expression in response to LPS stimulation. Differentially expressed genes (DEGs) were identified based on a False Discovery Rate (FDR) ≤ 0.05. Pathway and functional enrichment analysis of the DEGs was conducted using the SR-plot platform (Tang et al., 2023).
Results
The average RFI values of negative- and positive-RFI beef steers were -2.00 and 1.59 respectively. After sequence read processing, an average of 47,772,815 ± 4,792,832 reads per sample was generated (Supplementary Table 1). The PCA plots showing the distribution of the transcriptome data are shown in Supplementary Figure 1.
PBMC transcriptome without LPS stimulation
We determined the DEGs by comparing LPS-unstimulated PBMCs from both negative- and positive-RFI beef steers. In total, there were 3 DEGs (RPS3A, RBM44, and EDNRB) identified and were all upregulated in negative-RFI beef steers (FDR ≤ 0.05; Table 1). Results of the pathway analysis revealed that these genes are associated with cell development and differentiation (Figure 1).
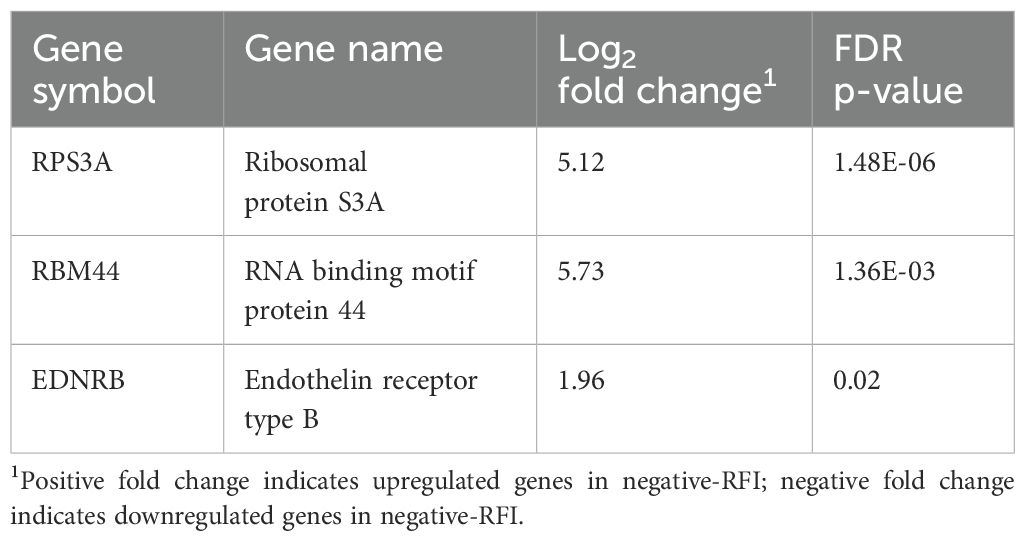
Table 1. Differentially expressed genes in negative or positive-RFI beef steers without LPS stimulation.
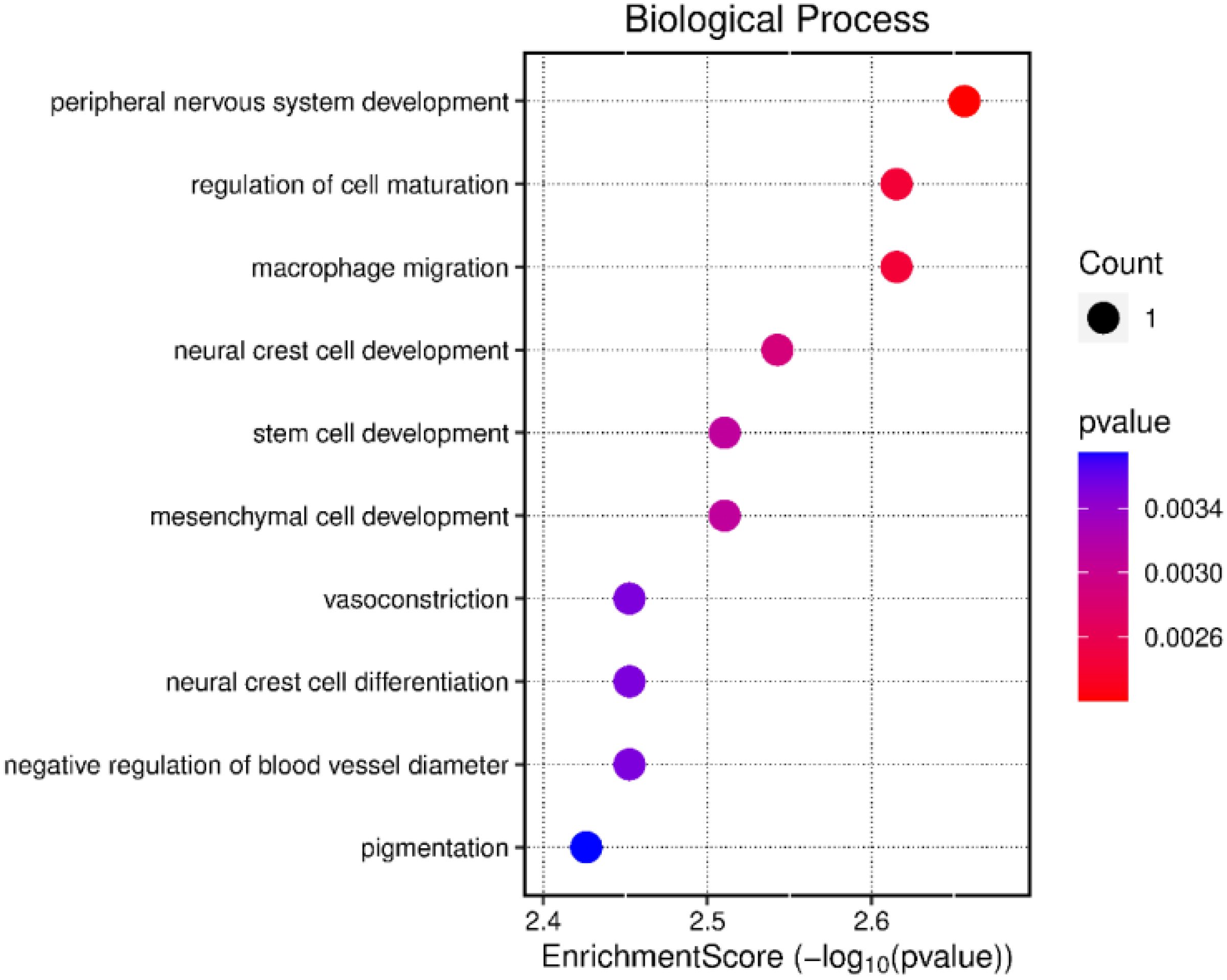
Figure 1. Functional analysis of the differentially expressed genes between beef steers with negative or positive residual feed intake prior to LPS stimulation.
Transcriptomic changes in negative-RFI beef steers following LPS stimulation
The gene expression profiles of LPS-stimulated PBMCs and the LPS-unstimulated control group from negative-RFI beef steers were compared and analyzed. We identified 37 differentially expressed genes (DEGs), with 9 upregulated and 28 downregulated after LPS stimulation (Table 2). Functional analysis revealed that these DEGs were associated with several biological processes including immune responses, defense mechanisms, inflammatory responses, and WBC migration (Figure 2).
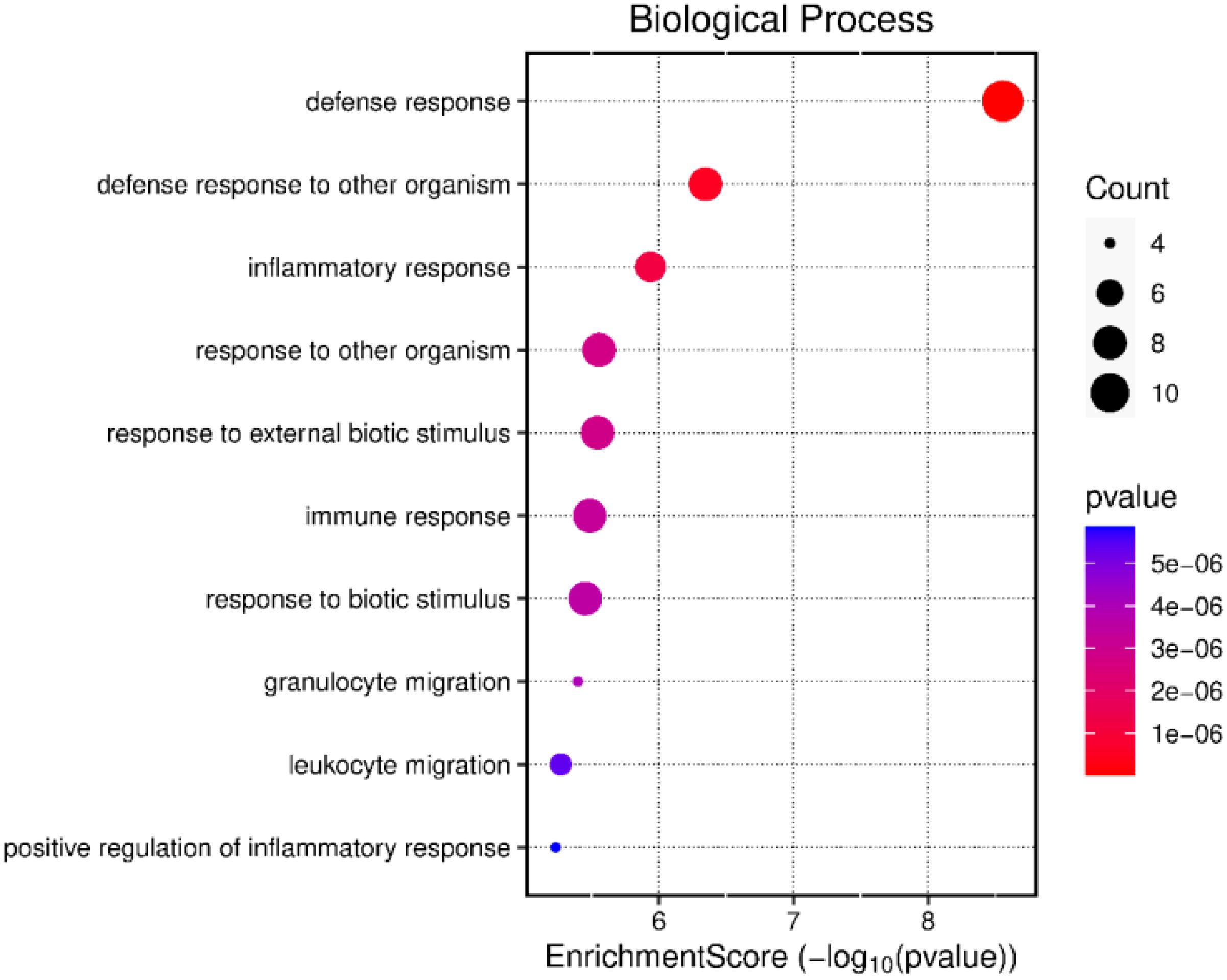
Figure 2. Gene ontology analysis of the differentially expressed genes in negative-RFI beef steers after LPS stimulation.
Transcriptomic changes in positive-RFI beef steers following LPS stimulation
The transcriptomic profiles of LPS-stimulated PBMCs and the LPS-unstimulated control group from positive-RFI beef steers were comparatively analyzed. In total, 9 DEGs were identified, all of which were upregulated after LPS stimulation (Table 3). The GO analysis revealed that the DEGs were primarily associated with biological processes including GTPase activity, T cell activation and differentiation, immune response-related cell surface receptor activation, lymphocyte differentiation, and positive regulation of inflammatory and adaptive immune response (Figure 3). The 5 differentially expressed genes shared by both negative-RFI and positive-RFI groups are CCL22, CD83, TRAF1, NFKBIZ, and RGS16 (Supplementary Figure 2).
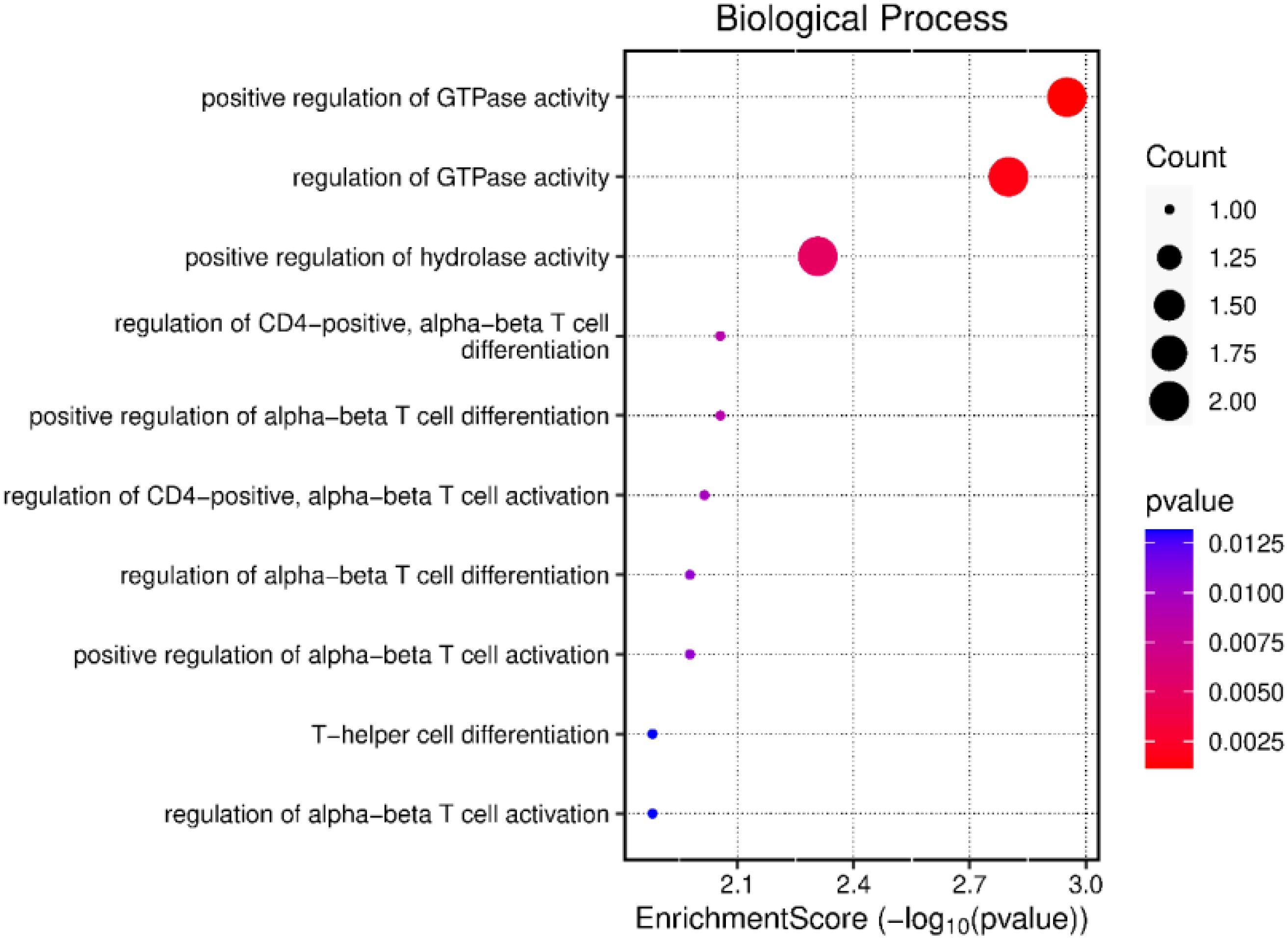
Figure 3. Gene ontology analysis of the differentially expressed genes in positive-RFI beef steers after LPS stimulation.
Discussion
Differentially expressed genes without LPS stimulation
The homeostatic immune response is essential for maintaining and balancing physiological systems, even before pathogen invasion (Davis, 2022). When immune pathways function properly, all physiological processes remain in a steady-state condition. Therefore, metabolic or nutritional status is one of the key factors that determine how defense mechanisms respond to pathogens (Palladino and Colombatto, 2022). In our study, we identified 3 DEGs between the negative and positive RFI—RPS3A, EDNRB, and RBM44. Ribosomal protein S3A (RPS3A) is known to exhibit significant correlations with CD4+ T cells, CD8+ T cells, and macrophages, potentially becoming a key gene involved in pro-inflammatory responses during a disease challenge. As a ribosomal protein gene, RPS3A contributes to the function and structure of ribosomes, as well as the initiation, elongation, and termination phases of protein translation. Studies have reported that this gene plays a critical role in biological functions such as the regulation of cell proliferation, cellular metabolism, and inflammation (Gao and Hardwidge, 2011; Slizhikova et al., 2005). RPS3A is required for LPS signal transduction at the time of inducing pro-inflammatory cytokine production (Li et al., 2018). Thus, the upregulation of this gene in negative-RFI beef steers could also indicate a more competent immune system by preparing the animals to mount a prompt pro-inflammatory response to disease, followed by a steady anti-inflammatory response.
Similarly, upregulation of EDNRB drives the enrichment of various biological processes like cell development and differentiation in negative-RFI PBMCs. EDNRB expression upregulates the production of endothelin receptor type B proteins, which regulate several biological processes including blood vessel development and function, hormone production and stimulation of cell growth and proliferation. In humans, it has been found that reduced expression of EDNRB is associated with proliferation of cancer cells (MedlinePlus, 2022; Wei et al., 2020). RBM44 is well-documented as being enriched in the testes, where it functions as an intercellular bridge component during meiosis and is essential for male fertility (Iwamori et al., 2011). However, its role in PBMCs remains unclear. The observed upregulation in immune cells may indicate a potential involvement in immune modulation, cellular stress response, or metabolic adaptation during inflammation. Further research, including functional validation studies, is needed to elucidate its specific role in PBMCs and its potential implications in immune responses.
Transcriptome changes in PBMCs after LPS stimulation in negative-RFI beef steers
The downregulation of CD14, TREM1, THBS1, S100A12, S100A8, S100A9, CXCL5, IL1RN, and CCL20 was observed in negative-RFI PBMCs after LPS stimulation. Cluster of differentiation 14 (CD14), a co-receptor of Toll-like receptor 4 (TLR-4), can recognize and interact with LPS, pathogen-associated molecular patterns (PAMPs), and damage-associated molecular patterns (DAMPs). Upon the presence of a pathogen, instead of signaling to other cells, CD14 presents LPS to various proteins, including the TLR-4 and MD-2 complex. Consequently, these complexes signal the transcription of pro-inflammatory cytokines to restore the immune system (Na et al., 2023). Consistent with other literature, the stimulation of human monocytes with LPS creates an increase in CD14 expression within 30 minutes, followed by a slow decrease after 1-3 hours. The downregulation of this gene after LPS stimulation could indicate that the PBMCs from the negative-RFI steers were prompt in responding to the challenge. Therefore, by the 2-hour mark post-stimulation, CD14 had already activated the respective inflammatory pathways, resulting in decreased expression (Marchant et al., 1992). TREM1 receptors, located on cell surfaces expressed on myeloid cells, also contribute to this decrease in gene expression. Their ability to amplify the immune response relies on enabling granulocyte and monocyte responses to pathogens. Similarly, THBS1 regulates immune cell function by interacting with T cell surface receptors and acts as a chemoattractant for neutrophils, monocytes, and macrophages to the site of infection. Therefore, a decline in the demand to activate inflammatory pathways from TREM1 and THBS1 consequently decreased CD14 expression (Colonna, 2023; Kaur and Roberts, 2024).
The differential expression of S100A12 was also observed. This protein, expressed by neutrophils and macrophages, supports innate immune responses. Upon LPS stimulation, the activity of this protein induces chemotactic activity, leukocyte recruitment, and activation of intracellular cascades for cytokine production. Literature indicates that high levels of S100A12 in human serum can be used as a marker for chronic inflammatory conditions, and both S100A8 and S100A9 exhibit antimicrobial, pro- and anti-inflammatory properties, suggesting their role in immunity is to contribute to homeostasis even when there is inflammation (Meijer et al., 2012; Wang et al., 2018). The activation of both the innate and adaptive immune systems can be further justified with the presence of different chemokines and cytokines. CXCL5 is a chemokine that uses innate immune cell trafficking to recruit neutrophils to infection sites to regulate host defense. Pro-inflammatory cytokines like TNF-α and IL-1β can activate NF-κB signaling, which increases CXCL5 production. This chemokine is considered a target in liver cancer, with antibodies against it serving as a treatment option that can suppress tumor growth, proliferation, and migration (Xia et al., 2015). In a study conducted by Dervishi et al. (2023), mice treated with subcutaneous LPS demonstrated upregulation of this gene in the spleen. Given that CXCL5 attracts neutrophils to infection sites, these then stimulate the production of CCL20, another essential chemokine involved in signaling immune cell trafficking in immature dendritic cells (Affò et al., 2014). Furthermore, the overexpression of CXCL5 can also be induced by IL-1β; however, because the role of IL-1RN inhibits the binding of IL-1A and IL-1β to the IL-1 receptor, the cellular responses that induce pro-inflammatory reactions are not triggered (Gómez-Flores-Ramos et al., 2014). The downregulation of CXCL5, IL-1RN, and CCL20 elucidates that PBMCs from negative-RFI beef steers activated the innate immune system’s line of defense promptly, hence the expression of these genes declined because they work together to mediate inflammatory responses within 2 hours post-stimulation.
The upregulation of some genes, such as CCL22, CD83, TRAF1, NFKBIZ, RSG16, CD60, and IL17A, was observed in negative-RFI beef steers after LPS stimulation. As previously discussed, CCL20 is necessary for signaling and cell migration, and the production of this chemokine is induced by IL17. This cytokine is essential for host defense against bacteria, and it has been shown to be upregulated in LPS-induced inflammation (Das and Khader, 2017). Literature has found the cross-communication of this interleukin with other innate immune cells to be essential in the switch to adaptive immunity if infection is not cleared. Its upregulation in PBMCs from negative-RFI beef steers demonstrates the effect it has on bridging T cell activation to neutrophil activation and migration (Zenobia and Hajishengallis, 2015). IL17 is a pro-inflammatory cytokine that promotes inflammation and protects against infection. CD4+ and CD8+ T cells can secrete IL17, and although chronic IL17 signaling can turn protective acute inflammation into chronic immunopathology, its function is essential and of interest in the development and progression of disease. Besides jumpstarting strong pro-inflammatory responses, IL17 can express anti-inflammatory cytokines like IL10 to create a balance and link T cell activation to neutrophil mobilization (Zenobia and Hajishengallis, 2015). CD60, which has not been discussed yet, is identified as a T cell marker commonly expressed on the surface of T lymphocytes, whose activation can be triggered through CD60 activity. Upon immune disruption, CD4+ T cells promoted by CD60 have been found to be predominantly efficient in the transformation of B cell differentiation into Ig-secreting cells (Bluth et al., 2005). Wada et al. (1998) analyzed the developmental changes of T cells with CD60 expression and found that this expression was upregulated in memory CD4+ T cells in comparison to memory CD8+ T cells. These memory cells are of utmost importance in the adaptive immune response because of their generation after exposure and their unique antigen recognition capabilities to provide protection upon re-exposure.
The functions of the previously discussed genes overall indicate a strong response from both innate and adaptive immunity to the pathogen. The pathway analysis result of all DEGs in negative-RFI beef steers revealed that these genes are involved in the innate immune response, inflammatory response, and WBC migration. They work together to maintain a balance between pro- and anti-inflammatory reactions with the goal to return to homeostasis. This prevents an excessive pro-inflammatory response, thereby, reducing energy demand and usage, potentially leading to improved feed efficiency to support growth and productivity (Wang et al., 2019). Studies have shown that negative-RFI beef steers have demonstrated improved responses to inflammation, expending less energy towards fighting pathogens compared to beef steers with positive-RFI (Arshad et al., 2024; Ferronato et al., 2024), thus allowing for a more efficient utilization of nutrients for growth (Serna-García et al., 2023). This is further supported by findings from Chen et al. (2011), where an upregulation of genes involved in cell migration and defense response occurs in negative-RFI bulls in comparison to positive-RFI, suggesting a larger biological response to challenges through innate immunity.
Transcriptome changes in PBMCs after LPS stimulation in positive-RFI beef steers
The upregulation of E1BK63, BCL2A1, CD40, and IFNLR1 was observed in positive-RFI PBMCs after LPS stimulation. Increased E1BK63 expression is known to participate in ribosomal assembly and pre-rRNA processing. Alteration to the expression of this gene may affect ribosome translational efficiency. For instance, overexpression of E1BK63 augments global protein translation and has an enhanced impact on the translation of transcripts encoding other ribosomal proteins (Ebright et al., 2020). Ribosomal proteins are involved in an organism’s physiological and pathological processes; they contain positively charged amino acid clusters that interact with the negatively charged bacterial surface of LPS (Chen et al., 2021). As a result, when exposed to LPS, E1BK63 promotes the generation of antimicrobial proteins that aid in clearing the pathogen; however, its overexpression has various implications. Recent studies indicate that upregulation of this gene is associated with cell proliferation in gastric cancer and metastasis in breast cancer (Ebright et al., 2020). Similarly, T helper (Th) cells produce IFN-λ, and IFNLR1 is a cytokine receptor that binds to it, promoting antiviral activity. Research in pigs with porcine reproductive and respiratory syndrome virus (PRRSV) showed an upregulation of IFN-λ after infecting their macrophages. In the presence of a viral infection or inflammation, IFNLR1 expression increases, as does IFN-λ, promoting signal transduction cascades and immunoregulatory functions (Qin et al., 2021). Evidence has shown that overexpression or sustained expression of IFNLR1 increases the amount of CXC chemokines generated in response to IFN-λ. These chemokines are crucial for inflammation and anti-tumor immunity, so their overexpression is positively associated with cancer metastasis, making them potential diagnostic and prognostic biomarkers (Wu et al., 2022).
In addition, the expression of BCL2A1 facilitates the survival of leukocytes to aid in inflammation. Several reports have outlined the importance of its upregulation for B lymphocyte function and survival upon CD40 signaling. Sustained expression of CD40 protects B cells from antigen receptor-mediated apoptosis (Craxton et al., 2000; Vogler, 2012). Altogether, these results indicate there are both direct and indirect effects on the adaptive immune system of the positive-RFI beef steers. When comparing the magnitude of gene upregulation after LPS stimulation in positive-RFI to the negative-RFI group, the differential expression of genes for the recruitment of cells and activation of pathways differs, suggesting that both groups combat the pathogen in different ways.
The pathway analysis result of all the DEGs in positive-RFI beef steers, including NFKBIZ, RGS16, and CD40 revealed that these genes are primarily involved in regulation and activation of the adaptive immune response and T cell proliferation. The innate response is immediate, while the adaptive response takes longer to develop. When the innate immune system is unable to clear pathogens, the adaptive immune system steps in to mount a more targeted and sustained response; however, the adaptive immune system responds more slowly but provides a more specific and long-lasting defense against pathogens, but this comes at the expense of higher energy expenditure than innate immune response. While both the innate and adaptive immune responses require energy to function, the adaptive immune response generally consumes more energy due to the need for extensive cell proliferation, high levels of protein synthesis, metabolic reprogramming, and the formation and maintenance of memory cells (McDade et al., 2016; Sherwood et al., 2022).
Conclusion
The differential response to in vitro LPS stimulation between negative-RFI and positive-RFI beef steers highlights significant variations in their innate and adaptive immune responses. Negative-RFI beef steers exhibited downregulation of genes like CD14, TREM1, THBS1, S100A12, and CXCL5, indicating a prompt and effective activation of their immune pathways, leading to a swift return to homeostasis. This efficient immune response aligns with their improved feed efficiency and nutrient utilization. Conversely, positive-RFI beef steers showed upregulation of genes such as E1BK63, BCL2A1, CD40, and IFNLR1, suggesting a heightened and sustained activation of immune pathways, particularly involving the adaptive immune response. The pronounced gene expression in positive-RFI beef steers points to a more prolonged and energetically costly immune response, potentially impacting their overall growth and productivity. These findings underscore the distinct immunological strategies employed by beef steers with differing RFI profiles, with negative-RFI beef steers demonstrating a more energy-efficient immune response that can support improved growth and productivity. While qPCR validation was not conducted, the transcriptomic data provide a comprehensive overview of gene expression changes in response to the LPS challenge. These findings offer valuable insights into the immune response and metabolic adaptations in RFI-divergent cattle, which can guide future studies incorporating targeted validation approaches.
Data availability statement
The datasets presented in this study can be found in online repositories. The names of the repository/repositories and accession number(s) can be found below: NCBI SRA, accession SAMN43321700.
Ethics statement
The animal study was approved by West Virginia University Animal Care and Use Committee (Protocol No. 2204052569). The study was conducted in accordance with the local legislation and institutional requirements.
Author contributions
YL: Data curation, Formal Analysis, Investigation, Methodology, Writing – original draft, Writing – review & editing. SJ: Data curation, Formal Analysis, Investigation, Methodology, Writing – review & editing. MI: Data curation, Formal Analysis, Investigation, Methodology, Writing – review & editing. GT: Data curation, Formal Analysis, Investigation, Methodology, Writing – review & editing. TS: Data curation, Formal Analysis, Investigation, Methodology, Writing – review & editing. ET: Data curation, Formal Analysis, Investigation, Methodology, Writing – review & editing. DO: Data curation, Formal Analysis, Investigation, Methodology, Writing – review & editing. LG: Funding acquisition, Writing – review & editing. OM: Formal Analysis, Methodology, Software, Writing – review & editing. IO: Conceptualization, Data curation, Formal Analysis, Funding acquisition, Investigation, Methodology, Project administration, Resources, Software, Supervision, Validation, Visualization, Writing – original draft, Writing – review & editing.
Funding
The author(s) declare that financial support was received for the research and/or publication of this article. The research study was funded by the U.S. Department of Agriculture hatch multi-state regional project W-3010.
Conflict of interest
The authors declare that the research was conducted in the absence of any commercial or financial relationships that could be construed as a potential conflict of interest.
The author(s) declared that they were an editorial board member of Frontiers, at the time of submission. This had no impact on the peer review process and the final decision.
Publisher’s note
All claims expressed in this article are solely those of the authors and do not necessarily represent those of their affiliated organizations, or those of the publisher, the editors and the reviewers. Any product that may be evaluated in this article, or claim that may be made by its manufacturer, is not guaranteed or endorsed by the publisher.
Supplementary material
The Supplementary Material for this article can be found online at: https://www.frontiersin.org/articles/10.3389/fanim.2025.1485446/full#supplementary-material
References
Adler M., Murani E., Ponsuksili S., Wimmers K. (2013). PBMC transcription profiles of pigs with divergent humoral immune responses and lean growth performance. Int. J. Biol. Sci. 9, 907–916. doi: 10.7150/ijbs.6769
Affò S., Morales-Ibanez O., Rodrigo-Torres D., Altamirano J., Blaya D., Dapito D. H., et al. (2014). CCL20 mediates lipopolysaccharide induced liver injury and is a potential driver of inflammation and fibrosis in alcoholic hepatitis. Gut 63, 1782–1792. doi: 10.1136/gutjnl-2013-306098
Arshad U., Kennedy K. M., Cid de la Paz M., Kendall S. J., Cangiano L. R., White H. M. (2024). Immune cells phenotype and bioenergetic measures in CD4+ T cells differ between high and low feed efficient dairy cows. Sci. Rep. 14, 15993. doi: 10.1038/s41598-024-66345-x
Arthur P. F., Archer J. A., Johnston D. J., Herd R. M., Richardson E. C., Parnell P. F. (2001). Genetic and phenotypic variance and covariance components for feed intake, feed efficiency, and other postweaning traits in Angus cattle. J. Anim. Sci. 79, 2805. doi: 10.2527/2001.79112805x
Bluth M. H., Norowitz K. B., Chice S., Shah V. N., Nowakowski M., Durkin H. G., et al. (2005). IgE, CD8+CD60+ T cells and IFN-α in human immunity to parvovirus B19 in selective igA deficiency. Hum. Immunol. 66, 1029–1038. doi: 10.1016/j.humimm.2005.07.009
Cantalapiedra-Hijar G., Abo-Ismail M., Carstens G. E., Guan L. L., Hegarty R., Kenny D. A., et al. (2018). Review: Biological determinants of between-animal variation in feed efficiency of growing beef cattle. Animal 12, s321–s335. doi: 10.1017/S1751731118001489
Cánovas A., Rincón G., Bevilacqua C., Islas-Trejo A., Brenaut P., Hovey R.C, et al. (2014). Comparison of five different RNA sources to examine the lactating bovine mammary gland transcriptome using RNA-Sequencing. Sci Rep 4, 5297. doi: 10.1038/srep05297
Chen Y., Gondro C., Quinn K., Herd R. M., Parnell P. F., Vanselow B. (2011). Global gene expression profiling reveals genes expressed differentially in cattle with high and low residual feed intake. Anim. Genet. 42, 475–490. doi: 10.1111/j.1365-2052.2011.02182.x
Chen C., Yuan J., Ji G., Zhang S., Gao Z. (2021). Amphioxus ribosomal proteins RPS15, RPS18, RPS19 and RPS30-precursor act as immune effectors via killing or agglutinating bacteria. Fish Shellfish Immunol. 118, 147–154. doi: 10.1016/j.fsi.2021.09.001
Colonna M. (2023). The biology of TREM receptors. Nat. Rev. Immunol. 23, 580–594. doi: 10.1038/s41577-023-00837-1
Craxton A., Chuang P. I., Shu G., Harlan J. M., Clark E. A. (2000). The CD40-inducible bcl-2 family member A1 protects B cells from antigen receptor-mediated apoptosis. Cell. Immunol. 200, 56–62. doi: 10.1006/cimm.2000.1616
Das S., Khader S. (2017). Yin and yang of interleukin-17 in host immunity to infection. F1000Research 6, 741. doi: 10.12688/f1000research.10862.1
Davis E. (2022). Immunometabolism and inflammation: A perspective on animal productivity. Anim. Front. 12, 5–7. doi: 10.1093/af/vfac060
Dervishi E., Hailemariam D., Goldansaz S. A., Ametaj B. N. (2023). Early-life exposure to lipopolysaccharide induces persistent changes in gene expression profiles in the liver and spleen of female FVB/N mice. Veterinary Sci. 10, 445. doi: 10.3390/vetsci10070445
Durunna O. N., Mujibi F. D. N., Goonewardene L., Okine E. K., Basarab J. A., Wang Z., et al. (2011). Feed efficiency differences and reranking in beef steers fed grower and finisher diets1. J. Anim. Sci. 89, 158–167. doi: 10.2527/jas.2009-2514
Ebright R. Y., Lee S., Wittner B. S., Niederhoffer K. L., Nicholson B. T., Bardia A., et al. (2020). Deregulation of ribosomal protein expression and translation promotes breast cancer metastasis. Science 367, 1468–1473. doi: 10.1126/science.aay0939
Ferronato G., Cattaneo L., Amato A., Minuti A., Loor J. J., Trevisi E., et al. (2024). Residual feed intake is related with metabolic and inflammatory response during the pre-weaning period in Italian Simmental calves. J Dairy Sci. 107, 1685–1693. doi: 10.3168/jds.2023-23617
Gao X., Hardwidge P. R. (2011). Ribosomal protein S3: A multifunctional target of attaching/effacing bacterial pathogens. Front. Microbiol. 2. doi: 10.3389/fmicb.2011.00137
Gómez-Flores-Ramos L., Torres-Flores J., Gallegos-Arreola M. P. (2014). IL1RN (interleukin 1 receptor antagonist). Atlas Genet Cytogenet Oncol Haematol. Available online at: http://atlasgeneticsoncology.org/gene/40953/il1rn-(interleukin-1-receptor-antagonist) (Accessed May 7, 2024).
Herd R. M., Arthur P. F. (2009). Physiological basis for residual feed intake1. J. Anim. Sci. 87, E64–E71. doi: 10.2527/jas.2008-1345
Herd R. M., Bishop S. C. (2000). Genetic variation in residual feed intake and its association with other production traits in British Hereford cattle. Livestock Production Sci. 63, 111–119. doi: 10.1016/S0301-6226(99)00122-0
Iwamori T., Lin Y.-N., Ma L., Iwamori N., Matzuk M. M. (2011). Identification and characterization of RBM44 as a novel intercellular bridge protein. PloS One 6, e17066. doi: 10.1371/journal.pone.0017066
Jacobs J.R., Middleton D., Greiner S.P., Bowdridge S.A. (2020). RNA‐Sequencing of ovine PBMC after exposure to Haemonchus contortus larval antigen. Parasite Immunology 42, e12697. doi: 10.1111/pim.12697
Kadarmideen H. N. (2014). Genomics to systems biology in animal and veterinary sciences: Progress, lessons and opportunities. Livestock Sci. 166, 232–248. doi: 10.1016/j.livsci.2014.04.028
Kaur S., Roberts D. D. (2024). Emerging functions of thrombospondin-1 in immunity. Semin. Cell Dev. Biol. 155, 22–31. doi: 10.1016/j.semcdb.2023.05.008
Kaveh D. A., Whelan A. O., Hogarth P. J. (2012). The duration of antigen-stimulation significantly alters the diversity of multifunctional CD4 T cells measured by intracellular cytokine staining. PloS One 7, e38926. doi: 10.1371/journal.pone.0038926
Kent-Dennis C., Aschenbach J. R., Griebel P. J., Penner G. B. (2020). Effects of lipopolysaccharide exposure in primary bovine ruminal epithelial cells. J. Dairy Sci. 103, 9587–9603. doi: 10.3168/jds.2020-18652
Koch R. M., Swiger L. A., Chambers D., Gregory K. E. (1963). Efficiency of feed use in beef cattle. J. Anim. Sci. 22, 486–494. doi: 10.2527/jas1963.222486x
Li Y.-H., Wang J., Liu Y., Qiu L., Li J.-Z., Hu H.-G., et al. (2018). Esculentoside A specifically binds to ribosomal protein S3a and impairs LPS-induced signaling in macrophages. Int. Immunopharmacol. 54, 254–260. doi: 10.1016/j.intimp.2017.11.018
Lippolis K. D., Cooke R. F., Schubach K. M., Marques R. S., Bohnert D. W. (2017). Effects of intravenous lipopolysaccharide administration on feed intake, ruminal forage degradability, and liquid parameters and physiological responses in beef cattle. J. Anim. Sci. 95, 2859–2870. doi: 10.2527/jas.2017.1502
Liu H., Feye K. M., Nguyen Y. T., Rakhshandeh A., Loving C. L., Dekkers J. C. M., et al. (2019). Acute systemic inflammatory response to lipopolysaccharide stimulation in pigs divergently selected for residual feed intake. BMC Genomics 20, 728. doi: 10.1186/s12864-019-6127-x
Marchant A., Duchow J., Delville J., Goldman M. (1992). Lipopolysaccharide induces up-regulation of CD14 molecule on monocytes in human whole blood. Eur. J. Immunol. 22, 1663–1665. doi: 10.1002/eji.1830220650
McDade T. W., Georgiev A. V., Kuzawa C. W. (2016). Trade-offs between acquired and innate immune defenses in humans. Evolution Medicine Public Health 2016, 1–16. doi: 10.1093/emph/eov033
MedlinePlus (2022). EDNRB gene (Bethesda (MD: National Library of Medicine (US). Available at: https://medlineplus.gov/genetics/gene/ednrb/references.
Meijer B., Gearry R. B., Day A. S. (2012). The role of S100A12 as a systemic marker of inflammation. Int. J. Inflammation2012, 1–6. doi: 10.1155/2012/907078
Na K., Oh B.-C., Jung Y. (2023). Multifaceted role of CD14 in innate immunity and tissue homeostasis. Cytokine Growth Factor Rev. 74, 100–107. doi: 10.1016/j.cytogfr.2023.08.008
Nordgreen J., Munsterhjelm C., Aae F., Popova A., Boysen P., Ranheim B., et al. (2018). The effect of lipopolysaccharide (LPS) on inflammatory markers in blood and brain and on behavior in individually-housed pigs. Physiol. Behav. 195, 98–111. doi: 10.1016/j.physbeh.2018.07.013
Onteru S. K., Gorbach D. M., Young J. M., Garrick D. J., Dekkers J. C. M., Rothschild M. F. (2013). Whole genome association studies of residual feed intake and related traits in the pig. PloS One 8, e61756. doi: 10.1371/journal.pone.0061756
Palladino R. A., Colombatto D. (2022). Immunometabolism in animal production: Building efficiency from health. Anim. Front. 12, 3–4. doi: 10.1093/af/vfac065
Qin M., Chen W., Li Z., Wang L., Ma L., Geng J., et al. (2021). Role of IFNLR1 gene in PRRSV infection of PAM cells. J. Veterinary Sci. 22, e39. doi: 10.4142/jvs.2021.22.e39
Serna-García M., Fonseca L. F. S., Panadero Romero J. J., Carretero Asuncion J., dos Santos Silva D. B., Salatta B. M., et al. (2023). Transcriptome profiling of the liver in nellore cattle phenotypically divergent for RFI in two genetic groups. Animals 13, 359. doi: 10.3390/ani13030359
Sherwood E. R., Burelbach K. R., McBride M. A., Stothers C. L., Owen A. M., Hernandez A., et al. (2022). Innate immune memory and the host response to infection. J. Immunol. 208, 785–792. doi: 10.4049/jimmunol.2101058
Slizhikova D. K., Vinogradova T. V., Sverdlov E. D. (2005). The NOLA2 and RPS3A genes as highly informative markers of human squamous cell carcinoma of lung. Russian J. Bioorganic Chem. 31, 178–182. doi: 10.1007/s11171-005-0024-6
Taiwo G. A., Idowu M., Denvir J., Cervantes A. P., Ogunade I. M. (2022b). Identification of key pathways associated with residual feed intake of beef cattle based on whole blood transcriptome data analyzed using gene set enrichment analysis. Front. Veterinary Sci. 9. doi: 10.3389/fvets.2022.848027
Taiwo G., Idowu M. D., Wilson M., Pech-Cervantes A., Estrada-Reyes Z. M., Ogunade I. M. (2022a). Residual feed intake in beef cattle is associated with differences in hepatic mRNA expression of fatty acid, amino acid, and mitochondrial energy metabolism genes. Front. Anim. Sci. 3. doi: 10.3389/fanim.2022.828591
Tang D., Chen M., Huang X., Zhang G., Zeng L., Zhang G, et al. (2023). SRplot: A free online platform for data visualization and graphing. PLoS ONE 18, e0294236. doi: 10.1371/journal.pone.0294236
Terry S. A., Basarab J. A., Guan L. L., McAllister T. A. (2021). Strategies to improve the efficiency of beef cattle production. Can. J. Anim. Sci. 101, 1–19. doi: 10.1139/cjas-2020-0022
Vogler M. (2012). BCL2A1: The underdog in the BCL2 family. Cell Death Differentiation 19, 67–74. doi: 10.1038/cdd.2011.158
Wada T., Seki H., Konno A., Ohta K., Nunogami K., Kaneda H., et al. (1998). Developmental changes and functional properties of human memory T cell subpopulations defined by CD60 expression. Cell. Immunol. 187, 117–123. doi: 10.1006/cimm.1998.1336
Wang A., Luan H. H., Medzhitov R. (2019). An evolutionary perspective on immunometabolism. Science 363, eaar3932. doi: 10.1126/science.aar3932
Wang S., Song R., Wang Z., Jing Z., Wang S., Ma J. (2018). S100A8/A9 in inflammation. Front. Immunol. 9. doi: 10.3389/fimmu.2018.01298
Wei F., Ge Y., Li W., Wang X., Chen B. (2020). Role of endothelin receptor type B (EDNRB) in lung adenocarcinoma. Thorac. Cancer 11, 1885–1890. doi: 10.1111/1759-7714.13474
Wu T., Yang W., Sun A., Wei Z., Lin Q. (2022). The role of CXC chemokines in cancer progression. Cancers 15, 167. doi: 10.3390/cancers15010167
Xia J., Xu X., Huang P., He M., Wang X. (2015). The potential of CXCL5 as a target for liver cancer – what do we know so far? Expert Opin. Ther. Targets 19, 141–146. doi: 10.1517/14728222.2014.993317
Keywords: animal, immune responses, LPS, feed efficiency, immune cells
Citation: Leal Y, Johnson S, Idowu M, Taiwo G, Sidney T, Treon E, Ologunagba D, Gratz LM, Morenikeji OB and Ogunade IM (2025) PBMC transcriptome changes in beef steers with negative or positive residual feed intake following in vitro LPS stimulation. Front. Anim. Sci. 6:1485446. doi: 10.3389/fanim.2025.1485446
Received: 23 August 2024; Accepted: 25 March 2025;
Published: 22 April 2025.
Edited by:
Mohan Mondal, Eastern Regional Station, IndiaReviewed by:
Amod Kumar, National Bureau of Animal Genetic Resources (NBAGR), IndiaRansom L. Baldwin, United States Department of Agriculture, United States
Copyright © 2025 Leal, Johnson, Idowu, Taiwo, Sidney, Treon, Ologunagba, Gratz, Morenikeji and Ogunade. This is an open-access article distributed under the terms of the Creative Commons Attribution License (CC BY). The use, distribution or reproduction in other forums is permitted, provided the original author(s) and the copyright owner(s) are credited and that the original publication in this journal is cited, in accordance with accepted academic practice. No use, distribution or reproduction is permitted which does not comply with these terms.
*Correspondence: Ibukun M. Ogunade, aWJ1a3VuLm9ndW5hZGVAbWFpbC53dnUuZWR1