1 Introduction
Smart grid technology is an advanced enhancement of the conventional power grid, characterized by improved communication, control, and computing technologies that optimize energy distribution. A smart grid is an improvement on the existing electric grid system, allowing for more intelligent controlling of electricity from the generation point to the consumer. Consequently, the Industrial Control Systems (ICS) of smart grids have become more exposed to cyber risks due to enhanced network integration and digitalization. The complexity of smart grids, with numerous distributed energy resources (DERs), sensors, and interconnected systems, makes the security problem challenging. The need for secure and resilient decentralized smart grids underscores the importance of new approaches that can enable the detection, prevention, and mitigation of cyber-attacks are desirable. Deep learning and smart grid cybersecurity based on decentralization provide a bright outlook, as they enhance the detection of anomaly cases and potential threats while also increasing the overall resilience of the grid.
2 The role of deep learning in cybersecurity
Smart grids represent a major evolution of conventional power grids that employ Information and Communication Technology (ICT) to optimize the delivery of electrical energy. Elements of smart grids include smart meters for consumers, automated distribution networks, and communication networks. Smart grids can be decentralized as it includes multiple DERs such as solar power, windmills, or energy storage systems, which are usually integrated at the edges of the smart grid. Such decentralization introduces additional challenges to the grid's physical structure and also increases the number of vectors through which a cyber threat can penetrate the network (Reuters, 2023). As a result, cybersecurity has emerged as a focal topic to ensure the safe and reliable functioning of smart grids. It has also demonstrated significant success in several contexts, largely due to deep learning's inclusion abilities to extract key features from accessed data (Ghiasi et al., 2023).
A major advantage of deep learning is that models are able to detect the abnormal flow of traffic since they hold knowledge of normal traffic flow patterns (Ortega-Fernandez and Liberati, 2023). Pattern recognition is another important factor, as deep learning networks can identify even complex patterns within a given data. Additionally, deep learning models are scalable, hence making them capable of analyzing large quantities of data produced by, for instance, smart grids (Reda et al., 2022).
3 Cybersecurity challenges
Decentralized smart grids are the most vulnerable because of the localized architecture and extensive connections with IoT gadgets. The different systems used in smart grids are not homogenous and have different protocols, which introduce security weaknesses; hence, the weaknesses are present (Thakkar and Lohiya, 2022). The lack of computational capacity in many IoT devices due to resource constraints precludes such approaches, and traditional security techniques cannot be implemented (Xia et al., 2022).
Despite the potential of deep learning in smart grid cybersecurity, there are a number of issues to address. Concerning a few key points, it is important to mention data confidentiality, as the training data can be considered sensitive. Another issue is the interpretability problem since, unlike traditional machine learning techniques, deep learning models are considered “black box” (Verma and Prakash, 2023). In addition, a limitation of deep learning is that it demands massive computation, which may not be readily feasible in low-power devices of smart grids (Vosughi et al., 2022). There is also a significant issue related to integration with legacy systems, as such systems might be incompatible with deep learning solutions (Esenogho et al., 2022).
4 Future directions
Future research directions encompass the development of smart grids that are decentralized and experience a higher level of risk related to cybersecurity because of the deployment of several IoT devices. Table 1 summarizes robust deep learning approaches for smart grid cybersecurity, highlighting their advantages, challenges, and future directions. While these methods show high accuracy in detecting cyber threats (ranging from 92% to 99.5%), they face issues such as high computational demands, vulnerability to adversarial attacks, and scalability concerns. Future research should focus on improving real-time integration, enhancing model interpretability, and developing more robust AI-driven cybersecurity frameworks as shown in Figure 1.
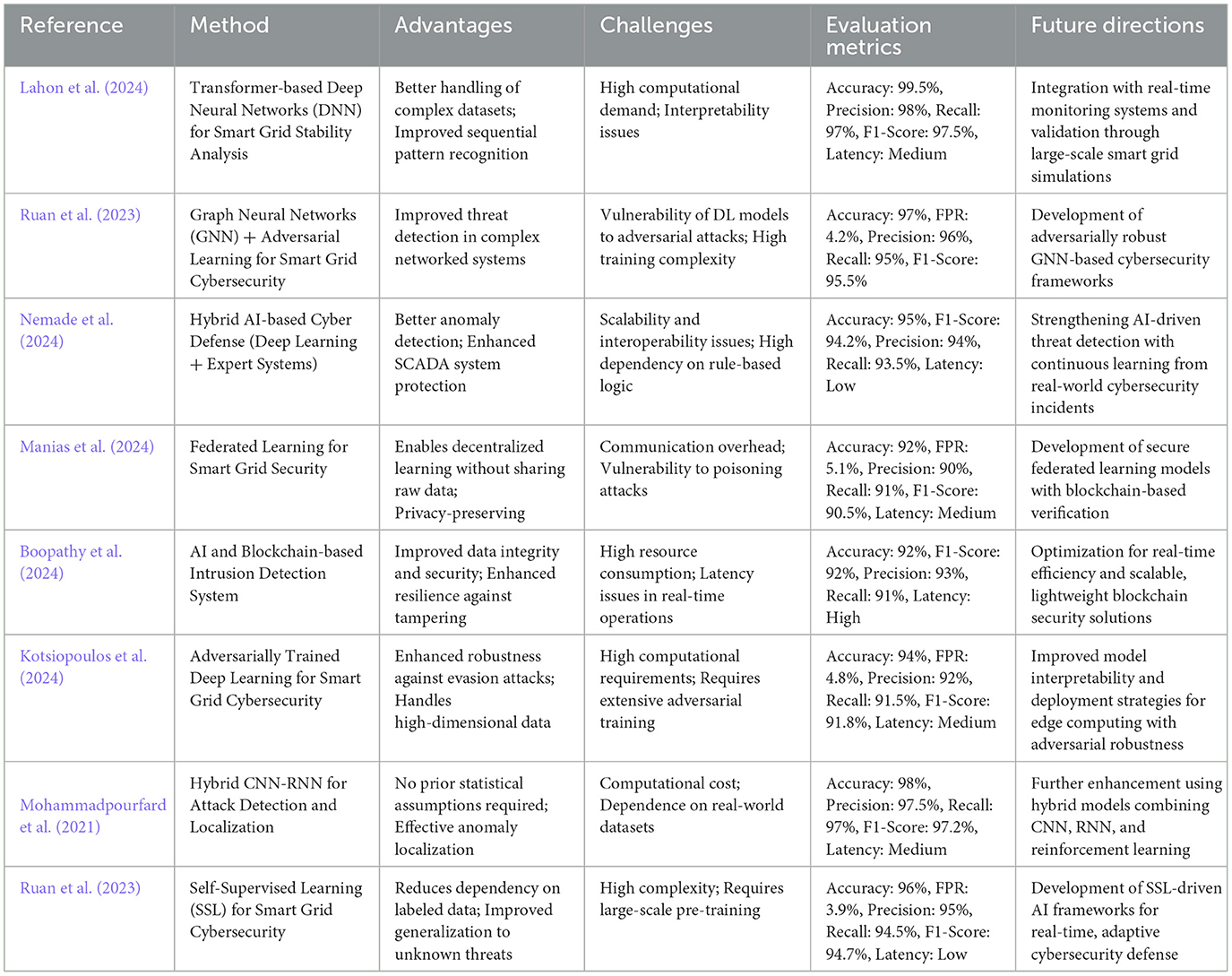
Table 1. Analysis of the robust methods of deep learning for decentralized smart grid cybersecurity.
The most suitable solution for smart grid cybersecurity protection combines federated learning, blockchain, and adversarial deep learning. With FL, grid nodes can participate in decentralized training processes without exchanging the actual data, thereby protecting their information security and privacy. The combination of blockchain technology and adversarial deep learning training creates an unalterable security framework that secures communication while building resistance against complex cyber threats. The implementation of this combined method becomes necessary because smart grids function through decentralized systems that connect numerous vulnerable IoT-enabled energy production networks to cyber security threats. Maximum security models become ineffective because they suffer from dimensional problems, privacy weaknesses, and developing electronic strike threats. Through an integration of FL and blockchain technology, organizations achieve real-time threat detection with adaptive capabilities and lower IT overhead costs. Industrial security in the energy sector needs sophisticated AI-enabled solutions that must scale effectively to defend against infrastructure attacks and disruptions to the power supply. By utilizing this model, organizations maintain autonomous cybersecurity operations that produce efficient proactive threat protection suitable for advanced smart grid systems against developing cyber-attacks.
5 Conclusion
Deep learning in decentralized smart grid cybersecurity is a revolutionary approach of handling vast and dynamic risks. Based on the real-time data processing capabilities and the pattern recognition ability of deep learning models, it is possible to improve anomaly detection density, threat prediction, and system robustness. However, challenges exist in using deep learning in this area, including the need for significant computational power, data security concerns, and difficulties related to the initiation and incorporation of integration of such complex technologies into existing systems. To overcome these challenges, new approaches must be developed more efficiently, with a focus on building effective privacy preservation mechanisms and ensuring seamless integration with other existing systems. Future work should focus on introducing sophisticated and flexible deep learning frameworks for smart grids that can function in a given distributed environment. This will enable the industry to progress and advance toward building a smarter grid that can address novel cyber threats and protect and enhance the reliability of energy distribution systems.
Author contributions
SV: Writing – original draft. AR: Writing – review & editing.
Funding
The author(s) declare that no financial support was received for the research and/or publication of this article.
Conflict of interest
The authors declare that the research was conducted in the absence of any commercial or financial relationships that could be construed as a potential conflict of interest.
Generative AI statement
The author(s) declare that no Gen AI was used in the creation of this manuscript.
Publisher's note
All claims expressed in this article are solely those of the authors and do not necessarily represent those of their affiliated organizations, or those of the publisher, the editors and the reviewers. Any product that may be evaluated in this article, or claim that may be made by its manufacturer, is not guaranteed or endorsed by the publisher.
References
Boopathy, P., Liyanage, M., Deepa, N., Velavali, M., Reddy, S., Maddikunta, P. K. R., et al. (2024). AI and blockchain integration for smart grid security. Comput. Sci. Rev. 51:100617. doi: 10.1016/j.cosrev.2024.100617
Esenogho, E., Djouani, K., and Kurien, A. M. (2022). Integrating artificial intelligence, Internet of Things, and 5G for next-generation smart grid: a survey of trends, challenges, and prospects. IEEE Access 10, 4794–4831. doi: 10.1109/ACCESS.2022.3140595
Ghiasi, M., Niknam, T., Wang, Z., Mehrandezh, M., Dehghani, M., Ghadimi, N., et al. (2023). A comprehensive review of cyber-attacks and defense mechanisms for improving security in smart grid energy systems: Past, present and future. Electr. Power Syst. Res. 215, 108975. doi: 10.1016/j.epsr.2022.108975
Kotsiopoulos, T., Sarigiannidis, P., and Ioannidis, D. (2024). Deep learning for smart grid cybersecurity: challenges and future directions. Comput. Sci. Rev. 40:100341. doi: 10.1016/j.cosrev.2020.100341
Lahon, P., Kandali, A. B., Barman, U., Konwar, R. J., Saha, D., Saikia, M. J., et al. (2024). Deep neural network-based smart grid stability analysis: enhancing grid resilience and performance. Energies 17, 2642. doi: 10.3390/en17112642
Manias, D. M., Saber, A. M., Radaideh, M. I., Gaber, A. T., Maniatakos, M., Zeineldin, H., et al. (2024). “Trends in smart grid cyber-physical security: components, threats, and solutions,” in IEEE Access. Piscataway, New Jersey: IEEE.
Mohammadpourfard, M., Genc, I., Lakshminarayana, S., and Konstantinou, C. (2021). “Attack detection and localization in smart grid with image-based deep learning,” in IEEE International Conference on Communications, Control, and Computing Technologies for Smart Grids (SmartGridComm) Piscataway, New Jersey: IEEE), 121–126
Nemade, B., Kishor Maharana, K., Kulkarni, V., Srivardhankumar, C., and Shelar, M. (2024). Revolutionizing smart grid security: a holistic cyber defense strategy. Front. Artif. Intell. 7:1476422. doi: 10.3389/frai.2024.1476422
Ortega-Fernandez, I., and Liberati, F. (2023). A review of denial of service attack and mitigation in the smart grid using reinforcement learning. Energies 16:635. doi: 10.3390/en16020635
Reda, H. T., Anwar, A., and Mahmood, A. (2022). Comprehensive survey and taxonomies of false data injection attacks in smart grids: attack models, targets, and impacts. Renew. Sustain. Energy Rev. 163, 112423. doi: 10.1016/j.rser.2022.112423
Reuters (2023). Russian Spies Behind Cyber-Attack on Ukraine Power Grid in 2022—Researchers. Canary Wharf: Reuters. Accessed: Jul. 11, 2024. Retrieved online at: https://www.reuters.com/technology/cybersecurity/russian-spiesbehind-cyberattack-ukrainian-power-grid-2022-researchers-2023-11-09/
Ruan, J., Liang, G., Zhao, J., Zhao, H., Qiu, J., Wen, F., et al. (2023). Deep learning for cybersecurity in smart grids: review and perspectives. Energy Conv. Econ. 4, 233–251. doi: 10.1049/enc2.12091
Thakkar, A., and Lohiya, R. (2022). A survey on intrusion detection system: feature selection, model, performance measures, application perspective, challenges, and future research directions. Artif. Intell. Rev. 55, 453–563. doi: 10.1007/s10462-021-10037-9
Verma, S., and Prakash, C. (2023). IoT security against network anomalies through ensemble of classifiers approach. Int. J. Recent Innov. Trends Comput. Commun. 11:54. doi: 10.17762/ijritcc.v11i7s.6976
Vosughi, A., Tamimi, A., King, A. B., Majumder, S., and Srivastava, A. K. (2022). Cyber–physical vulnerability and resiliency analysis for DER integration: a review, challenges and research needs. Renew. Sustain. Energy Rev. 168:112794. doi: 10.1016/j.rser.2022.112794
Keywords: smart grid, artificial intelligence, machine learning, block chain, deep learning
Citation: Verma S and Rao A (2025) A short report on deep learning synergy for decentralized smart grid cybersecurity. Front. Artif. Intell. 8:1557960. doi: 10.3389/frai.2025.1557960
Received: 09 January 2025; Accepted: 24 March 2025;
Published: 09 April 2025.
Edited by:
Suresh Kallam, Jain University, IndiaReviewed by:
Kenneth E. Okedu, Melbourne Institute of Technology, AustraliaDilip Motwani, University of Mumbai, India
Malvinder Singh Bali, Manipal University Jaipur, India
Copyright © 2025 Verma and Rao. This is an open-access article distributed under the terms of the Creative Commons Attribution License (CC BY). The use, distribution or reproduction in other forums is permitted, provided the original author(s) and the copyright owner(s) are credited and that the original publication in this journal is cited, in accordance with accepted academic practice. No use, distribution or reproduction is permitted which does not comply with these terms.
*Correspondence: Saurav Verma, c2F1cmF2dGhlbGVvQGdtYWlsLmNvbQ==