- 1Biomedical Engineering and Health Sciences Department, Faculty of Electrical Engineering, Universiti Teknologi Malaysia, Johor Bahru, Johor, Malaysia
- 2Advanced Diagnostics and Progressive Human Care, Biomedical Engineering and Health Sciences Department, Faculty of Electrical Engineering, Universiti Teknologi Malaysia, Johor Bahru, Johor, Malaysia
- 3Faculty of Computing, Universiti Teknologi Malaysia, Johor Bahru, Johor, Malaysia
- 4Department of Biotechnology, Kulliyyah of Science, International Islamic University Malaysia, Kuantan, Pahang, Malaysia
- 5Research Unit for Bioinformatics and Computational Biology (RUBIC), Kulliyyah of Science, International Islamic University Malaysia, Kuantan, Pahang, Malaysia
Antibodies are naturally produced safeguarding proteins that the immune system generates to fight against invasive invaders. For centuries, they have been produced artificially and utilized to eradicate various infectious diseases. Given the ongoing threat posed by COVID-19 pandemics worldwide, antibodies have become one of the most promising treatments to prevent infection and save millions of lives. Currently, in silico techniques provide an innovative approach for developing antibodies, which significantly impacts the formulation of antibodies. These techniques develop antibodies with great specificity and potency against diseases such as SARS-CoV-2 by using computational tools and algorithms. Conventional methods for designing and developing antibodies are frequently costly and time-consuming. However, in silico approach offers a contemporary, effective, and economical paradigm for creating next-generation antibodies, especially in accordance with recent developments in bioinformatics. By utilizing multiple antibody databases and high-throughput approaches, a unique antibody construct can be designed in silico, facilitating accurate, reliable, and secure antibody development for human use. Compared to their traditionally developed equivalents, a large number of in silico-designed antibodies have advanced swiftly to clinical trials and became accessible sooner. This article helps researchers develop SARS-CoV-2 antibodies more quickly and affordably by giving them access to current information on computational approaches for antibody creation.
1 Introduction
The Coronavirus Disease 2019 (COVID-19) pandemic, which is caused by the SARS-CoV-2 virus (Severe Acute Respiratory Syndrome Coronavirus 2), has already claimed the lives of approximately 6.8 million people so far and as of right now, there is no effective therapy for COVID-19 as the virus is emerging (Infectious Diseases Society of America, 2024). To control the disease progression, various types of antiviral drugs (Al-Tawfiq et al., 2020; Beigel et al., 2020; Gordon et al., 2021; Arbel et al., 2022) and antibodies (Safarzadeh Kozani et al., 2022; Keam, 2022) were prescribed to COVID-19 patients. Although antibodies offer protection with higher specificity against SARS-CoV-2 than drugs but their limitations point out the challenges in developing sustainable antibodies in the phase of rapid viral evolution (Van Regenmortel, 2014).
COVID-19 therapeutic antibodies developed to target the key components of SARS-CoV-2, Spike (S) protein, which interacts with ACE2 receptor protein on the cells in the respiratory tract during viral invasion (Pizzato et al., 2022). However, continuous structural changes of S protein of SARS-CoV-2 caused by rapid mutations render the effectiveness of the therapeutic antibodies. The antibodies which have been approved by EUA to be prescribed for COVID-19 patients, lost the approval as the mAb is no longer effective against currently emerging SARS-CoV-2 (Orders, 2022; Keam, 2022).
In this case, in silico technology paves promising approaches to design antibodies with our desired formats and customize the residues that favor higher binding affinity and good developability in a shorter time frame (Wolf Pérez et al., 2022). According to Moore, the phrase “in silico” refers to computer-assisted experimental procedures used in modern research (Moore, 2021). The integration of in silico technology into pharmaceutical research, notably in antibody designing, offers a sustainable approach and complementary avenue to traditional experimental methods that facilitates efficient antibody discovery for SARS-CoV-2 while conserving time and resources (Jabalia et al., 2021; Shaker et al., 2021; Ivanov et al., 2023).
2 Antibody discovery using in silico technology
Existing therapeutic antibodies for SARS-CoV-2 were discovered in laboratory through various approaches that involves in vitro technology. Hybridoma technology (Köhler and Milstein, 1975) and phage display (Smith and Petrenko, 1997) are employed to produce antibodies for SARS-CoV-2 with a wide range of application (Antipova et al., 2020; Kim et al., 2022; Somasundaram et al., 2020; Wang et al., 2023). Despite having many benefits to producing mAbs, in vitro technology poses limitations in terms of expenses as the methods mentioned above require sophisticated and resource-intensive high-throughput screening and characterization processes, which also consume adequate time (Moraes et al., 2021). In this case, in silico technology complements in vitro technology and can overtake several stages of conventional antibody discovery methods.
In silico antibody discovery comprises a multi-staged computational approach that accelerates the precision of antibody development. The process begins with the analysis of antibody sequences extracted from databases such as Protein Data Bank (PDB) (Bernstein et al., 1977), UniProt (UniProt Consortium, 2015) and other specified databases listed in Table 2. Modeling of 3D antibody structure is performed using predictive computational tools after sequence analysis to generate structural models with detailed spatial analysis. The next stage involves the evaluation of antibody interaction with targeted antigens through molecular docking. In this stage, high-affinity antibody candidates can be identified by predicting their interaction profiles. Finally, the developability of the antibodies will be evaluated via molecular dynamic simulation since the simulation refines the antibody-antigen complexes by examining their manufacturability. In recent times, in silico approach has been used widely in producing potential therapeutic options for COVID-19 through computational tools as presented in Table 1. In silico technology has been applied into SARS-CoV-2 antibody discovery in various stages of the process. Computational tools that can be used in different stages of SARS-CoV-2 antibody discovery are listed in Table 2.
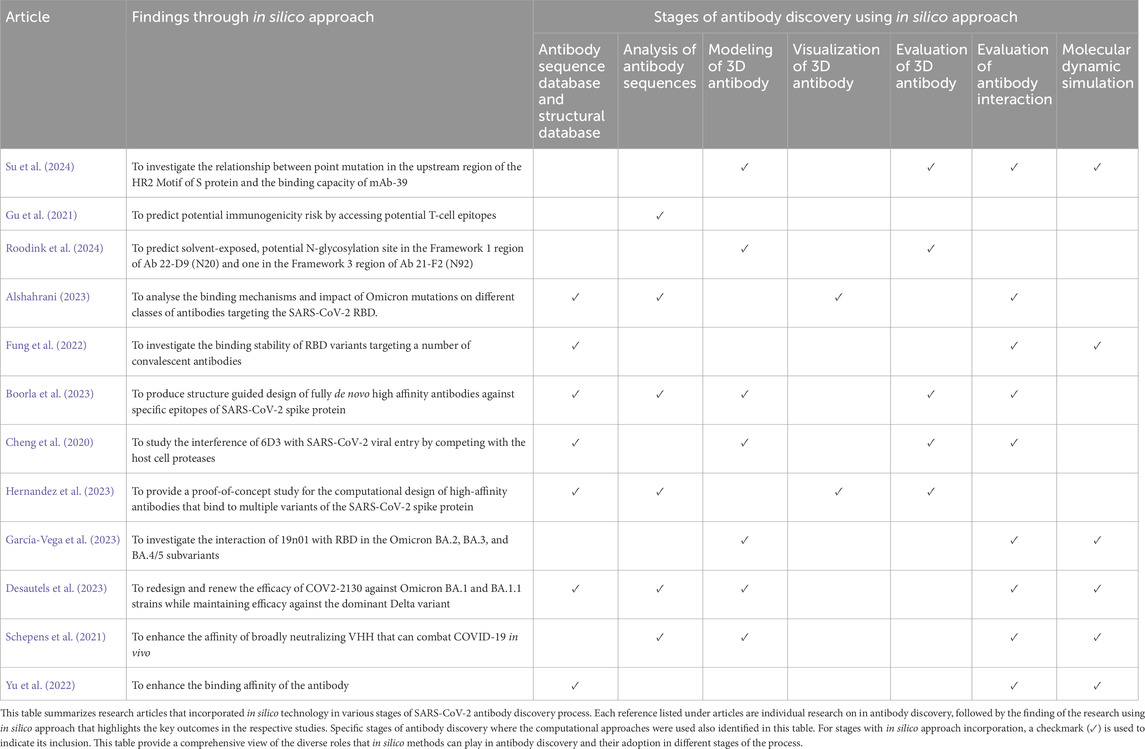
Table 1. Overview of in silico technology application in producing potential therapeutic options for COVID-19.
2.1 Analysis of antibody sequences
Sequences of antibody discovered as therapeutic option for COVID-19 are required to be analyzed before subjecting the sequence for further analysis. Since all variable domains fold into a series of beta strands joined by loops in a very similar 3D shape, the complementarity-determining regions (CDRs) are six of these loops at the top, where these regions develop loops that extend from the surface of the antibody, will result in direct contact with the antigen (Davies and Chacko, 1993). Numbering each residue according to a conventional approach is very helpful for sequence comparisons and engineering due to the continuity of the antibody structural similarity. Precise identification and characterization of these antibody regions are crucial in development and modification of antibodies (Patel et al., 2023). These annotated CDRs establish a significant degree of variation in antibody structure (Wong et al., 2019). Hence, it is critical for recognizing CDR to ensure its binding to a specific antigenic molecule before posing modifications to the antibody.
Numbering schemes with different approaches and set of applications have been developed to standardize the annotation of CDRs. An early yet widespread approach for annotating CDRs is the Kabat numbering scheme, which detects hypervariable regions and relies on the antibody sequences alignment (Kabat, 1991). The 3D structure of the antibodies is the foundation of Chothia numbering scheme (Chothia and Lesk, 1987) which emphasizes the structural locations of CDRs and the protected framework areas that sustain them. An enhanced version of the original Chothia scheme, the Martin scheme, introduces more structural insights and improves the numbering to cover a greater number of spots (Martin and Thornton, 1996), however, it has not been widely utilized. The well-established and comprehensive IMGT numbering scheme, annotates immunoglobulin and T cell receptors (Lefranc et al., 2015). It offers a standardized framework for comparing different species by ensuring consistency across species and antibody types by defining CDRs using both sequence and structural data.
Immunogenicity of the antibody sequences is also predicted to assess the immunogenic response of the therapeutic antibody which ensures safety and effectiveness. Immunogenicity prediction analysis helps in determining whether the antibody sequences exhibit low immunogenicity by identifying significant epitopes and ensuring that they fall below thresholds associated with strong immune activation. These antibodies can enhance their feasibility and reduce detrimental immune responses in various patient populations (Harris and Cohen, 2024).
ANARCI (Dunbar and Deane, 2015), an online tool that offers to annotate variable domains of antibodies from various species, enabling precise identification of CDRs and their alignment for immunogenicity analysis, is widely used in several SARS-CoV-2 studies (Wang et al., 2022; Xu et al., 2021; Zhou et al., 2023). Antibody region-specific alignment (AbRSA) (Li et al., 2019), is also a platform to perform sequence analysis by delimiting the CDRs and antibody numbering for numerous antibodies targeting viral particles (Dănăilă and Buiu, 2022; Dzimianski et al., 2023; Singh et al., 2023).
2.2 Modeling of 3D antibody
The successive unfolding process of protein folding transforms the protein sequences of the SARS-CoV-2 binding antibodies, which are mostly composed of a linear sequence of amino acids, into a functional three-dimensional antibody structure (Poluri et al., 2021). The arrangement of the amino acids determines its basic structure. From this linear arrangement, localized folding results in the formation of secondary structures including alpha helices and beta sheets, which are fuelled by hydrogen bonds between adjacent amino acids. The intricate three-dimensional tertiary structure is its repercussions of the continuous folding of the secondary structure together with the inclusion of loops and turns of the antibody (Rehman et al., 2022).
Protein folding analysis provides many useful insights about the interaction of the antibody especially through identifying the structure of CDR loop formations, but this multifaceted process requires expensive and specialized equipment, making it a challenging task before computational tools are being employed (Benjin and Ling, 2020; Brito and Archer, 2020). But as time passes, using in silico technology, where protein modeling has allowed for generally reliable predictions to be made (Srivastava et al., 2018). The goal of protein modeling is to make use of a range of computer methods to analyze amino acid sequences to predict the three-dimensional (3D) structure of the antibody sequences. Protein modeling provides distinctive approaches for predicting protein structures through a variety of tools that has been included on Table 2, which uses the protein sequences as an input (Agnihotry et al., 2022).
AlphaFold2 (Cheung et al., 2023; Ruff and Pappu, 2021; Skolnick et al., 2021) produces remarkably accurate 3D structure predictions using a neural network architecture that has been trained on a large database of structural and protein sequence data. This tool is utilized in various SARS-CoV-2-related studies that explore the binding behavior of its structural proteins (Ali and Caetano-Anollés, 2024; Jiao et al., 2023; Raisinghani et al., 2024). There are also several studies on the structural analysis of antibodies that prove the modeling capability of AlphaFold2 for antibody sequences (Du and Huang, 2023; Yin et al., 2022). SWISS-MODEL (Schwede et al., 2003) utilizes a homology-modeling approach that is performed iteratively until a satisfactory model structure is obtained. 3D structures of SARS-CoV-2 antibodies (Schepens et al., 2021; Beshnova et al., 2022) were successfully determined through this tool. MODELLER (Šali and Blundell, 1993) is a 3D modeling standalone tool, used to predict the 3D structure of SARS-CoV-2 antibodies (Mercurio et al., 2021; Yang et al., 2021) and restore missing residues in its structure (Martí et al., 2022; Giron et al., 2020). RoseTTAFold is one of the modeling tools that uses neural network-based techniques, incorporating connection between sequences, atomic coordinates, residue-residue orientations, and distances. This tool has been used in several studies on SARS-CoV-2 antibody discovery (Ford et al., 2022; Jing et al., 2024; Lubin et al., 2021). ABodyBuilder (Leem et al., 2016) is an antibody modeling software that incorporates multiple tools, including ABangle (Dunbar et al., 2013) and FREAD (Choi and Deane, 2010). Since this tool is specialized for antibody modeling, numerous studies employed ABodyBuilder to model the variable region of antibodies (Das et al., 2022; Das et al., 2023; Rouet et al., 2023) which also includes bispecific antibody (Ojha et al., 2022).
2.3 Evaluation of antibody interaction
The specificity of a novel or pre-existing antibody of SARS-CoV-2 can be accessed through validation in silico using computational tools. The binding properties of an antibody are primarily determined by the sequence and structure of CDRs through molecular docking. Molecular docking is performed using the analyzed and modeled 3D antibody structures to study the interaction by predicting the preferred orientation, affinity, and interaction of an antibody-antigen complex by analyzing intermolecular interactions (Koçer and Çelik, 2024).
Molecular docking is a process that anticipates atomic-level molecular interactions (Agu et al., 2023). Molecular docking can be performed with various types of biological molecules which include small molecules such as drugs, metabolites, ligands, inhibitors, ions (Jarad et al., 2023; Noreen et al., 2023), and complex molecules that comprise DNA, RNA, proteins, peptides, carbohydrates, nucleosides (Aziz et al., 2023; Madku et al., 2023; Weng et al., 2020). According to research by (Shahmirzaie et al., 2020), molecular docking has proven its capability of being a pioneering analysis to validate biological model interaction by providing binding site information. In the process of validation of antibody binding, molecular docking helps in predicting the preferred orientation of an antibody to the targeted antigen when these molecules are bound to each other to form a stable complex (Gaudreault et al., 2023).
Binding of an antibody exhibits both rigid and flexible properties which is essential for efficient antigen recognition and immune response (Fernández-Quintero et al., 2020). Electrostatic interactions and complementary structures lead to a relatively rigid and specific binding between the paratope and epitope where the rigidity ensures high-affinity binding and specificity (Zeng et al., 2023). On the other hand, the antibody also exhibits flexibility that facilitates conformational changes in the antigen and antibody. Flexibility allows the antibody to bind to a wide range of epitopes and identify antigens with various conformations by allowing it to accommodate variations in the antigen structure (Kilambi and Gray, 2017). An induced-fit mechanism takes place in binding conditions, where the conformational changes between the antigen and antibody are made upon binding to enhance their interactions. The flexibility of an antibody allows it to adapt to the structural alterations in the antigen and improves binding affinity (Bekker et al., 2020). In general, an antibody requires dynamic equilibrium between rigid and flexible phases upon its binding to the antigen.
RosettaDock is a docking approach that optimizes both rigid-body orientations and side-chain conformation (Lyskov and Gray, 2008). RosettaDock is used to perform docking of nanobodies against SARS-CoV-2 receptor-binding domain (RBD) (Yang et al., 2021), monoclonal antibodies against rare antigenic site of SARS-CoV-2 spike protein (Suryadevara et al., 2024) and a specific antibody against SARS-CoV-2 spike protein to improvise the binding affinity (Neamtu et al., 2023). ZDOCK uses Fast Fourier Transform (FFT) to yield less clash penalty in docking (Chen et al., 2003). Several studies employed ZDOCK to study the SARS-CoV-2 antibody-antigen interaction (Khan et al., 2020; Nath et al., 2021). HawkDock is an unique docking tool with integration of the ATTRACT docking algorithm and the MM/GBSA free energy that allows determination of antibody-antigen binding precisely (Weng et al., 2019). Docking is performed through this tool with nanobodies and therapeutic antibodies for interaction analysis (Shah and Woo, 2022; Yang et al., 2024). ClusPro is a widely used docking tool that has benchmarked against alternative docking tools in Critical Assessment of Predicted Interactions (CAPRI) studies (Kozakov et al., 2017). This tool employed to study the binding properties of SARS-CoV-2 spike protein RBD with nanobodies (Shang et al., 2024) and SARS-CoV-2 spike protein with monoclonal antibodies (Nath et al., 2021). High Ambiguity Driven Docking Approach (HADDOCK) harnesses biochemical and biophysical interaction data, including mutagenesis or chemical shift perturbation data from NMR titration experiments to obtain near-native results. Binding prediction of the antibodies discovered with the targeted site on SARS-CoV-2 is performed in several studies using this tool (Ford et al., 2022; Ford et al., 2023).
2.4 Developability evaluation of antibody
The developability of antibody models discovered using in silico approach for COVID-19 will be studied and validated as they can aligned with the real-time experimentally produced therapeutic antibodies. Molecular dynamics (MD) simulations offer a dynamic and comprehensive understanding of biomolecular behavior at the atomic level, and have developed to be an essential tool in the study of computational biophysics (Lemm et al., 2021). In the field of antibody design, MD simulations have shown to be very helpful as a reliable means of testing in silico designs, bridging the gap between computational predictions and experimental findings by providing insights into the structures.
MD simulations operate based on the basic principles of classical mechanics, which make use of Newton’s equations of motion to predict the motions of individual atoms in a molecular system (Shukla and Tripathi, 2020). MD simulations accurately depict the interactions between atoms, including the flexibility of bonds, angle bending, and non-bonded interactions such as van der Waals forces and electrostatics, by applying a force field, a mathematical model that defines the potential energy of the system (Badar et al., 2022). The force field selection is essential to the precision and dependability of MD simulations since it significantly impacts the simulation outcomes. Numerous force fields with unique strengths and applications have been developed over time. CHARMM force field is one of the most common and extensible force fields in computational chemistry which operates exceptionally well to simulate lipids, proteins, and nucleic acids (Brooks et al., 2009). AMBER force field is particularly utilized for proteins and nucleic acids (Wang et al., 2004). The goal of AMBER is to supply precise parameter sets for biomolecular systems. The temporary conformational state of antibody binding is not always visible in static crystal structures but only can be revealed by MD simulations. Accurate parameterization of these forcefields in MD simulation play pivotal roles in comprehending the principles underlying antibody binding and refining antibody architectures to enhance their affinity and specificity for target antigens (Shaw et al., 2010).
GROMACS, an open-source software package designed for molecular dynamics simulations of biochemical molecules including proteins, acts as an in silico to study the behavior of antibody and antibody-antigen complexes at the atomic level (Berendsen et al., 1995; Van Der Spoel et al., 2005). The stability of various SARS-CoV-2 antibody-antigen complexes, including complexes involving the SARS-CoV-2 S protein and bispecific antibodies, as well as the SARS-CoV-2 S protein trimer with monoclonal antibodies, was assessed by measuring the root-mean-square fluctuation (RMSF) of the complexes to quantify dynamic stability (Ford et al., 2022; Ford et al., 2023).
3 Discussion
The global response to the SARS-CoV-2 outbreak has emphasized the critical necessity of quick therapeutic progress. Handling SARS-CoV-2 live virus necessitates adherence to Biosafety Level 3 (BSL-3) laboratory standards as SARS-CoV-2 can be transmitted by air that can lead to respiratory transmission (Kaufer et al., 2020). Compliance with the biosafety regulations of BSL-3 adds to the time and cost of research as it requires a list of facilities and personal protective equipment (Loibner et al., 2021). In this case, in silico approach have grown to be valuable in antibody discovery of SARS-CoV-2.
The usage of computational tools complements various parts of the experimental approach of antibody discovery for SARS-CoV-2. The process of discovering new antibodies necessitates creating antibody libraries consisting of a pool of antibodies featuring various binding sites and screening them to select the antibody candidates with the best binding affinities (Kelley, 2020). Thus, the usage of molecular docking streamlines the process by cutting down the necessity to use experimental approach, which includes handling SARS-CoV-2 antigen or virus for repeated screening (Alshahrani, 2023; Boorla et al., 2023; Gaudreault et al., 2023).
Molecular dynamic simulation bridges the gap between the in silico-developed antibodies and experimentally produced antibodies by mimicking the near-native condition of the antibody (Jandova et al., 2021). Researchers can minimize the repeated usage of live SARS-CoV-2 virus and other experimental assays as these simulations reduce the dependence on experimental assessments while retaining a high level of accuracy (Jairajpuri et al., 2021). Determination of antibody 3D structure is also one of the most essential contributions of in silico approach in antibody discovery. 3D modelling is a useful complement to approaches such as cryo-electron microscopy (cryo-EM) and X-ray crystallography for predicting the three-dimensional structure of antibodies. Computational modeling of 3D structure of the antibodies offers a cost-effective alternative, as the equipment required for the conventional approach is expensive to acquire and maintain (Benjin and Ling, 2020; Brito and Archer, 2020).
Although implementation of in silico approach in SARS-CoV-2 antibody discovery significantly reduce the time and resource investments, transitioning from in silico predictions to experimentally validated antibodies present a few limitations. Biological systems are inherently complex, and in silico models often oversimplify these intricacies. Although in silico approaches can predict the near-native structure and conditions of antibodies, it unable to capture the complexity of the biological system such as glycosylation (Kashkooli et al., 2021). Hence, developing integrated workflows that combine in silico predictions with experimental validation can optimise the transition between these stages.
Moreover, the effectiveness of in silico tools heavily depends on the availability of high-quality training data. Rapid evolution of SARS-CoV-2 has resulted in limited repositories of updated experimentally validated sequences and structural data in public databases (Chen et al., 2022). Limited availability of the information may hinder the accuracy of the computational tools and the accuracy of the computational predicts is compromised by this shortage of data. Expansion of these databases and providing quality training datasets for computational tools are critical steps that enhance the performance of in silico tools (Norman et al., 2019; Khuat et al., 2024).
4 Conclusion
Antibody development is anticipated to accelerate at the greatest pace in upcoming years in life sciences, particularly in the fight against infectious diseases such as SARS-CoV-2. Researchers will be able to construct antibodies precisely but effortlessly due to the developments in bioinformatics and computer modeling. The in silico approach simplifies the process of antibody structure prediction and interaction analysis by providing a molecular dynamic simulation approach for validation. This method greatly improves the speed, economic performance, as well as effectiveness of the process of developing novel therapeutic antibodies. Although precision of computational assessments is reliant upon existing data and models, in silico technologies offer a quick and efficient means of prevention and treatment, that significantly reduce the worldwide burden of this infectious disease. The approaches are also having potential to resurface our knowledge of the immune system and antigen-antibody interaction advances. Overall, the idea of creating antibodies through in silico design has huge implications for the future prevention and management of SARS-CoV-2 and other infectious diseases.
Author contributions
TS: Conceptualization, Writing–original draft, Writing–review and editing. SM: Conceptualization, Funding acquisition, Supervision, Writing–review and editing. WC: Supervision, Writing–review and editing. KA: Supervision, Validation, Writing–review and editing.
Funding
The author(s) declare that financial support was received for the research, authorship, and/or publication of this article. The research is funded by Ministry of Higher Education under Fundamental Research Grant Scheme (FRGS) No: FRGS/1/2022/SKK06/UTM/02/3.
Acknowledgments
The authors would like to thank Ministry of Higher Education for providing financial support under Fundamental Research Grant Scheme (FRGS) No: FRGS/1/2022/SKK06/UTM/02/3. The title of the FRGS grant: Elucidation of antiviral properties of SARS-CoV-2 membrane and envelope proteins recombinant diabody. The present study is a review for the methodology of antibody discovery using in silico technology.
Conflict of interest
The authors declare that the research was conducted in the absence of any commercial or financial relationships that could be construed as a potential conflict of interest.
Generative AI statement
The author(s) declare that no Generative AI was used in the creation of this manuscript.
Publisher’s note
All claims expressed in this article are solely those of the authors and do not necessarily represent those of their affiliated organizations, or those of the publisher, the editors and the reviewers. Any product that may be evaluated in this article, or claim that may be made by its manufacturer, is not guaranteed or endorsed by the publisher.
References
Agnihotry, S., Pathak, R. K., Singh, D. B., Tiwari, A., and Hussain, I. (2022). “Chapter 11 - protein structure prediction,” in Bioinformatics. Editors D. B. Singh, and R. K. Pathak (Academic Press).
Agu, P. C., Afiukwa, C. A., Orji, O. U., Ezeh, E. M., Ofoke, I. H., Ogbu, C. O., et al. (2023). Molecular docking as a tool for the discovery of molecular targets of nutraceuticals in diseases management. Sci. Rep. 13, 13398. doi:10.1038/s41598-023-40160-2
Ali, M. A., and Caetano-Anollés, G. (2024). AlphaFold2 reveals structural patterns of seasonal haplotype diversification in SARS-CoV-2 spike protein variants. Biology 13, 134. doi:10.3390/biology13030134
Alshahrani, M. R. (2023). Computational analysis of antibody binding mechanisms to the omicron RBD of SARS-CoV-2 spike protein: identification of epitopes and hotspots for developing effective therapeutic strategies. Orange, California, USA: Chapman University Digital Commons.
Al-Tawfiq, J. A., Al-Homoud, A. H., and Memish, Z. A. (2020). Remdesivir as a possible therapeutic option for the COVID-19. Travel Med. Infect. Dis. 34, 101615. doi:10.1016/j.tmaid.2020.101615
Antipova, N. V., Larionova, T. D., Siniavin, A. E., Nikiforova, M. A., Gushchin, V. A., Babichenko, I. I., et al. (2020). Establishment of murine hybridoma cells producing antibodies against spike protein of SARS-CoV-2. Int. J. Mol. Sci. 21, 9167. doi:10.3390/ijms21239167
Arbel, R., Wolff Sagy, Y., Hoshen, M., Battat, E., Lavie, G., Sergienko, R., et al. (2022). Nirmatrelvir use and severe covid-19 outcomes during the omicron surge. N. Engl. J. Med. 387, 790–798. doi:10.1056/nejmoa2204919
Aziz, S., Waqas, M., Mohanta, T. K., Halim, S. A., Iqbal, A., Ali, A., et al. (2023). Identifying non-nucleoside inhibitors of RNA-dependent RNA-polymerase of SARS-CoV-2 through per-residue energy decomposition-based pharmacophore modeling, molecular docking, and molecular dynamics simulation. J. Infect. Public Health 16, 501–519. doi:10.1016/j.jiph.2023.02.009
Badar, M. S., Shamsi, S., Ahmed, J., and Alam, M. A. (2022). Molecular dynamics simulations: concept, methods, and applications. Transdisciplinarity: Springer.
Bairoch, A., and Apweiler, R. (2000). The SWISS-PROT protein sequence database and its supplement TrEMBL in 2000. Nucleic Acids Res. 28, 45–48. doi:10.1093/nar/28.1.45
Beigel, J. H., Tomashek, K. M., Dodd, L. E., Mehta, A. K., Zingman, B. S., Kalil, A. C., et al. (2020). Remdesivir for the treatment of covid-19 - final report. N. Engl. J. Med. 383, 1813–1826. doi:10.1056/nejmoa2007764
Bekker, G. J., Fukuda, I., Higo, J., and Kamiya, N. (2020). Mutual population-shift driven antibody-peptide binding elucidated by molecular dynamics simulations. Sci. Rep. 10, 1406. doi:10.1038/s41598-020-58320-z
Benjin, X., and Ling, L. (2020). Developments, applications, and prospects of cryo-electron microscopy. Protein Sci. 29, 872–882. doi:10.1002/pro.3805
Berendsen, H. J. C., Van Der Spoel, D., and Van Drunen, R. (1995). GROMACS: a message-passing parallel molecular dynamics implementation. Comput. Phys. Commun. 91, 43–56. doi:10.1016/0010-4655(95)00042-e
Bernstein, F. C., Koetzle, T. F., Williams, G. J., Meyer, E. F., Brice, M. D., Rodgers, J. R., et al. (1977). The Protein Data Bank: a computer-based archival file for macromolecular structures. J. Mol. Biol. 112, 535–542. doi:10.1016/s0022-2836(77)80200-3
Beshnova, D., Fang, Y., Du, M., Sun, Y., Du, F., Ye, J., et al. (2022). Computational approach for binding prediction of SARS-CoV-2 with neutralizing antibodies. Comput. Struct. Biotechnol. J. 20, 2212–2222. doi:10.1016/j.csbj.2022.04.038
Boorla, V. S., Chowdhury, R., Ramasubramanian, R., Ameglio, B., Frick, R., Gray, J. J., et al. (2023). De novo design and Rosetta-based assessment of high-affinity antibody variable regions (Fv) against the SARS-CoV-2 spike receptor binding domain (RBD). Proteins 91, 196–208. doi:10.1002/prot.26422
Brito, J. A., and Archer, M. (2020). “Chapter 10 - structural biology techniques: X-ray crystallography, cryo-electron microscopy, and small-angle X-ray scattering,” in Practical approaches to biological inorganic chemistry. Editors R. R. Crichton, and R. O. Louro Second Edition (Elsevier).
Brooks, B. R., Brooks, C. L., Mackerell, A. D., Nilsson, L., Petrella, R. J., Roux, B., et al. (2009). CHARMM: the biomolecular simulation program. J. Comput. Chem. 30, 1545–1614. doi:10.1002/jcc.21287
Chen, R., Li, L., and Weng, Z. (2003). ZDOCK: an initial-stage protein-docking algorithm. Proteins Struct. Funct. Bioinforma. 52, 80–87. doi:10.1002/prot.10389
Chen, Z., Azman, A. S., Chen, X., Zou, J., Tian, Y., Sun, R., et al. (2022). Global landscape of SARS-CoV-2 genomic surveillance and data sharing. Nat. Genet. 54, 499–507. doi:10.1038/s41588-022-01033-y
Cheng, M. H., Porritt, R. A., Rivas, M. N., Krieger, J. M., Ozdemir, A. B., Garcia, G., et al. (2020). A monoclonal antibody against staphylococcal enterotoxin B superantigen inhibits SARS-CoV-2 entry in vitro. bioRxiv, 2020.11.24.395079. doi:10.1101/2020.11.24.395079
Cheung, J., Wazir, S., Bell, D. R., Kochenderfer, J. N., Hendrickson, W. A., and Youkharibache, P. (2023). Crystal structure of a chimeric antigen receptor (CAR) scFv domain rearrangement forming a VL-VL dimer. Crystals 13, 710. doi:10.3390/cryst13040710
Choi, Y., and Deane, C. M. (2010). FREAD revisited: accurate loop structure prediction using a database search algorithm. Proteins 78, 1431–1440. doi:10.1002/prot.22658
Chothia, C., and Lesk, A. M. (1987). Canonical structures for the hypervariable regions of immunoglobulins. J. Mol. Biol. 196, 901–917. doi:10.1016/0022-2836(87)90412-8
Das, N. C., Chakraborty, P., Bayry, J., and Mukherjee, S. (2022). In silico analyses on the comparative potential of therapeutic human monoclonal antibodies against newly emerged SARS-CoV-2 variants bearing mutant spike protein. Front. Immunol. 12, 782506. doi:10.3389/fimmu.2021.782506
Das, N. C., Chakraborty, P., Bayry, J., and Mukherjee, S. (2023). Comparative binding ability of human monoclonal antibodies against Omicron variants of SARS-CoV-2: an in silico investigation. Antibodies 12, 17. doi:10.3390/antib12010017
Davies, D. R., and Chacko, S. (1993). Antibody structure. Accounts Chem. Res. 26, 421–427. doi:10.1021/ar00032a005
Dănăilă, V.-R., and Buiu, C. (2022). Prediction of HIV sensitivity to monoclonal antibodies using aminoacid sequences and deep learning. Bioinformatics 38, 4278–4285.
Desautels, T. A., Arrildt, K. T., Zemla, A. T., Lau, E. Y., Zhu, F., Ricci, D., et al. (2023). Computationally restoring the potency of a clinical antibody against SARS-CoV-2 Omicron subvariants. bioRxiv, 513237. doi:10.1101/2022.10.21.513237
DeLano, W. L. (2002). Pymol: An open-source molecular graphics tool. CCP4 Newsl. Prote. Crysta. 40, 82–92.
Dominguez, C., Boelens, R., and Bonvin, A. M. (2003). HADDOCK: a protein-protein docking approach based on biochemical or biophysical information. J. Am. Chem. Soc. 125, 1731–1737. doi:10.1021/ja026939x
Du, K., and Huang, H. (2023). Development of anti-PD-L1 antibody based on structure prediction of AlphaFold2. Front. Immunol. 14, 1275999. doi:10.3389/fimmu.2023.1275999
Dunbar, J., and Deane, C. M. (2015). ANARCI: antigen receptor numbering and receptor classification. Bioinformatics 32, 298–300. doi:10.1093/bioinformatics/btv552
Dunbar, J., Fuchs, A., Shi, J., and Deane, C. M. (2013). ABangle: characterising the VH-VL orientation in antibodies. Protein Eng. Des. Sel. 26, 611–620. doi:10.1093/protein/gzt020
Dzimianski, J. V., Han, J., Sautto, G. A., O’rourke, S. M., Cruz, J. M., Pierce, S. R., et al. (2023). Structural insights into the broad protection against H1 influenza viruses by a computationally optimized hemagglutinin vaccine. Communi. Bio. 6, 454.
Fernández-Quintero, M. L., Loeffler, J. R., Bacher, L. M., Waibl, F., Seidler, C. A., and Liedl, K. R. (2020). Local and global rigidification upon antibody affinity maturation. Front. Mol. Biosci. 7, 182. doi:10.3389/fmolb.2020.00182
Ford, C. T., Jacob Machado, D., and Janies, D. A. (2022). Predictions of the SARS-CoV-2 omicron variant (B. 1.1. 529) spike protein receptor-binding domain structure and neutralizing antibody interactions. Front. Virology 2, 830202. doi:10.3389/fviro.2022.830202
Ford, C. T., Yasa, S., Jacob Machado, D., White Iii, R. A., and Janies, D. A. (2023). Predicting changes in neutralizing antibody activity for SARS-CoV-2 XBB. 1.5 using in silico protein modeling. Front. Virology 3, 1172027. doi:10.3389/fviro.2023.1172027
Fung, K. M., Lai, S. J., Lin, T. L., and Tseng, T. S. (2022). Antigen-antibody complex-guided exploration of the hotspots conferring the immune-escaping ability of the SARS-CoV-2 RBD. Front. Mol. Biosci. 9, 797132. doi:10.3389/fmolb.2022.797132
García-Vega, M., Melgoza-González, E. A., Hernández-Valenzuela, S., Hinojosa-Trujillo, D., Reséndiz-Sandoval, M., Llamas-Covarrubias, M. A., et al. (2023). 19n01, a broadly neutralizing antibody against omicron BA.1, BA.2, BA.4/5, and other SARS-CoV-2 variants of concern. iScience 26, 106562. doi:10.1016/j.isci.2023.106562
Gaudreault, F., Corbeil, C. R., and Sulea, T. (2023). Enhanced antibody-antigen structure prediction from molecular docking using AlphaFold2. Sci. Rep. 13, 15107. doi:10.1038/s41598-023-42090-5
Giron, C. C., Laaksonen, A., and Da Silva, F. L. B. (2020). On the interactions of the receptor-binding domain of SARS-CoV-1 and SARS-CoV-2 spike proteins with monoclonal antibodies and the receptor ACE2. Virus Res. 285, 198021. doi:10.1016/j.virusres.2020.198021
Gordon, C. J., Tchesnokov, E. P., Schinazi, R. F., and Götte, M. (2021). Molnupiravir promotes SARS-CoV-2 mutagenesis via the RNA template. J. Biol. Chem. 297, 100770. doi:10.1016/j.jbc.2021.100770
Gu, C., Cao, X., Wang, Z., Hu, X., Yao, Y., Zhou, Y., et al. (2021). A human antibody of potent efficacy against SARS-CoV-2 in rhesus macaques showed strong blocking activity to B.1.351. mAbs 13, 1930636. doi:10.1080/19420862.2021.1930636
Harris, C. T., and Cohen, S. (2024). Reducing immunogenicity by design: approaches to minimize immunogenicity of monoclonal antibodies. BioDrugs 38, 205–226. doi:10.1007/s40259-023-00641-2
Hernandez, N. E., Jankowski, W., Frick, R., Kelow, S. P., Lubin, J. H., Simhadri, V., et al. (2023). Computational design of nanomolar-binding antibodies specific to multiple SARS-CoV-2 variants by engineering a specificity switch of antibody 80R using RosettaAntibodyDesign (RAbD) results in potential generalizable therapeutic antibodies for novel SARS-CoV-2 virus. Heliyon 9, e15032. doi:10.1016/j.heliyon.2023.e15032
Humphrey, W., Dalke, A., and Schulten, K. (1996). VMD: visual molecular dynamics. J. Molecu. Graph. 14, 33–38.
Hulo, N., Bairoch, A., Bulliard, V., Cerutti, L., De Castro, E., Langendijk-Genevaux, P. S., et al. (2006). The PROSITE database. Nucleic Acids Res. 34, D227–D230. doi:10.1093/nar/gkj063
Infectious Diseases Society of America (2024). COVID-19 Variant Update. Available at:https://www.idsociety.org/covid-19-real-time-learning-network/diagnostics/covid-19-variant-update/#/+/0/publishedDate_na_dt/desc/.
Ivanov, V., Lohachova, K., Kolesnik, Y., Zakharov, A., Yevsieieva, L., Kyrychenko, A., et al. (2023). Recent advances in computational drug discovery for therapy against coronavirus SARS-CoV-2. Sci. Pharm. Sci. doi:10.15587/2519-4852.2023.290318
Jabalia, N., Kumar, A., Kumar, V., and Rani, R. (2021). “In silico approach in drug design and drug discovery: an update,” in Innovations and implementations of computer aided drug discovery strategies in rational drug design. Editor S. K. SINGH (Singapore: Springer Singapore).
Jairajpuri, D. S., Hussain, A., Nasreen, K., Mohammad, T., Anjum, F., Rehman, M. T., et al. (2021). Identification of natural compounds as potent inhibitors of SARS-CoV-2 main protease using combined docking and molecular dynamics simulations. Saudi J. Biol. Sci. 28, 2423–2431. doi:10.1016/j.sjbs.2021.01.040
Jandova, Z., Vargiu, A. V., and Bonvin, A. M. J. J. (2021). Native or non-native protein–protein docking models? Molecular dynamics to the rescue. J. Chem. Theory Comput. 17, 5944–5954. doi:10.1021/acs.jctc.1c00336
Jarad, A. J., Dahi, M. A., Al-Noor, T. H., El-Ajaily, M. M., Al-Ayash, S. R., and Abdou, A. (2023). Synthesis, spectral studies, DFT, biological evaluation, molecular docking and dyeing performance of 1-(4-((2-amino-5-methoxy)diazenyl)phenyl) ethanone complexes with some metallic ions. J. Mol. Struct. 1287, 135703. doi:10.1016/j.molstruc.2023.135703
Jiao, Y., Xing, Y., and Sun, Y. (2023). Impact of E484Q and L452R mutations on structure and binding behavior of SARS-CoV-2 B. 1.617. 1 using deep learning AlphaFold2, molecular docking and dynamics simulation. Int. J. Mol. Sci. 24, 11564. doi:10.3390/ijms241411564
Jing, H., Gao, Z., Xu, S., Shen, T., Peng, Z., He, S., et al. (2024). Accurate prediction of antibody function and structure using bio-inspired antibody language model. Briefings Bioinforma. 25, bbae245. doi:10.1093/bib/bbae245
Kashkooli, F. M., Soltani, M., Souri, M., Meaney, C., and Kohandel, M. (2021). Nexus between in silico and in vivo models to enhance clinical translation of nanomedicine. Nano Today 36, 101057. doi:10.1016/j.nantod.2020.101057
Kaufer, A. M., Theis, T., Lau, K. A., Gray, J. L., and Rawlinson, W. D. (2020). Laboratory biosafety measures involving SARS-CoV-2 and the classification as a Risk Group 3 biological agent. Pathology 52, 790–795. doi:10.1016/j.pathol.2020.09.006
Keam, S. J. (2022). Tixagevimab + cilgavimab: first approval. Drugs 82, 1001–1010. doi:10.1007/s40265-022-01731-1
Kelley, B. (2020). Developing therapeutic monoclonal antibodies at pandemic pace. Nat. Biotechnol. 38, 540–545. doi:10.1038/s41587-020-0512-5
Khan, M. K. A., Pokharkar, N. B., Al-Khodairy, F. M., Al-Marshad, F. M., and Arif, J. M. (2020). Structural perspective on molecular interaction of IgG and IgA with spike and envelope proteins of SARS-CoV-2 and its implications to non-specific immunity. Biointerface Res. Appl. Chem. 11, 10923–10939. doi:10.33263/briac113.1092310939
Khuat, T. T., Bassett, R., Otte, E., Grevis-James, A., and Gabrys, B. (2024). Applications of machine learning in antibody discovery, process development, manufacturing and formulation: current trends, challenges, and opportunities. Comput. and Chem. Eng. 182, 108585. doi:10.1016/j.compchemeng.2024.108585
Kilambi, K. P., and Gray, J. J. (2017). Structure-based cross-docking analysis of antibody-antigen interactions. Sci. Rep. 7, 8145. doi:10.1038/s41598-017-08414-y
Kim, J. W., Min, S. W., Lee, J., Shin, H. G., Choi, H. L., Yang, H. R., et al. (2022). Development and characterization of phage-display-derived novel human monoclonal antibodies against the receptor binding domain of SARS-CoV-2. Biomedicines 10, 3274. doi:10.3390/biomedicines10123274
Koçer, İ., and Çelik, E. (2024). In silico analysis of the different variable domain oriented single-chain variable fragment antibody-antigen complexes. J. Biomol. Struct. Dyn. 42, 4699–4709. doi:10.1080/07391102.2023.2222191
Köhler, G., and Milstein, C. (1975). Continuous cultures of fused cells secreting antibody of predefined specificity. Nature 256, 495–497. doi:10.1038/256495a0
Kozakov, D., Hall, D. R., Xia, B., Porter, K. A., Padhorny, D., Yueh, C., et al. (2017). The ClusPro web server for protein–protein docking. Nat. Protoc. 12, 255–278. doi:10.1038/nprot.2016.169
Leem, J., Dunbar, J., Georges, G., Shi, J., and Deane, C. M. (2016). ABodyBuilder: automated antibody structure prediction with data-driven accuracy estimation. MAbs 8, 1259–1268. doi:10.1080/19420862.2016.1205773
Lefranc, M. P., Giudicelli, V., Duroux, P., Jabado-Michaloud, J., Folch, G., Aouinti, S., et al. (2015). IMGT®, the international ImMunoGeneTics information system® 25 years on. Nucleic Acids Res. 43, D413–D422. doi:10.1093/nar/gku1056
Lemm, D., Von Rudorff, G. F., and Von Lilienfeld, O. A. (2021). Machine learning based energy-free structure predictions of molecules, transition states, and solids. Nat. Commun. 12, 4468. doi:10.1038/s41467-021-24525-7
Li, L., Chen, S., Miao, Z., Liu, Y., Liu, X., Xiao, Z. X., et al. (2019). AbRSA: a robust tool for antibody numbering. Protein Sci. 28, 1524–1531. doi:10.1002/pro.3633
Liang, T., Jiang, C., Yuan, J., Othman, Y., Xie, X.-Q., and Feng, Z. (2022). Differential performance of RoseTTAFold in antibody modeling. Briefings Bioinforma. 23, bbac152. doi:10.1093/bib/bbac152
Lo Conte, L., Ailey, B., Hubbard, T. J., Brenner, S. E., Murzin, A. G., and Chothia, C. (2000). SCOP: a structural classification of proteins database. Nucleic Acids Res. 28, 257–259. doi:10.1093/nar/28.1.257
Loibner, M., Langner, C., Regitnig, P., Gorkiewicz, G., and Zatloukal, K. (2021). Biosafety requirements for autopsies of patients with COVID-19: example of a BSL-3 autopsy facility designed for highly pathogenic agents. Pathobiology 88, 37–45. doi:10.1159/000513438
Lubin, J. H., Markosian, C., Balamurugan, D., Pasqualini, R., Arap, W., Burley, S. K., et al. (2021). Structural models of SARS-CoV-2 Omicron variant in complex with ACE2 receptor or antibodies suggest altered binding interfaces. New York, USA: Cold Spring Harbor Laboratory.
Lyskov, S., and Gray, J. J. (2008). The RosettaDock server for local protein–protein docking. Nucleic acids Res. 36, W233–W238. doi:10.1093/nar/gkn216
Madku, S. R., Sahoo, B. K., Lavanya, K., Reddy, R. S., and Bodapati, A. T. S. (2023). DNA binding studies of antifungal drug posaconazole using spectroscopic and molecular docking methods. Int. J. Biol. Macromol. 225, 745–756. doi:10.1016/j.ijbiomac.2022.11.137
Martí, D., Alsina, M., Alemán, C., Bertran, O., Turon, P., and Torras, J. (2022). Unravelling the molecular interactions between the SARS-CoV-2 RBD spike protein and various specific monoclonal antibodies. Biochimie 193, 90–102. doi:10.1016/j.biochi.2021.10.013
Martin, A. C., and Thornton, J. M. (1996). Structural families in loops of homologous proteins: automatic classification, modelling and application to antibodies. J. Mol. Biol. 263, 800–815. doi:10.1006/jmbi.1996.0617
Mercurio, I., Tragni, V., Busto, F., De Grassi, A., and Pierri, C. L. (2021). Protein structure analysis of the interactions between SARS-CoV-2 spike protein and the human ACE2 receptor: from conformational changes to novel neutralizing antibodies. Cell. Mol. Life Sci. 78, 1501–1522. doi:10.1007/s00018-020-03580-1
Moore, S. (2021). What is in silico? Available at: https://www.news-medical.net/life-sciences/What-is-in-Silico.aspx.
Moraes, J. Z., Hamaguchi, B., Braggion, C., Speciale, E. R., Cesar, F. B. V., Soares, G., et al. (2021). Hybridoma technology: is it still useful? Curr. Res. Immunol. 2, 32–40. doi:10.1016/j.crimmu.2021.03.002
Nath, H., Mallick, A., Roy, S., Sukla, S., and Biswas, S. (2021). Computational modelling supports that dengue virus envelope antibodies can bind to SARS-CoV-2 receptor binding sites: is pre-exposure to dengue virus protective against COVID-19 severity? Comput. Struct. Biotechnol. J. 19, 459–466. doi:10.1016/j.csbj.2020.12.037
Neamtu, A., Mocci, F., Laaksonen, A., and Da Silva, F. L. B. (2023). Towards an optimal monoclonal antibody with higher binding affinity to the receptor-binding domain of SARS-CoV-2 spike proteins from different variants. Colloids Surfaces B Biointerfaces 221, 112986. doi:10.1016/j.colsurfb.2022.112986
Noreen, S., Sumrra, S. H., Chohan, Z. H., Mustafa, G., and Imran, M. (2023). Synthesis, characterization, molecular docking and network pharmacology of bioactive metallic sulfonamide-isatin ligands against promising drug targets. J. Mol. Struct. 1277, 134780. doi:10.1016/j.molstruc.2022.134780
Norman, R. A., Ambrosetti, F., Bonvin, A. M. J. J., Colwell, L. J., Kelm, S., Kumar, S., et al. (2019). Computational approaches to therapeutic antibody design: established methods and emerging trends. Briefings Bioinforma. 21, 1549–1567. doi:10.1093/bib/bbz095
Ojha, R., Gurjar, K., Ratnakar, T. S., Mishra, A., and Prajapati, V. K. (2022). Designing of a bispecific antibody against SARS-CoV-2 spike glycoprotein targeting human entry receptors DPP4 and ACE2. Hum. Immunol. 83, 346–355. doi:10.1016/j.humimm.2022.01.004
Orders, M. (2022). An EUA for bebtelovimab for treatment of COVID-19. Med. Lett. Drugs Ther. 64, 41–42.
Patel, R., Verma, P., Nagraj, A. K., Gavade, A., Sharma, O. P., and Patil, J. (2023). Significance of antibody numbering systems in the development of antibody engineering. Hum. Antibodies 31, 71–80. doi:10.3233/hab-230014
Pizzato, M., Baraldi, C., Boscato Sopetto, G., Finozzi, D., Gentile, C., Gentile, M. D., et al. (2022). SARS-CoV-2 and the host cell: a tale of interactions. Front. Virology 1. doi:10.3389/fviro.2021.815388
Poluri, K. M., Gulati, K., and Sarkar, S. (2021). “Structural and functional properties of proteins,” in Protein-protein interactions: principles and techniques, Vol. I. Singapore: Springer Singapore, 1–60. doi:10.1007/978-981-16-1594-8_1
Raisinghani, N., Alshahrani, M., Gupta, G., Xiao, S., Tao, P., and Verkhivker, G. (2024). Exploring conformational landscapes and binding mechanisms of convergent evolution for the SARS-CoV-2 spike Omicron variant complexes with the ACE2 receptor using AlphaFold2-based structural ensembles and molecular dynamics simulations. Phys. Chem. Chem. Phys. 26, 17720–17744. doi:10.1039/d4cp01372g
Raybould, M. I. J., Marks, C., Lewis, A. P., Shi, J., Bujotzek, A., Taddese, B., et al. (2020). Thera-SAbDab: the therapeutic structural antibody database. Nucleic Acids Res. 48, D383–d388. doi:10.1093/nar/gkz827
Rehman, I., Kerndt, C. C., and Botelho, S. (2022). Biochemistry, tertiary protein structure. Treasure Island, Florida, USA: StatPearls Publishing. StatPearls.
Roodink, I., Van Erp, M., Li, A., Potter, S., Van Duijnhoven, S. M. J., Smits, M., et al. (2024). Broad epitope coverage of therapeutic multi-antibody combinations targeting SARS-CoV-2 boosts in vivo protection and neutralization potency to corner an immune-evading virus. Biomedicines 12, 642. doi:10.3390/biomedicines12030642
Rouet, R., Henry, J. Y., Johansen, M. D., Sobti, M., Balachandran, H., Langley, D. B., et al. (2023). Broadly neutralizing SARS-CoV-2 antibodies through epitope-based selection from convalescent patients. Nat. Commun. 14, 687. doi:10.1038/s41467-023-36295-5
Ruff, K. M., and Pappu, R. V. (2021). AlphaFold and implications for intrinsically disordered proteins. J. Mol. Biol. 433, 167208. doi:10.1016/j.jmb.2021.167208
Safarzadeh Kozani, P., Sheikhi, M., Baharifar, N., Dashti Shokoohi, S., Sheikhi, S., Mirarefin, S. M. J., et al. (2022). Bebtelovimab: the FDA-approved monoclonal antibody for treating patients with mild-to-moderate COVID-19. J. Adv. Immunopharmacol. 2, e130706. doi:10.5812/tms-130706
Šali, A., and Blundell, T. L. (1993). Comparative protein modelling by satisfaction of spatial restraints. J. Mol. Biol. 234, 779–815. doi:10.1006/jmbi.1993.1626
Schepens, B., Van Schie, L., Nerinckx, W., Roose, K., Van Breedam, W., Fijalkowska, D., et al. (2021). An affinity-enhanced, broadly neutralizing heavy chain–only antibody protects against SARS-CoV-2 infection in animal models. Sci. Transl. Med. 13, eabi7826. doi:10.1126/scitranslmed.abi7826
Schwede, T., Kopp, J., Guex, N., and Peitsch, M. C. (2003). SWISS-MODEL: an automated protein homology-modeling server. Nucleic Acids Res. 31, 3381–3385. doi:10.1093/nar/gkg520
Shah, M., and Woo, H. G. (2022). Omicron: a heavily mutated SARS-CoV-2 variant exhibits stronger binding to ACE2 and potently escapes approved COVID-19 therapeutic antibodies. Front. Immunol. 12, 830527. doi:10.3389/fimmu.2021.830527
Shahmirzaie, M., Safarnejad, M. R., Rakhshandehroo, F., Safarpour, H., Shirazi, F. H., Zamanizadeh, H. R., et al. (2020). Generation and molecular docking analysis of specific single-chain variable fragments selected by phage display against the recombinant nucleocapsid protein of fig mosaic virus. J. Virol. Methods 276, 113796. doi:10.1016/j.jviromet.2019.113796
Shaker, B., Ahmad, S., Lee, J., Jung, C., and Na, D. (2021). In silico methods and tools for drug discovery. Comput. Biol. Med. 137, 104851. doi:10.1016/j.compbiomed.2021.104851
Shang, W., Hu, X., Lin, X., Li, S., Xiong, S., Huang, B., et al. (2024). Iterative in silico screening for optimizing stable conformation of anti-SARS-CoV-2 nanobodies. Pharmaceuticals 17, 424. doi:10.3390/ph17040424
Shaw, D. E., Maragakis, P., Lindorff-Larsen, K., Piana, S., Dror, R. O., Eastwood, M. P., et al. (2010). Atomic-level characterization of the structural dynamics of proteins. Science 330, 341–346. doi:10.1126/science.1187409
Shukla, R., and Tripathi, T. (2020). “Molecular dynamics simulation of protein and protein–ligand complexes,” in Computer-aided drug design. Editor D. B. SINGH (Singapore: Springer Singapore).
Skolnick, J., Gao, M., Zhou, H., and Singh, S. (2021). AlphaFold 2: why it works and its implications for understanding the relationships of protein sequence, structure, and function. J. Chem. Inf. Model 61, 4827–4831. doi:10.1021/acs.jcim.1c01114
Singh, S., Rao, A., Mishra, A., Mishra, A., and Prajapati, V. K. (2023). Multifaceted mutational immunotherapeutic approach to design therapeutic mAbs to combat monkeypox disease via integrated screening algorithms and antibody engineering. Molecu. System. Design. Enginee. 8, 1301–1318.
Smith, G. P., and Petrenko, V. A. (1997). Phage display. Chem. Rev. 97, 391–410. doi:10.1021/cr960065d
Somasundaram, R., Choraria, A., and Antonysamy, M. (2020). An approach towards development of monoclonal IgY antibodies against SARS CoV-2 spike protein (S) using phage display method: a review. Int. Immunopharmacol. 85, 106654. doi:10.1016/j.intimp.2020.106654
Srivastava, A., Nagai, T., Srivastava, A., Miyashita, O., and Tama, F. (2018). Role of computational methods in going beyond X-ray crystallography to explore protein structure and dynamics. Int. J. Mol. Sci. 19, 3401. doi:10.3390/ijms19113401
Su, H., Zhang, J., Yi, Z., Khan, S., Peng, M., Ye, L., et al. (2024). A human monoclonal antibody neutralizes SARS-CoV-2 Omicron variants by targeting the upstream region of spike protein HR2 motif. hLife 2, 126–140. doi:10.1016/j.hlife.2024.02.001
Suryadevara, N., Kose, N., Bangaru, S., Binshtein, E., Munt, J., Martinez, D. R., et al. (2024). Structural characterization of human monoclonal antibodies targeting uncommon antigenic sites on spike glycoprotein of SARS-CoV. J. Clin. Investigation, e178880. doi:10.1172/JCI178880
UniProt Consortium (2015). UniProt: a hub for protein information. Nucleic Acids Res. 43, D204–D212. doi:10.1093/nar/gku989
Van Der Spoel, D., Lindahl, E., Hess, B., Groenhof, G., Mark, A. E., and Berendsen, H. J. (2005). GROMACS: fast, flexible, and free. J. Comput. Chem. 26, 1701–1718. doi:10.1002/jcc.20291
Van Regenmortel, M. H. (2014). Specificity, polyspecificity, and heterospecificity of antibody-antigen recognition. J. Mol. Recognit. 27, 627–639. doi:10.1002/jmr.2394
Wang, J., Wolf, R. M., Caldwell, J. W., Kollman, P. A., and Case, D. A. (2004). Development and testing of a general amber force field. J. Comput. Chem. 25, 1157–1174. doi:10.1002/jcc.20035
Wang, Q., Peng, L., Nie, Y., Shu, Y., Zhang, H., Song, Z., et al. (2023). Hybridoma-derived neutralizing monoclonal antibodies against Beta and Delta variants of SARS-CoV-2 in vivo. Virol. Sin. 38, 257–267. doi:10.1016/j.virs.2022.12.007
Wang, Y., Yuan, M., Lv, H., Peng, J., Wilson, I. A., and Wu, N. C. (2022). A large-scale systematic survey reveals recurring molecular features of public antibody responses to SARS-CoV-2. Immunity 55, 1105–1117. e4. doi:10.1016/j.immuni.2022.03.019
Weng, G., Gao, J., Wang, Z., Wang, E., Hu, X., Yao, X., et al. (2020). Comprehensive evaluation of fourteen docking programs on protein-peptide complexes. J. Chem. Theory Comput. 16, 3959–3969. doi:10.1021/acs.jctc.9b01208
Weng, G., Wang, E., Wang, Z., Liu, H., Zhu, F., Li, D., et al. (2019). HawkDock: a web server to predict and analyze the protein–protein complex based on computational docking and MM/GBSA. Nucleic acids Res. 47, W322–W330. doi:10.1093/nar/gkz397
Wolf Pérez, A. M., Lorenzen, N., Vendruscolo, M., and Sormanni, P. (2022). Assessment of therapeutic antibody developability by combinations of in vitro and in silico methods. Methods Mol. Biol. 2313, 57–113. doi:10.1007/978-1-0716-1450-1_4
Wong, W. K., Leem, J., and Deane, C. M. (2019). Comparative analysis of the CDR loops of antigen receptors. Front. Immunol. 10, 2454. doi:10.3389/fimmu.2019.02454
Xu, J., Xu, K., Jung, S., Conte, A., Lieberman, J., Muecksch, F., et al. (2021). Nanobodies from camelid mice and llamas neutralize SARS-CoV-2 variants. Nature 595, 278–282. doi:10.1038/s41586-021-03676-z
Yang, J., Zhang, Z., Yang, F., Zhang, H., Wu, H., Zhu, F., et al. (2021). Computational design and modeling of nanobodies toward SARS-CoV-2 receptor binding domain, 98. Hoboken, New Jersey, USA: Chemical Biology and Drug Design, 1–18.
Yang, Q., Yan, M., Lin, J., Lu, Y., Lin, S., Li, Z., et al. (2024). Screening and affinity optimization of single domain antibody targeting the SARS-CoV-2 nucleocapsid protein. PeerJ 12, e17846. doi:10.7717/peerj.17846
Yin, R., Feng, B. Y., Varshney, A., and Pierce, B. G. (2022). Benchmarking AlphaFold for protein complex modeling reveals accuracy determinants. Protein Sci. 31, e4379. doi:10.1002/pro.4379
Yu, W., Zhong, N., Li, X., Ren, J., Wang, Y., Li, C., et al. (2022). Structure based affinity maturation and characterizing of SARS-CoV antibody CR3022 against SARS-CoV-2 by computational and experimental approaches. Viruses 14, 186. doi:10.3390/v14020186
Zeng, X., Bai, G., Sun, C., and Ma, B. (2023). Recent progress in antibody epitope prediction. Antibodies (Basel) 12, 52. doi:10.3390/antib12030052
Keywords: in silico, antibody, SARS-CoV-2, computational approach, bioinformatics, molecular dynamic simulation
Citation: Subramaniam T, Mualif SA, Chan WH and Abd Halim KB (2025) Unlocking the potential of in silico approach in designing antibodies against SARS-CoV-2. Front. Bioinform. 5:1533983. doi: 10.3389/fbinf.2025.1533983
Received: 25 November 2024; Accepted: 17 January 2025;
Published: 13 February 2025.
Edited by:
Vikram Dalal, Washington University in St. Louis, United StatesReviewed by:
Gunjan Saini, Purdue University, United StatesMeenakshi Tanwar, University of Maryland, United States
Copyright © 2025 Subramaniam, Mualif, Chan and Abd Halim. This is an open-access article distributed under the terms of the Creative Commons Attribution License (CC BY). The use, distribution or reproduction in other forums is permitted, provided the original author(s) and the copyright owner(s) are credited and that the original publication in this journal is cited, in accordance with accepted academic practice. No use, distribution or reproduction is permitted which does not comply with these terms.
*Correspondence: Siti Aisyah Mualif, YWlzeWFobXVhbGlmQHV0bS5teQ==