- 1Department of Translational Research and New Technologies in Medicine and Surgery, University of Pisa, Pisa, Italy
- 2Clinical Physiology Institute, The Italian National Research Council (CNR), Massa, Italy
- 3Department of Biomedical Sciences, University of Cagliari, Cagliari, Italy
Alzheimer’s disease (AD) is a complex neurodegenerative condition characterized by a multifaceted interplay of genetic, environmental, and pathological factors. Traditional diagnostic and research methods, including neuropsychological assessments, imaging, and cerebrospinal fluid (CSF) biomarkers, have advanced our understanding but remain limited by late-stage detection and challenges in modeling disease progression. The emergence of three-dimensional (3D) brain organoids (BOs) offers a transformative platform for bridging these gaps. BOs derived from patient-specific induced pluripotent stem cells (iPSCs) mimic the structural and functional complexities of the human brain. This advancement offers an alternative or complementary approach for studying AD pathology, including β-amyloid and tau protein aggregation, neuroinflammation, and aging processes. By integrating biological complexity with cutting-edge technological tools such as organ-on-a-chip systems, microelectrode arrays, and artificial intelligence-driven digital twins (DTs), it is hoped that BOs will facilitate real-time modeling of AD progression and response to interventions. These models capture central nervous system biomarkers and establish correlations with peripheral markers, fostering a holistic understanding of disease mechanisms. Furthermore, BOs provide a scalable and ethically sound alternative to animal models, advancing drug discovery and personalized therapeutic strategies. The convergence of BOs and DTs potentially represents a significant shift in AD research, enhancing predictive and preventive capacities through precise in vitro simulations of individual disease trajectories. This approach underscores the potential for personalized medicine, reducing the reliance on invasive diagnostics while promoting early intervention. As research progresses, integrating sporadic and familial AD models within this framework promises to refine our understanding of disease heterogeneity and drive innovations in treatment and care.
1 Introduction
The diagnosis of Alzheimer’s disease (AD) integrates medical history, neuropsychological assessments, imaging, and cerebrospinal fluid (CSF) analysis. A detailed clinical history examines cognitive and behavioral symptoms, progression, and risk factors (age, family history, and any comorbidities). Differential diagnoses help out in predicting pseudodementia, frontotemporal dementia, Lewy body dementia, and vascular dementia (Thangwaritorn et al., 2024). Neuropsychological assessments, such as the Mini-Mental State Examination (MMSE) (Arevalo-Rodriguez et al., 2021) and Montreal Cognitive Assessment (MoCA) (Ciesielska et al., 2016), provide a global cognitive screening, whereas specific tests assess memory, executive functions, and language. Clinical scales including Activities of Daily Living (ADL), Instrumental Activity of Daily Living (IADL) (Teng et al., 2023), and the Clinical Dementia Rating (CDR) (Cummings et al., 2023), evaluate functional autonomy and dementia severity. The CDR stages range from normal aging (CDR 0) to severe dementia (CDR 3), aiding in distinguishing Mild Cognitive Impairment (MCI) (CDR 0.5) from AD (Gkenios et al., 2022).
Imaging plays a pivotal role in diagnosis. Magnetic Resonance Imaging (MRI) allows to detect AD-related hippocampal and cortical atrophy, particularly in the temporo-parietal regions (Chandra et al., 2019). Positron emission tomography (PET) with fluorodeoxyglucose (FDG) reveals hypometabolism in medial temporal and parietal regions, while amyloid PET highlights β-amyloid deposition, a well-known hallmark of AD (Chételat et al., 2020). All of them are extremely informative in early or atypical disease presentations.
CSF biomarkers such as reduced β-amyloid 42 (Aβ42), increased total tau (T-Tau), and phosphorylated tau (P-Tau), are crucial for biological AD confirmation. The Aβ42/Aβ40 ratio enhances diagnostic precision, while additional biomarkers address neuroinflammation and oxidative stress (Olsson et al., 2016; Ossenkoppele et al., 2022; Dubois et al., 2023: Pascoal et al., 2024).
A potential AD diagnosis requires clinical symptoms and at least one positive biomarker, while a possible diagnosis applies to typical symptoms and inconclusive biomarkers. Definitive diagnosis is achieved post-mortem via neuropathological analysis. AD diagnosis remains challenging due to its overlap with mixed or vascular dementias, which necessitates a nuanced diagnostic approach (Zekry et al., 2002).
AD also embraces a spectrum of preclinical stages. Subjective Cognitive Impairment (SCI) and Mild Cognitive Impairment MCI represent prodromal conditions. SCI involves perceived cognitive deficits with neuroimaging evidence (Stewart, 2012; Wang et al., 2020), in contrast to MCI that denotes measurable cognitive decline with preserved function. MCI is a significant risk factor for AD, though not all cases progress to dementia (Jongsiriyanyong and Limpawattana, 2018).
Integrating clinical, neuropsychological, imaging, and biochemical biomarkers enhances diagnostic accuracy and informs targeted therapies. However, AD diagnosis often occurs late relative to underlying neurobiological changes, emphasizing the need for early identification and intervention.
Finally, we are now entering a pivotal era in which the evaluation of biomarkers in presymptomatic individuals will offer the biological foundation necessary to transition conventional plasma biomarkers into digital biomarkers (Cash et al., 2025; Aghdam et al., 2025; Jiao et al., 2025).
2 Exploring new frontiers in Alzheimer’s disease diagnosis: beyond the brain
Recommendation frameworks for diagnosing AD have been recently updated by the National Institute of Aging and the Alzheimer’s Association (NIA-AA) (Jack et al., 2024), based on the latest advancements that refine the previous document (Jack et al., 2018). On this matter, AD definition can be declined as a biological process that begins with the emergence of Alzheimer’s disease neuropathologic change (ADNPC) while individuals are still asymptomatic. As the neuropathologic burden progresses, clinical symptoms eventually appear and worsen. Early-changing biomarkers, such as amyloid PET-highlighted changes, CSF biomarkers, and p-Tau 217 (core 1 biomarker), indicate the presence of ADNPC. An abnormal result from a core 1 biomarker is sufficient for diagnosing AD and guiding clinical decisions. Later-changing core 2 biomarkers, including biofluids and tau PET, provide prognostic data that assist with confirming AD’s role in symptoms. The main goal is to establish objective criteria for diagnosing and staging AD thanks to the new advancements in biomarkers, to bridge the gap between research and clinical care settings. Currently, while the use of biomarkers enhances the in vivo diagnosis of AD, the approach has shifted from a syndromic model to a biological one, based on the [AT (N)] classification developed by the NIA-AA, that categorizes patients based on the presence of amyloid (A), tau protein (T), and neurodegeneration (N) (Jack et al., 2018). The ATN system is designed to be flexible, allowing for the addition of central and peripheral biomarkers as they become available. This is why some researchers advocate for including further molecular biomarkers, particularly those related to inflammation, in both the central nervous system (Xc) and the periphery (Xp) (Huang et al., 2022). This shifting paradigm aims to identify novel or unconventional diagnostic and prognostic biomarkers (Klyucherev et al., 2022) beyond the brain, especially in blood (Hampel et al., 2023) and CSF, or other biological fluids (Carmona-Iragui et al., 2024) to strengthen predictive and preventive strategies for AD (Khoury and Ghossoub, 2019; Baldini et al., 2022; Teunissen et al., 2022). For instance, cholesterol and its derivatives have emerged as significant biomarkers, linked to an increased risk of dementia in initially healthy older adults (Hussain et al., 2024). Moreover, microRNAs (miRNAs), crucial regulators of gene expression, have garnered attention for their potential clinical significance (Wei et al., 2020; Luo et al., 2022). Distinct miRNA profiles in the blood are valuable candidates for drawing correlations with various stages of AD, positioning them as useful targets for future interventions (Zhou et al., 2020; Bhatnagar et al., 2023). In the context of circulating and measurable chemical signals, recruiting exosomes and extracellular vesicles (EVs) containing miRNAs in the bloodstream has reinforced their potential as biomarkers for AD (Soares Martins et al., 2022; Abidin et al., 2023). By exploring biomarkers outside the traditional realms, researchers are paving the way for more comprehensive and early detection methods for AD, its clear-cut staging, and its discrimination against non-AD type dementias, offering hope for more effective treatment and prevention tools. Within this context, it is widely acknowledged that neuroinflammation plays a pivotal role in AD pathology. This pathway involves astrocytic and microglial activation, cytokine release, and alterations in the clearance of misfolded protein aggregates, all of which influence the onset and progression of AD. For instance, reactive astrogliosis (RA) exhibits a dual impact. While contributing to neurotoxicity and inflammation, it protects against neurotoxic agents and supports blood–brain barrier (BBB) repair (Singh, 2022). Moreover, systemic inflammasome activation (Rui et al., 2021) triggers a self-sustaining loop that leads the healthy brain to the Alzheimer’s manifestation through a cognitive impairment and finally to the Alzheimer’s manifestation, as recently confirmed by the role of the aberrant expression of Nucleotide-binding oligomerization domain (NOD), Leucine rich Repeat and Pyrin domain containing (NLRP) inflammasome activated caspase-1 (Beder et al., 2024). This statement intensifies the urgency of preclinical models, filling the gap between inconsistent preclinical AD animal models and the clinical appearance of the disease in humans.
3 The need for innovative experimental models: toward the modern age of 3D brain organoids
Despite their contributions to understanding AD mechanisms, both traditional animal models and transgenic ones fail to replicate the human brain’s intricate tissue structure, function, cellular diversity and pathology hallmarks as well as two-dimensional (2D) cell cultures merely contribute to the dissection of the involved molecular pathways (Hossain et al., 2024). In the last few years, ethical concerns and technical challenges have limited the study of the interactions between the human brain and peripheral organs; it has prompted the exploration of new experimental tools that could eventually reflect the brain exposure to several molecules, especially during the deterioration of BBB responsible for conveying harmful components to the already damaged brain (Chen et al., 2021).
The emergence of three-dimensional (3D) brain organoids (BOs), lab-grown structures developed through tissue engineering, have disclosed a revolutionary alternative to traditional AD models (Gonzalez et al., 2018; Kim et al., 2024). These mini encephalic organs mimic the in vivo physiology of the human brain, providing a more accurate representation of its structure and function (Hong et al., 2022; Jeong et al., 2023). Starting from blood or fibroblast samples and passing through the intermediate transformation into induced pluripotent stem cells (iPSCs) (Raja et al., 2016; Choe et al., 2024), various protocols for developing 3D BOs have flourished, differing in chemical composition, microenvironmental settings, and technological materials (Kwak et al., 2024). Indeed, whole-brain organoids and more specifically BOs of different regions can be built (e.g., dorsal forebrain or cerebral organoids (COs), respectively) (Del Dosso et al., 2020). However, over the past decade, more than 2,255 papers on “human brain organoids” have been published, underscoring the urgent need for a more unifying experimental methodology. These in vitro organs could really be leveraged for the advancement of basic and applied neuroscience only when comprehensive guidance and assertive advice on the design, execution, and sharing of experiments significantly will improve the reproducibility and utility of these models (Pașca et al., 2024). Recent findings have highlighted the importance of BOs in studying the fundamental mechanisms underlying AD. Notably, when these organoids are derived from AD patients, they retain biomarkers associated with the disease (Yan et al., 2018; Alić et al., 2021; Chen et al., 2021), even after undergoing a process of embryonic resetting through the use of iPSCs as intermediate cellular elements.
4 Patient-derived advanced BOs: combining biological and technological tools
The BOs exhibit certain features of human brain structure and AD-like pathology, serving as a tool to explore the connection between Alzheimer’s pathology and neural cell dysfunction that leads to cognitive decline. The morphological and biochemical analysis of BOs aligned with individual clinical histories creates a promising platform for modeling the patient-specific disease staging. Such experimental models offer the disease continuum from the incoming neurological deficits to AD diagnosis, which is still missing in the in vitro models despite the lack of vascularization and incomplete maturation. Meanwhile, at some point in the experimental study, BOs could express different recognized biomarkers, signaling the onset of the neurodegenerative process even when the clinical evidence is absent in the corresponding donor subjects and, thus, preventing in vivo invasive and expensive investigations. Furthermore, this innovative approach during the clinical follow-up will allow researchers to generate the clinically corresponding mini-brains to track clinical evolution. In contrast to clinical evaluations, the study of BOs and any biological fluids potentially analyzed for peripheral biomarkers can be carried out both prospectively and retrospectively, due to the possibility of storing them in certified biobanks.
A comprehensive understanding of AD through BOs hinges on merging cutting-edge biological and technological advancements (Boylin et al., 2024). We visually represent this in our proposed model of investigation (Figure 1). In the context of the biological field, these advancements aim to fulfill several critical objectives:
1. Recreating architectural complexity: BOs will replicate the cellular heterogeneity of the central nervous system (CNS), encompassing neuronal and glial phenotypes, and incorporating vascular components like endothelial cells to preserve BBB integrity.
2. Identifying CNS biomarkers: BOs will undergo molecular and morphological analyses to identify validated or potential AD biomarkers, previously detected in corresponding in vivo anatomical structures, including toxic protein aggregates such as β-amyloid and tau proteins, which are crucial for comparing AD hallmarks in vivo.
3. Correlating central and peripheral biomarkers: despite the lack of direct connections to other organs, BOs will reveal temporal patterns within the aging organoids and potential correlations between identified central biomarkers and peripheral ones found in blood samples of the same patients.
4. Analyzing immune responses: recruitment and pathological transformation of resident immune cells (astrocytes and microglia) will be qualitatively and quantitatively assessed to understand their detrimental pro-inflammatory effects.
5. Modeling aging processes: induction of cellular senescence in BOs will simulate gene expression changes and epigenetic modifications during aging, a significant risk factor for neurodegenerative diseases.
To ensure reproducibility and increase predictive capabilities, the following technological tools will be employed:
1. 3D microelectrode arrays, and high-resolution electrophysiology devices: they can record rhythmic activity with high spatio-temporal resolution and collectively synchronized electric signals.
2. Organ-on-a-chip devices: these devices will simulate physiological environments to optimize BO growth and homeostasis maintenance or alteration (see BBB defects).
3. Biocomputing simulations: utilizing computational models they can predict organoid behavior and response under varying conditions.
4. Optimized algorithms: the development of algorithms tailored for in silico-BOs interaction modeling will deepen the understanding of AD progression.
5. Creating Digital Twins (DTs) using artificial intelligence (AI).
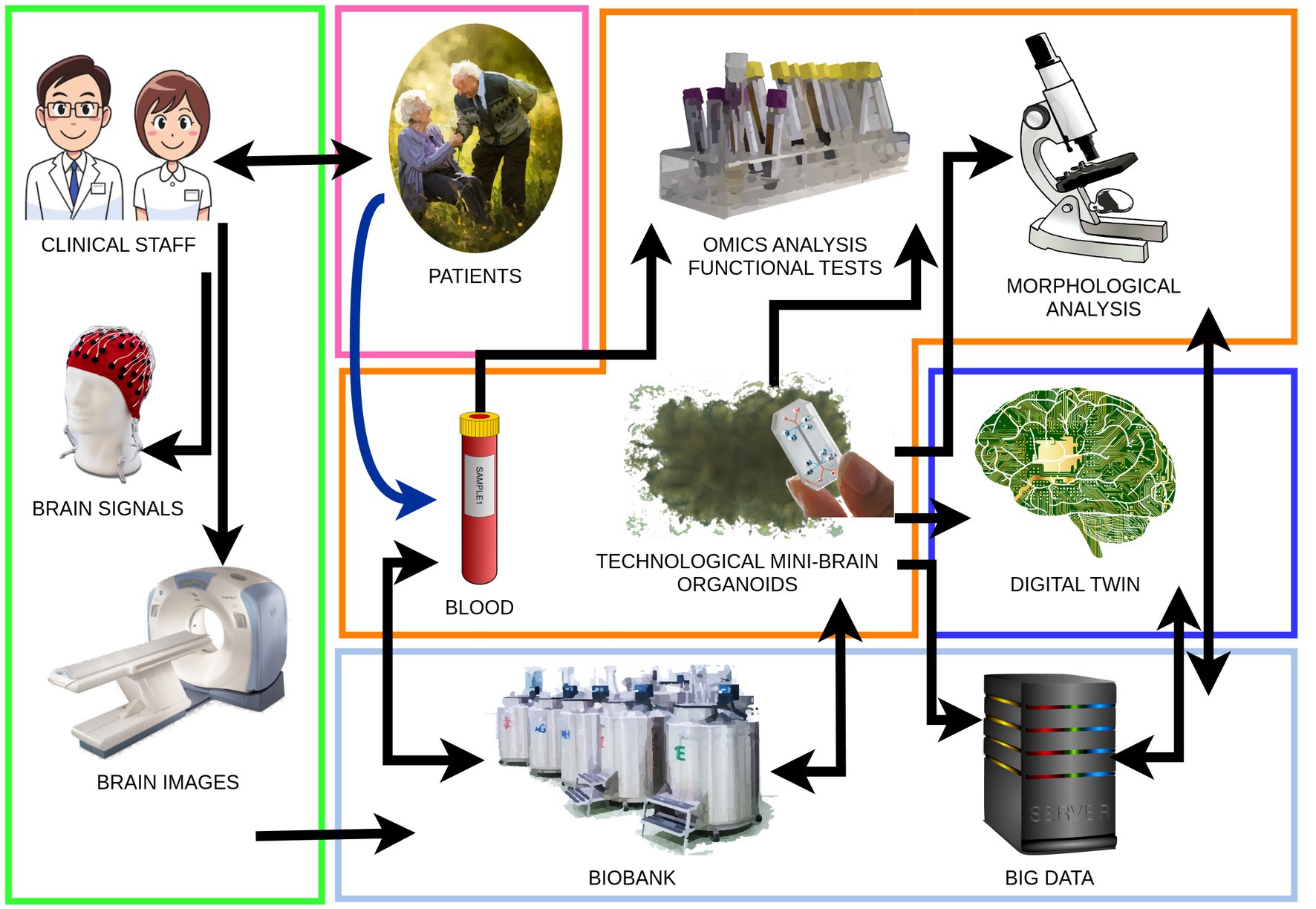
Figure 1. Brain organoids work-flow from patients to brain organoids and digital twins. Clinical staff: patient evaluation and classification through standard clinical tools and brain imaging. Blood: blood sampling, peripheral blood mononuclear cell extraction, and obtaining pluripotent stem cells to generate brain organoids. Technological mini-brain organoids: development and application of biotechnologies to achieve the structural complexity and cellular diversity of brain organoids. Analysis: diachronic execution of morphological analyses, omics analyses, and functional tests aimed at identifying AD biomarkers in brain organoids and blood of the organoid donor patients. Digital twin: creation of a mathematical model of the brain organoid that parallels its growth and degeneration. Data collection: collection of biological samples in a dedicated biobank and synthetic data, big data, in an open-access platform.
The term “digital twin” (DT) was introduced by Grieves (2019) to describe a digital entity capable of replicating certain properties of a physical object. Unlike traditional computer simulations, which rely on static mathematical models, DTs are virtual replicas of physical entities continuously updated with real-time data. This capability enables real-time monitoring, analysis, and intervention, making DTs more dynamic and interactive than conventional simulations. The development of these models relied heavily on imaging technologies and computational frameworks to mimic the structure and basic functions of organs. These early-stage DTs served as proof-of-concept demonstrations, showing how virtual models could enhance decision-making in medical practice.
AI-driven DTs have been applied in various medical and healthcare fields, including stroke progression (Allen et al., 2021), tissue culture (Möller and Pörtner, 2021), neurosurgery (Chumnanvej et al., 2024), and personalized dementia care (Wickramasinghe et al., 2022). Initially focused on simulating specific organs to address clinical challenges, DT technology has evolved to support personalized medicine by predicting patient-specific treatment responses. Early applications involved creating digital replicas of organs, such as the heart and lungs to enhance surgical procedures and drug effectiveness before real-world implementation (Vallée, 2023; El-Warrak and de Farias, 2024).
A major leap in DT technology came with the incorporation of molecular and genetic data, particularly transcriptomics which enables the analysis of gene expression patterns underpinning cellular and organ functions (Hansen et al., 2024). By integrating such data, DTs moved beyond anatomical simulations to model the intricate biological processes within organs clarifying disease mechanisms. Moreover, the development of validation models is enhanced by advanced predictive features. For instance, heart digital twins can predict arrhythmias, enabling personalized interventions (Thangaraj et al., 2024). Liver models provide valuable insights into disease progression, which helps optimize treatments and improve patient outcomes (Subramanian, 2020). Similarly, brain digital twins simulate neurological conditions, assisting in the planning of accurate and safe surgeries (Fekonja et al., 2024). Finally, the convergence of organoid technology and DT frameworks is one of the most exciting advancements in this field, pushing the boundaries for studying disease pathology, drug responses, and developmental biology (Heydari et al., 2021).
When combined with DT technology, organoids might offer an unparalleled platform for modeling complex biological systems and could provide unique insights into how certain genetic mutations or environmental factors influence developmental pathways and gain functions.
Nevertheless, DT technology and its application to organoids face several challenges. One major limitation is the computational complexity involved in creating accurate and scalable models. Simulating the intricate interplay of genetic, molecular, and physiological processes within an organoid requires significant computational power and advanced algorithms making the integration of diverse data types—such as transcriptomics, proteomics, and metabolomics—into a unified DT a concrete technical hurdle. However, the high computational demands of these advanced models can be effectively mitigated through the power of quantum computing, particularly with technologies such as the Majorana I processor, which offers unprecedented capabilities in handling such complexity (Nanda et al., 2024).
Another challenge is the need for standardized protocols and frameworks to ensure the reproducibility and reliability of DT models. Establishing regulatory guidelines and ethical standards will be critical as these technologies move closer to clinical application.
By improving and leveraging AI-driven analytics, researchers can automate the integration and interpretation of complex datasets, thereby enhancing the scalability and accuracy of DTs. Additionally, the development of more sophisticated organoid cultures—such as vascularized or multi-organ systems—will further expand the capabilities of DTs in simulating human biology.
5 Discussion
Preclinical studies currently rely on in vivo animal models, including transgenic and aged specimens, with 8,624,692 animals sacrificed for scientific purposes in the EU and Norway in 2020 (Commission Staff Working Document, 2023). BO-based research aims to reduce the use of these models, facilitating faster and easier scaling of therapeutic interventions for AD. We propose an alternative methodology using a virtual environment to test drug candidates before clinical trials, which lowers costs, minimizes ethical concerns of animal testing, and allows for individualized treatment strategies. Individual-derived BOs serve as valuable ex vivo targets for monitoring pharmacological interventions during disease progression, offering insights that may lead to personalized therapies.
In clinical applications related to AD and MCI, bridging the gap between genetic predispositions and sporadic factors remains essential. Understanding these complexities is crucial for developing effective interventions aimed at preserving mental acuity and mitigating AD progression. Modeling AD with BOs has primarily focused on familial AD cases. However, there is a growing need to include sporadic cases to enhance representativeness and accurately recapitulate the pathology (Selkoe and Hardy, 2016). Recently, some authors have outlined the minimal and ideal recommended standards for the quantitative analysis of organoids, focusing on ensuring rigor and reproducibility in human BOs research (Sandoval et al., 2024). While we refrain from replicating issues already exposed in a recent exhaustive paper (Cerneckis et al., 2023), we wish to warn the scientific community about some caveats and potential troubleshooting issues when translating findings to AD-affected patients.
One notable contradiction involves the aging process, a fundamental and widely recognized risk factor for neurodegenerative diseases. COs from AD patients are formed through the differentiation of embryonic stem cells (ESCs) or iPSCs, which, in turn, are derived from AD fibroblasts or blood cells via genetic reprogramming (Kunitomi et al., 2022). However, due to reasons that are both time-consuming and costly, studies based on organoid technology for AD research often rely on a limited number of iPSC cell lines, in stark contrast to the significantly larger number of patients enrolled in clinical studies. This raises the critical question about the robustness of the observed differences genuinely associated with AD-related pathological symptoms. An incomplete but promising solution would be to invest in research programs that use iPSCs derived from patients with sporadic AD. This approach could help create a more solid and reliable experimental design, aiming to minimize biochemical and morphological differences between various batches of cells.
For example, in a recent study by Lee et al. (2022), while certain features such as Aβ deposits aligned with histopathological findings, the expression levels of AD-related genes Microtubule-Associated Protein Tau (MAPT) and Amyloid Precursor Protein (APP) were similar when comparing iPSCs derived from normal and familial AD patients. Moreover, neuronal excitation tested by electrophysiological recording was downregulated in the AD group, in stark contrast to previous reports (Lin et al., 2018; Ghatak et al., 2019). This suggests that a 3-month culture period may be insufficient to fully capture AD’s histopathological hallmarks, but longer in vitro timelines are strongly dependent on sophisticated and reliable microfluidic systems to ensure the same vitality degree to the overall aged BOs. Research on the aging process has already been conducted, as the in vitro timeline does not match the in vivo aging pathway (Gordon et al., 2021; Hossain et al., 2024; Park et al., 2025). In addition, even though there is a close overlapping between the transcriptome/epigenome of BOs and human primary fetal tissues (Amiri et al., 2018), more detailed studies are needed for a faithful comparison between BOs and the aging brain, both in normal and pathological conditions. Thus, while human brain organoids as research tools provide advantages for studying AD, they also present numerous controversies and weaknesses that must be addressed (Sainz et al., 2025). Finally, the evolution of DT technology from simple anatomical models to complex simulations of BOs acts as a transformative shift in healthcare and biomedical research. The standpoint of this review is that DTs of BOs could be part of a fruitful strategy designed to a better comprehension and interpretation of biological phenomena underpinning AD appearance and progression. By integrating molecular data and leveraging organoid platforms, DTs would offer unprecedented opportunities for studying AD and possible in silico therapeutic personalized intervention prior, in alternative, or in parallel with human clinical trials.
Author contributions
CD: Conceptualization, Methodology, Writing – review & editing. MR: Methodology, Writing – review & editing. EG: Formal analysis, Writing – review & editing. MT: Formal analysis, Writing – review & editing. CM: Formal analysis, Writing – review & editing. DM: Conceptualization, Formal analysis, Writing – review & editing. AD: Conceptualization, Formal analysis, Methodology, Project administration, Writing – review & editing.
Funding
The author(s) declare that no financial support was received for the research and/or publication of this article.
Conflict of interest
The authors declare that the research was conducted in the absence of any commercial or financial relationships that could be construed as a potential conflict of interest.
Generative AI statement
The authors declare that no Gen AI was used in the creation of this manuscript.
Publisher’s note
All claims expressed in this article are solely those of the authors and do not necessarily represent those of their affiliated organizations, or those of the publisher, the editors and the reviewers. Any product that may be evaluated in this article, or claim that may be made by its manufacturer, is not guaranteed or endorsed by the publisher.
References
Abidin, S. Z., Mat Pauzi, N. A., Mansor, N. I., Mohd Isa, N. I., and Hamid, A. A. (2023). A new perspective on Alzheimer’s disease: microRNAs and circular RNAs. Front. Genet. 14:1231486. doi: 10.3389/fgene.2023.1231486
Aghdam, M. A., Bozdag, S., and Saeed, F. (2025). Alzheimer’s disease neuroimaging initiative. Machine-learning models for Alzheimer's disease diagnosis using neuroimaging data: survey, reproducibility, and generalizability evaluation. Brain Inform. 12:8. doi: 10.1186/s40708-025-00252-3
Alić, I., Goh, P. A., Murray, A., Portelius, E., Gkanatsiou, E., Gough, G., et al. (2021). Patient-specific Alzheimer-like pathology in trisomy 21 cerebral organoids reveals BACE2 as a gene dose-sensitive AD suppressor in human brain. Mol. Psychiatry 26, 5766–5788. doi: 10.1038/s41380-020-0806-5
Allen, A., Siefkas, A., Pellegrini, E., Burdick, H., Barnes, G., Calvert, J., et al. (2021). A digital twins machine learning model for forecasting disease progression in stroke patients. Appl. Sci. 11:5576. doi: 10.3390/app11125576
Amiri, A., Coppola, G., Scuderi, S., Wu, F., Roychowdhury, T., Liu, F., et al. (2018). Transcriptome and epigenome landscape of human cortical development modeled in organoids. Science 362:aat6720. doi: 10.1126/science.aat6720
Arevalo-Rodriguez, I., Smailagic, N., Roqué-Figuls, M., Ciapponi, A., Sanchez-Perez, E., Giannakou, A., et al. (2021). Mini-mental State examination (MMSE) for the early detection of dementia in people with mild cognitive impairment (MCI). Cochrane Database Syst. Rev. 2021:CD010783. doi: 10.1002/14651858.CD010783.pub3
Baldini, A., Greco, A., Lomi, M., Giannelli, R., Canale, P., Diana, A., et al. (2022). Blood Analytes as biomarkers of mechanisms involved in Alzheimer’s disease progression. Int. J. Mol. Sci. 23:13289. doi: 10.3390/ijms232113289
Beder, N., Belkhelfa, M., and Leklou, H. (2024). Involvement of inflammasomes in the pathogenesis of Alzheimer’s disease. J. Alzheimers Dis. 102, 11–29. doi: 10.1177/13872877241283677
Bhatnagar, D., Ladhe, S., and Kumar, D. (2023). Discerning the prospects of miRNAs as a multi-target therapeutic and diagnostic for Alzheimer’s disease. Mol. Neurobiol. 60, 5954–5974. doi: 10.1007/s12035-023-03446-0
Boylin, K., Aquino, G. V., Purdon, M., Abedi, K., Kasendra, M., and Barrile, R. (2024). Basic models to advanced systems: harnessing the power of organoids-based microphysiological models of the human brain. Biofabrication 16:032007. doi: 10.1088/1758-5090/ad4c08
Carmona-Iragui, M., O'Connor, A., Llibre-Guerra, J., Lao, P., Ashton, N. J., Fortea, J., et al. (2024). Clinical and research application of fluid biomarkers in autosomal dominant Alzheimer's disease and down syndrome. EBioMedicine 108:105327. doi: 10.1016/j.ebiom.2024.105327
Cash, D. M., Morgan, K. E., O'Connor, A., Veale, T. D., Malone, I. B., Poole, T., et al. (2025). Dominantly inherited Alzheimer network (DIAN). Sample size estimates for biomarker-based outcome measures in clinical trials in autosomal dominant Alzheimer's disease. J. Prev Alzheimers Dis. :100133. doi: 10.1016/j.tjpad.2025.100133
Cerneckis, J., Bu, G., and Shi, Y. (2023). Pushing the boundaries of brain organoids to study Alzheimer’s disease. Trends Mol. Med. 29, 659–672. doi: 10.1016/j.molmed.2023.05.007
Chandra, A., Dervenoulas, G., and Politis, M. (2019). Magnetic resonance imaging in Alzheimer’s disease and mild cognitive impairment. J. Neurol. 266, 1293–1302. doi: 10.1007/s00415-018-9016-3
Chen, X., Sun, G., Tian, E., Zhang, M., Davtyan, H., Beach, T. G., et al. (2021). Modeling sporadic Alzheimer's disease in human brain organoids under serum exposure. Adv. Sci. 8:e2101462. doi: 10.1002/advs.202101462
Chételat, G., Arbizu, J., Barthel, H., Garibotto, V., Law, I., Morbelli, S., et al. (2020). Amyloid-PET and 18F-FDG-PET in the diagnostic investigation of Alzheimer’s disease and other dementias. Lancet Neurol. 19, 951–962. doi: 10.1016/S1474-4422(20)30314-8
Choe, M. S., Yeo, H. C., Kim, J. S., Lee, J., Lee, H. J., Kim, H. R., et al. (2024). Simple modeling of familial Alzheimer's disease using human pluripotent stem cell-derived cerebral organoid technology. Stem Cell Res Ther 15:118. doi: 10.1186/s13287-024-03732-1
Chumnanvej, S., Chumnanvej, S., and Tripathi, S. (2024). Assessing the benefits of digital twins in neurosurgery: a systematic review. Neurosurg. Rev. 47:52. doi: 10.1007/s10143-023-02260-5
Ciesielska, N., Sokołowski, R., Mazur, E., Podhorecka, M., Polak-Szabela, A., and Kędziora-Kornatowska, K. (2016). Is the Montreal cognitive assessment (MoCA) test better suited than the Mini-mental State examination (MMSE) in mild cognitive impairment (MCI) detection among people aged over 60? Meta-analysis. Psychiatr. Pol. 50, 1039–1052. doi: 10.12740/PP/45368
Cummings, J., Hahn-Pedersen, J. H., Eichinger, C. S., Freeman, C., Clark, A., Tarazona, L. R. S., et al. (2023). Exploring the relationship between patient-relevant outcomes and Alzheimer’s disease progression assessed using the clinical dementia rating scale: a systematic literature review. Front. Neurol. 14:1208802. doi: 10.3389/fneur.2023.1208802
Del Dosso, A., Urenda, J.-P., Nguyen, T., and Quadrato, G. (2020). Upgrading the physiological relevance of human brain organoids. Neuron 107, 1014–1028. doi: 10.1016/j.neuron.2020.08.029
Dubois, B., von Arnim, C. A. F., Burnie, N., Bozeat, S., and Cummings, J. (2023). Biomarkers in Alzheimer's disease: role in early and differential diagnosis and recognition of atypical variants. Alzheimers Res. Ther. 15:175. doi: 10.1186/s13195-023-01314-6
El-Warrak, L., and de Farias, C. M. (2024). The State of the art of digital twins in health—a quick review of the literature. Computers 13:228. doi: 10.3390/computers13090228
Commission Staff Working Document. Summary Report on the statistics on the use of animals for scientific purposes in the Member States of the European Union and Norway in 2020. (2023) 84, 1–136. Available at: https://circabc.europa.eu/ui/group/8ee3c69a-bccb-4f22-89ca-277e35de7c63/library/10ad28d6-e17e-4367-b459-20883402cfcc/details. (Accessed March 31, 2023).
Fekonja, L. S., Schenk, R., Schröder, E., Tomasello, R., Tomšič, S., and Picht, T. (2024). The digital twin in neuroscience: from theory to tailored therapy. Front. Neurosci. 18:1454856. doi: 10.3389/fnins.2024.1454856
Ghatak, S., Dolatabadi, N., Trudler, D., Zhang, X., Wu, Y., Mohata, M., et al. (2019). Mechanisms of hyperexcitability in Alzheimer’s disease hiPSC-derived neurons and cerebral organoids vs isogenic controls. eLife 8:50333. doi: 10.7554/eLife.50333
Gkenios, G., Latsiou, K., Diamantaras, K., Chouvarda, I., and Tsolaki, M. (2022). Diagnosis of Alzheimer’s disease and mild cognitive impairment using EEG and recurrent neural networks. 2022 44th annual international conference of the IEEE engineering in Medicine & Biology Society (EMBC). 3179–3182.
Gonzalez, C., Armijo, E., Bravo-Alegria, J., Becerra-Calixto, A., Mays, C. E., and Soto, C. (2018). Modeling amyloid beta and tau pathology in human cerebral organoids. Mol. Psychiatry 23, 2363–2374. doi: 10.1038/s41380-018-0229-8
Gordon, A., Yoon, S. J., Tran, S. S., Makinson, C. D., Park, J. Y., Andersen, J., et al. (2021). Long-term maturation of human cortical organoids matches key early postnatal transitions. Nat. Neurosci. 24, 331–342. doi: 10.1038/s41593-021-00802-y
Grieves, M. W. (2019). “Complex systems engineering: Theory and practice” in Progress in astronautics and aeronautics. eds. S. Flumerfelt, K. G. Schwartz, D. Mavris, and S. Briceno (Reston, VA: American Institute of Aeronautics and Astronautics, Inc.).
Hampel, H., Hu, Y., Cummings, J., Mattke, S., Iwatsubo, T., Nakamura, A., et al. (2023). Blood-based biomarkers for Alzheimer's disease: current state and future use in a transformed global healthcare landscape. Neuron 111, 2781–2799. doi: 10.1016/j.neuron.2023.05.017
Hansen, J., Jain, A. R., Nenov, P., Robinson, P. N., and Iyengar, R. (2024). From transcriptomics to digital twins of organ function. Front. Cell Dev. Biol. 12:1240384. doi: 10.3389/fcell.2024.1240384
Heydari, Z., Moeinvaziri, F., Agarwal, T., Pooyan, P., Shpichka, A., Maiti, T. K., et al. (2021). Organoids: a novel modality in disease modeling. Biodes Manuf 4, 689–716. doi: 10.1007/s42242-021-00150-7
Hong, Y. J., Lee, S., Choi, J., Yoon, S. H., and Do, J. T. (2022). A simple method for generating cerebral organoids from human pluripotent stem cells. Int. J. Stem Cells 15, 95–103. doi: 10.15283/ijsc21195
Hossain, M. K., Kim, H.-R., and Chae, H. J. (2024). Aging phenotype in AD brain organoids: track to success and challenges. Ageing Res. Rev. 96:102256. doi: 10.1016/j.arr.2024.102256
Huang, S., Wang, Y.-J., and Guo, J. (2022). Biofluid biomarkers of Alzheimer’s disease: Progress, problems, and perspectives. Neurosci. Bull. 38, 677–691. doi: 10.1007/s12264-022-00836-7
Hussain, S. M., Robb, C., Tonkin, A. M., Lacaze, P., Chong, T. T.-J., Beilin, L. J., et al. (2024). Association of plasma high-density lipoprotein cholesterol level with risk of incident dementia: a cohort study of healthy older adults. Lancet Reg. Health West Pac. 43:100963. doi: 10.1016/j.lanwpc.2023.100963
Jack, C. R., Andrews, J. S., Beach, T. G., Buracchio, T., Dunn, B., Graf, A., et al. (2024). Revised criteria for diagnosis and staging of Alzheimer’s disease: Alzheimer’s association workgroup. Alzheimers Dement. 20, 5143–5169. doi: 10.1002/alz.13859
Jack, C. R., Bennett, D. A., Blennow, K., Carrillo, M. C., Dunn, B., Haeberlein, S. B., et al. (2018). NIA-AA research framework: toward a biological definition of Alzheimer’s disease. Alzheimers Dement. 14, 535–562. doi: 10.1016/j.jalz.2018.02.018
Jeong, E., Choi, S., and Cho, S.-W. (2023). Recent advances in brain organoid Technology for Human Brain Research. ACS Appl. Mater. Interfaces 15, 200–219. doi: 10.1021/acsami.2c17467
Jiao, B., Ouyang, Z., Xiao, X., Zhang, C., Xu, T., Yang, Q., et al. (2025). Development and validation of machine learning models with blood-based digital biomarkers for Alzheimer's disease diagnosis: a multicohort diagnostic study. EClinicalMedicine 81:103142. doi: 10.1016/j.eclinm.2025.103142
Jongsiriyanyong, S., and Limpawattana, P. (2018). Mild cognitive impairment in clinical practice: a review article. Am. J. Alzheimers Dis. Other Dement. 33, 500–507. doi: 10.1177/1533317518791401
Khoury, R., and Ghossoub, E. (2019). Diagnostic biomarkers of Alzheimer’s disease: a state-of-the-art review. Biomark Neuropsychiatry 1:100005. doi: 10.1016/j.bionps.2019.100005
Kim, Y., Kim, H., Cho, B., An, S., Kang, S., Kim, S., et al. (2024). Modeling APOE ε4 familial Alzheimer's disease in directly converted 3D brain organoids. Front. Aging Neurosci. 16:1435445. doi: 10.3389/fnagi.2024.1435445
Klyucherev, T. O., Olszewski, P., Shalimova, A. A., Chubarev, V. N., Tarasov, V. V., Attwood, M. M., et al. (2022). Advances in the development of new biomarkers for Alzheimer's disease. Transl. Neurodegener. 11:25. doi: 10.1186/s40035-022-00296-z
Kunitomi, A., Hirohata, R., Arreola, V., Osawa, M., Kato, T. M., Nomura, M., et al. (2022). Improved Sendai viral system for reprogramming to naive pluripotency. Cell Reports Methods 2:100317. doi: 10.1016/j.crmeth.2022.100317
Kwak, T., Park, S.-H., Lee, S., Shin, Y., Yoon, K.-J., Cho, S.-W., et al. (2024). Guidelines for manufacturing and application of organoids: brain. Int. J. Stem Cells 17, 158–181. doi: 10.15283/ijsc24056
Lee, J.-H., Yoo, G., Choi, J., Park, S.-H., Shin, H., Prasad, R., et al. (2022). Cell-line dependency in cerebral organoid induction: cautionary observations in Alzheimer’s disease patient-derived induced pluripotent stem cells. Mol. Brain 15:46. doi: 10.1186/s13041-022-00928-5
Lin, Y.-T., Seo, J., Gao, F., Feldman, H. M., Wen, H.-L., Penney, J., et al. (2018). APOE4 causes widespread molecular and cellular alterations associated with Alzheimer’s disease phenotypes in human iPSC-derived brain cell types. Neuron 98, 1141–1154.e7. doi: 10.1016/j.neuron.2018.05.008
Luo, Y., Fu, Y., Tan, T., Hu, J., Li, F., Liao, Z., et al. (2022). Screening of lncRNA-miRNA-mRNA Coexpression regulatory networks involved in acute traumatic coagulation dysfunction based on CTD, GeneCards, and PharmGKB databases. Oxidative Med. Cell. Longev. 2022, 1–13. doi: 10.1155/2022/7280312
Möller, J., and Pörtner, R. (2021). Digital twins for tissue culture techniques—concepts, expectations, and State of the art. PRO 9:447. doi: 10.3390/pr9030447
Nanda, T., Dhanalakshmi, B. K., and Lakshmi, B. N. (2024). An explorative cross sectional comprehensive survey on quantum computing. SN Comput. Sci. 5:1130. doi: 10.1007/s42979-024-03505-w
Olsson, B., Lautner, R., Andreasson, U., Öhrfelt, A., Portelius, E., Bjerke, M., et al. (2016). CSF and blood biomarkers for the diagnosis of Alzheimer’s disease: a systematic review and meta-analysis. Lancet Neurol. 15, 673–684. doi: 10.1016/S1474-4422(16)00070-3
Ossenkoppele, R., van der Kant, R., and Hansson, O. (2022). Tau biomarkers in Alzheimer’s disease: towards implementation in clinical practice and trials. Lancet Neurol. 21, 726–734. doi: 10.1016/S1474-4422(22)00168-5
Park, S., Min, C. H., Choi, E., Choi, J. S., Park, K., Han, S., et al. (2025). Long-term tracking of neural and oligodendroglial development in large-scale human cerebral organoids by noninvasive volumetric imaging. Sci. Rep. 15:2536. doi: 10.1038/s41598-025-85455-8
Pașca, S. P., Arlotta, P., Bateup, H. S., Camp, J. G., Cappello, S., Gage, F. H., et al. (2024). A framework for neural organoids, assembloids and transplantation studies. Nature 639, 315–320. doi: 10.1038/s41586-024-08487-6
Pascoal, T. A., Aguzzoli, C. S., Lussier, F. Z., Crivelli, L., Suemoto, C. K., Fortea, J., et al. (2024). Insights into the use of biomarkers in clinical trials in Alzheimer's disease. EBioMedicine 108:105322. doi: 10.1016/j.ebiom.2024.105322
Raja, W. K., Mungenast, A. E., Lin, Y. T., Ko, T., Abdurrob, F., Seo, J., et al. (2016). Self-organizing 3D human neural tissue derived from induced pluripotent stem cells recapitulate Alzheimer's disease phenotypes. PLoS One 11:e0161969. doi: 10.1371/journal.pone.0161969
Rui, W., Xiao, H., Fan, Y., Ma, Z., Xiao, M., Li, S., et al. (2021). Systemic inflammasome activation and pyroptosis associate with the progression of amnestic mild cognitive impairment and Alzheimer’s disease. J. Neuroinflammation 18:280. doi: 10.1186/s12974-021-02329-2
Sainz, A., Pérez, F., Pérez-Samartín, A., Panicker, M., Ruiz, A., and Matute, C. (2025). Pross and cons of human brain organoids to study Alzheimer's disease. Aging Dis. doi: 10.14336/AD.2024.1409 (Epub ahead of print).
Sandoval, S. O., Cappuccio, G., Kruth, K., Osenberg, S., Khalil, S. M., Méndez-Albelo, N. M., et al. (2024). Rigor and reproducibility in human brain organoid research: where we are and where we need to go. Stem Cell Reports 19, 796–816. doi: 10.1016/j.stemcr.2024.04.008
Selkoe, D. J., and Hardy, J. (2016). The amyloid hypothesis of Alzheimer’s disease at 25 years. EMBO Mol. Med. 8, 595–608. doi: 10.15252/emmm.201606210
Singh, D. (2022). Astrocytic and microglial cells as the modulators of neuroinflammation in Alzheimer’s disease. J. Neuroinflammation 19:206. doi: 10.1186/s12974-022-02565-0
Soares Martins, T., Marçalo, R., Da Cruze Silva, C. B., Trindade, D., Catita, J., Amado, F., et al. (2022). Novel exosome biomarker candidates for Alzheimer’s disease unravelled through mass spectrometry analysis. Mol. Neurobiol. 59, 2838–2854. doi: 10.1007/s12035-022-02762-1
Stewart, R. (2012). Subjective cognitive impairment. Curr. Opin. Psychiatry 25, 445–450. doi: 10.1097/YCO.0b013e3283586fd8
Subramanian, K. (2020). Digital twin for drug discovery and development—the virtual liver. J Indian Inst Sci 100, 653–662. doi: 10.1007/s41745-020-00185-2
Teng, E., Li, Y., Manser, P. T., Pickthorn, K., Butcher, B. D., Blendstrup, M., et al. (2023). Cross-sectional and longitudinal assessments of function in prodromal-to-mild Alzheimer’s disease: a comparison of the ADCS-ADL and A-IADL-Q scales. Alzheimer’s Dement. 15:e12452. doi: 10.1002/dad2.12452
Teunissen, C. E., Verberk, I. M. W., Thijssen, E. H., Vermunt, L., Hansson, O., Zetterberg, H., et al. (2022). Blood-based biomarkers for Alzheimer’s disease: towards clinical implementation. Lancet Neurol. 21, 66–77. doi: 10.1016/S1474-4422(21)00361-6
Thangaraj, P. M., Benson, S. H., Oikonomou, E. K., Asselbergs, F. W., and Khera, R. (2024). Cardiovascular care with digital twin technology in the era of generative artificial intelligence. Eur. Heart J. 45, 4808–4821. doi: 10.1093/eurheartj/ehae619
Thangwaritorn, S., Lee, C., Metchikoff, E., Razdan, V., Ghafary, S., Rivera, D., et al. (2024). A review of recent advances in the Management of Alzheimer’s disease. Cureus 16:e58416. doi: 10.7759/cureus.58416
Vallée, A. (2023). Digital twin for healthcare systems. Front. Digit Health 5:1253050. doi: 10.3389/fdgth.2023.1253050
Wang, X., Huang, W., Su, L., Xing, Y., Jessen, F., Sun, Y., et al. (2020). Neuroimaging advances regarding subjective cognitive decline in preclinical Alzheimer's disease. Mol. Neurodegener. 15:55. doi: 10.1186/s13024-020-00395-3
Wei, W., Wang, Z.-Y., Ma, L.-N., Zhang, T.-T., Cao, Y., and Li, H. (2020). MicroRNAs in Alzheimer’s disease: function and potential applications as diagnostic biomarkers. Front. Mol. Neurosci. 13:160. doi: 10.3389/fnmol.2020.00160
Wickramasinghe, N., Ulapane, N., Andargoli, A., Ossai, C., Shuakat, N., Nguyen, T., et al. (2022). Digital twins to enable better precision and personalized dementia care. JAMIA Open 5:72. doi: 10.1093/jamiaopen/ooac072
Yan, Y., Song, L., Bejoy, J., Zhao, J., Kanekiyo, T., Bu, G., et al. (2018). Modeling neurodegenerative microenvironment using cortical organoids derived from human stem cells. Tissue Eng. Part A 24, 1125–1137. doi: 10.1089/ten.TEA.2017.0423
Zekry, D., Hauw, J., and Gold, G. (2002). Mixed dementia: epidemiology, diagnosis, and treatment. J. Am. Geriatr. Soc. 50, 1431–1438. doi: 10.1046/j.1532-5415.2002.50367.x
Keywords: Alzheimer’s disease, neurodegeneration, personalized medicine, brain organoids, early diagnosis biomarker, neuroinflammation, digital twins
Citation: Dolciotti C, Righi M, Grecu E, Trucas M, Maxia C, Murtas D and Diana A (2025) The translational power of Alzheimer’s-based organoid models in personalized medicine: an integrated biological and digital approach embodying patient clinical history. Front. Cell. Neurosci. 19:1553642. doi: 10.3389/fncel.2025.1553642
Edited by:
Luca Raiteri, University of Genoa, ItalyReviewed by:
Vanessa Castelli, University of L’Aquila, ItalyCopyright © 2025 Dolciotti, Righi, Grecu, Trucas, Maxia, Murtas and Diana. This is an open-access article distributed under the terms of the Creative Commons Attribution License (CC BY). The use, distribution or reproduction in other forums is permitted, provided the original author(s) and the copyright owner(s) are credited and that the original publication in this journal is cited, in accordance with accepted academic practice. No use, distribution or reproduction is permitted which does not comply with these terms.
*Correspondence: Daniela Murtas, bXVydGFzQHVuaWNhLml0; Andrea Diana, ZGlhbmFAdW5pY2EuaXQ=; Marco Righi, bWFyY28ucmlnaGlAdW5pcGkuaXQ=